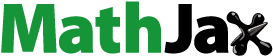
ABSTRACT
In the context of technological change and the ongoing transformation of the labour market, this paper investigates firms’ employer-provided continuing training provision for employees with different skill requirements. Following human capital theory, firms invest in training when expecting higher returns than costs. From a theoretical point of view, only investment in employees in high-skilled jobs is reasonable. Empirically, this is not always the case. Using firm-level data from the BIBB Establishment Panel on Training and Competence Development, a fractional logit model is applied to answer which role technology use and task profiles play in employees’ training participation. The results suggest that firms with a higher proportion of digital technology users provide more training. On the contrary, more working time spent with digital technologies is associated with less training. A potential explanation could be that after initial training in using digital technologies, there are substantial learning effects and employees become more experienced. Additionally, employees who more frequently perform complex tasks receive more training independent of their jobs’ general skill requirements.
Technological change and the need for training
Technological change, in particular computers being able to perform certain human tasks, largely affects the way we work. This ranges from the redefinition of processes in the production industry to a fast-growing service sector (Boden and Miles Citation2019; WTO Citation2019; Hirsch-Kreinsen Citation2016). While new jobs emerge, some jobs undergo major changes or even perish (Frey and Osborne Citation2017; Autor Citation2015).
Researchers argue that technological change in the post-industrial era of the twentieth century has gone along with an increasing demand for higher qualifications – the so-called skill-biased technological change (SBTC) (Acemoglu and Autor Citation2011; Goldin and Katz Citation2008; Acemoglu Citation2002; Katz and Autor Citation1999). However, the SBTC hypothesis fails to explain job polarisation recently observed in many western economies (Fernández-Macías and Hurley Citation2017; Goos, Manning, and Salomons Citation2014). By categorizing workers’ tasks into routine and nonroutine tasks, Autor, Levy, and Murnane (Citation2003) offer a framework to analyse the effects of computerisation on labour demand, i.e. computers substituting for human labour in routine tasks. Using the task framework, Goos, Manning, and Salomons (Citation2014) derive a model of routine-biased technological change (RBTC) being able to explain labour market polarisation.
Although the employment effects of technological change are subject to ongoing debate, most researchers agree that due to computerisation employees’ tasks are changing and gaining in complexity. Accordingly, many jobs show a rise in necessary qualifications and requirements (Goldin and Katz Citation2018; Arntz et al. Citation2016; Michaels, Natraj, and Van Reenen Citation2014; Goos and Manning Citation2007; Spitz-Oener Citation2006). The growing literature on new digital technologies and technological change points out the importance of lifelong learning in general (Merriam and Baumgartner Citation2020) and employer-provided training in particular (Kleinert and Wölfel Citation2018; Konings and Vanormelingen Citation2015; Nilsson and Rubenson Citation2014; Bresnahan, Brynjolfsson, and Hitt Citation2002). However, evidence on employer provided training in the context of recent technological change is still scarce.
This paper tries to address this research gap by analysing current firm-level data from Germany. To the best of our knowledge, it is the first to examine the relationship between employees’ job tasks, their use of digital technologies and employer-provided training from the aggregated firm perspective. We focus on two aspects. Firstly, we seek to answer how employees’ job tasks affect their participation in employer-provided training. Secondly, we want to investigate if firms’ overall technology-levelFootnote1 and employees’ use of digital technologies affect the participation in employer-provided training. We expect that a greater use of digital technologies by employees and more interactive/cognitive tasks relates to more participation in training. The idea behind this is that firms invest in their employees by providing them with the skills necessary for them to perform their jobs.
Continuing training in Germany
In Germany, between 50 and 85 percent of all firms offer continuing training to their employees, depending on the definition and data source (Mohr Citation2019; Dummert Citation2018; Seyda and Placke Citation2017), and training participation rates have grown over the last decades (BMBF Citation2017). However, employees’ participation largely depends on structural factors of the individual like qualification level (Seyda, Wallossek, and Zibrowius Citation2018; Kleinert and Wölfel Citation2018; Bellmann Citation2003). In the case of employer-provided training, the possibilities and support for employer-provided continuing training (Lukowski Citation2017; Leber Citation2009) as well as general firm characteristics like industry, size, industrial relations or technological infrastructure (Lukowski and Baum Citation2017; Leber Citation2009; Neubäumer, Kohaut, and Seidenspinner Citation2006; Brussig and Leber Citation2005) are additional factors. Moreover, formalised training structures and strong employee representation increase continuing training for all skill groups (Kleinert and Wölfel Citation2018; Wotschack Citation2019).
Continuing training in the context of tasks and technologies
According to human capital theory (Becker Citation1964), training activities are investments in human capital. Employers only invest in training when the expected returns exceed the costs (the principle of utility maximisation), especially due to higher returns from increased innovative capability, quality of their products and employee productivity (Almeida and Carneiro Citation2009; Behringer, Kampmann, and Käpplinger Citation2009; Becker Citation1964). Therefore, firms are more likely to invest in training measures for highly skilled employees because they expect higher returns (Behringer, Kampmann, and Käpplinger Citation2009). Consequently, this rational investment decision can explain the differences in training provided to different skill groups (Kleinert and Wölfel Citation2018; Boeren Citation2009; Behringer, Kampmann, and Käpplinger Citation2009). However, several studies that focus on the job-related tasks of different employee groups have shown that differences in training participation do not only exist between different skill groups, but also within skill groups (Görlitz and Tamm Citation2016a; Booth and Bryan Citation2007; Acemoglu and Pischke Citation1998, Citation1999). This cannot be explained by human capital theory. Therefore, research and theory not only shifted from classification into skill groups to the tasks employees perform but as well to the understanding, that the labour market is not perfect and wage compression exists (Picchio and van Ours Citation2011; Acemoglu and Pischke Citation1998, Citation1999).
For this approach, the differentiation between routine and non-routine tasks is crucial. In their theoretical model, Acemoglu and Pischke (Citation1999) argue that employees have varying productivity and employers have difficulty observing this. Therefore, they pay employees less than their productivity is actually worth. Since the output of routine work is easier to quantify than the output of non-routine work, it leads to greater wage compression for those with non-routine tasks. Greater wage compression leads to more investment resources for training – both general and specific – and therefore employers provide employees who perform non-routine tasks more frequently with more training (Acemoglu and Pischke Citation1999).
When combining the task approach and the assumption that routine tasks are more prone to substitution by technologies (Autor, Levy, and Murnane Citation2003), with the human capital theory, it can be argued, that it is not worthwhile to invest in employees with routine tasks, and firms should only invest in employees with non-routine tasks – independent of employees’ skill level. An additional explanation is that employees who exercise routine tasks need less updating of their skills due to fewer changes in their duties (Baethge and Baethge-Kinsky Citation2004; Shields Citation1998). When controlling for job tasks, the gap between high- and low-skilled employees shrinks drastically (Görlitz and Tamm Citation2016a). A recent study using individual-level data highlights the positive relationship between the performance of non-routine tasks and training participation, showing that employees in Germany whose task profiles are characterised by a high percentage of routine tasks participate less in employer-provided training, independent of the employees’ qualification level or prerequisites (Heß, Janssen, and Leber Citation2019).
A number of studies using firm-level data and focussing on employer-initiated or financed training support those findings. Employees performing routine tasks receive less continuing training than those performing non-routine/complex tasks (Tamm Citation2018; Görlitz and Tamm Citation2016a; Mohr, Troltsch, and Gerhards Citation2016). Moreover, after training, employees with a high initial share of routine work participate more in non-routine/complex tasks (Tamm Citation2018; Görlitz and Tamm Citation2016b).
In addition, analytical and interactive tasks are positively correlated with continuing training, whereas routine tasks are negatively correlated and, when the types of tasks are considered, skill-level is not significantly influencing the probability of receiving continuing training anymore (Kleinert and Wölfel Citation2018). We extend the existing evidence focussing on the firm’s perspective, i.e. employees’ aggregated task profiles.
This leads to the first hypothesis:
Firms are more likely to provide training if a job includes a high proportion of complex tasks, independent of the initial skill requirements.
One aspect of the human capital theory is that employers are only interested in investing in firm-specific training to prevent poaching by other firms. Accordingly, employers should only invest in training that cannot be used by other firms in order to exclusively gain from the increased productivity of the employee (Picchio and van Ours Citation2011; Becker Citation1964). The handling of new technologies is general skillsFootnote2 because employees can use these skills to find a new job, and digital technologies are in most cases not specific to a firm. According to human capital theory, employers would not invest in training for technological skills. However, employers need those skills to keep the company operating and thus investments in training for new technologies are indispensable. Moreover, the assumption that firms do not invest in general training cannot be supported for Germany, because poaching is not such a great danger in the German labour market (Müller Citation2014). Taking this and implications of the imperfect labour markets into account, employers in Germany should invest in training their employees for digital technologies.
A contrasting explanation to the human capital theory to explain employer-provided training is the configuration approach by Mintzberg (Citation1983). For this approach, continuing training is not a cost benefit calculation. Firms are embedded in several peripheral systems, e.g. economic, political, ecological and technological systems. Those systems can change and communicate their needs to the firm, which lead the firm to adapt to these changes and needs, in order to fit in. For example, technological change generates a qualification demand, which then leads to a reaction of the firm in form of continuing training for their employees (Käpplinger Citation2016). Altogether, this can explain why employers invest more in training for their employees, when more technologies are used.
In Germany, a rising need for continuing training in the context of digital technologies is evident (Seyda, Meinhard, and Placke Citation2018), and the empirical results for the different skill groups are mixed. Mohr, Troltsch, and Gerhards (Citation2016) discovered that with the introduction of new information and communication technology, technological change does not always lead to greater participation of employees in low-skilled jobs in continuing training. A possible reason for this could be the effects of the substitution of low-skilled employees by technologies.
Other studies show, that employees in jobs with lower skill requirements, especially those who have experienced a change in or the emergence of new services or computer programs at work, have the highest continuing training participation of this qualification group (Seyda, Wallossek, and Zibrowius Citation2018). Furthermore, firms with more intensive technology use have greater participation rates in continuing training for low-skilled employees than firms with less intensive technology use (Seyda, Meinhard, and Placke Citation2018; Troltsch and Lukowski Citation2017). However, a case study by Warnhoff and Krzywdzinski (Citation2018) emphasises that in a German industrial firm, employees with low qualifications are especially disregarded for continuing training in new digital technologies, whereas more highly qualified employees are being trained for the new digital requirements. Although there might be differences between employees in low- and high-skilled jobs, we expect:
(2) Firms with a higher level of technology use provide more training to their employees, independent of their initial skill requirements.
In sum, we hypothesise that, even though employees in low-skilled jobs receive less training in general, the execution of non-routine tasks and a higher technology use at their firm increases participation in continuing training.
Method
Data and sample
The BIBB Establishment Panel on Training and Competence Development (BIBB Training Panel) is an annual survey. It collects representative longitudinal data on the training activities of over 3500 establishments, from the employer’s perspective, in Germany. The data is usually collected through computer-assisted personal interviews (CAPI). The selection of establishments takes place via a disproportionately stratified sample of the statistical population of all establishments with one or more employees subject to mandatory social insurance contributions (for detailed information concerning the survey methodology cf. Gerhards, Mohr, and Troltsch Citation2012). The survey focuses especially on firms’ training activities, but employees’ use of digital technologies and employees’ tasks are surveyed as well.
In our analysis we use the data of the survey waves 2016 and 2017 (Gerhards, Mohr, and Troltsch Citation2018, Citation2020). Accordingly, only those establishments are considered in the analysis that participated in both waves. In the wave 2016 an additional sample of around 3500 establishments was interviewed via computer-assisted telephone interviews (CATI). In sum in around 7100 establishments took part in the survey wave 2016. In 2017, around 3700 establishments were questioned with CAPI. Due to the huge sample in 2016, a great percentage of those questioned in 2017, had been interviewed in 2016 as well. In total, we use the data of 3575 establishments in our analysis.
Measures
Participation in employer-provided continuing training
Employer-provided continuing training (employer-provided training) in the dataset includes training activities that are at least partially funded by employers and/or that fully or partly take place during an employee’s paid working time. The literature on continuing training differentiates between three forms of training: formal continuing training (or upgrading training) that leads to the achievement of formal qualifications, non-formal training that usually takes place in internal or external courses and informal forms of training such as workplace-based training (Behringer and Schönfeld Citation2014). The focus of this study is non-formal training, which is equal to employees’ participation in internal or external courses (plus seminars and workshops).
The participation rate can be distinguished for three different skill-requirement levels: employees in low-skilled, medium-skilled or high-skilled jobs. This differentiation is based on the subjective skill-level required for performing work tasks evaluated by the employer, rather than employees’ formal qualifications.
The participation rate (between 0% and 100%) of each skill group is the dependent variable for the estimation model. It is important to note that the participation rate is measured in the wave from 2017, which surveyed information from 2016, whereas the following independent variables are taken from the 2016 survey wave, which covered data from 2015. This timing is crucial to avoid a reverse causality bias.
Exposure to digital technologies
Three different indicators for employees’ exposure to digital technologies are used: (1) the portion of digital technology users, (2) the time spent using digital technologies and (3) the firm’s overall technology level. The first two indicators are included to measure average technology usage and time spent using technologies. Here the average portion of users and time-periods are given for each skill-requirement group.
The firm’s technology level is included because the potential need for training not only arises from employees using and spending time with digital technologies in general, but also from what kinds of technologies are used in the firm, since more advanced technologies may be more difficult to operate. Therefore, firms’ overall digital technology level acts as an indicator for the level of employees’ technology use. For measuring this, similarly to Weller, Lukowski, and Baum (Citation2018), an index ranging from 0 to 7 was constructed from the number of technologies being used in a firm (Level 1 comprises one or two technologies being used). The higher the score of a firm, the more likely they are to have more advanced digital technologies. Information on the use of the following digital technologies is provided:
Information and communication-related digital work devices or applications, such as computers, laptops, notebooks, smartphones or mobile telephones;
Digital network technology, such as the internet, intranet, email and content management systems;
Computer-aided tools or technologies for the creation of products and services, including machine tools, computer numerical control machines (CNC), computer-aided design (CAD) and measurement, analysis and diagnostic devices;
Digital technologies that relate to networking with customers, e.g. company websites with product overviews or service provision, online ordering or reservation systems and social media;
Digital technologies that relate to networking with suppliers, e.g. Enterprise resource management systems (ERP);
Digital technologies that relate to human resources or work organisation, such as human resource management tools and building and facilities management tools;
Digital technologies that relate to the collection, storage and processing of large quantities of data, e.g. big data and cloud computing;
Digital technologies that relate to data security and data protection.
The distribution of firms according to their technology level is displayed in .
Task profiles
As Autor, Levy, and Murnane (Citation2003) pointed out, tasks are a useful tool for observing job characteristics. The dataset surveys employees’ tasks from the firm’s perspective, e.g. managers. Information on employees’ task profiles are provided by indicating how often they perform certain tasks on a scale from 1 (never) to 5 (very often). The task module contains eight items being separately evaluated for the three skill-requirement groups. Conducting a factor analysis for each group, the same three underlying factors can be identified: (1) interactive/cognitive tasks, (2) routine tasks and (3) manual tasks (). Interactive/cognitive tasks in this analysis are a proxy for complex tasks.
Control variables
Structural variables like industry, size, region and if the firm offers vocational education and training are related to a firm’s technology-level (e.g. Baum and Lukowski Citation2019; Weller, Lukowski, and Baum Citation2018) and employees’ participation in employer-provided training (e.g. Lukowski and Baum Citation2017; Leber Citation2009), and therefore may influence employees’ tasks and should be controlled for. Additionally, firms’ overall investment is considered since training investment is part of overall investment decisions (Hansson Citation2004). Moreover, investment, continuing training and productivity are strongly interlinked (Zwick Citation2005; Hempell Citation2003).
Analysis
For the empirical analysis, a fractional logit model (Papke and Wooldridge Citation1996) is applied. This quasi-likelihood estimator is suitable for dependent variables ranging between 0 and 1.
As a dependent variable, the employees’ participation rate in employer-provided training in
is observed for each firm
. The expected value
is estimated, where
is a set of covariates at time
. The corresponding model can be described by
Note: UserDT: Proportion of digital technology users, TimeDT: Share of working time using digital technologies, TecLev: Firm’s technology-level, IntCogTasks: Interactive/Cognitive tasks, RouTasks: Routine tasks, ManTasks: Manual tasks, Inv: Firm’s overall investment, Ind: Firm’s industry, Size: Firm Size, Reg: Region, VET: provision of vocational education and training with
being the logistic function. The model is separately estimated for the three different skill-requirement groups. A detailed table of the characteristics of the variables in the model can be found in the and .
Results
displays the average proportion of each skill-requirement group in the firms and their participation rate in employer-provided training. Moreover, it shows these groups’ exposure to digital technologies. Employees with medium-skilled jobs participated in employer-provided training the most, whereas employees in low-skilled jobs participated the least. This group also used digital technology far less and spent the least amount of time with digital technologies.
Table 1. Employees participation in employer-provided training and usage of and time spent with digital technologies (in %).
Detailed regression results are reported in . For all employees, interactive/cognitive tasks revealed a highly significant positive relationship with participation in employer-provided training with an increase for all skill groups around 3–4%, whereas routine tasks only showed a positive influence on the participation rate of employees in low-skilled jobs (2%). The reasons behind this trend need to be further established. However, this does support the first hypothesis.
Table 2. Regression results (average marginal effects).
A positive relationship between the proportion of digital technology users and participation in employer-provided training for employees with low- (8%) and medium-skilled jobs (10%) can be observed. For employees with low-skilled jobs, this relationship is not significant. Still, if more employees in a firm use digital technologies, the firm on average provides more training.
This effect is the opposite for the amount of working time employees spend with digital technologies. The more time they spent using them, the lower their participation in employer-provided training (low-skilled jobs: 9%; medium-skilled jobs: 1%; high-skilled jobs: 7%).
A firm’s technology-level only increases the participation rate for employees in medium-skilled jobs (2%). The results in support of the second hypothesis are therefore mixed.
Incorporating additional continuing training variables in the model
One can argue that the general willingness of firms to train their employees can affect general participation rates (Heß, Janssen, and Leber Citation2019; Mohr, Troltsch, and Gerhards Citation2016), and the influence of technologies and tasks can affect participation rates for all employee groups. Therefore, in a second analysis, the training possibilities were included in a threefold manner. First, a dummy variable for whether a firm offered any type of training in 2016 and second a dummy variable for whether the firm offered upgrading training or informal training (e.g. workplace-based training) in addition to non-formal training in 2017 were created.
As shows, the offering of any form of training in the year before or other types of training in the same year influences the general participation in training of all employee groups. However, the general pattern that interactive/cognitive tasks increase the participation rates for all groups, similar to a greater proportion of digital technology users, remains. Again, a firm’s technology level only increases the participation rate of the medium-skilled employees. The only differences are that routine tasks do not influence the participation rate of employees with low-skilled jobs anymore, and that employees with high-skilled jobs now participate more in training when the share of technology users is greater.
Table 3. Regression results with extended training variables (average marginal effects).
Discussion
Technological change leads to changing working requirements, which require suitable employees. One strategy for employers is to train their existing staff in handling the new tasks and technologies, which, as this paper addresses, influences the impact employees’ tasks and technology use have on participation rates in employer-provided training for employees with low-, medium- and high-skilled jobs.
The results support that a frequent performance of interactive/cognitive tasks correlates with participation in employer-provided training – independent of employees’ skill requirements. For all three groups of employees (with low-, medium- and high-skilled jobs) we observe higher training participation rates in firms where employees more frequently perform complex tasks. In contrast to our expectation, the first model () shows that firms where employees with low-skilled jobs perform routine tasks more frequently tend to report higher training participation rates for this group. However, this finding is not replicated in the extended model. When controlling for other forms of continuing training and past-year training, firms with employees performing routine tasks more frequently do not report higher training rates. Therefore, in sum the findings support our first hypothesis that firms are more likely to provide training if job profiles are characterised by a high proportion of complex tasks and are in line with earlier findings (Tamm Citation2018; Görlitz and Tamm Citation2016a; Mohr, Troltsch, and Gerhards Citation2016).
The findings regarding the relationship between use of technologies and training participation rates in employer-provided continuing training (our second hypothesis) are not as clear as the relationship with complex tasks. The model includes several indicators for measuring the use of technologies.
With regard to the proportion of digital technology users the models for employees in low- and medium-skilled jobs show a positive relationship between the proportion of employees that use digital technologies in a firm and the participation rates of these groups in employer-provided training. For employees in high-skilled jobs, the evidence is mixed. We find no significant relationship between technology use and participation in employer-provided training in the first model, but in the extended model, we do.
A possible explanation might be that this group has a greater share of complex tasks, which are more decisive in their participation in employer-provided training than their technology use. Technological adoption might be incremental for this group, while changes have larger effects on the work of employees in low- and medium-skilled jobs. When including the additional continuing training variables, a greater proportion of digital technology users leads to a report of greater participation rates of employees in high-skilled jobs. Therefore, another explanation could be that employees in high-skilled jobs learn more often in informal settings than the other skill groups, due to different qualification requirements.
The influence of the other technology indicators does not fully support the second hypothesis. More working time spent with digital technologies is associated with less training for all employee groups, whereas a firm’s higher overall technology-level only goes along with a higher training participation rate of employees in medium-skilled jobs.
Learning effects might explain why general technology use goes along with greater training participation rates whereas time spent with technologies is related to lower participation rates. Spending more time with the same technologies could imply employees becoming more skilled in handling them through learning by doing. Employers could also anticipate that more provision of training is not economically reasonable and adjust accordingly.
The reason why firms’ overall technology level only is significant related to employees in medium-skilled jobs must be further investigated. Possibly, this group is more exposed to digital technologies and therefore needs more training. In addition, employees in medium-skilled jobs may lack the initial knowledge of employees in high-skilled jobs. Alternatively, the firm might be under pressure to adapt to the technological peripheral system stronger for employees in medium-skilled jobs.
In sum, the second hypothesis can only partially be supported. More technologies and their use are associated with more employer-provided training, although the effects differ in magnitude for the three groups. More working time spent with digital technologies on the other hand relates to less training.
With respect to human capital theory, the findings on tasks are in line with the Acemoglu-Pischke model of imperfect labour markets and stress the usefulness of the task framework in explaining differences in training participation within groups. Regarding technology use, the Acemoglu-Pischke model can explain, why a larger proportion of technology users is associated with a higher participation rate in employer-provided training. Employees with medium-skilled jobs being the only group, where firm’s overall technology use plays a role for training participation, could be explained by higher wage compression for this group. Whereas, for employees in high-skilled jobs, use of these technologies might be already included in the wage. More time spent with digital technologies being negatively correlated with employer-provided training is in line with the model, if one assumes that firms anticipate informal learning and adjust their investment in course-based training accordingly. The results are also in line with Mintzberg’s configuration theory. For the systems, complex tasks and technology represent needs for skills. In order to fulfil these needs, firms adjust by offering training.
Strengths and limitations
This study explores as one of the first the influence of technologies and their usage on employees’ participation in employer-provided continuing. Moreover, this study uniquely combines this technology use with employees’ tasks. Herby, it tries to address the research gap by analysing current firm-level data from Germany and analysing the perspective of the aggregated point of view of firms offering the training.
Another strength of this study is that its analysis differentiates between three different skill requirement levels. This is important because those groups vary greatly in their participation in continuing training (e.g. Kleinert and Wölfel Citation2018; Boeren Citation2009; Behringer, Kampmann, and Käpplinger Citation2009) and different effects from tasks and technologies are possible.
Finally, the BIBB Establishment Panel on Training and Competence Development offers a data set that is representative of firms located in Germany. In this sample, firms of all sizes are well represented. This is not always the case for firm-level surveys, which often focus on large enterprises and therefore underrepresent small firms. Since the German economy heavily relies on small and medium enterprises (SMEs), the inclusion of these firms is a major strength of this study.
However, with respect to size, this firm structure differs for other economies, which often have a higher share of large firms. For example, this is the case in most Anglo-Saxon countries. Therefore, generalisability of this study in an international context might be limited.
Moreover, the analyses provide results at an aggregate (firm) level and cannot take the individual characteristics and decisions of employees into account. In addition, private training activities, and training activities financed by the employer that are not considered continuing training by the respondent, are not measured.
A general critique of the task approach is the strict, clear-cut distinction between routine and non-routine tasks (Pfeiffer Citation2018; Fernández-Macías and Hurley Citation2014). By following this approach, we implicitly make a simplification of employees’ tasks.
Another limitation is that although different points in time for tasks, technology use and resulting continuing training participation were taken into account, the potential of the panel design was not used to its fullest. The causality of complex tasks, technology usage and training participation could still be reversed. In addition, tasks and technology use could influence each other in unknown ways that have not been considered here.
Lastly, only continuing training in internal or external courses were considered in this analysis. For other, informal forms of training, the results could be different. Moreover, the specific contents and purposes of the employer-provided continuing training are not known. Therefore, we cannot be certain, whether the training is always related to the technology use.
Conclusion
This analysis shows that employees’ use of digital technologies is associated with more training, whereas more time using them relates to less training. With these findings, we provide new insights on the relationship between technologies and training. Regarding the task framework, this study is able to confirm earlier research on tasks and training by focussing solely on the firm’s perspective. On one hand, it shows on an aggregated firm-level that more frequent performance of complex tasks is related to higher participation in employer-provided training. On the other hand, it demonstrates that results on employees’ tasks generally being elicited on an individual level can be replicated using firm-level data.
These results are important because employees working in jobs with low skill requirements in general perform more routine tasks (Tamm Citation2018) and participate the least in continuing training (Fouarge, Schils, and De Grip Citation2013; Kyndt et al. Citation2013; Bellmann Citation2003). Employers offer those, who already perform complex tasks, even more training. Therefore, it would be favourable, if employers focus more on training employees with a high proportion of routine tasks in performing non-routine tasks. Since routine tasks are prone to computerisation, employees in low-skilled jobs might be particularly in danger of replacement by technologies. Hence, in qualifying these employees and broadening their scope of tasks, firms might be able to fulfil their need for skilled personnel that is becoming rare in certain industries.
In our opinion, further research is needed to overcome the limitations of this study and to find out more about the relationship between technologies, tasks and training. For example, panel analysis could be used to investigate the dynamics between technology use, tasks and training participation and to provide a more detailed look into the influence of certain types of technology use. Additionally, further research should take into account moderations, mediations or other relations between technology use and task profiles. This analysis considered general firm investments, while upcoming research might especially investigate investments in training. Moreover, alternative and digital forms of training are on the rise (Sousa and Rocha Citation2019; Williamson Citation2017; Lee Citation2012) and hence should be considered in further research. Additional exploration on the individual level might also be needed.
In conclusion, a central implication of this study is that employer-provided training could be an important instrument for firms to develop their employees’ skills and prepare them for more complex tasks in times of technological change. In addition, training can be an effective tool to satisfy the need for skilled personnel. Therefore, we stress the importance of lifelong learning and constant training.
Acknowledgements
The authors would like to thank Sabine Pfeiffer and three anonymous referees for their critical remarks and helpful comments.
Disclosure statement
No potential conflict of interest was reported by the author(s).
Data availability statement
This paper uses data from the BIBB Training Panel – An Establishment Panel on Training and Competence Development 2016, doi:10.7803/371.16.1.2.10 and 2017, doi:10.7803/371.17.1.2.10. The Survey was conducted by the Federal Institute for Vocational Education and Training (BIBB). The data is available via Remote Data Access or On-site Use in Bonn at the Data Research Centre at the Federal Institute for Vocational Training and Education (BIBB-FDZ).
Additional information
Funding
Notes
1 Firms provide information on their use of selected digital technologies. A higher number of technologies used corresponds to a higher technology-level. A detailed list of the digital technologies considered in the paper can be found in the measures section.
2 In contrast to segmentation theory, which classifies technological skills as firm-specific skills (cf. Käpplinger Citation2016) we define technological skills as general skills. According to segmentation theory, firms who increasingly use new technologies have an expanding internal labour market and a shrinking external labour market ultimately leading to more continuing training offers (Käpplinger Citation2016). Although segmentation theory makes different assumptions, it comes to similar conclusions as human capital theory with respect to training.
References
- Acemoglu, Daron. 2002. “Directed Technical Change.” The Review of Economic Studies 69 (4): 781–809. doi: 10.1111/1467-937X.00226
- Acemoglu, Daron, and David H. Autor. 2011. “Skills, Tasks and Technologies: Implications for Employment and Earnings.” In Handbook of Labor Economics , Vol. 4B, edited by Orley Ashenfelter and David E. Card, 1043–1171. Amsterdam: Elsevier B.V.
- Acemoglu, Daron, and Jörn-Steffen Pischke. 1998. “Why Do Firms Train? Theory and Evidence.” The Quarterly Journal of Economics 113 (1): 79–119. doi: 10.1162/003355398555531
- Acemoglu, Daron, and Jörn-Steffen Pischke. 1999. “Beyond Becker: Training in Imperfect Labour Markets.” The Economic Journal 109 (453): 112–142. doi: 10.1111/1468-0297.00405
- Almeida, Rita, and Pedro Carneiro. 2009. “The Return to Firm Investments in Human Capital.” Labour Economics 16 (1): 97–106. doi: 10.1016/j.labeco.2008.06.002
- Arntz, Melanie, Terry Gregory, Simon Jansen, and Ulrich Zierahn. 2016. Tätigkeitswandel und Weiterbildungsbedarf in der digitalen Transformation. Mannheim: ZEW-Gutachten und Forschungsberichte.
- Autor, David H. 2015. “Why Are There Still So Many Jobs? The History and Future of Workplace Automation.” Journal of Economic Perspectives 29 (3): 3–30. doi: 10.1257/jep.29.3.3
- Autor, David H., Frank Levy, and Richard J. Murnane. 2003. “The Skill Content of Recent Technological Change: An Empirical Exploration.” The Quarterly Journal of Economics 118 (4): 1279–1333. doi: 10.1162/003355303322552801
- Baethge, Martin, and Volker Baethge-Kinsky. 2004. Der ungleiche Kampf um das lebenslange Lernen. Münster: Waxmann.
- Baum, Myriam, and Felix Lukowski. 2019. “Welche Rolle spielt Bildung im digitalen Transformationsprozess?” Berufsbildung in Wissenschaft und Praxis 48 (3): 4–5.
- Becker, Gary. 1964. Human Capital. A Theoretical and Empirical Analysis with Special Reference to Education. New York: Columbia University Press.
- Behringer, Friederike, Jara Kampmann, and Bernd Käpplinger. 2009. “Theoretische Erklärungsansätze zur betrieblichen Weiterbildungsbeteiligung.” In Betriebliche Weiterbildung – der Continuing Vocational Training Survey (CVTS) im Spiegel nationaler und europäischer Perspektiven. Zeitschrift für Berufs- und Wirtschaftspädagogik, edited by Friederike Behringer, Bernd Käpplinger, and Günter Pätzold, 35–52. Steiner Verlag: Stuttgart.
- Behringer, Friederike, and Gudrun Schönfeld. 2014. “Lebenslanges Lernen in Deutschland – Welche Lernformen nutzen die Erwerbstätigen?” Berufsbildung in Wissenschaft und Praxis 44 (5): 4–5.
- Bellmann, Lutz. 2003. Datenlage und Interpretation der Weiterbildung in Deutschland. Bielefeld: Bertelsmann.
- BMBF (Bundesministerium fur Bildung und Forschung). 2017. Weiterbildungsverhalten in Deutschland 2016. Ergebnisse des Adult Education Survey. Bonn: AES-Trendbericht.
- Boden, Mark, and Ian Miles. 2019. Services and the Knowledge-Based Economy. London: Routledge.
- Boeren, Ellen. 2009. “Adult Education Participation: the Matthew Principle.” Filosofija-sociologija 20 (2): 154–161.
- Booth, Alison L., and Mark L. Bryan. 2007. “Who Pays for General Training in Private Sector Britain?” In Aspects of Worker Well-Being. Vol. 26 of Research in Labor Economics, edited by Solomon W. Polachek and Olivier Bargain, 85–123. Oxford: Elsevier.
- Bresnahan, Timothy F., Erik Brynjolfsson, and Lorin M. Hitt. 2002. “Information Technology, Workplace Organization, and the Demand for Skilled Labor: Firm-Level Evidence.” The Quarterly Journal of Economics 117 (1): 339–376. doi: 10.1162/003355302753399526
- Brussig, Martin, and Ute Leber. 2005. “Betriebliche Determinanten formeller und informeller Weiterbildung im Vergleich.” Zeitschrift für Personalforschung 19 (1): 5–24.
- Dummert, Sandra. 2018. “Betriebliche Weiterbildungsbeteiligung und Weiterbildungsquote.” In Datenreport zum Berufsbildungsbericht 2018. Informationen und Analysen zur Entwicklung der beruflichen Bildung, 216–219. Bonn: Bundesinstitut für Berufsbildung.
- Fernández-Macías, Enrique, and John Hurley. 2014. European Jobs Monitor 2014: Drivers of Recent Job Polarisation and Upgrading in Europe. Luxembourg: Publications Office of the European Union.
- Fernández-Macías, Enrique, and John Hurley. 2017. “Routine-Biased Technical Change and job Polarization in Europe.” Socio-Economic Review 15 (3): 563–585.
- Fouarge, Didier, Trudie Schils, and Andris De Grip. 2013. “Why Do Low-Educated Workers Invest Less in Further Training?” Applied Economics 45 (18): 2587–2601. doi: 10.1080/00036846.2012.671926
- Frey, Carl B., and Michael A. Osborne. 2017. “The Future of Employment: How Susceptible Are Jobs to Computerisation?” Technological Forecasting and Social Change 114: 254–280. doi: 10.1016/j.techfore.2016.08.019
- Gerhards, Christian, Sabine Mohr, and Klaus Troltsch. 2012. “The BIBB Training Panel – An Establishment Panel on Training and Competence Development.” Schmollers Jahrbuch: Zeitschrift für Wirtschafts- und Sozialwissenschaften 132 (4): 635–652. doi: 10.3790/schm.132.4.635
- Gerhards, Christian, Sabine Mohr, and Klaus Troltsch. 2018. BIBB Training Panel – An Establishment Panel on Training and Competence Development 2016. GWA_1.0; Research Data Center at BIBB (ed., data access). Bonn: Federal Institute for Vocational Education and Training. doi:10.7803/371.16.1.2.10.
- Gerhards, Christian, Sabine Mohr, and Klaus Troltsch. 2020. BIBB Training Panel – An Establishment Panel on Training and Competence Development 2017. GWA_1.0; Research Data Center at BIBB (ed., data access). Bonn: Federal Institute for Vocational Education and Training. doi:10.7803/371.17.1.2.10.
- Goldin, Claudia, and Lawrence F. Katz. 2008. The Race Between Education and Technology. Cambridge: Harvard University Press.
- Goldin, Claudia, and Lawrence F. Katz. 2018. “The Race Between Education and Technology.” In Inequality in the 21st Century, edited by David Grusky and Jasmine Hill, 49–54. New York: Routledge.
- Goos, Maarten, and Alan Manning. 2007. “Lousy and Lovely Jobs: The Rising Polarization of Work in Britain.” The Review of Economics and Statistics 89 (1): 118–133. doi: 10.1162/rest.89.1.118
- Goos, Maarten, Alan Manning, and Anna Salomons. 2014. “Explaining Job Polarization: Routine-Biased Technological Change and Offshoring.” American Economic Review 104 (8): 2509–2526. doi: 10.1257/aer.104.8.2509
- Görlitz, Katja, and Marcus Tamm. 2016a. “Revisiting the Complementarity Between Education and Training: The Role of Job Tasks and Firm Effects.” Education Economics 24 (3): 261–279. doi: 10.1080/09645292.2015.1006182
- Görlitz, Katja, and Marcus Tamm. 2016b. “The Returns to Voucher-Financed Training on Wages, Employment and job Tasks.” Economics of Education Review 52: 51–62. doi: 10.1016/j.econedurev.2016.01.004
- Hansson, Bo. 2004. “Is It Time to Disclose Information About Human Capital Investments?” Available at SSRN: https://ssrn.com/abstract=615503.
- Hempell, Thomas. 2003. Do Computers Call for Training? Firm Level Evidence on Complementarities Between ICT and Human Capital Investments . ZEW Discussion Papers, No. 03-20. Mannheim: Zentrum für Europäische Wirtschaftsforschung (ZEW).
- Heß, Pascal, Simon Janssen, and Ute Leber. 2019. “Beschäftigte, deren Tätigkeiten durch Technologien ersetzbar sind, bilden sich seltener weiter.” IAB-Kurzbericht 16/2019.
- Hirsch-Kreinsen, Hartmut. 2016. “Digitization of Industrial Work: Development Paths and Prospects.” Journal for Labour Market Research 49 (1): 1–14. doi: 10.1007/s12651-016-0200-6
- Käpplinger, Bernd. 2016. Betriebliche Weiterbildung aus der Perspektive von Konfigurationstheorien. Bielefeld: W. Bertelsmann.
- Katz, Lawrence F., and David H. Autor. 1999. “Changes in the Wage Structure and Earnings Inequality.” In Handbook of Labor Economics Vol. 4B, edited by Orley Ashenfelter and David E. Card, 1463–1555. Amsterdam: Elsevier B.V.
- Kleinert, Corinna, and Oliver Wölfel. 2018. “Technologischer Wandel und Weiterbildungsteilnahme.” Berufsbildung in Wissenschaft und Praxis 47 (1): 11–15.
- Konings, Jozef, and Stijn Vanormelingen. 2015. “The Impact of Training on Productivity and Wages: Firm-Level Evidence.” Review of Economics and Statistics 97 (2): 485–497. doi: 10.1162/REST_a_00460
- Kyndt, Eva, Natalie Govaerts, Trees Claes, Jens De La Marche, and Filip Dochy. 2013. “What Motivates Low-Qualified Employees to Participate in Training and Development? A Mixed-Method Study on Their Learning Intentions.” Studies in Continuing Education 35 (3): 315–336. doi: 10.1080/0158037X.2013.764282
- Leber, Ute. 2009. “Betriebsgröße, Qualifikationsstruktur und Weiterbildungsbeteiligung – Ergebnisse aus dem IAB-Betriebspanel.” In Betriebliche Weiterbildung – der Continuing Vocational Training Survey (CVTS) im Spiegel nationaler und europäischer Perspektiven. Zeitschrift für Berufs- und Wirtschaftspädagogik, Beiheft 22, edited by Friederike Behringer, Bernd Käpplinger, and Günter Pätzold, 149–168. Stuttgart: Steiner Verlag.
- Lee, Kangdon. 2012. “Augmented Reality in Education and Training.” TechTrends 56 (2): 13–21. doi: 10.1007/s11528-012-0559-3
- Lukowski, Felix. 2017. “Anspruchsvoller arbeiten, mehr lernen?: Betriebliche Weiterbildung in Zeiten von Digitalisierung.” DIE-Zeitschrift für Erwachsenenbildung 24 (3): 42–44.
- Lukowski, Felix, and Myriam Baum. 2017. “Beschäftigte qualifizieren oder neu einstellen?: Strategien deutscher KMU, dem technologischen Wandel zu begegnen.” Berufsbildung in Wissenschaft und Praxis 46 (5): 13–17.
- Merriam, Sharan B., and Lisa M. Baumgartner. 2020. Learning in Adulthood: A Comprehensive Guide. Hoboken, NJ: John Wiley & Sons.
- Michaels, Guy, Ashwini Natraj, and John Van Reenen. 2014. “Has ICT Polarized Skill Demand? Evidence from Eleven Countries Over Twenty-Five Years.” Review of Economics and Statistics 96 (1): 60–77. doi: 10.1162/REST_a_00366
- Mintzberg, Henry. 1983. Power in and Around Organizations. Englewood Cliffs, NJ: Prentice-Hall.
- Mohr, Sabine. 2019. “Ergebnisse aus dem BIBB-Qualifizierungspanel zur betrieblichen Weiterbildung.” In Datenreport zum Berufsbildungsbericht 2019. Informationen und Analysen zur Entwicklung der beruflichen Bildung, 348–351. Bonn: Bundesinstitut für Berufsbildung.
- Mohr, Sabine, Klaus Troltsch, and Christian Gerhards. 2016. “Job Tasks and the Participation of Low-Skilled Employees in Employer-Provided Continuing Training in Germany.” Journal of Education and Work 29 (5): 562–583. doi: 10.1080/13639080.2015.1024640
- Müller, Norman. 2014. “Does CVT of Firms in Germany Suffer from Poaching?” Empirical Research in Vocational Education and Training 6 (1): 1–26. doi: 10.1186/1877-6345-6-1
- Neubäumer, Renate, Susanne Kohaut, and Margarete Seidenspinner. 2006. “Determinanten betrieblicher Weiterbildung. Ein ganzheitlicher Ansatz zur Erklärung des betrieblichen Weiterbildungsverhaltens und eine empirische Analyse für Westdeutschland.” Schmollers Jahrbuch: Zeitschrift für Wirtschafts- und Sozialwissenschaften 126 (3): 437–471. doi: 10.3790/schm.126.3.437
- Nilsson, Staffan, and Kjell Rubenson. 2014. “On the Determinants of Employment-Related Organised Education and Informal Learning.” Studies in Continuing Education 36 (3): 304–321. doi: 10.1080/0158037X.2014.904785
- Papke, Leslie E., and Jeffrey M. Wooldridge. 1996. “Econometric Methods for Fractional Response Variables with an Application to 401 (k) Plan Participation Rates.” Journal of Applied Econometrics 11 (6): 619–632. doi: 10.1002/(SICI)1099-1255(199611)11:6<619::AID-JAE418>3.0.CO;2-1
- Pfeiffer, Sabine. 2018. “Industry 4.0: Robotics and Contradictions.” In Technologies of Labour and the Politics of Contradiction, edited by Paško Bilić, Jaka Primorac, and Bjarki Valtýsson, 19–36. Cham: Palgrave.
- Picchio, Matteo, and Jan C. van Ours. 2011. “Market Imperfections and Firm-Sponsored Training.” Labour Economics 18 (5): 712–722. doi: 10.1016/j.labeco.2011.03.001
- Seyda, Susanne, David B. Meinhard, and Beate Placke. 2018. “Weiterbildung 4.0-Digitalisierung als Treiber und Innovator betrieblicher Weiterbildung.” IW-Trends–Vierteljahresschrift zur empirischen Wirtschaftsforschung 45 (1): 107–124.
- Seyda, Susanne, and Beate Placke. 2017. “Die neunte IW-Weiterbildungserhebung. Kosten und Nutzen betrieblicher Weiterbildung.” IW-Trends 44 (4): 3–19.
- Seyda, Susanne, Luisa Wallossek, and Michael Zibrowius. 2018. “Keine Ausbildung-keine Weiterbildung? Einflussfaktoren auf die Weiterbildungsbeteiligung von An- und Ungelernten.” IW-Analysen 122.
- Shields, Michael. 1998. “Changes in the Determinants of Employer-Funded Training for Full-Time Employees in Britain, 1984–94.” Oxford Bulletin of Economics and Statistics 60 (2): 189–214. doi: 10.1111/1468-0084.00094
- Sousa, Maria J., and Álvaro Rocha. 2019. “Leadership Styles and Skills Developed Through Game-Based Learning.” Journal of Business Research 94: 360–366. doi: 10.1016/j.jbusres.2018.01.057
- Spitz-Oener, Alexandra. 2006. “Technical Change, Job Tasks, and Rising Educational Demands: Looking Outside the Wage Structure.” Journal of Labor Economics 24 (2): 235–270. doi: 10.1086/499972
- Tamm, Marcus. 2018. “Training and Changes in Job Tasks.” Economics of Education Review 67: 137–147. doi: 10.1016/j.econedurev.2018.09.007
- Troltsch, Klaus, and Felix Lukowski. 2017. “Digitalisierungsprozesse, Ausbildungsbeteiligung, Fachkräfterekrutierung. Ergebnisse aus dem Betriebsbericht Qualifizierungspanel. Juli 2017.” Bundesinstitut für Berufsbildung. https://www.bibb.de/dokumente/pdf/Betriebsbericht_Erhebungswelle_2016.pdf.
- Warnhoff, Kathleen, and Martin Krzywdzinski. 2018. “Digitalisierung spaltet -Geringqualifizierte Beschäftigte haben weniger Zugang zu Weiterbildung.” WZB Mitteilungen 162: 58–60.
- Weller, Sabrina, Felix Lukowski, and Myriam Baum. 2018. “Digitalisierung in Betrieben und betriebliche Ausbildung – Ergebnisse aus dem BIBB-Qualifizierungspanel.” In Datenreport zum Berufsbildungsbericht 2018. Informationen und Analysen zur Entwicklung der beruflichen Bildung, 220–223. Bonn: Bundesinstitut für Berufsbildung.
- Williamson, Ben. 2017. Big Data in Education: The Digital Future of Learning, Policy and Practice. London: Sage.
- Wotschack, Philip. 2019. “When Do Companies Train Low-Skilled Workers? The Role of Institutional Arrangements at the Company and Sectoral Level.” British Journal of Industrial Relations. Advance access. doi:10.1111/bjir.12503.
- WTO (World Trade Organization). 2019. World Trade Statistical Review 2019. Geneva: World Trade Organization.
- Zwick, Thomas. 2005. “Continuing Vocational Training Forms and Establishment Productivity in Germany.” German Economic Review 6 (2): 155–184. doi: 10.1111/j.1465-6485.2005.00125.x