Abstract
Utilizing McKibben pneumatic artificial muscles is a widely accepted method to achieve compliance in applications where adaptability is desirable. Controlling their lengths in an open-loop based on pressure is still challenging, due to their non-linear nature, even more so if external perturbations can occur. Generating inflation or length feedback was, therefore, the goal of several previous sensor designs. However, preserving the actuator's flexibility and lightweight characteristics was not a typical consideration for those designs. This paper will describe our design of an inflation sensor made from conductive fabrics. Our design's minimalistic approach resulted in an easy-to-manufacture sensor that does not alter the actuator's shape or flexibility that also can be individually replaced. This design allows the sensor to be deployed into complex muscular systems, where rigid sensors potentially interfere with adjacent actuators. In this paper, we will describe the production of our sensor, its static response, and its dynamic response under load. The results of our experiments confirmed the viability of the usage of conductive fabrics for this type of sensor while conserving the desirable properties of McKibben pneumatic artificial muscles.
GRAPHICAL ABSTRACT
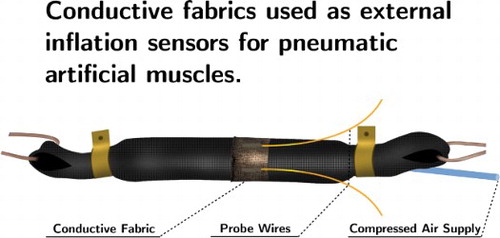
Acknowledgments
We thank Dr. Shunsuke Shigaki and Mr. Tsung-Yuan Chen for their valuable comments on visualizing our results.
Disclosure statement
No potential conflict of interest was reported by the author(s).
Additional information
Notes on contributors
Arne Hitzmann
Arne Hitzmann received his MSc in Computer Science from the Ostfalia University of Applied Sciences in 2016. Currently he is acquiring his PhD in Engineering from the Osaka University. His research interests are humanoid robotics, artificial intelligence and bioinspired control schemes.
Yanlin Wang
Yanlin Wang received her B.E. degree in mechanical engineering from Dalian University of Technology in 2017. Since 2019, she joined Prof. Hosoda's Adaptive Robotics Laboratory at Osaka University as a research student and is currently a master student. Her research interest is sensory reflex control on robots.
Tyler Kessler
Tyler Kessler received his bachelor's degree in Computer Engineering from Shepherd University. After working as a software engineer, he is currently pursuing a master's degree studying humanoid robotics at Osaka University's Graduate School of Engineering Science. His research goal is to utilize bioinspired mechanics to develop control schemes for humanoid robots.
Koh Hosoda
Koh Hosoda received his PhD degree in Mechanical Engineering from Kyoto University, Japan in 1993. He was an assistant professor of Mechanical Engineering Department from 1993 to 1997, and an associate professor of Graduate School of Engineering from 1997 to 2010, at Osaka University. He was a guest professor in Artificial Intelligence Laboratory, University of Zurich from April 1998 to March 1999. He was a group leader of JST Asada ERATO Project from 2005 to 2010. From 2010 to 2014, he was a professor of Graduate School of Information Science and Technology, Osaka University. Since 2014, he has been a professor of Graduate School of Engineering Science, Osaka University.