Abstract
Climate change is recognized to be one of the most serious challenges facing mankind today. Driven by anthropogenic activities, it is known to be a direct threat to our food and water supplies and an indirect threat to world security. Increase in the concentration of carbon dioxide and other greenhouse gases in the atmosphere will certainly affect hydrological regimes. The consequent global warming is expected to have major implications on water resources management. The objective of this research is to present a general approach for evaluating the impacts of potential climate change on streamflow in a river basin in the humid tropical zone of India. Large-scale global climate models (GCMs) are the best available tools to provide estimates of the effect of rising greenhouse gases on rainfall and temperature. However the spatial resolution of these models (250 km × 250 km) is not compatible with that of watershed hydrological models. Hence the outputs from GCMs have to be downscaled using regional climate models (RCMs), so as to project the output of a GCM to a finer resolution (50 km × 50 km). In the present work, the projections of a GCM for two scenarios, A2 and B2 are downscaled by a RCM to project future climate in a watershed. Projections for two important climate variables, viz. rainfall and temperature are made. These are then used as inputs for a physically-based hydrological model, SWAT, in order to evaluate the effect of climate change on streamflow and vegetative growth in a humid tropical watershed.
Citation Raneesh, K. Y. & Santosh, G. T. (2011) A study on the impact of climate change on streamflow at the watershed scale in the humid tropics. Hydrol. Sci. J. 56(6), 946–965.
Résumé
Il est admis que l'adaptation au changement climatique est l'un des plus grands défis actuels de l'humanité. Sous la pression des activités anthropiques, ce changement est une menace directe sur notre alimentation et notre approvisionnement en eau, et une menace indirecte pour la sécurité mondiale. L'augmentation de la concentration en dioxyde de carbone et autres gaz à effet de serre dans l'atmosphère va certainement affecter les régimes hydrologiques. Le réchauffement global devrait ainsi avoir des répercussions majeures sur la gestion des ressources en eau. L'objectif de cette étude est d'évaluer par une approche générale les impacts possibles du changement climatique sur les débits d'un bassin versant dans une région tropicale humide de l'Inde. Les modèles climatiques globaux à grande échelle (MCG) sont les meilleurs outils disponibles pour fournir des estimations de l'augmentation de l'effet de serre sur l'augmentation des précipitations et des températures. Mais la résolution spatiale de ces modèles (250 km × 250 km) n'est pas compatible avec celle des modèles hydrologiques. Les sorties des MCG doivent donc subir une réduction d'échelle en utilisant des modèles climatiques régionaux (MCR), de manière à projeter la sortie d'un MCG à une résolution plus fine (50 km × 50 km). Dans la présente étude, les projections de MCG pour deux scénarios, A2 et B2, sont réduites par un MCR pour prévoir le climat futur dans un bassin versant. On effectue la projection de deux variables climatiques importantes: les précipitations et la température. Elles sont utilisées ensuite en entrée d'un modèle hydrologique à base physique, SWAT, afin d'évaluer l'effet du changement climatique sur les débits et la croissance végétative dans un bassin tropical humide.
1 INTRODUCTION
Rising amount of greenhouse gases in the atmosphere can cause drastic changes in climate. This fact has resulted in a governing concern in the scientific community on how it would affect the water resources and water availability scenarios in different parts of the world. Climate variability and change are expected to alter regional hydrological conditions and result in a variety of impacts on water resource systems throughout the world. Large-scale global climate models (GCMs) are powerful tools for evaluating the impact of increasing CO2 concentrations in the atmosphere on climate, even though there is still considerable uncertainty in the results. The coarse resolution of the GCMs prohibits their direct use at local or regional scales. Also, most river basins are smaller than the grid dimensions (300 × 300 km) of currently used GCMs. Statistical downscaling using a pre-defined statistical relationship or dynamic downscaling using a regional climate model (RCM) nested in a GCM is necessary to assess hydrological impacts of climate change (Marengo et al. Citation2009). The application of statistical downscaling methods is hindered by the lack of long-term precipitation records needed to fit statistical models of precipitation occurrence and amount. Additionally, the density of raingauges is generally low. Regional climate models are the best tools for dynamic downscaling of climate features in order to make projections for a particular region (Jones et al. Citation2004). The PRECIS (Providing Regional Climate for Impact Studies) model is a high-resolution (<50 km), coupled ocean–atmosphere RCM designed by the UK Met Office (Jones et al. Citation2004) specifically for use by non-climate scientists to produce detailed climate scenarios for impact assessment studies. The PRECIS model has been validated extensively at both regional and catchment scales in different parts of the world and represents well the mean climate (Gosain et al. Citation2006, Mileham et al. Citation2008).
Linsley (Citation1960) identified determination of the response of a catchment to climate change as the most serious problem facing water resource managers. Nemec and Schaake (Citation1982) analysed the sensitivity of water resource systems to climate variation by performing simulations in a deterministic framework. The impact of climate change on streamflow in arid and humid basins was reported. At present, projections of freshwater resources in post-climate-change scenarios are expressed in terms of annual river discharges (Kamga Citation2001, Legesse et al. Citation2003, Vörösmarty et al. Citation2005, Messager et al. Citation2006, Kundzewicz et al. Citation2008). Hydrological models are forced with RCM data to address the impact of climate change on the hydrological response of river basins (Jacob Citation2001, de Wit et al. Citation2007, Mileham et al. Citation2009), because observations are scarce at the spatial and temporal resolutions at which the spatially-distributed models are run. In many of the climate impact studies, the hydrological model is forced with RCM data, without an attempt to assess the quality of the RCM data (Middelkoop et al. Citation2001, Arnell et al. Citation2003). Obviously, the reliability of the spatially-distributed model output is strongly dependent on the quality of the climate forcing data. Christensen et al. (Citation2008) stated that one inherent source of uncertainty comes from the RCM's inability to simulate present-day climate conditions accurately. Therefore, it is of major importance that the RCM output is validated with historical observations, before running the hydrological model with the RCM data. Obviously, the reliability of the spatially-distributed model output is strongly dependent on the quality of the climate forcing data. Applying a bias correction to the RCM data often seems necessary to match the RCM data with the observed meteorological data (Shabalova et al. Citation2003, Kleinn et al. Citation2005, Leander and Buishand Citation2007).
Vegetative growth is affected directly by the changing concentrations of atmospheric gases, primarily CO2, and also affected indirectly by the influence of changing atmospheric gas composition on regional climate and local weather patterns (Thomson et al. Citation2005). Current indications are that global agricultural production will not decline significantly with a doubling of atmospheric CO2 concentrations. However, the direct and indirect effects of rising CO2 levels may have significant consequences for regional production that would require adapting crops and crop varieties grown as well as agricultural management practices (Reilly et al. Citation2001). While the increase in CO2 concentrations is directly measurable, the direct impact on plant growth remains unclear. In experiments in controlled environments, and to a lesser extent in field studies, a CO2 fertilization effect has been observed; agricultural crops grown at higher concentrations of CO2 experience increased growth rates, improved water-use efficiency and higher yields (Allen et al. Citation1998, Maroco et al. Citation1999). However, most studies of this effect have evaluated the response of single crops or fields through one growing season where climate and soil conditions are held constant. Because plant response to CO2 depends on other limitations in the environment, such as water and nutrient availability, and soil characteristics, uncertainty remains about how accurately and consistently the same effects can be applied to simulations over landscape scales. A higher concentration of atmospheric CO2 is expected to cause a decline in plant transpiration and improve water-use efficiency, which would leave more water available for runoff (Wigley and Jones Citation1985). However, the higher CO2 would, if water and nutrients are available, also increase plant cover in some areas, increasing the proportion of precipitation consumed by plants (Allen et al. Citation1991, McCabe and Wolock Citation1992). The effects of climate change on water resources and vegetation are closely linked, and must be considered together.
Climate change is expected to have an impact on hydrological systems because of changes in precipitation, temperature and evapotranspiration, which are the primary input variables for the terrestrial part of the hydrological cycle. The most important sources and driving forces of climate change are at the regional scale. Considering that the political and technical measures to adapt to climate change also take place at a regional level, regional-scale studies on climate change are a matter of great concern (Varis et al. Citation2004). The use of several simulations from the same RCM with varying emissions scenarios and GCM forcings better reflects the uncertainty in the range of possible future climate change (Raisanen et al. Citation2004, Van den Hurk et al. Citation2005, Salathe Citation2005). Unfortunately, the number of simulations from an RCM is often limited and only available for certain time periods because climate model simulations are computationally demanding. Regional climate models contain the same representations of atmospheric dynamical and physical processes as GCMs. The typical grid size of RCM simulations has been 25 or 50 km. For RCMs with a typical grid spacing of 25–50 km, this equates to providing information on scales of 100 km although this also depends on other factors such as season and topography. Linked to the spatial resolution of RCMs, there is also a minimum temporal scale on which RCMs can provide meaningful information. The linkage between RCMs and hydrological models requires spatial resolutions of less than 10 km in order to get results which are more dependable. Hence, further downscaling of climate data to the level of regional hydrological models is the need of the hour.
Quantitative estimation of the hydrological effects of climate change will be helpful in understanding potential water resource problems and making better planning decisions. With economic development and increase in population, the conflict between water use and water supply will become increasingly grave in the future. Understanding the possible impacts of climate change on water resources is of utmost importance for ensuring their appropriate management and utilization. For this purpose, an appropriate hydrological model, the SWAT (Soil and Water Assessment Tool) is identified and this model is run with the projections from the RCM as input besides the watershed parameters. The main objective of this study is to evaluate the effect of climate change on future streamflow at a river gauge station Kuniyil, on the Chaliyar River in Kerala, India. The AVSWAT2000 (ArcView Interface of the Soil and Water Assessment Tool), a physically-based distributed hydrological model, is used to perform watershed simulation and project streamflow at the station. The model was initially calibrated and subsequently its performance was validated using observed streamflow records at the Kuniyil gauge station during the period 1987–2006. Thereafter, temperature and precipitation in climate change scenarios A2 and B2 were projected using the RCM named PRECIS. These projections were corrected for bias and then input into the SWAT model to compute streamflow and evapotranspiration in the post-climate-change period, 2071–2100. Even though using RCM outputs in a hydrological model is not a new topic, there have been few climate change impact studies on watersheds in humid tropical zones. An investigation into the effect of climate change on vegetative growth is also conducted.
2 MATERIALS AND METHODS
2.1 Characteristics of the study area
The River Chaliyar is the fourth longest river in Kerala, India, with a length of about 170 km. The geographical area of the part of the river basin in Kerala () is 2530 km2 and it lies between latitude 11°06′–11°36′N and longitude 75°48′–76°33′E. The basin exhibits undulating topography with steep slopes. Physiographically, the basin can be divided into three regions: lowlands, mostly comprising the coastal belt; midlands, comprising mostly laterites; and highlands, covered by hard rocks. The main river starts from the Elambalari hills at an altitude of 2067 m a.m.s.l. The climate in the basin is typically that of the humid tropics. The southwest monsoon (June–August) contributes about 60% and the northeast monsoon (September–November) contributes 25% of the annual rainfall respectively. The remaining 15% is received as pre-monsoon showers during the months April–May. December–March is considered to be the dry period. The average annual precipitation in the basin is 3012 mm and the maximum and minimum temperatures are 34°C and 24°C respectively. The annual average relative humidity ranges from 60% (minimum) to 90% (maximum) in summer; the corresponding values for winter are 65% and 85%. The predominant land-use is agriculture (60.04%) and forests (38.74%). Urban areas, pastures, waste lands and rocky areas comprise less than 1% of the total area. The elevation of the watershed varies from about 20 m a.m.s.l. in the lowland areas to around 2250 m in the hilltops; with a mean elevation of 338 m and standard deviation of 458 m. With regard to the type of soils, the basin is dominated by loam texture (42.74%), followed by clay (28.66%), clay loam (24.18%) and sandy loam (4.42%). Details pertaining to land-use and soil classifications are presented in and , respectively.
2.2 Data collection and analysis
Hydrological, data such as streamflow and sediment load at the Kuniyil gauging station of the Central Water Commission (CWC), were collected for a period of 26 years from 1981 to 2006. Also, meteorological data, including daily rainfall, temperature, relative humidity and solar radiation at the Kottaparamba Observatory of the Centre for Water Resources Development and Management (CWRDM), were collected for the same period. shows the location of these stations. Meteorological data from a single station were only used in the simulation. This was necessitated since no other stations were located in the basin. The Chaliyar River basin is located in Malappuram and Kozhikode districts of Kerala and Nilgiris District of Tamil Nadu. This area is covered in the following GTS maps (toposheets) published by the Survey of India (SOI): 49M14, 49M15, 49M16, 58A2, 58A3, 58A4, 58A6, 58A7, 58A8, 58A10, 58A11 and 58A12. These maps were collected and digitized using the ArcGIS 9.0 software. These were then geo-referenced and the geo-referenced maps were digitized using the editor toolbar in ArcGIS 9.0. After preparing the contour layer, the digital elevation model (DEM) of the basin was derived in grid format using the ArcInfo software. The DEM () was used for establishing sub-watershed boundaries and obtaining the elevations of points. summarizes the input data used in this study.
Table 1 Summary of input data for the SWAT model
2.3 Brief description of SWAT
The SWAT model was developed by the US Department of Agriculture (USDA) to assess the impact of land management practices on water, sediment and agricultural chemical yields in large complex watersheds with varying soils, land-use and management conditions over long periods of time. The SWAT model has been extensively used, is in the public domain and can be considered as becoming a standard tool in spatial decision support systems (Schuol and Abbaspour Citation2007, Keshta et al. Citation2009, Cibin et al. Citation2010). The SWAT model represents all the components of the hydrological cycle, including: rainfall, snow, interception storage, surface runoff, infiltration, evapotranspiration (ET), lateral flow, percolation, pond and reservoir water balances, shallow and deep aquifers, and channel routing. It includes sediment production based on a modified version of the Universal Soil Loss Equation (USLE) and routing of sediments in river channels. The crop growth model in SWAT is a simplification of the EPIC (Erosion Productivity Impact Calculator) model with the concepts of phenological crop development based on daily accumulated energy units, harvest index for partitioning grain yield, Monteith's approach for potential biomass and water, nutrient and temperature stress adjustments. The SWAT model tracks the movement and transformation of several forms of nitrogen and phosphorus in the watershed. It also tracks the movement and decay of pesticides. The SWAT model has a modular set-up and it is beyond the scope of this paper to enter into detail on each of these modules; readers are referred to the theoretical documentation (Neitsch et al. Citation2001). The SWAT modelling approach has been extensively used since 1993, mainly by hydrologists, for watershed hydrology related issues (Srinivasan et al. Citation1998, Santhi et al. Citation2001, Cao et al. Citation2006, Schuol and Abbaspour Citation2007, Keshta et al. Citation2009, Cibin et al. Citation2010). Alansi et al. (Citation2009) showed that SWAT was able to simulate and forecast streamflow in the Upper Bernam humid tropical river basin successfully. This model was adopted in this study to evaluate the impacts of climate change on the streamflow and vegetative growth in the Chaliyar River basin.
For modelling purposes, a watershed may be partitioned into a number of sub-watersheds or sub-basins. The part of the Chaliyar River basin in Kerala is delineated into a number of sub-basins using a 20-m digital elevation model (DEM) created in ArcInfo grid format and the stream network map. By partitioning the watershed into sub-basins, the user is able to reference different areas of the watershed to one another spatially. Input information for each sub-basin is grouped or organized into the following categories: climate; hydrological response units or HRUs; ponds/wetlands; groundwater; and the main channel, or reach, draining the sub-basin. Hydrological response units are lumped land areas within the sub-basin that have unique land-use, soil, and management combinations. Once the HRU distribution has been defined, the weather data to be used in a watershed simulation must be imported into SWAT. The SWAT model requires daily data of minimum and maximum temperatures, precipitation, solar radiation, wind speed and relative humidity. It also uses long-term monthly averages of data. In addition, statistical parameters are generated from the long-term daily data in order to simulate missing observations. Surface runoff from daily rainfall was estimated with the modified Soil Conservation Service Curve Number (SCS-CN) method. This method estimates the amount of runoff based on local land use, soil type and the antecedent moisture condition. The Green-Ampt method of estimating infiltration is an alternative option for estimating surface runoff and infiltration (requires sub-daily weather data). Channel routing is simulated using either the variable-storage method or the Muskingum method. The channel sediment routing equation uses a modification of Bagnold's sediment transport equation that estimates the transport concentration capacity as a function of velocity. The model either deposits excess sediment or re-entrains sediment through channel erosion depending on the sediment load entering the channel.
2.3.1 Estimating evapotranspiration in SWAT
Evapotranspiration includes: evaporation from rivers and lakes, bare soil and vegetative surfaces; evaporation from within the leaves of plants (transpiration); and sublimation from ice and snow surfaces. The model computes evaporation from soils and plants separately as described by Ritchie (Citation1972). Potential soil water evaporation is estimated as a function of potential evapotranspiration and leaf area index (area of plant leaves relative to the area of the HRU). Potential evapotranspiration (ET) is the rate at which evapotranspiration would occur from a large area completely and uniformly covered with growing vegetation that has access to an unlimited supply of soil water. This rate is assumed to be unaffected by microclimatic processes such as advection or heat-storage effects. The model offers three options for estimating potential evapotranspiration: Hargreaves (Hargreaves et al. Citation1985), Priestley-Taylor (Priestley and Taylor Citation1972), and Penman-Monteith (Monteith Citation1965). In this study, the Penman-Monteith method was used to estimate ET. Actual soil water evaporation is estimated by using exponential functions of soil depth and water content. Plant transpiration is simulated as a linear function of potential evapotranspiration and leaf area index.
2.3.2 Crop growth model in SWAT
The categories specified in the land-use/land-cover map should be reclassified into SWAT land-cover/crop-types as shown in Three options are available in the model for reclassifying the categories. The first option is to use US Geological Survey (USGS) category codes when creating the map (or use a USGS land-use/land-cover map). The interface provides an ArcView table that identifies different SWAT land-cover/plant-types used to model the various USGS land uses. The second option is to select the SWAT default database land-cover/crop-type or urban code for each category when the land-cover/land-use map theme is loaded in the interface. The third option is to create a look-up table that identifies the four-letter SWAT code for the different categories of land-cover/land-use on the map. Once a land-use SWAT code has been assigned to all map categories, reclassification can be done. In this study, the second option was used. The crop growth parameters for a land-cover/crop-type are stored in a database (crop.dat) file in SWAT. A four-letter code is used to represent the land-cover/crop-type. The codes in the land-cover/crop-type database are used by the GIS interface to link the land-use/land-cover maps to SWAT crop types. When adding a new crop species or land-cover category, the four-letter code for the new crop must be unique. The land-cover/crop-type database contains information needed by SWAT to simulate the growth of a particular crop. The growth parameters in the crop growth database define plant growth under ideal conditions and quantify the impact of water stresses on plant growth. Differences in growth between plant species are defined by the parameters contained in the crop growth database. Several parameters are specified for more than 70 crop/vegetation types in the crop database attached to the SWAT model, i.e. biomass-energy ratio, harvest index, base and optimal temperature for plant growth, maximum leaf area index (LAI), fraction of growing season when LAI declines, maximum root depth, and potential heat units required for maturity of crop. The SWAT land-cover/crop-type codes along with the crop growth parameter values for the study area are listed in
Table 2 Details of land-use/land cover classifications from SWAT
Table 3 Crop growth values linked to SWAT database
The model is able to differentiate between annual and perennial plants. Annual plants grow from the planting date to the harvest date or until the accumulated heat units equal the potential heat units (PHU) for the plant. Perennial plants maintain their root systems throughout the year, becoming dormant in the winter months. They resume growth when the average daily air temperature exceeds the minimum, or base temperature required. The plant growth model is used to assess removal of water and nutrients from the root zone, transpiration and biomass/yield production. Potential plant growth and yield are usually not achieved due to constraints imposed by the environment. The model estimates stresses caused by water, nutrients and temperature. The potential increase in plant biomass on a given day is defined as the increase in biomass under ideal growing conditions. The potential increase in biomass in a day is a function of intercepted energy and the efficiency of the plant in converting energy to biomass. Energy interception is estimated as a function of solar radiation and the leaf area index. The SWAT model allows the user to define management practices taking place in every HRU, as well as the beginning and the end of the growing season, the timing and amounts of fertilizer, pesticide and irrigation applications and the timing of tillage operations. At the end of the growing season, the biomass may be removed from the HRU as yield or placed on the surface as residue.
The SWAT model calculates the maximum daily increase in plant biomass allowed by the daily solar radiation incident on the field. The amount of solar radiation captured by the crop is a function of the LAI and the amount converted into plant biomass is a function of the radiation use efficiency (RUE) which is crop specific. Solar radiation also provides the energy that drives ET. Crop growth is simulated by calculating the potential daily photosynthetic production of biomass. The daily potential growth is retarded by stresses caused by shortages of radiation, water and nutrients, by temperature extremes and by inadequate soil aeration. Planting and harvesting dates are based on accumulated heat units during the growing season, and therefore vary with different climate scenarios. Crop yields are estimated by multiplying above-ground biomass at maturity by a harvest index. In this study, the crop parameters (potential heat units, base and optimal temperatures, length of the growing season, leaf area development parameters) for the crops in the study area were linked to appropriate crops in the SWAT database to obtain the general growth and water use patterns. Forest trees and agricultural crops (row, close grown and land generic) are the dominant in the area. The values of radiation use efficiencies and harvest indices were adjusted to obtain biomass and yield production figures close to the average values reported in literature (KAU Citation2002, Monteith Citation1977). In order to assess the impact of climate change on agricultural productivity, SWAT incorporates equations that adjust RUE for elevated atmospheric CO2 concentrations. Values must be entered for parameters; CO2HI—elevated CO2 atmospheric concentration (ppmv), and BIOEHI—radiation use efficiency at elevated CO2 atmospheric concentration value (kg ha-1 MJ-1 m-2) in the plant database. For simulations in which elevated CO2 levels are not modelled, CO2HI should be set to some number greater than 330 ppmv and BIOEHI should be set to some number greater than BIO_E—radiation use efficiency in ambient CO2 (kg ha-1 MJ-1 m-2). Values of these parameters were obtained from the literature (Challinor et al. Citation2006, Cramer et al. Citation2001, Houghton Citation2003, Bondeau et al. Citation2007).
2.4 Regional climate models and climate change scenarios
Although many methods for downscaling of large-scale climate projections have emerged, very few of these methods have been implemented in complex regions such as humid tropical mountain areas (Buytaert et al. Citation2010). The RCMs operate at a typical resolution of 50 km or lower, and can capture the spatio-temporal variability of climate in much greater detail than GCMs. By providing more realistic simulations of present and future climate change compared to coarser resolution GCMs, the RCMs can help provide better understanding of the impact these changes will have on water resources. Regional climate models are based on the same model physics as the driving GCM and, therefore, do not require a dense observational network, as is needed for statistical downscaling. However, careful model validation with observational data is still a necessary pre-requisite to ensure that the RCMs accurately portray present-day spatio-temporal climate variability, in particular along mountain ranges, where orography strongly affects seasonal distributions of precipitation. Regional climate models have been used successfully for climate change studies over South America (Marengo et al. Citation2009, Soares and Marengo Citation2009), but their application is still in its early stages (Urrutia and Vuille Citation2009). A major drawback of RCMs is that they are computationally expensive and rather complex to implement.
The Intergovernmental Panel on Climate Change (IPCC) published a set of emissions scenarios in the Special Report on Emissions Scenarios (SRES) (Nakicenovic et al. Citation2000) to serve as the basis for assessment of future climate change. Among all the SRES scenarios, four marker scenarios (A1, A2, B1 and B2) are by far the most frequently used (Van Vuuren and O'Neill Citation2006). The A1 and B1 scenarios emphasize ongoing globalization and project a homogeneous world, while the A2 and B2 scenarios lay emphasis on social, economic and environmental development on both a regional and a local basis, and project a heterogeneous world. In this study, the scenarios A2 and B2 were adopted for investigating the effect of climate change on watershed hydrology. The A2 scenario projects high population growth and slow economic and technological development, while the B2 scenario projects slower population growth, rapid economic development, and lays more emphasis on environmental protection. Emissions of greenhouse gases (GHGs) and other driving forces were quantified in the Fourth Assessment Report of the IPCC (IPCC Citation2007) for use in climate simulations using GCMs. PRECIS is an atmospheric and land surface model of limited area and high resolution developed at the Hadley Centre and is sponsored by the UK Department for Environment, Food and Rural Affairs (Defra), the UK Department for International Development (DFID) and the United Nations Development Programme (UNDP) (Jones et al. Citation2004). This RCM is a hydrostatic, primitive equation grid-point model containing 19 levels described by a hybrid vertical coordinate. The present version of PRECIS has a horizontal resolution of 50 km. It has the provision to include the sulphur cycle and can generate outputs for more than 150 variables.
In this study, PRECIS was run with a horizontal resolution of 50 km for the present climate (1981–2010) using different baseline lateral boundary conditions (LBCs) and for future scenarios (2071–2100) based on the SRES of the IPCC. PRECIS was forced at its lateral boundaries by the simulations of a high-resolution global model (HadAM3H) with a horizontal resolution of 150 km × 150 km. The HadAM3H is an atmosphere-only GCM which has been derived from the atmospheric component of HadCM3, the Hadley Centre's state-of-the-art coupled model which has a horizontal resolution of 3.75° longitude by 2.5° latitude. The Indian Institute of Tropical Meteorology (IITM), Pune, in collaboration with the Hadley Centre, has developed future scenarios for India (Rupa Kumar et al. Citation2006). Climate data for both A2 and B2 scenarios were collected and utilized in this study. The location of Kerala for PRECIS simulations and the Chaliyar River basin having a drainage area of 2530 km2 falling in one grid location of 50 km × 50 km are presented in .
Daily data on precipitation and temperature (maximum and minimum) obtained from this grid location were used in the SWAT model to assess the impact of climate change on basin hydrology. The PRECIS grid was superimposed on the basin to derive the mean of the inputs at the centre of the square grid. This data set was then provided at the weather station location in the SWAT model.
3 RESULTS AND DISCUSSION
3.1 Bias correction of PRECIS data
Several methods have been identified to correct the bias in RCM data. Leander and Buishand (Citation2007) applied a power law transform, which corrects for the coefficient of variation (CV) and the mean of the precipitation values. A problem with the use of RCMs for hydrological purposes is that the simulated precipitation differs systematically from the observed precipitation (Frei et al. Citation2003). Hay et al. (Citation2002) proposed the use of the gamma distribution to match the modelled daily precipitation with the observed daily precipitation. After bias correction, the corrected data can be used for streamflow simulations using a semi-distributed hydrological model. Hay et al. (Citation2002) observed that the corrected precipitation data did not contain the day-to-day variability which was present in the observed data set. The method developed by Leander and Buishand (Citation2007) for bias correction was used in this study to correct temperature and precipitation data. The SWAT model, however, needs other meteorological forcing data as well, such as solar radiation, wind speed and relative humidity. Unfortunately projected data were not available for these variables and so simulations were performed using SWAT simulated values. In this work, bias correction was performed at a temporal resolution of one day and at a grid size of 50 km × 50 km.
Leander and Buishand (Citation2007) reported that a relatively simple non-linear correction, adjusting both the biases in the mean and variability, leads to a better reproduction of observed extreme daily and multi-day precipitation amounts than the commonly used linear scaling correction. This method of bias correction does not correct for the fraction of wet and dry days and lag inverse autocorrelation. A power transformation was used in this study, which corrects the CV as well as the mean. The bias correction was determined for the downscaled PRECIS data for the period 1981–2005. After bias correction, the most important statistics (CV, mean and standard deviation) of the PRECIS data were compared with those of the observed values. Each PRECIS daily precipitation amount P is transformed to a corrected P* using the equation:
The effect of sampling variability is reduced by determining the parameters a and b for every five-day period of the year, including data for the years that were available (Leander and Buishand Citation2007). Determination of the parameter b was performed iteratively. It was determined such that the coefficient of variation of the projected daily precipitation matched that of the observed daily precipitation. Thus, the coefficient of variation is only a function of parameter b and is expressed as:
The parameter a is then determined such that the mean of the transformed daily values matches with the observed mean. The parameter a thus depends on b. The parameter b depends only on the coefficient of variation and is independent of the value of parameter a. The values for a and b for the A2 scenario were 0.1506 and 1.0344 and the corresponding values for the B2 scenario were 0.1679 and 1.1062.
For correcting the daily temperature, a different technique was used. The correction of temperature involves shifting and scaling to adjust the mean and variance (Leander and Buishand Citation2007). For the basin, the corrected daily temperature T* was obtained as:
where TU is the uncorrected daily temperature from PRECIS, TO is the observed daily average temperature, TM is the corresponding daily average temperature obtained from PRECIS and SD is the standard deviation. Again coefficient of variation and mean (AVG) were determined for each five-day block of the year separately, as in the case of bias correction of daily precipitation. and present the bias-corrected future scenario precipitation and temperature (2071–2100) and the present-day (1981–2005) corresponding daily values, respectively.
3.2 Calibration and validation of SWAT
The AVSWAT2000 model was calibrated using observed streamflow records for a 15-year period from January 1987 to December 2001. The parameters selected in this study for sensitivity analysis were based on the description of the study area and literature (Heuvelmans et al. Citation2004, Chu and Shirmohammadi Citation2004, Gosain et al. Citation2006). The model parameters were adjusted manually by trial and error based on certain statistical indicators and the characteristics of the study area. The statistical criteria used to evaluate the hydrological goodness-of-fit were the coefficient of determination (R 2) and the Nash-Sutcliffe efficiency (E NS) (Nash and Sutcliffe Citation1970). The coefficient of determination is an index of the degree of linear association between the observed and the simulated values. The Nash-Sutcliffe efficiency indicates how well the plot of observed vs simulated data is close to the 1:1 line. It is the most commonly used coefficient in SWAT calibrations (Gassman et al. Citation2007). The optimal value of the model efficiency is 1. To capture the uncertainty in the parameter values, two additional runs were performed: one with a combination of the extreme parameter value settings that would result in maximum streamflow from the basin and one with a combination that would result in minimum flow. presents the parameters in the order of sensitivity and whether these have been increased or increased from the default values in the SWAT database. After calibrating the model, validation of the model was performed using data for another 5-year period (2002–2006). The results of model calibration for streamflow at the Kuniyil gauging station are presented in (a) and (b). It can be seen that the model slightly underestimates flow in all the years. The Nash-Sutcliffe efficiency calculated for the calibration period was 0.667. The plot of simulated vs observed annual streamflow shows an R 2 value of 0.932. The availability of accurate meteorological and hydrological data along with good estimates of parameters determines the accuracy of model simulation results. Arnold et al. (Citation1998) cited spatial variability in precipitation data as one of the major limitations to large area hydrological modelling. An important limitation of this study is the limited spatial resolution of hydrological and meteorological data. (a) and (b) presents the results of model validation. The Nash-Sutcliffe efficiency for streamflow in the validation period was 0.719. The R 2 value for the validation phase was 0.996. Hence it can be stated that the calibrated model performed reasonably well.
Table 4 Overview of parameter changes for calibration of SWAT
3.3 Results for climate change scenarios
Streamflows in the climate change period (2071–2100) were projected with the help of the hydrological model SWAT using bias-corrected rainfall and temperature data for the A2 and B2 scenarios projected by the PRECIS model. In the A2 scenario, for the southwest monsoon period, an average increase in temperature by 2°C and decrease in rainfall by 11.50% resulted in an increase in potential evapotranspiration by 1.14% from the present day average values. For the same period, in the B2 scenario, an average increase in temperature by 1°C and decrease in rainfall by 8.79% resulted in an increase in potential evapotranspiration by 1.12%. The streamflow showed a slightly decreasing trend: 7.53% decrease in the A2 and 4.62% decrease in the B2 scenario. Similar trends were projected in the northeast monsoon period also. In the case of the A2 scenario, an average decrease in rainfall by 8.70% with an increase in temperature of 2°C caused an increase in potential evapotranspiration by 1.09% and a decrease of 4.31% in streamflow from the present day average values. In the B2 scenario, an increase in average temperature of 1°C with a decrease of 4.73% in rainfall caused an increase in 1.01% in evapotranspiration and a decrease of 3.01% in streamflow.
(a) and (b) presents the variations in monthly streamflow and rainfall, and temperature and ET in the A2 scenario from the present-day values, respectively. The corresponding variations in the B2 scenario from the present-day values are presented in (a) and (b), respectively. A very small decrease is observed for both summer and pre-monsoon period streamflow in the Chaliyar River basin due to high surface warming in the future, even though there is a slight increase in the rainfall. In the A2 scenario, precipitation showed a 1.60% increase from the present-day average value; the corresponding value in the B2 scenario was 1.40%. The average temperature increased by 3°C and 2°C from the present-day average values in the A2 and B2 scenarios, respectively. Evapotranspiration values showed an increase of 1.05% and 1.03% in the A2 and B2 scenarios, respectively. The results indicate that, although there is no drastic change in the monsoon streamflow, the occurrence of high flows will decrease significantly because of high surface warming and, therefore, there will be a decrease in monthly streamflow. It is worth mentioning that the projected streamflow presented is due to a single RCM using two scenarios and it is widely recognized that disagreements between different RCM outputs represent a significant source of uncertainty (Wilby and Harris Citation2006, Ghosh and Majumdar Citation2008). Therefore, over-reliance on a single RCM could lead to inappropriate planning and adaptation responses. Also, there are extremely large variations in precipitation over very short horizontal distances in the river basin. Moreover, there could be water transfers from outside the river basin, but these are not incorporated in the model. From a model quality perspective, it could be that the hydrological processes related to groundwater movement and storage are insufficiently represented and do not capture regional fluxes. This demands further research and a regional groundwater model could describe the water balance better.
Fig. 11 (a) Observed and projected monthly streamflow and rainfall—A2 scenario; and (b) observed and projected monthly average temperature and ET—A2 scenario; average of 25 years.
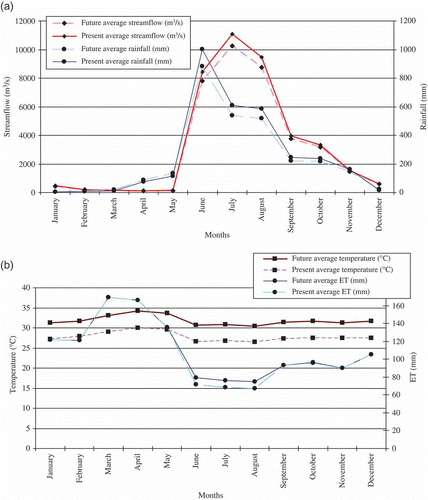
Fig. 12 (a) B2 scenario—observed and projected monthly streamflow and rainfall; and (b) observed and projected monthly average temperature and ET, average of 25 years.
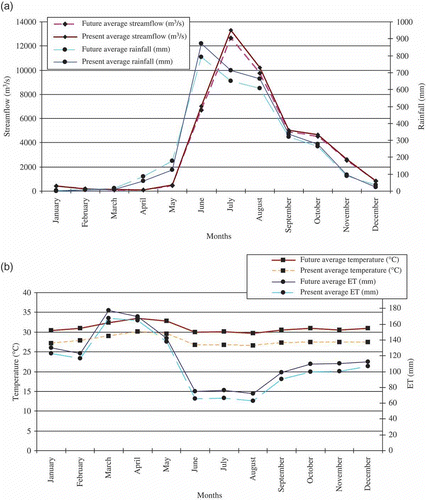
The land-use/land-cover was not changed in the SWAT simulations for future scenarios. In both the A2 and B2 scenarios, biomass production was found to increase to some extent as the CO2 levels increased. Even though temperature increase was found to cause water stress in the summer period, it was offset by the slight increase in precipitation during these months. Biomass production was found to be slightly greater in the A2 than the B2 scenario and this may be due to the higher levels of CO2 in the former case. Moreover ET in the A2 scenario was slightly lower than that in B2. The amount of water removed by transpiration from plants in the A2 scenario was less when compared to the B2 scenario. The Penman-Monteith method was used in SWAT simulations and it accounts for the effects of changing atmospheric CO2 in the transpiration computations (in the range of 330–660 ppmv). The impact of CO2 on leaf stomatal conductance was computed following the modification suggested by Easterling et al. (Citation1992). It assumes a 40% reduction in leaf conductance at an atmospheric CO2 concentration of 660 ppmv, as reported by Morison and Gifford (Citation1983). The impact of elevated CO2 levels on transpiration was then accounted for by simulating the effect of vapour pressure deficit on leaf stomatal conductance, based on the approach used by Stockle et al. (Citation1992). Crop yields show a non-uniform sensitivity to changes in temperature and precipitation, depending on the regional characteristics of climate change and crop species (Hattermann et al. Citation2007). The differences are the highest when comparing plants such as crops and trees having a different CO2 metabolism. Overall, plants have a higher potential biomass production under climate change; this is induced mainly by the increase in temperature and CO2, whereas trees are adapted to low water availability. The reductions in yield are only simulated when the decrease in precipitation is the strongest. While the rate of increase in crop yields appear to be declining generally (Wollenweber et al. Citation2005), projections of future trends in farming practices, plant breeding, and development of genetically modified organisms, are all uncertain.
The basin response to an annual average 3°C increase in temperature and 8% decrease in precipitation may be a function of factors other than ET. Net radiation effects, timing of precipitation during the year, and changes in the forest canopy may all play significant roles in controlling average streamflow (Graham et al. Citation1990). This evaluation has been based on analysis performed using a hydrological model in a potential climate change scenario. The analysis simply illustrates the trend and magnitude of streamflow changes in a drainage basin related to possible changes in temperature and precipitation. Additional analyses are needed that extend this work with refined climate change models, hydrological models that include more direct coupling between the land surface and the atmosphere, and evaluation of seasonal patterns in addition to average annual changes.
4 CONCLUSIONS
In this study, the impact of potential climate change on streamflow in the Chaliyar River basin at the Kuniyil gauging station was analysed for the projected climate change scenarios A2 and B2 outlined by the IPCC. The future climate variables were generated using an RCM, PRECIS and corrected for bias. These were translated to streamflow using the hydrological model, AVSWAT2000. PRECIS does indeed capture local gradients better than global models, but locally the model is prone to large discrepancies between observed and modelled precipitation. It is concluded that a further increase in resolution is necessary to represent local gradients properly. Furthermore, to assess the uncertainty in downscaling, an ensemble of regional climate models should be implemented. It is observed that even though streamflow exhibited a declining trend in these two scenarios, it is not so severe as to adversely affect agricultural production in the basin. Also, it is observed that in both scenarios crop yield increased from the present day observations due to an increase in CO2 concentrations. Even though temperature increase was observed, it was compensated by projected showers in the summer and pre-monsoon periods along with availability of nutrients. It may be noted that the land-use/land-cover and management practices were not changed in the SWAT simulations using data for climate change scenarios. However, there are uncertainties in the projected streamflow values because of uncertainties in both future emission scenarios and uncertainties in RCM projections (Maurer and Duffy Citation2005, Zierl and Bugmann Citation2005). Arnell et al. (Citation2001) suggested that future scenarios provide extremely valuable insights into the sensitivity of hydrological systems to changes in climate. Wolock and McCabe (Citation1999) further stated that sensitivity studies of temperature and precipitation variations can provide important insight into the responses and vulnerabilities of different hydrological systems to climate change, especially when there is a great deal of uncertainty in the available GCM projections. Hence the projected streamflows can only provide a rough indication of likely changes in streamflow patterns. However, the general results of this analysis can be identified and incorporated into water resources management plans for the future so as to promote more sustainable water use in the river basin.
Acknowledgements
The authors are grateful to the Department for Environment, Food and Rural Affairs (Defra), Government of UK, for sponsoring the joint Indo–UK programme on Climate Change, and the Ministry of Environment and Forests (MoEF), Government of India, for coordinating its implementation. Thanks are due to the Hadley Centre for Climate Prediction and Research, UK Met Office, for making available the regional model, PRECIS. Support for the PRECIS simulation data sets was provided by the Indian Institute of Tropical Meteorology, Pune. Thanks are also due to reviewers who helped in improving this research paper.
REFERENCES
- Alansi , A.W. 2009 . Validation of SWAT model for stream flow simulation and forecasting in Upper Bernam humid tropical river basin. Malaysia . Hydrology and Earth System Sciences Discussion , 6 : 7581 – 7609 .
- Allen , L.H. , Valle , R.R. , Jones , J.W. and Jones , P.H. 1998 . Soybean leaf water potential responses to carbon dioxide and drought . Agronomy Journal , 90 : 375 – 383 .
- Allen , R.G. , Gichuki , F.N. and Rosenzweig , C. 1991 . CO2 induced climatic changes and irrigation water requirements . Journal of Water Resources Planning and Management , 117 ( 2 ) : 157 – 178 .
- Arnell , N.W. 2001 . “ Hydrology and Water Resources ” . In Climate change 2001: Impacts, adaptation, and vulnerability , Edited by: McCarthy , J. J. 191 – 233 . Cambridge : Cambridge University Press . Chapter 4
- Arnell , N.W. 2003 . Socio-economic scenarios for climate change impacts assessments: characterising the SRES storylines . Global Environmental Change , 14 : 3 – 20 .
- Arnold , J.G. , Srinivasan , R. , Muttiah , R.S. and Williams , J.R. 1998 . Large area hydrologic modeling and assessment, Part I: model development . Journal of the American Water Resources Association , 34 ( 1 ) : 73 – 89 .
- Bondeau , A. 2007 . Modelling the role of agriculture for the 20th century global terrestrial carbon balance . Global Change Biology , 13 : 679 – 706 . doi: doi:10.1111/j.1365-2486.2006.01305.x
- Buytaert, W., et al., 2010. Uncertainties in climate change projections and regional downscaling: implications for water resources management. Hydrology and Earth System Sciences, 7, 1821–1848.. http://www.hydrol-earth-syst-sci-discuss.net/7/1821/2010 (http://www.hydrol-earth-syst-sci-discuss.net/7/1821/2010)
- Cao , W. , Bowden , B.W. and Davie , T. 2006 . Multi-variable and multi-site calibration and validation of SWAT in a large mountainous catchment with high spatial variability . Hydrological Processes , 20 : 1057 – 1073 .
- Challinor , A.J. , Wheeler , T.R. and Osborne , T.M. 2006 . “ Assessing the vulnerability of crop productivity to climate change thresholds using an integrated crop-climate model ” . In Avoiding dangerous climate change , Edited by: Schellnhuber , H.J. , Cramer , W. and Nakicenovic , N. Cambridge : Cambridge University Press .
- Christensen , J. , Boberg , F. , Christensen , O. and Lucas-Picher , P. 2008 . On the need for bias correction of regional climate change projections of temperature and precipitation . Geophysical Research Letters , 35 : L20709 doi: doi:10.1029/2008GL035694
- Chu , T.W. and Shirmohammadi , A. 2004 . Evaluation of the SWAT model's hydrology component in the piedmont physiographic region of Maryland . Transactions of the American Society of Agricultural Engineers , 47 ( 4 ) : 1057 – 1073 .
- Cibin , R. , Sudheer , K.P. and Chaubey , I. 2010 . Sensitivity and identifiability of stream flow generation parameters of the SWAT model . Hydrological Processes , doi: doi:10.1002/hyp.7568
- Cramer , W. , Bondeau , A. and Woodward , F.I. 2001 . Global response of terrestrial ecosystem structure and function to CO2 and climate change: results from six dynamic global vegetation models . Global Change Biology , 7 : 357 – 373 .
- de Wit , M. , van den Hurk , B. , Warmerdam , P. , Torfs , P. , Roulin , E. and van Deursen , W. 2007 . Impact of climate change on low-flows in the River Meuse . Climatic Change , 82 : 351 – 372 . doi: doi:10.1007/s10584-006-9195-2
- Easterling , W.E. 1992 . Preparing the Erosion Productivity Impact Calculator (EPIC) model to simulate crop response to climate change and the direct effects of CO2 . Agricultural and Forest Meteorology , 59 ( 1–2 ) : 17 – 34 .
- Frei , C. , Christensen , J.H. , Déqué , M. , Jacob , D. , Jones , R.G. and Vidale , P.L. 2003 . Daily precipitation statistics in regional climate models: evaluation and intercomparison for the European Alps . Journal of Geophysical Research , 108 ( D3 ) : 4124 doi: doi:10.1029/2002JD002287
- Gassman , P.W. , Reyes , M. , Green , C.H. and Arnold , J.G. 2007 . The Soil and Water Assessment Tool: Historical Development, Applications, and Future Directions. Iowa State University: Center for Agricultural and Rural Development . CARD Working Paper 07-WP 443 ,
- Ghosh , S. and Mujumdar , P.P. 2008 . Statistical downscaling of GCM simulations to streamflow using relevance vector machine . Advances in Water Resources , 31 : 132 – 146 .
- Gosain , A.K. , Rao , S. and Basuray , D. 2006 . Climate change impact assessment on hydrology of Indian river basins . Current Science , 90 ( 3 ) : 346 – 353 .
- Graham , R.L. , Turner , M.G and Dale , V.H. 1990 . How increasing CO, and climate change affect forests . BioScience , 40 ( 8 ) : 575 – 587 .
- Hargreaves , G.L. , Hargreaves , G.H. and Riley , J.P. 1985 . Agricultural benefits for Senegal River Basin . Journal of Irrigation and Drainage Engineering , 111 ( 2 ) : 113 – 124 .
- Hattermann , F.F. 2007 . Impacts of global change on water-related sectors and society in a trans-boundary central European river basin—Part 1: project framework and impacts on agriculture . Advances in Geosciences , 11 : 85 – 92 .
- Hay , L.E. 2002 . Use of regional climate model output for hydrologic simulations . Journal of Hydrometeorology , 3 : 571 – 590 .
- Heuvelmans , G. , Muys , B. and Feyen , J. 2004 . Evaluation of hydrological model parameter transferability for simulating the impact of land use on catchment hydrology . Physics and Chemistry of the Earth , 29 : 739 – 747 .
- Houghton , R.A. 2003 . Revised estimates of the annual net flux of carbon to the atmosphere from changes in land use and land management 1850–2000 . Tellus Ser. B, Chemical and Physical Meteorology , 55 : 378 – 390 .
- IPCC (Intergovernmental Panel on Climate Change), 2007. Climate change 2007: The physical science basis. Contribution of Working Group I to the Fourth Assessment Report of the Intergovernmental Panel on Climate Change. Cambridge: Cambridge University Press. http://ipcc-wg1.ucar.edu/wg1/wg1-report.html (http://ipcc-wg1.ucar.edu/wg1/wg1-report.html)
- Jacob , D. 2001 . A note to the simulation of the annual and inter-annual variability of the water budget over the Baltic Sea drainage basin . Meteorology and Atmospheric Physics , 77 : 61 – 73 .
- Jones , R.G. 2004 . Generating high resolution climate change scenarios using PRECIS , Exeter : Met Office Hadley Centre .
- Kamga , F.M. 2001 . Impact of greenhouse gas induced climate change on the runoff of the upper Benue River (Cameroon) . Journal of Hydrology , 252 : 145 – 156 .
- KAU (Kerala Agricultural University) . 2002 . Package of practice recommendations: crops , 12th , Edited by: Jose , A.I. Mannuthy, Trichur, Kerala, , India : Kerala Agricultural University, Directorate of Extension .
- Keshta , N. , Elshorbagy , A. and Carey , S. 2009 . A generic system dynamics model for simulating and evaluating the hydrological performance of reconstructed watersheds . Hydrology and Earth System Sciences , 13 : 865 – 881 .
- Kleinn , J. 2005 . Hydrologic simulations in the Rhine basin driven by a regional climate model . Journal of Geophysical Research , 110 : D04102 doi: doi:10.1029/2004JD005143
- Kundzewicz , Z.W. 2008 . The implications of projected climate change for freshwater resources and their management . Hydrological Sciences Journal , 53 ( 1 ) : 3 – 10 .
- Leander , R. and Buishand , T. 2007 . Resampling of regional climate model output for the simulation of extreme river flows . Journal of Hydrology , 332 : 487 – 496 . doi: doi:10.1016/j.jhydrol.2006.08.006
- Legesse , D. , Vallet-Coulomb , C. and Gasse , F. 2003 . Hydrological response of a catchment to climate and land use changes in tropical Africa: case study South Central Ethiopia . Journal of Hydrology , 275 : 67 – 85 .
- Linsley , R.K. 1960 . “ Computation of a synthetic streamflow record on a digital computer ” . In Surface water, Proceedings of the Helsinki Symposium, 1960 , Vol. 51 , 526 – 538 . Wallingford : IAHS Press, IAHS Publ .
- Marengo , J.A. , Jones , R. , Alves , L.M. and Valverde , M.C. 2009 . Future change of temperature and precipitation extremes in South America as derived from the PRECIS regional climate modeling system . International Journal of Climatology , 29 : 2241 – 2255 .
- Maroco , J.P. , Edwards , G.E. and Ku , M.S.B. 1999 . Photosynthetic acclimation of maize to growth under elevated levels of carbon dioxide . Planta , 210 : 115 – 125 .
- Maurer , E.P. and Duffy , P.B. 2005 . Uncertainty in projections of streamflow changes due to climate change in California . Geophysical Research Letters , 32 ( 3 ) : L03704 doi: doi:10.1029/2004GL021462
- McCabe , G.J. and Wolock , D.M. 1992 . Sensitivity of irrigation demand in a humid-temperate region to hypothetical climatic change . Water Resources Bulletin , 28 ( 3 ) : 535 – 543 .
- Messager , C. 2006 . Influence of observed and RCM-simulated precipitation on the water discharge over the Sirba basin, Burkina Faso, Niger . Climate Dynamics , 27 ( 2–3 ) : 199 – 214 .
- Middelkoop , H. 2001 . Impact of climate change on hydrological regimes and water resources management in the Rhine Basin . Climatic Change , 49 : 105 – 128 .
- Mileham , L.J. , Taylor , R.G. , Thompson , J. , Todd , M. and Tindimugaya , C. 2008 . Impact of rainfall distribution on the parameterisation of a soil-moisture balance model of groundwater recharge in equatorial Africa . Journal of Hydrology , 359 : 46 – 58 .
- Mileham , L. , Richard , G.T. , Todd , M. , Tindimugaya , C. and Thompson , J. 2009 . The impact of climate change on groundwater recharge and runoff in a humid, equatorial catchment: sensitivity of projections to rainfall intensity . Hydrological Sciences Journal , 54 ( 4 ) : 727 – 738 .
- Monteith , J.L. 1965 . “ Evaporation and the environment. The state and movement of water in living organisms ” . In 19th Symposium of the Society for Experimental Biology , 205 – 234 . Cambridge : Cambridge Univ. Press .
- Monteith , J.L. 1977 . Climate and the efficiency of crop production in Britain . Philosophical Transactions of the Royal Society, London , 281 : 277 – 329 .
- Morison , J.I.L. and Gifford , R.M. 1983 . Stomatal sensitivity to carbon dioxide and humidity . Plant Physiology , 71 : 789 – 796 .
- Nakicenovic , N. 2000 . IPCC special report on emissions scenarios , Cambridge : Cambridge University Press .
- Nash , J.E. and Sutcliffe , J.V. 1970 . River flow forecasting through conceptual models: Part I. A discussion of principles . Journal of Hydrology , 10 ( 3 ) : 282 – 290 .
- Neitsch, S.L., Arnold, J.G., Kiniry, J.R. and Williams, J.R., 2001. Soil and Water Assessment Tool theoretical documentation, version 2000. Temple, TX: Blackland Research Center, Texas Agricultural Experiment Station. http://www.brc.tamus.edu/swat/swat2000doc.html (http://www.brc.tamus.edu/swat/swat2000doc.html)
- Nemec , J. and Schaake , J.S. 1982 . Sensitivity of water systems to climate variation . Hydrological Sciences Journal , 27 : 327 – 343 .
- Priestley , C.H.B. and Taylor , R.J. 1972 . On the assessment of surface heat flux and evaporation using large-scale parameters . Monthly Weather Review , 100 : 81 – 92 .
- Raisanen , J. 2004 . European climate in the late twenty-first century: Regional simulations with two driving global models and two forcing scenarios . Climate Dynamics , 22 ( 1 ) : 13 – 31 .
- Reilly , J. , Tubiello , F. , McCarl , B. and Melillo , J. 2001 . “ Climate change and agriculture in the United States ” . In Climate change impacts on the United States: The potential consequences of climate variability and change , Edited by: The National Assessment Synthesis Team . Cambridge : Cambridge University Press .
- Ritchie , J.T. 1972 . A model for predicting evaporation from a row crop with incomplete cover . Water Resources Research , 8 : 1204 – 1213 .
- Rupa Kumar , K. 2006 . High-resolution climate change scenarios for India for the 21st century . Current Science , 90 ( 3 ) : 334 – 345 .
- Salathe , E.P. 2005 . Downscaling simulations of future global climate with application to hydrologic modeling . International Journal of Climatology , 25 ( 4 ) : 419 – 436 .
- Santhi , C. 2001 . Validation of the SWAT model on a large river basin with point and nonpoint sources . Journal of the American Water Resources Associatino , 37 ( 5 ) : 1169 – 1188 .
- Schuol , J. and Abbaspour , K.C. 2007 . Using monthly weather statistics to generate daily data in a SWAT model application to West Africa . Ecological Modeling , 201 : 301 – 311 .
- Shabalova , M. , van Deursen , W. and Buishand , T. 2003 . Assessing future discharge of the River Rhine using regional climate model integrations and a hydrological model . Climate Research , 23 : 233 – 246 .
- Soares , W.R. and Marengo , J.A. 2009 . Assesments of moisture fluxes east of the Andes in South America in a global warming scenario . International Journal of Climatology , 29 : 1395 – 1414 .
- Srinivasan , R. , Ramanarayanan , T.S. , Arnold , J.G. and Bednarz , S.T. 1998 . Large area hydrologic modeling and assessment part II: model application . Journal of the American Water Resources Association , 34 ( 1 ) : 91 – 101 .
- Stockle , C.O. , Williams , J.R. , Rosenberg , N.J. and Jones , C.A. 1992 . A method for estimating the direct and climatic effects of rising atmospheric carbon dioxide on growth and yield of crops: Part I—Modification of the EPIC model for climate change analysis . Agricultural Systems , 38 : 225 – 238 .
- Thomson , A.M. , Rosenberg , N.J. , Izaurralde , R.C. and Brown , R.A. 2005 . Climate change impacts for the conterminous USA: an integrated assessment: Part 2—Models and validation . Climatic Change , 69 : 27 – 41 .
- Urrutia , R. and Vuille , M. 2009 . Climate change projections for the tropical Andes using a regional climate model: temperature and precipitation simulations for the end of the 21st century . Journal of Geophysical Research , 114 : D02108 doi: doi:10.1029/2008JD011021
- Van den Hurk , B. 2005 . Soil control on runoff response to climate change in regional climate model simulations . Journal of Climate , 18 ( 17 ) : 3536 – 3551 .
- Van Vuuren , P.D. and O'Neill , B.C. 2006 . The consistency of IPCC's SRES scenarios to recent literature and recent projections . Climatic Change , 75 : 9 – 46 .
- Varis , O. , Kajander , T. and Lemmelä , R. 2004 . Climate and water: from climate models to water resources management and vice versa . Climatic Change , 66 ( 3 ) : 321 – 344 .
- Vörösmarty , C.J. , Douglas , E.M. , Green , P.A. and Revenga , C. 2005 . Geospatial indicators of emerging water stress: an application to Africa . Ambio , 34 : 230 – 236 .
- Wigley , T.M. and Jones , P.D. 1985 . Influences of precipitation changes and direct CO2 effects on streamflow . Nature , 314 : 149 – 152 .
- Wilby , R.L. and Harris , I. 2006 . A framework for assessing uncertainties in climate change impacts: low-flow scenarios for the River Thames, UK . Water Resources Research , 42 : W02419 doi: doi:10.1029/2005WR004065
- Wollenweber , B. , Lubberstedt , T. and Porter , J.R. 2005 . Need for multidisciplinary research towards a second green revolution . Current Opinion in Plant Biology , 8 : 337 – 341 .
- Wolock , D.M. and McCabe , G.J. 1999 . Estimates of runoff using water-balance and atmospheric general circulation models . Journal of the American Water Resources Association , 35 ( 6 ) : 1341 – 1350 .
- Zierl , B. and Bugmann , H. 2005 . Global change impacts on hydrological processes in Alpine catchments . Water Resources Research , 41 ( 2 ) : 396 – 408 .