Abstract
A physically-based hillslope hydrological model with shallow overland flow and rapid subsurface stormflow components was developed and calibrated using field experiments conducted on a preferential path nested hillslope in northeast India. Virtual experiments were carried out to perform sensitivity analysis of the model using the automated parameter estimation (PEST) algorithm. Different physical parameters of the model were varied to study the resulting effects on overland flow and subsurface stormflow responses from the theoretical hillslopes. It was observed that topographical shapes had significant effects on overland flow hydrographs. The slope profiles, surface storage, relief, rainfall intensity and infiltration rates primarily controlled the overland flow response of the hillslopes. Prompt subsurface stormflow responses were mainly dominated by lateral preferential flow, as soil matrix flow rates were very slow. Rainfall intensity and soil macropore structures were the most influential parameters on subsurface stormflow. The number of connected soil macropores was a more sensitive parameter than the size of macropores. In hillslopes with highly active vertical and lateral preferential pathways, saturation excess overland flow was not evident. However, saturation excess overland flow was generated if the lateral macropores were disconnected. Under such conditions, rainfall intensity, duration and preferential flow rate governed the process of saturation excess overland flow generation from hillslopes.
Editor D. Koutsoyiannis; Associate editor C. Perrin
Résumé
Un modèle hydrologique de versant, fondé physiquement, ayant des composantes de ruissellement de surface et d’écoulement souterrain rapide lors d’événements pluvieux, a été développé et calé en utilisant des expérimentations de terrain conduites sur des versants emboités présentant des chemins d’écoulement préférentiel, situés dans le Nord-Est de l’Inde. Nous avons mené des expériences virtuelles pour réaliser une analyse de sensibilité du modèle en utilisant l’algorithme automatisé d’estimation des paramètres (PEST). Nous avons fait varier différents paramètres physiques du modèle pour étudier les effets résultants sur les réponses du ruissellement de surface et de l’écoulement souterrain événementiel au niveau des versants théoriques. Nous avons pu observer que les formes topographiques ont des effets significatifs sur les hydrogrammes du ruissellement de surface. Les profils de pente, le stockage en surface, le relief, l’intensité de la pluie et les taux d’infiltration contrôlent en premier lieu la réponse de ruissellement de surface des versants. Les réponses rapides de l’écoulement souterrain événementiel sont principalement dominées par des écoulements préférentiels latéraux, les taux d’écoulement dans la matrice de sol étant très lents. Les paramètres les plus influents sur les écoulements souterrains événementiels sont l’intensité de la pluie et la structure des macropores du sol. Le nombre de macropores du sol connectés est un paramètre plus sensible que la taille des macropores. Sur les versants présentant des chemins préférentiels latéraux et verticaux très actifs, le ruissellement de surface par saturation n’est pas évident. Cependant, le ruissellement de surface par saturation est généré si les macropores latéraux sont déconnectés. Dans ces conditions, l’intensité de la pluie, sa durée et les taux d’écoulement préférentiel gouvernent le processus de génération du ruissellement de surface par excès de saturation sur les versants.
INTRODUCTION
Hillslope hydrological processes in tropics and subtropics
In humid tropical and subtropical forested hilly watersheds, the surface and subsurface runoff responses are primarily controlled by the climate and geophysical characteristics of hillslopes. Hillslope hydrological processes are known to be highly interdependent with complex interactions between them. Depending on the physical characteristics of watersheds and storm characteristics, some hydrological processes may be dominant. These are the critical hydrological processes, which principally control the rate and volume of runoff generated from the watersheds. Therefore, identification of the critical hydrological processes and their modelling is the key towards developing reasonably accurate rainfall–runoff models at the hillslope or watershed scale. In this context, physically-based hydrological models at the hillslope or the plot scale have been developed and used by many researchers under different conditions (Bronstert and Plate Citation1997, Faeh et al. Citation1997, Ticehurst et al. Citation2003, Adams et al. Citation2005, Weiler and McDonnell Citation2007, Sarkar et al. Citation2008, Sarkar and Dutta Citation2009, Citation2012).
In humid tropics and subtropics, highly active runoff generation processes are largely affected by the presence of natural pipes within the soil (Chappell Citation2010). Generally, in hilly tropical and subtropical regions the dominating hydrological processes are: water infiltration and percolation through soil macopores and matrix (Germann and Beven Citation1985, Beven and Clarke Citation1986, Gerke and van Genuchten Citation1993), shallow overland flow (Govindaraju et al. Citation1992, Ruan and Illangasekare Citation1998, Wang et al. Citation2002, Liu and Singh Citation2004, Gomi et al. Citation2008) and subsurface stormflow through soil (Beven Citation1981, Sloan and Moore Citation1984, Germann et al. Citation1986, Fan and Bras Citation1998, Kim et al. Citation2005, Uchida et al. Citation2005, Weiler and McDonnell Citation2007, Lin and Zhou Citation2008, Anderson et al. Citation2009). The severity and dominance of these processes depend on a number of factors, namely storm characteristics, soil physical properties, topography, vegetation, antecedent moisture conditions and other physical characteristics of watersheds.
Model sensitivity analysis and virtual experiments
The primary criteria for the satisfactory performance of any hydrological model are proper identification and reasonable mathematical representation of the physical processes. The model must also be calibrated and validated before its application. Often, due to the highly complex and random nature of hydrological processes, it is necessary to make some simplifying assumptions in the course of model development. The choice of assumptions again depends on the present state of knowledge and the acceptable degree of precision expected in model predictions. Once the modeller is satisfied with the calibration and validation of a model, it is necessary to have an idea of the sensitivity of the model input parameters. This information can help the modeller to calibrate the model for a new location. In the case of physically-based hydrological models, one of the secondary criteria is the measurement accuracy of the captured field data. A small error in the measurement of a sensitive parameter might lead to a significant error in the model predictions. Therefore, in complex hydrological models it is important that a sensitivity analysis is carried out to identify the most ‘sensitive’ model parameters. For improved process conceptualization in hillslope hydrology, virtual experiments can be a useful tool for model sensitivity analysis. Weiler and McDonnell (Citation2004) defined virtual experiments as a “numerical experiment with a model driven by collective field intelligence”. Virtual experiments are also referred to as physically-linked simulation studies, which are useful to investigate the effect of individual processes on a complex system (Coenders-Gerrits et al. Citation2013).
To perform sensitivity analysis either analytical methods or repeated random sampling techniques, like Monte Carlo analysis, can be used (Bootlink et al. Citation1998). However, in the case of physically-based complex hydrological models, virtual experiments are preferred over other methods of sensitivity analysis (Freeze Citation1972a, Citation1972b, Beven Citation1985, Burt and Butcher Citation1986, McCord and Stephens Citation1987, Wallach and Zaslavsky Citation1991, Jackson Citation1992, Ritsema et al. Citation1996, Hook Citation1998, Mishra et al. Citation2008). In this process, simulations are carried out keeping all model input parameters at their respective base values, except for the parameter being tested. The effect of changing the input parameter on model outputs is studied to determine how ‘sensitive’ the output is to that parameter (Ticehurst et al. Citation2003). Independent automated calibration algorithms, like parameter estimation (PEST), can be used to assist in the sensitivity analysis of models (Doherty Citation2001, Doherty and Johnston Citation2003).
Environmental challenges in northeast India
Northeast India is dominated by the vast vegetated hillslopes and floodplains of the Brahmaputra river system. The region falls under the humid subtropics with very high average annual rainfall (more than 1600 mm/year), which is mainly concentrated in the monsoon season (Singh et al. Citation2004). In general, the hillslopes have shallow soils with highly active vertical and lateral preferential flow networks. Frequent spells of rainy days during the monsoon keep the topsoil nearly saturated for most of the season. Therefore, high-intensity rainfall events usually occur under wet antecedent soil conditions to generate a shallow depth of overland flow and a very high rate of subsurface stormflow from the hillslopes. These climate and hydrogeological regimes are primarily responsible for hydrological extremes in the region, such as flash floods, landslides and subsurface erosion. To gain insight into these highly interdependent and complex regional hillslope processes, a physically-based, plot-scale hillslope hydrological model was developed. The model has been used to conceptualize overland flow and subsurface stormflow responses of the hillslopes under wet antecedent soil conditions (Sarkar et al. Citation2008, Sarkar and Dutta Citation2012). The basic input parameters of the model, namely infiltration rate, topographical features, saturated hydraulic conductivity, drainable porosity and soil macropore structures, are to be physically derived from field and laboratory experiments. Therefore, the model can also be calibrated and used in other humid tropical or subtropical regions having similar climate and hydrogeological conditions. However, in the process of calibration, the model parameters should be optimized interactively using an inverse modelling approach. In practice, it is essential to have an idea of the sensitivity of input parameters so that the model can be calibrated easily. Therefore, in the present investigation, virtual experiments have been used to study the sensitivity of model parameters with respect to rainfall intensity, topographical shapes, relief, number of connected soil macropores, and macropore size on surface and subsurface runoff generation processes. The virtual experiments also provided a basis for evaluating the performance of the model under a wide range of physical conditions, which are difficult to replicate under actual field conditions.
Contrasting the proposed model with some existing models
Over the last couple of decades a significant number of studies have been carried out on different aspects of hillslope hydrology, such as overland flow, streamflow, vertical and lateral preferential flow, non-equilibrium flow and dual-porosity flow. Despite this research attention, the underlying processes of surface and subsurface runoff generation in many hillslopes are not well understood. It is often difficult to quantify lateral subsurface flow through soil macropores due to a lack of information about the architecture of subsurface flow paths. Failure to understand the reasons for discrepancies between observed data and model predictions is also a cause of great concern (Beven and Germann Citation2013). To address this paradox, it is important that the selected model should reasonably represent the dominant hydrological processes of the region. This has encouraged the development of a number of physically-based hydrological models around the world to address local or regional hydrological issues.
TOPMODEL is one of the oldest and most popular topography-based distributed hydrological models (Beven Citation1997). The model uses a topographic index to represent catchment dynamics. TOPMODEL is described as a set of conceptual tools to simulate surface and subsurface hydrological processes. Over the years, TOPMODEL has been modified to include kinematic wave routing of subsurface flow (Beven and Freer Citation2001). Using a generalized topographic index, the model has been used to predict shallow subsurface stormflow response from forested headwater catchments (Scanlon et al. Citation2000). However, the model does not directly represent the processes of vertical and lateral preferential flow through soil. HYDROTEL is another physically-based, spatially-distributed hydrological model, which can handle remote sensing and geographic information system data (Fortin et al. Citation2001). The model is suitable for real-time forecasting of streamflow, quantification of hydrological effects due to physical changes and simulation of the spatial distribution of soil moisture, evapotranspiration, subsurface runoff and vertical water budget. The model does not incorporate the physical processes of soil macropore flow and the minimum possible time step (1 h) is too large to represent fast preferential flow processes. A two-dimensional (2-D), physically-distributed hillslope hydrological model (HILLS) was presented to quantify infiltration, overland flow, saturated and unsaturated subsurface flow, evapotranspiration and vertical drainage (Smith and Hebbert Citation1983). The model uses rainfall intensity and duration, permeability of soil layers, soil water storage capacity and topographic data as input parameters. However, the model only considers saturated water flow through the soil matrix (without macropore flow) to compute lateral subsurface flow. A physically-based numerical hillslope model (QSOIL) was proposed based on the double porosity concept to describe infiltration and runoff processes (Weiler et al. Citation1998). The model has modules for overland flow, macropore flow, matrix flow, interaction and pipe flow. Some important physical parameters of the model are: surface roughness coefficient, soil macroporosity parameters, flow velocity through macropores and soil matrix parameters. The model can simulate a one-dimensional (1-D) infiltration process and 2-D infiltration and runoff processes. A 1-D model MACRO was developed to describe unsteady flow of water and solutes in soil (Jarvis and Larsbo Citation2003). It is a dual-porosity model, which considers interaction between the soil matrix and macropore domains. The main input parameters required by the model are hydraulic conductivity, rainfall, soil data, solute concentration and degree of saturation of soil. The model can simulate complete water balance, root water uptake, seepage, drainage and solute transport. However, it does not include the process of lateral preferential flow through soil macropores. Tsutsumi et al. (Citation2005) developed a 3-D steady-state hillslope lateral flow model using both soil matrix flow and pipe flow. Though the model accounted for different types of pipeflow, both groundwater levels and preferential flow were simulated under steady-state conditions. Weiler and McDonnell (Citation2007) presented a conceptual hillslope hydrological model to show that lateral preferential flow can be parameterized within a process-based model framework. Wienhöfer and Zehe (Citation2012) proposed a hillslope-scale, rapid lateral preferential flow model. The water and solute transport processes were modelled by representing field observed soil macropore characteristics explicitly in the 2-D model CATFLOW. Davies and Beven (Citation2012) presented a Multiple Interactive Pathways (MIPs) model to represent the effects of soil heterogeneities and preferential flow pathways. The model predictions compared well with kinematic wave representation of flow processes on shallow hillslopes. Dusek et al. (Citation2012) used a dual-continuum approach to model hillslope-scale shallow subsurface runoff from headwater catchments without any overland flow. The effect of preferential flow was represented in the model by combining a 1-D vertical dual-continuum model with a 1-D lateral flow equation. Buttle and McDonald (Citation2002) also explored the coupling of vertical and lateral preferential flows through field observations of runoff.
From the above discussions, it is clear that there are several hillslope hydrological models available with certain capabilities and limitations. The proposed physically-based hillslope hydrological model has the following features which distinguish it from other models:
The model is specifically suitable for dealing with flashy hillslope hydrological systems driven by highly interconnected vertical and lateral preferential flow networks in nearly-saturated shallow soils under very high intensity rainfall events.
Subsurface stormflow can be obtained at the bottom of the slope in terms of saturated soil matrix flow and lateral preferential flow hydrographs.
The physical aspects of the model have been developed with respect to the climate, soil and dominant hillslope hydrological processes of northeast India and thus it should be useful in dealing with the extreme hydrological events of the region.
MODEL DEVELOPMENT, CALIBRATION AND PERFORMANCE EVALUATION
A physically-based, plot-scale hillslope hydrological model with shallow overland flow (Sarkar et al. Citation2008) and subsurface stormflow (Sarkar and Dutta Citation2012) components under wet antecedent soil conditions was developed (). The model was calibrated based on physical runoff experiments conducted on a wet, vegetated, preferential flow path nested hillslope plot in northeast India. A unique feature of the model is that most of its input parameters can be measured physically from simple field and laboratory experiments. The model could capture the extreme hydrological conditions of the study area for natural storm events as well as for plot-scale runoff simulation experiments conducted with a sheet flow generation system (Sarkar et al. Citation2008, Sarkar Citation2011, Sarkar and Dutta Citation2012).
Physical runoff experiments
An undisturbed vegetated hillslope plot, 18 × 6 m in size, was selected in the Brahmaputra River basin (26°12′N latitude and 91°42′E longitude) of India for conducting in situ runoff simulation experiments. The land cover of the plot was dominated by close growing grasses and shrubs. Depending on climatic conditions, the degree of vegetation in the plot was observed to vary seasonally. Laboratory dye tracer infiltration tests conducted with soil samples collected from the hillslope plot showed the existence of a highly active preferential flow network in the hillslope soil (Shougrakpam et al. Citation2010). Macropores of 1–2 and 3–4 mm in size, were found to be dominant (Sarkar Citation2011).
A sheet flow generation system was used to conduct runoff simulation experiments on the hillslope plot (Sarkar et al. Citation2008, Sarkar and Dutta Citation2012). A total of 49 runoff experiments were conducted in different seasons with varying rainfall intensities (59–406 mm/h) under three vegetation conditions: sparse, moderate and dense. Quantitative parameters such as plant height, stem density, percent ground cover and average root depth were used to classify the vegetation condition of the hillslope plot (Sarkar et al. Citation2008). For sparse vegetation conditions, the stem density, plant height and ground cover were <800 stems per m2, 15 cm and 60%, respectively. However, under dense vegetation conditions, the respective values were >2000 stems per m2, 30 cm and 80%. Intermediate values of these parameters indicated moderate vegetation conditions. The average rooting depth for sparse, moderate and dense vegetation conditions was taken as <35 cm, 35–60 cm and >60 cm, respectively. Before each experiment a wet antecedent condition was established in the plot. The hillslope plot was instrumented with a profile-probe moisture meter to monitor soil moisture conditions at four points located centrally along the length of the plot. A total of 25 piezometers were installed along five transects across the plot to monitor the build-up and recession of the perched water table over the impermeable layer, which was present at 1 m depth b.g.l. (Sarkar and Dutta Citation2012). Volumetric measurements were taken at the downstream end of the slope to measure the outflow hydrograph generated from the hillslope (Sarkar et al. Citation2008).
The observed overland flow rates from the hillslope plot () showed an initial time lag and then, due to wet antecedent conditions, within a short time the outflow became almost constant representing near-steady infiltration conditions. The minor fluctuations in outflow rate were mainly due to observational errors, which usually occur in field experiments conducted on a relatively large plot area (Liu and Singh Citation2004, Sarkar et al. Citation2008). However, the fluctuations that could be observed about the mean outflow rate were negligible (). For the runoff simulation experiment shown in , after some initial time lag the outflow rates showed a coefficient of variation of 6.6%. Thus, neglecting minor fluctuations in outflow rates, after the lag period the difference between mean outflow rate and constant inflow rate approximately represented the spatially-averaged steady infiltration rate over the hillslope plot (Sarkar et al. Citation2008, Sarkar Citation2011). The steady infiltration rate (fb) was found to be closely related to the inflow intensity (I) of the runoff and the degree of vegetation. The linear relationships between I (mm/h) and fb (mm/h) in equations (1)–(3) were derived from in situ plot-scale runoff simulation experiments conducted under different vegetation conditions using a sheet flow generation system (Sarkar et al. Citation2008). The relationships were established from, respectively, 10, 11 and 13 experiments conducted under sparse, moderate and dense vegetation conditions of the hillslope plot.
Fig. 2 Observed overland flow rates for a plot-scale runoff simulation experiment conducted with constant inflow intensity of 97 mm/h and 51 min duration under dense vegetation condition.
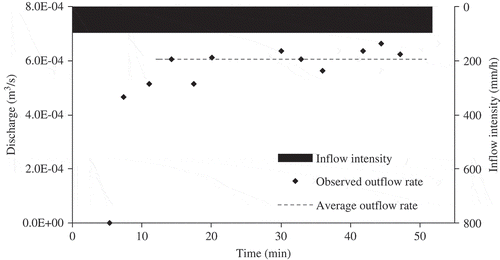
Overland flow component
The overland flow component of the model solves 1-D shallow overland flow equations with diffusive wave approximation using a finite volume technique (Jain et al. Citation2005). Neglecting the inertia term, 1-D shallow overland flow equation was written as:
Subsurface stormflow component
The subsurface stormflow component of the model integrated rapid lateral preferential flow and saturated soil matrix flow (Sarkar and Dutta Citation2012). Soil moisture storage capacity function, Sc(x), for a uniform hillslope facet was defined as (Fan and Bras Citation1998):
The total lateral flow per unit length of the actively flowing macropores at a given time was computed as (Sarkar and Dutta Citation2012):
Equation (11) was solved numerically using a finite difference method considering the Warming-Beam upwind scheme (Niyogi et al. Citation2005). The model was used to compute subsurface storage at time steps of 0.05 h in each grid cell and subsurface lateral flow response at the bottom of the hillslope as saturated matrix flow and lateral preferential flow. At any given time step, the storage within the hillslope was used to calculate the saturated depth over the impermeable layer. The basic input data for the subsurface stormflow component of the model are: rainfall intensity, physical dimension and topographic data of the plot, d, ks, ε, average rate of recharge (favg) to the saturated layer and soil macropore structure. The soil macropore structure depends on flow network, pore size, connectivity and spatial distribution of pipes within the soil profile (Weiler and McDonnell Citation2007, Chappell Citation2010, Krzeminska et al. 2012). In the present model, soil macropore structures were defined by the number, diameter and depth-wise distribution of connected pipes in different soil layers.
Illustrations of model performance
The model predictions compared well with the observed surface and subsurface flow response of the experimental hillslope plot (Sarkar et al. Citation2008, Sarkar and Dutta Citation2012). shows observed and simulated overland flow hydrographs for one of the experiments conducted with an inflow intensity of 241 mm/h and a duration of 30 min. shows observed and predicted runoff coefficients for some experiments conducted under sparse, moderate and dense vegetation conditions of the hillslope plot (Sarkar Citation2011). The observed and simulated runoff coefficients compared well for most of the experiments. Runoff coefficients and steady outflow rates were significantly lower under dense vegetation conditions, compared with sparse and moderate vegetation conditions, This could be attributed to the enhanced preferential flow rate through well-connected soil macropores, developed mainly by densely populated plant roots (Sarkar et al. Citation2008, Shougrakpam et al. Citation2010).
Fig. 3 Observed and simulated outflow rates for a plot-scale runoff experiment conducted with constant inflow intensity of 241 mm/h and 30 min duration under moderate vegetation condition.
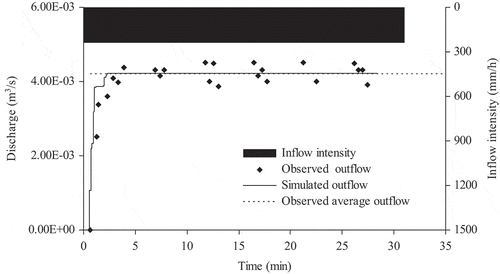
Table 1 Observed and simulated overland flow characteristics for some of the runoff experiments conducted on the experimental hillslope plot.
To evaluate the subsurface stormflow component of the model, average saturated depths, at five points along the centroid of the plot, were estimated by averaging observed piezometer readings at the five transects. These average depths at different time steps were compared with the simulated water table depths (Sarkar and Dutta Citation2012). In order to calibrate the model for the experimental hillslope plot, simulations were run with different arbitrary soil macropore structures, defined by assuming various combinations of m, N and ravg. For different rates of recharge the number of hydraulically effective macropores in each soil layer was varied to match the observed and simulated temporal variations of water table profile in the experimental plot (Sarkar and Dutta Citation2012). To ensure a good match between observed and predicted saturated profiles, a statistical parameter referred to as the performance index (PI) was used (Lin and Cunningham III 1995):
Table 2 Observed and predicted water table depths above the impermeable layer for an experiment conducted with an average steady recharge rate of 139 mm/h for 48 min duration under dense vegetation conditions. PI: performance index.
THE THEORETICAL HILLSLOPE PLOTS
To conduct a parametric study of the hillslope hydrological model, different theoretical hillslope plots were considered. The length of the theoretical hillslope plot was taken as 100 m, with a uniform width of 22 m. The relief between the upslope and downslope points was taken as 20 m. A uniform soil depth of 1 m was taken by assuming that the surface profile curvature of the terrain was parallel to the bedrock profile. It is usual practice to have such an assumption in case the soil depth function is unknown (Troch et al. Citation2002). It was further assumed that the hydrological behaviour of the theoretical hillslope was identical to that of the experimental hillslope plot used for the calibration of the hillslope hydrological model (Sarkar et al. Citation2008, Sarkar and Dutta Citation2012). Therefore, the physical relationships derived from in situ experiments conducted on the hillslope plot were also considered to be valid for the theoretical hillslopes.
Topographical variations of hillslopes
The geometric surface of a hillslope can be approximately defined by a continuous function. The curvature in the gradient direction is known as profile curvature and in the width direction as plan curvature. The profile curvature defines the change in slope angle and thereby controls the change of velocity of water flowing down the slope curve, whereas the plan curvature reflects the change in aspect angle to influence the divergence or convergence of water flow. The following form of bivariate quadratic function was used for defining plan and profile curvatures of the theoretical hillslopes (Troch et al. Citation2002, Rezzoug et al. 2005):
METHOD OF SENSITIVITY ANALYSIS
To perform sensitivity analysis, the independent automated calibration algorithm PEST (Doherty Citation2001, Doherty and Johnston Citation2003) was used; PEST uses the Gauss-Marquardt-Levenberg method for parameter estimation and provides useful information about parameter sensitivity. The PEST algorithm can be used efficiently in calibration and sensitivity analysis of hydrological models (Doherty and Skahill Citation2006, Bahremand and De Smedt Citation2008). The PEST companion program SENSAN was used to study the sensitivity of the model parameters following a one-at-a-time approach. The sensitivity of the parameters with respect to rainfall intensity (i), relief (H), hillslope topography/bedrock profile curvature parameter (α), radius of macropores (ravg) and number of hydraulically effective macropores in a soil layer per unit width of slope (N) was tested on overland flow and subsurface stormflow responses. The base values of the model parameters were taken as follows: i = 150 mm/h, H = 20 m, α = 1, ravg = 1.5 mm, and N = 14. The sensitivity of these parameters on peak overland flow rate (Qp), time to peak (tp), time of recession (tr), peak matrix flow rate (Qp(mat)), peak macropore flow rate (Qp(mac)), and maximum saturated depth over the impermeable layer (hs) was quantified by estimating the normalized sensitivity coefficient (NSC). If Φb is the model output corresponding to the parameter base value Ψb, and Φ and Ψ are the model output and parameter values, respectively, pertaining to a particular model run, then NSC can be calculated as follows:
RESULTS AND DISCUSSION
Overland flow response from hillslopes
The overland flow response from the theoretical hillslopes was simulated with different rainfall intensities in the range 100–300 mm/h. The effects of topographical shape were clearly evident on the overland flow hydrographs generated at the bottom of the hillslopes. It was considered that the theoretical hillslopes had dense vegetation cover and a highly macroporous soil, similar to the experimental hillslope plot. It was also assumed that the rainfall intensity (i) was equivalent to the inflow intensity (I) of runoff as both represent water input to the hillslope plot. Thus, for different rainfall intensities, the values of average fb were computed using the functional relationship (equation (3)) derived from the plot-scale runoff simulation experiments performed in the field (Sarkar et al. Citation2008). The rainfall intensity in excess of fb generated overland flow from the hillslope and the infiltration excess flow was routed to the outlet of the slope with an average Manning’s roughness coefficient (n) value of 0.04. The infiltrated water was assumed to percolate rapidly through the vertical macropores of soil to recharge the temporary saturated zone (i.e. fb ≈ favg) formed over the impermeable layer at 1 m depth below ground level (b.g.l.). In all the simulations the duration of rainfall was taken as 30 min.
shows some of the simulated overland flow hydrographs for Type 1, Type 2 and Type 3 hillslopes. All three hillslopes showed similar shapes of hydrographs: discharge at the slope outlet gradually increased from zero as contributions from upslope points started reaching the outlet and, subsequently, reached a maximum value and became constant as long as the rainfall continued. This was due to the assumed steady rainfall and constant recharge condition of the plot. After cessation of the rainfall, discharge gradually reduced with the reduction of storage within the hillslopes. For all hillslopes, with an increase in rainfall intensity, Qp increased and tp decreased.
Fig. 5 (a)–(c) Overland flow hydrographs from different hillslopes under varying rainfall intensities.
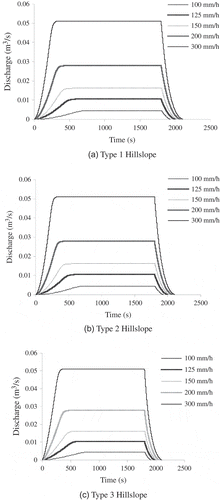
Overland flow hydrographs were also simulated by varying the relief of the hillslopes in the range 5–40 m. For example, keeping other conditions constant, the relief of the three types of hillslopes was changed from 20 to 10 m and the resulting DEMs were generated. Then, considering a rainfall intensity of 150 mm/h, overland flow hydrographs could be simulated. Comparison between these runoff hydrographs showed that, in all three types of hillslope, similar steady peak outflow conditions were attained ().
Table 3 Effect of change in relief on overland flow hydrographs for i = 150 mm/h.
The sensitivity of the model input parameters i, H and α on the simulated overland flow hydrograph components, namely, Qp, tp and tr is enumerated in , in terms of the respective NSC values.
Table 4 Estimated normalized sensitivity coefficients (NSC) for the tested parameters of the model.
Discussion of overland flow results
A comparison of the hydrograph ordinates, generated from the three hillslopes (), clearly shows the effects of profile curvature on overland flow. The peak rate of discharge for a given rainfall intensity remained constant for all three hillslopes. This was expected as a steady rainfall and recharge condition over an extended duration was simulated over the plot. However, different profile curvatures showed significant effects on tp and tr. The lowest tp values were obtained for the Type 2 hillslope, as the flow length was minimum for a straight slope profile. Comparatively higher values of tp were evident from the Type 1 hillslope, as the flow length of a concave slope profile is more than that of a straight profile. The Type 3 hillslope had maximum slope length and thus showed maximum values of tp. Similarly, the recession time was also controlled by the hillslope profile curvatures. As flow recession started after the cessation of rainfall, the storage of water at that particular instant primarily controlled the value of tr. Clearly, the Type 3 hillslope had the least surface storage due to its convex profile and the Type 1 hillslope had maximum storage capacity owing to its concave profile. Therefore, tr values were least for the Type 3 hillslope and maximum for the Type 1 hillslope. The Type 2 hillslope exhibited intermediate values of tr due to its moderate surface storage property. It is possible that the length of flow also plays a role in the recession time of hillslopes. However, the control of hillslope storage on recession time was found to be more dominant than the effect of flow length.
The effect of changing relief was also reflected in tp and tr values. As hillslope relief was reduced from 20 to 10 m, the values of tp and tr were increased due to flattening of the slope (). However, for different hillslope profiles, both tp and tr followed similar trends to those discussed in the preceding paragraph.
From the values of NSC given in , it is apparent that i was the most sensitive parameter for Qp, and the parameters H and α did not have any effect on the peak rate of overland flow. Time to peak was sensitive to i and to H, whereas tr was largely controlled by the profile curvature parameter. The measures of parameter sensitivities were found to be in agreement with the simulated trends of overland flow characteristics discussed in the previous paragraphs.
Subsurface stormflow response from hillslopes
The subsurface stormflow responses of hillslopes with different physical conditions of the plot were simulated under varying intensities of rainfall. The average rate of recharge to the saturated layer was obtained from the same functional relationship (equation (3)) used in overland flow simulations assuming favg ≈ fb. The values of N for different rainfall intensities were computed using equation (16) assuming that the theoretical hillslope soil had the same order of macroporosity as that of the experimental plot.
It was observed that, with increasing rainfall intensities, both soil macropore and matrix flow rates increased ( and ). In both the cases the peak flow occurred after the cessation of recharge, as at this point the saturated depth above the impermeable layer was the maximum. However, the magnitude of macropore flow was much higher than that of matrix flow. The simulations also showed that the bedrock profile curvature did not have a significant effect on macropore flow rate. Macropore flow strongly depended on the number of active connected macropores in the soil (Sarkar and Dutta Citation2009, Citation2012). Therefore, minor variations in saturated depth owing to different curvatures of bedrock profile were insignificant. However, matrix flow rates were affected by different profile curvatures due to variations in the hydraulic gradient ().
The bedrock topography of the theoretical hillslopes was approximated by the surface topography. Therefore, the effect of bedrock profile curvature on subsurface stormflow was investigated by varying the relief of the hillslopes along the bedrock profile. In the present modelling concept, as macropore flow was incorporated as a sink term, it was not affected much by variations in relief. However, peak matrix flow rates were found to decrease with the reduction of relief (). Ticehurst et al. (Citation2003) also reported similar results from the simulations of the HILLS model.
Table 5 Effect of change in relief on matrix flow hydrographs for i = 150 mm/h.
The effects of change in active macropore size and number were also evaluated. First, keeping the values of N the same, the size of macropores was doubled. It can be observed from that doubling macropore size did not change hs significantly. Consequently, the increase in macropore flow rate was small. However, keeping macropore size the same, if the values of N were increased, hs changed considerably resulting in higher macropore flow.
Table 6 Effect of change in soil macroporosity on subsurface stormflow for the Type 2 hillslope.
The estimated NSC values () provided a clear idea of the sensitivity of tested parameters on subsurface stormflow characteristics. Bedrock relief and profile curvatures were identified as very sensitive parameters for saturated matrix flow through soil. Compared to ravg, N was a more sensitive parameter to control the lateral preferential flow from the hillslopes. In general, rainfall intensity was a sensitive parameter for the subsurface stormflow characteristics of the hillslopes. The maximum saturation build-up over the impermeable layer was primarily controlled by rainfall intensity and soil macroporosity.
Discussion of subsurface stormflow results
From the model simulations it could be observed that peak discharge through the soil macropores was quite comparable to the peak rate of overland flow. Anderson et al. (Citation2010) also observed similar trends and reported that lateral preferential flow had first-order control on subsurface flow. Hopp et al. (Citation2011) found that in humid temperate regions, approximately two-thirds of precipitation occurred as rapid lateral subsurface flow.
enumerates variations in the formation of a saturated profile above the impermeable layer. Saturation excess overland flow occurs when the saturated depth above the impermeable layer exceeds the soil depth. In all cases it could be observed that the depths of saturated profile were less than the soil depth. Even under the simulated extreme rainfall conditions, saturation excess overland flow was not evident. Therefore, in hillslopes where high macroporosity results in quick vertical as well as lateral transmission of infiltrated water, the process of saturation excess overland flow might not occur in spite of having saturated soil conditions and high rainfall intensity. However, it is also worth mentioning that saturation excess overland flow was evident for simulations with no lateral macroporosity (i.e. N = 0) and prolonged recharge with a high vertical preferential infiltration rate (). Thus, if the lateral macroporosity of a hillslope is disturbed, but the vertical macropores are still active to rapidly convey the infiltrated water towards the impermeable layer, saturation excess overland flow might occur. It is also worth mentioning here that in natural undisturbed forested hillslopes, the case of discontinuity of lateral macropores might not be relevant. But, there are specific instances of discontinuity of lateral macropores under shifting cultivation systems. In northeast India shifting cultivation is popularly practiced in forested hillslopes. Usually, the steep upslope forest areas are kept undisturbed and shifting cultivation is performed in relatively flatter downslope locations following such steps as: clearing the forest, burning the litter, land preparation, cultivation of crops, and abandonment of land. Once the land is abandoned, natural forest vegetation starts to grow back. However, shifting cultivation has long-lasting effects on soil structure and is known to significantly disturb the natural continuity of lateral macropores (Shougrakpam et al. Citation2010). Under such conditions, the downslope locations are unable to transmit high lateral subsurface flow coming from the upslope forested hillslopes through a well-connected network of macropores. Consequently, saturation excess overland flow occurs. The assumption of discontinued lateral macropores was made to simulate these conditions reported in literature (Shougrakpam et al. Citation2010).
Table 7 Maximum height of water table above the impermeable bed under different rainfall intensities.
Table 8 Occurrence of saturation excess overland flow with no lateral macropores for the Type 2 hillslope.
Subsurface simulations and sensitivity analysis results also indicated that when compared to N, macropore size was not a very sensitive parameter of the model. In the process of characterization of soil macroporosity for the experimental hillslope plot, an average macropore diameter of 1.5 mm was selected from the dominating macropore size groups 1–2 mm and 3–4 mm. Here, the selection of sizes 2 mm or 3 mm for macropore diameter, would not have changed the macropore flow rate significantly (Sarkar Citation2011). But, the selection of N was much more critical as it had a pronounced effect on macropore flow. Under different soil and hydrogeological conditions the value of N can change significantly owing to different land use and land cover, management practices, and varying rainfall intensities (Sharma et al. Citation2013). Therefore, experimental data should be analysed carefully in order to define the lateral macroporosity of soil under different physical and hydrogeological hillslope conditions.
Significance and limitations of the modelling concept
The hillslope hydrological model discussed in this investigation was developed based on a physical understanding of some of the key hydrological processes in northeast India. The modelling approach conceptualized surface and subsurface runoff generation processes from wet vegetated hillslopes of the region under high intensity rainfall events. The model was able to describe the shallow overland flow and rapid subsurface stormflow generated from the experimental hillslope plot reasonably well. The role of rapid subsurface stormflow as the root cause behind the typical hydrological extremes prevailing in the region could also be elucidated in a comprehensive manner. The results of the virtual experiments, conducted under different theoretical conditions of hillslopes, were useful in identifying the sensitive parameters of the model. The strength of the model lies in the fact that most of its input parameters can be measured from simple field and laboratory experiments. At a different location with similar hydrological regimes, the functional relationships describing input parameters of the model might vary. Once these relationships are experimentally derived, the effects of spatial variability in the model input parameters can be handled. Thus, the model can be easily calibrated and used in other humid tropical or subtropical hillslopes having a similar physical and hydrological scenario.
However, there are some specific limitations regarding the applicability of the model. Considering the regional climate and hydrogeological conditions of northeast India, the model assumes a steady infiltration rate under wet antecedent hillslope conditions. This particular assumption may not be valid for hillslopes in other parts of the world. The application of the model is grossly limited to vegetated hillslopes having a nearly saturated soil layer. The model may not be suitable in arid, semiarid or dry tropical regions, as processes such as variable infiltration rate and unsaturated water flow through the soil were not considered in the model. The process of saturation excess overland flow (Dunne and Black Citation1970) was also not included in the model. Saturation excess overland flow is evident in hillslopes when the water influx to the soil exceeds its absorption and transmission capacity. In many tropical and subtropical watersheds such a flow mechanism occurs. However, under highly active vertical and lateral preferential flow conditions the transmission and absorption capacity of the soil can be so high that saturation excess runoff might not be generated. The experimental evidence from the present hillslope plot also indicated that, with highly active preferential flow conditions, saturation excess overland flow might not occur even under high intensity storm events and wet hillslope soils.
In the proposed model the subsurface flow concept was driven by the dynamics of the perched water table formed over the impermeable layer, which was assumed to be present at a shallow depth. In the absence of a similar subsurface geological formation, the subsurface flow mechanisms would be difficult to explain using the proposed concept of the model. The presence of an impermeable layer in the soil profile is known to have a significant effect on lateral subsurface flow generation. Weyman (Citation1973) identified that the presence of impermeable layer is essential for the initiation of lateral flow. The variations in soil depth affect the subsurface water storage capacity of hillslopes and thus control the lateral storm flow responses. Shallower soils are saturated more quickly and generate higher subsurface lateral flow (Mosley Citation1979). Freer et al. (Citation2002) identified that the bedrock topography may significantly control the mechanism of subsurface storm flow generation from hillslopes. Ticehurst et al. (Citation2003) also reported that soil depth was the most sensitive parameter of the HILLS model. In the present investigation, the experimental hillslope plot had a soil depth of 1 m and this uniform soil depth was considered for the virtual experiments as well. The effects of changing the depth of the impermeable layer on surface and subsurface flow processes needs to be investigated in detail by conducting physical experiments on other hillslopes in the region having different soil depths.
To address the issue of up-scaling the microscale observations to mesoscale, it is most important that the connectivity functions between the hydrological response units in both spatial and temporal domains are adequately understood and represented. Blöschl and Sivapalan (Citation1995) discussed the importance of catchment heterogeneity and the implication of uncertainty in up-scaling of hydrological systems. Distributed parameter models were identified as a way of linking process-level understanding to different scales. Dimensional analysis and similarity concepts might also be useful in handling complex hydrological processes. Stomph et al. (Citation2002) observed that the Hortonian runoff per unit slope length reduced with the increase in slope length. With the help of a simple process-based model, the experimental results were validated and laboratory results were extrapolated to hillslope-scale. Jencso et al. (Citation2009) used a relationship representing hydrological connectivity between hillslopes and streams to transfer the plot-scale findings to landscape-scale and catchment-scale runoff dynamics. Landscape structures showed first-order control on runoff source area and catchment response. Smith et al. (Citation2010) presented a conceptual model to identify the dynamic hydrological connectivity at the hillslope-scale. The spatial and temporal variability of flow resistance captured from plot-scale experiments were extrapolated to hillslope-scale using a morphological runoff zone framework. The hillslope processes, driven by strong preferential flow conditions, are highly complex and interdependent in nature. Therefore, the process of extrapolating such plot-scale results to hillslope-scale or watershed-scale is a critical task and perhaps a holistic approach is required to address the issue in future. There is scope for further improvement of the model by incorporating these features into the model.
CONCLUSIONS
The present investigation demonstrated the sensitivity analysis of a plot-scale hillslope hydrological model through virtual experiments conducted with theoretical hillslopes representing different physical and hydrogeological conditions. Topographical variations and vegetation cover had a significant effect on overland flow response from the theoretical hillslopes. Higher rainfall intensities produced a greater peak discharge with a shorter time to peak. Comparison of overland flow hydrographs showed the effects of profile curvature on tp and tr. Time to peak was mainly controlled by variations in the slope length and gradient, whereas time of recession was mainly a function of storage within the hillslope plot. A reduction of overall relief of the hillslopes, led to increases in the values of tp and tr.
The subsurface stormflow responses of the theoretical hillslopes were analysed assuming that the experimental relationships were valid for the theoretical hillslopes. The matrix and macropore flow hydrographs showed that with increasing rainfall intensities the contributions of both matrix and macropore flows in subsurface stormflow increased. The peak of both hydrographs occurred immediately after the cessation of recharge. However, the macropore flow rates were much higher than the soil matrix flow rates. Macropore flow rates were almost comparable to overland flow rates generated from the hillslopes. There is strong evidence that subsurface stormflow can dominate over surface runoff under favourable conditions (Sloan and Moore Citation1984, Zhang et al. 2006, Scherrer et al. 2007). Unlike macropore flow rates, soil matrix flow rates were influenced by profile curvatures due to changes in hydraulic gradient. In highly macroporous hillslopes, even under extreme recharge conditions, saturation excess overland flow was not evident due to rapid vertical and lateral transmission of infiltrated water. Saturation excess overland flow occurred only under conditions of prolonged recharge with very high rates of vertical water movement and no active lateral preferential flow paths. Therefore, disturbed hillslopes, where human interventions (e.g. shifting cultivation) often lead to discontinuity of lateral macropores, might generate saturation excess overland flow (Shougrakpam et al. Citation2010). Soil matrix flow rates were found to decrease with the reduction in relief. Doubling the macropore diameter did not significantly affect the saturated depth of water above the impermeable layer and macropore flow rates. However, keeping the diameter constant, any change in the value of N had a considerable influence on macropore flow rates.
The computed parametric sensitivity estimates indicated that rainfall intensity was a very sensitive parameter of the model for both overland flow and subsurface stormflow responses. Hillslope topographic profile was the most sensitive parameter to control the recession of overland flow. Peak saturated matrix flow rates largely depended on hillslope relief and topography. Compared to macropore diameter, the number of connected soil macropores was a more sensitive parameter of the model.
The proposed modelling concept was intended to capture the typical hydrological regimes of the forested hillslopes in northeast India. However, considering the physical basis of model development it should be possible to calibrate and apply the model to different forested hillslopes representing similar climate, soil, and hydrological systems.
Acknowledgements
The authors are thankful to Dr S. Panigrahy and Dr J.S. Parihar, Space Applications Centre, Indian Space Research Organization (ISRO), Ahmedabad, India.
Additional information
Funding
REFERENCES
- Adams, R., et al., 2005. Using a rainfall simulator and a physically based hydrological model to investigate runoff processes in a hillslope. Hydrological Processes, 19 (11), 2209–2223. doi:10.1002/hyp.5670.
- Anderson, A.E., et al., 2009. Dye staining and excavation of a lateral preferential flow network. Hydrology and Earth System Sciences, 13 (6), 935–944. doi:10.5194/hess-13-935-2009.
- Anderson, A.E., et al., 2010. Piezometric response in zones of a watershed with lateral preferential flow as a first-order control on subsurface flow. Hydrological Processes, 24 (16), 2237–2247. doi:10.1002/hyp.7662.
- Bahremand, A. and De Smedt, F., 2008. Distributed hydrological modeling and sensitivity analysis in Torysa Watershed, Slovakia. Water Resources Management, 22 (3), 393–408. doi:10.1007/s11269-007-9168-x.
- Beven, K., 1981. Kinematic subsurface stormflow. Water Resources Research, 17 (5), 1419–1424. doi:10.1029/WR017i005p01419.
- Beven, K., 1985. Distributed models. In: M.G. Anderson and T.P. Burt, eds. Hydrological forecasting. Chichester: Wiley, 405–435.
- Beven, K., 1997. TOPMODEL: a critique. Hydrological Processes, 11 (9), 1069–1085. doi:10.1002/(SICI)1099-1085(199707)11:9<1069::AID-HYP545>3.0.CO;2-O.
- Beven, K.J. and Clarke, R.T., 1986. On the variation of infiltration into a homogeneous soil matrix containing a population of macropores. Water Resources Research, 22 (3), 383–388. doi:10.1029/WR022i003p00383.
- Beven, K. and Freer, J., 2001. A dynamic TOPMODEL. Hydrological Processes, 15 (10), 1993–2011. doi:10.1002/hyp.252.
- Beven, K. and Germann, P., 2013. Macropores and water flow in soils revisited. Water Resources Research, 49 (6), 3071–3092. doi:10.1002/wrcr.20156.
- Blöschl, G. and Sivapalan, M., 1995. Scale issues in hydrological modelling: A review. Hydrological Processes, 9 (3–4), 251–290. doi:10.1002/hyp.3360090305.
- Bootlink, H.W.G., Bouma, J., and Droogers, P., 1998. Use of fractals to describe soil structure. In: S.H. Magdi and M. Liwang, eds. Physical non equilibrium in soils: modeling and application. Chelsea, MI: Ann Arbor Press, 157–198.
- Bronstert, A. and Plate, E.J., 1997. Modelling of runoff generation and soil moisture dynamics for hillslopes and micro-catchments. Journal of Hydrology, 198 (1–4), 177–195. doi:10.1016/S0022-1694(96)03306-9.
- Burt, T. and Butcher, D., 1986. Stimulation from simulation? A teaching model of hillslope hydrology for use on microcomputers. Journal of Geography in Higher Education, 10 (1), 23–39. doi:10.1080/03098268608708953.
- Buttle, J.M. and McDonald, D.J., 2002. Coupled vertical and lateral preferential flow on a forested slope. Water Resources Research, 38 (5), 18-1–18-16. doi:10.1029/2001WR000773.
- Chappell, N.A., 2010. Soil pipe distribution and hydrological functioning within the humid tropics: a synthesis. Hydrological Processes, 24 (12), 1567–1581. doi:10.1002/hyp.7579.
- Chaudhry, M.H., 1993. Open channel flow. Englewood Cliffs, NJ: Prentice-Hall.
- Chow, V.T., 1959. Open-channel hydraulics. New York: McGraw-Hill.
- Coenders-Gerrits, A.M.J., et al., 2013. The effect of spatial throughfall patterns on soil moisture patterns at the hillslope scale. Hydrology and Earth System Sciences, 17 (5), 1749–1763. doi:10.5194/hess-17-1749-2013.
- Davies, J. and Beven, K., 2012. Comparison of a multiple interacting pathways model with a classical kinematic wave subsurface flow solution. Hydrological Sciences Journal, 57 (2), 203–216. doi:10.1080/02626667.2011.645476.
- Dikau, R., 1989. The application of a digital relief model to landform analysis in geomorphology. In: J. Raper, ed. Three dimensional applications in geographical information systems. London: Taylor and Francis, 51–77.
- Doherty, J., 2001. PEST-ASP User’s manual. Brisbane, Australia: Watermark Numerical Computing.
- Doherty, J. and Johnston, J.M., 2003. Methodologies for calibration and predictive analysis of a watershed model. JAWRA Journal of the American Water Resources Association, 39 (2), 251–265. doi:10.1111/j.1752-1688.2003.tb04381.x.
- Doherty, J. and Skahill, B.E., 2006. An advanced regularization methodology for use in watershed model calibration. Journal of Hydrology, 327 (3–4), 564–577. doi:10.1016/j.jhydrol.2005.11.058.
- Dunne, T. and Black, R.D., 1970. An experimental investigation of runoff production in permeable soils. Water Resources Research, 6 (2), 478–490. doi:10.1029/WR006i002p00478.
- Dusek, J., et al., 2012. Combining dual-continuum approach with diffusion wave model to include a preferential flow component in hillslope scale modeling of shallow subsurface runoff. Advances in Water Resources, 44, 113–125. doi:10.1016/j.advwatres.2012.05.006.
- Faeh, A.O., Scherrer, S., and Naef, F., 1997. A combined field and numerical approach to investigate flow processes in natural macroporous soils under extreme precipitation. Hydrology and Earth System Sciences, 1 (4), 787–800. doi:10.5194/hess-1-787-1997.
- Fan, Y. and Bras, R.L., 1998. Analytical solutions to hillslope subsurface storm flow and saturation overland flow. Water Resources Research, 34 (4), 921–927. doi:10.1029/97WR03516.
- Fortin, J., et al., 2001. Distributed watershed model compatible with remote sensing and GIS data. I: description of model. Journal of Hydrologic Engineering, 6 (2), 91–99. doi:10.1061/(ASCE)1084-0699(2001)6:2(91).
- Freer, J., et al., 2002. The role of bedrock topography on subsurface storm flow. Water Resources Research, 38 (12), 5-1–5-16. doi:10.1029/2001WR000872.
- Freeze, R.A., 1972a. Role of subsurface flow in generating surface runoff: 1. Base flow contributions to channel flow. Water Resources Research, 8 (3), 609–623. doi:10.1029/WR008i003p00609.
- Freeze, R.A., 1972b. Role of subsurface flow in generating surface runoff: 2. Upstream source areas. Water Resources Research, 8 (5), 1272–1283. doi:10.1029/WR008i005p01272.
- Gerke, H.H. and van Genuchten, M.T., 1993. A dual-porosity model for simulating the preferential movement of water and solutes in structured porous media. Water Resources Research, 29 (2), 305–319. doi:10.1029/92WR02339.
- Germann, P.F. and Beven, K., 1985. Kinematic wave approximation to infiltration into soils with sorbing macropores. Water Resources Research, 21 (7), 990–996. doi:10.1029/WR021i007p00990.
- Germann, P.F., Pierce, R.S., and Beven, K., 1986. Kinematic wave approximation to the initiation of subsurface stormflow in a sloping forest soil. Advances in Water Resources, 9 (2), 70–76. doi:10.1016/0309-1708(86)90012-6.
- Gomi, T., et al., 2008. Characteristics of overland flow generation on steep forested hillslopes of central Japan. Journal of Hydrology, 361 (3–4), 275–290. doi:10.1016/j.jhydrol.2008.07.045.
- Govindaraju, R.S., Kavvas, M.L., and Tayfur, G., 1992. A simplified model for two dimensional overland flows. Advances in Water Resources, 15 (2), 133–141. doi:10.1016/0309-1708(92)90040-9.
- Hook, R., 1998. Landscape structure and function – fundamental causes of land and water degradation. In: J. Williams, R. Hook, and H. Gascoigne, eds. Farming action catchment reaction. Victoria, Australia: CSIRO.
- Hopp, L., McDonnell, J.J., and Condon, P., 2011. Lateral subsurface flow in a soil cover over waste rock in a humid temperate environment. Vadose Zone Journal, 10 (1), 332–344. doi:10.2136/vzj2010.0094.
- Jackson, C.R., 1992. Hillslope infiltration and lateral downslope unsaturated flow. Water Resources Research, 28 (9), 2533–2539. doi:10.1029/92WR00664.
- Jain, M.K., Kothyari, U.C., and Ranga Raju, K.G.R., 2005. GIS based distributed model for soil erosion and rate of sediment outflow from catchments. Journal of Hydraulic Engineering, 131 (9), 755–769. doi:10.1061/(ASCE)0733-9429(2005)131:9(755).
- Jarvis, N. and Larsbo, M., 2003. MACRO 5.0. A model of water flow and solute transport in macroporous soil. Technical description. Sweden: Swedish University of Agricultural Sciences, Department of Soil Science, Division of Environmental Physics.
- Jencso, K.G., et al., 2009. Hydrologic connectivity between landscapes and streams: transferring reach- and plot-scale understanding to the catchment scale. Water Resources Research, 45 (4), W04428. doi:10.1029/2008WR007225.
- Kim, H.J., Sidle, R.C., and Moore, R.D., 2005. Shallow lateral flow from a forested hillslope: influence of antecedent wetness. Catena, 60 (3), 293–306. doi:10.1016/j.catena.2004.12.005.
- Krzeminska, D.M., et al., 2012. A conceptual model of the hydrological influence of fissures on landslide activity. Hydrology and Earth System Sciences, 16 (6), 1561–1576. doi:10.5194/hess-16-1561-2012.
- Lin, H. and Zhou, X., 2008. Evidence of subsurface preferential flow using soil hydrologic monitoring in the Shale Hills catchment. European Journal of Soil Science, 59 (1), 34–49. doi:10.1111/j.1365-2389.2007.00988.x.
- Lin, Y. and Cunningham, G.A. III, 1995. A new approach to fuzzy-neural system modeling. IEEE Transactions on Fuzzy Systems, 3 (2), 190–198. doi:10.1109/91.388173.
- Liu, Q.Q. and Singh, V.P., 2004. Effect of microtopography, slope length and gradient, and vegetative cover on overland flow through simulation. Journal of Hydrologic Engineering, 9 (5), 375–382. doi:10.1061/(ASCE)1084-0699(2004)9:5(375).
- McCord, J.T. and Stephens, D.B., 1987. Lateral moisture flow beneath a sandy hillslope without an apparent impeding layer. Hydrological Processes, 1 (3), 225–238. doi:10.1002/hyp.3360010302.
- Mishra, S.K., et al., 2008. A physically based hydrological model for paddy agriculture dominated hilly watersheds in tropical region. Journal of Hydrology, 357 (3–4), 389–404. doi:10.1016/j.jhydrol.2008.05.019.
- Mosley, M.P., 1979. Streamflow generation in a forested watershed, New Zealand. Water Resources Research, 15 (4), 795–806. doi:10.1029/WR015i004p00795.
- Niyogi, P., Chakraborty, S.K., and Laha, M.K., 2005. Introduction to computational fluid dynamics. Delhi: Pearson Education (Singapore), Indian Branch.
- Rezzoug, A., et al., 2005. Field measurement of soil moisture dynamics and numerical simulation using the kinematic wave approximation. Advances in Water Resources, 28 (9), 917–926. doi:10.1016/j.advwatres.2005.02.010.
- Ritsema, C.J., Oostindie, K., and Stolte, J., 1996. Evaluation of vertical and lateral flow through agricultural loessial hillslopes using a two-dimensional computer simulation model. Hydrological Processes, 10 (8), 1091–1105. doi:10.1002/(SICI)1099-1085(199608)10:8<1091::AID-HYP414>3.0.CO;2-J.
- Ruan, H. and Illangasekare, T.H., 1998. A model to couple overland flow and infiltration into macroporous vadose zone. Journal of Hydrology, 210 (1–4), 116–127. doi:10.1016/S0022-1694(98)00179-6.
- Sarkar, R., 2011. Hydrological response of a preferential infiltration dominated natural hillslope in Brahmaputra river basin. Thesis (PhD). Indian Institute of Technology Guwahati, India.
- Sarkar, R. and Dutta, S., 2009. An experimental and modelling investigation of macropore dominated subsurface stormflow in vegetated hillslopes of northeast India. In: K.K. Yilmaz, I. Yucel, H.V. Gupta, T. Wagener, D. Yang, H. Savenije, C. Neale, H. Kunstmann, and J. Pomeroy, eds. New approaches to hydrological prediction in data-sparse regions. IAHS Publication 333.Wallingford: IAHS Press, 145–152.
- Sarkar, R. and Dutta, S., 2012. Field investigation and modeling of rapid subsurface stormflow through preferential pathways in a vegetated hillslope of northeast India. Journal of Hydrologic Engineering, 17 (2), 333–341. doi:10.1061/(ASCE)HE.1943-5584.0000431.
- Sarkar, R., Dutta, S., and Panigrahy, S., 2008. Characterizing overland flow on a preferential infiltration dominated hillslope: case study. Journal of Hydrologic Engineering, 13 (7), 563–569. doi:10.1061/(ASCE)1084-0699(2008)13:7(563).
- Scanlon, T.M., et al., 2000. Shallow subsurface storm flow in a forested headwater catchment: observations and modeling using a modified TOPMODEL. Water Resources Research, 36 (9), 2575–2586. doi:10.1029/2000WR900125.
- Scherrer, S., et al., 2007. Formation of runoff at the hillslope scale during intense precipitation. Hydrology and Earth System Sciences, 11 (2), 907–922. doi:10.5194/hess-11-907-2007.
- Sharma, R.D., Sarkar, R., and Dutta, S., 2013. Run-off generation from fields with different land use and land covers under extreme storm events. Current Science, 104 (8), 1046–1053.
- Shougrakpam, S., Sarkar, R., and Dutta, S., 2010. An experimental investigation to characterise soil macroporosity under different land use and land covers of northeast India. Journal of Earth System Science, 119 (5), 655–674. doi:10.1007/s12040-010-0042-5.
- Singh, V.P., Sharma, N., and Ojha, C.S.P., 2004. The Brahmaputra basin water resources. Dordrecht, The Netherlands: Kluwer.
- Sloan, P.G. and Moore, I.D., 1984. Modeling subsurface stormflow on steeply sloping forested watersheds. Water Resources Research, 20 (12), 1815–1822. doi:10.1029/WR020i012p01815.
- Smith, M.W., Bracken, L.J., and Cox, N.J., 2010. Toward a dynamic representation of hydrological connectivity at the hillslope scale in semiarid areas. Water Resources Research, 46 (12), W12540. doi:10.1029/2009WR008496.
- Smith, R.E. and Hebbert, R.H.B., 1983. Mathematical simulation of interdependent surface and subsurface hydrologic processes. Water Resources Research, 19 (4), 987–1001. doi:10.1029/WR019i004p00987.
- Stomph, T.J., et al., 2002. Scale effects of Hortonian overland flow and rainfall–runoff dynamics: laboratory validation of a process-based model. Earth Surface Processes and Landforms, 27 (8), 847–855. doi:10.1002/esp.356.
- Ticehurst, J.L., Cresswell, H.P., and Jakeman, A.J., 2003. Using a physically based model to conduct a sensitivity analysis of subsurface lateral flow in south-east Australia. Environmental Modelling & Software, 18 (8–9), 729–740. doi:10.1016/S1364-8152(03)00075-6.
- Troch, P., van Loon, E., and Hilberts, A., 2002. Analytical solutions to a hillslope-storage kinematic wave equation for subsurface flow. Advances in Water Resources, 25 (6), 637–649. doi:10.1016/S0309-1708(02)00017-9.
- Tsutsumi, D., Sidle, R.C., and Kosugi, K., 2005. Development of a simple lateral preferential flow model with steady state application in hillslope soils. Water Resources Research, 41 (12), W12420. doi:10.1029/2004WR003877.
- Uchida, T., Meerveld, I.T., and McDonnell, J.J., 2005. The role of lateral pipe flow in hillslope runoff response: An intercomparison of non-linear hillslope response. Journal of Hydrology, 311 (1–4), 117–133. doi:10.1016/j.jhydrol.2005.01.012.
- Wallach, R. and Zaslavsky, D., 1991. Lateral flow in a layered profile of an infinite uniform slope. Water Resources Research, 27 (8), 1809–1818. doi:10.1029/91WR00879.
- Wang, G.T., et al., 2002. Modelling overland flow based on Saint-Venant equations for a discretized hillslope system. Hydrological Processes, 16 (12), 2409–2421. doi:10.1002/hyp.1010.
- Weiler, M. and McDonnell, J., 2004. Virtual experiments: a new approach for improving process conceptualization in hillslope hydrology. Journal of Hydrology, 285 (1–4), 3–18. doi:10.1016/S0022-1694(03)00271-3.
- Weiler, M. and McDonnell, J.J., 2007. Conceptualizing lateral preferential flow and flow networks and simulating the effects on gauged and ungauged hillslopes. Water Resources Research, 43 (3), W03403. doi:10.1029/2006WR004867.
- Weiler, M., Naef, F., and Leibundgut, C., 1998. Study of runoff generation on hillslopes using tracer experiments and a physically-based numerical hillslope model. In: K. Kovar, U. Tappeiner, N.E. Peters, and R.G. Craig, eds. Hydrology, water resources and ecology in headwaters. IAHS Publication 248. Wallingford: IAHS Press, 353–362.
- Weyman, D.R., 1973. Measurements of the downslope flow of water in a soil. Journal of Hydrology, 20 (3), 267–288. doi:10.1016/0022-1694(73)90065-6.
- Wienhöfer, J. and Zehe, E., 2012. Modelling rapid subsurface flow at the hillslope scale with explicit representation of preferential flow paths. In: EGU General Assembly Conference Abstracts, 14, 12650.
- Zhang, G.P., et al., 2006. Modelling subsurface storm flow with the Representative Elementary Watershed (REW) approach: application to the Alzette River Basin. Hydrology and Earth System Sciences, 10 (6), 937–955. doi:10.5194/hess-10-937-2006.