Abstract
Controversy surrounding the use of race-conscious admissions can be partially resolved with improved empirical knowledge of the effects of racial diversity in educational settings. We use a national sample of law students nested in 64 law schools to test the complex and largely untested theory regarding the effects of educational diversity on student outcomes. Social scientists who study these outcomes frequently encounter both latent variables and nested data within a single analysis. Yet, until recently, an appropriate modeling technique has been computationally infeasible, and consequently few applied researchers have estimated appropriate models to test their theories, sometimes limiting the scope of their research question. Our results, based on disaggregated multilevel structural equation models, show that racial diversity is related to a reduction in prejudiced attitudes and increased perceived exposure to diverse ideas and that these effects are mediated by more frequent interpersonal contact with diverse peers. These findings provide support for the idea that administrative manipulation of educational diversity may lead to improved student outcomes. Admitting a racially/ethnically diverse student body provides an educational experience that encourages increased exposure to diverse ideas and belief systems.
This study was motivated by an issue of contemporary relevance in the legal system: the benefits of educational diversity (Citation Gratz v. Bollinger, 2003; Citation Grutter v. Bollinger, 2003; Citation Meredith v. Jefferson Co. Board of Education, 2007; Citation Parents v. Seattle, 2007). Theory and previous empirical research suggest that increased levels of racial diversity within a school improve student outcomes by (a) reducing prejudiced attitudes and (b) increasing students' exposure to a variety of viewpoints (i.e., diversity of ideas; CitationAntonio, 2001; CitationAntonio et al., 2004; CitationBowen & Bok, 2000; CitationChang, Denson, Sáenz, & Misa, 2006; CitationGurin, Dey, Hurtado, & Gurin, 2002; CitationHolzer, Neumark, & Besharov, 2006; CitationNiemann & Maruyama, 2005). The effects of racial diversity are thought to be mediated by intergroup contact with peers (“contact theory”; CitationAllport, 1954).
Several complexities are inextricably linked to these hypotheses. First, both of the criterion variables (prejudiced attitudes and perceived diversity of ideas) are not directly observable and should be defined as latent variables to capture all aspects of the underlying constructs and to eliminate measurement error (e.g., CitationBollen, 1989). Second, students are naturally nested within schools. In this research area we do not consider such nesting a nuisance; rather, we are interested in disentangling the school-level effects from student-level effects. For instance, it is of interest to know the proportion of the variance in prejudice reduction that is explained by intergroup contact at the school level versus the proportion explained by individual-level intergroup contact. Failure to separate these school-level and individual-level effects forces us to rely on the untenable assumption that effects are identical across levels (e.g., CitationRaudenbush & Bryk, 2002). Finally, it is desirable to test the measurement and structural invariance of these models across race/ethnicity subsamples to test hypotheses about group differences in measurement or effects.
Some recent technical and computational developments have enabled the implementation of full information maximum likelihood (FIML) approaches to estimating disaggregated structural equation models with multilevel data (e.g., Citationdu Toit & du Toit, 2007; CitationLee & Poon, 1998; CitationLiang & Bentler, 2004). In this article we use a large, nationally representative sample of law students to test substantive theory on the role of educational diversity in higher education settings and to identify some existing practical limitations of employing disaggregated multilevel structural equation models within a real-world data situation. Finally, we use the disaggregated multilevel structural equation modeling approach and compare findings with a simpler but sometimes less appropriate aggregated modeling approach.
This study evaluates the mediated effects of racial diversity on prejudiced attitudes and perceived exposure to a diversity of ideas through intergroup contact in a large sample of law students drawn from a nationwide study of law schools. Hypothesized mediational relationships between racial diversity, intergroup contact, prejudiced attitudes, and perceived diversity of ideas are tested using a series of models that capture different aspects of the data. Population-average structural equation models and disaggregated structural equation models are briefly compared.
STATEMENT OF HYPOTHESES
Ethnic/racial diversity alone is not always sufficient to benefit student learning (e.g., CitationAllport, 1954; CitationGurin et al., 2002; CitationHolzer & Neumark, 2006; CitationNiemann & Maruyama, 2005). Supportive environments also appear to be essential for harvesting the benefits of diversity. The results of this study should provide policymakers with knowledge about which types of diversity (i.e., school composition, intergroup contact) may influence student sociopolitical attitudes and perceived educational experiences so that they can allocate limited educational resources to pursuing the most efficacious practices. Our two main hypotheses are as follows:
Intergroup contact reduces prejudice
In 1954, Allport outlined optimal conditions for reducing prejudice in environments containing diverse groups of people, a perspective that has come to be known as “contact theory.” According to Allport, mere contact between groups is not sufficient to reduce prejudice because “prejudice screens and interprets our perceptions” (p. 252). Meaningful interactions with so-called outgroups are required for prejudice reduction to occur. Allport theorized that not only must people be frequently exposed to members of an outgroup in casual situations but also that groups should (a) be of equivalent social standing in a cooperative environment; (b) share common goals; (c) cooperate; and (d) be supported and encouraged by an authority figure, institution, or custom.
A number of social psychologists have presented evidence in support of contact theory. CitationDeutsch and Collins (1951) randomly assigned African American and White families to racially integrated or racially segregated public housing. White families that were randomly assigned to the integrated condition reported more positive attitudes toward African Americans than the segregated group. This experiment worked because residents were living together in a communal, informal environment; participants were of equal status; and racial integration was normative within the public housing community. CitationAronson and Bridgeman (1979) described a “jigsaw” instructional technique that supports cooperative interaction among diverse groups of students within classrooms. By requiring students to cooperate and depend upon one another to complete assignments, Aronson and Bridgeman showed that their intervention increased empathy and interpersonal attraction across ethnic/racial groups.
In a recent meta-analysis of 515 studies of contact theory, CitationPettigrew and Tropp (2006) confirmed that increased contact with outgroups, even when not under the optimal conditions specified by Allport, showed a consistent, small-to-medium effect in reducing prejudice and increasing positive attitudes about an outgroup. Further, Pettigrew and Tropp found that the benefits of frequent contact generalize beyond the specific outgroup of exposure (e.g., to other ethnic/racial groups). The majority of studies investigating effects of intergroup contact have not tested prejudice reduction using a longitudinal design; however, studies that have done so report sustained effects.
Here, we examine whether frequent, informal interactions with diverse peers reduce ethnic/racial and social class prejudice among law students. CitationFestinger and Kelley (1951) indicated that proximity to diverse outgroups is a necessary but not sufficient condition for fostering intergroup contact. Thus, as some degree of racial diversity is a necessary condition for intergroup contact to exist, we suggest that racial diversity affects school-level variance in intergroup contact. In turn, intergroup contact explains primarily individual-level variance in prejudice, as increased intergroup contact alters individual-level prejudiced cognitions rather than the sociological environment.
Racial diversity promotes perceived diversity of ideas
In 2003, the Supreme Court ruled that racial diversity in law school benefits all students because it enables an intellectual and social exchange of a variety of beliefs and values among students who have had different life experiences (Citation Grutter v. Bollinger, 2003). In a diverse student body each student contributes to the learning of other students by providing a different perspective on issues. Furthermore, as Lempert testified in the Citation Grutter v. Bollinger (2003) case, students who have experienced a lifetime of racial discrimination may be able to offer perspectives not available to most White students. In support of this argument, CitationGurin et al. (2002) provided preliminary evidence showing that contact with people from a variety of racial groups is prospectively related to improved educational achievement and that such intergroup engagement is also related to an increased sense of social democracy/civic responsibility.
We hypothesized that, after taking into account the attributes of the law schools that students choose to attend (enrollment, sector, and selectivity) and student attributes (age, gender, race/ethnicity, standardized test scores, socioeconomic status, and political orientation), more racially diverse schools will be characterized by greater diversity of ideas. Racial diversity can only explain school-level variance in perceived diversity of ideas; however, the effect of racial diversity on perceived diversity of ideas may also be mediated by intergroup contact.
METHODOLOGICAL BACKGROUND
When a research question involves a nested analysis with only observable variables, a standard multilevel model, with appropriate centering, can permit separation of group-level effects from individual-level effects (CitationEnders & Tofighi, 2007; CitationKreft, de Leeuw, & Aiken, 1995). However, when the variables of interest are not directly measurable, it would be undesirable to give up estimation of measurement models for the sake of accounting for clustering. Although it is possible to parameterize a latent variable model with clustered data as a multilevel model, practical limitations make it difficult to incorporate latent variables into these models (CitationBauer, 2003).
CitationMuthén and Satorra (1995) discussed two approaches to modeling complex data in a latent variable framework: aggregated and disaggregated analyses. Aggregated analytical methods are useful for developing a population-average model that does not generalize to any particular sampling unit. Disaggregated analyses estimate variability in Level 1 variables across independent sampling units so that estimates particularize to individuals within a sample (e.g., students). Disaggregated analyses are far more informative but are also more difficult to estimate and they are more sensitive to model misspecification.
Disaggregated multilevel structural equation models (MSEMs) were first developed by CitationGoldstein and McDonald (1988). CitationMcDonald and Goldstein (1989) developed an analytical solution to obtain true maximum likelihood estimates for balanced and unbalanced MSEMs with continuous data; however, these solutions were impractical to implement and were not widely available. In the same year, CitationMuthén (1989) presented a maximum likelihood-based estimator (MUML) that provided an approximate solution for unbalanced data that was computationally more feasible than McDonald and Goldstein's true maximum likelihood estimator. However, MUML could not handle missing data or categorical outcomes. A number of researchers subsequently proposed estimation techniques for specific MSEM formulations throughout the 1990s and early 2000s (e.g., Citationdu Toit & du Toit, 2007; CitationLee & Poon, 1998; CitationRaudenbush, 1995). In 2004, Liang and Bentler expanded Lee and Poon's technique by deriving an accelerated expectation maximization (EM) algorithm that was useful for obtaining FIML solutions with a generalized MSEM formulation. This relatively recent development has increased the feasibility of implementing MSEM analyses with missing data and has become incorporated into a number of software programs.Footnote 1
The presence of categorical data provides an added complexity to the estimation of MSEMs. The issue of noncontinuous data in MSEMs was only recently confronted in the literature by CitationRabe-Hesketh, Skrondal, and Pickles (2004; also CitationSkrondal & Rabe-Hesketh, 2004). When latent variable indicators are categorical, there is no closed-form solution to the marginal likelihood (CitationRabe-Hesketh, Skrondal, & Pickles, 2005). In this case, the likelihood must be approximated by a computationally intensive numerical integration procedure.
A number of quantitative researchers have published expository papers demonstrating empirical examples of the use of the MSEM procedure (e.g., CitationKaplan & Elliott, 1997; CitationLiang & Bentler, 2004; B. O. CitationMuthén, 1994; CitationMuthén, Khoo, & Gustafsson, 1997; CitationSkrondal & Rabe-Hesketh, 2004). However, these examples have involved relatively idyllic data scenarios, for example, involving very simple confirmatory factor analytic or multitrait multiindicator structures. In addition, all examples except those in Skrondal and Rabe-Hesketh predated the ability to model categorical data (e.g., MSEM for categorical data was not implemented in Mplus until 2004 in Version 3).
METHOD
Sample and Participants
National data were collected from law students during their law school orientation in the fall of 2004 and again during the spring of 2007, prior to their graduation, as part of the Educational Diversity Project (EDP). Sixty-four law schools participated in our study. Institutional characteristics of the schools were provided by the American Bar Association (ABA) on law schools. Demographics, background characteristics, and institutional characteristics of the students and law schools in the sample are described in .
TABLE 1 Baseline Sample Characteristics
Fifty nationally representative, ABA-approved U. S. law schools were identified using two methods. Schools that were identified as having very high minority populations (N = 7) were oversampled from the 184 ABA-approved U.S. law schools. An additional 46 schools from the remaining 177 schools were randomly drawn. Of these schools, 1 was ineligible to participate and 2 were nonresponsive. In the schools with high minority representations, average student response rates were 75.5%, and student response rates at the remaining schools were 51.8% on average. Higher response rates in the former are partially attributable to the administration method; all students in the high minority representation sample completed surveys during law school orientation, although the other sample included some students who completed the surveys during orientation and others who took surveys home with them.Footnote 2
Administrators from 16 additional ABA-approved law schools volunteered to participate in the sample after hearing about EDP through a presentation at an annual Law School Admissions Council meeting or after reading about the study from a newsletter widely distributed to admissions counselors. Volunteer schools are not a probability sample of law schools in the United States, but they represent 12 states spanning the continental United States and characteristics of students attending these schools do not differ significantly from those of student attending schools selected in the nationally representative sample. On average, 58.2% of law students per volunteer school completed baseline surveys. Of 4,865 viable baseline participants, to date 2,695 have either completed the follow-up survey (N = 2,180) or were confirmed to have left law school prior to spring 2007 (N = 515). Taking into account the known reasons for attrition (permission to recontact not granted, invalid e-mail addresses, or law school dropout), 54.0% of nonresponders did not complete the follow-up survey for reasons that are unknown to the EDP. A listwise or pairwise deletion of cases for which follow-up data are missing might provide severely biased results if the missingness mechanism is not completely at random (CitationLittle & Rubin, 2002).
Information about the most sensitive outcome variables was collected at the baseline assessment along with many student demographic measures. Controlling for these baseline variables, nonresponse to the follow-up items is less likely to be a function of unobserved data on the outcome variables of interest. The nonresponse mechanisms that are not related to the observed covariates (i.e., dropout is potentially related to bar examination and job search-related stressors) should be uncorrelated with diversity experiences in law school. Thus, it might be reasonable to assume that missingness on the follow-up surveys is covariate-dependent and is thus ignorably missing, or at least not severely nonignorably missing (e.g., CitationLittle, 1995). If this is true, FIML estimation technique, which uses all available case data, should not provide excessively biased parameter estimates (e.g., CitationArbuckle, 1996; CitationEnders & Bandalos, 2001; CitationWothke, 2000).
Measures
Institutional characteristics
Attributes of the law school verified from ABA databases included racial diversity index (described later), school enrollment, percentage of applications accepted, and sector (public or private).
Background characteristics
Student self-reported demographics from the EDP baseline survey included age, ethnic/racial minority status, gender, Law School Admission Test (LSAT) score, political orientation (a 5-point scale ranging from 1 (extremely liberal) to 5 (extremely conservative)), and relative family household income during childhood (a 5-point scale ranging from 1 (far below average) to 5 (far above average). Self-reported age, race/ethnicity, gender, and LSAT scores were verified with the Law School Admission Council databases.
Baseline and follow-up attitudes
On both the baseline and follow-up EDP surveys, students answered questions pertaining to their sociopolitical beliefs on a Likert-type scale ranging from 1 (strongly disagree) to 5 (strongly agree). At both timepoints, students rated how much they agreed with the following statements: “In America today, every person has an equal opportunity to achieve success”; “Because Irish, Italians, Jews, and many other minorities overcame prejudice and worked their way up, Blacks should do the same without any special favors”; and “People at the bottom of the economic scale are probably lazier than those at the top.” These items comprise the prejudice factor.Footnote 3 Students who score high on the prejudiced attitudes factor are inclined to attribute economic success and failure to individual characteristics while ignoring social and historical barriers or facilitators of success.
Three diversities
Education diversity is multifaceted; it cannot be represented by a single variable or dimension. Three types of diversity were measured in this study: (1) racial heterogeneity (Racial Diversity Index; RDI), (2) frequency of contact with diverse peers (intergroup contact), and (3) perceived diversity of ideas. RDI is measured at the school level, and the other two diversities contain variance at both the individual and school level.
RDI is calculated by summing the squares of the proportions (p) of each ethnic/racial group (k = 1 … K) in a school and subtracting that number from one (Equation (Equation1); CitationLieberson, 1969).Footnote 4 If measured in this way, RDI indicates the proportion of students within a school who do not share a common race/ethnicity and is thus a succinct quantitative summary of ethnic/racial heterogeneity within a school. This formulation creates a variable that is large (closer to one) when a school has many medium-size ethnic/racial groups (i.e., heterogeneity) and is small (closer to zero) if there is a dominant ethnic/racial group (i.e., homogeneity).
The intergroup contact measure is based on student reports of frequency of interactions with peers of various ethnic/racial backgrounds during college (measured at baseline) and law school (measured at follow-up). On a 3-point scale from 1 (never) to 3 (frequently), students rated how often they interacted with African American, Asian American, Hispanic/Latino, Native American, and White peers. Frequencies were summed across the groups to create an approximately normally distributed variable ranging from 5 to 15.
Finally, the perceived diversity of ideas latent construct was composed of four indicators. On a 5-point Likert-type agreement scale, students rated the quality of class discussions at their school, how much their school is characterized by respectful exchange of political views, how much their school is characterized by respect for expression of diverse beliefs, and how open their school is to new ideas.
Time Trends
In this study, we did not have enough repeated assessment occasions to model trajectories of change over time (e.g., CitationBollen & Curran, 2006). However, we did have two assessments of both intergroup contact (experiences prior to law school and experiences during law school) and two assessments of prejudiced attitudes (upon entry to law school and upon graduation from law school). These assessments are sufficient to model residualized change.Footnote 5 First, we looked descriptively at how attitudes and experiences changed for law students over time. The mean structure of these measures was fairly constant over time for law students. A paired-samples t test, which did not take the multilevel nature of the data into account, indicated no significant change for any of the items with the exception that there is stronger agreement at follow-up for the item “People at the bottom of the economic scale are probably lazier than those at the top.” This difference is probably not attributable to increased prejudiced attitudes but rather to the specific variance in the item (e.g., law students have spent many years in school to achieve economic success). Although trends in the aggregate sample are interesting, our interest is in determining whether we can model individual perturbances in residualized change scores by investigating the effects of predictors such as racial diversity.
Model Description
After the preliminary step of estimating an aggregated structural model, we evaluated a disaggregated model. The first issue within this modeling framework concerned defining the measurement models for the latent constructs. CitationGoldstein and McDonald (1988) suggested estimating a common factor model at each data level:
The model is further simplified if there is no systematic unique variance in an item response residing at the school level (i.e., the expected value of Θ B is zero). Imposing this restriction implies that, at the school level, the only true variance in an item results from the common factor and not from any other source that is consistent for all students nested within a school. For example, within a given school, students' responses to an item measuring prejudiced attitudes would be conditionally independent after accounting for the school-level prejudice factor if the between-level residual covariance matrix is assumed to be 0. The motivation for imposing this restriction is model parsimony.
If both restrictions are tested and found to be permissible, the measurement model reduces to the more interpretable “Variance Components” formulation described by CitationRabe-Hesketh et al. (2004) and CitationSkrondal and Rabe-Hesketh (2004):
For the structural model considered in this study, latent variables (η ij , prejudiced attitudes and perceived diversity of ideas at follow-up) are regressed on student-level measured variables (x ij ; baseline prejudiced attitudes, intergroup contact during law school, intergroup contact during college, minority status, gender, LSAT score, age, political orientation, and childhood household income) and school-level measured variables (z j ; RDI, selectivity, and enrollment):
The corresponding data model for the latent response of a given item is
Analysis Plan
Educational diversity theory suggests that, after controlling for school-level variables such as enrollment, selectivity, and sector, and after controlling for student-level background characteristics such as age, minority status, gender, LSAT scores, political orientation, relative family household income during childhood, and baseline prejudice, higher levels of racial diversity within a school should directly and indirectly result in lower prejudice at follow-up than would otherwise be expected. That is, holding all else equal, students attending schools with higher racial diversity should have lower average posttest prejudice scores than their peers attending law schools with less racial diversity. Likewise, holding all else equal, student attending law schools that have more racial diversity should perceive that they were exposed to more diverse ideas than students attending less racially diverse law schools. These effects should be at least partially mediated by intergroup contact. School-level racial diversity affects group-level variance in these outcomes, whereas intergroup contact has the potential to predict either student-level and school-level variance in the outcomes.
Intergroup contact is hypothesized as a mediating construct in the model; however, items assessing intergroup contact consist of retrospective measures assessed concurrently with the items measuring perceived diversity of ideas and prejudiced attitudes at follow-up. Given this study design limitation, we are unable to infer a causal link between the proposed mediator and either of the outcomes (CitationHolland, 1988). Yet, we are interested in testing the existence of an indirect effect of racial diversity in schools on student outcomes (prejudiced attitudes and perceived diversity of ideas) through individual experiences of intergroup contact during law school. Thus, we chose to estimate a model with a directional arrow from intergroup contact to the other variables measured at follow-up but caution that we cannot establish directionality with survey methods.
Multiple-groups analysis
It is conceivable that the pattern of results might be moderated by the student race/ethnicity. Although there were a large number of White respondents at follow-up (1,113), only 125 African American, 108 Asian American, 71 Mexican/Hispanic/Latino, and 116 multiracial students responded to the follow-up survey. Unfortunately, the unbalanced nature of these data indicate that the statistical power to detect differences between minority groups in a multiple groups analysis is too low; however, given that Whites are generally the majority racial group in a school, it is sensible to distinguish between ethnic/racial minorities and Whites.
As a first step in the multiple-group MSEM analysis, measurement invariance was tested for minorities and Whites following the procedure specified CitationMillsap and Tein (2004) for multiple-group categorical data. Measurement invariance for ordinal data requires that two criteria are met: (a) items must have the same relationship to the latent variable for both groups (i.e., equal factor loadings for all items; equivalently, no differential item functioning in the slope parameters), and (b) conditional on a particular value of the latent variable, minorities and Whites must endorse a level of the item at equal rates (i.e., no differential item functioning in the thresholds). Once measurement invariance was confirmed, two structural models were tested. The first model constrained the structural paths of interest to equality (i.e., the paths originating from intergroup contact were constrained to be equal). The second model allowed these paths to be freely estimated across the two ethnic/racial groups. School-level effects are, by definition, constant for all individuals within a school and therefore cannot vary across ethnic/racial groups within schools. Model fit was compared using likelihood ratio tests with the Satorra-Bentler correction for nonnormality (CitationSatorra & Bentler, 1999).
Estimation
Mplus Version 5 (CitationMuthén & Muthén, 1998–2007) was used to estimate all structural equation models (SEMs) in this study. An FIML estimator for nonnormal and dependent data (MLR) was used for all analyses, but the weighted least squares mean and variance adjusted (WLSMV) estimator was also used to provide an idea about chi-square-based global model fit. The MLR estimator is asymptotically equivalent to the estimator proposed by CitationYuan and Bentler (2000). Adaptive Gauss-Hermite quadrature with five integration points was used to numerically evaluate the likelihood.
RESULTS
Preliminary results from the population-average model support the hypothesis that racial diversity increases intergroup contact (B = 3.12, SE =.60, β =.24)Footnote 7 and that intergroup contact increases perceived diversity of ideas (B =.11, SE =.02, β =.17) and decreases prejudiced attitudes (B = −.06, SE =.02, β = −.07). The effect of racial diversity on prejudiced attitudes and perceived diversity of ideas was entirely indirect in the aggregated model, suggesting that, for the average student, the mechanistic effects of racial diversity relating prejudiced attitudes and the perceived openness of the intellectual atmosphere is entirely due to increased peer-to-peer contact. This result seems to coincide with CitationAllport's (1954) work on prejudice reduction; he argued that prejudice reduction can only occur in the presence of constructive intergroup contact. As is shown, however, these results are not in perfect agreement with the results from the disaggregated model. LSAT scores, enrollment, and school sector were not related to the outcome variables of interest; thus, these were not included in subsequent models.
As a first step in conducting the disaggregated analysis, we evaluated two-level measurement models for the latent variables. The information criteria for the perceived diversity of ideas construct were slightly lower for the Between and Within formulation (AIC = 20,309.63, BIC = 20,446.06, sample-size adjusted BIC = 20,369.81) than for the Variance Components formulation (AIC = 20,381.23, BIC = 20,489.24, sample-size adjusted BIC = 20,428.88); however, in light of Heywood cases for between-level residuals, and because the Between and Within model converged to a potential saddle point, the Variance Components formulation was selected. Evaluation of the appropriate measurement model specification for the prejudiced attitudes factor was more straightforward. The information criteria indices indicated that the Variance Components model provided a superior fit (AIC = 16,020.54, BIC = 16,111.48, sample-size adjusted BIC = 16,060.64) to the Between and Within model (AIC = 16,023.50, BIC = 16,137.18, sample-size adjusted BIC = 16,073.64). The Variance Components formulation was selected for both latent variables; this implies that prejudiced attitudes and perceived diversity of ideas constitute the same construct across the individual and school levels and that no specific variance contributes to items at the school level.
The intraclass correlation (ICC) is estimated for latent variables by dividing the estimated variance at the between level by the total latent variable variance (between- plus within-variance) in the unconditional measurement model. As noted by CitationRaudenbush, Rowan, and Kang (1991), ICCs are generally larger for latent variables than for measured variables due to the disattenuation for measurement error. The ICC for perceived diversity of ideas was low (.03). It was higher (.14) for the prejudiced attitudes factor. The ICC indicates the proportion of the variance in the latent variable that resides at the school level, or the correlation among students resulting simply from attending the same law school. The ICC for intergroup contact during law school was estimated to be. 22.
Parameter estimates from the disaggregated model are shown in . The results are graphically displayed in . As expected, RDI was significantly related to an increased expected value of intergroup contact during law school (B = 2.95, SE =.78, β =.57). Unsurprisingly (because it is purely a school-level effect), the effect in the disaggregated model is approximately the same in magnitude as the effect found in the aggregated model. Racial diversity was hypothesized to affect perceived diversity of ideas and prejudiced attitudes through an indirect relationship with intergroup contact during law school. The strength of the relation between intergroup contact and perceived diversity of ideas was moderate but was statistically significant and positive (B =.17, SE =.02, β =.21), and the relation between intergroup contact and prejudiced attitudes was small but statistically significantly negative (B = −.10, SE =.01, β = −.14); both of these effects are larger in magnitude than those found in the aggregated model due to the disaggregation of school-level and individual-level effects.
TABLE 2 Parameter Estimates for Hypothesized and Significant Paths from the Disaggregated Educational Diversity Model
FIGURE 1 Final model. Standardized, statistically significant paths are shown. All exogenous variables are allowed to correlate (not shown). Small circles represent disturbances at the student and school levels. Variables with variance solely at the school level are shown outside the box and variables that vary across students and schools are shown inside the box.
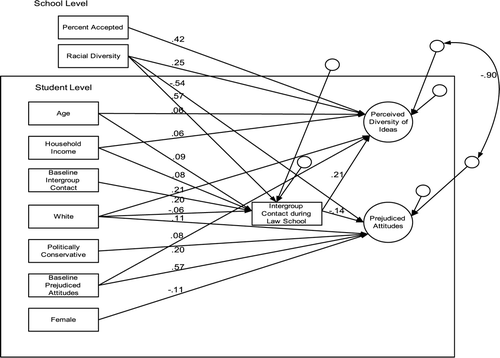
The indirect effect of RDI on prejudiced attitudes through intergroup contact was statistically significant (B1 *B2 = −.30, SE =.08) as was the indirect effect of RDI on perceived diversity of ideas (B1 *B2 =.50, SE =.14).Footnote 8 The direct effect of RDI on prejudiced attitudes at follow-up was fairly strongly negative (B = −.72, SE =.32, β = −.54); however, no direct effect of RDI was found for perceived diversity of ideas. No direct effect of RDI was found in the aggregated model, and so a researcher estimating only an aggregated model would have concluded that the benefits of racial diversity are fully explained through intergroup contact. The disaggregated model suggests that a large part of the total effect of racial diversity on prejudice attitudes remains unexplained after taking intergroup contact into account.
The prejudiced attitudes construct was negatively correlated with perceived diversity of ideas at the school level (B = −.04, SE =.02, β = −.90); prejudiced attitudes was not significantly correlated with perceived diversity of ideas at the individual level. Intergroup contact was not significantly correlated with perceived diversity of ideas or prejudiced attitudes at the school level.
The proportion of between-school variance in intergroup contact during law school was estimated to be .13 in the final model, a decrease from the unconditional ICC of .22. This change is an indication that more between-level variation than within-level variation in intergroup contact was explained in the final model. School-level racial diversity and selectivity are responsible for explaining the between-level variance. The proportion of variance at the between-level in prejudiced attitudes in the final model is .05, a decrease from .14 in the unconditional model. The proportion of variance in perceived diversity of ideas at the between level had a slight increase from .03 in the unconditional model to .05 in the final model, indicating that the model explained more of the individual-level variation in perceived diversity of ideas (i.e., by student-level background variables and by intergroup contact during law school).
Once the final single group model was determined, measurement and structural invariance across racial/ethnic groups was tested. Measurement invariance is a prerequisite for inferring structural invariance (CitationMillsap & Meredith, 2007). A likelihood ratio test (LRT) with the Satorra-Bentler scaling correction factor for nonnormality was used to compare the measurement variant and invariant models. The chi-square statistic was nonsignificant for both perceived diversity of ideas (χ2 (13) = 1.07) and for prejudiced attitudes (χ2 (9) = 1.13), indicating measurement invariance by race/ethnicity. Next, two structural models were compared, one with freely estimated paths for all within-level parameters and one with important structural paths constrained to equality across groups. The corrected LRT was nonsignificant (χ2 (3) = 1.52), indicating structural invariance of the mechanism of diversity was similar for minority students and White students. That is, the effect of intergroup contact on prejudiced attitudes and the effect of intergroup contact on perceived diversity of ideas is not a function of ethnic/racial minority status. Thus, a single group model remained the most parsimonious model of educational diversity in law schools.
displays the relation between racial diversity and the endogenous variables (prejudiced attitudes and perceived diversity of ideas) after partialing out the effects of other variables in the model. The five points indicated on the lines represent the observed quartiles for RDI in our sample. imparts the practical significance of racial diversity within law schools on the measured student outcomes. As shown, the effect of increasing RDI from the 25th to 75th percentile is associated with a −.25 standard deviation decrease in prejudiced attitudes and a .27 standard deviation increase in perceived diversity of ideas. Thus, the total effect is moderately small but is consistent with CitationPettigrew and Tropp's (2006) findings and could be increased with conscious effort on the part of school administrators to strengthen the relation between the mediator, intergroup contact, and student outcomes.
DISCUSSION
In this investigation, we aimed to contribute to the existing research and policy dialogue on the influence of educational diversity on attitudes and experiences in higher education by employing current methods in MSEM with a nationally representative sample of law students within a naturalistic setting. In this section, we first consider our substantive findings and the implications of these findings for diversity in higher education. Next, we focus on disaggregated MSEM and discuss practical implementation issues, provide advice for applied researchers, and offer directions for future developments in the methodology.
Substantive Implications
Our research questions were motivated by recent legal challenges to the use of affirmative action in education. With this analysis, we wanted to (a) examine whether or not there was empirical support for the benefits of racial diversity in education and (b) determine mediating mechanisms that could potentially serve as leverage points for school administrators. The latter, although beneficial for any academic administrator wishing to boost the effects of educational diversity, will become particularly important should race-conscious admissions policies continue to be strongly challenged across the United States. Indeed, educational institutions are now investigating race-blind alternatives to increasing diversity. At the University of California at Berkeley Law School, for instance, CitationShultz and Zedeck (2008) have identified “effectiveness factors” that can be used as alternatives to traditional indicators such as test scores to assess whether prospective law students will become effective lawyers.
Study Limitations
To measure racial diversity within schools, we used a single quantitative summary of ethnic/racial heterogeneity (RDI). This index indicates the probability that any two randomly selected students differ in race/ethnicity within a school (CitationLieberson, 1969). From this standpoint, RDI provides valuable information about the structural diversity within a school; however, there are substantive limitations to the measure .9 Namely, a school with representation from a greater number of ethnic/racial groups will have a higher RDI score, even if the proportional representation of majority students is held constant. Given that geography may impact the number of ethnic/racial groups that are represented, schools residing in coastal or border states are likely to have higher average RDI scores. Law schools may desire to construct classes that share ethnic/racial characteristics of their states rather than of the nation. This goal may or may not conflict with the goal of maximizing RDI, depending on the state. On the other hand, it may be the case that representation of a greater number of ethnic/racial groups, holding the proportion of minorities constant, has benefits in terms of reductions in outgroup prejudice and increases in diversity of ideas. This is an issue that deserves attention in future research.
Because it is not possible to manipulate experimentally racial diversity in law schools, we cannot rule out the possibility of selection bias. We attempted to minimize this inherent limitation with the inclusion of potentially confounding covariates in our observational longitudinal design. For instance, by controlling for baseline indicators of prejudice and intergroup contact, conclusions regarding the effects of educational diversity on student outcomes were not influenced by preexisting individual differences on the measures. In addition, we explored whether we could rule out self-selection of law students into racially diverse schools as an alternative explanation for our data by examining verified admissions and matriculation records. In those analyses, we observed that students, regardless of race/ethnicity and gender, generally elected to attend the most selective school to which they were accepted, not the most racially diverse school: odds were about 50:1 that the average student in our sample chose to attend the most selective school to which they were admitted, regardless of individual sociodemographic factors.
SUBSTANTIVE CONCLUSIONS AND IMPLICATIONS
The first major implication of this study is that racial diversity provides measurable benefits for students: students who attend more racially diverse law schools are more likely to have increased exposure to a novel ideas, and they are likely to be less prejudiced against outgroups than students at less diverse schools. Furthermore, the finding of no ethnic/racial group differences in the structure of the effects suggested that these benefits accrue for all students, regardless of race/ethnicity. Assuming that most institutions of higher education strive toward facilitating an exchange of new and diverse ideas, administrators should focus on achieving a racially diverse student body to the extent possible. Additionally, increasing the racial heterogeneity of the student body may also disabuse law students of prejudiced attitudes before they enter into the workforce, a goal identified by Justice O'Connor in her Grutter (2003) decision.
Second, our finding that the benefits of racial diversity are partially mediated by intergroup contact (in accordance with contact theory) suggest a mechanism through which educators can maximize the benefits of racial diversity within their school while working with a fixed level of racial diversity in the student body. By actively encouraging cooperative interactions between students of different ethnic and racial backgrounds, students will be exposed to a wider array of new ideas and outgroup prejudices will dissipate. For example, law schools may encourage students to form discussion groups with peers whose viewpoints are known to differ from their own. This particular technique was used in an experimental study conducted by CitationAntonio et al. (2004), who found that undergraduate students who were randomly assigned to racially diverse focus groups developed a higher degree of integrative complexity in their perspectives on an array of issues compared with the racially homogenous groups. This study shows that a similar type of effect generalizes to a large-scale, longitudinal study of naturally occurring law school interactions inside and outside of the classroom; students who have more frequent encounters with diverse peers rate their educational experience being more intellectually varied and open. Administrators in higher education settings may also encourage student group leaders to recruit actively students of diverse racial/ethnic backgrounds to participate in extracurricular student groups (e.g., mock court, student journals).
The findings from this study warrant future targeted study on effects of specific educational contact experiences and the nature of the contact that would lead to reduced prejudice and greater intellectual complexity.
Methodological Implications
Multilevel modelers are familiar with the consequences of ignoring nestedness in data, which include inflated standard errors and high rates of Type I error (e.g., CitationRaudenbush & Bryk, 2002). Structural equation modelers are equally well versed in the pitfalls inherent in failing to use common factor models to model unobserved constructs. Using measured variables in place of latent constructs can result in lower reliability and reduced construct validity (e.g., CitationHoyle & Robinson, 2004). Measurement models, on the other hand, provide a statistical way to model unique error variance directly in items (CitationThurstone, 1947). Psychologists frequently encounter nested data, either due to intentional sampling strategies or because of naturally occurring nestedness within the population. MSEM, to the extent that it is theoretically developed and computationally practical, is able to handle both of these situations simultaneously.
Practical Implementation Issues and Suggestions
An obvious downside to using the disaggregated approach is that numerical integration is required to implement maximum likelihood estimation when data are ordinal, as they clearly are for these survey items. Item response theorists, who have conducted nonlinear factor analysis for decades, have developed a number of numerical estimation techniques that provide unbiased, precise, and stable estimates when only one or two random effects are present (see CitationMislevy, 1986; CitationSwygert, McLeod, & Thissen, 2001; CitationWirth & Edwards, 2007). Researchers dealing with only a couple of factors have a choice between using upwards of 15 quadrature points or using fewer adaptive quadrature points. Analysts with more complex models do not have such a luxury. In our analyses, we were extremely frugal with allowing dimensions of integration. Instead of using a measurement model for baseline prejudiced attitudes, we computed a composite score; instead of declaring intergroup contact as Poisson-distributed at baseline and at follow-up, we treated it as a continuous variable; the Variance Components measurement model formulation was used instead of the more complex Between and Within measurement model formulation. Despite these analytic choices, there were still five dimensions of numerical integration required to evaluate the likelihood of the structural models. Each additional quadrature point increases computational demand by an exponential power, so only five adaptive quadrature points were used for model estimation. CitationSchilling and Bock (2005) were able to recover population parameter values with as few as two adaptive quadrature points, implying that our parameter estimates are reasonably sound.Footnote 10
METHODOLOGICAL CONCLUSIONS
Theoretically and as our findings show, there is a major advantage to using a disaggregated MSEM approach to handling complex survey data over the aggregated (i.e., population average) approach. The population-average approach is useful for drawing inferences about average trends that may appear in a population, but it is not useful for making inferences about individual variability within the population (CitationB. O. Muthén & Satorra, 1995). The disaggregated approach enables more finely tuned inferences by partitioning variance at the between level from the variance at the within level. This analysis provides (a) the correlation between students that is associated with attending the same school (intraclass correlation; ICC); (b) the variability that exists across schools and across individuals; and (c) a distinction between, and estimates of, the within-level and between-level effects. Although we were able to draw the same general conclusions from the aggregated and the disaggregated models in our study, the standardized effect sizes of the hypothesized paths in the aggregated model were typically about half of those estimated in the disaggregated models. We attribute this difference to the incorrect assumption of the aggregated model that the individual-level effects are equivalent to school-level effects that is necessary to combine effect estimates. In the disaggregated model, however, we partitioned the between-level covariances from the individual-level relationships and we were able to conduct more precise tests of the theory surrounding educational diversity.
ACKNOWLEDGMENTS
This article is based on the master's thesis of Nisha C. Gottfredson under the direction of A. T. Panter. We thank the Educational Diversity Project research team as well as Daniel Bauer and Patrick Curran. This study received funding from the Law School Admission Council (LSAC). The opinions and conclusions contained in this article are ours and do not necessarily reflect the position or policy of LSAC.
Notes
1An alternative to FIML estimation is a diagonally weighted least squares statistic, WLSMV (CitationMuthén, du Toit, & Spisic, 1997; CitationL. K. Muthén & Muthén, 1998–2007; CitationWirth & Edwards, 2007). This limited information estimator uses pairwise-deletion, is less efficient than FIML, and requires assumptions about missingness mechanisms that may be untenable in many research contexts (i.e., missing completely at random (MCAR); CitationRubin, 1976). However, there are two benefits to using the WLSMV estimator. First, computational time is not dependent on the number of random effects in the model, so it is more practical for estimating very complex models. Second, the two-stage estimation procedure enables the estimation of global fit statistics that are not currently available with maximum likelihood estimation methods within a software package (CitationAsparouhov & Muthén, 2007). Thus, we note the availability of WLSMV for researchers who have complete data, data that can be assumed to be MCAR, or who wish to multiply impute their data prior to analysis to avoid assuming an MCAR missing data process; however, we utilize an FIML estimator to obtain parameter estimates.
2Contact the authors for additional information regarding the sample and procedure for the Year 3 follow-up. Sampling and procedures for the baseline assessment can be found in CitationPanter, Daye, Allen, and Wightman (2006).
3Measurement invariance in the prejudiced attitudes factor was confirmed across time; however, we used a composite score for the baseline measure to help reduce the dimensionality of estimation. The items that measure prejudiced attitudes in this study would be expected to measure attitudes of group dominance or perceptions of a meritocracy. CitationFederico and Sidanius (2002) have identified two main components that account for dominant group members' objections to policies such as affirmative action. The first, “principled conservatism,” is linked to individualistic work ethic and politically conservative values. The second is “general group-dominance,” a desire to maintain a privileged position at the expense of other groups. CitationReyna, Henry, Korfmacher, and Tucker (2005) report that these two attitudes tend to co-occur; outgroup-based stereotypes may mediate the effect of conservatism on anti affirmative-action attitudes. After controlling for an individual's political orientation, measures of perceptions of a meritocracy should remain predictive of prejudice against economically and socially disadvantaged groups.
4Race/ethnicity proportions were obtained using known institutional characteristics from the ABA database.
5We chose to model change in outcome variables by regressing attitudes at Time 2 on attitudes at Time 1, thereby partialing initial attitudes from the follow-up attitude measures so that the estimated effects of educational diversity on attitudes were not confounded with initial attitude status (unlike change scores; see CitationMacKinnon, 2008, for a discussion of alternative methods for modeling longitudinal change with two timepoints).
6Both the AIC and the BIC penalize for overparameterization in favor of more parsimonious models. The primary reason for using the BIC is to choose the model that is closer to the “true” population generating model for the data (i.e., given the data, which model has the highest likelihood). The AIC maximizes predictive ability for future studies (CitationKuha, 2004).
7Standardized regression coefficients (labeled β) are reported to provide an indication of the effect size. The path coefficients are standardized with respect to the variance of the latent variables, as described in L. K. Muthén & Muthén, 2004.
*p <.05.
**p <.01.
***p <.001.
8 CitationPreacher and Leonardelli's (2001) online Sobel test of mediation effects was used to estimate the indirect effects. Although it would have been preferable to obtain bootstrapped estimates of the mediated effects, such an approach would have been computationally infeasible for this analysis.
9We thank an anonymous reviewer for prompting this discussion.
10Empirical evidence supported the use of Variance Components over the Between and Within specification; however, if unique variance existed at the school level and was unmodeled, the results may be slightly biased. The unmodeled variance would be pushed into factor loading or factor variance estimates, deflating factor loading estimates or increasing factor variance estimates. Inflated factor variances would result in a downwardly biased estimate of the proportion of variance explained. Given that estimation problems emerged in the fitting of the Between and Within model, we conjecture that any unmodeled specific variance at the school level would be quite minimal.
REFERENCES
- Akaike , H. 1974 . A new look at the statistical model identification . IEEE Transactions on Automatic Control , 19 : 716 – 723 .
- Allport , G. W. 1954 . The nature of prejudice , New York : Addison-Wesley .
- Antonio , A. L. 2001 . The role of interracial interaction in the development of leadership skills and cultural knowledge and understanding . Research in Higher Education , 42 : 593 – 617 .
- Antonio , A. L. , Chang , M. L. , Hakuta , K. , Kenny , D. A. , Levin , S. and Milem , J. F. 2004 . Effects of racial diversity on complex thinking in college students . Psychological Science , 15 : 507 – 510 .
- Arbuckle , J. L. 1996 . “ Full information estimation in the presence of incomplete data ” . In Advanced structural equation modeling , Edited by: Marcoulides , G. A. and Schumacker , R. E. 243 – 277 . Mahwah , NJ : Erlbaum .
- Aronson , E. and Bridgeman , D. 1979 . Jigsaw groups and the desegregated classroom: In pursuit of common goals . Personality and Social Psychology Bulletin , 5 : 438 – 446 .
- Asparouhov , T. and Muthén , B. Computationally efficient estimation of multilevel high-dimensional latent variable models . Proceedings of the 2007 JSM meeting in Salt Lake City, Utah, Section on Statistics in Epidemiology .
- Bauer , D. J. 2003 . Estimating multilevel linear models as structural equation models . Journal of Educational and Behavioral Statistics , 28 : 135 – 167 .
- Bollen , K. A. 1989 . Structural equations with latent variables , New York : Wiley .
- Bollen , K. A. , Bauer , D. J. , Christ , S. L. and Edwards , M. C. “ An overview of structural equations models and recent extensions ” . In Recent developments in social science statistics , Edited by: Kolenikov , S , Steinley , D. and Thombs , L. New York : Wiley . (in press)
- Bollen , K. A. and Curran , P. J. 2006 . Latent curve models: A structural equation perspective , Hoboken , NJ : Wiley .
- Bowen , W. G. and Bok , D. 2000 . The shape of the river: Long-term consequences of considering race in college or university admissions , Princeton , NJ : Princeton University Press .
- Chang , M. J. , Denson , N. , Sáenz , V. and Misa , K. 2006 . The educational benefits of sustaining cross-racial interaction among undergraduates . The Journal of Higher Education , 77 : 430 – 55 .
- Deutsch , M. and Collins , M. E. 1951 . Interracial housing: A psychological evaluation of a social experiment , Minneapolis : University of Minnesota Press .
- du Toit , S. H. and du Toit , M. 2007 . “ Multilevel structural equation modeling ” . In Handbook of multilevel analysis , Edited by: de Leeuw , J. and Meijer , E. 435 – 478 . New York : Springer .
- Enders , C. K. and Bandalos , D. L. 2001 . The relative performance of full information maximum likelihood estimation for missing data in structural equation models . Structural Equation Modeling , 8 : 430 – 457 .
- Enders , C. K. and Tofighi , D. 2007 . Centering predictor variables in cross-sectional multilevel models: A new look at old issues . Psychological Methods , 12 : 121 – 138 .
- Federico , C. M. and Sidanius , J. 2002 . Racism, ideology, and affirmative action revisited: The antecedents and consequences of “principled objections” to affirmative action . Journal of Personality and Social Psychology , 82 : 488 – 502 .
- Festinger , L. and Kelley , H. H. 1951 . Changing attitudes through social contact , Ann Arbor : University of Michigan, Institute for Social Research .
- Goldstein , H. and McDonald , R. P. 1988 . A general model for the analysis of multilevel data . Psychometrica , 53 : 435 – 467 .
- 2003 . Gratz. v. Bollinger, 539 U.S. 244
- 2003 . Grutter v. Bollinger, 539 U.S. 306
- Gurin , P. , Dey , E. L. , Hurtado , S. and Gurin , G. 2002 . Diversity and higher education: Theory and impact on educational outcomes . Harvard Educational Review , 72 : 330 – 366 .
- Holland , P. W. 1988 . Causal inference, path analysis, and recursive structural equations models . Sociological Methodology , 18 : 449 – 484 .
- Holzer , H. J. and Neumark , D. 2006 . Affirmative action: What do we know? . Journal of Policy Analysis and Management , 26 : 463 – 490 .
- Holzer , H. J. , Neumark , D. and Besharov , D. J. 2006 . Affirmative action: What do we know? . Journal of Policy Analysis and Management , 25 : 663 – 690 .
- Hoyle , R. H. and Robinson , J. C. 2004 . “ Mediated and moderated effects in social psychological research: Measurement, design, and analysis issues ” . In Sage handbook of methods in social psychology , Edited by: Sansone , C. , Morf , C. C. and Panter , A. T. 213 – 233 . Thousand Oaks , CA : Sage .
- Kaplan , D. and Elliott , P. R. 1997 . A didactic example of multilevel structural equation modeling applicable to the study of organizations . Structural Equation Modeling , 4 : 1 – 24 .
- Kreft , I. G. , de Leeuw , J. and Aiken , L. S. 1995 . The effect of different forms of centering in hierarchical linear models . Multivariate Behavioral Research , 30 : 1 – 21 .
- Kuha , J. 2004 . AIC and BIC: Comparisons of assumptions and performance . Sociological Methods & Research , 33 : 188 – 229 .
- Lee , S. Y. and Poon , W. Y. 1998 . Analysis of two-level structural equation models via EM type algorithms . Statistica Sinica , 8 : 749 – 766 .
- Liang , J. and Bentler , P. M. 2004 . An EM algorithm for fitting two-level structural equation models . Psychometrika , 69 : 101 – 122 .
- Lieberson , S. 1969 . Measuring population diversity . American Sociological Review , 34 : 850 – 862 .
- Little , R. J. A. 1995 . Modeling the drop-out mechanism in longitudinal studies . Journal of the American Statistical Association , 90 : 1112 – 1121 .
- Little , R. J. A. and Rubin , D. B. 2002 . Statistical analysis with missing data , Hoboken , NJ : Wiley .
- MacKinnon , D. P. 2008 . “ Longitudinal mediation models ” . In Introduction to statistical mediation analysis , Edited by: MacKinnon , D. P. 193 – 236 . Mahwah , NJ : Lawrence Erlbaum Associates .
- McDonald , R. P. and Goldstein , H. 1989 . Balanced versus unbalanced designs for linear structural relations in two-level data . British Journal of Mathematical and Statistical Psychology , 42 : 215 – 232 .
- Mehta , P. D. and Neale , M. C. 2005 . People are variables too: Multilevel structural equations modeling . Psychological Methods , 10 : 259 – 284 .
- Meredith v. Jefferson Co. Board of Education. (2007). 551 U.S. 05-915 (2007)
- Millsap , R. E. and Meredith , W. 2007 . “ Factorial invariance: Historical perspectives and new problems ” . In Factor analysis at 100: Historical developments and future directions , Edited by: Cudeck , R. and MacCallum , R. C. 131 – 152 . Mahwah , NJ : Erlbaum .
- Millsap , R. E. and Tein , J. Y. 2004 . Assessing factorial invariance in ordered-categorical measures . Multivariate Behavioral Research , 39 : 479 – 515 .
- Mislevy , R. J. 1986 . Bayes modal estimation in item response models . Psychometrika , 51 : 177 – 195 .
- Muthén , B. O. 1989 . Latent variable modeling in heterogeneous populations . Psychometrika , 54 : 557 – 558 .
- Muthén , B. O. 1994 . Multilevel covariance structure analysis . Sociological Methods & Research , 22 : 376 – 398 .
- Muthén , B. O. , duToit , S. H. C. and Spisic , D. 1997 . Robust inference using weighted least squares and quadratic estimating equations in latent variable modeling with categorical and continuous outcomes . Psychometrika , Retrieved from http://www.gseis.ucla.edu/faculty/muthen/full_paper_list.htm
- Muthén , B. O. , Khoo , S. T. and Gustafsson , J. E. 1997 . Multilevel latent variable modeling in multiple populations . Sociological Methods & Research , Retrieved from http://www.gseis.ucla.edu/faculty/muthen/full_paper_list.htm
- Muthén , B. O. and Satorra , A. 1995 . Complex sample data in structural equation modeling . Sociological Methodology , 25 : 267 – 316 .
- Muthén , L. K. and Muthén , B. O. 1998–2007 . Mplus user's guide, , 5th ed. , Los Angeles : Author .
- Niemann , Y. F. and Maruyama , G. 2005 . Inequities in higher education: Issues and promising practices in a world ambivalent about affirmative action . Journal of Social Issues , 61 : 407 – 26 .
- Panter , A. T. , Daye , C. E. , Allen , W. R. and Wightman , L. F. 2006 . An empirical study of the relationship between race and educational diversity in law schools: The Educational Diversity Project (Sampling, Methodology, and Procedures) , Chapel Hill , NC : The Educational Diversity Project . Technical report
- 2007 . Parents Involved in Community Schools v. Seattle School District No. 1, 551 U.S. 05-908
- Pettigrew , T. F. and Tropp , L. R. 2006 . A meta-analytic test of intergroup contact theory . Journal of Personality and Social Psychology , 90 : 751 – 783 .
- Preacher , K. J. and Leonardelli , G. J. March 2001 . Calculation for the Sobel test: An interactive calculation tool for mediation tests [Computer software] March , Available from http://www.quantpsy.org
- Rabe-Hesketh , S. , Skrondal , A. and Pickles , A. 2004 . Generalized multilevel structural equation modeling . Psychometrika , 69 : 167 – 190 .
- Rabe-Hesketh , S. , Skrondal , A. and Pickles , A. 2005 . Maximum likelihood estimation of limited and discrete dependent variable models with nested random effects . Journal of Econometrics , 128 : 301 – 323 .
- Raudenbush , S. W. 1995 . Maximum likelihood estimation for unbalanced multilevel covariance structure models via the EM algorithm . British Journal of Mathematical and Statistical Psychology , 48 : 359 – 370 .
- Raudenbush , S. W. and Bryk , A. S. 2002 . Hierarchical linear models: Applications and data analysis methods , Thousand Oaks , CA : Sage .
- Raudenbush , S. W. , Rowan , B. K. and Kang , S. J. 1991 . A multilevel, multivariate model for studying school climate with estimation via the EM algorithm and application to U.S. high-school data . Journal of Educational Statistics , 16 : 295 – 330 .
- Reyna , C. , Henry , P. J. , Korfmacher , W. and Tucker , A. 2005 . Examining the principles in principled conservatism: The role of responsibility stereotypes as cues for deservingness in racial policy decisions . Journal of Personality and Social Psychology , 90 : 109 – 128 .
- Rubin , D. B. 1976 . Inference and missing data . Biometrika , 63 : 581 – 592 .
- Satorra , A. and Bentler , P. 1999 . A scaled difference chi-square test statistic for moment structure analysis . Psychometrika , 66 : 507 – 514 .
- Schilling , S. and Bock , R. D. 2005 . High-dimensional maximum marginal likelihood item factor analysis by adaptive quadrature . Psychometrika , 70 : 533 – 555 .
- Schwarz , G. 1978 . Estimating the dimension of a model . Annals of Statistics , 6 : 461 – 464 .
- Shultz , M. M. and Zedeck , S. 2008 . Final report: Identification, development, and validation of predictors for successful lawyering Retrieved December 10, 2008, from http://www.law.berkeley.edu/
- Skrondal , A. and Rabe-Hesketh , S. 2004 . Generalized latent variable modeling: Multilevel, longitudinal and structural equations models , Boca Raton , FL : Chapman & Hall .
- Stoel , R. D. , Garre , F. G. , Dolan , C. and van den Wittenboer , G. 2006 . On the likelihood ratio test in structural equation modeling when parameters are subject to boundary constraints . Psychological Methods , 11 : 439 – 455 .
- Swygert , K. , McLeod , L. D. and Thissen , D. 2001 . “ Factor analysis for items scored in more than two categories ” . In Test scoring , Edited by: Thissen , D. and Wainer , H. 217 – 250 . Hillsdale , NJ : Erlbaum .
- Thurstone , L. L. 1947 . Multiple factor analysis , Chicago : University of Chicago Press .
- Wirth , R. J. and Edwards , M. C. 2007 . Item factor analysis: Current approaches and future directions . Psychological Methods , 12 : 58 – 79 .
- Wothke , W. 2000 . “ Longitudinal and multi-group modeling with missing data ” . In Modeling longitudinal and multiple group data: Practical issues, applied approaches and specific examples , Edited by: Little , T. D. , Schnabel , K. U. and Baumert , J. 219 – 240 . Mahwah , NJ : Erlbaum .
- Yuan , K. H. and Bentler , P. M. 2000 . Three likelihood-based methods for mean and covariance structure analysis with nonnormal missing data . Sociological Methodology , 28 : 363 – 396 .