ABSTRACT
Background: Alcohol use disorder (AUD) treatments, including medications, are increasingly offered via telehealth.
Objective: This study characterizes 90-day treatment retention and changes in objectively measured blood alcohol concentration (BAC) in a large cohort receiving AUD telehealth.
Methods: Patients received AUD treatment through Ria, a virtual (telehealth) program offering AUD treatment that is tailored to patient goals (e.g. abstinence or controlled drinking). Patients were encouraged to complete breathalyzer readings twice daily for measurement-based care. We characterized rates of 90-day treatment retention (i.e. completing a BAC reading or medical/coaching encounter on the 90th day or later) and used growth curve analyses to model changes in daily estimated peak BAC over 90 days.
Results: Of 4121 patients (51.5% women), 50.1% had 90-day treatment retention (n = 2066, 52.2% women). Most patients received prescriptions for AUD medications (84.6%) and completed encounters with medical providers (86.7%) and coaches (86.1%). Patients with 90-day retention provided 184,817 BAC readings in the first 90 days. Growth curve analyses revealed significant reductions in daily estimated peak BAC (p < .001) from a mean of 0.092 (day 1) to 0.038 (day 90). Similar magnitudes of BAC reduction were observed for men and women and for patients with abstinence and controlled drinking goals.
Conclusion: Telehealth appears to be a viable approach to delivering AUD treatments in a manner that promotes drinking reductions. Telehealth approaches can yield reductions in objectively measured BAC, including for some patient subgroups that have historically faced greater stigma in AUD treatment settings, such as women and people with non-abstinence drinking goals.
Introduction
Approximately 13.9% of adults in the United States (US) annually meet criteria for alcohol use disorder (AUD) (Citation1), however, only one in five people with AUD receives alcohol treatment (Citation2). Numerous barriers can inhibit treatment-seeking, including stigma, limited or no access to treatment providers, and difficulty getting to treatment due to time or travel requirements (Citation3,Citation4). Moreover, even when patients access AUD treatment programs, they often are not offered evidence-based treatments; for example, less than half of US drug and alcohol treatment programs report offering medications for AUD (Citation5) despite their efficacy (Citation6) and recommended use by clinical guidelines (Citation7,Citation8).
Barriers to AUD treatment may be even more salient for some patient subgroups. For example, women are often subject to greater stigma for having AUD, experience less social support for seeking treatment, face more structural barriers to accessing treatment (e.g., childcare responsibilities, threats of losing child custody, etc.), and are less likely to receive treatment for AUD than men (Citation9,Citation10). Additionally, many people with AUD who want to reduce their drinking without abstaining completely (e.g., people with a controlled drinking goal) often do not seek treatment, potentially due to incongruence of their goals with the abstinence-focused goals of many treatment programs. Yet, when patients are offered treatments that are accepting of controlled drinking goals, they typically experience similar drinking reductions and social functioning outcomes as those that are observed in abstinence-focused treatments (Citation11,Citation12).
Telehealth may help increase access to AUD treatment services, including treatment encounters with addiction medicine specialists who can offer evidence-based treatments like medications for AUD. Telehealth also can facilitate treatment delivery in a manner that is more private, flexible, geographically available, and less time-intensive than traditional in-person services. Systematic reviews indicate that addiction treatments delivered by telehealth can be effective in reducing substance use and increasing treatment accessibility and patient satisfaction (Citation13,Citation14); however, these studies have often studied telehealth as an add-on to other treatment programs (e.g., aftercare delivered via telehealth) rather than as primary treatment. Further, a recent systematic review suggested that the majority of patients in clinical trials of telehealth treatments for substance use disorders had minimal or no utilization of the telehealth services that were offered (Citation14).
Building on the potential benefits of telehealth, the Ria treatment platform was launched in 2017 to provide AUD treatment from addiction medicine specialists through a completely virtual (telehealth) format. The program offers treatment that is tailored to the goals of patients (e.g., including goals of abstinence or controlled drinking) and offers prescriptions for medications for AUD, regular visits with addiction medicine specialists and recovery coaches, didactic learning materials, and breathalyzer readings of blood alcohol concentration (BAC) to facilitate treatment progress monitoring.
An early observational study of patients in the Ria treatment program showed that 55% of patients who started the program were retained in treatment for 90 days or longer and that patients who were retained for 90 days or longer (n = 77) had significant reductions in daily BAC over the first 90 days of treatment (mean BAC = 0.090 on day 1, mean BAC = 0.045 on day 90) (Citation15). However, due to the small sample size of that initial study, the precision and generalizability of the findings were limited and it was not possible to evaluate how 90-day treatment retention or BAC reductions may have differed across patient subgroups.
The goal of the current study was to characterize 90-day treatment retention and BAC reductions in a large cohort of participants who received AUD telehealth through the Ria treatment platform. First, we characterized 90-day treatment retention rates among all patients who initiated treatment, then compared the patient demographics, treatment goals, and clinical services received by patients with and without 90-day retention. Then, for patients with 90-day treatment retention, we characterized the trajectories of change in daily estimated peak BAC over the first 90 days of treatment when BAC data were available. Following this, we characterized whether the trajectories of change in daily estimated peak BAC over the first 90 days differed across patient subgroups defined by gender (men, women) and drinking goal (e.g., abstinence, controlled drinking, or unknown drinking goal).
To our awareness, this is the largest longitudinal observational study to provide information about treatment retention and changes in objectively measured BAC during AUD treatment delivered entirely via telehealth. The findings of this study in turn may help provide a more precise understanding of treatment retention and drinking reductions in telehealth-based AUD treatments, including for patient subgroups that face particularly salient treatment barriers (women; patients with controlled drinking goals), which in turn may help inform efforts to reduce barriers to accessing AUD treatment and improving retention and effectiveness of AUD treatments delivered via telehealth.
Materials and methods
Setting and participants
The current study sample included all patients who enrolled in the Ria platform during a study catchment period of 01/2017 to 12/2021. To be eligible for the treatment program, patients had to report symptoms consistent with AUD (assessment procedures described in Treatment Program section below), have an American Society of Addiction Medicine (ASAM) criteria score of 1.0 to 3.1, report a desire to reduce or abstain from drinking, have access to technology that that would facilitate receiving treatment services via telehealth (e.g., smartphone, tablet device, or computer) and live in a US state where a Ria clinician was licensed. Patients could pay for treatment through a combination of self-pay and insurance. Patients with emergent medical or psychiatric conditions were eligible for treatment entry only when they were deemed medically or psychiatrically stable enough to safely participate. Patients with high risk for complicated alcohol withdrawal (e.g., seizures, delirium tremens) were referred to medical detoxification programs prior to treatment entry.
Treatment program
The Ria treatment program offered management of AUD entirely via telehealth through a smartphone application. Patients completed intake assessments by videoconference with a medical provider or nurse who had expertise in addiction medicine (physician or psychiatric nurse practitioner). The intake assessment included a semi-structured clinical interview that followed a standardized template that included a detailed assessment of alcohol and substance use history, mental status, suicide risk, and medical, psychiatric, and social histories. The medical provider determined whether the patient met criteria for alcohol dependence based on International Classification of Diseases 10th revision criteria (Citation16) (code F10.2x) and assigned an ASAM level of care criteria score. At follow-up appointments with medical providers, patients could receive prescriptions for AUD medications followed by ongoing medication management. A follow-up medical appointment was usually scheduled 1–2 weeks after the intake, then usually followed by monthly appointments for the next three months and appointments every 2–3 months thereafter. However, the frequency of appointments and the duration of treatment were variable. Patients were free to remain in the treatment program as long as they desired. Treatment goals were periodically reviewed with care teams to ascertain whether goals were being met and to discuss whether changes in treatment plans or termination of treatment were warranted.
Throughout the program, patients had access to a recovery coach with certified addiction counseling credentials. Coaching encounters were typically completed by telephone, videoconference, or text message. Coaching appointments were typically scheduled weekly for the first three months, then typically decreased in frequency to biweekly or monthly. Patients also had access to cognitive-behavioral therapy-based learning modules.
Patients received a Bluetooth enabled breathalyzer (BACtrack C6 or BACtrack Mobile) to measure BAC up to twice daily. BAC readings were prompted by a smartphone application in the morning and at night, at times reflecting usual time of waking and going to bed. When BAC readings detected alcohol use, the application prompted patients to self-report when they took their last drink so peak BAC could be estimated (details in Measures section below). Longitudinal BAC measures could be viewed by patients through the smartphone application and by clinicians through a clinical dashboard to help monitor treatment progress. The protocol did not include procedures that ensured BAC readings were always completed by the identified patient; however, the risk for patients submitting false BAC readings was believed to be low because (1) patients were not penalized for missed BAC readings or for completing BAC readings that indicated heavy drinking, (2) clinicians anecdotally reported that breathalyzer results appeared to correlate with patient self-reports, and (3) many patients reported that seeing their breathalyzer results and tracking them over time helped motivate their treatment efforts. Additionally, patients typically self-referred to Ria Health on a voluntary basis without mandated treatment (e.g., not required by courts or transportation authorities).
Measures
The current study utilized data that was originally collected for clinical care by the treatment program. The use of previously collected, de-identified clinical data for the current study was considered non-human subjects research and issued an exemption by the University of Washington’s Institutional Review Board.
BAC readings were obtained from Bluetooth enabled breathalyzers that were transmitted by internet to the treatment program’s internal servers. Peak BAC was estimated via the Widmark equation (Citation17) based on recorded BAC readings and patients’ reports of the timing of their last drink. Estimated peak BAC values>0.50 were considered potential overestimates and were winsorized to values of 0.50 prior to analysis (0.03% of BAC readings). For the current study, on days with multiple BAC readings we retained the highest peak BAC estimate so the data would reflect estimated daily peak BAC. Sensitivity analyses that modeled BAC trajectories under different assumptions about potential drinking on days without BAC data are described below in the Analysis Plan and in the eSupplement.
We defined a 90-day observation period for every participant, with day 1 being the first day that a BAC reading was recorded within 30 days of initiating the treatment program. Patients were considered to have 90-day retention if they completed one or more BAC reading, medical encounter, and/or coaching encounter on or after the 90th day after the first BAC reading.
Age, gender, and drinking goal (abstinence, controlled drinking, or unknown drinking goal) were typically self-reported during intake assessments and were obtained from the treatment program’s electronic medical records. Data on race and ethnicity were not collected. Medication orders and clinical encounters with medical providers and coaches were counted for each patient over the 90-day observation period.
Analysis plan
First, we characterized 90-day treatment retention rates for all patients who initiated the treatment program. (Note: although the treatment program was not limited to 90 days, we modeled outcomes over 90 days to match an earlier observation study (Citation15) and because other studies of AUD treatments often utilize similar timeframes.) We used descriptive statistics to characterize patient demographics (gender, age), treatment goals, and treatment services during the 90-day observation period (medication orders, medical encounters, and coaching encounters) for patients with and without 90-day treatment retention. We examined the distributions of outcome measures to confirm their suitability for planned parametric statistical analyses. Differences between patient subgroups with and without 90-day treatment retention were tested using Fisher’s exact tests for categorical measures and independent samples t-tests for continuous measures.
Trajectories of daily estimated peak BAC were estimated among patients with 90-day treatment retention. The trajectory analyses only included patients with 90-day retention because (1) BAC data were collected as part of the treatment program and therefore availability of BAC data over the full 90-day period was contingent on 90-day treatment retention, and (2) evaluating changes in BAC for non-retained patients could yield biased results and invalid conclusions if non-retention was correlated with the amount of change in BAC (Citation18,Citation19). Therefore, results of the BAC trajectory analyses must be interpreted as reflecting changes in BAC only among patients with 90-day treatment retention.
BAC trajectory analyses were conducted using estimated daily peak BAC over the 90-day observation period. Prior to modeling BAC trajectories, we calculated the number of days with BAC data available over the 90-day observation period and used analysis of variance and independent sample t-tests to evaluate whether the number of days with BAC data differed across subgroups defined by gender and drinking goal or across days 1–30, days 31–60, and days 61–90.
Latent growth curve analyses were used to model the trajectories of daily estimated peak BAC over the 90-day observation period. These models were fit using mixed effect models with restricted maximum likelihood estimation via the lmerTest package in R (Citation20,Citation21). Informed by descriptive analyses and our earlier study (Citation15), we used a piecewise trajectory modeling approach that allowed the growth curves to account for potentially nonlinear trajectories of change over time (i.e., faster rates of change in the first two weeks treatment, slower rates of change in subsequent weeks). Specifically, the piecewise models were chosen to identify two linear trajectories, one to model more rapid changes in daily estimated peak BAC over days 1–14 and another to model the slower changes over days 15–90. We selected this two-part piecewise linear trajectory model for its interpretability (i.e., model coefficients reflect mean rates of change), the trajectories observed in the raw data, and because it yielded better model convergence compared to alternative trajectory parameterizations (e.g., quadratic, cubic). The two linear trajectories were modeled using both fixed effects (which model the mean trajectory across all patients) and random effects (which model variability in trajectories for each patient).
Subsequent analyses evaluated whether the trajectories of change in daily estimated peak BAC were significantly moderated by gender and treatment goal. These analyses were conducted by adding gender × time and drinking goal × time interactions to the model, as well as the main effects of gender and drinking goal. Moderating effects of gender and drinking goal were each tested separately. We plotted the model-estimated growth trajectories along with the observed mean values of daily estimated peak BAC to illustrate the modeled growth trajectories and to evaluate whether the modeled trajectories mapped onto the observed mean values of estimated daily peak BAC.
The maximum likelihood estimator utilized all available daily estimated peak BAC values, including when patients were missing BAC measures on one or more days. Similar to multiple imputation methods (Citation18), maximum likelihood yields unbiased growth trajectory results if BAC data are missing at random or missing completely at random (e.g., if missingness is assumed to be unrelated to the amount of drinking on a particular day). However, the assumption of data missing at random or completely at random is not empirically testable. Therefore, we conducted sensitivity analyses to examine whether BAC reductions would still be observed across different scenarios of abstinence and heavy drinking on days that did not have BAC data available (e.g., on days with missing BAC data, we tested different models that assumed heavy drinking, abstinence, or a mixture of heavy drinking and abstinence, then re-estimated the growth curve trajectories). We also examined whether the trajectories of change in daily estimated peak BAC significantly differed between patient subgroups with high, medium, or low amounts of BAC data available to test if trajectories appeared to be moderated by the amount of BAC data provided (see eSupplement).
Results
Description of sample
4121 patients enrolled in the treatment program during the study catchment period. Among them, 2066 (50.1%) met this study’s definition of 90-day retention. provides descriptive information about the demographics, treatment goals, and treatment services utilized by patients with and without 90-day retention.
Table 1. Patient demographics and clinical services received in the first 90 days of treatment program.
In both subgroups, slightly more than half of patients were women, although patients with 90-day retention had a slightly higher proportion of men and a lower proportion of patients with an unknown gender compared to patients without 90-day retention. Patients were, on average, in their mid-to-late 40’s, and those with 90-day retention were slightly older (2.65 year mean difference) than patients without 90-day retention. Controlled drinking was the most endorsed goal for both subgroups; patients with 90-day retention more often had a goal of controlled drinking and less often had an unknown drinking goal compared to patients without 90-day retention. In a logistic regression model that included gender, drinking goal, and age as simultaneous predictors of 90-day retention, all three measures remained significant independent predictors of 90-day retention (see eSupplement Table S1).
Nearly all patients with 90-day retention received at least one medication prescription, medical appointment, and coaching session (). Approximately three quarters of patients without 90-day retention received at least one medication prescription, medical appointment, and/or coaching session. For both subgroups, naltrexone was the most prescribed medication, followed by thiamine and gabapentin. Additional information about services received after the 90-day retention period are provided in the eSupplement (Table S2).
Availability of BAC measures
In total, patients with 90-day retention provided 184,817 BAC readings over the first 90 treatment days. The first BAC reading (considered “day 1” for the 90-day outcome period in the current study) occurred a mean of 5.07 days after treatment initiation (SD = 4.52). On average, each patient provided BAC data on 54.43 days (SD = 25.42) during the 90-day observation period. On days that a BAC was provided, 45.2% of days had one BAC reading and 54.8% had two or more BAC readings. There was a small but significant difference between the number of days with BAC data for women (M = 53.12 days, SD = 24.80) versus men (M = 55.86 days, SD = 26.02, p = .01). There were no differences in the number of days with BAC data between patients with an abstinence goal (M = 54.99, SD = 25.43), a controlled drinking goal (M = 54.39, SD = 25.51), or an unknown drinking goal (M = 53.93, SD = 25.08; p = .85). There were significant differences in the availability of BAC data over the three consecutive 30-day observation windows (p < .001), such that BAC data was more often available during treatment days 1–30 (M = 23.44 days, SD = 7.06) compared to treatment days 31–60 (M = 17.22 days, SD = 10.20) and treatment days 61–90 (M = 13.77 days, SD = 10.74).
Trajectories of daily estimated peak BAC
and provide results from a growth curve model representing changes in daily estimated peak BAC for patients with 90-day treatment retention. On average, daily estimated peak BAC decreased significantly (p < .001) from day 1 (model-estimated peak BAC = 0.092) to day 14 (model-estimated peak BAC = 0.057), then continued to decrease through day 90 (model-estimated mean peak BAC = 0.038, p < .001). Sensitivity analyses indicated that significant reductions in BAC would still be observed over the 90-day treatment period even if days without BAC data were assumed to be heavy drinking days, abstinence days, or a mixture of heavy drinking and abstinence days (see eSupplement Figures S1-S11). Additional models indicated that patients with “low” data availability (i.e., patients with 41 or fewer days with BAC data) had slightly smaller reductions in daily estimated peak BAC compared to patients with “medium” or “high” data availability (i.e., patients with 42–72 or 73–90 days with BAC data); however, overall there were large and significant reductions in daily estimated peak BAC observed for each of the “low,” “medium,” and “high” data availability subgroups (see eSupplement Table S3 and Figure S12).
Figure 1. Trajectory of daily estimated peak BAC among patients with 90-day treatment retention.
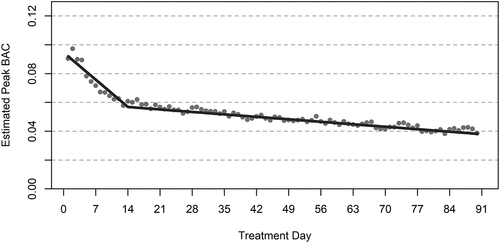
Table 2. Growth curve model results showing significant reductions in daily estimated peak BAC over the 90 day observation period.
Changes in BAC by gender and drinking goal
Moderation analyses indicated that the trajectories of daily estimated peak BAC did not significantly differ for men and women (see and ) but differed slightly for patients with different treatment goals (see and ). Compared to patients with an abstinence goal, estimated peak BAC was, on average, 0.018 percentage points higher for patients with a controlled drinking goal and 0.010 percentage points higher for patients with unknown drinking goal throughout the full 90-day observation period. However, there were no differences in the rates of change in BAC over time (i.e., difference in slopes) for patients with different drinking goals – that is, on average, patients with different drinking goals experienced similar magnitudes of reduction in daily estimated peak BAC over time.
Figure 2. Trajectories of daily estimated peak BAC by gender among patients with 90-day treatment retention.
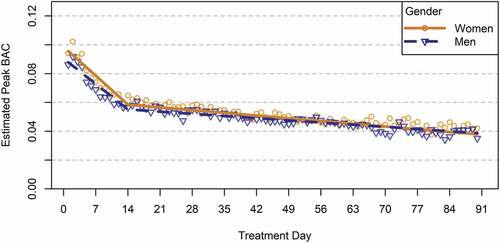
Figure 3. Trajectories of daily estimated peak BAC by treatment goal among patients with 90-day treatment retention.
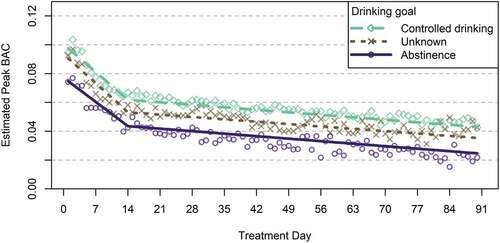
Table 3. Growth curve model results showing that changes in BAC were not significantly moderated by gender.
Table 4. Growth curve model results showing that changes in BAC were not significantly moderated by drinking goal.
Discussion
The need for social distancing during the COVID-19 pandemic and changes in policies for telehealth reimbursement have led to drastic increases in the utilization of telehealth services, particularly for mental health and addiction-related concerns (Citation22,Citation23). During the first 10 months of the pandemic (March-December 2020), it was estimated that approximately half of all AUD treatment sessions among commercially insured adults in the US were conducted via telehealth (Citation24), and patients have reported high satisfaction with receiving addiction treatment services via telehealth during the pandemic (Citation25). It is likely that telehealth will continue to be a viable approach to offering AUD treatment that many patients will prefer even after the COVID-19 public health emergency has ended.
Telehealth provides many advantages over traditional treatment modalities, including fewer barriers to accessing care and being widely available to individuals who have access to internet and/or smartphone technologies (Citation25,Citation26). The program studied here used breathalyzers connected to smartphones by Bluetooth, but many aspects of the program did not require smartphones (e.g., some encounters could be completed using cellular or landline phones), and it is possible that telehealth modalities that do not require high-speed internet (which is often less available in rural areas) or smartphones can be used to engage patients and help them reduce their drinking.
Results of the current study suggest that a fully remote telehealth program may be a viable means of helping patients access medical care, coaching, and medications for AUD. Many patients remained engaged in telehealth for 90 days or longer and those with 90-day retention had significant and large reductions in estimated daily peak BAC, on average. These outcomes suggest that telehealth may be a viable approach for engaging patients in AUD treatment and helping them reduce their alcohol consumption. These findings are promising when considering that telehealth modalities may also help overcome limitations associated with traditional in-person treatment, including barriers that prevent individuals from accessing treatment altogether (e.g., limited geographic availability, travel burden, stigma) and barriers that prevent patients from receiving evidence-based treatments even when they enter treatment (e.g., limited use of evidence-based medications for AUD in many settings) (Citation25,Citation26).
Results of the current study also suggest that telehealth can benefit women and people with controlled drinking goals. Although women are generally less likely to receive AUD treatment than men due to stigma and other structural barriers (Citation9,Citation10,Citation27), more than half of the patients in the current sample were women, and women attained similar reductions in daily estimated peak BAC as men. Likewise, controlled drinking was the most commonly endorsed treatment goal, and patients with controlled drinking goals attained similar reductions in daily estimated peak BAC compared to patients with abstinence goals or unknown drinking goals.
Limitations and strengths
The current study has important limitations. Notably, it was an observational study without randomization or a control group; therefore, we could not test the efficacy of the treatment program against a control condition. Additionally, the data were originally collected for clinical care (rather than for research) which resulted in missing data for BAC, gender, and drinking goal for some patients. BAC data were increasingly unavailable as patients got further into treatment, and the treatment procedures did not include steps to verify that BAC readings were submitted by identified patients. Because BAC data collection was contingent on being in treatment, we could not validly estimate BAC trajectories for patients without 90-day treatment retention. We did not have access to structured measures of race, geographical location, health insurance, education, employment or other comorbidities for the current research. All patients had to have access to technology required for telehealth, and therefore results may not generalize to other patients without such access; notably, however, smartphone ownership in the United States continues to rise with 85% of US adults owning smartphones as of February 2021 (Citation28).
The current study also has noteworthy strengths. The large cohort allowed for finer precision in our analyses and allowed us to evaluate differences across patient subgroups. The use of existing data collected for clinical care (rather than research) allowed us to include people who may not be interested in participating in alcohol research or who may not be able to complete research assessment batteries, increasing generalizability of the findings. The trajectory analyses utilized objectively measured BAC, and a series of sensitivity analyses indicated that reductions in BAC were likely robust across different scenarios of potential drinking outcomes on days without BAC data.
Conclusion
Telehealth can help address barriers to AUD treatment by offering services that are perceived as more private, flexible, and geographically available. In this large observational study, approximately half of patients who participated in a telehealth program offering medical and coaching services for AUD were retained in treatment for at least 90 days. Nearly all patients with 90-retention received a medication that can be used to treat AUD, and on average, patients with 90-day treatment retention achieved substantial reductions in their drinking based on an objective BAC measure. Telehealth may help facilitate similar magnitudes of BAC reduction for men and women and for patients with goals of abstinence and controlled drinking. Telehealth can be an engaging approach to delivering evidence-based AUD treatments – including medications for AUD and encounters with addiction medicine providers and recovery coaches – and helping patients with AUD achieve meaningful reductions in alcohol use.
Disclosure statement
Kevin A. Hallgren declares no conflicts of interest.
Gantt P. Galloway is employed by Ria Health and has been granted equity in Ria Health.
Katie Witkiewitz is a member Alcohol Clinical Trials Initiative (ACTIVE) Workgroup, which in the past 36 months has had activities that were supported by Alkermes, Dicerna, Ethypharm, Lundbeck, Mitsubishi, and Otsuka.
Paul Linde is employed by Ria Health and has been granted equity in Ria Health.
Bob Nix is employed by Ria Health and has been granted equity in Ria Health.
John E. Mendelson is employed by Ria Health and has been granted equity in Ria Health.
Additional information
Funding
References
- Grant BF, Goldstein RB, Saha TD, Chou SP, Jung J, Zhang H, Pickering RP, Ruan WJ, Smith, SM, Huang B, Hasin DS. Epidemiology of DSM-5 Alcohol Use Disorder: results from the National Epidemiologic Survey on Alcohol and Related Conditions III. JAMA Psychiatry. 2015;72:757–66.
- Mekonen T, Chan GCK, Connor J, Hall W, Hides L, Leung J. Treatment rates for alcohol use disorders: a systematic review and meta-analysis. Addiction. 2021;116:2617–34. doi:10.1111/add.15357.
- Saunders SM, Zygowicz KM, D’angelo BR. Person-related and treatment-related barriers to alcohol treatment. J Subst Abuse Treat. 2006;30:261–70. doi:10.1016/j.jsat.2006.01.003.
- Cunningham JA, Sobell LC, Sobell MB, Agrawal S, Toneatto T. Barriers to treatment: why alcohol and drug abusers delay or never seek treatment. Addict Behav. 1993;18:347–53. doi:10.1016/0306-4603(93)90036-9.
- Abraham AJ, Yarbrough CR. Availability of medications for the treatment of alcohol use disorder in U.S. counties, 2016–2019. J Stud Alcohol Drugs. 2021;82:689–99. doi:10.15288/jsad.2021.82.689.
- Kranzler HR, Soyka M. Diagnosis and pharmacotherapy of alcohol use disorder: a review. JAMA. 2018;320:815–24. doi:10.1001/jama.2018.11406.
- Substance Abuse and Mental Health Services Administration and National Institute on Alcohol Abuse and Alcoholism. Medication for the treatment of alcohol use disorder: a brief guide. 2015 [accessed 2022 Sept 27]. https://store.samhsa.gov/sites/default/files/d7/priv/sma15-4907.pdf
- National Institute on Alcohol Abuse and Alcoholism. Core Resource on Alcohol. 2022 [accessed 2022 Jun 3]. https://www.niaaa.nih.gov/health-professionals-communities/core-resource-on-alcohol
- McCaul M, Roach D, Hasin D, Weisner C, Chang G, Sinha R. Alcohol and women: a brief overview. Alcohol Clin Exp Res. 2019;43:774–79. doi:10.1111/acer.13985.
- Alvanzo AAH, Storr CL, Mojtabai R, Green KM, Pacek LR, La Flair LN, Cullen BA, Crum RM. Gender and race/ethnicity differences for initiation of alcohol-related service use among persons with alcohol dependence. Drug Alcohol Depend. 2014;140:48–55. doi:10.1016/j.drugalcdep.2014.03.010.
- Henssler J, Müller M, Carreira H, Bschor T, Heinz A, Baethge C. Controlled drinking—non-abstinent versus abstinent treatment goals in alcohol use disorder: a systematic review, meta-analysis and meta-regression. Addiction. 2021;116:1973–87. doi:10.1111/add.15329.
- Substance Abuse and Mental Health Services Administration. Key substance use and mental health indicators in the United States: results from the 2019 National Survey on drug use and health. 2020 [accessed 2022 Sept 27]. https://www.samhsa.gov/data/sites/default/files/reports/rpt29393/2019NSDUHFFRPDFWHTML/2019NSDUHFFR090120.htm.
- Kruse CS, Lee K, Watson JB, Lobo LG, Stoppelmoor AG, Oyibo SE. Measures of effectiveness, efficiency, and quality of telemedicine in the management of alcohol abuse, addiction, and rehabilitation: systematic review. J Med Internet Res. 2020;22:e13252. doi:10.2196/13252.
- Young LB. Telemedicine interventions for substance-use disorder: a literature review. J Telemed Telecare. 2012;18:47–53. doi:10.1258/jtt.2011.110608.
- Mitchell MM, Mendelson J, Gryczynski J, Carswell SB, Schwartz RP. A novel telehealth platform for alcohol use disorder treatment: preliminary evidence of reductions in drinking. Am J Drug Alcohol Abuse. 2020;46:297–303. doi:10.1080/00952990.2019.1658197.
- World Health Organization. ICD-10: international statistical classification of diseases and related health problems: tenth revision. World Health Organization; 2004 [accessed 2022 Dec 9]. https://apps.who.int/iris/handle/10665/42980
- Maskell PD, Korb A-S. Revised equations allowing the estimation of the uncertainty associated with the total body water version of the Widmark equation. J Forensic Sci. 2022;67:358–62. doi:10.1111/1556-4029.14859.
- Hallgren KA, Witkiewitz K. Missing data in alcohol clinical trials: a comparison of methods. Alcohol Clin Exp Res. 2013;37:2152–60. doi:10.1111/acer.12205.
- Hallgren KA, Witkiewitz K, Kranzler HR, Falk DE, Litten RZ, O’Malley SS, Anton RF. Missing data in alcohol clinical trials with binary outcomes. Alcohol Clin Exp Res. 2016;40:1548–57. doi:10.1111/acer.13106.
- Bates D, Mächler M, Bolker B, Walker, S. Fitting linear mixed-effects models using lme4. arXiv preprint arXiv:14065823. 2014.
- Kuznetsova A, Brockhoff PB, Christensen RH. lmerTest package: tests in linear mixed effects models. J Stat Softw. 2017;82:1–26. doi:10.18637/jss.v082.i13.
- Patel SY, Mehrotra A, Huskamp HA, Uscher-Pines L, Ganguli I, Barnett ML. Variation in telemedicine use and outpatient care during the COVID-19 pandemic in the United States: study examines variation in total US outpatient visits and telemedicine use across patient demographics, specialties, and conditions during the COVID-19 pandemic. Health Aff. 2021;40:349–58. doi:10.1377/hlthaff.2020.01786.
- Lo J, Amin K, Cox, C, Panchal, N, Miller, BF. Telehealth has played an outsized role meeting mental health needs during the COVID-19 pandemic. KFF; 2022, [accessed 2022 Sept 27]. https://www.kff.org/coronavirus-covid-19/issue-brief/telehealth-has-played-an-outsized-role-meeting-mental-health-needs-during-the-covid-19-pandemic/
- Busch AB, Mehrotra A, Greenfield SF, Uscher-Pines L, Rose S, Huskamp HA. A cohort study examining changes in treatment patterns for alcohol use disorder among commercially insured adults in the United States during the COVID-19 pandemic. J Subst Abuse Treat. 2023;144:108920. doi:10.1016/j.jsat.2022.108920.
- Sugarman DE, Busch AB, McHugh RK, Bogunovic OJ, Trinh CD, Weiss RD, Greenfield SF. Patients’ perceptions of telehealth services for outpatient treatment of substance use disorders during the COVID-19 pandemic. Am J Addict. 2021;30:445–52. doi:10.1111/ajad.13207.
- Davis CN, O’neill SE. Treatment of alcohol use problems among rural populations: a review of barriers and considerations for increasing access to quality care. Curr Addict Rep. Epub ahead of print 22 Nov 2022;9:432–44. doi:10.1007/s40429-022-00454-3.
- Greenfield SF, Brooks AJ, Gordon SM, Green CA, Kropp F, McHugh RK, Lincoln M, Hien D, Miele GM. Substance abuse treatment entry, retention, and outcome in women: a review of the literature. Drug Alcohol Depend. 2007;86:1–21. doi:10.1016/j.drugalcdep.2006.05.012.
- NW 1615 L. St, Washington S 800, Inquiries D 20036 U-419-4300 | M-419-4372 | M. Mobile Fact Sheet. Pew Research Center: Internet, Science & Tech; 2018 [accessed 2018 Sept 18]. http://www.pewinternet.org/fact-sheet/mobile/