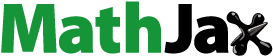
Abstract
Steel-making and continuous casting (SCC) processes are considered the main bottlenecks in the steel industry. We consider planning and scheduling problems in SCC processes and provide an overview of the literature with our commentary. The planning problem has as goal to create out of customer orders production units for the various production processes. The scheduling problem assigns these production units to the production facilities over different time intervals. We review a large number of planning and scheduling papers and propose two basic models for the planning and the scheduling problems that incorporate the most common and essential features. We describe for each problem the respective constraints and objectives as well as the practical implications in an actual production environment. We also analyse the methodologies used in their solutions, including decomposition strategies. In the last section, we present the conclusions of our survey and propose new research directions.
1. Introduction
The steel industry is clearly one of the more important process industries (Kadlec et al., Citation2009). Because of its high fixed costs, an efficient utilisation of equipment is desirable, which in practice leads to various operational problems. Many steel production processes have been controlled and managed by specialised engineers (Ruf et al., Citation2022), and the know-how in steel production has remained a tacit knowledge (Merten et al., Citation2022). Thus, digitalisation has been a catalyst for transforming tacit knowledge into explicit knowledge. For this purpose, several decision support systems or expert systems have been developed to systematise professional knowledge (Zhang et al., Citation2011). Such systems help engineers deal with various optimisation problems inherent in production, for example, planning, scheduling, and shipping. Classical optimisation problems in steel production have primarily as goal an increase in production efficiency and customer satisfaction. In addition, energy-related objectives have received much attention in order to achieve a sustainable production since the steel industry is the world’s largest CO2 producer and the second largest energy consumer (Branca et al., Citation2020).
The steel production process consists of iron-making, steel-making, continuous casting, hot rolling, and cold rolling, see . In the iron-making stage, iron ore is smelted with coke in a blast furnace to make pig iron that contains impurities. The steel-making stage makes steel by purifying pig iron and adjusting the chemical composition of substances such as carbon, sulfur, manganese, etc. The transfer unit of molten steel in a ladle is called a charge. The continuous casting transforms molten steel into slabs, which are intermediate products in cuboid form. In the continuous casting process, a series of charges—called a cast—are poured sequentially into the tundish of a continuous caster. In the hot rolling stage, slabs are heated and rolled into rectangular sheets. The sheets are turned into either coils or plates dependent upon their thickness after rolling. A sheet thinner than a certain thickness (e.g., 6 mm) is wound into coil products, whereas a thicker one is cut into plate products. shows the shapes of the coil and plate products. In the cold rolling stage, hot coils are unwound, re-rolled, and rewound when producing cold coils. Although the production processes of coils and plates are similar, their requirements are different, so they are produced separately.
Figure 1. The steel production process.
This figure explains the entire steel production process, which consists of iron-making, steel-making, continuous casting and rolling.
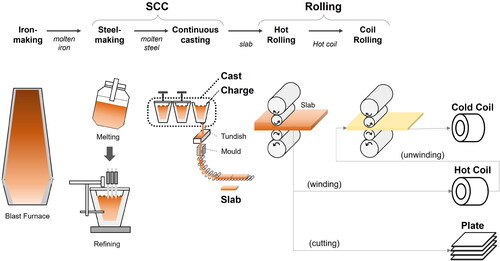
Not all steel plants have production facilities that cover the entire steel production process. A steel plant with all the processes is called an “integrated steel mill”; it produces molten iron through a blast furnace and produces molten steel from molten iron through a basic oxygen furnace (BOF). On the other hand, steel plants called “mini-mills” produce steel by melting steel scrap using an electric arc furnace (EAF). Notably, 75% of steel produced worldwide is produced in integrated mills, whereas 25% is produced in mini-mills (Sun, Wang, Zheng, et al., 2020; Sun, Wang, Zhou, et al. 2020). Both integrated steel mills and mini-mills use continuous casting processes when producing slabs from molten steel. Since molten steel cannot be stored, it must go through the continuous casting process without any interruption; the continuous casting process comes immediately after the steel-making process. Some companies may not have steel-making facilities but do have rolling plants. They purchase slabs (or hot coils) from other steel companies and roll them to produce coils and plates. categorises the phases of steel production according to the production facilities being utilised. A distinction is made between three phases of the steel production process. The optimisation problems incorporating multiple phases have not been analysed yet in detail because the problems within each phase separately are already very complicated.
Table 1. Steel plants classification.
Our focus is on the steel-making and continuous casting (SCC) processes in Phase II. They are considered bottleneck processes that do affect overall productivity (Tang et al., Citation2002). We consider in what follows planning and scheduling problems in SCC processes. The planning problem has as objective to transform orders into production batches (e.g., in the form of slabs, charges, and casts) for each production process. Since intermediate and final products are produced in batches, planners must consider certain physical and chemical characteristics of the orders and intermediate products, and the relationships between batches in the different processes. The scheduling problem determines the machine assignments and the start times of the batches in each process. Since the planning problem’s output is the scheduling problem’s input, the two problems are interrelated. However, considering both problems together is highly complex, and only a few such attempts have been made in the past. Although both problems regard productivity and customer satisfaction as their main objectives, they are formulated differently. We collect and summarise papers dealing with SCC planning and scheduling to obtain insights into each phase and discuss the potential of integration.
To the best of our knowledge, no survey has appeared reviewing planning problems in SCC processes; a few papers have provided surveys on scheduling problems in SCC processes. Tang et al. (Citation2001) and García-Menéndez et al. (Citation2020) reviewed scheduling problems in SCC processes. Tang et al. (Citation2001) described SCC processes and the related scheduling problems clearly, but the problems they considered were relatively simple since it was published two decades ago. García-Menéndez et al. (Citation2020) focused on reporting trends in SCC scheduling problems with a statistical analysis of the characteristics considered. Thus, they did not explicitly formulate optimisation problems nor present a comparative analysis of the various methodologies. Hong et al. (Citation2022) recently reviewed SCC scheduling papers, including constraints, objectives, and methodologies. In this paper, we provide a comprehensive and critical survey on SCC planning problems and SCC scheduling problems, focusing more on the limitations of past research, and propose future research directions.
There are surveys that consider the other phases of the production process. For Phase I, a few of the optimisation problems, such as the gas byproduct problem (Kim et al., Citation2003), and the blast furnace control problem (Ariyama & Sato, Citation2006), have been considered. van Beek (Citation2018) comprehensively reviewed the problems of this phase. Phase III involves planning and scheduling problems in rolling processes, such as Cowling and Rezig (Citation2000). Several papers surveyed optimisation problems in the various phases. Dutta and Fourer (Citation2001) presented a survey of optimisation problems in the steel industry based on case studies. Xu et al. (Citation2021) reviewed deterministic optimisation problems and Iglesias-Escudero et al. (Citation2019) reviewed optimisation problems under uncertainty. Although they do cover SCC planning and scheduling problems, they do not go into much detail due to the broad scope of the surveys.
We find some areas where collaboration between academia and industry with regard to SCC planning and scheduling should have been closer. We believe such missing links are the primary limitations of existing studies. We also propose future research directions to overcome such limitations. For the planning problem, previous papers tend to focus too much on finding critical features and theoretical structures that fit well within known optimisation formulations. Thus, these papers generally deal with problems that exclude several practical (but important) features, and algorithms to solve them may work well only in very few environments. We propose several practical planning problems and more generalised solution methods that may be of more interest to the industry. For the scheduling problem, on the other hand, previous papers mainly focused on solving plant-specific problems. The relative importance of theoretical structures and practical features of problems have rarely been considered; solution methods applied to other generic scheduling problems, which may also be effective for SCC scheduling problems, have not been commonly utilised. Therefore, the versatility of the proposed methodologies is generally not guaranteed, and any comparison among them may not be straightforward. We focus on the essential features of the scheduling problem and discuss previous solution methods in detail, which may help establish a more formal problem structure. We present the most promising algorithm in the existing literature and offer ideas on how to improve this algorithm by considering generic solution methodologies that have been applied to other scheduling problems similar to the SCC scheduling problem. We also propose several problems as future research topics.
The contribution of this article is the following. We formally define the planning and scheduling problems related to SCC processes focusing on their objectives, constraints, and solution methodologies. The proposed taxonomy can help practitioners and theorists communicate with each other. Since some papers dealt with optimisation problems in specific plants, it is hard to consider every problem with all its specific features. Therefore, we propose two core models that take into account the essential and most common constraints and objectives that may be meaningful to experts in both industry and academia. We classify problems that consider additional practical features based on the models presented. We investigate the methodologies used for each problem in detail and identify various decomposition strategies that are being used. We also highlight the decomposition approaches that may be useful for solving problems to be encountered in the future. We summarize previous papers on each problem and propose future research directions to overcome specific limitations.
The remainder of this article is organised as follows. In Section 2, we explain SCC processes in more detail and the business processes directly related to the planning problem. We summarise and categorise the methodologies used in selected papers. In Section 3, we subdivide the planning problem into two separate problems and analyse their objectives, constraints and methodologies. In Section 4, we introduce a core SCC scheduling problem having the more essential features and review problems with additional practical features. We also analyse problems under uncertainty and with energy considerations. Finally, we summarise the results and present the implications of the survey, including new research opportunities in Section 5.
2. Preliminaries
2.1. Planning and scheduling processes
In this section, we describe the various steel production processes in more detail. shows SCC processes that consist of steel-making and continuous casting. The steel-making stage is further subdivided into melting and refining processes. Firstly, iron is purified and turned into steel in either by an EAF or a BOF through a reduction of oxygen in the melting process. Then, the steel is brought to the refining process via ladles, and its micro-ingredients are adjusted. Refining has as goal a homogenisation of the steel charge with regard to temperature and chemical composition, as well as an increase in the alloy yield, and decarburisation, etc. (Chakrabarti, Citation2006). The final chemical composition of the steel coming out of the refining stage is referred to as the steel grade and it satisfies given metallurgical characteristics. Each plant may have its own distinct refining processes (Kikuchi, Citation2020), and charges must go through some, if not all, of them in order to satisfy customers’ requirements. The sequence of the refining processes of a charge is called a “route.” For example, Missbauer et al. (Citation2009) considered a plant with three distinct refining processes, namely Conditioning Stand (CS), Ladle Furnace (LF), and Ruhrstahl Heraeus (RH) (Vacuum degassing units). The possible routes include just LF, just RH, LF → RH, CS → RH, etc. After refining, the molten steel in a charge is brought to a continuous caster and poured into it. The tundish is the buffer between the charge and the mould, which controls the speed of the steel flow and plays a vital role in maintaining the quality of the continuous cast steel (Sahai, Citation2016). The molten steel flows into moulds and is converted into slabs. In the rolling process, the slabs are transformed into coils and plates. Although rolling is not within the scope of this article, we have to understand the rolling process for planning purposes. shows the rolling processes for each product.
Figure 2. Adjusting the widths of coils and plates.
The figure shows the rolling process of coils and plates.
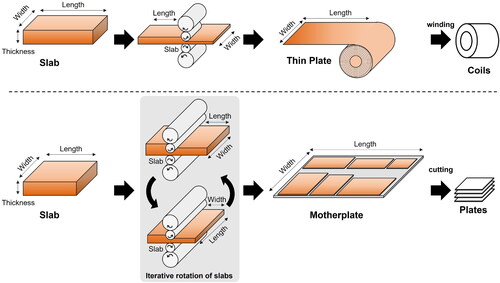
As shown in , a slab has a width, length, and thickness. Since all slabs from one cast go through the same mould, they must have the same thickness and width but may have a different length. However, the width of slabs can be adjusted in the rolling process when producing the final products to be made, implying that the slab’s width and that of the final products do not have to be the same (Tang et al., Citation2014). For coil products, only rolling in the length direction can be done, and a little trim is allowed for width adjustment. Thus, the allowable width range of slabs when producing coils is relatively narrow. On the other hand, for plates, rolling can be done in both the length and width directions. Transforming slabs to the desired plate shape is possible, and thus the width range of these slabs may be relatively wide. The rectangular intermediate sheets for plates converted from slabs are referred to as motherplates. A motherplate is cut into multiple plates.
Before production begins, planners must decide the order configuration and the number of casts to generate to fulfil orders. This business process is called a planning process, and the optimisation problem in this process is called a planning problem. illustrates the planning process. When a customer places an order (for coils or plates), the company first tries to fulfil the order by either matching it to existing slabs in inventory or by creating new ones (Dawande et al., Citation2004). If an existing slab is used, its dimension is predetermined. However, when a slab is newly made, its shape can still be determined. Coil products are assigned to slabs based on the size in the length direction (1-dimensional (1D)). Plate products are assigned to motherplates, based on the width and length sizes (2-dimensional (2D)). Afterwards, slabs are combined into charges and casts. Since a cast is a sequential batch of charges, the sequence of charges within a cast must also be determined. Other details with regard to this problem are discussed in Section 3.
Figure 3. The planning process.
It is a figure explaining the planning process, which consists of product design into slabs and slab batch into charges and casts.
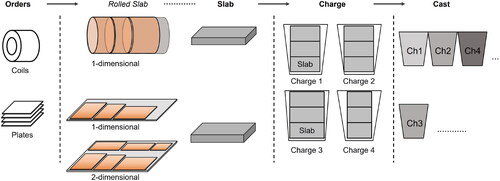
The SCC planning problem has as goal the assignment of orders to charges and casts, and the SCC scheduling problem establishes a production schedule of the charges and casts. In the planning problem, planners decide on order assignments to slabs, slab assignments to charges and charge assignments to casts. Afterwards, engineers create a production schedule of charges and casts, determining machine assignments, sequences, and start times. The two problems are closely related since the output of the planning problem (charges and casts configuration) is an input to the scheduling problem. In practice, however, the two problems are solved sequentially since each problem is hard. Besides, the decision-makers who have to deal with the two problems may reside in different departments. The planning decision-making is close to the sales department whereas the scheduling decision-making is close to the operations department. Therefore, we consider the two problems in Sections 3 and 4 separately, and discuss integrated versions in Section 4.4. explains the list of abbreviations used in this paper; see Appendix C for detailed meanings of technical terms.
Table 2. List of abbreviations.
2.2. Methodologies
Before discussing the papers, we classify the methodologies used to solve the problems and propose abbreviations. Our methodology classification is as follows.
Exact methods: Methods that solve problems optimally. Modelling-based approaches (e.g., Mixed Integer Programming (MIP), Constraint Programming (CP)) and commercial solvers are frequently used.
Mathematical programming heuristics: Methods that use mathematical models as either a module or a subroutine. The Lagrangian relaxation method (Fisher, Citation1981) is a popular example.
Heuristics: Methods that find a feasible solution in a short time using a combinatorial algorithm. One type of such a generic algorithm is a simple priority rule (or greedy algorithm) or a combination of such rules. The other type is an application-specific heuristic for a specific problem utilizing its unique features.
Metaheuristics (single-solution): Methods that iteratively improve a given initial solution based on neighborhood search. Tabu search (Glover & Laguna, Citation1998), restricting some solution moves, is an example.
Metaheuristics (population-based): Metaheuristic methods that generate a solution pool (multiple solutions). Each solution is represented as a particular encoding and evaluated heuristically via a decoding algorithm. The solution pool is updated through a neighbourhood search, merging distinct solutions to escape from local optima. A genetic algorithm (Whitley, Citation1994) with crossover and mutation procedures is a popular example. For multi-objective problems, solutions are selected to approximate a Pareto frontier.
We classify methods according to the five types described above. For ease of classification, we denote each type of method by a number. summarises the methods considered in this article and their abbreviations.
Table 3. The classification of solution methods.
3. Planning problems
We first describe the planning problems in SCC processes. As shown in , planning problems fall mainly into three categories: assigning orders to slabs, assigning slabs to charges and assigning and sequencing charges within casts. The optimisation problem of assigning customer orders to slabs is called the slab design problem, and assigning slabs to charges and charges to casts are called the charge batching problem and the cast batching problem, respectively. Although the slab design problem is closely related to the other two problems, we consider them in this paper separately.
The most critical aspects of the planning problem are the trade-offs between well-known objectives. The primary objectives for the planning process are sales maximisation, cost minimisation, and maximisation of customer satisfaction. Inserting as many orders as possible into casts is desirable for sales maximisation. Reducing the slacks of intermediate products (e.g., slabs), maximising an efficient use of expensive parts (e.g., tundish), and minimising the dissimilarity costs between orders are critical for cost minimisation. Meeting the due dates of orders is important for customer satisfaction. Since trades-offs between these objectives are heavily dependent upon by the production environment, each plant uses specialised methodologies for its planning problems.
3.1. Slab design problems
The slab design problem assigns orders to slabs taking many practical considerations into account. The target slabs in the problem may either be newly generated or already in inventory. While integrated mill and mini-mill companies can design new slabs based on their current orders, rolling companies can only buy slabs from other companies and match their orders to the slabs they purchase. The slab design problem may be of one of two types. One type is the problem of assigning orders to slabs of a fixed size, and the other type is the problem of assigning plate orders to a (2D) motherplate. Although plate orders have 2D sizes, the design problem can be simplified as a 1D problem.
A 1D slab design problem assigns orders to slabs with a size constraint. Except for Dawande et al. (Citation2004), all the problems have assumed slabs in inventory, so their size is a fixed constant. Maximising the profit of assigned orders and minimising the residual part (slack) of slabs (or minimising the number of slabs) are important objectives. Since the problem may deal with both coil and plate products, the types of orders are in general not explicitly specified. However, Balakrishnan and Geunes (Citation2003), Song (Citation2014), and Kasapinov et al. (Citation2014) noted that their orders were plate orders and assumed some specific practical properties. On the other hand, Vasko et al. (Citation1994) and Luo and Tang (Citation2005) assumed an arbitrary set of orders instead of unit orders and assigned slabs to such order sets.
summarises the design problems according to production environment (Env.), objectives (Obj.), and algorithms (Alg.). Some papers consider eligibility constraints between orders and slabs due to their physical properties (width, thickness) or their chemical (steel grade) characteristics. After having undergone the rolling, orders in a slab may be moved to different locations in the plant dependent upon the finishing process, which is abstracted as a “colour” for an order. If a slab contains orders with two different colours, then it must be cut and sent to another site in the plant. The colour constraint on slabs implies that a slab cannot have orders with more than two colours to restrict the time-consuming and cumbersome cutting process.
Table 4. Slab design papers.
About half of the papers consider variable-sized slabs, which either may be provided as multiple fixed (F) candidates or may be freely adjusted (A). Only Dawande et al. (Citation2004) considered adjustable slabs in a 1D slab design problem and viewed slab size as a decision variable. Order sizes can also be adjusted considering physical restrictions (minimum and maximum size) imposed by the facilities’ constraints. In some cases, when the residual weight of a slab is more than a given value, it can be held in inventory and reused. In this case, recycling to minimise the slack of slabs is a possible strategy.
summarises the methodologies used in the batching problem. Since the slab design problem is more complex than the bin packing problem of Garey and Johnson (Citation1979), the problem is NP-hard. Specifically, the core problem can be viewed as a knapsack problem with colour constraints and open problem instances were first introduced in MIPLIB (https://miplib.zib.de/instance_details_mkc.html). The slab design problem is a problem of more theoretical interest, so methodologies taking advantage of specific problem structures are of interest. Thus, only Luo and Tang (Citation2005) proposed a single-solution metaheuristic that consists of an initial greedy heuristic followed up by tabu search.
Table 5. The number of papers on classified methodologies for the slab design problem.
Since the slab design has been included in CSPLib (https://www.csplib.org/), which is a library of test problems for constraint solvers, the problem has been solved through constraint programming. After Frisch et al. (Citation2001) presented a constraint programming approach, many other papers have proposed methods to improve the quality of solutions. For example, Schaus et al. (Citation2011) used constraint-based local search to delete symmetry. Other exact methods based on MIP have been adopted by Dawande and Kalagnanam (Citation1998) and Crévits et al. (Citation2019). Dawande and Kalagnanam (Citation1998) proposed valid inequalities describing the structure of the problem and Crévits et al. (Citation2019) proposed a MIP model for special cases.
As far as the non-exact methods are concerned, heuristic approaches based on specific problem characteristics are widely used. For example, Kalagnanam et al. (Citation2000) proposed a reformulation by splitting the maximum order weight into multiple production units of equal size and applying a matching-based heuristic. Similarly, Vasko et al. (Citation1994) proposed a transportation problem formulation and applied a rounding heuristic to the solution. Mathematical programming heuristics are also widely used. They consist of a formulation of the problem, a strengthening of the formulation, and a decomposition. For example, Balakrishnan and Geunes (Citation2003) used Lagrangian relaxation that allows an order to be assigned to more than one slab. Forrest et al. (Citation2006) applied column generation with the subproblem being a simplified knapsack problem.
Although a 1D slab design has been applied to plate orders, it may result in a larger slack, especially when the plates’ widths are significantly smaller than those of the motherplates. This motivates a slab design problem based on a 2D packing of orders. This problem deals with the assignment of 2D plate orders to a 2D motherplate. Because the plates’ thicknesses assigned to the same motherplate must be identical, the problem aggregates plates taking this into account in a preliminary step.
A guillotine cut is a cut from one end of the motherplate to the other end, without cutting any of the assigned plates. depicts a guillotine cut constraint. If plates in a motherplate are assigned in a non-guillotine shape, cutting the motherplate becomes more difficult requiring specialised equipment. Thus, guillotine cut constraints are usually imposed on motherplates. Segments of a motherplate created by guillotine cuts are referred to as levels of the motherplate. Rotating plates and packing such plates in a motherplate are typically not considered, because the length of a plate is usually larger than the width. Moreover, rotating could affect the quality of the plates. Plants that put a heavy weight on a minimisation of the slack of slabs may allow rotation of plates.
Figure 4. Guillotine cut constraint.
The figure explains a guillotine cut in a 2-dimensional rectangular sheet.
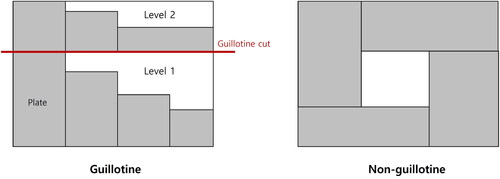
The 2D slab design problem is a famous variant of the ordinary 2D packing problem. Specifically, the problem is a 2D variable-sized orthogonal bin packing problem with guillotine cut constraints (Furini & Malaguti, Citation2013). Due to its wide applicability, many methodologies have already been developed in previous papers for 2D packing problems. Lodi et al. (Citation2002) and Iori et al. (Citation2021) have provided survey papers on this topic. There are 2D slab design problems assuming a steel production context. Vasko et al. (Citation1989) and Vasko et al. (Citation1991) solved a practical problem at Bethlehem Steel via a heuristic. Mehdi et al. (Citation2022) solved an online problem at Artco Steel via a heuristic method.
A recent variant of a 2D slab design problem in a steel production environment deals with the assignment of plates to motherplates with the sizes of the motherplates being adjustable. In this problem, a motherplate has a maximum area constraint, and one can adjust its width and length within a given range. Park (Citation2007) formulated the problem as a MIP and proposed a level-packing heuristic algorithm. He first used a heuristic to assign plates to create levels and then heuristically assigned the levels (partial configurations of motherplates) to motherplates. Wy and Kim (Citation2010) solved the same problem using an improved level-generation algorithm based on weighted bipartite matching. After that, Zhang et al. (Citation2021) solved a variation that allows for rotation through column generation; they also used a heuristic approach to reduce the dimensions of the pricing sub-problem. Two other papers considered additional practical features: Dash et al. (Citation2007) used column generation to solve the problem with various other practical considerations, including due dates. Recently, Zhao et al. (Citation2023) solved the practical problem that takes into account the detailed physical constraints of the plates assigned to the same motherplate, such as the maximum difference of the widths of the plates at the same level. They formulated the problem as a mixed integer nonlinear programming model with generalised disjunctive programming constraints and suggested a logic-based outer approximation algorithm to solve the problem.
We find that the production environment determines the ranges of the sizes of the motherplates as parameters or decision variables. Plants with just a few plate types achieve high productivity and minimise dissimilarity costs by maintaining a fixed set of motherplates’ sizes that are easy to produce. For plants with many motherplate types, such an approach could generate too much slack. Thus, adjusting the size of the motherplate may be required, even allowing for lower production efficiency. In practice, a compromise plan could be helpful since the order environment plays an important role. For example, a plant may decide on specific motherplate widths/lengths sizes and select one as a decision variable. Thus, engineers should consider the production environment when defining the problem.
Previous papers have mainly focused on developing algorithms for more abstract problems that only capture the core features. The performance of these algorithms was measured using known open instances. However, these studies may exclude important practical features and cannot be applied to real problems directly. We introduce several practical considerations that should be included in future research. The problem of matching slabs to order sets deserves more interest since a slab corresponds to a coil in general. The 2D slab design problem with adjustable motherplates will receive more attention and should be considered with a focus on minimising slack in the mass customisation era. A frequently used level-packing approach for solving the problem should be modified since a motherplate with more than two levels causes a heavy cutting load and is not preferable in practice. We recommend including a penalty in the objective function for adding levels to a motherplate. We also suggest considering more practical features such as Zhao et al. (Citation2023).
3.2. Charge and cast batching problems
After orders are converted into slabs, planners aggregate the slabs into charges and casts. Batching problems are further classified as charge batching, cast batching, and integrated batching. These batching problems are more sensitive to the production environment than the design problem. A batching problem formulated for a specific plant is typically hard to classify. Thus, we introduce a core model of the integrated batching problem with the most essential constraints and the most important objectives, referred to as the Steel-making and Continuous Casting Batching Model (SCC-BM). Appendix A presents a MIP formulation of the SCC-BM. Based on the SCC-BM, we can classify problems considering other practical constraints, objectives, and solution methodologies.
We first introduce the SCC-BM’s input parameters, constraints, and objectives. Each slab has a width range, a steel grade and a weight determined by its orders’ specifications. The following constraints are considered in the SCC-BM.
The width of a slab must be determined within a width range and all slabs in a cast (charge) must have a common width. The common width that is established is also called a cast (charge) width.
A maximum charge weight is in effect a capacity constraint.
A minimum charge weight is in effect for productivity reasons.
Due to the tundish’s lifespan, a cast has a maximum capacity (usually expressed in the number of charges).
The steel grades of the slabs in a charge must be identical because slabs in a charge undergo the same steel-making process.
Steel grades of adjacent charges in a cast must be the same or similar. We enforce this condition through a compatible steel grades constraint. Adjacent charges in a cast must be poured sequentially into a tundish. If the two charges have completely different steel grades, a transition zone may result in a productivity loss.
illustrates the SCC-BM with an example. The SCC-BM considers width, capacity, and steel grade constraints. Specifically, a width constraint is a major difficulty since width ranges for slabs with multiple steel grades that have compatible relationships should be considered simultaneously. As shown in the figure, there are 15 slabs as input, and 3 charges and 2 casts as output. Each slab has its steel grade and width range. For example, slab 1 has steel grade SG1 and width range [w1, w4]. Regarding all slabs, there are two steel grades (SG1 and SG2) and nine candidate widths (w1–w9). We produce three charges and two casts, and two slabs ({6, 7}) are not used. Charge 1 consists of slabs {1, 2, 3, 4, 5} and its steel grade and width are SG1 and w4, respectively. Charge 2 consists of slabs {8, 9, 10} and its steel grade and width are SG2 and w4, respectively. Charge 3 consists of slabs {11, 12, 13, 14, 15} and its steel grade and width are SG2 and w7, respectively. Cast 1 consists of Charges 1 and 2, and Cast 2 consists of Charge 3 only.
Figure 5. An illustrative example of the SCC-BM.
It is a figure explaining batching from slabs to charges and casts considering width and steel grade constraints.
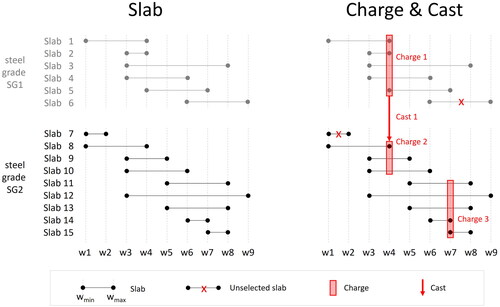
We propose two main objectives for the SCC-BM. One is to assign as many slabs as possible to charges. Every paper has either as an objective the maximisation of the weight of assigned slabs (or, equivalently, the minimisation of the weight of unassigned slabs) or the constraint of having to assign all slabs. Another objective is to maximise the utilisation of a tundish since its fixed cost is very high. Thus, minimising the number of casts or maximising the number of charges in a cast is of interest. Even though the width range of a slab could be considered continuous in an abstract sense, the width range for each slab is given as a set of specific width sizes because of technical limitations in practice (Tang et al., Citation2014; Lee et al., Citation2023). Note that the formulation of Lee et al. (Citation2023) is an extension of the SCC-BM.
lists other practical features that have been considered in the literature. Constraints and objectives are abbreviated as (con) and (obj), respectively. Five practical features are considered for each type of batching problem. Among these features, three are related to the similarities of the slabs in a batch (charge or cast). Although we assume that a batch has only one width in SCC-BM, in practice, shrinking the width during the continuous casting process is in some plants possible with a specific type of mould. A batch may contain then multiple widths even though there may be restrictions on the number of distinct widths in a batch, the minimum number of slabs with the same width, etc. Shrinking width creates trapezoid-shaped slabs in the production process, and parts of them would be waste. Thus, the differences between widths are penalised. Slabs have due dates based on their order specifications. Since slabs in a cast are produced around the same time, minimising the difference in due dates between slabs is considered an objective in some papers. Some papers consider the production route similarity of slabs in a cast to prevent congestion during the production process.
Table 6. Charge and cast batching papers.
For a stable operation, balancing production capacity between facilities is required. Since every slab has a predetermined production route based on its order specifications, the production amount sent on each production route can be determined in the batching process. Some papers consider minimum and maximum weight constraints on slabs for each production route. Another condition of a continuous caster may also be considered: A strand refers to the path of the molten steel after it passes through the mould to form a slab. Some continuous casters have two strands and a mould for each strand so that a charge flows to both strands. The slabs in a charge that emanate from one of the two strands are referred to as a half-charge. Although all slabs in a half-charge must have the same width, the widths of the two strands can be different. Some papers consider the constraint that the completion times of the steel flows in each strand must be synchronised within a given time span (Box & Herbe, Citation1988; Lee et al., Citation2023).
The charge batching problem considers two practical features. A certain number of slabs is required to batch the slabs as a charge. However, since slabs in a charge must have the same steel grade, slabs with uncommon steel grades are hard to batch. To deal with this situation, one can replace the steel grade of a slab with a higher one. Although this could increase productivity, such an upgrade involves a cost, so the trade-offs between these costs have to be carefully analysed. Another way to resolve the situation is to use open slabs, which are slabs that have not yet any order assigned to it. Since these open slabs will be used for filling future orders, their specifications are much more flexible. Since creating open slabs incurs inventory holding costs, and may increase waste in any matching, a similar trade-off must be analysed.
The cast batching problem considers three practical features. Some plants consider the minimum number of charges as a constraint to ensure a certain level of the tundish utilisation. When width changes in a cast are possible, some plants restrict the number of width changes to control the costs. Some plants consider grade compatibility only as a 0-1 variable; other plants consider the combination of different widths a cost.
Since batching problems are hard to solve optimally, non-exact methods are often used. A formal proof of NP-hardness of the charge batching problem can be done by a reduction to the bin-packing problem (Garey & Johnson, Citation1979), and that of the cast batching problem by a reduction to the Hamiltonian path problem (Tang et al., Citation2014). Two papers used exact methods to solve batching problems. Lee et al. (Citation2004) proposed a polynomial-time algorithm for a specialised charge batching problem; they considered slabs with one steel grade and analysed the problem of assigning all slabs that are partially usable to a minimal number of charges. Tang et al. (Citation2014) considered a cast batching problem with width change constraints. They applied a branch and price algorithm to exactly solve the problem, and used a structural property to reduce the solution space of the pricing sub-problem. summarises the methodologies used for the batching problem. Note that no pure heuristic methods have been applied to batching problems since improving a solution with other metaheuristics is straightforward and effective.
Table 7. The number of papers on classified methodologies for each batching problem.
For the charge batching problem, population-based metaheuristics are mostly used. For a representation of the problem, a permutation of the slabs is usually considered; see Xue et al. (Citation2005) and Yuan et al. (Citation2020). The decoding algorithm sequentially assigns slabs, accumulates weight, and then creates a new charge when the weight exceeds the weight limit. Yang and Li (Citation2014) transformed the problem into a Travelling Salesman Problem (TSP) and applied a cross-entropy algorithm. The solution is improved through either insertions or swaps of slabs. Another approach is through Lagrangian relaxation. After Tang et al. (Citation1996) formulated a MIP model, Tang and Jiang (Citation2009) proposed a Langrangian relaxation. They relaxed the constraint that a slab can be assigned to only one charge and solved the subproblem via dynamic programming. Li et al. (Citation2019) and L. Sun et al. (Citation2020) used an augmented Lagrangian relaxation method for the same MIP model with more objectives. Other methodologies include single-solution metaheuristics. For example, Dong et al. (Citation2010) used a modified next-fit decreasing algorithm as a constructive heuristic and applied a neighbourhood search similar to a population-based metaheuristic. Tang et al. (Citation2011) and Yi et al. (Citation2021) proposed a set of patterns, which are combinations of slab widths and steel grades and then developed pattern-based models and algorithms to handle the pattern variables.
For the cast batching problem, various methods have been used. Two papers used a column generation algorithm that consists of a pattern-based master problem and pattern-generating subproblems. Chang et al. (Citation2000) considered a complex master problem and derived simple bounded-variable knapsack subproblems. Yi et al. (Citation2019) considered a master problem in the form of set-partitioning and solved complex subproblems through a MIP model. Tang and Luo (Citation2007) and Dong et al. (Citation2012) applied a single-solution metaheuristic that consists of greedy initial heuristics and neighbourhood searches via insertions and swaps of charges. Long et al. (Citation2018b) solved the batching problem with a variable neighbourhood search and then solved the scheduling problem by directly solving a MIP. Xue et al. (Citation2006) and Zhang et al. (Citation2011) transformed the problem into a TSP and then applied population-based metaheuristics.
For the integrated batching problem, three papers used matheuristics in which mathematical programming is used in some parts of the algorithm. In Dash et al. (Citation2007) and Song (Citation2014), mathematical programs were used with other heuristic algorithms. Lee et al. (Citation2023) decomposed the problem into two subproblems, and formulated and solved these as two different MIP models. Tang and Wang (Citation2008) applied a greedy heuristic to the charge batching problem and used the solution as an input to the cast batching problem. They applied tabu search to solve the cast batching problem with some constraints being relaxed to get a feasible solution. Ma et al. (Citation2014) considered a problem of assigning slabs to charges, charges to semi-casts, and semi-casts to casts. They applied a simple dispatching algorithm of grouping slabs to semi-casts and then an ACO algorithm to generate casts. Ogata et al. (Citation2015) and Y.-Z. Wang et al. (Citation2022) proposed a framework for assigning slabs to casts and dividing casts into charges. Yadollahpour and ArbabShirani (Citation2016) proposed a two-phase complex integrated batching problem: in the first phase, they used constraint programming to aggregate orders into casts, assign them to continuous casters, and determine the start times of casts; in the second phase, they used a heuristic to divide casts into charges.
We find that proposed solution methodologies may presume an input with little diversity in widths and steel grades. More than half of charge batching papers applied constructive greedy algorithms, and more than two-thirds of the papers sequentially solved the charge and cast batching problem. Such greedy approaches may yield solutions that may be far from the global optimum solution especially when the input data has a high diversity. The greedy method for charge batching with heterogeneous orders may increase the number of open slabs. For the integrated batching problem, more slabs being assigned to charges implies more widths being selected in charge batching. However, charges with widths selected only for charge batching are not likely grouped into a small number of casts; solving the charge and cast batching problems separately usually generates a solution far from the global optimum. It highlights the necessity of an integrated problem to deal with diversified slabs, carefully considering the trade-offs between two core objectives. We also recommend considering the bi-strand condition since two common widths in a charge help handle more diversified width ranges of slabs. Although various solution methodologies have been developed according to the relative importance of the two core objectives, the efficient utilisation of tundishes is generally considered more critical since not only is the fixed cost of a tundish high, but it also reduces the setup time, which would increase production efficiency (Tang et al., Citation2014).
A promising solution methodology has been presented in the literature considering this, and which can be applied to single and bi-strand conditions. The key idea is to create intermediate containers called frames that correspond to charges and casts with predetermined widths and steel grades. This approach can decompose the problem into two parts: generating frames based on information of slabs and assigning slabs to the generated frames. Since a frame’s width and steel grade is given, eligibility relationships between slabs and frames have to be determined; assigning slabs to these frames can be done quickly. There are two approaches for generating frames. One approach is to introduce pattern variables corresponding to every frame. Tang et al. (Citation2011) considered the new batching unit (viewed as a frame) “charge lot” of a candidate width and steel grade. They then proposed a MIP formulation determining the assignment of slabs to such charge lots. They combine a Lagrangian approach that relaxes constraints for the single assignment of slabs and then apply column generation to solve the problem. Yi et al. (Citation2021) formulated the problem as a pattern-based MIP model and applied a genetic algorithm to the model. Another approach is to create a few promising frames by considering the ranges of widths and steel grades for the input slabs. Dash et al. (Citation2007) created half-charges for the bi-strand problem and generated charges through matching. Then, they used an extended branch and bound algorithm to create cast frames and used a MIP model to select suitable candidates. Lee et al. (Citation2023) aggregated slabs with the same steel grade and the same width range, and called such units “slab groups,” which are fractionally used to generate frames. After frames have been created, both papers use a MIP model to assign slabs to the frames generated. These solution methodologies, especially the second one, can capture the overall configuration of slabs with a few promising frames; generate a decent solution in a reasonable time, even with highly heterogeneous slabs.
4. The scheduling problem
The operational problem of generating a production schedule for charges and casts is called the SCC scheduling problem. We formulate a core model that includes the most common features found in the literature. Other practical features, including uncertainty and energy-related issues, are also discussed.
Recall that SCC processes comprise melting, refining, and continuous casting processes. Since each stage may have multiple machines, the SCC scheduling problem may be considered a variant of the hybrid flow shop scheduling problem, which focuses at each stage on machine assignments and sequences of charges. The first stage corresponds to the melting process and the last stage corresponds to the continuous casting process. There may be one or more stages in between corresponding to refining processes. Several surveys on hybrid flow shop scheduling have appeared in the literature since the problem is relevant in many other production environments, see Linn and Zhang (Citation1999), Ribas et al. (Citation2010), Ruiz and Vázquez-Rodríguez (Citation2010) and Neufeld et al. (Citation2023). Although some hybrid flow shop problems considered sequential batches, such as Xuan and Li (Citation2013), the batch process in the last stage clearly differentiates the SCC scheduling problem from the standard hybrid flow shop problem. In the continuous casting process, charges in a cast must be processed in a predetermined order on the same machine without any idle times in between. If, in practice, there is an idle time between two charges in a cast (which is referred to as “cast break”), the tundish deteriorates and may need to be replaced at a cost (Niu et al., Citation2022). Thus, a cast break is usually considered a hard constraint, but its violation is sometimes considered a penalty term in the objective. Since a cast’s start time seriously restricts the start times of its charges in all previous stages, the SCC scheduling problem is more constrained than the generic hybrid flow shop scheduling problem. Thus, the methodologies applicable to the standard hybrid flow shop problem cannot be directly applied to the SCC scheduling problem.
In Section 4.1, we present the deterministic SCC scheduling problem without taking into account any uncertainty or energy considerations. The papers that consider the problem with uncertainty are discussed in Section 4.2, and the papers that consider energy-related issues are discussed in Section 4.3. Finally, a few papers that consider integrated planning and scheduling problems are analysed in Section 4.4.
4.1. Deterministic SCC production scheduling
In this section, we propose a core model for the SCC scheduling problem and then classify papers dealing with deterministic SCC scheduling according to the practical features they subsume. The core model for the SCC scheduling problem is referred to as the Steel-making and Continuous Casting Scheduling Model (SCC-SM). The features considered in the SCC-SM are the following.
All charges follow the same route.
Each charge has a transportation time between two consecutive stages.
All machines in a stage are identical.
The intermediate buffers between stages are unlimited.
The sequence of charges in a cast is predetermined and the charges are processed sequentially without any idle time in the continuous casting stage.
A sequence-independent setup time is required before processing a cast.
After a charge has been transported from one stage to the next, it should be processed without any delay. If a charge has to wait for a long time, it has to be heated in the intermediate buffer to prevent a solidification of the steel, which may affect the quality and result in a high energy cost. A variable called “waiting time” or “sojourn time” is used in the SCC-SM in order to minimise various objectives (e.g., heat loss). The waiting time of a charge is the time between the completion time at one stage and its start time at the next stage minus its transportation time. Sojourn time is the time between the completion time at the first stage and the start time at the last stage. Note that minimising these two objectives are equivalent unless waiting times have different weights dependent upon the stage. Since delaying the start times of charges does not affect the waiting time objective negatively, one or more objectives, such as makespan, total completion time, etc., are also included to prevent any unnecessary delay. shows an example of the SCC-SM with two casts, five charges and three stages. We present a MIP formulation of the SCC-SM in Appendix B, that can be easily extended to more general settings such as Hong et al. (Citation2022).
Figure 6. An illustrative example of the SCC-SM.
The figure illustrates the core model of the SCC scheduling problem, showing transportation time, setup time and waiting time.
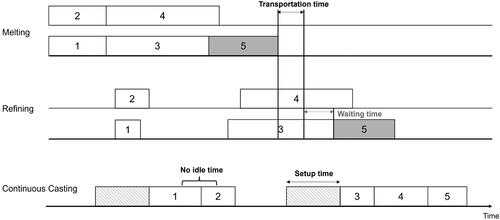
We classify papers in according to other special features that are considered in SCC scheduling. The environment (Env.) column specifies the basic features of the scheduling problem. As for Route type, “I(identical)” implies that all charges have the same route. “S(skippable)” implies that each charge has a predetermined route that may skip some refining processes, and “A(alternative)” implies the possibility of alternative routes by selecting one of multiple routes as part of the decisions for a charge. As for machine type, “D(dedicated)” implies for each charge a dedicated machine at each stage, “P(parallel)” implies identical parallel machines at each stage, and “R(unrelated)” implies unrelated parallel machines. The Ca-CC fix implies that assignments of casts to particular casters may be predetermined. This setting emerges when specifications of the continuous casters are distinct, and the sequence of casts in the dedicated caster may also be predetermined.
Table 8. Deterministic SCC scheduling papers.
We impose four constraints that are often considered. Release dates determine the earliest possible start times of charges or casts. Maximum waiting times are upper bounds on allowable waiting times between stages. Controllable processing times imply a certain freedom in determining processing times of charges at each stage (within a given range), which may be taken advantage of to prevent a violation of constraints regarding maximum waiting times or cast breaks. Ladle constraints imply constraints on the buffers between stages. It may be either due to blocking between stages (e.g., Pacciarelli & Pranzo, Citation2004) or to resource constraints on ladles as transporters (e.g., Missbauer et al., Citation2009). Generic scheduling objectives such as makespan, total completion time, total earliness, and total tardiness are usually considered with waiting time objectives. ‘W(weighted)’ and ‘S(simple)’ refer to total weighted completion time and total completion time, respectively.
classifies the papers based on the methodologies for specific route and machine environments. The table shows how the route and machine environments affect the methods used. If the environment is restricted, such as identical routes or dedicated machine environments, then exact methods are typically used. Population-based metaheuristics are mostly used when the environment gets more complicated, such as identical routes and identical parallel machine environments. However, when the environment gets even more complex, the effectiveness of this methodology gradually decreases. For example, population-based metaheuristics have been used to solve problems that assume only one skippable route in an unrelated machine environment. Moreover, only Atighehchian et al. (Citation2009) considered using this method with an unrelated machine environment. Thus, to deal with complicated problems, heuristics and matheuristics are typically used.
Table 9. The number of papers on classified methodologies for route and machine environment.
Since a 2-stage hybrid flow shop problem is NP-hard (Gupta, Citation1988), non-exact methods are more often used; however, a few papers have considered exact methods such as MIP, CP, and even LP. They formulate the problem as a MIP or CP model and directly apply a commercial solver. While most papers focus on restricted problems, Gay et al. (Citation2014) applied CP to solve the problem with skippable routes. Some papers, such as Takahashi et al. (Citation1989) and Tang et al. (Citation2000), used LP to solve the restricted scheduling problem with machine assignments and charge sequences at each stage being predetermined by an expert’s know-how.
Population-based metaheuristics have been mostly used for moderately constrained problems. For the representation of the solution, a charge (job) permutation encoding is frequently used since more complicated encodings tend to yield poor results (Oĝuz & Ercan, Citation2005). Charges in an encoding are sequentially assigned to the first available machine in the decoding process. Because of the sequential batch in the last stage, SCC-specific encodings have also been proposed. Jiang et al. (Citation2015) considered permutations of casts that contain sub-permutations of charges within the cast, representing the charge sequences and processing speeds for the respective cast. Similarly, Pan (Citation2016) proposed an encoding that represents casts and charges indicated by the cast index. Note that a key difference is that Pan (Citation2016) allows for alternatively sequencing of charges in different casts, while Jiang et al. (Citation2015) do not. More sophisticated encodings have also been proposed, including for each stage charge sequences (Long et al., Citation2018c; Li et al., Citation2018) or machine assignments (De Moerloose & Maenhout, Citation2023).
In the hybrid flow shop problem, list scheduling could work well, as shown by Hakimzadeh Abyaneh and Zandieh (Citation2012). Thus, Li et al. (Citation2014) applied this decoding algorithm to the SCC scheduling problem. However, as Jiang et al. (Citation2015) noted, since preventing a cast break in the last stage is important, applying this decoding heuristic to SCC scheduling could yield unsatisfactory solutions. Thus, other papers have frequently used the decoding method referred to as forward-backward scheduling by Pan et al. (Citation2013): They first sort the charges in non-decreasing order of their start times, which had been determined by considering the target due dates of the casts; then, they schedule in a forward manner each charge to its earliest start time at each stage. They end up fixing the last charge’s start time at the last stage for each cast and modify other charges’ start times to prevent cast breaks at the last stage. There are papers that used the same decoding algorithm (Peng et al., Citation2018) or a modified version of the algorithm (Long et al., Citation2016, Citation2018c). For the cast permutation encoding of Pan (Citation2016) and Jiang et al. (Citation2015), a backward scheduling algorithm was proposed. The algorithm first fixes the start time of the cast at the last stage, and it creates in a backward manner a schedule of charges. Then, the algorithm adjusts the start times of charges to attain feasibility or to improve the solution. A more complicated decoding algorithm must be used for papers that considered the maximum waiting time constraint. Thus, only a few papers applied population-based metaheuristics to problems with such a constraint. To consider this feature, a more sophisticated decoding algorithm is required. For example, see Fazel Zarandi and Dorry (Citation2018), who determined the machine assignments in a first phase and applied a fuzzy LP in a subsequent phase.
Swapping and inserting a charge (Han, Tang, Zhang, and Li, Citation2020) or charges (Li et al., Citation2014) have been considered in order to generate neighbouring solutions. The same movements have also been applied to casts (Li et al., Citation2018). The mutation technique of gene-based algorithms, such as Sbihi and Chemangui (Citation2018), represents such a process. Solutions that have not been improved upon in a number of generations may be replaced to enhance the variety of solutions. For example, Peng et al. (Citation2018) considered random swapping in these solutions. Regeneration of sub-populations (Pan, Citation2016) and cross-overs in gene-based algorithms (Wang et al., Citation2021) are examples of such approaches. Long et al. (Citation2016) proposed two LP models to enhance the quality and diversity of solutions, respectively. The solution pool is updated via a roulette wheel selection process considering its fitness. Although these methods work well for the makespan and earliness and tardiness, it is hard to avoid with such limited movements being stuck in local optima with the total (weighted) completion time objective. Thus, no previous paper has used such methods for this objective.
Mathematical programming heuristics have frequently been used, especially for more complicated problems. The Lagrangian relaxation method is the one mostly used among the methodologies in this category and it consists of constraint relaxation, subproblem solving, Lagrangian multiplier updating, and conflict resolution. The formulation of a problem significantly affects the performance of an algorithm. Although there are many different types of scheduling formulations, only time-indexed and precedence-based formulations have been used. A time-indexed formulation includes variables for assigning charges to discretised time horizons, and the capacity considers precedences between charges on machines at each stage. In contrast, the precedence-based formulation includes variables to assign charges to machines and to determine sequences between charges. For more types of scheduling formulations, see Unlu and Mason (Citation2010).
Papers with time-indexed formulations consider three types of constraints for relaxation: (i) machine capacities, (ii) sequences between casts, and (iii) charge sequences within a cast. The number of relaxed constraints determines the decomposition of the subproblems. A problem is decomposed into job-level subproblems when all three sets of constraints are relaxed. A problem is decomposed into batch-level subproblems when the two sets of constraints (i) and (ii) are relaxed. A problem is decomposed into machine-level subproblems when only constraint set (i) is relaxed (Sun & Yu, Citation2015). Dynamic programming approaches have been applied to each subproblem to get exact solutions. As the level of relaxation is higher, solving the problem is getting more manageable, but the achieved lower bound may be less tight. Thus, choosing an appropriate level of decomposition considering the production environment is critical. For example, Tang et al. (Citation2002) chose the job-level subproblem and Xuan and Tang (Citation2007) chose the batch-level subproblem since their objectives are different. On the other hand, a precedence-based formulation cannot be decomposed into subproblems of casts or charges even though the precedence constraints of charges or casts are relaxed. Instead, these models were solved using the structures of objective functions. For example, Mao et al. (Citation2014b) decomposed objectives and solved two tractable subproblems. Cui and Luo (Citation2017), discovered a concave-convex structure in the problem’s relaxed objective and solved the problem utilizing known methods for such a structure. After solving the subproblems, the Lagrangian multipliers are updated via subgradient descent, and the algorithm solves the subproblem again with an updated multiplier. Improved updating algorithms, such as the deflected subgradient method have been proposed to evade the famous zigzag phenomenon (Mao et al., Citation2015). The algorithm merges all the solutions of the decomposed subproblems and resolves any conflict through a simple list scheduling algorithm.
Other matheuristics consist of formulating the problem as a MIP, decomposing the problem, and solving the subproblems sequentially. Harjunkoski and Grossmann (Citation2001) formulated the problem as a MIP and solved the cast-level subproblem to get a partial schedule. Then they proposed another MIP to merge these partial schedules in order to generate a feasible schedule. They also used an LP to improve the solution. In addition, Li et al. (Citation2012) proposed an event-based MIP model, which determined the start time of every event point and assigned casts to every event point. They divided the time horizon into several segments, assigned casts to each segment, and then solved the problem for each time segment separately.
There also have been papers proposing pure heuristic methods. Most of these papers separate the assignments and sequencing of charges from determining the charge start times. For example, Yang et al. (Citation2020) proposed a heuristic that consists of assigning charges to machines via a heuristic and resolves conflicts through linear programming. Other methods are developed by introducing an alternative graph (Pacciarelli & Pranzo, Citation2004) and a combinatorial auction (Kumar et al., Citation2006), based on the problem structure. For the deterministic SCC scheduling problem, only Zhao et al. (Citation2011) applied a single-solution metaheuristic algorithm.
Each paper in the literature tends to focus on a solution methodology that shows a good practical performance for a specific problem. Features that may distinguish the problem, such as a batch constraint or a waiting time objective, have not been used to derive theoretical results (e.g., lower bounds, valid inequalities) which may help in the development of solution methodologies for more general scheduling problems. In addition, only a few papers have presented solution methodologies used for similar scheduling problems (e.g., flexible flow shop, flexible job shop), which may work well for SCC problems as well. A lack of theoretical analyses makes the development of a generic algorithm difficult and may make it hard for engineers to select an appropriate algorithm. We analyse previous solution methodologies using various decomposition approaches in detail to capture the critical theoretical structures that can help with the development of an algorithm. We then introduce a promising solution methodology from previous literature, which utilises such structures as well as background knowledge regarding similar scheduling problems.
We can make a distinction between three decomposition strategies: job decomposition, assignment-sequence decomposition, and stage decomposition. A job decomposition strategy highly depends on the steel production process, which determines the schedules of each charge or cast sequentially. Numao and Morishita (Citation1991) and Tang et al. (Citation2000) were the first to propose such a strategy. They scheduled each cast and generated a sub-schedule, then merged the sub-schedules and resolved the conflicts of the sub-schedules by modifying the charge sequences and applying an LP. After that, this job decomposition idea was applied to Lagrangian relaxation methods. The basic scheduling unit, however, can be either a cast (Xuan & Tang, Citation2007), as previously considered, or it can be further decomposed into charges (Mao et al., Citation2015). This decomposition idea has also been applied to matheuristics, such as Harjunkoski and Grossmann (Citation2001) and Hong et al. (Citation2022). They both created sub-schedules for each cast and then aggregated the sub-schedules.
An assignment-sequence decomposition strategy first decides on the machine assignments and then on the charge sequences. Thus, selecting a machine and a charge sequence in each stage is the primary concern for this approach since determining the start times of charges can be done afterwards with an LP. The strategy is mostly applied to heuristic methods utilizing the problem structure. Machine assignments and sequences can be determined easily following a priority list, considering the load on the machines (Wang et al., Citation2010). A problem-specific algorithm, such as dynamic programming by Sun and Wang (Citation2013), also can be used. After that, the start times of charges are determined via an LP (Missbauer et al., Citation2009; Long et al., Citation2018c) or Nonlinear Programming (NLP) (Atighehchian et al., Citation2009).
According to stage decomposition, the schedule of the continuous casting stage is generated independently from the other stages. After determining the cast sequences at the last stage, the charge sequences in the previous stages are likely to be fixed since the charge sequences within casts are predetermined. This observation is the key idea behind this decomposition strategy. Decomposition strategies are frequently considered in decoding heuristics in population-based metaheuristics. For example, when the machine assignments and cast sequences at the continuous caster are fixed, the forward-backward scheduling of Pan et al. (Citation2013) can be directly applied. The backward scheduling approach of Pan (Citation2016) may be another option in such environment. Besides the three decomposition strategies, a rolling horizon approach (Ovacik & Uzsoy, Citation1994) was proposed that partitions the scheduling horizon into time segments, assigns casts to the time segments, and then schedules each time segment separately. Although this decomposition strategy is frequently used in other scheduling settings, only Li et al. (Citation2012) and Hong et al. (Citation2022) applied this strategy in an SCC context (since the time scale for the problem is relatively short).
We believe that the iterated greedy algorithm of Hong et al. (Citation2022) is a promising solution methodology that can be applied to any deterministic SCC scheduling problem. They consider the SCC scheduling problem with unrelated machines and skippable routes, which can be easily transformed into other problems. They first built sub-schedules for individual casts and merged them sequentially using a MIP. After that, they applied two improvement heuristics based on a decomposition of casts and time segments, respectively. Since this algorithm consists of various effective decomposition strategies from the literature and also follows the well-known Large Neighbourhood Search (LNS) of Shaw (Citation1998) frequently used in scheduling (Kovacs et al., Citation2012; He et al., Citation2020), we may expect a certain level of performance. We also propose two possible extensions of the algorithm. Firstly, we may change the parameters to improve the algorithm in an adaptive way, applying an Adaptive Large Neighbourhood Search (ALNS) idea. Secondly, replacing a MIP with a CP may improve the algorithm’s performance since CP generally performs better for shop scheduling problems (Naderi et al., Citation2023). We refer to Pacino and Van Hentenryck (Citation2011), who apply CP with ALNS to flexible job shop problems, to demonstrate the potential of these suggested extensions.
4.2. SCC production scheduling with uncertainty
In this section, we deal with SCC papers that consider uncertainties in actual production settings. These uncertainties can be either predictable or unpredictable (Yu & Qi, Citation2004). Predictable uncertainties typically have a distribution or a range of potential deviations in the values of certain parameters, mainly in processing times. On the other hand, unpredictable uncertainties include disruptions, mostly on machines. There are three approaches to deal with uncertainty: the proactive approach, the reactive approach, and the predictive & reactive approach (Ouelhadj & Petrovic, Citation2009). summarises SCC scheduling papers that consider uncertainties.
Table 10. SCC scheduling papers with uncertainty.
The proactive approach considers predictable uncertainties in parameters before generating a schedule. It implies an a priori decision-making that is based on a method of creating a robust schedule with regard to the uncertainties. A mathematical programming heuristic approach, such as a robust optimisation framework to minimise a worst-case performance, or a stochastic programming approach to ensure a specific performance is frequently used. Ye et al. (Citation2014) considered uncertain processing times and an uncertain number of charges in a cast. They modified the event-based MIP models of Li et al. (Citation2012) and proposed two approaches: (i) a robust optimisation and (ii) a two-stage scenario-based stochastic programming approach. Niu et al. (Citation2022) considered uncertain processing times in a distributionally robust optimisation framework, where an exact distribution of processing times is unknown but it belongs to a specific distributional set. They determined machine assignments and sequencing variables by tabu search and solved the subproblems via chance-constrained LPs. Only Lv et al. (Citation2022) used a cross-entropy algorithm, assuming fuzzy processing times. They proposed six dispatching algorithms as heuristics for decoding and presented global search algorithms.
Unlike the proactive approach, the rescheduling approach deals with unpredictable uncertainties, such as machine disruptions that make a running schedule infeasible. It involves a dynamic adaptation of the existing schedule to generate a new feasible schedule with minor modification. contains the rescheduling objectives columns: “TD (Time Deviation)” for minimising the deviations in start times or processing times of charges if they are controllable; “MD (Machine Deviation)” for minimising the deviations in the machine assignments or charges in each stage; “T&M (Time and Machine deviation)” for minimising both deviations. When a disruption occurs, charges that have not been completed are rescheduled using a similar method as the deterministic scheduling problem but with deviation penalties included. Yu and Pan (Citation2012) proposed a three-stage heuristic that consists of forward scheduling and backward scheduling. Mao et al. (Citation2014a) formulated the problem as a time-indexed MIP and proposed a Lagrangian relaxation method using the decomposition strategy of Tang et al. (Citation2002).
In practice, however, engineers must deal with both types of uncertainties. Thus, the predictive and reactive approach has been introduced. The fundamental idea of the approach is to combine offline proactive scheduling (for dealing with potential uncertainties) with online rescheduling (for dealing with events that actually happened). One typical example is to create an initial schedule and check its robustness with regard to uncertainties via simulation (Worapradya & Thanakijkasem, Citation2015; Fanti et al., Citation2016). Long et al. (Citation2020) predicted parameters offline and conducted dynamic rescheduling via an evolutionary algorithm. Hao et al. (Citation2015) proposed a new soft-decision scheduling approach. It involves creating a tentative soft schedule, saving only important abstract partial information of charge priority that is later used in dynamic online dispatching. They updated their soft schedule when a critical event occurred. The predictive and reactive scheduling methods are more flexible in dealing with unexpected disruptions than the proactive methods because the start times of charges are not fixed. Due to its practical impact, many other papers have used since then the soft scheduling method (Jiang et al., Citation2016; Jiang et al., Citation2017).
Previous papers have considered machine breaks as unexpected events that should be managed either by the reactive or the predictive and reactive approach. However, in general, minor disruptions on machines can be predicted, and rescheduling after a disruption invokes an operational cost. There have been many studies on establishing a robust measure, which implies the ability to maintain a specific performance (Goren & Sabuncuoglu, Citation2008), as well as solution methodologies to optimize the performance measure. Applying these techniques to the SCC scheduling problem may be of interest. Liu et al. (Citation2007), Goren and Sabuncuoglu (Citation2009) and Xiong et al. (Citation2013) provide additional references.
4.3. SCC production scheduling with energy-related characteristics
In this section, we review the papers that consider energy-related characteristics. Energy-related papers have two main objectives: One is to minimise the total energy consumption and the other is to minimise the total energy cost that varies over time due to changes in start times of charges. summarises these papers.
Table 11. Energy-related SCC scheduling papers.
To reduce energy consumption, the heat loss of charges between stages is the main consideration in the minimisation of energy loss. Minimising the waiting time is the primary objective similar to the deterministic problem considered by Xu et al. (Citation2020), Lian et al. (Citation2021), and Lu and Qiao (Citation2022). The idle times of machines and the idle times of ladles are measures of energy loss (Wang et al., Citation2015). These features may worsen the waiting times or tardinesses since they could delay the start times of charges. Thus, the trade-offs between objectives have to be carefully managed. For example, Tan et al. (Citation2020) solved the problem of minimising the waiting times of charges and the idle times of machines and ladles. They used a scatter search method considering a decomposition of machine assignments and charge sequences with start times of charges. Another approach to reduce production energy loss is assigning charges to machines with minimum energy consumption. In such a setting a conflict between minimising energy consumption and production cost may occur (K. Wang et al., Citation2022). For example, Han, Tang, Zhang, and Cao (Citation2020) considered a scheduling problem to minimise the energy cost on machines and ladles with different unit costs. They used the migrating bird optimisation algorithm with a decoding heuristic consisting of forward-backward dispatching to determine the machine assignments of charges and another rule-based heuristic to determine the ladle assignments of charges.
Twelve papers consider minimising energy (electricity) costs that vary over time. Although deterministic scheduling problems typically assume that the continuous casting is the most constrained and most important stage, the melting stage from the EAF is considered equally important in such problems since 88% of energy for the entire SCC process is used at that stage. These papers assume that the entire scheduling horizon is partitioned into several time segments with different energy costs per unit time. Thus, a schedule that assigns charges to time segments with a low energy cost per unit time may require a different solution methodology. For example, Tan et al. (Citation2013) proposed a decomposition of the scheduling aspect and the energy consumption aspect. They first created a schedule for each cast considering the makespan objective and then applied a mathematical model to determine start times of all casts considering the time dependent energy costs. Instead of a heuristic, a distinct MIP model can be used to solve this problem. For that, Hadera and Harjunkoski (Citation2013) and Zhang et al. (Citation2017) used a resource-task network (RTN) based MIP model, which is specifically designed for reducing energy costs. In addition to time dependent energy costs, Hadera et al. (Citation2015) and Hadera et al. (Citation2016) have considered matching decisions with regard to energy sources and machines in a particular period. For example, Hadera et al. (Citation2015) proposed four electricity sources: long-term contract, short-term contract, spot market and onsite generation depending on the time interval and the price in each time interval. Thus, decisions of selecting energy sources in each time interval are included in the problem. Hadera et al. (Citation2015) and Hadera et al. (Citation2016) captured this issue, and proposed an effective decomposition matheuristic for the problem. Thus, they used a matheuristic to determine the machine assignments of charges in the EAF, and then determine other machine assignments in other stages and the exact start times.
Due to the increasing importance of sustainable production, researchers have started to focus more and more on energy-efficient scheduling. Thus, these scheduling techniques can be applied to SCC scheduling as well. One example is the speed-scaling mechanism of Wu and Che (Citation2020) that relates a machine’s processing speed to its energy consumption. In general, a lower processing speed implies a lower energy consumption. Even though the condition in SCC scheduling may differ from the previous study, establishing such a relationship may be a new research direction since some SCC scheduling problems do consider controllable processing times. Rescheduling could be problematic in the future when a schedule includes the decision of energy consumption since the supply of energy may not be easily adaptable (Lian et al., Citation2022); especially when energy is supplied from various sources such as Hadera et al. (Citation2015). Thus, we propose a rescheduling problem considering energy consumption as a future research direction.
4.4. SCC scheduling integrated with a batching decision
A few papers considered the cast batching and scheduling problems as one integrated problem. However, these papers do not provide a sufficient justification for solving both problems simultaneously, and do not present a critical relationship between the two problems. Hamada et al. (Citation1995) considered the batching problem of minimising the number of charges in a cast with the scheduling problem of minimising the makespan. Ouelhadj et al. (Citation2003) considered an integrated problem of batching and scheduling under uncertainty, and proposed a predictive and reactive scheduling framework for the problem. Armellini et al. (Citation2020) proposed an integrated problem of batching and scheduling along with energy-related decisions on ladle constraints. Although the batching decisions do indeed affect the scheduling decisions, especially with regard to energy consumption (Xu et al., Citation2018), a clear tradeoff between the objectives for the batching and scheduling problems should be investigated in future research to justify an integration of the two problems. For example, Ozturk (Citation2022) deals with a scheduling problem of parallel batches included in a serial batch. The problem also considers a family of jobs, where only jobs from the same family can belong to the same serial batch. When a particular steel grade is considered a family, this problem can have a structure similar to the integrated SCC batching and scheduling problem. If the objective of the scheduling problem is to minimize total weighted tardiness, the batch assignment decisions directly affect the objective. If the solution to a batching problem assigns jobs with varying due dates to the same batch, the objective value of the scheduling problem cannot be good because each job has its own due date. This implies that the similarity of due dates should be considered when creating batches for an integrated SCC batching and scheduling problem.
5. Conclusion
5.1. Summary
In this article, we discussed, classified, and analysed planning and scheduling problems in SCC processes. We proposed two core models for the batching problem and for the scheduling problem; both models have the essential features of the two problems. We also relate the constraints and objectives of the problems to practical considerations. We reviewed frequently used methodologies to solve these problems, focusing on various decomposition strategies. We also suggested some promising new solution methodologies.
A planning problem includes the design problem of assigning orders to slabs and the batching problem of assigning slabs to charges and casts. Since slab design problems have a theoretical modelling base, many previously known methodologies have been used. A steel-specific slab design problem with an adjustable motherplate is one variant that can be considered in the future. Developing practical methods for the problem can be beneficial since it can dramatically reduce the slacks from the slabs. Since the batching problem is well defined for any specific plant, we propose a fundamental core model in Appendix A. Developing solution methodologies for the core model would help solve more general problems. The introduction of frames of charges and casts based on width range and steel grade information may serve as an input for the solution methodologies. The frames are fully described using corresponding pattern variables; in previous studies only a subset was created from partial slab information. Since the core model is a pure binary problem, we expect the column-generation approach to work well for large problem instances.
The scheduling problem is a variant of the hybrid flow shop problem with non-idle sequential batches in the last stage. We mainly reviewed deterministic SCC scheduling problems, and then investigated problems under uncertainty, problems with energy-related characteristics, and integrated problems with batching decisions. We reviewed three major decomposition approaches applicable to the deterministic problems with key ideas. Although only a few papers have considered the application of multiple decomposition approaches to a problem, it will become an essential method when problems become more complicated. Although many proactive scheduling approaches have been proposed for other production environments, only seven papers have used such a method in this area. Since frequent dynamic rescheduling may negatively affect the performance of the initial plan and may lead to severe production instability (Hao et al., Citation2015), proactive approaches may benefit engineers in practice.
5.2. Discussion
Because of the practical importance of SCC planning and scheduling, we predict that this field will continue to grow. Based on our analysis, we can present conclusions and identify promising future research directions for industry and academia. From an industry perspective, the following research directions are of interest.
In addition to performance and computational efficiency, implementation and maintenance can also be important aspects of an algorithm. A research group could keep the implementation within the company, for example, Baosteel (Tang et al., Citation2014), or could do it via a third-party software company such as IBM (Dash et al., Citation2007), Solentia (Missbauer et al., Citation2009) and Danieli (Armellini et al., Citation2020). The implementer must help decision-makers in the plant maintain the system. Otherwise, it remains in use only for a limited time and will be ignored altogether when the production environment changes. For that, a continuous update of the skills of the workforce is required (see Branca et al., Citation2020), and a system should allow users to easily modify the generated solutions through interactive Graphics User Interfaces (GUIs). We refer to the above papers as practical cases for those readers who are interested in implementations.
The SCC planning and scheduling problem usually involves multiple objectives, and thus practitioners may want to find solution candidates that lie on the Pareto frontier. Thus, research on multi-objective SCC planning and scheduling needs more attention. Research considering hybrid algorithms generating diversified solutions, such as Long et al. (Citation2016) may be a promising direction.
It may be essential to holistically compare practical performances of solution methodologies regarding problem settings, instance sizes, runtimes, years of publication (to account for computing power), optimality gaps, etc. This may help practitioners with the development of algorithms for their production environments.
From an academic perspective, the following research directions are of interest.
Although every batching problem assumes that slabs are prescribed in advance, considering a penalty for wide width ranges in a slab design problem may facilitate slabs to be more easily batched into charges and casts; it shows the possibility of integrating the design problem with the batching problem.
A few papers have used exact methods to deal with batching problems and scheduling problems due to their complexity. Exact methods could capture the problem’s fundamental structure and serve as a basis for developing efficient heuristic algorithms (Neufeld et al., Citation2023). Because of the two core models proposed, we recommend constructing efficient exact algorithms for these models as a first trial.
The SCC planning and scheduling problem is to some extent connected to the rolling process. For example, Li et al. (Citation2019) considered using slabs for warming up the rolling facilities. Also, rolling slabs just after the continuous casting stage reduces energy consumption by skipping the reheating process of slabs in inventory (Cowling & Rezig, Citation2000). However, even with such relationships, the optimisation problems of rolling processes are usually solved separately from that of SCC processes. We suggest formulating and solving the integrated optimisation problem as a future research opportunity, such as Xu et al. (Citation2012) and Lin et al. (Citation2016).
Comparing algorithms for SCC planning and scheduling problems in the literature is very difficult because of plant-specific constraints. To the best of our knowledge, no planning paper has disclosed its instances. Armellini et al. (Citation2020) and Hong et al. (Citation2022) are the only papers that disclose problem instances for SCC scheduling. We would strongly encourage a standardisation of models and a preparation of benchmark instances for fair comparisons in academic research.
Disclosure statement
No potential conflict of interest was reported by the author(s).
Additional information
Funding
References
- Ariyama, T., & Sato, M. (2006). Optimization of ironmaking process for reducing CO2 emissions in the integrated steel works. ISIJ International, 46(12), 1736–1744. https://doi.org/10.2355/isijinternational.46.1736
- Armellini, D., Borzone, P., Ceschia, S., Di Gaspero, L., & Schaerf, A. (2020). Modeling and solving the steelmaking and casting scheduling problem. International Transactions in Operational Research, 27(1), 57–90. https://doi.org/10.1111/itor.12595
- Atighehchian, A., Bijari, M., & Tarkesh, H. (2009). A novel hybrid algorithm for scheduling steel-making continuous casting production. Computers & Operations Research, 36(8), 2450–2461. https://doi.org/10.1016/j.cor.2008.10.010
- Balakrishnan, A., & Geunes, J. (2003). Production planning with flexible product specifications: An application to specialty steel manufacturing. Operations Research, 51(1), 94–112. https://doi.org/10.1287/opre.51.1.94.12791
- Bellabdaoui, A., & Teghem, J. (2006). A mixed-integer linear programming model for the continuous casting planning. International Journal of Production Economics, 104(2), 260–270. https://doi.org/10.1016/j.ijpe.2004.10.016
- Box, R. E., & Herbe, D. G., Jr. (1988). A scheduling model for LTV Steel’s Cleveland Works’ twin strand continuous slab caster. Interfaces, 18(1), 42–56. https://doi.org/10.1287/inte.18.1.42
- Branca, T. A., Fornai, B., Colla, V., Murri, M. M., Streppa, E., & Schröder, A. J. (2020). The challenge of digitalization in the steel sector. Metals, 10(2), 288. https://doi.org/10.3390/met10020288
- Cao, J., Pan, R., Xia, X., Shao, X., & Wang, X. (2021). An efficient scheduling approach for an iron-steel plant equipped with self-generation equipment under time-of-use electricity tariffs. Swarm and Evolutionary Computation, 60, 100764. https://doi.org/10.1016/j.swevo.2020.100764
- Casado, S., Laguna, M., Pacheco, J., & Puche, J. C. (2020). Grouping products for the optimization of production processes: A case in the steel manufacturing industry. European Journal of Operational Research, 286(1), 190–202. https://doi.org/10.1016/j.ejor.2020.03.010
- Chakrabarti, A. K. (2006). Steel making. PHI Learning Pvt. Ltd.
- Chang, S. Y., Chang, M.-R., & Hong, Y. (2000). A lot grouping algorithm for a continuous slab caster in an integrated steel mill. Production Planning & Control, 11(4), 363–368. https://doi.org/10.1080/095372800232090
- Cowling, P., & Rezig, W. (2000). Integration of continuous caster and hot strip mill planning for steel production. Journal of Scheduling, 3(4), 185–208. https://doi.org/10.1002/1099-1425(200007/08)3:4<185::AID-JOS42>3.0.CO;2-G
- Crévits, I., Hanaf I, A Ridha Mahjoub, S., Taktak, R., Wilbaut, C. (2019). A special case of variable-sized bin packing problem with color constraints. In 2019 6th International Conference on Control, Decision and Information Technologies (pp. 1150–1154). IEEE
- Cui, H., & Luo, X. (2017). An improved Lagrangian relaxation approach to scheduling steelmaking-continuous casting process. Computers & Chemical Engineering, 106, 133–146. https://doi.org/10.1016/j.compchemeng.2017.05.026
- Cui, H., Luo, X., & Wang, Y. (2020). Scheduling of steelmaking-continuous casting process using deflected surrogate Lagrangian relaxation approach and DC Algorithm. Computers & Industrial Engineering, 140, 106271. https://doi.org/10.1016/j.cie.2020.106271
- Cui, H., Luo, X., & Wang, Y. (2022). Scheduling of steelmaking-continuous casting process with different processing routes using effective surrogate Lagrangian relaxation approach and improved concave-convex procedure. International Journal of Production Research, 60(11), 3435–3460. https://doi.org/10.1080/00207543.2021.1924408
- Dash, S., Kalagnanam, J., Reddy, C., & Song, S. H. (2007). Production design for plate products in the steel industry. IBM Journal of Research and Development, 51(3.4), 345–362. https://doi.org/10.1147/rd.513.0345
- Dawande, M., & Kalagnanam, J. (1998). The multiple knapsack problem with color constraints. IBM Technical Report RC21138.
- Dawande, M., Kalagnanam, J., Lee, H. S., Reddy, C., Siegel, S., & Trumbo, M. (2004). The slab-design problem in the steel industry. Interfaces, 34(3), 215–225. https://doi.org/10.1287/inte.1040.0077
- De Moerloose, P., & Maenhout, B. (2023). A two-stage local search heuristic for solving the steelmaking continuous casting scheduling problem with dual shared-resource and blocking constraints. Operational Research, 23(1), 2. https://doi.org/10.1007/s12351-023-00760-2
- Dong, H., Huang, M., Ip, W. H., & Wang, X. (2010). On the integrated charge planning with flexible jobs in primary steelmaking processes. International Journal of Production Research, 48(21), 6499–6535. https://doi.org/10.1080/00207540903246656
- Dong, H., Huang, M., Ip, W. H., & Wang, X. (2012). Improved variable neighbourhood search for integrated tundish planning in primary steelmaking processes. International Journal of Production Research, 50(20), 5747–5761. https://doi.org/10.1080/00207543.2011.624563
- Dorn, J., Kerr, R., & Thalhammer, G. (1995). Reactive scheduling: Improving the robustness of schedules and restricting the effects of shop floor disturbances by fuzzy reasoning. International Journal of Human-Computer Studies, 42(6), 687–704. https://doi.org/10.1006/ijhc.1995.1031
- Dutta, G., & Fourer, R. (2001). A survey of mathematical programming applications in integrated steel plants. Manufacturing & Service Operations Management, 3(4), 387–400. https://doi.org/10.1287/msom.3.4.387.9972
- Fanti, M. P., Rotunno, G., Stecco, G., Ukovich, W., & Mininel, S. (2016). An integrated system for production scheduling in steelmaking and casting plants. IEEE Transactions on Automation Science and Engineering, 13(2), 1112–1128. https://doi.org/10.1109/TASE.2015.2477362
- Fazel Zarandi, M. H., & Dorry, F. (2018). A hybrid fuzzy PSO algorithm for solving steelmaking-continuous casting scheduling problem. International Journal of Fuzzy Systems, 20(1), 219–235. https://doi.org/10.1007/s40815-017-0331-0
- Fisher, M. L. (1981). The Lagrangian relaxation method for solving integer programming problems. Management Science, 27(1), 1–18. https://doi.org/10.1287/mnsc.27.1.1
- Forrest, J. J., Kalagnanam, J., & Ladanyi, L. (2006). A column-generation approach to the multiple knapsack problem with color constraints. INFORMS Journal on Computing, 18(1), 129–134. https://doi.org/10.1287/ijoc.1040.0078
- Fraizzoli, D., Ramin, D., Brusaferri, A. (2020). A new modeling approach to include EAF flexibility in the energy-aware scheduling of steelmaking process. In 2020 7th International Conference on Control, Decision and Information Technologies (vol. 1, pp. 1063–1068).
- Frisch, A. M., Miguel, I., & Walsh, T. (2001). Symmetry and implied constraints in the steel mill slab design problem. In Proc. CP’01 workshop on modelling and problem formulation.
- Furini, F., & Malaguti, E. (2013). Models for the two-dimensional two-stage cutting stock problem with multiple stock size. Computers & Operations Research, 40(8), 1953–1962. https://doi.org/10.1016/j.cor.2013.02.026
- Gajic, D., Hadera, H., Onofri, L., Harjunkoski, I., & Di Gennaro, S. (2017). Implementation of an integrated production and electricity optimization system in melt shop. Journal of Cleaner Production, 155, 39–46. https://doi.org/10.1016/j.jclepro.2016.09.170
- García-Menéndez, D., Morán-Palacios, H., Ortega-Fernández, F., & Díaz-Piloñeta, M. (2020). Scheduling in continuous steelmaking casting: A systematic review. ISIJ International, 60(6), 1097–1107. https://doi.org/10.2355/isijinternational.ISIJINT-2019-574
- Garey, M. R., & Johnson, D. S. (1979). Computers and intractability: A guide to the theory of NP-completeness (1st ed.). W. H. Freeman.
- Gargani, A., Refalo, P. (2007). An efficient model and strategy for the steel mill slab design problem. In International Conference on Principles and Practice of Constraint Programming (pp. 77–89). Springer.
- Gay, S., Schaus, P., & Smedt, V. D. (2014). Continuous casting scheduling with constraint programming. In B. O’Sullivan (ed.), Principles and practice of constraint programming (vol. 8656, pp. 831–845). Springer International Publishing.
- Glover, F., & Laguna, M. (1998). Tabu search. In Handbook of combinatorial optimization (pp. 2093–2229). Springer.
- Goren, S., & Sabuncuoglu, I. (2008). Robustness and stability measures for scheduling: Single-machine environment. IIE Transactions, 40(1), 66–83. https://doi.org/10.1080/07408170701283198
- Goren, S., & Sabuncuoglu, I. (2009). Optimization of schedule robustness and stability under random machine breakdowns and processing time variability. IIE Transactions, 42(3), 203–220. https://doi.org/10.1080/07408170903171035
- Guo, D., & Li, T. (2007). Rescheduling algorithm for steelmaking-continuous casting [Paper presentation]. In 2007 2nd IEEE Conference on Industrial Electronics and Applications (pp. 1421–1425). IEEE. https://doi.org/10.1109/ICIEA.2007.4318640
- Gupta, J. N. (1988). Two-stage, hybrid flowshop scheduling problem. Journal of the Operational Research Society, 39(4), 359–364. https://doi.org/10.1057/jors.1988.63
- Hadera, H., & Harjunkoski, I. (2013). Continuous-time batch scheduling approach for optimizing electricity consumption cost. In A. Kraslawski, & I. Turunen (Eds.), Computer aided chemical engineering. Volume 32 of 23 European Symposium on Computer Aided Process Engineering (pp. 403–408). Elsevier.
- Hadera, H., Harjunkoski, I., Sand, G., Grossmann, I. E., & Engell, S. (2015). Optimization of steel production scheduling with complex time-sensitive electricity cost. Computers & Chemical Engineering, 76, 117–136. https://doi.org/10.1016/j.compchemeng.2015.02.004
- Hadera, H., Labrik, R., Sand, G., Engell, S., & Harjunkoski, I. (2016). An improved energy-awareness formulation for general precedence continuous-time scheduling models. Industrial & Engineering Chemistry Research, 55(5), 1336–1346. https://doi.org/10.1021/acs.iecr.5b03239
- Hakimzadeh Abyaneh, S., & Zandieh, M. (2012). Bi-objective hybrid flow shop scheduling with sequence-dependent setup times and limited buffers. The International Journal of Advanced Manufacturing Technology, 58(1–4), 309–325. https://doi.org/10.1007/s00170-011-3368-5
- Hamada, K., Baba, T., Sato, K., & Yufu, M. (1995). Hybridizing a genetic algorithm with rule-based reasoning for production planning. IEEE Expert, 10(5), 60–67. https://doi.org/10.1109/64.464934
- Han, D., Tang, Q., Zhang, Z., & Cao, J. (2020). Energy-efficient integration optimization of production scheduling and ladle dispatching in steelmaking plants. IEEE Access, 8, 176170–176187. https://doi.org/10.1109/ACCESS.2020.3027018
- Han, D., Tang, Q., Zhang, Z., & Li, Z. (2020). An improved migrating birds optimization algorithm for a hybrid flow shop scheduling within steel plants. Mathematics, 8(10), 1661. https://doi.org/10.3390/math8101661
- Han, D., Tang, Q., Zhang, Z., Yuan, L., Rakovitis, N., Li, D., & Li, J. (2021). An efficient augmented Lagrange multiplier method for steelmaking and continuous casting production scheduling. Chemical Engineering Research and Design, 168, 169–192. https://doi.org/10.1016/j.cherd.2021.01.035
- Hao, J., Liu, M., Jiang, S.-L., & Wu, C. (2015). A soft-decision based two-layered scheduling approach for uncertain steelmaking-continuous casting process. European Journal of Operational Research, 244(3), 966–979. https://doi.org/10.1016/j.ejor.2015.02.026
- Harjunkoski, I., & Grossmann, I. E. (2001). A decomposition approach for the scheduling of a steel plant production. Computers & Chemical Engineering, 25(11–12), 1647–1660. https://doi.org/10.1016/S0098-1354(01)00729-3
- Harjunkoski, I., & Sand, G. (2008). Flexible and configurable MILP-models for meltshop scheduling optimization. In B. Braunschweig, & X. Joulia (Eds.), Computer aided chemical engineering. Volume 25 of 18th European symposium on computer aided process engineering (pp. 677–682). Elsevier.
- He, L., de Weerdt, M., & Yorke-Smith, N. (2020). Time/sequence-dependent scheduling: The design and evaluation of a general purpose tabu-based adaptive large neighbourhood search algorithm. Journal of Intelligent Manufacturing, 31(4), 1051–1078. https://doi.org/10.1007/s10845-019-01518-4
- He, S., Li, D., Zhang, H., Gong, F., Chen, S., Ren, K. (2021). Research on modeling and solving algorithm of steelmaking continuous casting production scheduling. In 2021 7th International Symposium on Mechatronics and Industrial Informatics (pp. 188–192).
- Heinz, S., Schlechte, T., Stephan, R., & Winkler, M. (2012). Solving steel mill slab design problems. Constraints, 17(1), 39–50. https://doi.org/10.1007/s10601-011-9113-8
- Hnich, B., Kiziltan, Z., Miguel, I., & Walsh, T. (2004). Hybrid modelling for robust solving. Annals of Operations Research, 130(1–4), 19–39. https://doi.org/10.1023/B:ANOR.0000032568.51115.0d
- Hong, J., Moon, K., Lee, K., Lee, K., & Pinedo, M. L. (2022). An iterated greedy matheuristic for scheduling in steelmaking-continuous casting process. International Journal of Production Research, 60(2), 623–643. https://doi.org/10.1080/00207543.2021.1975839
- Huegler, P. A., & Hartman, J. C. (2007). Fulfilling orders for steel plates from existing inventory. Journal of the Operational Research Society, 58(9), 1156–1166. https://doi.org/10.1057/palgrave.jors.2602274
- Huimin, G., Jianchao, Z. (2007). Cooperative particle swarm optimization for steel making scheduling. In Third international conference on natural computation (vol. 4, pp. 522–526). IEEE.
- Iglesias-Escudero, M., Villanueva-Balsera, J., Ortega-Fernandez, F., & Rodriguez-Montequín, V. (2019). Planning and scheduling with uncertainty in the steel sector: A review. Applied Sciences, 9(13), 2692. https://doi.org/10.3390/app9132692
- Iori, M., De Lima, V. L., Martello, S., Miyazawa, F. K., & Monaci, M. (2021). Exact solution techniques for two-dimensional cutting and packing. European Journal of Operational Research, 289(2), 399–415. https://doi.org/10.1016/j.ejor.2020.06.050
- Jansen, B. J. (1998). The graphical user interface. ACM SIGCHI Bulletin, 30(2), 22–26. https://doi.org/10.1145/279044.279051
- Jiang, S.-L., Liu, M., & Hao, J. (2017). A two-phase soft optimization method for the uncertain scheduling problem in the steelmaking industry. IEEE Transactions on Systems, Man, and Cybernetics: Systems, 47(3), 416–431. https://doi.org/10.1109/TSMC.2015.2503388
- Jiang, S.-L., Liu, M., Hao, J., & Qian, W. (2015). A bi-layer optimization approach for a hybrid flow shop scheduling problem involving controllable processing times in the steelmaking industry. Computers & Industrial Engineering, 87, 518–531. https://doi.org/10.1016/j.cie.2015.06.002
- Jiang, S.-L., Liu, M., Lin, J., & Zhong, H. (2016). A prediction-based online soft scheduling algorithm for the real-world steelmaking-continuous casting production. Knowledge-Based Systems, 111, 159–172. https://doi.org/10.1016/j.knosys.2016.08.010
- Jiang, S.-L., & Liu, Q. (2017). An adaptive sampling-estimation of distribution algorithm for robust scheduling in the steelmaking-continuous casting process [Paper presentation]. In 2017 13th IEEE conference on automation science and engineering (pp. 440–445).
- Jiang, S.-L., Liu, Q., Bogle, I. D. L., & Zheng, Z. (2023). A self-learning based dynamic multi-objective evolutionary algorithm for resilient scheduling problems in steelmaking plants. IEEE Transactions on Automation Science and Engineering, 20(2), 832–845. https://doi.org/10.1109/TASE.2022.3168385
- Jiang, S.-L., Zheng, Z., & Liu, M. (2017). A multi-stage dynamic soft scheduling algorithm for the uncertain steelmaking-continuous casting scheduling problem. Applied Soft Computing, 60, 722–736. https://doi.org/10.1016/j.asoc.2017.07.016
- Jiang, S.-L., Zheng, Z., & Liu, M. (2018). A preference-inspired multi-objective soft scheduling algorithm for the practical steelmaking-continuous casting production. Computers & Industrial Engineering, 115, 582–594. https://doi.org/10.1016/j.cie.2017.10.028
- Jin, Y., Niu, D., Li, Q., & Shi, H. (2021). Research on scheduling method of steelmaking-continuous casting production with limited waiting time and stage skipping. In 2021 36th youth academic annual conference of Chinese association of automation (pp. 604–609). IEEE.
- Kadlec, P., Gabrys, B., & Strandt, S. (2009). Data-driven soft sensors in the process industry. Computers & Chemical Engineering, 33(4), 795–814. https://doi.org/10.1016/j.compchemeng.2008.12.012
- Kalagnanam, J. R., Dawande, M. W., Trumbo, M., & Lee, H. S. (1998). Inventory matching problems in the steel industry. IBM Technical Report, Computer Science/Mathematics, 21171.
- Kalagnanam, J. R., Dawande, M. W., Trumbo, M., & Lee, H. S. (2000). The surplus inventory matching problem in the process industry. Operations Research, 48(4), 505–516. https://doi.org/10.1287/opre.48.4.505.12425
- Kammammettu, S., & Li, Z. (2018). Multistage adaptive optimization for steelmaking and continuous casting scheduling under processing time uncertainty. IFAC-PapersOnLine, 51(21), 262–267. https://doi.org/10.1016/j.ifacol.2018.09.429
- Kasapinov, L., Jovanov, M., & Trajanov, D. (2014). A new approach for maximum utilization of the mother steel plates for rectangular steel plates orders. In 2014 37th international convention on information and communication technology, electronics and microelectronics (pp. 959–965). IEEE.
- Kikuchi, N. (2020). Development and prospects of refining techniques in steelmaking process. ISIJ International, 60(12), 2731–2744. https://doi.org/10.2355/isijinternational.ISIJINT-2020-186
- Kim, J. H., Yi, H.-S., & Han, C. (2003). A novel MILP model for plantwide multiperiod optimization of byproduct gas supply system in the iron-and steel-making process. Chemical Engineering Research and Design, 81(8), 1015–1025. https://doi.org/10.1205/026387603322482248
- Kovacs, A. A., Parragh, S. N., Doerner, K. F., & Hartl, R. F. (2012). Adaptive large neighborhood search for service technician routing and scheduling problems. Journal of Scheduling, 15(5), 579–600. https://doi.org/10.1007/s10951-011-0246-9
- Kumar, V., Kumar, S., Tiwari, M. K., & Chan, F. T. S. (2006). Auction-based approach to resolve the scheduling problem in the steel making process. International Journal of Production Research, 44(8), 1503–1522. https://doi.org/10.1080/00207540500434713
- Lee, K., Chang, S. Y., & Hong, Y. (2004). Continuous slab caster scheduling and interval graphs. Production Planning & Control, 15(5), 495–501. https://doi.org/10.1080/09537280410001714279
- Lee, M., Hong, J., Kim, H., Bang, I., Lee, K., Kim, B.-I., & Kim, J. (2023). An integrated batching problem for steel plate manufacturing with bi-strand casting. International Journal of Production Research, 61(3), 955–975. https://doi.org/10.1080/00207543.2021.2021456
- Li, C., Yuan, B., Ren, J., Sun, L., & Wang, J. (2019). Research on optimization of charge batch planning based on augmented Lagrangian relaxation algorithm. IFAC-PapersOnLine, 52(1), 814–819. https://doi.org/10.1016/j.ifacol.2019.06.162
- Li, J., Xiao, X., Tang, Q., & Floudas, C. A. (2012). Production scheduling of a large-scale steelmaking continuous casting process via unit-specific event-based continuous-time models: Short-term and medium-term scheduling. Industrial & Engineering Chemistry Research, 51(21), 7300–7319. https://doi.org/10.1021/ie2015944
- Li, J-q., Pan, Q-k., Mao, K., & Suganthan, P. N. (2014). Solving the steelmaking casting problem using an effective fruit fly optimisation algorithm. Knowledge-Based Systems, 72, 28–36. https://doi.org/10.1016/j.knosys.2014.08.022
- Li, J., Duan, P., Sang, H., Wang, S., Liu, Z., & Duan, P. (2018). An efficient optimization algorithm for resource-constrained steelmaking scheduling problems. IEEE Access, 6, 33883–33894. https://doi.org/10.1109/ACCESS.2018.2840512
- Li, J., Pan, Q-k., & Mao, K. (2016). A hybrid fruit fly optimization algorithm for the realistic hybrid flowshop rescheduling problem in steelmaking systems. IEEE Transactions on Automation Science and Engineering, 13(2), 932–949. https://doi.org/10.1109/TASE.2015.2425404
- Li, T., Li, Y., Zhang, Q., Gao, X., & Dai, S. (2005). Constraint programming approach to steelmaking-making process scheduling. Communications of the IIMA, 5(3), 9.
- Lian, X., Zheng, Z., & Gao, X. (2022). Steel plant rescheduling considering the balance of oxygen supply and demand under converter failure. Computers & Chemical Engineering, 168, 108064. https://doi.org/10.1016/j.compchemeng.2022.108064
- Lian, X., Zheng, Z., Wang, C., & Gao, X. (2021). An energy-efficient hybrid flow shop scheduling problem in steelmaking plants. Computers & Industrial Engineering, 162, 107683. https://doi.org/10.1016/j.cie.2021.107683
- Lin, J., Liu, M., Hao, J., & Jiang, S.-L. (2016). A multi-objective optimization approach for integrated production planning under interval uncertainties in the steel industry. Computers & Operations Research, 72, 189–203. https://doi.org/10.1016/j.cor.2016.03.002
- Linn, R., & Zhang, W. (1999). Hybrid flow shop scheduling: A survey. Computers & Industrial Engineering, 37(1-2), 57–61. https://doi.org/10.1016/S0360-8352(99)00023-6
- Liu, L., Gu, H-y., & Xi, Y-g (2007). Robust and stable scheduling of a single machine with random machine breakdowns. The International Journal of Advanced Manufacturing Technology, 31(7–8), 645–654. https://doi.org/10.1007/s00170-005-0237-0
- Liu, W., Pang, X., Li, H., & Sun, L. (2022). Ladle intelligent re-scheduling method in steelmaking–refining–continuous casting production process based on BP neural network working condition estimation. The International Journal of Advanced Manufacturing Technology, 122(1), 65–85. https://doi.org/10.1007/s00170-021-08327-1
- Lodi, A., Martello, S., & Monaci, M. (2002). Two-dimensional packing problems: A survey. European Journal of Operational Research, 141(2), 241–252. https://doi.org/10.1016/S0377-2217(02)00123-6
- Long, J., Hong, Y., Bai, Y., Zhang, S., & Sun, Z. (2018a). Practical production scheduling for hot metal pretreatment-steelmaking-continuous casting process involving preventive maintenance consideration. IEEE Access, 6, 57017–57029. https://doi.org/10.1109/ACCESS.2018.2873422
- Long, J., Sun, Z., Chen, H., Bai, Y., & Hong, Y. (2018b). Variable neighborhood search for integrated determination of charge batching and casting start time in steel plants. Journal of Intelligent & Fuzzy Systems, 34(6), 3821–3832. https://doi.org/10.3233/JIFS-169554
- Long, J., Sun, Z., Pardalos, P. M., Bai, Y., Zhang, S., & Li, C. (2020). A robust dynamic scheduling approach based on release time series forecasting for the steelmaking-continuous casting production. Applied Soft Computing, 92, 106271. https://doi.org/10.1016/j.asoc.2020.106271
- Long, J., Zheng, Z., & Gao, X. (2017). Dynamic scheduling in steelmaking-continuous casting production for continuous caster breakdown. International Journal of Production Research, 55(11), 3197–3216. https://doi.org/10.1080/00207543.2016.1268277
- Long, J., Zheng, Z., Gao, X., & Pardalos, P. M. (2016). A hybrid multi-objective evolutionary algorithm based on NSGA-II for practical scheduling with release times in steel plants. Journal of the Operational Research Society, 67(9), 1184–1199. https://doi.org/10.1057/jors.2016.17
- Long, J., Zheng, Z., Gao, X., & Pardalos, P. M. (2018c). Scheduling a realistic hybrid flow shop with stage skipping and adjustable processing time in steel plants. Applied Soft Computing, 64, 536–549. https://doi.org/10.1016/j.asoc.2017.12.044
- Lu, H., & Qiao, F. (2022). An efficient adaptive genetic algorithm for energy saving in the hybrid flow shop scheduling with batch production at last stage. Expert Systems, 39(2), e12678. https://doi.org/10.1111/exsy.12678
- Luo, J., & Tang, L. (2005). Slab matching problem: A tabu search approach [Paper presentation]. In 2005 ICSC Congress on computational intelligence methods and applications. IEEE.
- Lv, Y., Qian, B., Hu, R., Jin, H.-P., & Zhang, Z.-Q. (2022). An enhanced cross-entropy algorithm for the green scheduling problem of steelmaking and continuous casting with uncertain processing time. Computers & Industrial Engineering, 171, 108445. https://doi.org/10.1016/j.cie.2022.108445
- Ma, T., Luo, X., & Chai, T. (2014). Modeling and hybrid optimization of batching planning system for steelmaking-continuous casting process. IEEE/CAA Journal of Automatica Sinica, 1(2), 113–126.
- Mao, K., Pan, Q-k., Chai, T., & Luh, P. B. (2015). An effective subgradient method for scheduling a steelmaking-continuous casting process. IEEE Transactions on Automation Science and Engineering, 12(3), 1140–1152. https://doi.org/10.1109/TASE.2014.2332511
- Mao, K., Pan, Q.-K., Pang, X., & Chai, T. (2014a). An effective Lagrangian relaxation approach for rescheduling a steelmaking-continuous casting process. Control Engineering Practice, 30, 67–77. https://doi.org/10.1016/j.conengprac.2014.06.003
- Mao, K., Pan, Q-k., Pang, X., & Chai, T. (2014b). A novel Lagrangian relaxation approach for a hybrid flowshop scheduling problem in the steelmaking-continuous casting process. European Journal of Operational Research, 236(1), 51–60. https://doi.org/10.1016/j.ejor.2013.11.010
- Maravelias, C. T. (2021). Chemical production scheduling: Mixed-integer programming models and methods. Cambridge University Press.
- Mehdi, M. M., Wang, L., & Willems, S. P. (2022). Developing a maximum inscribed rectangle heuristic to satisfy rush orders for heavy plate steel. INFORMS Journal on Applied Analytics, 52(3), 283–294. https://doi.org/10.1287/inte.2021.1086
- Merten, D. C., Hütt, M.-T., & Uygun, Y. (2022). A network analysis of decision strategies of human experts in steel manufacturing. Computers & Industrial Engineering, 168, 108120. https://doi.org/10.1016/j.cie.2022.108120
- Missbauer, H., Hauber, W., & Stadler, W. (2009). A scheduling system for the steelmaking-continuous casting process. A case study from the steel-making industry. International Journal of Production Research, 47(15), 4147–4172. https://doi.org/10.1080/00207540801950136
- Naderi, B., Ruiz, R., & Roshanaei, V. (2023). Mixed-Integer programming vs. Constraint programming for shop scheduling problems: New results and outlook. INFORMS Journal on Computing, 35(4), 817–843. https://doi.org/10.1287/ijoc.2023.1287
- Nakhaeinejad, M. (2019). Production scheduling optimization algorithm for the steel-making continuous casting processes. Advances in Industrial Engineering, 53(4), 127–147.
- Neufeld, J. S., Schulz, S., & Buscher, U. (2023). A systematic review of multi-objective hybrid flow shop scheduling. European Journal of Operational Research, 309(1), 1–23. https://doi.org/10.1016/j.ejor.2022.08.009
- Niu, S., Song, S., & Chiong, R. (2022). A distributionally robust scheduling approach for uncertain steelmaking and continuous casting processes. IEEE Transactions on Systems, Man, and Cybernetics: Systems, 52(6), 3900–3914. https://doi.org/10.1109/TSMC.2021.3079133
- Numao, M., & Morishita, S.-I. (1991). Cooperative scheduling and its application to steelmaking processes. IEEE Transactions on Industrial Electronics, 38(2), 150–155. https://doi.org/10.1109/41.88909
- Ogata, T., Okubo, T., Nagai, H., Yamamoto, M., Sugi, M., & Ota, J. (2015). A novel algorithm for continuous steel casting scheduling with focus on quality property constraint and slab width maximization. International Journal of Automation Technology, 9(3), 235–247. https://doi.org/10.20965/ijat.2015.p0235
- Oĝuz, C., & Ercan, M. F. (2005). A genetic algorithm for hybrid flow-shop scheduling with multiprocessor tasks. Journal of Scheduling, 8(4), 323–351. https://doi.org/10.1007/s10951-005-1640-y
- Ouelhadj, D., Cowling, P. I., Petrovic, S. (2003). Utility and stability measures for agent-based dynamic scheduling of steel continuous casting. In 2003 IEEE international conference on robotics and automation (Cat. No.03CH37422), (vol. 1, pp. 175–180).
- Ouelhadj, D., & Petrovic, S. (2009). A survey of dynamic scheduling in manufacturing systems. Journal of Scheduling, 12(4), 417–431. https://doi.org/10.1007/s10951-008-0090-8
- Ovacik, I. M., & Uzsoy, R. (1994). Rolling horizon algorithms for a single-machine dynamic scheduling problem with sequence-dependent setup times. International Journal of Production Research, 32(6), 1243–1263. https://doi.org/10.1080/00207549408956998
- Ozturk, O. (2022). When serial batch scheduling involves parallel batching decisions: A branch and price scheme. Computers & Operations Research, 137, 105514. https://doi.org/10.1016/j.cor.2021.105514
- Pacciarelli, D. (2002). Alternative graph formulation for solving complex factory-scheduling problems. International Journal of Production Research, 40(15), 3641–3653. https://doi.org/10.1080/00207540210136478
- Pacciarelli, D., & Pranzo, M. (2004). Production scheduling in a steelmaking-continuous casting plant. Computers & Chemical Engineering, 28(12), 2823–2835. https://doi.org/10.1016/j.compchemeng.2004.08.031
- Pacino, D., & Van Hentenryck, P. (2011). Large neighborhood search and adaptive randomized decompositions for flexible jobshop scheduling [Paper presentation]. In Twenty-second international joint conference on artificial intelligence.
- Pan, Q-k. (2016). An effective co-evolutionary artificial bee colony algorithm for steelmaking-continuous casting scheduling. European Journal of Operational Research, 250(3), 702–714. https://doi.org/10.1016/j.ejor.2015.10.007
- Pan, Q.-K., Wang, L., Mao, K., Zhao, J.-H., & Zhang, M. (2013). An effective artificial bee colony algorithm for a real-world hybrid flowshop problem in steelmaking process. IEEE Transactions on Automation Science and Engineering, 10(2), 307–322. https://doi.org/10.1109/TASE.2012.2204874
- Pan, R., Wang, Q., Li, Z., Cao, J., & Zhang, Y. (2022). Steelmaking-continuous casting scheduling problem with multi-position refining furnaces under time-of-use tariffs. Annals of Operations Research, 310(1), 119–151. https://doi.org/10.1007/s10479-021-04217-7
- Pang, X-f., Gao, L., Pan, Q-k., Tian, W-h., & Yu, S-p (2017). A novel Lagrangian relaxation level approach for scheduling steelmaking-refining-continuous casting production. Journal of Central South University, 24(2), 467–477. https://doi.org/10.1007/s11771-017-3449-1
- Park, H. (2002). Production scheduling in integrated steel mills. PhD diss., Pohang University of Science and Technology.
- Park, S. (2007). Two-dimensional bin packing problems in steel industry. PhD diss., Pohang University of Science and Technology.
- Peng, K., Deng, X., Zhang, C., Pan, Q-k., Ren, L., & Pang, X. (2020). An improved imperialist competitive algorithm for hybrid flowshop rescheduling in steelmaking-refining-continuous casting process. Measurement and Control, 53(9–10), 1920–1928. https://doi.org/10.1177/0020294020960187
- Peng, K., Deng, X., Zhang, C., Shen, W., Song, Y., Mou, J., & Liu, A. (2022). Elite solutions and tabu assisted variable neighbourhood descent for rescheduling problems in the steelmaking-refining-continuous casting process. Flexible Services and Manufacturing Journal. https://doi.org/10.1007/s10696-022-09465-8
- Peng, K., Pan, Q-k., Gao, L., Zhang, B., & Pang, X. (2018). An improved artificial bee colony algorithm for real-world hybrid flowshop rescheduling in steelmaking-refining-continuous casting process. Computers & Industrial Engineering, 122, 235–250. https://doi.org/10.1016/j.cie.2018.05.056
- Peng, K., Pan, Q., & Zhang, B. (2018). An improved artificial bee colony algorithm for steelmaking- refining-continuous casting scheduling problem. Chinese Journal of Chemical Engineering, 26(8), 1727–1735. https://doi.org/10.1016/j.cjche.2018.06.008
- Rahal, S., Li, Z., & Papageorgiou, D. J. (2020). Proactive and reactive scheduling of the steelmaking and continuous casting process through adaptive robust optimization. Computers & Chemical Engineering, 133, 106658. https://doi.org/10.1016/j.compchemeng.2019.106658
- Ribas, I., Leisten, R., & Framiñan, J. M. (2010). Review and classification of hybrid flow shop scheduling problems from a production system and a solutions procedure perspective. Computers & Operations Research, 37(8), 1439–1454. https://doi.org/10.1016/j.cor.2009.11.001
- Ruf, C., Bard, J. F., & Kolisch, R. (2022). Workforce capacity planning with hierarchical skills, long-term training, and random resignations. International Journal of Production Research, 60(2), 783–807. https://doi.org/10.1080/00207543.2021.2017058
- Ruiz, R., & Vázquez-Rodríguez, J. A. (2010). The hybrid flow shop scheduling problem. European Journal of Operational Research, 205(1), 1–18. https://doi.org/10.1016/j.ejor.2009.09.024
- Sahai, Y. (2016). Tundish technology for casting clean steel: A review. Metallurgical and Materials Transactions B, 47(4), 2095–2106. https://doi.org/10.1007/s11663-016-0648-3
- Hornig, S., & Javier, E. (2013). Scheduling multi-stage batch production systems with continuity constraints: The steelmaking and continuous casting system. PhD diss, Publikationsserver der RWTH Aachen University.
- Sbihi, A., Bellabdaoui, A., & Teghem, J. (2014). Solving a mixed integer linear program with times setup for the steel-continuous casting planning and scheduling problem. International Journal of Production Research, 52(24), 7276–7296. https://doi.org/10.1080/00207543.2014.919421
- Sbihi, A., & Chemangui, M. (2018). A genetic algorithm for the steel continuous casting with inter-sequence dependent setups and dedicated machines. RAIRO - Operations Research, 52(4–5), 1351–1376. https://doi.org/10.1051/ro/2018023
- Schaus, P., Van Hentenryck, P., Monette, J.-N., Coffrin, C., Michel, L., & Deville, Y. (2011). Solving steel mill slab problems with constraint-based techniques: CP, LNS, and CBLS. Constraints, 16(2), 125–147. https://doi.org/10.1007/s10601-010-9100-5
- Shaw, P. (1998). Using constraint programming and local search methods to solve vehicle routing problems. In International conference on principles and practice of constraint programming (pp. 417–431). Springer.
- Shuang-Ping, W., & An-Jun, X. (2022). An improved elitist GA-based solution for integrated batch planning problem in a steelmaking plant. ISIJ International, 62(6), 1227–1238. https://doi.org/10.2355/isijinternational.ISIJINT-2021-137
- Song, S. H. (2009). A nested column generation algorithm to the meta slab allocation problem in the steel making industry. International Journal of Production Research, 47(13), 3625–3638. https://doi.org/10.1080/00207540801968625
- Song, S. H. (2014). An integrated formulation for hierarchical cast design problems in the steel making industry. International Journal of Production Research, 52(5), 1443–1454. https://doi.org/10.1080/00207543.2013.844374
- Sun, L., Jin, H., & Li, Y. (2018). Research on scheduling of iron and steel scrap steelmaking and continuous casting process aiming at power saving and carbon emissions reducing. IEEE Robotics and Automation Letters, 3(4), 3105–3112. https://doi.org/10.1109/LRA.2018.2849500
- Sun, L., Liu, W., Xu, B., Chai, T. (2010). The scheduling of steel-making and continuous casting process using branch and cut method via CPLEX optimization. In 5th international conference on computer sciences and convergence information technology (pp. 716–721).
- Sun, L., & Luan, F. (2015). Near optimal scheduling of steel-making and continuous casting process based on charge splitting policy. IFAC-PapersOnLine, 48(3), 1610–1615. https://doi.org/10.1016/j.ifacol.2015.06.316
- Sun, L., Luan, F., Ying, Y., & Mao, K. (2017). Rescheduling optimization of steelmaking-continuous casting process based on the Lagrangian heuristic algorithm. Journal of Industrial & Management Optimization, 13(3), 1431–1448. https://doi.org/10.3934/jimo.2016081
- Sun, L., & Wang, X. (2013). Application of mix optimization scheduling approach for steelmaking-continuous casting process based on actual steelmaking industry. Journal of Iron and Steel Research International, 20(10), 1–9. https://doi.org/10.1016/S1006-706X(13)60168-5
- Sun, L., & Yu, S. (2015). Scheduling a real-world hybrid flow shop with variable processing times using Lagrangian relaxation. The International Journal of Advanced Manufacturing Technology, 78(9-12), 1961–1970. https://doi.org/10.1007/s00170-014-6754-y
- Sun, L., Yu, Y., Li, C., Stephen Cloete, D., & Bai, S. (2020). Research on decision support method for charge batch planning of steelmaking-continuous casting under Lagrangian framework. IFAC-PapersOnLine, 53(2), 11138–11143. https://doi.org/10.1016/j.ifacol.2020.12.278
- Sun, W., Wang, Q., Zheng, Z., & Cai, J. (2020). Material–energy–emission nexus in the integrated iron and steel industry. Energy Conversion and Management, 213, 112828. https://doi.org/10.1016/j.enconman.2020.112828
- Sun, W., Wang, Q., Zhou, Y., & Wu, J. (2020). Material and energy flows of the iron and steel industry: Status quo, challenges and perspectives. Applied Energy, 268, 114946. https://doi.org/10.1016/j.apenergy.2020.114946
- Takahashi, T., Konishi, M., Tamura, S., Hanaoka, H., & Nakagawa, H. (1989). Scheduling for steel making process using mathematical programming method. IFAC Proceedings Volumes, 22(11), 129–134. https://doi.org/10.1016/S1474-6670(17)53099-2
- Tan, Y., Huang, Y., & Liu, S. (2013). Two-stage mathematical programming approach for steelmaking process scheduling under variable electricity price. Journal of Iron and Steel Research International, 20(7), 1–8. https://doi.org/10.1016/S1006-706X(13)60118-1
- Tan, Y., & Liu, S. (2014). Models and optimisation approaches for scheduling steelmaking–refining–continuous casting production under variable electricity price. International Journal of Production Research, 52(4), 1032–1049. https://doi.org/10.1080/00207543.2013.828179
- Tan, Y., Zhou, M., Zhang, Y., Guo, X., Qi, L., & Wang, Y. (2020). Hybrid scatter search algorithm for optimal and energy-efficient steelmaking-continuous casting. IEEE Transactions on Automation Science and Engineering, 17(4), 1814–1828. https://doi.org/10.1109/TASE.2020.2979079
- Tang, L., & Jiang, S. (2009). The charge batching planning problem in steelmaking process using Lagrangian relaxation algorithm. Industrial & Engineering Chemistry Research, 48(16), 7780–7787. https://doi.org/10.1021/ie801454k
- Tang, L., Liu, J., Rong, A., & Yang, Z. (2000). A mathematical programming model for scheduling steelmaking-continuous casting production. European Journal of Operational Research, 120(2), 423–435. https://doi.org/10.1016/S0377-2217(99)00041-7
- Tang, L., Liu, J., Rong, A., & Yang, Z. (2001). A review of planning and scheduling systems and methods for integrated steel production. European Journal of Operational Research, 133(1), 1–20. https://doi.org/10.1016/S0377-2217(00)00240-X
- Tang, L., Luh, P. B., Liu, J., & Fang, L. (2002). Steel-making process scheduling using Lagrangian relaxation. International Journal of Production Research, 40(1), 55–70. https://doi.org/10.1080/00207540110073000
- Tang, L., & Luo, J. (2007). A new ILS algorithm for cast planning problem in steel industry. ISIJ International, 47(3), 443–452. https://doi.org/10.2355/isijinternational.47.443
- Tang, L., Meng, Y., Wang, G., Chen, Z.-L., Liu, J., Hu, G., Chen, L., & Zhang, B. (2014). Operations research transforms Baosteel’s operations. Interfaces, 44(1), 22–38. https://doi.org/10.1287/inte.2013.0719
- Tang, L., & Wang, G. (2008). Decision support system for the batching problems of steelmaking and continuous-casting production. Omega, 36(6), 976–991. https://doi.org/10.1016/j.omega.2007.11.002
- Tang, L., Wang, G., & Chen, Z.-L. (2014). Integrated charge batching and casting width selection at Baosteel. Operations Research, 62(4), 772–787. https://doi.org/10.1287/opre.2014.1278
- Tang, L., Wang, G., Liu, J., & Liu, J. (2011). A combination of Lagrangian relaxation and column generation for order batching in steelmaking and continuous-casting production. Naval Research Logistics (NRL), 58(4), 370–388. https://doi.org/10.1002/nav.20452
- Tang, L., Yang, Z., & Wang, M. (1996). Model and algorithm of the furnace charge plan for steelmaking continuous casting production scheduling. Journal of Northeastern University, 17(4), 440–445.
- Tang, L., Zhao, Y., & Liu, J. (2014). An improved differential evolution algorithm for practical dynamic scheduling in steelmaking-continuous casting production. IEEE Transactions on Evolutionary Computation, 18(2), 209–225. https://doi.org/10.1109/TEVC.2013.2250977
- Unlu, Y., & Mason, S. J. (2010). Evaluation of mixed integer programming formulations for non-preemptive parallel machine scheduling problems. Computers & Industrial Engineering, 58(4), 785–800. https://doi.org/10.1016/j.cie.2010.02.012
- van Beek, M. (2018). A review of mathematical programming in integrated iron-and steelmaking. Chemie Ingenieur Technik, 90(10), 1568–1575. https://doi.org/10.1002/cite.201800027
- Van Hentenryck, P., Michel, L. (2008). The steel mill slab design problem revisited. In International conference on integration of artificial intelligence (AI) and operations research (or) techniques in constraint programming (pp. 377–381). Springer.
- Vasko, F. J., Cregger, M. L., Stott, K. L., & Richard Woodyatt, L. (1994). Assigning slabs to orders: An example of appropriate model formulation. Computers & Industrial Engineering, 26(4), 797–800. https://doi.org/10.1016/0360-8352(94)90015-9
- Vasko, F. J., Newhart, D. D., & Stott, K. L., Jr. (1999). A hierarchical approach for one-dimensional cutting stock problems in the steel industry that maximizes yield and minimizes overgrading. European Journal of Operational Research, 114(1), 72–82. https://doi.org/10.1016/S0377-2217(98)00035-6
- Vasko, F. J., Wolf, F. E., & Pflugrad, J. A. (1991). An efficient heuristic for planning mother plate requirements at Bethlehem Steel. Interfaces, 21(2), 1–7. https://doi.org/10.1287/inte.21.2.1
- Vasko, F. J., Wolf, F. E., & Stott, K. L. (1989). A practical solution to a fuzzy two-dimensional cutting stock problem. Fuzzy Sets and Systems, 29(3), 259–275. https://doi.org/10.1016/0165-0114(89)90039-0
- Wang, G., Guo, Q., Xu, W., & Tang, L. (2022). A graph partitioning based cooperative coevolution for the batching problem in steelmaking production. International Journal of Production Research, 60(19), 5876–5891. https://doi.org/10.1080/00207543.2021.1973137
- Wang, G-r., Li, Q-q., & Wang, L-h (2015). An improved cross entropy algorithm for steelmaking-continuous casting production scheduling with complicated technological routes. Journal of Central South University, 22(8), 2998–3007. https://doi.org/10.1007/s11771-015-2836-8
- Wang, Q., Wang, H., & Huang, M. (2021). An effective evolutionary algorithm for steelmaking and continuous casting scheduling [Paper presentation]. In 2021 33rd Chinese Control and Decision Conference (pp. 4039–4044).
- Wang, X., Chai, T., Zheng, B., Wang, H. (2010). Two-stage optimized scheduling method and application for steelmaking and continuous casting. In Proceedings of the 2010 American control conference (pp. 6109–6114).
- Wang, Y.-Z., Zheng, Z., Zhu, M.-M., Zhang, K.-T., & Gao, X.-Q. (2022). An integrated production batch planning approach for steelmaking-continuous casting with cast batching plan as the core. Computers & Industrial Engineering, 173, 108636. https://doi.org/10.1016/j.cie.2022.108636
- Wang, K., Che, A., & Feng, J. (2022). Energy-efficient unrelated parallel machine scheduling with general position-based deterioration. International Journal of Production Research, 61(17), 5886–5900. https://doi.org/10.1080/00207543.2022.2118887
- Whitley, D. (1994). A genetic algorithm tutorial. Statistics and Computing, 4(2), 65–85. https://doi.org/10.1007/BF00175354
- Worapradya, K., & Thanakijkasem, P. (2015). Proactive scheduling for steelmaking-continuous casting plant with uncertain machine breakdown using distribution-based robustness and decomposed artificial neural network. Asia-Pacific Journal of Operational Research, 32(02), 1550010. https://doi.org/10.1142/S0217595915500104
- Wu, X., & Che, A. (2020). Energy-efficient no-wait permutation flow shop scheduling by adaptive multi-objective variable neighborhood search. Omega, 94, 102117. https://doi.org/10.1016/j.omega.2019.102117
- Wy, J., & Kim, B.-I. (2010). Two-staged guillotine cut, two-dimensional bin packing optimisation with flexible bin size for steel mother plate design. International Journal of Production Research, 48(22), 6799–6820. https://doi.org/10.1080/00207540903317523
- Xiong, J., Xing, L-n., & Chen, Y-w (2013). Robust scheduling for multi-objective flexible job-shop problems with random machine breakdowns. International Journal of Production Economics, 141(1), 112–126. https://doi.org/10.1016/j.ijpe.2012.04.015
- Xu, C., Sand, G., Harjunkoski, I., & Engell, S. (2012). A new heuristic for plant-wide schedule coordination problems: The intersection coordination heuristic. Computers & Chemical Engineering, 42, 152–167. https://doi.org/10.1016/j.compchemeng.2011.12.014
- Xu, W., Tang, L., & Pistikopoulos, E. N. (2018). Modeling and solution for steelmaking scheduling with batching decisions and energy constraints. Computers & Chemical Engineering, 116, 368–384. https://doi.org/10.1016/j.compchemeng.2018.03.010
- Xu, Z-j., Zheng, Z., & Gao, X-q (2021). Operation optimization of the steel manufacturing process: A brief review. International Journal of Minerals, Metallurgy and Materials, 28(8), 1274–1287. https://doi.org/10.1007/s12613-021-2273-7
- Xu, Z., Zheng, Z., & Gao, X. (2020). Energy-efficient steelmaking-continuous casting scheduling problem with temperature constraints and its solution using a multi-objective hybrid genetic algorithm with local search. Applied Soft Computing, 95, 106554. https://doi.org/10.1016/j.asoc.2020.106554
- Xuan, H., & Li, B. (2013). Scheduling dynamic hybrid flowshop with serial batching machines. Journal of the Operational Research Society, 64(6), 825–832. https://doi.org/10.1057/jors.2012.64
- Xuan, H., & Tang, L. (2007). Scheduling a hybrid flowshop with batch production at the last stage. Computers & Operations Research, 34(9), 2718–2733. https://doi.org/10.1016/j.cor.2005.10.014
- Xue, Y-c., Mei, Z-q., & Yang, Q. (2006). Optimum steelmaking cast plan with unknown cast number based on the pseudo TSP model [Paper presentation]. In 2006 IEEE international conference on systems, man and cybernetics (vol. 5, pp. 3721–3726). IEEE. https://doi.org/10.1109/ICSMC.2006.384708
- Xue, Y.-C., Wang, X., & Li, S.-Y. (2005). Improved genetic algorithm for integrated steelmaking optimum charge plan. IFAC Proceedings Volumes, 38(1), 61–66. https://doi.org/10.3182/20050703-6-CZ-1902.01494
- Yadollahpour, M. R., & ArbabShirani, B. (2016). A comprehensive solution for continuous casting production planning and scheduling. The International Journal of Advanced Manufacturing Technology, 82(1-4), 211–226. https://doi.org/10.1007/s00170-015-7314-9
- Yang, F., & Li, Q. (2014). Improved cross entropy algorithm for the optimum of charge planning problem. Abstract and Applied Analysis, 2014(7), 1–5. https://doi.org/10.1155/2014/857521
- Yang, J-p., Wang, B-l., Liu, Q., Guan, M., Li, T-k., Gao, S., Guo, W-d., & Liu, Q. (2020). “Scheduling model for the practical steelmaking-continuous casting production and heuristic algorithm based on the optimization of “Furnace-caster Matching” mode. ISIJ International, 60(6), 1213–1224. https://doi.org/10.2355/isijinternational.ISIJINT-2019-423
- Yang, J., Wang, B., Zou, C., Li, X., Li, T., & Liu, Q. (2018). Optimal charge planning model of steelmaking based on multi-objective evolutionary algorithm. Metals, 8(7), 483. https://doi.org/10.3390/met8070483
- Ye, Y., Li, J., Li, Z., Tang, Q., Xiao, X., & Floudas, C. A. (2014). Robust optimization and stochastic programming approaches for medium-term production scheduling of a large-scale steelmaking continuous casting process under demand uncertainty. Computers & Chemical Engineering, 66, 165–185. https://doi.org/10.1016/j.compchemeng.2014.02.028
- Yi, J., Jia, S-j., & Du, B. (2021). Hybrid optimization for charge planning problem in twin strands continuous casting production. Journal of Iron and Steel Research International, 28(5), 520–529. https://doi.org/10.1007/s42243-021-00578-2
- Yi, J., Jia, S-j., Du, B., & Liu, Q. (2019). Multi-objective model and optimization algorithm based on column generation for continuous casting production planning. Journal of Iron and Steel Research International, 26(3), 242–250. https://doi.org/10.1007/s42243-018-0143-y
- Yu, G., & Qi, X. (2004). Disruption management: Framework, models and applications. World Scientific.
- Yu, S-p., & Pan, Q-k (2012). A rescheduling method for operation time delay disturbance in steelmaking and continuous casting production process. Journal of Iron and Steel Research International, 19(12), 33–41. https://doi.org/10.1016/S1006-706X(13)60029-1
- Yu, S., Chai, T., & Tang, Y. (2016). An effective heuristic rescheduling method for steelmaking and continuous casting production process with multirefining modes. IEEE Transactions on Systems, Man, and Cybernetics: Systems, 46(12), 1675–1688. https://doi.org/10.1109/TSMC.2016.2604081
- Yu, S., Chai, T., Wang, H., Pang, X., & Zheng, B. (2011). Dynamic optimal scheduling method and its application for converter fault in steelmaking and continuous casting production process. IFAC Proceedings Volumes, 44(1), 9506–9511. https://doi.org/10.3182/20110828-6-IT-1002.01793
- Yuan, F., Wu, S., Song, W., & Xu, A. (2020). Charge plan model for steelmaking-continuous casting section. Metals, 10(9), 1196. https://doi.org/10.3390/met10091196
- Zhang, Q., Liu, S., Zhang, R., & Qin, S. (2021). Column generation algorithms for mother plate design in steel plants. Or Spectrum, 43(1), 127–153. https://doi.org/10.1007/s00291-020-00610-z
- Zhang, R., Lu, J., & Zhang, G. (2011). A knowledge-based multi-role decision support system for ore blending cost optimization of blast furnaces. European Journal of Operational Research, 215(1), 194–203. https://doi.org/10.1016/j.ejor.2011.05.037
- Zhang, R., Lu, K., Huang, K., & Wang, D. (2011). Graph-based model of cast planning problem and its optimization [Paper presentation]. In 2011 Chinese control and decision conference (pp. 1606–1611). IEEE.
- Zhang, X., Hug, G., & Harjunkoski, I. (2017). Cost-effective scheduling of steel plants with flexible EAFs. IEEE Transactions on Smart Grid, 8(1), 239–249. https://doi.org/10.1109/TSG.2016.2575000
- Zhao, S., Meng, Y., Su, L., Liu, J., & Tang, L. (2023). Optimal plate design problem in steel production. International Journal of Production Research, 61(5), 1575–1590. https://doi.org/10.1080/00207543.2022.2043566
- Zhao, Y., Jia, F. Y., Wang, G. S., Wang, L. J. (2011). A hybrid tabu search for steelmaking-continuous casting production scheduling problem. In 2011 International Symposium on Advanced Control of Industrial Processes (pp. 535–540). IEEE
- Zhu, W., Li, Y., Sun, L., Hou, T., Bai, S., Yuan, B., Zhao, L. (2021). Research on batch planning method of steelmaking-continuous casting tundish. In 2021 3rd international conference on industrial artificial intelligence (pp. 1–6). IEEE
Appendix A:
Core batching model for steel-making and continous casting
This section presents a MIP formulation of Steel-making and Continuous Casting Batching Model (SCC-BM) in Section 3.2.
Table
The objective Equation(A1)(A1)
(A1) implies maximising the total weight of assigned slabs and minimising the number of used casts. Constraints Equation(A2)
(A2)
(A2) mean that a slab can have one width and can be assigned to at most one charge. Constraints Equation(A3)
(A3)
(A3) ensure charges in a cast are sequentially used. Constraints Equation(A4)
(A4)
(A4) ensure sequential usage of casts because the first charge in a cast is used if and only if the cast is used. Constraints Equation(A5)
(A5)
(A5) explain that if a cast is used, one cast width must be selected. Constraints Equation(A6)
(A6)
(A6) mean that a slab can be assigned to a cast when the slab can be made of the selected cast width. Constraints Equation(A7)
(A7)
(A7) ensure that if a cast is used, that cast contains at least one slab made with the selected cast width. Constraints Equation(A8)
(A8)
(A8) and Equation(A9)
(A9)
(A9) express a charge’s minimum and maximum weight capacity constraints, respectively. Constraints Equation(A10)
(A10)
(A10) imply that a charge must have exactly one steel grade if that charge is used. Constraints Equation(A11)
(A11)
(A11) mean the steel grade of slabs must be identical to the steel grade of the charge including them. Constraints Equation(A12)
(A12)
(A12) imply that adjacent charges in a cast must have compatible steel grades. Constraints Equation(A13)
(A13)
(A13) –Equation(A16)
(A16)
(A16) present the domains of variables.
Appendix B:
Core scheduling model for steel-making and continuous casting
This section presents a MIP formulation of Steel-making and Continuous Casting Scheduling Model (SCC-SM) in Section 4.1.
Table
The objective Equation(B1)(B1)
(B1) is the minimisation of the total weighted penalty. Each term denotes the penalty for cast break, total waiting time, total earliness, and total tardiness, respectively. Constraints Equation(B2)
(B2)
(B2) ensure that a charge is assigned to exactly one machine at each stage on its route. Constraints Equation(B3)
(B3)
(B3) and Equation(B4)
(B4)
(B4) ensure that one of any two charges must precede the other one if and only if they are assigned to the same machine at a stage. Constraints Equation(B5)
(B5)
(B5) imply that the charges in a cast must be assigned to the same machine at the last stage, and constraints Equation(B6)
(B6)
(B6) restrict their precedence to conform with the given sequence. Constraints Equation(B7)
(B7)
(B7) mean that a charge can be processed only after its release time at a stage. Constraints Equation(B8)
(B8)
(B8) ensure that a charge will start its processing only after its preceding charges processed on the same machine have been completed. Constraints Equation(B9)
(B9)
(B9) mean that the first charge of a cast needs a setup time after completing the last charge of the preceding cast on the same machine at the last stage. Constraints Equation(B10)
(B10)
(B10) and Equation(B11)
(B11)
(B11) imply that the difference of the completion times at two consecutive stages is the sum of waiting time, transportation time, and the processing time at the latter stage. Constraints Equation(B12)
(B12)
(B12) define the idle time between two consecutive charges in a cast at the last stage. By setting a large penalty value π1, the idle time supposedly goes to zero when possible. Constraints Equation(B13)
(B13)
(B13) are from the definition of the earliness and the tardiness of a charge. Constraints Equation(B14)
(B14)
(B14) –Equation(B19)
(B19)
(B19) present the domains of variables.
Appendix C:
Glossary
This glossary explains technical terms that appeared in the manuscript. The glossary is made based on the following URLs:
World Steel Association, http://www.worldsteel.org
American Iron and Steel Institute https://www.steel.org
Ministry of Steel in India https://steel.gov.in/en/
Danieli https://www.danieli.com/en/
We also consider Park (Citation2002) as an additional reference.
A
Alloy Steel. An iron-based mixture is considered to be an alloy steel when manganese is greater than 1.65%, silicon over 0.5%, copper above 0.6%, or other minimum quantities of alloying elements such as chromium, nickel, molybdenum, or tungsten are present. An enormous variety of distinct properties can be created for the steel by substituting these elements in the recipe.
B
Blast Furnace. A towering cylinder lined with heat-resistant (refractory) bricks, used by integrated steel mills to smelt iron from iron ore. Its name comes from the “blast” of hot air and gases forced up through the iron ore, coke, and limestone that load the furnace.
Basic Oxygen Furnace (BOF). A furnace that refines a charge of molten iron and scrap into steel. The BOF, as it is called, blows pure oxygen into the charge, creating a vigorous chemical reaction to refine the metal.
Breakage. Cracks or separation of the steel.
C
Continuous Casting (CC). A process for solidifying steel in the form of a continuous strand rather than individual ingots. Molten steel is poured into open-bottomed, water-cooled moulds. As the molten steel passes through the mould, the outer shell solidifies.
Coke. Coke is the residual solid product obtained upon the carbonisation of coking coal. Depending upon property, coke is known as Hard Coke, Soft Coke, and Metallurgical Coke.
Coil. A metal sheet that has been wound. Once rolled flat, the metal is more than one-quarter mile long; coils are the most efficient way to store and transport sheet steel.
Cold Rolling. Passing a sheet or strip that has previously been hot rolled and picked through cold rolls (below the softening temperature of the metal). Cold rolling makes a product that is thinner, smoother, and stronger than can be made by hot rolling alone.
Conditioning Stand (CS). Conditioning stand is one method of degassing process, which removes unwanted gases from the liquid, which could produce any number of imperfections and defects.
D
Decarburization. A process for further refinement of stainless steel through reduction of carbon content.
E
Electric Arc Furnace (EAF). A furnace that melts steel scrap using the heat generated by a high power electric arc. During the melting process, elements are added to achieve the correct chemistry and oxygen is blown into the furnace to purify the steel.
F
Furnace. The cylindrical or rectangular heating device.
G
Graphic User Interface (GUI) (Jansen, Citation1998). A Graphic User Interface is a type of computer-human interface on a computer.
H
Hot Rolling. Rolling of Steel above the re-crystallisation temperature of steel (normally above 1000 °C) to produce Hot Rolled Long products/Flat Products from semis. Ingots are also hot rolled to get semis. At times blooms are also hot rolled to produce Billets. Rolling Mills used for hot rolling are known as Hot Rolling Mills.
I
Iron Ore. Mineral containing enough iron to be a commercially viable source of the element for use in steelmaking. Except for fragments of meteorites found on Earth, iron is not a free element; instead, it is trapped underneath the Earth’s crust in its oxidized form.
Integrated Steel Mill. Large-scale plant combining iron smelting and steelmaking facilities, usually based on a basic oxygen furnace. May also include systems for turning steel into finished products.
L
Ladle Furnace. The main purpose of the LF is to raise steel temperature and to control the chemical composition between tapping and casting, removing and/or modifying inclusions.
M
Mini-mill. Steel mills that melt scrap metal to produce commodity products. The processes after the continuous casting are the same as integrated steel mills, but they differ greatly in regard to their minimum efficient size, labour relations, product markets, and management style.
P
Pig Iron. The name for the melted iron produced in a blast furnace that contains a large quantity of carbon (above 1.5%). Long ago, when molten iron was poured through a trench underground to flow into shallow earthen holes, the arrangement looked like newborn pigs suckling. The central channel became known as the “sow,” and the moulds were “pigs.”
Plate. Sheet steel with a width of more than eight inches, with a thickness ranging from one quarter of an inch to more than one foot.
R
Refining. Processes that control the ratio of micro-ingredients of steel.
Resource-Task Network (RTN). A type of network representation of a production environment. See Maravelias (Citation2021) for details.
Ruhrstahl Heraeus. The Ruhrstahl-Heraeus (RH) process, also called Vacuum Circulation Process, is the vacuum process commonly used in integrated steel plants. It was developed in response to BOF steel plants’ need to treat large ladles for short cycle times.
Rolling. Rolling is a process in which slabs are passed through rolls to become thinner and be the final products (plates and coils).
Rolling Mill. Equipment that reduces and transforms the shape of semi-finished or intermediate steel products by passing the material through a gap between rolls that is smaller than the entering materials.
Re-rolling. Another term for cold rolling.
S
Steel-making.The hot metal, tapped from the blast furnace has a carbon content of between 4 and 5% as well as impurities such as phosphorus and sulfur that negatively impact its strength and durability. This hot metal is charged into a basic oxygen furnace or “converter” along with scrap. A high-pressure stream of pure oxygen is then injected into the hot metal, transforming impurities into gases and slag. The result of this relatively brief process is steel that’s ready to be formed into semi-finished products.
Slab. A semi-finished steel product that is hot-rolled down from an ingot or strand cast. It is wide and rectangular in shape. (9 inches thick, 32–48 inches wide by 220–398 inches long) Slabs are used in the manufacture of sheets, strips, plates and other flat-rolled steel products.
Strand. (1) A component of a hydraulic system, which is made up of a number of stacks. (2) Sets of rolls. (3) (Batch Anneal) Same as “stool.”
T
Tundish. The shallow refractory-lined basin on top of the continuous caster. It receives the liquid steel from the ladle, prior to the cast, allowing the operator to precisely regulate the flow of metal into the mould.
V
Vacuum Degassing. An advanced steel refining facility that removes oxygen, hydrogen and nitrogen under low pressures (in a vacuum) to produce ultra-low-carbon steel for demanding electrical and automotive applications. Normally performed in the ladle, the removal of dissolved gases results in cleaner, higher quality, more pure steel (see Ladle Metallurgy).