ABSTRACT
Background: Neighborhood-, school-, and peer-contexts play an important role in adolescent alcohol use behaviors. Methodological advances permit simultaneous modeling of these contexts to understand their relative and joint importance. Few empirical studies include these contexts, and studies that do typically: examine each context separately; include contexts for the sole purpose of accounting for clustering in the data; or do not disaggregate by sex.
Objectives: This study takes an eco-epidemiologic approach to examine the role of socio-contextual contributions to variance in adolescent alcohol use. The primary parameters of interest are therefore variance rather than beta parameters (i.e. random rather than fixed effects). Sex-stratified models are also used to understand how each context may matter differently for male and female adolescents.
Method: Data come from the National Longitudinal Study of Adolescent to Adult Health (n = 8,534 females, n = 8,102 males). We conduct social network analysis and traditional and cross-classified multilevel models (CCMM) in the full and sex-disaggregated samples.
Results: In final CCMM, peer groups, schools, and neighborhoods contributed 10.5%, 10.8%, and 0.4%, respectively, to total variation in adolescent alcohol use. Results do not differ widely by gender.
Conclusions: Peer groups and schools emerge as more salient contributing contexts relative to neighborhoods in adolescent alcohol use for males and females. These findings have both methodological and practical implications. Multilevel modeling can model contexts simultaneously to prevent the overestimation of variance in youth alcohol use explained by each context. Primary prevention strategies addressing youth alcohol use should focus on schools and peer networks.
Introduction
Adolescence (ages 10–19) is second only to infancy in terms of the physical, biological, and neurocognitive changes that occur in the human body (Citation1). It is also a time in life when most substance use is first initiated (Citation2). Although alcohol use may have some positively perceived purposes among adolescents, its use may translate into serious risks (Citation2). For example, alcohol use in adolescence may interfere with normal brain development and increase the risk of developing alcohol use disorders later in life (Citation3). Understanding the factors that contribute to adolescent alcohol use aid in the prevention of negative outcomes in adolescence and across the lifecourse (Citation4).
The framing of factors that explain variation in health outcomes as originating purely at the individual level is in line with much of the traditional epidemiologic research (Citation5–7). This is despite the fact that individuals are embedded in social and geographic contexts and many health outcomes are linked to a number of causal factors that range from the individual-level (e.g., genetic makeup) to community-levels (e.g., peer groups and neighborhoods) (Citation8,Citation9). The application of an eco-epidemiologic theoretical framework that situates adolescents within the places they play, learn, and live demands an examination of both compositional and contextual effects (Citation7,Citation10). Compositional effects are characteristics of the individuals that collectively comprise a group or sub-population (e.g., average number of drinks in a month), while contextual effects reflect the nature of the contexts within which individuals socialize, study, and reside (e.g., number of alcohol outlets in a neighborhood) (Citation10).
Empirical evidence supports the idea that variation in adolescent behaviors can be attributed to multilevel factors, beyond, and yet simultaneously inclusive of, individual traits and behaviors. Specific to the adolescent alcohol literature, contextual effects have been shown to influence adolescent alcohol use behaviors through: (a) peers (see, e.g., Citation11,Citation12); (b) schools (see, e.g., Citation13–15); and (c) neighborhoods (see, e.g., Citation16–19).
Despite a recognition of the importance that these multiple contexts play in adolescent alcohol use, there remains a limited body of empirical evidence examining contextual contributions to variation in alcohol use outcomes. Rather than treating clustering within the data as a nuisance or something to control for in statistical models, clustering is of substantive interest in its own right and allows investigators the ability to understand the extent to which variation in outcome measures may be explained by peers, schools, and neighborhoods. Studies that do include an examination of variance parameters for substantive reasons typically examine the contexts in which adolescents socialize, learn, and reside in isolation rather than modeling all three together; to the authors’ knowledge, the simultaneous consideration of peer groups, schools, and neighborhoods on adolescent alcohol use has not been conducted. This leaves gaps in our understanding of the relative and joint importance of these contexts in explaining variation in adolescent alcohol use. Failing to analyze these contexts simultaneously may lead to omitted context bias, or the false attribution of the variance associated with the omitted context(s) to contexts included in a multilevel model (Citation20). It may also lead to the misinterpretation of the optimal contexts that must be targeted for prevention efforts. Finally, although males and females initiate alcohol use in adolescence at comparable rates (Citation21), in comparison to adult females, adult males drink alcohol more often, drink greater amounts, and are more likely to engage in binge drinking (Citation22,Citation23). Understanding whether variation in adolescent alcohol use at each contextual-level differs by sex may improve understanding of the causes underlying sex disparities in adult alcohol use.
To respond to these gaps, we conduct analyses to fulfill both methodological and substantive study aims. The first is to provide a methodological examination using both traditional multilevel models (MLM) and their extension, cross-classified multilevel models (CCMM). Cross-classified multilevel models permit the simultaneous analysis of non-hierarchically nested multilevel data (Citation24), a type of data structure commonly found in social and community settings, wherein individuals do not perfectly nest within increasingly broad social groupings. For example, as opposed to a nested hierarchy where all adolescents from the same two neighborhoods would go to one school, in a cross-classified data structure, adolescents who attend the same school may not all reside within the same neighborhood and those from the same neighborhood may not all attend the same school. The use of MLM and CCMM affords the opportunity to examine the extent to which peer group, school, and neighborhood contexts contribute to the variance in alcohol use during adolescence when they are modeled in isolation of the other contexts as well as when they are modeled simultaneously in CCMM. This focus on contributions to variance, or the models’ random effects – rather than to beta coefficients, or the models’ fixed effects – has its origins in multilevel eco-epidemiologic approaches that seek to understand the determinants of individual heterogeneity, rather than to identify probabilistic risk factors (Citation20,Citation25). The results stemming from this methodological contribution provides an opportunity to also empirically evaluate the individual and joint contributions these contexts make in adolescent alcohol use. The substantive aim of this work is thus to aid in the disentanglement of the overlapping influences of these contexts, thereby increasing precision and clarity in our assessment of which of these contexts matter relative to the others in the prevention of adolescent alcohol use.
Methods
Given the methodological focus of this study, we conduct analysis using data from the National Longitudinal Study of Adolescent to Adult Health (Add Health), a longitudinal cohort study of adolescents in grades 7 through 12 in Wave I (1994–1995), recruited from a nationally representative sample of schools in the United States (Citation26). This dataset is one of the few nationally representative datasets available in the world that enables researchers to study the joint contributions of peer social networks, schools and neighborhoods alongside adolescent health outcomes, and therefore the only dataset that will allow us to examine our research aims. The primary sampling frame for Add Health was derived from the Quality Education Database (QED) and was used to select a stratified sample of 80 high schools and 52 middle schools representative of US schools with respect to region of country, urbanicity, school size, type (public, private, parochial), and ethnic mix (Citation27).
In Wave I, in-school questionnaires were administered to 90,118 students to assess school context and activities, school-based peer friendship networks, and a limited set of individual characteristics such as certain health conditions and risk behaviors (Citation28). The peer network data from the in-school questionnaires enable researchers the ability to construct a fairly complete web of social relationships, known as a sociocentric social network graph (Citation28). In contrast to egocentric networks, which provide a narrower perspective of only the contacts of individual respondents (or “egos”) (Citation29), sociocentric studies permit calculation of the entire network structure, allowing for the study of the mechanisms through which social networks may affect health-related attitudes, behaviors, and outcomes (Citation30). This method represents a significant improvement in the way youth friendships are traditionally modeled (Citation31).
Add Health also conducted a more extensive at-home follow-up survey in Wave I with a random sub-sample of students, stratified by grade and sex (n = 20,745) (Citation27). This sub-sample (or “core sample”) was followed longitudinally, and it is from this sub-sample that data on neighborhoods are available. Given the peer network data come from the in-school sample and the neighborhood data come from the at-home or core sample, we first use in-school Wave I data to conduct the social network analysis to develop the socio-centric network graphs. We subsequently conduct the primary analyses (multilevel models) using data from adolescents who participated in both the in-school and in-home survey, and who had complete information on the outcome, contextual predictors, and covariates. This resulted in a final analytic sample of n = 16,636, with missingness on alcohol use (n = 248), race (n = 10), parental education (n = 274), age (n = 15), sex (n = 2), region (n = 378), school (n = 407), neighborhood (n = 198), and peer group (n = 3,549). Given the centrality of peer networks to the present study, respondents who did not report having friends, who refused to nominate friends, or who nominated themselves as a friend were not able to be assigned a peer group and could not therefore be included in analyses examining the role of peer groups. As shown in Supplemental Table A, respondents who were not assigned a peer group (i.e., “isolates”) do not differ in alcohol use or demographic characteristics as compared to adolescents who did have a peer group.
The parameters of interest in this research are the contexts’ contributions to the overall variance of the outcome (as opposed to any single predictor’s beta coefficient). This is because the overall research aim is to understand the influence of neighborhood, school, and peer contexts on adolescent alcohol use. As such, the primary statistics of concern are the models’ random effects and are presented in both the total variance explained via Variance Partition Coefficients (VPCs) and Median Odds Ratios (MORs). VPCs measure the proportion of total variance in the outcome that is attributable to the area or contextual-level (i.e., the correlation between two observations within the same cluster) (Citation32,Citation33). The MORs translate area-level variance into the widely-used odds ratio scale, and is provided due to this scale’s consistent and intuitive interpretation. The MOR is defined as the median value of the odds ratio between the context at highest risk and the context at lowest risk of adolescent alcohol use when randomly picking out two areas (Citation34), and shows the extent to which the variation in individual probability of adolescent alcohol use is explained by context (i.e., peer group, school, and neighborhood).
Measures
Outcome: adolescent alcohol use
Adolescents reported whether they had ever had a drink of beer, wine, or liquor – not just a sip or taste of someone else’s drink – more than 2 or 3 times in their lifetime. Respondents who answered yes to this question were subsequently asked how often they drank alcohol in the past year: “During the past 12 months, on how many days did you drink alcohol?” Categorial response options included: 0 = never, 1 = 1–2 days in the past 12 months, 2 = once a month or less (3–12 times in the past 12 months), 3 = 2–3 days a month, 4 = 1–2 days a week, 5 = 3–5 days a week, and 6 = almost every day. Given this count variable was over-dispersed and zero-inflated Poisson models did not converge in the cross-classified multilevel model using Markov Chain Monte Carlo estimation procedures, the outcome was operationalized in final analyses as a binary measure of past year alcohol use: (0) “No alcohol use,” those who did not drink alcohol in the past year (including those who self-reported never use); and (1) “Alcohol use,” those who drank alcohol 1–2 days or more in the past year.
Exposure variables
This study is concerned with the eco-epidemiologic examination of socio-contextual contributions to variance in adolescent alcohol use. As such, the primary exposures of interest are modeled not as fixed effects, but rather as random effects and their contribution to the total variance in our outcome measure. The following three contexts are included as contextual levels in MLM and CCMM:
Neighborhood
The Add Health data provide several possible definitions of neighborhood – census block, census tract, and county. In line with previous research on neighborhoods (Citation20,Citation35–37), we choose to model the neighborhood context using census tract identifiers.
School
Schools were modeled using the unique identification numbers for each of the 80 high schools and 52 middle schools are provided in the Add Health sample.
Peer group
Finally, the peer group context was modeled using unique social network community identifiers as specified via an algorithm applied to data from respondents’ nominations of up to five of their best male and five best female friends from the school roster (using ID codes specific to each student). Although the concept of peer groups is familiar, their quantitative identification is a complex task, and precise formulas that partition sociocentric network graphs into smaller social network communities or peer groups are known to be computationally intractable (Citation38–40). As such, numerous algorithmic heuristics have been devised to partition the larger network graphs into smaller network communities with densely connected nodes, and only sparse connections to nodes of other communities (Citation39,Citation40). Given that network analysis may be conducted on myriad types of networks – from social networks, to citations of scientific papers, to webpage networks on the internet – the type of algorithm chosen depends in part on the research aims and discipline of the investigator. This study builds upon previous social epidemiologic research with adolescent school-based social networks using Add Health data by Evans et al. (2016) (Citation20). This prior research applied two different network community detection algorithms to the Add Health data—k-clique percolation (Citation40) and modularity maximization (Citation41) to examine which algorithm would be best-suited for the social network data in Add Health. The study demonstrated that while overall findings were robust to the algorithm, the modularity maximization algorithm resulted in network communities with smaller variation in size and was chosen for primary analyses (Citation20). Further, the modularity maximization algorithm is computationally faster than other algorithms (Citation41) and the modularity (i.e., the density of links inside communities as compared to links between communities) achieved is robust to overall network size, making it a versatile algorithm for use on various sizes of social networks (Citation41). Given these characteristics and the algorithm’s use in previous research with the Add Health data, the modularity maximization algorithm is used in the present analysis for the quantitative identification of adolescent peer groups.
Socio-demographics
Adjusted models include the following individual-level covariates: age, self-reported race/ethnicity (Hispanic, White, Black or African American, Asian or Pacific Islander, American Indian or Native American, and “Other”), and socio-economic status, as measured by parental education level. U.S. region (West, Midwest, South, and Northeast) is also included as a covariate given variation in drinking by state and US region (Citation42). Given the importance of sex as a known social stratifier in American society, and enduring sex disparities in US population patterning of alcohol use (Citation43), sex is included as a stratifying variable in models.
Analysis
Models
Each student in Add Health belongs to three contexts or higher-level units: one neighborhood, one school, and one social network community, and these contexts are non-hierarchically structured. For example, students from neighborhood A may attend different schools; students from school B may reside in different neighborhoods; and adolescents in the same social network community may attend different schools and reside in different neighborhoods. Given the nonhierarchical nature of these multiple contexts, cross-classified multilevel modeling is best suited for these analyses. To test the difference between traditional MLM and CCMM, each context is first specified individually in MLM. Next, two contexts are modeled together in two-way CCMM, followed by a null three-way random-intercepts logit CCMM is specified. The final set of models incorporate relevant covariates and sex-stratified results.
Social network community detection
As mentioned above, in order to quantitatively identify adolescent peer groups, we use the modularity maximization algorithm (Citation41) for social network community (or peer group) detection. As the name implies, the algorithm attempts to maximize modularity, or the density of ties (i.e., links or edges) inside communities as compared to ties between communities, calculated by the number of edges falling within groups minus the expected number in an equivalent network with edges placed at random (Citation44). Given the Wave I in-school instrument used a saturated sample design, with nearly all adolescents in the school interviewed, the resulting near-complete network data provides a strong basis for confident evaluation of social network community effects.
Initial social network analysis is performed in Python 3.6 (Citation45) using the NetworkX package (Citation46). Python 3.6 is used for subsequent network community detection using the community_multilevel algorithm from the IGraph library (Citation47).
Multilevel analyses were conducted in MLwiN version 3.00 (Citation48) via Stata version 13.1 (Citation49) using the runmlwin program (Citation50). CCMM were fit with Bayesian Markov Chain Monte Carlo (MCMC) estimation procedures (Citation51) using the Metropolis-Hastings algorithm. CCMM were first fit using second order penalized quasi-likelihood methods (PQL2) and Iterative Generalized Least Squares (IGLS) estimation to provide the Bayesian MCMC procedure with initialization values. Non-informative priors and burn-in of 500 iterations and monitoring chain of 5,000 iterations were used in all analyses. A complete case analysis is conducted, with a final analytic sample of n = 16,636 adolescents.
Results
The multilevel structure of the data is summarized in . Adolescents in this analytic sample come from 2,112 neighborhoods, 125 schools, and 870 peer groups. On average, there are 19 adolescents in each peer group. Characteristics of the adolescents in the sample are provided in . As shown, the average age of respondents was 16.1 years old. Slightly more than half of the sample was female (51.3% female, 48.7% male), with a majority of adolescents self-reporting White racial identity. Just under one half (47.8%) of respondents reported using alcohol in the 12 months preceding the survey. When disaggregated by age, however, we see variation in the proportion of adolescents who used alcohol in the past year. As shown in , the proportion of adolescents drinking alcohol steadily increased by age. examines adolescent alcohol use by sex and age, illustrating similar alcohol use trajectories by age between the sexes, and a slightly greater proportion of females than males in this sample reporting alcohol use at ages 12–16 and more males than females reporting alcohol use in the past year at ages 17 and 18 years (with statistically significant differences between sexes seen only at ages 14 and 18).
Figure 1. Past year alcohol use increases incrementally during adolescents (12 to 18 years).a.
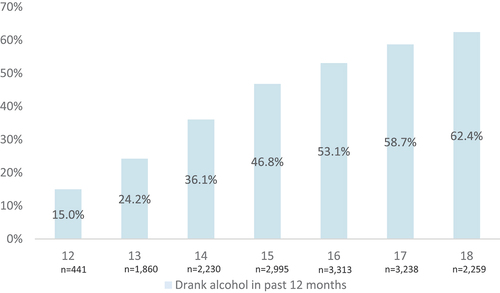
Figure 2. Past year alcohol use by sex, showing similar trajectories by age between male and female adolescents.a.
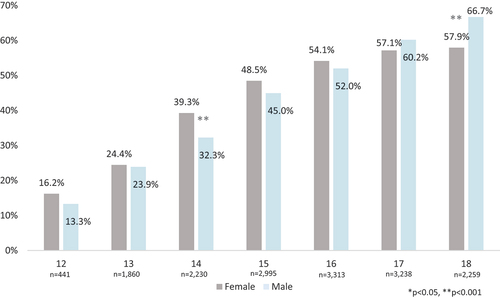
Table 1. Multilevel data structure of Add Health: adolescents situated within peer groups, schools, and neighborhoods.
Table 2. Adolescent characteristics.
provides results for the null MLM (Models 1–3) as well as null CCMM (Models 4–7). Statistics for both Variance Partition Coefficients (VPCs) and Median Odds Ratios (MORs) are presented. VPCs measure the proportion of total variance in the outcome that is attributable to the area or contextual-level (i.e., the correlation between two observations within the same cluster) (Citation33). The MOR shows the extent to which the variation in individual probability of adolescent alcohol use is explained by context (i.e., peer group, school, and neighborhood) and can be conceptualized as the increased risk (in median) that an individual would have if switching peer groups or moving to another school or neighborhood with a higher risk (Citation32). If the MOR is equal to one, this indicates no differences between contexts.
Table 3. Adolescent alcohol use. Random effects results from null two-level hierarchical multilevel models (MLM) (Models 1–3); Null two-way (Models 4–6) and three-way (Model 7) cross-classified multilevel models (CCMM).
In traditional hierarchical two-level null multilevel models (Models 1–3), we find that all three contexts contribute to the total variance in likelihood of adolescent alcohol use, with network contributing 17.2%, school 12.5%, and neighborhood contributing 5.6% to the total variation in adolescent alcohol use when each context is modeled separately. The MOR for peer groups is 2.20, which shows that in the median case the residual heterogeneity between peer groups increased individual odds of alcohol use by 2.20 times when randomly selecting two adolescents in different peer groups. This is, if an adolescent moved into a peer group with a higher probability of alcohol use, their risk of alcohol use would (in median) increase 2.20 times (95% CrI: 2.07, 2.34). This is compared to MORs of 1.92 for schools and 1.53 for neighborhoods in the null MLM (Models 1–3).
Models 4, 5 and 6 examine the cross-classified contexts two at a time using CCMM. As shown in , when a second context is added to the first, the contexts’ MORs decrease but remain statistically significant. For example, when modeled alone the MOR for peer group (Model 1) was 2.20, but dropped to 1.80 when modeled with the school context (Model 4) and to 2.19 when modeled with the neighborhood context (Model 5). Model 7 examines all three contexts simultaneously. This results in further changes to the MORs for all three of the contexts as compared to both the MLM and the two-level CCMM, however all MORs remain statistically significant. The MOR for peer groups in the unadjusted three-way CCMM (Model 7) is 1.81 (95% CrI: 1.70, 1.92), for schools it is 1.82 (95% CrI: 1.65, 2.03), and for neighborhoods it is 1.11 (95% CrI: 1.04, 1.19).
After adjusting for relevant individual-level covariates (sex, age, race/ethnicity, parental education, and US region), we see in Model 8 () that the MORs for peer group, school, and neighborhood drop slightly to 1.54, 1.40, and 1.15, respectively. Each contexts’ MOR remains statistically significant. When disaggregated by sex (Models 9 and 10), MORs remain largely the same: peer group MORs are 1.51 for males and 1.59 for females; school MORs are 1.38 for males and 1.42 for females; and neighborhood MORs are 1.08 for males and 1.13 for females. This indicates that sex does not modify the associations between adolescent alcohol use and the variance explained by peer group, school, and neighborhood contexts.
Table 4. Adolescent alcohol use. Random effects results from final, fully adjusted three-way cross-classified multilevel model (CCMM), with the full sample (Model 8) a, and sex-disaggregated models (males: Model 9; females; Model 10).b.
Discussion
Findings from this study demonstrate that peer group, school, and neighborhood contexts each contribute to variation in adolescent alcohol use outcomes among adolescents in middle school and high school. Importantly, however, this study models these contexts in a joint statistical model, and for the first time provides a simultaneous examination of the three contexts’ contributions to variation in adolescent alcohol use. Results indicate that, when considered alongside school and neighborhood influences, peer groups remain the most salient context in explaining adolescent alcohol use in this US school-based sample. These findings provide strong evidence in support of prior research that asserts social context, especially that of peer groups, is an especially important context in shaping the likelihood of onset of adolescent alcohol use (Citation52,Citation53).
Findings also highlight the advantage of applying eco-epidemiologic frameworks to the etiology of adolescent alcohol use, as permitted via the use of more advanced analytic methodologies such as cross-classified multilevel modeling and social network analysis. Results demonstrate the methodological importance of modeling all available contexts within a dataset simultaneously in order to avoid omitted context bias, or the error made in falsely attributing the variance to one or more area-level or community contexts included in a model when it should in fact be attributed to a context not factored into a model (Citation20). Results from the three-way CCMM document that contextual effects of peer groups, schools, and neighborhoods are inter-related and may thus yield overestimated effects on the outcome of interest if modeled in isolation. Had any one of the contexts not been included in the multilevel models, the MOR for the included context(s) would have been overestimated. For example, when modeled in isolation, the MOR for peer group was 2.20, but this parameter fell roughly 18% (to 1.81) when modeled jointly with schools and neighborhoods.
Results from sex-stratified models did not indicate large differences in MORs between males and females. This is seemingly in conflict with previous research that demonstrates the importance of adolescent sex and sex composition of their social network as a determinant of adolescents’ substance use behaviors (Citation54). However, study findings in fact highlight the importance in distinguishing between compositional and contextual-level effects in our understanding of the etiology of health behaviors. Our findings indicate that peer group-, school-, and neighborhood-contexts do indeed contribute to variation in adolescent alcohol use, and that they contribute in roughly similar proportions to variance in the outcomes of male and female alcohol use (i.e., they have similar contextual effects by sex). The mechanisms of action at play within the contexts may, however, vary by sex. Indeed, scholars have proposed numerous explanations for the mechanisms through which sex may modify social interactions within peer groups (Citation54). For example, male peer groups may more strongly enforce gender norms related to binge drinking as compared to female peer groups. Empirical work examining the role of gender on alcohol socialization suggests the mechanisms are highly complex and this is a promising and necessary area for additional research (Citation55).
Study findings also demonstrate the importance of multilevel and multi-context approaches of alcohol risk prevention for adolescents, particularly through engagement of peer networks and schools. These findings correspond with reviews of alcohol risk prevention efforts with adolescents, which demonstrate the value of peer and school-based interventions (Citation56,Citation57), and support potential integration of these approaches into programming designed to address adolescent alcohol initiation. While beyond the scope of the present study, a direction for future research is to determine whether the contribution of these contexts may differ as youth progress from onset of or initial alcohol use to binge drinking, the extent to which the contextual influences vary over the course of adolescence as prevalence of alcohol involvement becomes more common (i.e., with age), whether certain network characteristics (e.g., degree centrality and properties of nodes) may differentially impact outcomes, and how drinking behaviors in adolescence differ between social isolates (i.e., adolescents who do not report having school-based friends) and those with more robust peer group connections. Another avenue for further research is to include contextual-level predictors within CCMM to examine the extent to which certain characteristics of the contexts may explain the variance at these contextual levels. Any included predictor variable in statistical models will of course explain a proportion of the variance seen at each level in the CCMM, but subsequent research is needed to examine what variables explain the most variation. This study lays the foundation for this subsequent work in the examination of contextual-level predictors by demonstrating that there is a need for investigators to take these contexts – and their characteristics – into consideration in future data collection efforts and statistical analysis.
Limitations of the current study include the use of cross-sectional data that precludes assumptions of causality as well as reliance solely on adolescents’ self-report of alcohol use, which may be subject to social desirability and recall biases. The Add Health Wave 1 data, while necessary for our methodological investigation, are quite dated and thus caution is made regarding generalizability beyond this time period in the US (mid-1990s). Further considerations of the results in relation to the age of the data include the fact that adolescent alcohol use has declined substantially in the US since the 1990s, from 50.8% of 9th through 12th grade students reporting alcohol use in 1991 to 29.2% in 2019 (Citation58). Despite these decreases, it is likely that the influence of in-person peer group, school, and neighborhood contexts continue to play a role in variation in adolescent alcohol use, though more recent data are needed to validate this assumption. A further need is new nationally representative data that combines in-person peer groups with online, social media-based. Given that adolescent socialization occurs both in-person and virtually, and that alcohol-related media content is related to alcohol consumption, new and more holistic peer network data are required to better understand how to tailor interventions related to social influence among adolescents (Citation59).
Conclusion
The contributions of this research are both methodological and substantive. Study findings offer important support in how to advance alcohol epidemiologic methods by employing CCMM to examine multilevel causal frameworks when examining health risks for adolescents. Findings also lend support in highlighting the value of multilevel interventions for the prevention of alcohol use and misuse among US adolescents.
Disclosure statement
No potential conflict of interest was reported by the authors.
Additional information
Funding
References
- Steinberg L, Dahl R, Keating D, Kupfer DJ, Masten AS, Pine DS. Psychopathology in adolescence: Integrating affective neuroscience with the study of context. In: D. Cicchetti, D. Cohen, editors. Developmental psychopathology. Vol. 2. 2nd ed. New York: Wiley; 2006.
- Schulenberg J, Maslowsky J, Patrick ME, Martz M. Substance use in the context of adolescent development. In: Zucker RA, Brown SA, editors. The Oxford handbook of adolescent substance abuse. New York: Oxford University Press; 2019.
- Crews FT, Vetreno RP, Broadwater MA, Robinson DL, Morrow LA. Adolescent alcohol exposure persistently impacts adult neurobiology and behavior. Pharmacol Rev. 2016;68:1074–109. doi:10.1124/pr.115.012138.
- Duncan SC, Alpert A, Duncan TE, Hops H. Adolescent alcohol use development and young adult outcomes. Drug Alcohol Depen. 1997;49:39–48. doi:10.1016/S0376-8716(97)00137-3.
- Diez-Roux A. Bringing context back into epidemiology: variables and fallacies in multilevel analysis. Am J Public Health. 1998;88:216–22. doi:10.2105/AJPH.88.2.216.
- Lukes S. Sociological theory and philosophical analysis. Palgrave Macmillan: London; 1970. p. 76–88. doi:10.1007/978-1-349-15388-6_5.
- Susser M, Susser E. Choosing a future for epidemiology. 1996. Am J Public Health. 2015;105:1313–15.
- Berkman LF, Kawachi I, Glymour M. Social epidemiology. 2nd ed. Oxford University Press: New York; 2014. doi:10.1093/med/9780195377903.001.0001.
- Krieger N. Epidemiology and the people’s health: theory and context. New York: Oxford University Press; 2011.
- Diez-Roux A. A glossary for multilevel analysis. J Epidemiol Commun H. 2002;56:588–94. doi:10.1136/jech.56.8.588.
- Cox MJ, DiBello AM, Meisel MK, Ott MQ, Kenney SR, Clark MA, Barnett NP. Do misperceptions of peer drinking influence personal drinking behavior? Results from a complete social network of first-year college students. Psychol Addict Behav. 2019;33:297–303. doi:10.1037/adb0000455.
- Jose R, Hipp JR, Butts CT, Wang C, Lakon CM, Van Horn L. A multi-contextual examination of non-school friendships and their impact on adolescent deviance and alcohol use. PLos One. 2021;16:e0245837–e0245837. doi:10.1371/journal.pone.0245837.
- Sudhinaraset M, Wigglesworth C, Takeuchi DT. Social and cultural contexts of alcohol use: influences in a social-ecological framework. Alcohol Res. 2016;38:35–45.
- Tomczyk S, Isensee B, Hanewinkel R. Moderation, mediation - or even both? School climate and the association between peer and adolescent alcohol use. Addict Behav. 2015;51:120–26. doi:10.1016/j.addbeh.2015.07.026.
- Burdzovic Andreas J, Jackson KM. Adolescent alcohol use before and after the high school transition. Alcohol Clin Exp Res. 2015;39:1034–41. doi:10.1111/acer.12730.
- Slutske WS, Deutsch AR, Piasecki TM. Neighborhood contextual factors, alcohol use, and alcohol problems in the United States: evidence from a nationally-representative study of young adults. Alcohol Clin Exp Res. 2016;40:1010–19. doi:10.1111/acer.13033.
- Bendtsen P, Damsgaard MT, Tolstrup JS, Ersbøll AK, Holstein BE. Adolescent alcohol use reflects community-level alcohol consumption irrespective of parental drinking. J Adolesc Health. 2013;53:368–73. doi:10.1016/j.jadohealth.2013.04.021.
- Fairman BJ, Goldstein RB, Simons-Morton BG, Haynie DL, Liu D, Hingson RW, Gilman SE. Neighbourhood context and binge drinking from adolescence into early adulthood in a US national cohort. Int J Epidemiol. 2020;49:103–12. doi:10.1093/ije/dyz133.
- Joshi S, Schmidt NM, Thyden NH, Glymour MM, Nelson TF, Haynes D, Osypuk TL. Do alcohol outlets mediate the effects of the moving to opportunity experiment on adolescent excessive drinking? A secondary analysis of a randomized controlled trial. Subst Use Misuse. 2022;57:1788–96. doi:10.1080/10826084.2022.2115847.
- Evans CR, Onnela JP, Williams DR, Subramanian SV. Multiple contexts and adolescent body mass index: schools, neighborhoods, and social networks. Social Sci Med. 2016;162:21–31. doi:10.1016/j.socscimed.2016.06.002.
- Kuhn C. Emergence of sex differences in the development of substance use and abuse during adolescence. Pharmacology & Therapeutics. 2015;153:55–78. doi:10.1016/j.pharmthera.2015.06.003.
- Courtenay WH. Dying to be men: pyschosocial, environmental, and biobehavioral directions in promoting the health of men and boys. New York: Routledge; 2011.
- Pinkhasov RM, Wong J, Kashanian J, Lee M, Samadi DB, Pinkhasov MM, Shabsigh R. Are men shortchanged on health? Perspective on health care utilization and health risk behavior in men and women in the United States. Int J Clin Pract. 2010;64:475–87. doi:10.1111/j.1742-1241.2009.02290.x.
- Barker KM, Dunn EC, Richmond TK, Ahmed S, Hawrilenko M, Evans CR. Cross-classified multilevel models (CCMM) in health research: a systematic review of published empirical studies. Soc Sci Med Popul Health. 2020;12:100661. doi:10.1016/j.ssmph.2020.100661.
- Merlo J. Invited commentary: multilevel analysis of individual heterogeneity—A fundamental critique of the current probabilistic risk factor epidemiology. Am J Epidemiol. 2014;180:208–12. doi:10.1093/aje/kwu108.
- Harris KM, Halpern CT, Whitsel E, Hussey J, Tabor J, Entzel P, Udry JR. The national longitudinal study of adolescent health: research design. 2009. http://www.cpc.unc.edu/projects/addhealth/design
- Harris KM, et al. Add Health: the national longitudinal study of adolescent to adult health. 2015.
- Harris KM. Design features of Add Health. Chapel Hill (NC): Carolina Population Center, University of North Carolina at Chapel Hill; 2005.
- Marsden PV. Network data and measurement. Annu Rev Sociol. 1990;16:435–63. doi:10.1146/annurev.so.16.080190.002251.
- Perkins JM, Subramanian SV, Christakis NA. Social networks and health: a systematic review of sociocentric network studies in low- and middle-income countries. Social Sci Med. 2015;125:60–78. doi:10.1016/j.socscimed.2014.08.019.
- Cavanagh SE. The sexual debut of girls in early adolescence: the intersection of race, pubertal timing, and friendship group characteristics. J Res Adolesc. 2004;14:285–312. doi:10.1111/j.1532-7795.2004.00076.x.
- Merlo J, et al. A brief conceptual tutorial of multilevel analysis in social epidemiology: using measures of clustering in multilevel logistic regression to investigate contextual phenomena. J Epidemiol Commun H. 2006;60:290–97.
- Subramanian SV, Jones K. Multilevel statistical models: concepts and applications. Boston: Harvard School of Public Health & Centre for Multilevel Modelling, University of Bristol; 2012.
- Merlo J, Bengtsson-Boström K, Lindblad U, Råstam L, Melander O. Multilevel analysis of systolic blood pressure and ACE gene I/D polymorphism in 438 Swedish families – a public health perspective. BMC Med Genet. 2006;7:14. doi:10.1186/1471-2350-7-14.
- Cubbin C, Santelli J, Brindis CD, Braveman P. Neighborhood context and sexual behaviors among adolescents: findings from the national longitudinal study of adolescent health. Perspect Sex Reprod Health. 2005;37:125–34. doi:10.1363/3712505.
- Peterson CE, Sastry N, Pebley AR, Ghosh-Dastidar B, Williamson S, Lara-Cinisomo S. The Los Angeles family and neighborhood survey: codebook. RAND Working Paper Series DRU-2400/2-1-LAFANS; 2004.
- Dunn EC, Milliren CE, Evans CR, Subramanian SV, Richmond TK. Disentangling the relative influence of schools and neighborhoods on adolescents’ risk for depressive symptoms. Am J Public Health. 2015;105:732–40. doi:10.2105/AJPH.2014.302374.
- Granovetter M. The strength of weak ties. Am J Sociol. 1973;78:1360–80. doi:10.1086/225469.
- Porter MA, Onnela J-P, Mucha PJ. Communities in networks. Not Am Math Soc. 2009;56:1082–97.
- Palla G, Derényi I, Farkas I, Vicsek T. Uncovering the overlapping community structure of complex networks in nature and society. Nature. 2005;435:814–18. doi:10.1038/nature03607.
- Blondel VD, Guillaume J-L, Lambiotte R, Lefebvre E. Fast unfolding of communities in large networks. J Stat Mech. 2008;10:P10008. doi:10.1088/1742-5468/2008/10/P10008.
- Lipari RN, Van Horn SL, Hughes A, Williams M. Underage binge drinking varies within and across states. Center for Behavioral Health Statistics and Quality (CBHSQ) Report. Rockville (MD): Substance Abuse and Mental Health Services Administration (SAMHSA); 2017.
- Keyes KM, Kaur N, Kreski NT, Chen Q, Martins SS, Hasin D, Olfson M, Mauro PM. Temporal trends in alcohol, cannabis, and simultaneous use among 12th-grade U.S. adolescents from 2000 to 2020: differences by sex, parental education, and race and ethnicity. Alcohol Clin Exp Res. 2022;46:1677–86. doi:10.1111/acer.14914.
- Newman MEJ. Modularity and community structure in networks. Proc Natl Acad Sci USA. 2006;103:8577–82. doi:10.1073/pnas.0601602103.
- Anaconda Software D. Computer software. Vers. 3 6. Continuum Analytics; 2016.
- Hagberg AA, Schult DA, Swart PJ. Proceedings of the 7th Python in Science Conference (SciPy2008), Varoquaux G, T Vaught, and J Millman, editors. Pasadena, CA; 2008. p. 11–15.
- Csardi G, Nepusz T. The igraph software package for complex network research. Int J Complex Syst. 2006;1695:1–9.
- Charlton C, Rasbash J, Browne WJ, Healy M, Cameron B. MLwiN version 3.00. Centre for Multilevel Modeling, University of Bristol; 2017.
- StataCorp. Stata statistical software: release 17. College Station (TX): StataCorp LP; 2021.
- Leckie G, Charlton C. Runmlwin: a program to run the MLwiN multilevel modeling software from within stata. J Stat Softw. 2012;52. doi:10.18637/jss.v052.i11.
- Browne WJ. MCMC estimation in MLwiN v3.00. Centre for Multilevel Modeling, University of Bristol; 2017.
- Jackson KM, Sartor CE. The Natural Course of Substance Use and Dependence. In: Sher KJ, editor. The Oxford handbook of substance use and substance use disorders. Vol. 1. Oxford: Oxford University Press; 2016.
- Lewis A, Neighbors C, Lindgren K, Buckingham KG, Hoang M. Social influences on adolescent and young adult alcohol use. New York: Nova Science Publishers, Inc; 2010. p. 1–80.
- Jacobs W, Goodson P, Barry AE, McLeroy KR. The role of gender in adolescents’ social networks and alcohol, tobacco, and drug use: a systematic review. J Sch Health. 2016;86:322–33. doi:10.1111/josh.12381.
- Deutsch AR, Steinley D, Slutske WS. The role of gender and friends’ gender on peer socialization of adolescent drinking: a prospective multilevel social network analysis. J Youth Adolesc. 2014;43:1421–35. doi:10.1007/s10964-013-0048-9.
- Hunter RF, de la Haye K, Murray JM, Badham J, Valente TW, Clarke M, Kee F. Social network interventions for health behaviours and outcomes: a systematic review and meta-analysis. PLoS Med. 2019;16:e1002890. doi:10.1371/journal.pmed.1002890.
- McMillan C, Schaefer DR. Comparing targeting strategies for network-based adolescent drinking interventions: a simulation approach. Social Sci Med. 2021;282:114136. doi:10.1016/j.socscimed.2021.114136.
- Centers for Disease Control and Prevention. Trends in the prevalence of alcohol use: national YRBS 1991-2019. Centers for Disease Control and Prevention, National Center for HIV/AIDS, Viral Hepatitis, STD, and TB Prevention, Division of Adolescent and School Health; 2020.
- Strowger M, Braitman AL. Using social network methodology to examine the effects of exposure to alcohol-related social media content on alcohol use: a critical review. Exp Clin Psychopharmacol. 2022;31:280. doi:10.1037/pha0000561.