ABSTRACT
This study explored the sequential interplay between challenges and regulatory processes in high- and low-performing collaborative groups. 66 students from a Finnish higher education institution participated in a collaborative task in groups of three. Approximately 34 h of video data were coded. The sequential analysis revealed that both groups had higher sequential transitions between cognitive regulation and emotional/motivational regulation, rather than cognitive challenges. The high-performing groups demonstrated a stronger sequential link between emotional/motivational regulation and cognitive regulation than the low-performing groups did when faced with cognitive challenges. The study establishes a theoretical grounding and advances our understanding of regulated learning. Since collaborative learning tasks are highly adopted in the higher education context, especially in the Nordic region, this study has practical implications for higher education in these countries and beyond as they seek to develop pedagogical methodologies and customised support to help collaborative groups resolve challenges by initiating regulatory processes.
Introduction
The rapid growth in globalisation has turned us towards collaboration to resolve complex challenges (Järvelä et al., Citation2020). Collaborative learning settings are considered important to inculcate the vital skills needed to succeed in the twenty-first century. Therefore, various education systems have been adopting pedagogical methodologies, such as constructive teaching strategies that include problem- and practice-oriented learning and enquiry-based learning, to equip students with collaborative learning skills (Cukurova et al., Citation2018).
In collaborative learning, learners face various types of challenges (Hadwin et al., Citation2018). These challenges can include, for example, cognitive challenges, such as understanding the task goals and content and selecting appropriate strategies to progress with the task (Koivuniemi et al., Citation2018; Näykki et al., Citation2014). Emotional and motivational challenges, such as anxiety, frustration, and a lack of self-efficacy, are also observed in collaborative learning settings (Järvenoja et al., Citation2019; Järvenoja et al., 2013). To succeed in collaborative learning, research has shown that learners should advance deliberate initiatives and efforts to plan, monitor, control, and evaluate individual as well as group members’ collective cognitive, motivational, emotional, and behavioural aspects, as this would help in tackling the challenges they face during collaboration. The literature calls this process the regulation of learning (Hadwin et al., Citation2010; Järvelä et al., Citation2008; Volet & Mansfield, Citation2006). In collaborative learning, research has placed great weight on understanding and unfolding the regulation of learning, as it ensures joint knowledge construction and prepares learners to take on challenges to attain their learning goals (Malmberg et al., Citation2017).
As in many other settings, in collaborative learning, learning happens in sequence. The sequentiality of learning could be more precisely explained as a series of events/actions in collaborative learning (i.e., how and when learning processes occur, which event/action leads to another, and what comes after; Bannert et al., Citation2013). Unfolding the sequences of learning advances the theoretical framework of regulated learning, as it reveals the interplay between the challenges groups face and the existing regulatory processes (i.e., planning, monitoring, and controlling); the process of unfolding also explains which regulatory process is more efficient and has stronger sequential relations with challenges (Malmberg et al., Citation2017).
Most empirical studies on collaborative learning have primarily investigated regulatory processes in terms of sequentiality. In this regard, Chang et al.’s (Citation2017) exploration of regulatory processes found unsuccessful collaborative groups to have a sequential association of monitoring and reflecting with planning and executing. In contrast, successful groups developed a sequential relationship of monitoring and reflecting with understanding, exploring, formulating, and representation. Malmberg et al. (Citation2017) also explored the sequential relationship between regulatory and executive processes. In terms of sequences, the researchers found a substantial relationship between the regulatory processes of planning and monitoring. Molenaar and Chiu (Citation2014) also aimed to explore the sequentiality of regulatory processes, and according to their findings, planning was correlated with low cognition, which was then correlated with high cognition. By applying process mining, Schoor and Bannert’s (Citation2012) study also examined regulatory processes, but the process models of the two profiles of groups (low- and high-achieving dyads) in their study revealed no differences regarding sequential relations.
Although the aforementioned studies have advanced theoretical and methodological understandings regarding the sequential nature of regulatory processes, there is a scarcity of research examining the sequential nature of regulatory processes in relation to the challenges that groups face during collaborative work. Therefore, we argue that examining regulatory processes in a sequential relationship with challenges by categorising collaborative groups (i.e., low and high performance) is vital to advance the theoretical and methodological grounding of regulated learning and to outline the educational implications for designing pedagogical approaches, improving learning content, and providing customised support to collaborative groups with different performance profiles in Nordic higher education. Although some studies have investigated the sequential relationships between challenges and regulatory processes, these studies are limited in two ways. First, they have focused on a single profile of collaborative groups, and second, they only investigate the sequentiality of challenges in relation to emotion regulation (Järvenoja et al., Citation2019). To advance the research on this topic, our second argument is that the regulatory processes of cognition (i.e., planning, monitoring, and controlling) and emotion/motivation has not been adequately investigated. We thus find it important to investigate the sequential relationship between cognitive and emotional/motivational challenges. This will be achieved by categorising collaborative groups into high- and low-performing ones using state-of-the-art process mining methodologies.
Our last argument relates to the Nordic educational perspective in terms of the educational implications for higher education. Many studies in the higher education context that are situated in the Nordic region have found that collaborative learning is challenging; learners face different challenges, such as those of a cognitive (Koivuniemi et al., Citation2018), socio-cognitive (Näykki et al., Citation2021b), emotional (Järvenoja et al., Citation2019), socio-emotional (Näykki et al., Citation2014), and motivational nature (Järvenoja et al., Citation2017). In the context of teacher education in the Nordic region, many teacher education students are still prepared in a so-called traditional teacher-led schooling culture, which often makes them inactive in knowledge construction and unable to meet the true demands of collaborative learning, as well as unprepared to implement educational technology as a means of support for collaborative learning (Häkkinen et al., Citation2017; Näykki et al., Citation2021a). Hence, as the present study is situated in the Nordic region, it has some educational implications for designing collaborative learning in higher education in the Nordic region.
Theoretical framework
Socially shared regulation of learning (SSRL)
Challenges in collaborative learning urge learners to initiate regulation (Hadwin et al., Citation2011). Such regulation and its corresponding challenges can be described within the theoretical framework of the socially shared regulation of learning (SSRL; Järvelä, Järvenoja, et al., Citation2016). In terms of regulation, SSRL posits that learners jointly share their beliefs, prior knowledge, and understanding of a task and its content to negotiate and plan strategies to initiate the regulation of individual and joint cognitive, motivational, emotional, and behavioural aspects ( Järvelä & Hadwin, Citation2013; Järvelä, Kirschner, et al., Citation2016).
SSRL occurs at individual as well as group levels, enabling learners to attain their joint goals (Hadwin et al., Citation2011). Individual and group regulatory processes are interdependent. Each learner in a group contribute to their own understanding of the task, beliefs, and knowledge to negotiate strategies for regulating not only their own cognitive, emotional, motivational, and behavioural aspects, but also those of the group member as a whole (Hadwin et al., Citation2011; Järvelä et al., Citation2019). The theoretical grounding of SSRL posits that individual regulatory processes become shared at the group level, with learners working together to establish task objectives, monitor their progress, and optimise their performance (Järvenoja et al., Citation2020; Zhang et al., Citation2021).
To succeed in a collaborative task, learners must focus on SSRL and regulate different domains of learning, such as cognition (i.e., for applying strategies and developing task perception), motivation, and emotion (i.e., for developing a sense of willingness, inclusion, and interest in working together to meet the requirements of the task and sustain socio-emotional balance and social coherence; Malmberg et al., Citation2015). SSRL’s theoretical grounding explains how learners operate and sustain the regulatory processes of planning, monitoring, and controlling for not only their shared learning, but also for joint understanding and socio-emotional cohesiveness (Järvelä & Hadwin, Citation2013; Panadero & Järvelä, Citation2015). For example, initiating the regulatory processes of monitoring could help not only to meet the standards of the task, but also to check group progress, ensure understanding, and apply further strategies to proceed with the task (Kempler-Rogat & Linnenbrink-Garcia, Citation2011).
Sequential nature of regulation in collaborative learning
In collaborative learning, learners create different types of events, such as those that are cognitive, motivational, and emotional. These events can be categorised into different types of challenges and regulations. These events take place in a series of connections and sequentially influence each other, forming different paths of regulatory processes and challenges that are sequentially connected. According to Järvelä, Järvenoja, et al. (Citation2016), research puts weight on the significance of unfolding these paths to open the “black box” of learning processes and to gain insights from the sequential patterns of regulatory processes and challenges in collaborative learning. Kapur (Citation2011) explains how unfolding sequential patterns of interactions (i.e., challenges and regulatory processes) in collaborative learning might answer certain challenging questions in educational research, such as how learners plan and monitor their learning activities, how they apply strategies to control their learning and attend to joint learning objectives, how collaborative groups react (or not) to any particular type of challenge, and which challenges or regulatory actions are more frequently prominent in terms of sequences (Bakhtiar et al., Citation2013; Knight et al., Citation2017).
Although unpacking regulatory processes has been challenging, investigating them in terms of sequences could be helpful in increasing learning outcomes (Kapur, Citation2011). Keeping this in consideration, a few studies have empirically examined the sequential nature of regulatory processes in collaborative learning (Chang et al., Citation2017; Järvenoja et al., Citation2019; Malmberg et al., Citation2017; Molenaar & Chiu, Citation2014; Paans et al., Citation2019a; Schoor & Bannert, Citation2012; Zhang et al., Citation2021). Specifically, Chang et al. (Citation2017) examined collaborative problem solving in terms of a sequential relationship by capturing the learning constructs of “exploring & understanding”, “representation & formulating”, “planning & executing”, and “monitoring & reflecting” in two profiles of collaborative groups (i.e., successful and unsuccessful groups). The authors applied lag-sequential analysis and found that unsuccessful groups had sequential patterns between “planning & executing” and “monitoring & reflecting”. At the same time, successful groups developed a sequential relationship of “monitoring & reflecting” with “exploring & understanding” and “representation & formulating”. In terms of frequency, the study revealed more occurrences of “monitoring & reflecting” in the learning activities of successful groups than unsuccessful ones. In contrast, unsuccessful groups superseded successful groups in terms of the frequency of “monitoring & reflecting”.
In another study, Malmberg et al. (Citation2017) investigated the sequential relationship between executive processes and regulation in collaborative learning. They used video data to record the research constructs of planning, monitoring, and task execution. With the help of lag-sequential analysis, they discovered that socially shared monitoring facilitated knowledge construction in learners. Their study also found a strong association between planning and monitoring. Contrary, in Järvenoja et al.’s (Citation2019) study, the authors investigated how cognitive, emotional, and motivational challenges were related to emotion regulation strategies, including task structuring, increasing awareness, encouragement, and social reinforcement. Through their sequential analysis, the authors found that cognitive challenges were often occurred independently of emotional and motivational challenges. This study also revealed that emotional regulation strategies tended to be more closely connected to each other than to cognitive challenges. Finally, the researchers found that learners encountered more cognitive challenges when resolving emotional and motivational challenges.
Zhang et al. (Citation2021) applied epistemic network analysis to investigate regulated learning between high- and low-performing groups. The researchers found that both groups engaged in similar sequential patterns dealing with planning, monitoring, and evaluating. However, the high-performing groups benefited more by initiating patterns between positive and negative emotions as compared to the low-performing groups. The analysis also revealed that the high-performing groups established stronger connections between monitoring and socio-emotional regulation compared to the low-performing groups.
Although each of these studies investigated regulatory processes in sequentiality, the sequential relationship between challenges and regulatory processes was not the focus of any of them. Research emphasises the importance of investigating regulations in sequential relationships with challenges, as it might provide insightful perspectives on learners’ interactions when they face challenges (Bakhtiar et al., Citation2013; Järvelä, Järvenoja, et al., Citation2016; Kapur, Citation2011). Further, the sequential relationship between cognitive regulation and challenges has remained beyond the scope of most studies (Chang et al., Citation2017; Malmberg et al., Citation2017; Molenaar & Chiu, Citation2014; Paans et al., Citation2019b; Schoor & Bannert, Citation2012; Su et al., Citation2018; Zhang et al., Citation2021). Moreover, there is scarce literature on regulated learning and the empirical grounding of the sequential pathways of the regulatory processes of emotion/motivation in relation to cognition, especially with respect to how cognitive and emotional/motivational challenges could be investigated in categorised (i.e., high- and low-performing) collaborative groups in higher education.
Considering this research gap, for the present study, the participants were categorised into high- and low-performing groups, as the literature suggests that learners’ responses and actions vary according to learning tasks and learning contexts. Furthermore, pedagogical approaches also differently influence the regulatory behaviour of learners (Hadwin et al., Citation2018; Järvelä & Hadwin, Citation2013; Zhang et al., Citation2021). In addition, the collaborative groups were categorised to make this research parallel to ongoing studies that have been central to the sequential analysis of regulated learning in collaborative learning settings (Chang et al., Citation2017; Paans et al., Citation2019a; Su et al., Citation2018; Zhang et al., Citation2021). In terms of group categorisation, the present study was designed by taking into account the practical implications of two extreme collaborative groups. For instance, the findings of the regulatory actions of two categorised groups (i.e., high and low performers) when confronting challenges could help in the design of tailored learning content, pedagogical approaches, and learning environments to maximise student learning outcomes. Hence, groups were categorised to advance the theoretical groundings of regulated learning processes and to understand their key features (Zhang et al., Citation2021).
We argue that there is a gap in the research on the sequential relationship between cognitive regulation (such as planning, monitoring, and controlling) and emotional/motivational regulation in response to cognitive and emotional/motivational challenges in collaborative learning at the higher education level. Furthermore, the literature on regulated learning in higher education collaborative settings lacks empirical evidence on how high- and low-performing groups respond to and develop sequential pathways of regulatory processes when faced with diverse challenges. The connection between the regulatory processes of emotion/motivation and cognition in sequential responses to different challenges remains empirically understudied.
Hence, the present study has attempted to bridge this research gap. First, it focuses on the vital phenomena of cognitive and emotional/motivational challenges and regulatory processes in face-to-face collaborative learning in higher education. Second, a sample of collaborative groups was categorised into high- and low-performing ones. Although some studies have examined the sequentiality between challenges and regulatory processes (Järvenoja et al., Citation2019), they have not shed any insight into how challenges and regulation interplay in high- and low-performing groups. Lastly, in collaborative learning, mostly group interactions are captured from video data by applying time segmentation of 20 or 30 s, or even five minutes (Isohätälä et al., Citation2020; Järvenoja et al., Citation2019; Mänty et al., Citation2020; Näykki et al., Citation2014; Sinha et al., Citation2015; Sullivan & Wilson, Citation2015). However, in the present study, a fine-grained approach was applied to systematically process and capture episodes/events and code them into categories at the micro level. Through this approach, even a one-second event/episode in which a combination of words was meaningful and relevant to the coding category, such as “Oh my God!”, was coded.
To address this research gap, the present research aims to investigate how cognitive and emotional/motivational challenges and regulation develop into sequential pathways in high-performing groups (HPGs) and low-performing groups (LPGs). Specifically, the study addresses the following research questions:
RQ1: How do high- and low-performing groups differ in terms of the frequency and duration of different challenges and regulations observed during face-to-face collaborative learning?
RQ2: How do sequential pathways of cognitive and emotional/motivational challenges and cognitive and emotional/motivational regulation differ in high- and low-performing groups?
Methodology
Participants and the task
The participants in the study (mean age = 27.8; SD age = 5.43; female = 41, male = 33) were university students enrolled in different higher education international degree programmes, including those at the bachelor’s (n = 5), master’s (n = 52), and PhD levels (n = 16), offered by the University of Oulu, Finland. The participants were from 35 different countries. Out of 73 participants, 66 completed the collaborative task in groups of three. The remaining participants completed the task in groups of two, either due to a participant failing to engage in the experiment or leaving the collaborative task uncompleted for personal reasons. To avoid the confounding effects of differing team sizes, only groups with three members were included in the data analysis.
A simulation tailorshop task was given to each group to work on in collaboration. The task simulated a critical problem-solving scenario of running a garment company and raising its value as much as possible by the end of 12 months (Danner et al., Citation2011), specifically by manipulating complex connections between 24 variables. The simulated company’s value was directly and indirectly affected by the 24 variables, such as raw material cost, shirt price, shop rent, store locations, and employee wages. The overall value of the business was determined by the amount of money in its bank account, the value of its outlet sales, the value of its raw materials and garment products, and the value of its machinery sales. Based on the participants’ manipulation of the variables, the simulation updated the value of the business after each month.
The simulation was designed to take place in two phases. The first phase involved exploration, during which the participants ran the tailorshop company for six simulated months. This helped them become acquainted with the simulation and the relationship between the company’s value and the provided variables. The second performance phase restarted the simulation from the beginning and ran for 12 months. In this phase, changes in company values were tracked according to changes in variables. The present study captured the group interactions (utterances) of both phases. On average, HPGs took 101.6 min to complete the task, compared to 87.3 min for LPGs.
Data collection
The researchers distributed fliers on the university campus and posted announcements on social media sites to recruit participants. A free lunch ticket was given to each participant in exchange for their participation. As the participants were students in different degree programmes, the dates of their availability to participate varied, which made it impossible for researchers to randomly assign participants to collaborative groups. Therefore, based on their availability on the same dates, participants were assigned to collaborative groups.
The LeaF Research Infrastructure (https://www.oulu.fi/leaf-eng/) at the University of Oulu is a state-of-the-art facility designed for research in collaborative learning. For the present study, it was used to collect video data from three groups simultaneously, with three soundproof rooms partitioned for this purpose. Upon arrival at the facility, participants were given a consent form to fill out and sign before being introduced to their group members and directed to the data collection room. In the room, they were seated in front of a touchscreen desktop computer and given instructions on the group task objectives by a researcher reading from a pre-written script. There was no time limit for completing the task, and on average, it took the collaborative groups 96 min (SD = 28.08). All of the group’s interactions during the task were recorded on video, and the computer screen was also recorded to observe how the groups interacted with the simulation interface.
Data analysis
Categorisation of high- and low-performing groups
The analysis of the trend score of the company’s value in each month showed the performance of the groups (Danner et al., Citation2011). Specifically, a rise in the company’s value showed that the group was successful. Therefore, the trend score was computed by adding the number of months in which the group raised the value of the company. Since the simulation task consisted of 12 months, the range of the trend scores was between 0 and 12. To categorise HPGs and LPGs, the trend scores of the groups were analysed with a K-mean cluster analysis. High performance was indicated by trend scores that exceeded five, and those below five were associated with low performance (see Appendix 1).
Video qualitative analysis
The video data for all 22 groups consisted of approximately 34 h. By taking account of the theoretical framework of SSRL, the coding scheme (see Appendix 2) was formed by adopting codes from prior studies (Järvenoja et al., Citation2019; Kempler-Rogat & Linnenbrink-Garcia, Citation2011; Ucan & Webb, Citation2015).
The data were analysed by capturing the research variables (see Appendix 2) from the utterances of the participants (Heirweg et al., Citation2020). Each captured episode/event was considered a group-level event (Järvenoja et al., Citation2019; Mänty et al., Citation2020). Instead of relying on a time-segmented window of 20 or 30 s (Isohätälä et al., Citation2020) or five minutes (Sinha et al., Citation2015; Sullivan & Wilson, Citation2015), we adopted a fine-grained approach to capture meaningful utterances/sentences that were related to the research variables of the study (Heirweg et al., Citation2020). This allowed us to capture episodes/events as short as 1 s.
To ensure the reliability of the data analysis, a coding scheme and criteria were discussed, explained with examples, and agreed upon with SSRL expert researchers. The primary investigator of the study coded all the video data through the lens of the coding scheme. One of the co-authors coded the video data of three random groups to ensure the consistency of the coding. The author has a broad understanding of the theoretical frameworks of challenges and regulated learning in collaborative learning. The kappa value of the three random groups’ data (13.6% of the whole dataset) was checked to ensure inter-rater reliability between the two researchers. Acceptable Cohen’s kappa (K) values (see Appendix 3) were achieved for all coding variables (Fleiss, Citation1981). The coding category of evaluation was omitted from the inter-rater reliability, as it occurred once in each category of the groups.
Comparison of HPGs and LPGs in terms of the frequency and duration of challenges and regulation
The absolute frequencies of each coding category from both groups were calculated into minimum, maximum, standard deviation, mean, and time-weighted scores to compare the categories between the groups. In addition, the HPGs’ and LPGs’ time durations (in seconds) for each coding category were also calculated. We also calculated two types of time-weighted scores: (a) time-weighted frequency and (b) time-weighted duration. Time-weighted frequency was computed by dividing the absolute frequency of each coding category by the group task time duration (in seconds) separately for each HPG and LPG. Time-weighted duration, in contrast, was calculated by dividing the total duration spent on each coding category by the group task time, which was done separately for each group in both categories. The evaluation coding category was excluded because it had only one event in both groups.
Several Mann–Whitney U tests were conducted on frequencies, durations (in seconds), time-weighted durations, and time-weighted frequencies to investigate whether there were statistically significant differences between HPGs and LPGs regarding the frequencies and durations of each coding category. As the size of the groups was small, instead of independent samples t-tests, we applied Mann–Whitney U tests.
Process mining analysis
The captured events/episodes from the video data were formed into event log data that were run through the “BupaR” package in R software to visualise the sequentiality of all coding categories (Janssenswillen et al., Citation2019). The package plots process models that reveal insights into the sequences between events. The process models of the high- and low-performing groups, plotted through the absolute frequencies of the groups, are shown in and , respectively. The process models demonstrate two features: (a) the boxes show the absolute frequency of coding categories, and (b) the bidirectional pathways (arrows) show sequences between events (i.e., coding categories in the boxes). The “play” and “stop” signs indicate the start and end of the process, respectively. The unidirectional arrow in the process models, which originates from the box and follows the same box (i.e., coding category), is considered a recurrent pattern (i.e., a sequential loop within). This demonstrates that the category followed itself in connection or succession.
Figure 1. Process model of HPGs showing pathways/sequential relationships among categories (boxes) and bidirectional paths (arrows). The paths (arrows) refer to the sequence in which events occurred, and their thickness indicates the stronger relationship between the events (boxes). The dashed paths highlight the interactions between different pathways. The number on the paths refers to the absolute frequency of the research variable.
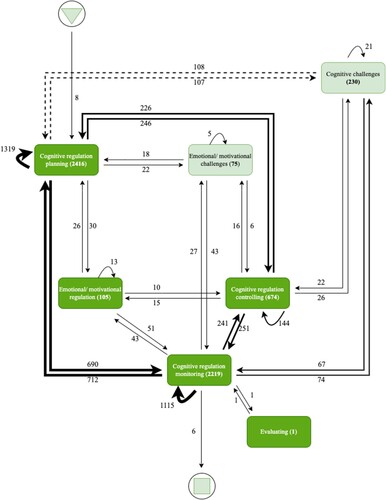
Figure 2. Process model of LPGs showing pathways/sequential relationships among coding categories (boxes) and bidirectional paths (arrows). The paths (arrows) refer to the sequence in which events occurred, and their thickness indicates the stronger relationship between the events (boxes). The dashed paths highlight the interactions between different pathways. The number on the paths refers to the absolute frequency of the research variable.
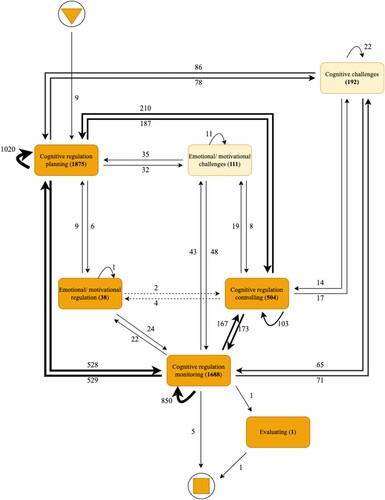
To follow the current trends of using process mining in recent studies (Heirweg et al., Citation2020; Paans et al., Citation2019a; Sonnenberg & Bannert, Citation2015), we retained only significant events and paths, which were quite significant in terms of frequency, that frequently occurred and were closely connected and correlated in the process models of HPGs and LPGs. Retaining the percentage of absolute frequency of events and paths has not been fixed by any criteria so far in educational research (Heirweg et al., Citation2020). Nevertheless, the general guidelines by Fluxicon (Citation2019) were followed to avoid complexity and showed only significantly important correlations in terms of the absolute frequencies of events and paths in the process models of HPGs and LPGs.
Results
Frequency- and duration-wise differences between high- and low-performing groups (RQ1)
and show the statistical results for frequency and duration, respectively. In terms of absolute frequency, with total events of 5,720 in HPGs and 4,409 in LPGS, cognitive regulation (i.e., planning, monitoring, and controlling) was the most frequent activity in both groups. This means that difficulties with cognitive regulation were more frequently higher in numbers compared to challenges in both categorised groups.
Table 1. Frequency statistics of coding categories in high and low performing groups.
Table 2. Duration (seconds) statistics of coding categories in high and low performing groups.
Frequency and duration of cognitive regulation
Regarding the sub-categories of cognitive regulation, the absolute frequency of planning was the most observed, with 2,416 events in the HPGs and 1,875 in the LPGs. In terms of duration, planning was also the most time-consuming regulatory process initiated by both groups. Regarding duration and frequency, monitoring had the second most observable events, with absolute frequencies of 2,219 and 1,688 in HPGs and LPGs, respectively. Both groups had proportionally quite similar events for controlling learning in terms of absolute frequencies and durations.
Frequency and duration of cognitive and emotional/motivational challenges
Cognitive challenges (230 events in HPGs and 192 in LPGs) were observed very rarely in terms of their absolute frequencies and durations. Duration-wise, the time spent by both groups confronting cognitive challenges was in proportion to its absolute frequency value. LPGs encountered higher frequencies of emotional/motivational challenges (111) as compared to HPGs (75). In proportion to absolute frequency, HPGs spent 86 s less on the challenges, whereas LPGs spent 127 s.
Frequency and duration of emotional/motivational regulation
Unlike cognitive regulation, emotional/motivational regulation had a significantly low absolute frequency value. HPGs had 105 events, whereas LPGs had 38 events. shows the duration spent by both groups on emotional/motivational regulation, which is in proportion to the absolute frequency value.
Mann–Whitney U test, time-weighted frequency, and time-weighted duration
Along with the Mann–Whitney U test, the time-weighted frequency and time-weighted duration of all coding categories (excluding evaluation) were calculated to analyse the values of the frequencies and durations and to check if there were differences between the groups regarding the coding categories. and do not present any statistically significant differences between the groups.
Table 3. Mann-Whitney U values of absolute frequency and duration (seconds) of coding categories in high and low performing groups.
Table 4. Mann-Whitney U values of time-weighed frequency and time-weighed duration of coding categories in high and low performing groups.
This study shares similarities with prior studies in learning regulation, which also failed to find statistically significant differences between different categories of groups, such as successful vs. unsuccessful or high vs. low performers. For instance, Schoor and Bannert (Citation2012) found no significant statistical difference in the frequencies of coding categories such as planning, monitoring, coordination, and regulation of motivation between successful and less successful groups. Similarly, Paans et al. (Citation2019a) found no statistical differences in the frequencies of coding categories, such as reading, monitoring, evaluation, and supporting. In addition, Bannert et al. (Citation2013) found no statistical differences in the frequencies of coding categories, such as orientation, searching, planning, monitoring, and evaluating. Malmberg et al. (Citation2017) computed Mann–Whitney U tests to compare the duration spent on planning, monitoring, and task execution by three collaborative groups, but to a large extent, the researchers did not find statistically significant results.
Nevertheless, Bannert et al. (Citation2013) found that successful groups had higher frequencies of planning, monitoring, and motivation compared to unsuccessful groups, which aligns with the present study’s findings that HPGs had higher frequencies of planning, monitoring, and emotional/motivational regulation compared to LPGs. Similarly, Paans et al. (Citation2019a) found that low-challenge dyads, who faced fewer challenges, like the HPGs in this study, had more cognitive aspects and fewer obstacles than high-challenge dyads, who faced more challenges, like the LPGs.
Differences in terms of sequential pathways of cognitive and emotional/motivational challenges and cognitive and emotional/motivational regulation between high- and low-performing groups (RQ2)
The process models shown in and of the high- and low-performing groups respectively show a similar starting pathway, which is a cognitive regulatory process. This finding is parallel with previous studies indicating that group-level metacognitive monitoring help learners initiate the adaptive regulation of collaborative learning (Nguyen et al., Citation2023; Sobocinski et al., Citation2021). However, both groups had different sequential pathways between challenges and regulation.
The sequential pathways initiating from monitoring to cognitive challenges were quite the same in both groups. This shows that, like HPGs, LPGs were mindful to monitor their progress to attain their learning goals. However, while research emphasise that monitoring is an important regulatory process that helps learners develop strategies to control their learning, LPGs of this study were unable to develop strategies when faced with cognitive challenges during the task (Isohätälä et al., Citation2020). Collaborative learning environments require groups to respond to challenges and create opportunities for regulating cognitive processes. However, the LPGs struggled to respond to cognitive challenges that may have hindered their performance during the task.
In collaborative learning, research demonstrates that learners are generally unable to recognise a demand to regulate learning (Järvenoja et al., Citation2017). In this study, this process of regulatory control was observed only among HPGs, as LPGs were less aware of and less successful in regulating their learning. Moreover, monitoring has been observed to lead to controlling activities (Molenaar & Chiu, Citation2014). In the present study, both groups exhibited similarities in the sequentiality of monitoring with cognitive challenges. Nevertheless, only HPGs manifested a stronger sequential relationship between controlling and cognitive challenges. One possible explanation for the weaker sequential connection between controlling and cognitive challenges among LPGs is their poor monitoring skills, as previous research has shown that poor monitoring can hinder learners from initiating control (Molenaar & Chiu, Citation2014).
Sequential pathways between cognitive regulation and emotional/motivational regulation
Both groups had different sequential pathways between cognitive regulation (i.e., planning, monitoring, and controlling) and emotional/motivational regulation. LPGs manifested fewer sequential pathways between cognitive regulation and emotional/motivational regulation compared to HPGs. The process map of LPGs shows a less sequential relationship (i.e., nine and six frequencies) between planning and emotional/motivational regulation as compared to HPGs, which had 30 and 20 frequencies.
Regarding monitoring, HPGs transited more frequently between the sequential pathways of monitoring and emotional/motivational regulation with the frequency values of 51 and 43. LPGs, on the same pathways, had frequency values that were almost half as high (i.e., 22 and 24). The pathways between controlling and emotional/motivational regulation were weak in the process model of LPGs, with frequency values of two and four. However, HPGs, in the same situation, had stronger pathways in terms of frequency (i.e., 10 and 15). As prior studies have found a complex relationship between metacognitive experience for cognitive regulation and the presentation of feelings and judgements while performing a collaborative task (Dindar et al., Citation2020), this study further reveals that HPGs differ from LPGs regarding the relationship between cognitive regulation and emotional/motivational regulation. Further experimental investigations are needed to quantify the effect of the relationship between cognitive regulation and emotional/motivational regulation on learning performance.
Sequential pathways between emotional/motivational challenges and cognitive regulation
Both groups differed in the sequential pathways between emotional/motivational challenges and cognitive regulation. LPGs manifested more sequential pathways (i.e., 35 and 32 in frequency) between planning and challenges compared to HPGs, whose frequency values were 22 and 18. Similarly, with frequency values of 43 and 48, the process model of LPGs showed more sequential pathways between cognitive regulation monitoring and emotional/motivational challenges than HPGs. The sequential pathways between cognitive regulation controlling and emotional/motivational challenges were developed more frequently in LPGs than in HPGs in terms of frequency. These results could confirm the hypothesis that LPGs are less effective in performing the SSRL than HPGs when they face emotional/motivational challenges in learning.
Discussion
Research has shown that regulation in collaborative learning has several benefits (Malmberg et al., Citation2017). However, there is a scarcity of empirical evidence in the literature examining the sequential relationship between challenges and regulation in high- and low-performing groups. Therefore, in this study, we aimed to investigate the frequency, duration, and sequential relationship between cognitive and emotional/motivational challenges and regulation in high- and low-performing groups.
While the findings of this study, which included several Mann–Whitney U tests, did not yield statistically significant differences in the frequency and duration of the coding categories between high- and low-performing collaborative groups, evidence was provided in line with the previous studies (e.g., Schoor & Bannert, Citation2012; Bannert et al., 2014; Paans et al., Citation2019a) for the argument that the success of collaborative groups should not be solely determined by the duration or the amount of regulation. Research in education has emphasised the role of reporting insignificant results to accumulate knowledge towards evaluating and refining theories and existing findings (Moalosi, Citation2013). Like earlier studies, our study did not find statistically significant differences between the groups in terms of coding category frequencies, yet the findings enhanced our theoretical and methodological knowledge by highlighting the need to explore the regulatory processes in investigating learning regulation. Therefore, this study can also be viewed in a similar light, as it contributes to our understanding of the theoretical and methodological framework of regulated learning by uncovering the sequential patterns between challenges and regulation.
Importantly, in this study, we demonstrated that the sequential relationships between challenges and regulation should be considered to better understand the regulatory process between high- and low-performing groups. The current study’s focus on the sequential patterns of challenges and regulation in high- and low-performing collaborative groups is a departure from Schoor and Bannert’s (Citation2012) research, which found no difference in the sequential pathways of planning, monitoring, evaluation, and motivation between low- and high-achieving dyads. In contrast, the process models of the current study’s high- and low-performing groups demonstrated distinct sequential pathways between cognitive and emotional/motivational challenges and regulation. This result highlights the importance of considering the specific patterns of regulation and challenges within collaborative groups when evaluating their performance, rather than just the frequency or duration of these processes.
Furthermore, this study utilised a fine-grained approach to coding categories from group utterances, which allowed us to consider event duration in process mining. Our findings are in line with previous research (e.g., Su et al., Citation2018), especially in terms of the number of sequential pathways between regulatory processes, which included monitoring and socio-emotional regulation. Su et al. found that low-performing groups had more sequential pathways between regulatory processes, which is similar the present study’s findings that LPGs had more patterns of cognitive challenges. At the same time, the HPGs in the present study developed strong sequential pathways between cognitive and emotional/motivational regulation to counter challenges, which differs from Su et al.’s findings. Su et al. found no significant differences in the frequency of cognitive challenges between high- and low-performing groups. However, the present study found that HPGs had higher frequencies of cognitive challenges than LPGs.
Research shows that initiating the regulatory process of planning is vital to broadening understanding in collaborative learning (Kempler-Rogat & Linnenbrink-Garcia, Citation2011). This could be an indicator of performance during collaborative learning (Janssen et al., Citation2012). The theoretical framework of regulated learning posits that planning is initiated not only to understand the task but also to clarify learning conditions, including learners’ perceptions of the task, its content, and the design roadmap to achieve learning objectives (Kempler-Rogat & Linnenbrink-Garcia, Citation2011; Malmberg et al., Citation2017). Through this theoretical lens, HPGs could have realised that they should initiate planning purposefully, as the process models demonstrated that HPGs had more sequential transitions between planning and cognitive challenges in contrast to the LPGs. One reason LPGs may not have actively initiated planning to counter cognitive challenges is that they may have been unaware of precisely when to initiate planning to proceed with the learning task and tackle the challenges.
The theoretical framework of regulated learning in collaborative learning settings places weight on emotional regulation as a means of succeeding in collaboration (Järvenoja et al., Citation2019; Näykki et al., Citation2014). Initiating the process of emotion regulation helps learners apply different regulatory strategies, such as formulating shared perceptions through the interpretation and observation of emotional responses. This in turn leads to a sense of social inclusion, which decreases socio-emotional conflict (Bakhtiar et al., Citation2018; Järvenoja et al., Citation2015; Kwon et al., Citation2014). In addition, joint emotion regulation develops collaborative synchrony, which leads to socially shared regulated learning (Järvenoja et al., Citation2019). Based on these theoretical perspectives, it can be argued that the HPGs were more aware of the emergence of cognitive challenges and utilised the SSRL to address them by initiating emotion/motivational regulation.
Further, HPGs not only relied on cognitive regulation, but also actively applied different regulatory strategies (emotional/motivational in this case). This finding is in line with the work of Zheng and Yu (Citation2016), who found that high achievers were active in implementing different regulatory strategies (i.e., monitoring, controlling, and goal setting) to achieve their learning objectives.
Regarding emotional and motivational challenges, the present study found that the stronger and more frequent sequential transitions were between emotional/motivational challenges and cognitive regulation rather than emotional/motivational regulation. Research has shown that the emergence of regulatory processes during learning depends on the task and its context (Winne, Citation2014), as well as on the demands of the learning situation (Järvenoja et al., Citation2015, Citation2018). Therefore, it can be inferred that both categorised groups were mindful of the context and situation and used the regulatory processes of cognition, rather than those of emotion/motivation, to address emotional/motivational challenges. In collaborative learning, research has shown that the cognitive aspects of regulation are more prominent than emotional regulation (Kwon et al., Citation2014; Ucan & Webb, Citation2015). Additionally, the findings and context of this study suggest that emotional and motivational challenges may be related to the cognitive aspects of collaborative tasks. Emotional/motivational challenges could be considered the result of failing to proceed with the task rather than the emergence of socio-emotional conflicts between the members of collaborative groups. Therefore, it can be argued that emotional/motivational challenges drove group members to run regulatory processes of cognition rather than emotion/motivation.
The process models revealed a surprising finding, in that the sequential patterns between emotional/motivational challenges and cognitive regulation were stronger in LPGs than in HPGs. LPGs made almost twice as many sequential transitions as HPGs between emotional/motivational challenges and planning. This is consistent with the literature suggesting that low performers often struggle to develop effective regulatory strategies to overcome challenges and make progress in tasks, often relying on a trial-and-error approach (Beheshitha et al., Citation2015; Chang et al., Citation2017; Hong & Liu, Citation2003). The process model showed that LPGs frequently switched back and forth between emotional/motivational challenges and planning because they were unable to develop effective strategies to improve their performance. This led to the emergence of further emotional/motivational challenges. Similar results were found in previous research by Beheshitha et al. (Citation2015) and Heirweg et al. (Citation2020), who found that surface learners and low achievers, respectively, often failed to develop effective strategies and relied on a trial-and-error approach. Therefore, the result of LPGs’ more frequent sequential switch between emotional/motivational challenges and planning is consistent with previous research.
It is interesting to note that the findings of this study and Zhang et al.’s (Citation2021) are in agreement regarding the importance of monitoring in collaborative learning. Both studies suggest that monitoring plays a crucial role in collaborative learning, and that HPGs tend to have stronger patterns of monitoring than LPGs. Additionally, both studies suggested that monitoring is associated with establishing a positive emotional collaborative environment. These findings highlight the importance of monitoring and emotional regulation in collaborative learning and suggest that these skills should be explicitly taught and practiced in collaborative learning settings.
The theoretical framework of SSRL further suggests that collaborative groups work together to regulate cognitive, motivational, emotional, and/or behavioural aspects to overcome challenges (Hadwin et al., Citation2018). However, the process models of both groups, especially HPGs, show stronger sequential patterns between cognitive and emotional/motivational regulation than between cognitive and emotional/motivational challenges. According to Järvelä, Kirschner, et al. (Citation2016), regulatory processes are typically initiated by cognitive factors. The findings of this study support that both groups show a higher frequency of sequential connections between cognitive and emotional/motivational regulation.
In addition to planning and monitoring, the process model of HPGs shows that, they also develop stronger sequential connections for controlling compared to LPGs. To some extent, this result is parallel with the findings of Kempler-Rogat and Linnenbrink-Garcia (Citation2011). The researchers concluded that synergy between planning and monitoring helps collaborative groups achieve their joint learning goals. However, the process model of HPGs expands on this understanding by demonstrating that the synergistic inclusion of controlling the learning process, in conjunction with planning and monitoring, helps groups sustain their collaboration and achieve their joint learning objectives.
Finally, the results showed that the sequential connections between emotional/motivational challenges and regulation were not particularly insightful. This contrasts with the results of Ucan and Webb’s (Citation2015) study. The researchers suggested that regulatory processes of emotion and motivation are triggered by socially challenging situations. However, we propose that emotional/motivational regulation may emerge when collaborative groups face cognitive difficulties related to learning. This proposition is supported by the theoretical grounding that regulatory processes are typically initiated by cognitive aspects (Järvelä, Kirschner, et al., Citation2016). Thus, the process models of this study illustrate that emotional/motivational regulation is more strongly and sequentially linked with planning, monitoring, and controlling than with either type of challenge. It can thus be inferred that when facing challenges, collaborative groups first engage in regulatory actions (i.e., planning, monitoring, and controlling) to overcome the challenges. The regulatory process of emotion/motivation emerges as a result of these regulatory actions, rather than directly because of the challenges themselves.
Implications
We envision some concrete educational and practical implications of the study for furthering pedagogical methods, helping improve learning content, and designing customised support for Nordic higher education collaborative groups. Studies suggest that knowledge constructions could be improved if collaborative learning environments are integrated with certain elements that trigger an SSRL (Järvelä & Hadwin, Citation2013; Järvelä et al., Citation2013). Through this lens, the present study provides empirical evidence of the learning processes (i.e., challenges and regulation) of low- and high-performing collaborative groups in higher education. This in turn provides directions for developing supportive elements that may help groups initiate regulatory processes during learning in a collaborative learning environment. For instructional developers in higher education, the results of the present study imply that pedagogical methodologies and learning content may have prompts to not only encourage learners to monitor group progress, but also control learning by initiating strategies to deal with challenges. The results also suggest that scaffolding should be integrated to support collaborative groups that lack the skills to encounter challenges. Since this study applied process analysis, instructional designers could glean some benefits, for example, by integrating prompts or scripts into learning content that may encourage collaborative groups to resolve challenges by initiating regulatory processes. Another implication is that the duration of regulation may not need to be considered when designing prompts or scripts for collaborative learning, as it was not a determining factor for either the high- or low-performing groups in the study.
The field of learning analytics research is devoted to creating customised and specific feedback for students (Gaševic et al., Citation2016). This study contributes to the theoretical understanding of SRL by addressing its difficulties; in doing so, it can assist in the development of personalised and timely feedback tools for collaborative groups in higher education. This study also shows that high- and low-performing groups differ in how they regulate challenges, so grouping students beforehand and providing tailored support for learning regulation could be beneficial for collaborative learning tasks.
This study’s theoretical implications suggest that the duration of regulation of learning does not have a significant impact on the success or failure of collaborative learning. Furthermore, emotional or motivational challenges do not always result in emotional or motivational regulation, indicating that other factors may be at play. Additionally, the findings support the idea that cognitive regulation can be initiated by emotional or motivational challenges rather than just cognitive ones, providing a new perspective on the relationship between emotional and cognitive regulation.
Conclusion
The sequential relationship between challenges and cognitive and emotional/motivational regulation in collaborative learning settings are still underexplored. To address this gap, the present study applyed a process mining methodology to investigate the sequential relationship between challenges and regulation in high- and low-performing collaborative groups. The results revealed that HPGs developed stronger connections between cognitive and emotional/motivational regulation to tackle cognitive challenges, unlike LPGs. Both groups monitored their learning, but HPGs were able to directly apply successful strategies, while LPGs relied on trial and error. The study suggests that the amount of time spent on learning regulation is not necessarily an indicator of success or failure in collaborative learning. The findings extend prior research and deepen our understanding of regulated learning and its relationship to challenges in a higher education context. Additionally, the results have practical implications for designing personalised support and feedback, pedagogical methodologies, and learning content in collaborative learning environments at the higher education level.
Limitations and future work
This study has some limitations that make generalisation of the findings challenging. First, the study used only video data, which has limitations in analysing specific behaviour and the reasons for its manifestation in particular patterns and connections in learning processes (Järvenoja et al., Citation2019). Future studies should use multimodal datasets to increase the generalizability of the results. Second, some groups were familiar with each other and were from the same degree programme, which may have influenced their collaboration and learning processes. Future studies should take this into account. Third, the study did not capture the sequential relationship of off-task activities with challenges and regulation due to the design of the learning task and the video data of the groups. Examining off-task activities could have been informative in exploring whether collaborative groups manifested sequential patterns of off-task activities when facing challenges. Future research could also capture off-task activities to explore the sequentialities among different variables. Lastly, the process mining methodology is insufficient for examining the temporality of the occurrence of research variables. Therefore, future studies could use temporal methods in combination with process mining to address this issue.
Acknowledgements
We would like to thank Mohammad Saqr for helping first author learn and apply process mining methodology.
Disclosure statement
No potential conflict of interest was reported by the author(s).
References
- Bakhtiar, A., Webster, E. A., & Hadwin, A. F. (2018). Regulation and socio-emotional interactions in a positive and a negative group climate. Metacognition and learning, 13(1), 57–90. https://doi.org/10.1007/s11409-017-9178-x
- Bannert, M., Reimann, P., & Sonnenberg, C. (2013). Process mining techniques for analysing patterns and strategies in students’ self-regulated learning. Metacognition and Learning, 9(2), 161–185. https://doi.org/10.1007/s11409-013-9107-6
- Beheshitha, S. S., Gašević, D., & Hatala, M. (2015, March). A process mining approach to linking the study of aptitude and event facets of self-regulated learning. Proceedings of the Fifth International Conference on Learning Analytics and Knowledge (pp. 265–269). https://doi.org/10.1145/2723576.2723628
- Chang, C., Chang, M., Chiu, B., Liu, C., Fan Chiang, S., Wen, C., Hwang, F.-K., Wu, Y.-T., Chao, P.-Y., Lai, C.-H., Wu, S.-W., Chang, C.-K., & Chen, W. (2017). An analysis of student collaborative problem solving activities mediated by collaborative simulations. Computers & Education, 114, 222–235. https://doi.org/10.1016/j.compedu.2017.07.008
- Cukurova, M., Luckin, R., Millán, E., & Mavrikis, M. (2018). The NISPI framework: Analysing collaborative problem-solving from students’ physical interactions. Computers & Education, 116, 93–109. https://doi.org/10.1016/j.compedu.2017.08.007
- Danner, D., Hagemann, D., Schankin, A., Hager, M., & Funke, J. (2011). Beyond IQ: A latent state-trait analysis of general intelligence, dynamic decision making, and implicit learning. Intelligence (Norwood), 39(5), 323–334. https://doi.org/10.1016/j.intell.2011.06.004
- Dindar, M., Järvelä, S., & Järvenoja, H. (2020). Interplay of metacognitive experiences and performance in collaborative problem solving. Computers & Education, 154, 103922. https://doi.org/10.1016/j.compedu.2020.103922
- Fleiss, J. (1981). Statistical examples for rates and proportions. Wiley.
- Fluxicon. (2019). Disco user’s guide. Retrieved January 7, 2019, from https://fuxicon.com/disco/fles/Disco-User-Guide.pdf
- Gašević, D., Dawson, S., Rogers, T., & Gasevic, D. (2016). Learning analytics should not promote one size fits all: The effects of instructional conditions in predicting academic success. The Internet and Higher Education, 28, 68–84. https://doi.org/10.1016/j.iheduc.2015.10.002
- Hadwin, A. F., Bakhtiar, A., & Miller, M. (2018). Challenges in online collaboration: Effects of scripting shared task perceptions. International Journal of Computer-Supported Collaborative Learning, 13(3), 301–329. https://doi.org/10.1007/s11412-018-9279-9
- Hadwin, A. F., Järvelä, S., & Miller, M. (2011). Self-regulated, co-regulated, and socially shared regulation of learning. In B. J. Zimmerman & D. H. Schunk (Eds.), Handbook of self-regulation of learning and performance (pp. 65–84). Routledge.
- Hadwin, A. F., Oshige, M., Gress, C. L., & Winne, P. H. (2010). Innovative ways for using gStudy to orchestrate and research social aspects of self-regulated learning. Computers in Human Behavior, 26(5), 794–805. https://doi.org/10.1016/j.chb.2007.06.007
- Häkkinen, P., Järvelä, S., Mäkitalo-Siegl, K., Ahonen, A., Näykki, P., & Valtonen, T. (2017). Preparing teacher-students for twenty-first-century learning practices (PREP 21): A framework for enhancing collaborative problem-solving and strategic learning skills. Teachers and Teaching, Theory and Practice, 23(1), 25–41. https://doi.org/10.1080/13540602.2016.1203772
- Heirweg, S., De Smul, M., Merchie, E., Devos, G., & Van Keer, H. (2020). Mine the process: Investigating the cyclical nature of upper primary school students’ self-regulated learning. Instructional Science, 48(4), 337–369. https://doi.org/10.1007/s11251-020-09519-0
- Hong, J., & Liu, M. (2003). A study on thinking strategy between experts and novices of computer games. Computers in Human Behavior, 19(2), 245–258. https://doi.org/10.1016/S0747-5632(02)00013-4
- Isohätälä, J., Näykki, P., & Järvelä, S. (2020). Convergences of joint, positive interactions and regulation in collaborative learning. Small Group Research, 51(2), 229–264. https://doi.org/10.1177/1046496419867760
- Janssen, J., Erkens, G., Kirschner, P. A., & Kanselaar, G. (2012). Task-related and social regulation during online collaborative learning. Metacognition and Learning, 7(1), 25–43. https://doi.org/10.1007/s11409-010-9061-5
- Janssenswillen, G., Depaire, B., Swennen, M., Jans, M., & Vanhoof, K. (2019). Bupar: Enabling reproducible business process analysis. Knowledge-based Systems, 163, 927–930. https://doi.org/10.1016/j.knosys.2018.10.018
- Järvelä, S., Gašević, G., Seppänen, T., Pechenizkiy, M., & Kirschner, P. A. (2020). Bridging learning sciences, machine learning and affective computing for understanding cognition and affect in collaborative learning. British Journal of Educational Technology, 51(6), 2391–2406. https://doi.org/10.1111/bjet.12917
- Järvelä, S., & Hadwin, A. F. (2013). New Frontiers: Regulating learning in CSCL. Educational Psychologist, 48(1), 25–39. https://doi.org/10.1080/00461520.2012.748006
- Järvelä, S., Järvenoja, H., & Malmberg, J. (2019). Capturing the dynamic and cyclical nature of regulation: Methodological progress in understanding socially shared regulation in learning. International Journal of Computer-Supported Collaborative Learning, 14(4), 425–441. https://doi.org/10.1007/s11412-019-09313-2
- Järvelä, S., Järvenoja, H., Malmberg, J., Isohätälä, J., & Sobocinski, M. (2016). How do types of interaction and phases of self-regulated learning set a stage for collaborative engagement? Learning and Instruction, 43, 39–51. https://doi.org/10.1016/j.learninstruc.2016.01.005
- Järvelä, S., Järvenoja, H., & Näykki, P. (2013). Analyzing regulation of motivation as an individual and social process. In M. Vauras & S. Volet (Eds.), Interpersonal regulation of learning and motivation: Methodological advances (pp. 170–188). New York: Routledge.
- Järvelä, S., Järvenoja, H., & Veermans, M. (2008). Understanding the dynamics of motivation in socially shared learning. International Journal of Educational Research, 47(2), 122–135. https://doi.org/10.1016/j.ijer.2007.11.012
- Järvelä, S., Kirschner, P. A., Hadwin, A., Järvenoja, H., Malmberg, J., Miller, M., & Laru, J. (2016). Socially shared regulation of learning in CSCL: Understanding and prompting individual- and group-level shared regulatory activities. International Journal of Computer-Supported Collaborative Learning, 11(3), 263–280. https://doi.org/10.1007/s11412-016-9238-2
- Järvenoja, H., Järvelä, S., & Malmberg, J. (2015). Understanding regulated learning in situative and contextual frameworks. Educational Psychologist, 50(3), 204–219. https://doi.org/10.1080/00461520.2015.1075400
- Järvenoja, H., Järvelä, S., & Malmberg, J. (2017). Supporting groups’ emotion and motivation regulation during collaborative learning. Learning and Instruction, 70, 101090. https://doi.org/10.1016/j.learninstruc.2017.11.004
- Järvenoja, H., Järvelä, S., & Malmberg, J. (2020). Supporting groups’ emotion and motivation regulation during collaborative learning. Learning and Instruction, 70, 101090. https://doi.org/10.1016/j.learninstruc.2017.11.004
- Järvenoja, H., Järvelä, S., Törmänen, T., Näykki, P., Malmberg, J., Kurki, K., & Isohätälä, J. (2018). Capturing motivation and emotion regulation during a learning process. Frontline Learning Research, 6(3), 85–104. https://doi.org/10.14786/flr.v6i3.369
- Järvenoja, H., Näykki, P., & Törmänen, T. (2019). Emotional regulation in collaborative learning: When do higher education students activate group level regulation in the face of challenges? Studies in Higher Education (Dorchester-on-Thames), 44(10), 1747–1757. https://doi.org/10.1080/03075079.2019.1665318
- Kapur, M. (2011). Temporality matters: Advancing a method for analyzing problem-solving processes in a computer-supported collaborative environment. International Journal of Computer-Supported Collaborative Learning, 6(1), 39–56. https://doi.org/10.1007/s11412-011-9109-9
- Kempler-Rogat, T., & Linnenbrink-Garcia, L. (2011). Socially shared regulation in collaborative groups: An analysis of the interplay between quality of social regulation and group processes. Cognition and Instruction, 29(4), 375–415. https://doi.org/10.1080/07370008.2011.607930
- Knight, S., Friend Wise, A., & Chen, B. (2017). Time for change: Why learning analytics needs temporal analysis. Journal of Learning Analytics, 4(3). https://doi.org/10.18608/jla.2017.43.2
- Koivuniemi, M., Järvenoja, H., & Järvelä, S. (2018). Teacher education students’ strategic activities in challenging collaborative learning situations. Learning, Culture and Social Interaction, 19, 109–123. https://doi.org/10.1016/j.lcsi.2018.05.002
- Kwon, K., Liu, Y., & Johnson, L. P. (2014). Group regulation and social-emotional interactions observed in computer supported collaborative learning: Comparison between good vs. Poor collaborators. Computers and Education, 78, 185–200. https://doi.org/10.1016/j.compedu.2014.06.004
- Malmberg, J., Järvelä, S., & Järvenoja, H. (2017). Capturing temporal and sequential patterns of self-, co-, and socially shared regulation in the context of collaborative learning. Contemporary Educational Psychology, 49, 160–174. https://doi.org/10.1016/j.cedpsych.2017.01.009
- Malmberg, J., Järvelä, S., Järvenoja, H., & Panadero, E. (2015). Promoting socially shared regulation of learning in CSCL: Progress of socially shared regulation among high- and low-performing groups. Computers in Human Behavior, 52, 562–572. https://doi.org/10.1016/j.chb.2015.03.082
- Mänty, K., Järvenoja, H., & Törmänen, T. (2020). Socio-emotional interaction in collaborative learning: Combining individual emotional experiences and group-level emotion regulation. International Journal of Educational Research, 102, 101589. https://doi.org/10.1016/j.ijer.2020.101589
- Moalosi, S. W. T. (2013). Teachers self efficacy: Is reporting non-significant results essential? Journal of International Education Research (JIER), 9(4), 397–406. https://doi.org/10.19030/jier.v9i4.8266
- Molenaar, I., & Chiu, M. M. (2014). Dissecting sequences of regulation and cognition: Statistical discourse analysis of primary school children’s collaborative learning. Metacognition and Learning, 9, 137–160. https://doi.org/10.1007/s11409-013-9105-8.
- Näykki, P., Isohätälä, J., & Järvelä, S. (2021b). “You really brought all your feelings out” – scaffolding students to identify the socio-emotional and socio-cognitive challenges in collaborative learning. Learning, Culture and Social Interaction, 30, 100536. https://doi.org/10.1016/j.lcsi.2021.100536
- Näykki, P., Järvelä, S., Kirschner, P., & Järvenoja, H. (2014). Socio-emotional conflict in collaborative learning—A process-oriented case study in a higher education context. International Journal of Educational Research, 68, 1–14. https://doi.org/10.1016/j.ijer.2014.07.001
- Näykki, P., Kontturi, H., Seppänen, V., Impiö, N., & Järvelä, S. (2021a). Teachers as learners - a qualitative exploration of pre-service and in-service teachers’ continuous learning community OpenDigi. Journal of Education for Teaching, 47, 495–512. https://doi.org/10.1080/02607476.2021.1904777
- Nguyen, A., Järvelä, S., Rosé, C., Järvenoja, H., & Malmberg, J. (2023). Examining socially shared regulation and shared physiological arousal events with multimodal learning analytics. British Journal of Educational Technology, 54(1), 293–312. https://doi.org/10.1111/bjet.13280
- Paans, C., Molenaar, I., Segers, E., & Verhoeven, L. (2019b). Temporal variation in children's self-regulated hypermedia learning. Computers in Human Behavior, 96, 246–258. https://doi.org/10.1016/j.chb.2018.04.002
- Paans, C., Onan, E., Molenaar, I., Verhoeven, L., & Segers, E. (2019a). How social challenges affect children’s regulation and assignment quality in hypermedia: A process mining study. Metacognition and Learning, 14(2), 189–213. https://doi.org/10.1007/s11409-019-09204-9
- Panadero, E., & Järvelä, S. (2015). Socially shared regulation of learning: A review. European Psychologist, 20, 190–203. https://doi.org/10.1027/1016-9040/a000226
- Schoor, C., & Bannert, M. (2012). Exploring regulatory processes during a computer-supported collaborative learning task using process mining. Computers in Human Behavior, 28(4), 1321–1331. https://doi.org/10.1016/j.chb.2012.02.016
- Sinha, S., Rogat, T. K., Adams-Wiggins, K. R., & Hmelo-Silver, C. E. (2015). Collaborative group engagement in a computer-supported inquiry learning environment. International Journal of Computer-Supported Collaborative Learning, 10(3), 273–307. https://doi.org/10.1007/s11412-015-9218-y
- Sobocinski, M., Malmberg, J., & Järvelä, S. (2021). Exploring adaptation in socially-shared regulation of learning using video and heart rate data. Technology, Knowledge and Learning, 27(2), 385–404. https://doi.org/10.1007/s10758-021-09526-1
- Sonnenberg, C., & Bannert, M. (2015). Discovering the effects of metacognitive prompts on the sequential structure of SRL-processes using process mining techniques. Journal of Learning Analytics, 2(1). https://doi.org/10.18608/jla.2015.21.5
- Su, Y., Li, Y., Hu, H., & Rose, C. P. (2018). Exploring college English language learners’ self and social regulation of learning during wiki-supported collaborative reading activities. International Journal of Computer-Supported Collaborative Learning, 13, 13–35. https://doi.org/10.1007/s11412-018-9269-y
- Sullivan, F. R., & Wilson, N. C. (2015). Playful talk: Negotiating opportunities to learn in collaborative groups. Journal of the Learning Sciences, 24(1), 5–52. https://doi.org/10.1080/10508406.2013.839945
- Ucan, S., & Webb, M. (2015). Social regulation of learning during collaborative inquiry learning in science: How does it emerge and what are its functions? International Journal of Science Education, 37(15), 2503–2532. https://doi.org/10.1080/09500693.2015.1083634
- Volet, S., & Mansfield, C. (2006). Group work at university: Significance of personal goals in the regulation strategies of students with positive and negative appraisals. Higher Education Research & Development, 25(4), 341–356. https://doi.org/10.1080/07294360600947301
- Winne, P. H. (2014). Issues in researching self-regulated learning as patterns of events. Metacognition and Learning, 9(2), 229–237. https://doi.org/10.1007/s11409-014-9113-3
- Zhang, S., Chen, J., Wen, Y., Chen, H., Gao, Q., & Wang, Q. (2021). Capturing regulatory patterns in online collaborative learning: A network analytic approach. International Journal of Computer-Supported Collaborative Learning, 16(1), 37–66. https://doi.org/10.1007/s11412-021-09339-5
- Zheng, L., & Yu, J. (2016). Exploring the behavioral patterns of Co-regulation in mobile computer-supported collaborative learning. Smart Learning Environments, 3(1), 1–20. https://doi.org/10.1186/s40561-016-0024-4