Abstract
New information on the timing of past ruptures, size of single-event displacements and Holocene dextral slip rate of the Wairarapa and Ōhariu faults has become available from recent geological studies. This information is used to evaluate the conditional probability of rupture over the next 100 yr using four different recurrence–time models, allowing for data and parameter uncertainties. The sensitivity of estimates to data and distributional assumptions is examined. The southern Wairarapa Fault has a probability of rupture in the next 100 yr of c. 7.7, 1.3, 2.3 and 4.3% under the exponential, lognormal, Weibull and inverse Gaussian models, respectively, based on preferred data inputs. Corresponding estimates for the Ōhariu Fault are c. 4.3, 5.1, 3.9 and 5.1%. A logic tree with subjectively assigned weights is suggested to combine such differing time-dependent estimates. This gives a 100-yr conditional probability of rupture of c. 3.0% for the southern Wairarapa Fault and c. 4.9% for the Ōhariu Fault.
Introduction
The Wairarapa Fault ruptured in the AD 1855 magnitude 8+ Wairarapa, New Zealand, earthquake which generated severe levels of shaking (Modified Mercalli Instensity [MMI] ≥ IX) throughout much of the Wellington region (Grapes & Downes Citation1997). The Ōhariu Fault extends through Porirua city and within c. 5 km of central Wellington city (). Both these active faults – the Wairarapa Fault and the Ōhariu Fault – pose a significant risk to life, property and societal infrastructure. In fact, perceived earthquake hazard and loss in the Wellington region is dominated by these two faults, along with the Wellington–Hutt Valley segment of the Wellington Fault, at return periods greater than a few hundred years (Smith & Harmsen Citation2010; Stirling et al. Citation2012). Accordingly, the conditional probability of rupture of the Wairarapa Fault and the Ōhariu Fault is a matter of great importance. A re-evaluation of the conditional probability of rupture of the southern Wairarapa Fault and of the Ōhariu Fault is the primary goal of this article. The term ‘southern Wairarapa Fault’ is used here to mean that portion of the Wairarapa Fault southwest of its intersection with the Carterton and Masterton faults ().
Figure 1 Site locations and active faults covered in this study. A, Tectonic setting of New Zealand with the Alpine Fault, Marlborough Fault System, Hikurangi subduction zone, and Taupó Volcanic Zone denoted, respectively, by the abbreviations AF, MFS, HSZ and TVZ. Numbered arrows depict azimuth and rate of Pacific Plate motion relative to Australian Plate. B, Active faults of central New Zealand, with the southern Wairarapa Fault highlighted in bold red (including the AD 1855 rupture of the Wharekauhau Fault), the Ōhariu Fault highlighted in bold yellow, and the Wellington–Hutt Valley segment of the Wellington Fault highlighted in bold blue. Site locations (white dots) are as follows (in alphabetical order): CC, Cross Creek; LK, Lake Kohangapiripiri; MK, MacKays Crossing; OV, Ōhariu Valley; PB, Pigeon Bush; RL, Riverslea; TH, Turakirae Head; WR, Waiohine River. Carterton and Masterton faults are labelled Cf and Mf, respectively. The urban centres (black dots) of Porirua city and Wellington city are shown, respectively, as P and W.
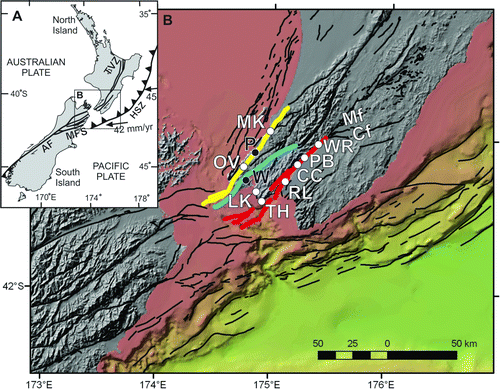
The basic statistical method adopted in this study is that of Rhoades et al. (Citation1994), with modifications described by Rhoades & Van Dissen (Citation2003) and applied by Rhoades et al. (Citation2004) to the major faults in the Wellington area, including the Wairarapa and Ōhariu faults and, more recently, applied by Rhoades et al. (Citation2011) to the Wellington–Hutt Valley segment of the Wellington Fault. In this method, the probability of rupture of the fault in some future period of interest is expressed as a single value that accounts for both data and parameter uncertainties. As in previous studies, a range of different recurrence–time distributions is considered – namely the exponential, lognormal, Weibull and inverse Gaussian (or Brownian passage-time) distributions.
• | The exponential recurrence–time distribution corresponds to a stationary Poisson process commonly adopted for seismic hazard analysis, in which the hazard is time-invariant. | ||||
• | The lognormal model has been widely used for rupture recurrence (Nishenko & Buland Citation1987). For this model the hazard is zero immediately after a rupture, rises gradually to a peak and then tails off asymptotically to zero as the elapsed time greatly exceeds the mean recurrence interval. | ||||
• | The Weibull distribution is widely used in failure–time modelling for manufactured items, and was proposed as a model for fault-rupture recurrence by Hagiwara (Citation1974). For the range of values of the shape parameter considered here, the hazard under this model increases monotonically from zero immediately following a rupture until the time of the next failure. | ||||
• | The inverse Gaussian (Brownian passage–time) distribution was proposed by Ellsworth et al. (Citation1999) and Matthews et al. (Citation2002) as a physically realistic model of earthquake occurrence, and at present appears to be the most generally accepted time–variable model (National Institute of Advanced Industrial Science and Technology Citation2007; Field et al. Citation2009). For this model the hazard is zero immediately after a rupture, rises gradually to a peak and then tails off asymptotically to a positive constant as the elapsed time greatly exceeds the mean recurrence interval. |
The Rhoades & Van Dissen (Citation2003) methodology requires knowledge of the distribution of the long-term average slip rate and its uncertainty, the mean single-event displacement and its uncertainty, and the dates of known recent ruptures and their uncertainties. It also requires specification of prior distributions for the parameters of the recurrence–time distributions. The prior distributions adopted here are constructed in the same way as those used by Rhoades & Van Dissen (Citation2003) and Rhoades et al. (Citation2004, Citation2011) for the exponential, lognormal, Weibull and inverse Gaussian models.
The principal differences between the present evaluation and that of Rhoades et al. (Citation2004) lie in the significant amount of new geological data acquired along both the southern Wairarapa Fault and the Ōhariu Fault since 2004, much of it as part of the ‘It's Our Fault’ project (Van Dissen et al. Citation2009, Citation2010). These new data provided information affecting estimates of the size of the average single-event displacement and the long-term average slip rate of the respective faults, and hence their respective average recurrence interval between ruptures. They also provided new information affecting estimates of the times of occurrence of recent past ruptures of the faults. These new data and results are summarised below as they pertain to our re-evaluation of conditional probability of rupture of the southern Wairarapa Fault and the Ōhariu Fault.
The available geological evidence on the uncertainties in the timing of past ruptures is represented by simple geometrical distributions (uniform, when there is only a bounding interval; triangular, when there is a preferred date within a bounding interval; or trapezoidal, when there is preferred range within a wider bounding interval). Attempting more detailed descriptions of the uncertainties would add additional complexity without significantly impacting on the conditional probability estimates (see below). The conditional probabilities are expected to be more sensitive to the distributions of average single-event displacements and slip rates. Considering alternative specifications of these distributions, where such alternatives could reasonably be entertained, is the main focus of a sensitivity analysis of the conditional probability estimates.
Wairarapa Fault input data
Timing of past surface ruptures
Combining results from the historic record, trenching investigations at Riverslea, Cross Creek and Pigeon Bush (Little et al. Citation2009), uplifted beach ridges at Turakirae Head (McSaveney et al. Citation2006), and lake disturbances at Lake Kó;hangapiripiri (Cochran et al. Citation2007) the timing of six ruptures of the Wairarapa Fault can be constrained (). These are assumed here to be the six most recent surface ruptures. The age constraints for these ruptures are summarised below.
Most recent event
The most recent rupture of this portion of the Wairarapa Fault was in AD 1855 (155 yr ago, using AD 2010 as a datum) (; ).
Event II
Constraints on the timing of the penultimate surface rupture come from Riverslea, Cross Creek and Pigeon Bush (Little et al. Citation2009), with Cross Creek providing the best control (800–920 cal yr BP) (cal yr BP = calendar years before AD 1950). This event is not recognised at Turakirae Head, or at Lake Kó;hangapiripiri. The Cross Creek age range is defined by the minimum and maximum 95% confidence limits of the respective bounding C-14 ages for this event (in this case, ages from samples CC-1-14 and CC-1-13; Little et al. Citation2009). Using AD 2010 as a datum, the best estimate for the timing of Event II is 860–980 yr ago. For our calculations of conditional probability of rupture we adopt a uniform probability distribution between 860 and 980 yr for Event II.
Event III
There are three sites along the southern Wairarapa Fault that constrain the timing of Event III: Cross Creek (2110–2340 cal yr BP; Little et al. Citation2009), Turakirae Head (2060–2380 cal yr BP; BR3 of McSaveney et al. Citation2006), Lake Kó;hangapiripiri (2000–2300 cal yr BP; transition D–E of Cochran et al. Citation2007). Taken collectively, and using AD 2010 as a datum, the best estimate for the timing of Event III is 2170–2360 yr ago (). In our probability calculations we adopt a uniform probability distribution between 2170 and 2360 yr for this event ().
Table 1 Preferred distributions of input data for computation of conditional probability of rupture the southern Wairarapa Fault and the Ōhariu Fault.
Event IV
Event IV is recorded at Cross Creek and Lake Kó;hangapiripiri, but not at Turakirae Head. At Cross Creek, Event IV has an age of 3070–3690 cal yr BP (Little et al. Citation2009), and at Lake Kó;hangapiripiri it has an age of 3300–3900 cal yr BP (transition C–D of Cochran et al. Citation2007). Taken collectively, and using AD 2010 as a datum, the best estimate for the timing of Event IV is 3360–3750 yr ago. In our probability calculations we adopt a uniform probability distribution between 3360 and 3750 yr for Event IV.
Event V
This surface rupture event is recorded at Cross Creek (4840–5210 cal yr BP; Little et al. Citation2009), Turakirae Head (4710–5350 cal yr BP; BR4 of McSaveney et al. Citation2006) and Lake Kó;hangapiripiri (4800–5400 cal yr BP; transition B–C of Cochran et al. Citation2007). Taken collectively, and using AD 2010 as a datum, the best estimate for the timing of Event V is 4900–5270 yr ago. In our probability calculations we adopt a uniform probability distribution between 4900 and 5270 yr for Event V.
Event VI
The timing of Event VI is constrained at Turakirae Head (6610–6920 cal yr BP; BR5 of McSaveney et al. Citation2006) and Lake Kó;hangapiripiri (6300–7250 cal yr BP; transition A–B of Cochran et al. Citation2007); the Cross Creek record of Little et al. (Citation2009) does not go back this far in time. Taken collectively, and using AD 2010 as a datum, the best estimate for the timing of Event VI is 6670–6980 yr ago. In our probability calculations we adopt a uniform probability distribution between 6670 and 6980 yr for Event VI.
Slip rate
At Waiohine River there is a flight of alluvial terraces that are progressively displaced, both vertically and dextrally, by the Wairarapa Fault (Lensen & Vella Citation1971; Grapes & Wellman Citation1988; Carne et al. Citation2011). This is the only site along the Wairarapa Fault, south of its intersection with the Carterton and Masterton faults, where well preserved and dated landforms having an age of > 10 ka are cut and offset by the fault in a place where it consists of a single strand. Only at this site can long-term dextral slip rate be estimated. In this section, we summarise age and displacement data for two offset terraces at Waiohine River (the Waiohine surface and the next youngest terrace, Terrace B), and use these data to collectively constrain a dextral slip rate for the Wairarapa Fault at this locality.
Waiohine surface age
In southeastern North Island, the most extensive and lowest fluvial aggradation terrace is termed the Waiohine terrace comprising, collectively, its top – the Waiohine Surface – and its gravely aggradation fill – the Waiohine Gravel (Vella Citation1963). The Waiohine terrace is regarded as equivalent to the Ohakean terrace, both surface and fill, elsewhere in the North Island (Palmer Citation1984). The age of aggradation, cessation of aggradation and subsequent abandonment of the Ohakean (=Waiohine) terrace has been the subject of a good deal of recent investigation (Formento-Trigilio et al. Citation2003; Litchfield & Berryman Citation2005; Wang & Grapes Citation2008; Carne et al. Citation2011; see also references cited therein). Litchfield & Berryman (Citation2005) interpret the timing of abandonment of the Ohakean terrace in the three largest catchments in southeastern North Island to be 15–20 ka. Whereas, in some smaller subcatchments and/or locations closer to the range front, abandonment appears to be younger, c. 10–12 ka (Wang & Grapes Citation2008; Little et al. Citation2009).
At Waiohine River itself, Wang & Grapes (Citation2008) report three luminescence ages for the Waiohine terrace from silts with some fine to medium gravel that directly overlie coarse alluvial gravel. The gravel content of the sampled silt sequence suggest that these samples are more likely to have been collected from the top of the aggradation fill package, or from overbank alluvial deposits, rather than from loess. The three samples were all collected from within 0.6–0.8 m from the ground surface, and have ages of 10.0±0.8, 10.2±1.2 and 13.0±0.9 ka (samples MR-1, Wh-2 and Wh-1, respectively, of Wang & Grapes Citation2008). At 2σ uncertainty, all three samples overlap in the age range of 11.2–11.6 ka. We regard this, c. 11.4 ka, as the best estimate for the age of abandonment of the Waiohine terrace at this locality. For a minimum bounding age for the abandonment of the Waiohine terrace at this locality we adopt a value of 10 ka, based on the mean of the youngest of the three dates listed above. As a maximum bounding age we adopt a value of 17.5 ka, based on the mid-point of the Litchfield & Berryman (Citation2005) 15–20 ka age estimate for the cessation of aggradation in the lower North Island. For the purposes of our slip-rate calculations, we adopt a triangular probability distribution for the age of abandonment of the Waiohine terrace at Waiohine River with maximum probability at 11.4 ka, and with minimum and maximum bounds at 10 and 17.5 ka, respectively.
Waiohine surface displacement
As described in Carne et al. (Citation2011), there is no direct measurement of dextral displacement of the Waiohine surface at Waiohine River, but one can be approximated by applying the horizontal to vertical displacement ratio derived from younger offset terraces in the Waiohine sequence to the known vertical displacement of the Waiohine surface. Wellman (Citation1955), and more recently Carne et al. (Citation2011), have done just this, and Carne et al. estimate a dextral displacement of 129±18 m for the Waiohine surface which encompasses the c. 120 m estimate of Wellman (Citation1955). For the purposes of our slip-rate calculations, we adopt a triangular probability distribution for the estimated dextral displacement of the Waiohine surface with maximum probability at 129 m, and minimum and maximum bounds at 111 and 147 m, respectively.
Waiohine surface dextral slip rate
The above estimates of Waiohine surface displacement (triangular distribution: 111 m, 129 m, 147 m) and age (triangular distribution: 10 ka, 11.4 ka 17.5 ka) yield a Wairarapa Fault dextral slip rate with a mean of 10.1 mm/yr and standard deviation (SD) of 1.4 mm/yr (A). This rate and its uncertainty were determined by drawing numerous independent paired Monte Carlo samples from the distributions of displacement and age, and for each paired sample dividing the displacement by age. The 95% confidence bounds for the slip rate are 7.6 and 12.7 mm/yr.
Figure 3 Histograms of Monte Carlo samples from Wairarapa Fault slip rate distributions estimated from Waiohine River terrace data. A, Slip rate estimated from Waiohine surface displacement and age (mean 10.1 mm/yr, SD 1.4 mm/yr); B, Minimum slip rate estimated from Terrace B displacement and maximum age; C, Waiohine surface slip-rate values excluded by Terrace B minimum slip-rate values; D, Combined, preferred, Wairarapa Fault slip-rate values (mean 10.7 mm/yr, SD 1.0 mm/yr) based on Waiohine surface slip-rate values that are consistent with Terrace B minimum slip rate.
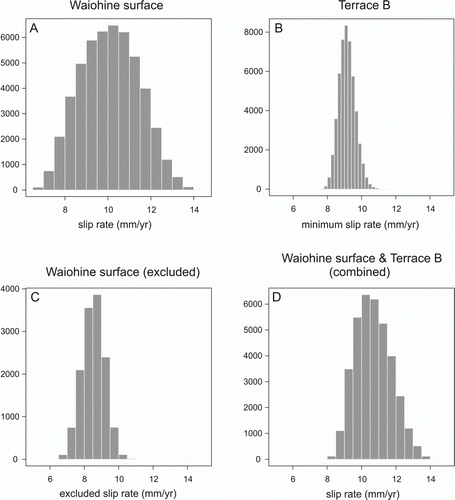
Terrace B age
At Waiohine River the next terrace below the Waiohine surface is Terrace B (adopting the nomenclature of Lensen & Vella Citation1971 and Carne et al. Citation2011). Terrace B is younger than the Waiohine surface and, accordingly, the three optically stimulated luminescence (OSL) ages of Wang & Grapes (Citation2008) (10.0±0.8, 10.2±1.2, 13.0±0.9 ka) for the Waiohine surface provide, almost certainly, maximum age constraints for the abandonment of younger Terrace B. Averaging the three OSL ages of Wang & Grapes (Citation2008) and allowing for their uncertainties, we obtain an estimate of 11.1±0.6 ka for the maximum age of abandonment of Terrace B.
Carne et al. (Citation2011) dated four OSL samples from Terrace B. Three of these samples returned ages older than the ages of the Wang & Grapes (Citation2008) samples collected from the higher Waiohine terrace. We suspect that these three samples of Carne et al. (Citation2011) were incompletely bleached, and have retuned ages that are older than the true age of deposition. The fourth sample of Carne et al. (Citation2011) from Terrace B was collected from a silt c. 0.5 m above the gravel tread of Terrace B, and yielded an age of 7.4±0.6 ka (sample Wai-10b; Carne et al. Citation2011). The age of this sample is about half that of the next youngest Terrace B sample of Carne et al. (Citation2011), and is stratigraphical consistent with the Wang & Grapes (Citation2008) ages from the older Waiohine terrace. It is possible that the 7.4±0.6 ka age of sample Wai-10b (Carne et al. Citation2011) provides a reasonable estimate for the age of abandonment of Terrace B, but there are two conflicting aspects of the sample that make it difficult to be sure of this. The other three Terrace B samples of Carne et al. (Citation2011) appear to be incompletely bleached, and it is possible that sample Wai-10b, though significantly younger, could also be incompletely bleached. Thus, it is possible that Terrace B could be younger than 7.4±0.6 ka. Conversely, sample Wai-10b was collected from c. 0.5 m above the gravel tread of Terrace B, and may provide only a minimum age for the abandonment of Terrace B. Thus, it is possible that Terrace B could be older than 7.4±0.6 ka. Because of the ambiguity surrounding the interpretation of sample Wai-10b, we do not use its age of 7.4±0.6 ka in our attempts to constrain the age of Terrace B. As such, we are left only with the three ages of Wang & Grapes (Citation2008) from the older Waiohine terrace that provide maximum constraints on the age of abandonment of Terrace B. For the purposes of our slip-rate calculations, we adopt a maximum age of abandonment of Terrace B of 11.1±0.6 ka.
Terrace B displacement
Carne et al. (Citation2011) documents a dextral displacement of Terrace B of 101±3 m. For the same terrace, Grapes & Wellman (Citation1988) report a dextral displacement of 97 m, and Lensen & Vella (Citation1971) report a displacement of 99 m. For our slip-rate calculations, we use the Carne et al. (Citation2011) value and adopt a triangular probability distribution for the dextral displacement of Terrace B with maximum probability at 101 m, and minimum and maximum bounds at 98 and 104 m, respectively.
Terrace B dextral slip rate
By sampling from the above estimates of Terrace B displacement (triangular distribution: 98 m, 101 m, 104 m) and maximum age (normal distribution: mean 11.1 ka and SD 0.6 ka), the distribution of a minimum bound on Wairarapa Fault dextral slip rate is found, with a mean of 9.2 mm/yr and a SD of 0.5 mm/yr (B).
Combined Wairarapa Fault dextral slip rate at Waiohine River
Numerous random samples were drawn in parallel from the above distributions for the Waiohine surface dextral slip rate and the Terrace B minimum dextral slip rate. The combined distribution was formed by retaining only those Waiohine surface slip rate samples which exceeded the corresponding Terrace B minimum slip rate samples (see C). The resulting combined, preferred, dextral slip-rate distribution has a mean of 10.7 mm/yr and a standard deviation (SD) of 1.0 mm/yr (D). Minimum and maximum 95% confidence bounds on the combined dextral slip rate are c. 8.9 and 12.8 mm/yr, respectively. This distribution is regarded as lognormally distributed in our calculation of the conditional probability of rupture ().
Sensitivity runs – slip rate
Given the uncertainties regarding Wairarapa Fault dextral slip rate, and to gauge the sensitivity of the conditional probability results to slip rates other than our preferred 10.7±1.0 mm/yr (), we undertake sensitivity runs adopting the following elevated and reduced rates ():
1. | elevated rate normally distributed about the +2σ value of the preferred rate with the same SD as the preferred rate (i.e. an elevated rate of 12.7±1.0 mm/yr), and | ||||
2. | a reduced rate normally distributed about the −2σ value of the preferred rate with the same SD as the preferred rate (i.e. 8.7±1.0 mm/yr). |
Table 2 Input data variations considered in the sensitivity studies for the southern Wairarapa Fault and the Ōhariu Fault.
Single-event displacement
South of Carterton, there are three sites on the Wairarapa Fault where it is both expressed as a single strand, and where dextral displacements resulting from both the Most Recent and the Penultimate (Event II) surface ruptures can be measured at the same location. Two of these sites are near Pigeon Bush (Pigeon Bush 1 and Pigeon Bush 2 sites of Rodgers & Little Citation2006), and the other is at Waiohine River (Carne et al. Citation2011) c. 16 km to the northeast. These displacements are summarised in , and based on these, an average single-event dextral displacement of 13.6 m is estimated for this portion of the fault with a between-site SD of 2.66 m and a between-event SD of 1.31 m.
Table 3 Co-located one-event (most recent) and two-event (most recent plus penultimate) dextral displacements for single-strand sites on the southern Wairarapa Fault, and derived estimates of displacement in the penultimate event.
The implied between-event coefficient of variation (CoV) (c. 0.1) is low compared with previous studies in which the multiple single-event displacements have been measured at a single site (Hecker & Abrahamson Citation2002). Because the c. 0.1 between-event CoV is based on only two events, it is not a robust statistic, and we prefer to use the global average value of 0.35 from the study of Hecker & Abrahamson (Citation2002), which corresponds to a between-event SD of 4.76 m. Therefore, the observed displacement in each event is regarded as having a between event variance of 4.762 and an additional variance component of 2.662/2 to account for the site-to-site variation (it being the average over two sites). Summing these gives a total variance of 26.2 m2 for the displacement in each event. The mean displacement over two events thus has a SD of (26.2/2)0.5, or 3.6 m. Accordingly, in our probability computations we adopt a mean single-event displacement of 13.6±3.6 m (), and regard it as lognormally distributed, since it must be non-negative.
Sensitivity runs – single-event displacement
In Rhoades et al. (Citation2004), an upper value of the CoV of single-event displacement of 0.57 was considered. To afford comparability with this earlier study, a high-end value of 0.57 for the CoV of single-event displacement is considered as part of our sensitivity analysis ().
Ōhariu Fault input data
Timing of past surface ruptures
Most Recent Event
Litchfield et al. (Citation2004) constrain the timing of the most recent metre-scale surface rupture of the Ōhariu Fault to be at 1000–1050 cal yr BP. Using AD 2010 as a datum, the best estimate for the timing of this event is 1060–1110 yr ago. In our probability calculations, we adopt a uniform probability distribution between 1060 and 1110 yr for this surface rupture event (; ).
Figure 4 Input data distributions for the times of past surface ruptures on the Ōhariu Fault. A, preferred values and distributions. B, sensitivity options, including the possible occurrence of Event FB and an alternative probability distribution for the timing of Event II.
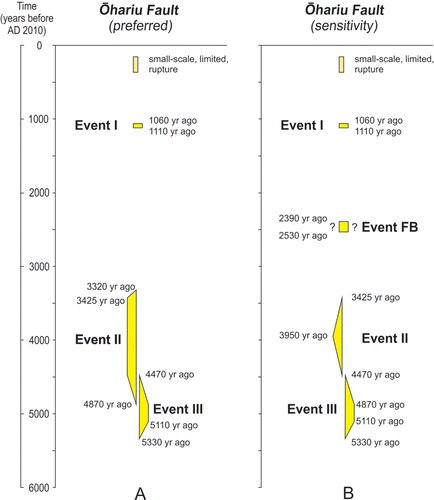
Litchfield et al. (Citation2010) document the occurrence of a much younger decimetre-scale surface rupture of a limited along-strike length of the Ōhariu Fault. The timing of this small-scale rupture is post-360 yr ago (AD 2010 datum), and it may represent either a small primary Ōhariu Fault rupture, or a triggered-slip event associated with a large earthquake on a nearby fault. Because of the small size of this young rupture, and its apparent limited extent, we do not incorporate this event into our calculations of conditional probability of rupture which aim to estimate the hazard of rupture of metre-scale displacement, i.e. large magnitude, Ōhariu Fault earthquakes.
Event II
The timing of Event II is constrained to have occurred between 3260 and 4810 cal yr BP (Litchfield et al. Citation2006). In our calculations of conditional probability we adopt a trapezoidal-shaped probability distribution for the timing of Event II (). The minimum bound of the age range of Event II (3320 yr ago; AD 2010 datum) is defined by the minimum of the 95% calibrated age range of the relevant bounding radiocarbon age (T1-8 of Litchfield et al. Citation2006). The probability distribution rises to a maximum at the mid-point of the 95% calibrated age range of the relevant bounding radiocarbon age (3425 yr ago; T1-8 of Litchfield et al. Citation2006). The maximum bound of the age range of Event II (4870 yr ago; AD 2010 datum) is defined by the maximum of the 95% calibrated age range of the relevant bounding radiocarbon age (T1-5 of Litchfield et al. Citation2006). From its maximum bound, the probability distribution rises to a maximum at 4470 yr ago (AD 2010 datum) based on the minimum of the 95% calibrated age range of the relevant bounding radiocarbon age (T1-5 of Litchfield et al. Citation2006). We chose the minimum of the 95% calibrated age range of sample T1-5 as the point at which maximum probability is reached, as apposed to the mid-point of its age range, because sample T1-5 provides not only the maximum bounding age for Event II but also the minimum bounding age for Event III (see Litchfield et al. Citation2006 for more detail).
Event III
The timing of Event III is constrained to have occurred between 4410 and 5270 cal yr BP (Litchfield et al. Citation2006). In our calculations of conditional probability, we adopt a trapezoidal-shaped probability distribution for the timing of Event III (). The minimum bound of the age range of Event III (4470 yr ago; AD 2010 datum) is defined by the minimum of the 95% calibrated age range of the relevant bounding radiocarbon age (T1-5 of Litchfield et al. Citation2006). The probability distribution rises to a maximum at the maximum of the 95% calibrated age range of the relevant bounding radiocarbon age (4870 yr ago; T1-5 of Litchfield et al. Citation2006). The maximum bound of the age range of Event III (5330 yr ago; AD 2010 datum) is defined by the maximum of the 95% calibrated age range of the relevant bounding radiocarbon age (T1-6 of Litchfield et al. Citation2006). From its maximum bound, the probability distribution rises to a maximum at 5110 yr ago (AD 2010 datum) based on the mid-point of the 95% calibrated age range of the relevant bounding radiocarbon age (T1-6 of Litchfield et al. Citation2006).
Sensitivity runs – timing of past surface ruptures
In our sensitivity analyses, we entertain the following two modifications to Ōhariu Fault surface rupture event timings ().
1. | Heron et al. (Citation1998) identify and date (2330–2470 cal yr BP) a buried forest/root horizon in their Ōhariu Valley 2 trench. They tentatively suggest that burial of this forest was the result of rupture of the Ōhariu Fault which caused growth of the fault scarp, ponding behind the uphill-facing scarp and subsequent tree death in the ponded area. However, neither a buried forest horizon nor a rupture event of this age have been identified in any of the other trenches on the Ōhariu Fault. Thus, despite the fact that the age of death of the forest/root layer coincides with the timing of a synchronous, apparently coseismic, subsidence event in nearby water bodies (Cochran et al. Citation2007), the nature of this event still remains speculative. The forest burial could have been the result of a non-tectonic event such as a flood or a landslide, or an earthquake event not related to rupture of the Ōhariu Fault. Because an Ōhariu Fault surface rupture event at 2330–2470 cal yr BP has not been documented in any other trench across the fault, we do not include this event in our preferred chronology of past surface rupture event timings (A). Nevertheless, in some of our sensitivity runs we do entertain the possible occurrence of this event: Event FB (FB for forest burial) at 2390–2530 yr ago (AD 2010 datum) with a uniform probability distribution between these bounding ages (B). | ||||
2. | In the trench that documents the occurrence and constrains the timing of Event II (MacKays Crossing trench T1 of Litchfield et al. Citation2006) the stratigraphic and structural relationships suggest that Event II could have occurred half-way in time between the ages of the two bounding radiocarbon dates. As such, in some of our sensitivity runs we entertain an alternative age distribution for Event II; triangular, instead of trapezoidal, with minimum and maximum bounds of 3425 and 4470 yr ago, respectively, and maximum probability at 3950 yr ago (AD 2010 datum) (B). |
Slip rate
The dextral slip rate value used in Rhoades et al. (Citation2004), based on results presented in Van Dissen & Berryman (Citation1996) and Heron et al. (Citation1998), is still relevant and the best available: 1–2 mm/yr, uniformly distributed between these two bounds ().
Sensitivity runs – slip rate
In order to gauge the sensitivity of the Ōhariu Fault conditional probability results to slip-rate distributions other than our preferred uniform distribution between 1–2 mm/yr, we undertake sensitivity runs adopting the following three alternative slip-rate distributions ():
1. | A slip-rate distribution that favours the middle of preferred range: 1.5±0.5 mm/yr, normally distributed. | ||||
2. | A slip-rate distribution that favours the slower end of the preferred range: one-sided triangular distribution with minimum and maximum bounds of 1 and 2 mm/yr, respectively, and maximum probability at 1 mm/yr. | ||||
3. | A slip-rate distribution that favours the faster end of the preferred range: one-sided triangular distribution with minimum and maximum bounds of 1 and 2 mm/yr, respectively, and maximum probability at 2 mm/yr. |
Single-event displacement
As summarised in Heron et al. (Citation1998) and Litchfield et al. (Citation2006), the best estimate for mean single-event displacement is 3.7 m (this is based on displacements assumed to relate only to the most recent surface rupture). In our probability calculations, we adopt a CoV of single-event displacement of 0.35 based on Hecker & Abrahamson (Citation2002). Accordingly, in our conditional probability calculations, our preferred value for single-event displacement is 3.7±1.3 m ().
Sensitivity runs – single-event displacement
In Rhoades et al. (Citation2004) an upper value of CoV of single-event displacement of 0.57 was considered. To afford comparability with this earlier study, a high-end value of 0.57 for CoV is considered as part of our sensitivity analyses. This implies a single-event displacement value of 3.7±2.1 m ().
Statistical method
The statistical method applied here is the same as that described by Rhoades & Van Dissen (Citation2003) in a study of the conditional probability of rupture of the Alpine Fault, and applied by Rhoades et al. (Citation2004, Citation2011) to the major upper-plate faults of the Wellington region. This method is an elaboration of that proposed by Rhoades et al. (Citation1994) for taking account of uncertainties in both data and parameter values when estimating the time-varying probability of rupture of a fault or fault segment. The reader is referred to Rhoades & Van Dissen (Citation2003) for details of the method, which is outlined briefly below.
The estimation begins with a prior distribution for the value of the adjustable parameters in each recurrence–time distribution. For the exponential model, there is only one parameter: the mean. The other distributions considered each have two parameters: the mean and one other. The prior distribution of the mean is determined from the input distributions of the mean single-event displacement and the average slip rate. For the lognormal model, the second parameter is the coefficient of variation, for which the prior distribution is taken as uniform on the interval (0,1). For the Weibull distribution, the second parameter is a shape parameter c, which is such that if c>1, the hazard increases (rather than decreases) as the elapsed time since the most recent rupture increases. The prior distribution for c is defined by taking 1/c to be distributed uniformly on the interval (0,1). For the inverse Gaussian model, the second parameter is a dispersion parameter called the aperiodicity, for which a generic value of 0.5 was suggested by Ellsworth et al. (Citation1999). Values close to 0 correspond to regular, i.e. near periodic, recurrence. The prior distribution of the aperiodicity is taken as uniform on (0,1).
A large sample of size n is drawn from the input data distributions of the mean single-event displacement, the average slip rate, and the times of past ruptures. We thus have n data samples, where a single sample comprises a set of values, one from each data input distribution. For each such data sample x , a sample of size m is drawn from the conditional distribution of the parameters θ of each recurrence–time distribution α, given x , and the probability density f (t| θ, x ;α) is evaluated. For each data sample, the probability density (t| x ;α) of the time from the last rupture to the next is calculated by mixing (i.e. averaging) the probability over the parameter samples, i.e.
Then for each recurrence–time model, the hazard function at time t since the last rupture is obtained by mixing (i.e., averaging) the hazard functions over the data sample.
Note that for a given cumulative distribution function F(t) and corresponding probability density f(t), the hazard function h(t), which has units of earthquakes per unit time, is given by
The conditional probability of an earthquake occurring in a time interval (t1, t2) of interest for a given model α is given by
An implication of the above method is that the final distribution of the mean recurrence interval is affected by the input data distributions of the times of past ruptures and the recurrence–time model, as well as its prior distribution. It follows that the final distribution can differ from the prior distribution of the mean recurrence interval determined from the input distributions for the single-event displacement and average slip rate, and can also differ between different recurrence–time models. Thus, even in the case of the exponential recurrence–time distribution, for which the hazard is in principle time-invariant, the mean recurrence interval will not coincide exactly with the estimate that would be obtained from a knowledge only of the distributions of the single-event displacement and average slip rate.
In this study we take n=100, and m=200. These values were found to be large enough to give stable results for all models, both for estimation of the hazard rate with the preferred input data distributions and for the sensitivity analysis.
Conditional probability results
Wairarapa Fault
The estimated conditional probabilities of rupture under each of the four recurrence–time distributions are shown for time intervals of 1, 20, 50 and 100 yr in , using the data input values in . The conditional probabilities under the four different recurrence–time models are not similar (; ). The hazard rate under the exponential model over the next 100 yr is always higher than under the lognormal, Weibull and inverse Gaussian models, and it is static; whereas, the hazard rate under the lognormal, Weibull and inverse Gaussian models is always increasing. Also, the hazard rate under the lognormal model is about half that of the Weibull model, over the next 100 yr, and the hazard rate under the inverse Gaussian model is higher than both the lognormal and Weibull models and has an appreciably faster positive rate of change. That is, over the next 100 yr, hazard increases fastest and changes the most under the inverse Gaussian model compared to the other three models.
Figure 5 Variation of hazard of rupture of the southern Wairarapa Fault with time, from AD 2010 to 2110, averaged over sampled data distributions, under the exponential, lognormal, Weibull and inverse Gaussian recurrence–time distributions. Data distributions as in .
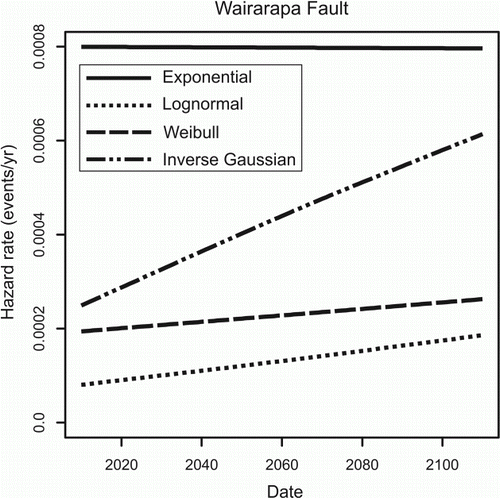
Table 4 Estimated conditional probability of rupture of the southern Wairarapa Fault and the Ōhariu Fault during time intervals starting in AD 2010, allowing for uncertainties in data and parameter values. Input data from . Also listed are the conditional probability of rupture results for the Wellington–Hutt Valley segment of the Wellington Fault from Rhoades et al. (Citation2011), and a combined time-dependent result for each fault derived from the following models with the following weights: inverse Gaussian (0.5 wt), lognormal (0.3 wt) and Weibull (0.2 wt).
Over the next 100 yr, the probability of rupture of the southern Wairarapa Fault is c. 7.7% under the exponential model, and c. 1.3%, c. 2.3% and c. 4.3% under the lognormal, Weibull and inverse Gaussian models, respectively ().
Sensitivity results – southern Wairarapa Fault
In order to show the sensitivity of the southern Wairarapa Fault probability results to particular elements of the data, the conditional probabilities were also calculated for data sets with variations on those in , considering six different combinations of data inputs, as described in . The reasons for these variations have already been discussed above. The variations considered were: (1) exploring the effect of elevated and reduced slip rates; and (2) adjusting the CoV of single event displacements to the high-end value of 0.57 considered by Rhoades et al. (Citation2004).
The results of the sensitivity study are summarised in , which shows the 100 yr conditional probabilities for each of the six input data variations ().
Figure 6 Sensitivity study results: probability of rupture of the southern Wairarapa Fault in the next 100 yr from AD 2010, using data input variations as outlined in . Bold black circles are preferred conditional probability results based on preferred data input and distributions of .
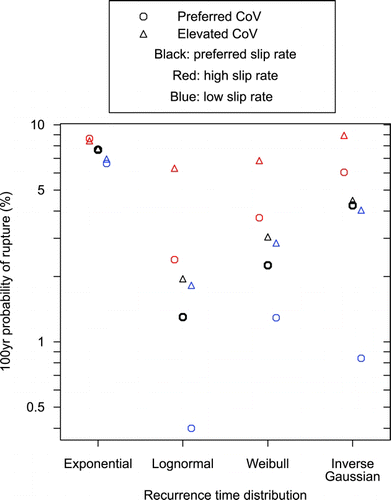
The probabilities in span a wide range, with a factor of more than 20 between the highest and lowest estimates. For all distributions other than exponential, the probabilities are highly sensitive to the data variations. The slip rate has a large impact on the results. As expected, raising (lowering) the slip rate increases (decreases) the probability of rupture for all distributions, by shortening (lengthening) the mean recurrence interval. For the exponential distribution the change in the probability is by c. 10%. For the other distributions it is by a factor ranging from c. 1.5 to c. 5, except that when the CoV of single-event displacement is elevated the probability is reduced by < 10% when the slip rate is lowered. The effect of elevating the CoV is almost negligible for the exponential distribution. For the other distributions the effect is large, up to a factor of five, and is greater when the slip rate is elevated or lowered than with the preferred slip rate. The lognormal probabilities are generally the most sensitive to variation of the CoV. For all distributions the lowest probability is obtained when both the CoV of single-event displacement takes the preferred value and the slip rate is lowered.
Ōhariu Fault
The estimated conditional probabilities of rupture of the Ōhariu Fault under each of the four recurrence–time distributions are shown for time intervals of 1, 20, 50 and 100 yr in , using the data input values in . The conditional probabilities under the four different recurrence–time models are not too dissimilar. Over the next 100 yr, the probability of rupture of the Ōhariu Fault is c. 4.3% under the exponential model, and c. 5.1%, c. 3.9% and c. 5.1% under the lognormal, Weibull and inverse Gaussian models, respectively (). Nevertheless, shows that the hazard rate under the exponential model is almost static for the next 100 yr, but that under the lognormal, Weibull and inverse Gaussian models it is on an increasing trend. Also, compared with the exponential model the conditional probability of rupture under the lognormal and inverse Gaussian models is slightly higher while under the Weibull model it is slightly lower.
Figure 7 Variation of hazard of rupture of the Ōhariu Fault with time, from AD 2010 to 2110, averaged over sampled data distributions (), under the exponential, lognormal, Weibull and inverse Gaussian recurrence–time distributions.
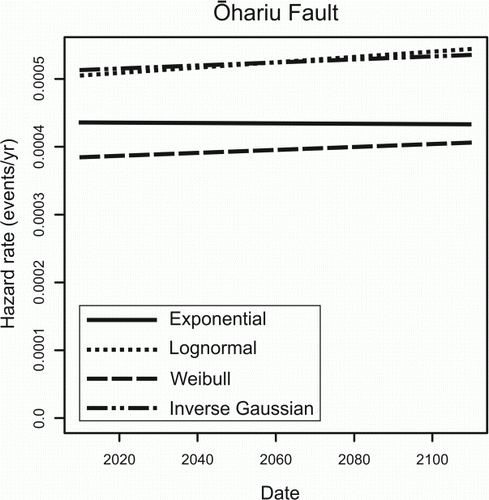
Sensitivity results – Ōhariu Fault
As was done for the southern Wairarapa Fault, we explore the sensitivity of the Ōhariu Fault results by calculating a suite of conditional probabilities based on 32 alternative data input combination, as outlined in , which differ from our preferred data inputs (). The reasons for these variations have already been discussed above. The variations considered were: (1) including Event FB, (2) adjustments to the distribution for the time of occurrence of Event II; (3) exploring the effect of three alternative slip-rate distributions, and (4) adjusting the CoV of single event displacements to the high-end value of 0.57 considered by Rhoades et al. (Citation2004).
The results of the Ōhariu Fault sensitivity study are summarised in , which shows the 100 yr conditional probabilities for each of the 32 input data variations outlined in .
Figure 8 Sensitivity study results: probability of rupture of the Ōhariu Fault in the next 100 yr from AD 2010, using data input variations as outlined in . Bold black circles are preferred conditional probability results based on preferred data input and distributions of .
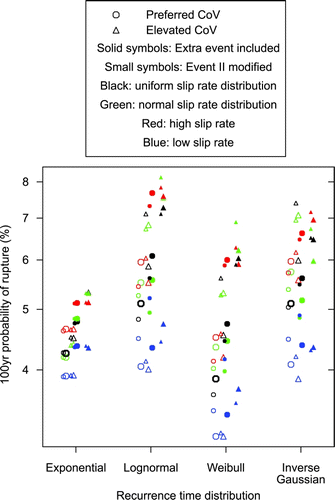
Generally, the results for the Ōhariu Fault are not as sensitive as those for the Wairarapa Fault; there is only a factor of c. 2.5 between the highest and lowest probabilities plotted in . As expected, the exponential model is less sensitive to data variations than the other models. However, it is somewhat sensitive both to changes in the slip rate and inclusion of the extra event. Inclusion of the extra event mostly increases the probability of rupture for all models and for most data combinations. However, modification of the Event II data has only a minor effect in most cases, although in some cases it increases and in other cases decreases the probability of rupture. Changes to the slip-rate distribution have consistent effects across all models. The high slip rate and normally distributed slip-rate cases produce higher probabilities than the preferred slip-rate distribution, and the low slip-rate case produces lower probabilities. For the lognormal, Weibull and inverse Gaussian models these variations in the slip-rate affect the probability by a factor of up to 1.5 compared with the preferred slip-rate distribution.
Discussion
The National Seismic Hazard Model of New Zealand (Stirling et al. Citation2012) has recently been updated. In this update, conditional probability of rupture of the Wairarapa Fault, Ōhariu Fault and Wellington–Hutt Valley segment of the Wellington Fault are being incorporated into the hazard assessment. This article provides documentation and results of the conditional probability of rupture evaluations for the southern Wairarapa Fault and the Ōhariu Fault (). Rhoades et al. (Citation2011) provide conditional probability documentation and results for the Wellington–Hutt Valley segment of the Wellington Fault and, for comparative purposes, we include their results in also.
In the calculations of conditional probability of rupture of the southern Wairarapa Fault, Ōhariu Fault and Wellington–Hutt Valley segment of the Wellington Fault results are presented for four recurrence time models – exponential, lognormal, Weibull and inverse Gaussian (). The latter three are all time–variable models, but the probability estimates they yield for a specific fault are not always similar, in fact, sometimes they are quite different. For example, the lognormal and inverse Gaussian results for the southern Wairarapa Fault differ by more than a factor of three (; ). So, the question arises as to which model's results, or combination of results, should be used in the National Seismic Hazard Model update, and other time-dependent earthquake hazard and loss applications. Fitzenz et al. (Citation2010, Citation2012) proposed a Bayesian framework for deciding the best combination of models. This is not adopted here, for the reason that the number of known past interevent times is small for these faults, and therefore the Bayesian weightings are not likely to be robust. It would be unsatisfactory, with this small amount of data, to adopt different model weightings for each fault in the National Seismic Hazard Model. Therefore at this stage, it is preferred to apply expert judgement to decide how to combine the models.
The inverse Gaussian model appears to be the most favoured at the moment. For example, UCERF 2 (Field et al. Citation2009) gives the inverse Gaussian model the greatest prevalence and weighting for type-A faults. (Type-A faults in UCERF 2 are those that have known slip rates and paleoseismic estimates of recurrence interval.) The southern Wairarapa Fault and Wellington–Hutt Valley segment of the Wellington Fault would be classified as type-A faults, and probably also the Ōhariu Fault. This could potentially be used as an argument favouring the exclusive use of the inverse Gaussian results in for evaluating the time–variable hazard of rupture of the Wairarapa, Wellington and Ōhariu faults. That said, other models, such as lognormal and Weibull are still being used and favoured by some (e.g. Robinson et al. Citation2011; Rundle et al. Citation2006). At present, given the significant unknowns regarding earthquake behaviour, fault interactions and the like, it is not possible to state unequivocally which of the three models is most realistic. This suggests to us that consideration should be given to combining the results of the three time–variable models in some agreed on, and consistent, fashion, instead of relying on the results of a single model. We propose that the conditional probabilities of rupture listed in should be applied in practice using a logic tree, with subjectively assigned weights to each recurrence time distribution as follows: inverse Gaussian (0.5 wt), lognormal (0.3 wt), Weibull (0.2 wt). The ramifications of this proposition for the southern Wairarapa Fault, Ōhariu Fault and Wellington–Hutt Valley segment of the Wellington Fault are listed in the respective ‘combined time-dependent result’ rows of .
presents a comparison of the ‘combined time-dependent result’ for the three faults listed in along with their time-invariant results (i.e. exponential results). shows that, for the Wairarapa Fault, the current time-dependent hazard rate, represented by the combined model, is only about a quarter of the long-term average hazard rate, represented by the exponential model, and although rising, will remain below half the long-term average rate for the next 100 yr. For the Ōhariu Fault, the time-dependent hazard is currently c. 20% above the long-term average rate and is gradually increasing. Although in the long run this fault presents a lower hazard than the other two faults, for at least the next 100 yr it has a higher hazard rate than the Wairarapa Fault. For the Wellington Fault, which has the highest long-term hazard of these three faults, the time-dependent rate is currently similar to the long-term average rate and will continue to rise over the next 100 yr to c. 25% above the long-term average rate.
Figure 9 Comparison of hazard of rupture with time, from AD 2010 to 2110, of the southern Wairarapa Fault, Ōhariu Fault and Wellington–Hutt Valley segment of the Wellington Fault under the exponential recurrence–time distribution, and a combined time-dependent result derived from the following models with the following weights: inverse Gaussian (0.5 wt), lognormal (0.3 wt) and Weibull (0.2 wt) (see ).
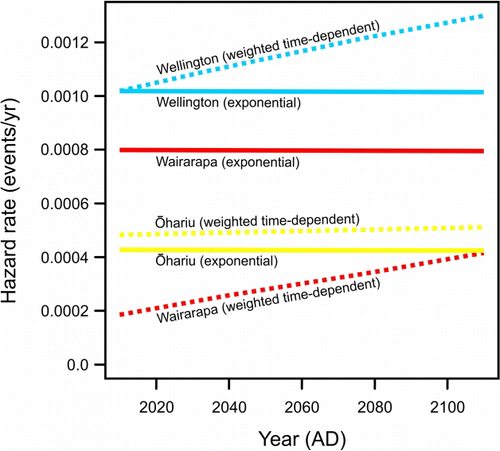
Conclusions
The newly acquired data on the southern Wairarapa and Ōhariu faults has helped to refine estimates of the probability of rupture of these faults over the next 100 yr, and also to show the sensitivity of those estimates to the input data and recurrence–time model adopted. Overall, the results show that the time-dependent probability of rupture of the southern Wairarapa Fault, at c. 3.0% for the next 100 years, is currently well below its long-term average value of c. 7.7% for a 100 yr period. The present estimate is quite sensitive to the values the long-term average slip rate and the CoV of the average single-event displacement. The estimate could therefore be made more robust if stronger observational support for these values could be obtained. The results also show that the time-dependent probability of rupture of the Ōhariu Fault, at c. 4.9% for the next 100 yr, is currently slightly above its long-term average value of 4.3% for a 100 yr period. This estimate is also somewhat sensitive the value of the average slip rate. However, taking the sensitivity analysis into account, both of these faults currently have a lower hazard of rupture than the Wellington–Hutt Valley segment of the Wellington Fault, for which the probability the time-dependent probability of rupture is c. 11% for the next 100 yr, slightly greater than the long-term average.
The weighted conditional probability estimates from these faults are being incorporated into the National Seismic Hazard Model, replacing previous time-invariant estimates. Similar conditional probability estimates from other mapped faults will be added as they become available.
Acknowledgements
This project was funded by the It's Our Fault programme and the Natural Hazards Research Platform. Discussions surrounding this investigation with Kelvin Berryman, Graeme McVerry, Warwick Smith, Mark Stirling and Terry Webb improved its scope and focus. We thank Kate Clark, John Townend and two anonymous journal reviewers for their formal reviews of, and consequent improvements to, this manuscript.
References
- Carne RC , Little TA , Rieser U 2011 . Using displaced river terraces to determine Late Quaternary slip rate for the central Wairarapa Fault at Waiohine River, New Zealand . New Zealand Journal of Geology and Geophysics 54 : 217 – 236 . doi: 10.1080/00288306.2010.532224 .
- Cochran , U , Hannah , M , Harper , M , Van Dissen , R , Berryman , K and Begg , J . 2007 . Detection of large, Holocene earthquakes using diatom analysis of costal sedimentary sequences, Wellington, New Zealand . Quaternary Science Reviews , 26 : 1129 – 1147 . doi: 10.1016/j.quascirev.2007.01.008
- Ellsworth WL , Matthews MV , Nadeau RM , Nishenko SP , Reasenberg PA , Simpson RW 1999 . A physically-based earthquake recurrence model for estimation of long-term earthquake probabilities . US Geological Survey Open-File Report 99-522 . 22 p.
- Fitzenz DD , Ferry MA , Jalobeanu A. 2010 . Long-term slip history discriminates among occurrence models for seismic hazard assessment . Geophysical Research Letters 37 : L20307 . doi: 10.1029/2010GL044071 .
- Fitzenz DD , Jalobeanu A , Ferry MA. 2012 . A Bayesian framework to rank and combine candidate recurrence models for specific faults . Bulletin of the Seismological Society of America 102 : 936 – 947 . doi: 10.1785/0120110087 .
- Field EH , Dawson TE , Felzer KR , Frankel AD , Gupta V , Jordan TH , Parsons T , Petersen MD , Stein RS , Weldon II RJ , Wills CJ 2009 . Uniform California earthquake rupture forecast, version 2 (UCERF 2) . Bulletin of the Seismological Society of America 99 : 2053 – 2107 . doi: 10.1785/0120080049 .
- Formento-Trigilio , ML , Burbank , DW , Nicol , A , Shulmeister , J and Reiser , U . 2003 . River response to an active fold-and-thrust belt in a convergent margin setting, North Island, New Zealand . Geomorphology , 49 : 125 – 152 . doi: 10.1016/S0169-555X(02)00167-8
- Grapes , R and Downes , G . 1997 . The 1855 Wairarapa, New Zealand, earthquake – analysis of historical data . Bulletin of the New Zealand Society for Earthquake Engineering , 30 : 271 – 368 .
- Grapes RH , Wellman HW 1988 . The Wairarapa Fault . Victoria University of Wellington Geology Board of Studies . v. 4 . 54 p.
- Hagiwara , Y . 1974 . Probability of earthquake occurrence as obtained from a Weibull distribution of crustal strain . Tectonophysics , 23 : 313 – 318 . doi: 10.1016/0040-1951(74)90030-4
- Hecker S , Abrahamson N 2002 . Characteristic fault rupture: implications for fault rupture hazard analysis . Paper presented at the Pacific Earthquake Engineering Research Centre (PEER) Lifelines Research Meeting , 24 June 2002 .
- Heron , D , Van Dissen , R and Sawa , M . 1998 . Late Quaternary movement on the Ōhariu Fault, Tongue Point to MacKays Crossing, North Island, New Zealand . New Zealand Journal of Geology and Geophysics , 41 : 419 – 439 . doi: 10.1080/00288306.1998.9514820
- Lensen , GJ and Vella , P . 1971 . The Waiohine River faulted terrace sequence. In: Recent crustal movements . Royal Society of New Zealand Bulletin , 9 : 117 – 119 .
- Litchfield , NJ and Berryman , KR . 2005 . Correlation of fluvial terraces within the Hikurangi Margin, New Zealand: implications for climate and baselevel controls . Geomorphology , 68 : 291 – 313 . doi: 10.1016/j.geomorph.2004.12.001
- Litchfield N , Van Dissen R , Hemphill-Haley M , Townsend D , Heron D 2010 . Post c. 300 year rupture of the Ōhariu Fault in Ōhariu Valley, New Zealand . New Zealand Journal of Geology and Geophysics 53 : 43 – 56 . doi: 10.1080/00288301003631780 .
- Litchfield , N , Van Dissen , R , Heron , D and Rhoades , D . 2006 . Constraints on the timing of the three most recent surface rupture events and recurrence interval for the Ōhariu Fault: trenching results from MacKays Crossing, Wellington, New Zealand . New Zealand Journal of Geology and Geophysics , 49 : 57 – 61 . doi: 10.1080/00288306.2006.9515147
- Litchfield , N , Van Dissen , R , Langridge , R , Heron , D and Prentice , C . 2004 . Timing of the most recent surface rupture event on the Ōhariu Fault near Paraparaumu, New Zealand . New Zealand Journal of Geology and Geophysics , 47 : 123 – 127 . doi: 10.1080/00288306.2004.9515041
- Little TA , Van Dissen R , Schermer E , Carne R 2009 . Late Holocene surface ruptures on the southern Wairarapa Fault, New Zealand: link between earthquakes and the raising of beach ridges on a rocky coast . Lithosphere 1 : 4 – 28 . doi: 10.1130/L7.1 .
- McSaveney , MJ , Graham , IJ , Begg , JG , Beu , AG , Hull , AG , Kim , K and Zondervan , A . 2006 . Late Holocene uplift of beach ridges at Turakirae Head, south Wellington coast, New Zealand . New Zealand Journal of Geology and Geophysics , 49 : 337 – 358 . doi: 10.1080/00288306.2006.9515172
- Matthews , MV , Ellsworth , WL and Reasenberg , PA . 2002 . A Brownian model for recurrent earthquakes . Bulletin of the Seismological Society of America , 92 : 2233 – 2250 . doi: 10.1785/0120010267
- National Institute of Advanced Industrial Science and Technology 2007 . Active fault database of Japan, 13 December 2008 version . Research Information Database DB095, National Institute of Advanced Industrial Science and Technology . http://riodb02.ibase.aist.go.jp/activefault/index_e.html
- Nishenko , SP and Buland , R . 1987 . A generic recurrence interval distribution for earthquake forecasting . Bulletin of the Seismological Society of America , 77 : 1382 – 1399 .
- Palmer , AS . 1984 . Quaternary geology of the Wairarapa. Guide for field trips . Geological Society of New Zealand Miscellaneous Publication , 318 : 20 – 52 .
- Rhoades DA , Stirling MW , Schweig E , Van Dissen RJ 2004 . Time-varying earthquake hazard in the Wellington region . Institute of Geological & Nuclear Sciences Client Report 2004/141 ( prepared for the Earthquake Commission ).
- Rhoades , DA and Van Dissen , RJ . 2003 . Estimates of the time-varying hazard of rupture of the Alpine Fault, New Zealand, allowing for uncertainties . New Zealand Journal of Geology and Geophysics , 46 : 479 – 488 . doi: 10.1080/00288306.2003.9515023
- Rhoades , DA , Van Dissen , RJ and Dowrick , DJ . 1994 . On the handling of uncertainties in estimating the hazard of rupture on a fault segment. Journal of Geophysical Research . Solid Earth , 99 ( B7 ) : 13701 – 13712 .
- Rhoades , DA , Van Dissen , RJ , Langridge , RM , Little , TA , Ninis , D , Smith , EGC and Robinson , R . 2011 . Re-evaluation of conditional probability of rupture of the Wellington–Hutt Valley segment of the Wellington Fault . Bulletin of the New Zealand Society for Earthquake Engineering , 44 : 77 – 86 .
- Robinson R , Van Dissen R , Litchfield N 2011 . Using synthetic seismicity to evaluate seismic hazard in the Wellington region, New Zealand . Geophysical Journal International 187 : 510 – 528 . doi: 10.1111/j.1365-246X.2011.05161.x .
- Rodgers DW , Little TA 2006 . World's largest co-seismic strike-slip offset: the 1855 rupture of the Wairarapa Fault, New Zealand, and implications for displacement/length scaling of continental earthquakes . Journal of Geophysical Research 111 : B12408 . doi: 10.1029/2005JB004065 .
- Rundle PB , Rundle JB , Tiampo KF , Donnellan A , Turcotte DL 2006 . Virtual California: fault model, frictional parameters, application . Pure and Applied Geophysics 163 : 1819 – 1846 . doi: 10.1007/s00024-006-0099-x .
- Smith WD , Harmsen SC 2010 . Displaying seismic deaggregation: the importance of the various sources . Seismological Research Letters 81 : 488 – 497 . doi: 10.1785/gssrl.81.3.488 .
- Stirling M , McVerry G , Gerstenberger M , Litchfield N , Van Dissen R , Berryman K , Barnes P , Wallace L , Bradley B , Villamor P , Langridge R , Lamarche G , Nodder S , Reyners M , Rhoades D , Smith W , Nicol A , Pettinga J , Clark K , Jacobs K 2012 . National seismic hazard model for New Zealand: 2010 update . Bulletin of the Seismological Society of America 102 : 1514 – 1542 . doi: 10.1785/0120110170 .
- Van Dissen R , Barnes P , Beavan J , Cousins J , Dellow G , Francois-Holden C , Fry B , Langridge R , Litchfield N , Little T , McVerry G , Ninis D , Rhoades D , Robinson R , Saunders W , Villamor P , Wilson K , Barker P , Berryman K , Benites R , Brackley H , Bradley B , Carne R , Cochran U , Hemphill-Haley M , King A , Lamarche G , Palmer N , Perrin N , Pondard N , Rattenbury M , Read S , Semmens S , Smith E , Stephenson W , Wallace L , Webb T , Zhao J 2010 . It's our fault: better defining earthquake risk in Wellington . Proceedings of the 11th IAEG Congress , Auckland , New Zealand 5–10 September 2010 . Pp. 737 – 746 .
- Van Dissen , RJ and Berryman , KR . 1996 . Surface rupture earthquakes over the last c. 1000 years in the Wellington region, New Zealand, and implications for ground shaking hazard . Journal of Geophysical Research , 101 : 5999 – 6019 . doi: 10.1029/95JB02391
- Van Dissen R , Berryman K , King A , Webb T , Brackley H , Barnes P , Beavan J , Benites R , Barker P , Carne R , Cochran U , Dellow G , Fry B , Hemphill-Haley M , Francois-Holden C , Lamarche G , Langridge R , Litchfield N , Little T , McVerry G , Ninis D , Palmer N , Perrin N , Pondard N , Semmens S , Stephenson W , Robinson R , Villamor P , Wallace L , Wilson K 2009 . It's our fault: better defining the earthquake risk in Wellington – Results to date and a look to the future . Proceedings of the New Zealand Society for Earthquake Engineering Technical Conference , Christchurch , New Zealand 3–5 April 2009 . Paper No. 48 , 8 p.
- Vella , P . 1963 . Upper Pleistocene succession in the inland part of Wairarapa Valley, New Zealand . Transactions of the Royal Society of New Zealand , 2 ( 4 ) : 63 – 78 .
- Wang , N and Grapes , R . 2008 . Infrared-stimulated luminescence dating of late Quaternary aggradation surfaces and their deformation along an active fault, southern North Island of New Zealand . Geomorphology , 96 : 86 – 104 . doi: 10.1016/j.geomorph.2007.07.016
- Wellman , HW . 1955 . New Zealand Quaternary tectonic . Geologische Rundschau , 43 : 248 – 257 . doi: 10.1007/BF01764108