ABSTRACT
An overlapping set of brain regions in parietal and frontal cortex are engaged by different types of tasks and stimuli: (i) making inferences about the physical structure and dynamics of the world, (ii) passively viewing, or actively interacting with, manipulable objects, and (iii) planning and execution of reaching and grasping actions. We suggest the observed neural overlap is because a common superordinate computation is engaged by each of those different tasks: A forward model of physical reasoning about how first-person actions will affect the world and be affected by unfolding physical events. This perspective offers an account of why some physical predictions are systematically incorrect – there can be a mismatch between how physical scenarios are experimentally framed and the native format of the inferences generated by the brain’s first-person physics engine. This perspective generates new empirical expectations about the conditions under which physical reasoning may exhibit systematic biases.
Three tasks that engage a common network
The motivation for this paper is the empirical observation summarized in : An overlapping set of brain regions in parietal and frontal cortex are engaged when, Panel 1A: Making inferences about the physical structure and dynamics of the world (Fischer et al., Citation2016; Schwettmann et al., Citation2019); viewing, naming or pantomiming the use of manipulable objects (Chao & Martin, Citation2000), and planning reach-to-grasp actions (Culham et al., Citation2003). Those three findings have each been broadly replicated, and the argument of this paper takes them at face value.
Figure 1. A common network of brain regions supports manipulable object representation, intuitive physics inferences, and action planning. (A) Regions in parietal and frontal cortex that are engaged during intuitive physics inferences (Fischer, Citation2020; Navarro-Cebrian & Fischer, Citation2022). Those regions are more active during physical prediction than during difficulty-matched tasks requiring prediction in other domains (Fischer et al., Citation2016). (B) The network that is more active during viewing of manipulable objects compared to animals, places and faces (for the original observation, see Chao & Martin, Citation2000; data from Kristensen et al., Citation2016). (C) Regions engaged in reaching and grasping, from (Culham et al., Citation2003, see also Gallivan & Culham, Citation2015). While the task demands and stimuli used to localize the three networks are markedly different on their surface, they engage overlapping parietal and frontal areas. Of particular note, is the role of the supramarginal gyrus across intuitive physics, manipulable object representation, action planning and execution, and (not shown) phonological processing (Oberhuber et al., Citation2016)
.
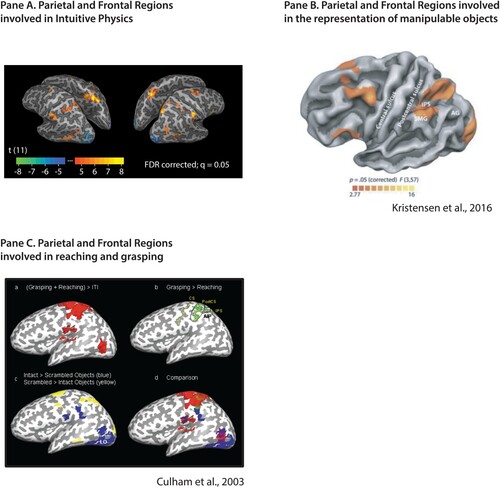
The goal of this paper is to sketch a proposal about why those three tasks seem to co-locate in the brain. We argue that the parieto-frontal network shown in , centred on the supramarginal gyrus, supports a common computation that is engaged across physical reasoning, manipulable object representation, and action planning. That common computation is a forward model of physical reasoning about how first-person actions will effect changes in the world, and how actions will in turn be constrained by unfolding physical events.
This proposal is based on the idea that the brain has a “physics engine” which supports inferences about what will happen next in a scene (Battaglia et al., Citation2013; Pramod et al., Citation2022). Within the framework of thinking of the brain’s physics engine as a type of forward model, we emphasize the first-person reference frame that supports action.
Specialization of function – for what?
A dominant paradigm in cognitive neuroscience involves identifying and studying brain areas that show differential activity for a particular type of stimulus or process, compared with “theoretically relevant” control conditions. What qualifies as “theoretically relevant” depends on the hypothesis being tested and on assumptions about what the brain region(s) in question do(es). For instance, the same stimulus rotated 90 degrees in its orientation may be a relevant baseline for an orientation-tuned cell in early visual cortex, while the appropriate control in high-level visual areas may be a stimulus from a completely different semantic category (e.g., the relevant baseline for an image of a cat, in the fusiform gyrus, may be a picture of a house).
The regions in were defined using different types of experimental stimuli and control conditions. The contrast for physical reasoning (Panel 1A) compared judgments about where the majority of blocks would land if unstable block towers were to fall, to difficulty-matched judgments of the visual features of the same stimuli (whether there were more blue or yellow blocks present in the tower). The comparison for manipulable objects (Panel1B) contrasted naming handheld graspable and manipulable objects (fork, glass, hammer) against naming faces, animals and places. The grasp-related areas (Panel 1C) were identified by subtracting activity for reach-to-touch actions from activity during reach-to-grasp actions. The notable heterogeneity of stimuli and tasks across the experiments summarized in motivates the present proposal, which seeks to “zoom out” in thinking about what those regions are doing. This point has been made before in many other contexts: Specificity of a region for a given computation is a theoretical hypothesis that must be inferred from a pattern of responses. Our proposal, for the brain regions discussed herein, is that the “theoretically relevant pattern of responses” is broader than what has been considered in any of the respective sub-fields that generated the findings summarized in .
Perception in the service of predicting the future
Visual processing comprises separable operations over distinct dimensions of the visual input – form, motion, colour, depth, location, orientation, axis correspondence, and so on. A high-level organizing principle within the visual system – orthogonal to many of those dimensions – distinguishes “vision for perception” from “vision for action”. The occipito-temporal pathway, or ventral stream, supports detailed perception and visual recognition, while a subcortical and dorsal occipito-parietal pathway supports object localization and visual analysis in the service of concurrently unfolding actions (Goodale et al., Citation1991; Goodale & Milner, Citation1992; Schneider, Citation1969; Ungerleider & Mishkin, Citation1982; for further discussion on how best to characterize the visual streams see Freud et al., Citation2020; Livingstone & Hubel, Citation1988; Mahon, Citationin press; Merigan & Maunsell, Citation1993; Pisella et al., Citation2006; Schenk, Citation2006; Xu, Citation2018).
Everyday interactions with objects involve processing of visual form, surface-texture and material properties, object location in various reference frames, action goals, object identity, object function, lexical semantics, linguistic forms, and learned motor competencies and skills. Functional object use involves the orchestration of that entire diversity of representations in the service of behaviour. Those separable processes are supported by brain regions across the ventral and dorsal visual streams. A subset of the regions engaged by these processes overlap with regions involved in action planning. In particular, the left ventral precentral gyrus (premotor cortex), the left supramarginal gyrus, and the anterior IPS bilaterally (but stronger in the left).
The first wave of empirical reports describing the neural representation of manipulable objects emphasized that the relevant parietal and frontal areas were also involved in action planning and execution (Chao & Martin, Citation2000; Culham et al., Citation2003; Mahon et al., Citation2007; Noppeney et al., Citation2006). Indeed, it has been debated whether manipulable object representations obligatorily involve a form of motor simulation. Generalizing over much discussion, there is broad agreement that object-directed actions are a type of knowledge (“knowledge” understood broadly) that is automatically engaged when thinking about manipulable objects (Martin, Citation2016). The key point is that past discussions have been premised on the view that “manipulable objects (‘tools’) engage the action system”. In other words, with respect to parietal-frontal areas, and in particular the supramarginal gyrus, it has been assumed the processes indexed by activity in those regions have to do with action or motor-relevant processes.
An alternative view is that processing of manipulable objects and action planning both drive inferences about the next state of the world. The brain does not wait until “after perception is over” to determine how to interact with the environment – and it does not wait until after action is over to understand how an action may affect the world. The default posture of the system is to be continuously inferring what will happen next in the environment. Those inferences inform action and perception. Which is to say, implicit physical reasoning is constantly evaluating how potential actions will interact with the world, and what the next state of the world is likely to be. The process is ongoing and iterative. Physical inferences drive further perceptual analysis, which drives further physical reasoning. On this view, the computations supported by the supramarginal gyrus (and other regions in the network) may be better thought of as implementing a forward model that supports first-person inferences about future states of the world.
There has been much discussion about whether parietal and frontal areas are specialized for “tools” as a category. But to say that a region is specialized for processing tools amounts to little more than a redescription of the data – i.e., the experimental conditions under which disproportionately high levels of activity are observed. “Tools”, or manipulable objects, describes a set (or category) of graspable objects for which the function and manner of manipulation and visual structure are all tightly related (Mahon et al., Citation2007). The system is not specialized for manipulable objects per se. Rather, there are certain computations that are demanded of successful processing of manipulable objects and which are not demanded by processing of animals, faces or places (Mahon, Citation2020).
Physical reasoning is often tested in contexts in which the layout is unfamiliar – using novel objects to push the system into a state where it has to reason, de novo, about the behaviours of objects based on their structure and dynamics. This de novo reasoning itself may be a key component of the underlying processes. For instance, Weisberg, van Turennout, and Martin (Citation2007) showed that learning form-function relations for novel objects drove activity in the same regions highlighted in (Weisberg et al., Citation2007; see also Martin & Weisberg, Citation2003 for a study using Michotte causality driving parts of the network).
There is considerable neuropsychological evidence that lesions involving the fronto-parietal network highlighted in are associated with specific grasping or object manipulation impairments. In particular, patients with upper limb apraxia have an impairment in using objects that cannot be attributed to basic sensory or motor deficits (Rothi et al., Citation1991; Rumiati et al., Citation2001). Upper limb apraxia is classically associated with damage to the left supramarginal gyrus, in the inferior parietal lobule (Goldenberg, Citation2014; Gonzalez-Rothi & Heilman, Citation1996). By contrast, aIPS supports the computation of hand postures for object-directed grasping (Binkofski et al., Citation1998; Culham et al., Citation2003). Some authors have argued that upper limb apraxia is fundamentally a disruption to “de novo mechanical problem solving abilities” (Goldenberg & Spatt, Citation2009); such accounts emphasize that complex object-directed actions are not stored as integral representations but are rather built on the fly.
The “errors” of intuitive physics result from a feature, not a glitch, in the system
In certain circumstances, naïve observers make dramatic errors in their judgments about the physical contents and dynamics of seemingly simple scenarios (Caramazza et al., Citation1981; Gilden & Proffitt, Citation1994; Ludwin-Peery et al., Citation2020, Citation2021; McCloskey et al., Citation1980). These errors in physical reasoning have generally been viewed as revealing a glitch in the system – an incorrect or incomplete mental model of physics that leads to misconceptions about the latent physical structure of a scene or the way its physical events will unfold. However, these errors might instead be viewed as a consequence of a mismatch between the native format of the computations that support intuitive physics reasoning in everyday life, and the format of the hypothetical scenarios used to cue explicit responses and descriptions of how physical interactions will unfold. The “errors” of intuitive physics judgements could result from what is otherwise a “feature” – a dedicated system that supports in situ and first-person inferences about how the state of the world may change.
A classic paradigm asks participants to diagram the trajectory of a moving object in the absence of external forces – for instance, a ball launched through a curved tube seen from directly overhead. People often draw a curved path as though the tube would impart a persisting curvilinear trajectory to the ball (McCloskey et al., Citation1980). Diagrams of curvilinear motion in this scenario are at odds with natural physical behaviour, where the ball would maintain a straight path in the absence of external forces. The prevalence and magnitude of errors in people’s reports has presented a puzzle, especially alongside other circumstances in which physical predictions are accurate and highly precise. The format of the scenario might be key to understanding why it leads to incorrect responses – the scenario forces the observer to represent the movement in a specific allocentric reference frame. By hypothesis, that reference frame is disconnected from the format of the inferences the system has available.
Consider what would be involved in observing the Newtonian dynamics of a large, faraway object – for example, a boulder tumbling down a mountain, or a tree falling after it has been chopped down. The retinal sizes of such objects can be the same as those of nearby objects on one’s desk, and the same physical laws apply to both the distant and nearby objects. Yet the observed physical dynamics are quite different in their visual patterns. The boulder appears to fall in slow motion because its visual acceleration profile does not match the acceleration of falling objects that are close to the observer. These are the types of divergent cues that may be generated by separable systems about what will happen as the event unfolds.
Another classic finding is the “straight down belief:” people diagram the path of an object after it is released by a walking person as falling straight down (McCloskey et al., Citation1983). In a similar manner to the scenario above, these errors in prediction could be due to the requirement to report the results of the event from the 3rd person perspective – a format that may not align with how the system represents the event internally. If physical predictions are informed by a system that sees the world in the first person, then participants’ answers are actually correct: The ball does fall straight down from the perspective of the person walking. The “erroneous” judgement is a product of the mental model that participants have for completing the task, which operates first and foremost in the service of physical inference for first-person interactions.
On this view, the intuitions of intuitive physics cannot help but be influenced by the “suggestions” generated from a first-person perspective on how the state of the world may change. The computations of the dorsal stream are inherently in the first person – and the dorsal stream is one source for the generation of such inferences. The “suggestions” that the dorsal stream may make to the rest of the brain are in the service of fitting actions to the current state of the world.
The tasks used in intuitive physics studies often ask for declarative and explicit judgements about how the world will be. By hypothesis, it is the inescapability of our physical reasoning from the suggestions made by the dorsal stream that causes some physical judgments to be systematically incorrect when queried in scenarios that run counter to the expectations of the system. Physical reasoning itself is not a dorsal process – the physical reasoning task is an explicit and declarative perceptual task. The physical reasoning task is equivalent to asking about the perceptual consequences of what will happen in the world.
There is an intriguing and instructive analogy between participants’ erroneous judgements in physical reasoning tasks and some visual illusions, such as the Ebbinghaus/Titchener size constancy illusion (Titchener, Citation1901). Size constancy illusions are not a glitch in the system – they are a result of a feature (size constancy) that is a key element of a stable perceptual experience of the world. Errors in intuitive physical reasoning, like the Ebbinghaus/Titchener visual illusion, expose an aspect of how the system works: Some computations are applied in a compulsory manner. “Compulsory” in this context implies both “automatic” and “in a manner that is relatively encapsulated”, from declarative knowledge that is also held about the world. For instance, knowing about the Ebbinghaus/Titchener illusion, even as one is staring at it, does not make it stop.
This framing of the source of some erroneous intuitive physics judgements generates several predictions about the experimental conditions under which people will display accurate or mistaken physical inferences. Some of these find preliminary support and some, we hope, will spur future research:
The accuracy of some physical predictions will be modulated by the reference frame in which the scenario is depicted. The directional prediction is that scenarios that are presented in egocentric frames will yield more accurate predictions than those presented in allocentric frames. Some of the examples above align with that expectation, and other studies have also highlighted cases where physical reasoning can fail for scenarios that are depicted as being outside peri-personal space, or the space in which visually guided actions typically operate (Ludwin-Peery et al., Citation2020).
Some systematically erroneous physical inferences should become accurate when the judgement is rendered by the participant via an action. Returning to the analogy to the Ebbinghaus/Titchener illusion, an important finding is that the illusory effect is less pronounced when the size judgment is rendered implicitly via spontaneous grip aperture during a reach-to-grasp action. The same illusory stimulus that generates a size constancy illusion in perception tricks the hand less during a visually guided grasp (Aglioti et al., Citation1995; Goodale, Citation2011). In this example and others, a task that asks participants to report on their perceptual experience can yield responses that are systematically incorrect. Similarly, we suggest, physical reasoning performance should improve if judgements about the unfolding physics of an event are collected via actions on the part of participants.
Prediction 2 is in line with an argument put forward by Smith and colleagues (Smith et al., Citation2018), and finds some preliminary empirical support from several studies, including one in this special issue (Neupärtl et al., Citation2022). When sliding a puck toward a target (Neupärtl et al., Citation2022) or moving a bin to catch a falling object (Smith et al., Citation2013), participants produce actions that are in line with Newtonian physics even when their explicit reports or categorical judgments about the scenarios are erroneous. Even for judgments about the behaviour of liquids in containers, which are notoriously challenging, pantomiming an action on the container can lead to substantially improved predictions (Schwartz & Black, Citation1999).
It is important to note that not all studies that used natural actions to probe physical predictions found improvements in accuracy, compared to explicit report (McCloskey & Kohl, Citation1983). Understanding the boundary conditions that allow the system to generate the correct judgements for a given physical event will provide important constraints on hypotheses about the causes of systematic biases.
What we are (and are not) arguing
We assume that the dorsal stream is not cognitively penetrable. Our argument is not in conflict with the view that the dorsal stream is (relatively) informationally encapsulated. Indeed, we would subscribe to a robust version of the view that declarative knowledge systems can intervene only on inputs and outputs to the dorsal stream but not internal machinations, and that dorsal stream computations do not have access to semantic interpretations of the world. The dorsal stream is focused on processing visual information in support of real-time actions by the hand, eye, body – it is a type of “lidar” for the body (see discussion in Mahon & Wu, Citation2015; Mahon, Citationin press).
As noted, it is important that the same naïve observers who make incorrect physical inferences in certain scenarios (e.g., “the ball will drop straight down”) will make the correct actions in the first person (e.g., catch the same ball). Intuitive physics inferences are shaped by information being generated by the dorsal stream; performing the correct action in the first person to catch the ball, and thus displaying veridical underlying representation of the ball’s trajectory, is a dorsal process. When the task used to query physical inference is aligned with the suggestions of the dorsal stream, there is no conflict, and performance is not systematically wrong.
While the “physics engine in the brain” might be informed by dorsal stream processing, intuitive physics judgements as they are typically studied are not “dorsal stream inferences”. The explicit, declarative nature of participants’ reports in most intuitive physics tasks (e.g., drawing the path a falling object will take, or predicting the position where it will land) cannot draw directly on dorsal processes because those processes are siloed from broader declarative knowledge. This disconnect is (we suggest) precisely why many intuitive physics judgements can be systematically wrong. One simply cannot intervene to stop the information from being generated in a particular way, just like one cannot stop the perceptual (ventral) visual system from generating the Ebbinghaus/Titchener illusion. The physical “inferences” that guide a reach to an object’s centre of mass, or help one dodge a snowball, play out in the dorsal stream; however, declarative tasks that ask for reports about those inferences cannot access the contents of the computations for explicit report. Explicit reports might be garden-pathed by dorsal stream contributions, but the garden-pathing can be more of a hindrance than a benefit for explicit tasks in an allocentric format. On this view, some intuitive physics errors reflect a type of illusion, that ironically, is made possible by a system (dorsal stream) that specializes in representing reality veridically.
Physical reasoning is not just visual mental imagery. It feels natural to think about physical prediction as a movie playing out in one’s head – a form of visual mental imagery. And, of course, we would not disagree that visual mental imagery can be engaged in some physical reasoning tasks. However, does having more vivid mental imagery allow for better predictions? Or at the very least, is a lack of mental imagery an impediment for accurately forecasting physical events? Recent findings (Washington & Fischer, Citation2021) suggest that vivid visual imagery does not predict good performance on intuitive physics tasks, and, if anything, can be slightly detrimental. Perhaps the vividly “seen” outcomes in mental imagery can lead judgements astray, as some sources of physical intuitions do not manifest as images (e.g., a catching action of the ball whose trajectory is being predicted). Similarly, recent work has shown that intuitive physics is not simply a special case of spatial cognition (Mitko & Fischer, Citation2020) – the two domains make distinct contributions to individual differences in performance on physical reasoning tasks.
This is not to say that imagery itself is the source of errors in physical reasoning. The source of the miscalculation could be generated by systems having nothing to do with imagery per se. Imagery may be where the error is detected in intuitive physics tasks; where an error is detected is not always where it arises.
The role of visual mental imagery in supporting intuitive physics reasoning is of direct relevance to our proposal – as visual mental imagery engages bilateral posterior parietal regions. The regions that are engaged in visual mental imagery are posterior and superior to the parietal regions highlighted in . Xu (Citation2018) has argued for a new framework to understand the nature and source of posterior parietal visual representations. On Xu’s proposal, while ventral stream representations achieve invariance in order to provide a stable basis for perception, the role of the posterior parietal cortex is to process visual representations in a manner that is tuned to the current task – “adaptive” visual processing. A component of Xu’s proposal is that posterior parietal visual representations are dependent on connectivity with occipitotemporal areas, potentially via the vertical occipital fasciculus (Kravitz et al., Citation2013; Yeatman et al., Citation2014).
Thus, and to be perfectly speculative, when the task used to query the intuitive physics judgements imposes an allocentric frame, the system is pushed toward having to use its mechanisms for adaptive visual manipulation to solve the task. That system of adaptive visual manipulation (mental imagery) is like a white board for physical reasoning. The task solution is disconnected from some of the first-person inferences that are being generated.
Indeed, we can process and make predictions about events that are not first person – it’s not that we cannot perceive, think about, and make predictions in allocentric frames. A growing literature has implicated regions of lateral posterior temporo-occipital cortex that process event structure driven by biological agents, and event structure driven by mechanical interactions among inanimate entitles (Beauchamp et al., Citation2002; Beauchamp et al., Citation2003; Wurm et al., Citation2017). It is an open question as to why those lateral temporo-occipital regions are not able to support veridical physical reasoning in scenarios that yield systematically wrong intuitive physics judgements. Nonetheless, such systems live alongside the endogenous first-person physics engine that we have argued is supported by the fronto-parietal network highlighted in .
What does “overlap” even mean?
Our argument is (unapologetically) rooted in a form of “reverse inference” (Poldrack, Citation2011), and based on a simple-minded construal of “neural overlap”. “Neural overlap” is in turn based on empirical observations that have not (yet) been demonstrated within the same individuals (across tasks). The empirical generalization on which we have premised our proposal is that the supramarginal gyrus, together with other parietal and frontal areas, is reliably engaged across different tasks (). We have argued for the strongest form of our proposal: There is a superordinate computation shared by those tasks. Here we unpack some challenges faced by this proposal. The intention is not to put such concerns to rest, but to expose the vulnerabilities of our proposal as clearly as possible, with the goal of motivating empirical studies that might resolve the issues.Footnote1
There is precedent in the field for the general structure of our argument: Observations of neural overlap are used to support inferences of common computations. There are also clear examples where prior claims of computational overlap, motivated by neural overlap, have been empirically disconfirmed. Briefly reviewing a few examples will help frame expectations for evaluating the current proposal.
Perhaps the most widely discussed example comes from the class of proposals that are broadly descended from Motor Theories of Perception (Liberman et al., Citation1967): Observations that motor production processes (and their brain regions) are automatically active during perception have been taken as evidence that production process are (constitutively) part of perception. The “mirroring” hypothesis argues that a motor computation is involved in both action (by definition, as it is the motor system) and perception and recognition (Rizzolatti & Fogassi, Citation2014). The “mirroring nature” of some neural responses in some motor areas has been invoked as an explanation of how we recognize speech sounds, the sounds of bodily actions, and visual observations of hand actions (among other applications; di Pellegrino et al., Citation1992; Galantucci et al., Citation2006; Pazzaglia et al., Citation2008; for broader and critical discussion, see Dinstein et al., Citation2008; Hauk, Citation2016; Hickok, Citation2009; Negri et al., Citation2007). Stepping back from Motor Theories of Perception: There are “motor simulation” approaches to meaning representation that emphasize the role of motor processing in knowledge representation (Gallese & Lakoff, Citation2005; Glenberg, Citation2015; Pulvermuller, Citation2013). Those proposals assume that, for instance word meanings that refer to actions (kick, punch, etc.), depend on the concurrent simulation of the corresponding motor processes. The evidence for such simulationist accounts of meaning representation is that the motor system is automatically active during the processing of meaning of those words (Hauk et al., Citation2004). A third group of proposals emphasizes the role of motor processes and regions in motor imagery (see review and discussion in Hetu et al., Citation2013).
There are thus three related groups of “motor simulationist” proposals that all start with an observation of overlap – namely that motor processes/regions are active during action perception | understanding | imagery.Footnote2 All three proposals assume a common superordinate (in this case motor) computation is drawn upon across tasks.
“Motor simulationist” theories are vulnerable to empirical disconfirmation in at least two ways: (i) show that transient or long-term disruption of the motor region in question does not disrupt perception | meaning representation | imagery; and/or (ii) disconfirm the empirical premise of “overlap”, for instance with methods or techniques with greater spatial sensitivity. We briefly consider two examples here to illustrate how such tests could be applied to our proposal and some implications.
An example test using lesion evidence. The observation that listening to speech sounds leads to activity of speech motor areas has been argued to support the claim that motor production processes (in speech) are constitutively involved in perception of speech (D'Ausilio et al., Citation2009; Galantucci et al., Citation2006). Stasenko and colleagues (Citation2015) found that a brain lesion to the speech motor system can disrupts speech motor ability while completely sparing the ability to perceive speech sounds (see also Rogalsky et al., Citation2011). Such patients cannot reliably produce “pear” versus “bear”, but have no difficulty discriminating those minimal pairs (p/b). “Motor simulationist” theories predict that perceptual processes will (necessarily) be disrupted in the measure to which the primary motor processes are disrupted. Thus, such observations are incompatible with the claim that motor simulation is a necessary part of perception (see discussion in Lotto et al., Citation2009; Stasenko et al., Citation2013).
An example test of the claim of overlap: Persichetti and colleagues (Persichetti et al., Citation2020) applied a new functional MRI method called vascular space occupancy (VASO) to the question of whether there is overlap between motor production and motor imagery within primary motor cortex. VASO measures cerebral blood volume and has higher contrast-to-noise at high spatial resolution than conventional BOLD fMRI (Huber et al., Citation2017). VASO has the sensitivity to distinguish superficial laminar activity, associated with afferent inputs, from deep laminar activity, associated with efferent outputs (Huber et al., Citation2017). The technique is thus suited to test for intra-cortical overlap. Using that technique, Persichetti and collogues found that overt hand actions (finger tapping) led to activity in both superficial and deep layers, while motor imagery engaged only superficial layers. Thus, the theoretically predicted overlap was not found. By comparison, conventional BOLD fMRI with the same experiment produces robust “overlap”, but only because the technique does not distinguish the different cortical layers where overlap is not present. While Persichetti and colleagues focused on motor imagery, their findings motivate similar tests of other motor simulationist theories (see discussion in Mahon, Citation2020).
The bottom-line of this short discussion cautions against inferring computational overlap from neural overlap – that gambit is bound to fail as new technology dissects the functional organization of the brain at finer and finer scales of spatial resolution. Let us (perhaps safely) assume, for the sake of argument, that a future empirical study will disconfirm the assumption of “overlap” on which our proposal is premised.
For instance, lets us assume that a traditional (e.g., 3 mm voxel size) BOLD fMRI study confirms our core prediction that there will be overlap, within individual brains, in the supramarginal gyrus for tool representation, intuitive physics, and grasping. Imagine then a subsequent study, using higher resolution BOLD fMRI (or, for instance, VASO) finds such areas of “overlap” can be separated into smaller subregions that are task specific. Imagine this hypothetical study finds one subregion (or single unit, or cortical layer) involved in tool representation, while another subregion is involved in intuitive physics judgements (and so on). Empirically, it would be clear that the “overlap” was only apparent, and due to the (comparatively) low spatial resolution of conventional BOLD fMRI. How would such an outcome relate to the hypothesis that there is a superordinate computation that is common across tasks, and which is implemented by the supramarginal gyrus, together with the broader network in ?
Consider the following analogy. Gambling is legal in some locations in the United States. One of those locations is the entire state of Nevada. In this example, Nevada is analogous to the supramarginal gyrus, and the Nevada law that makes gambling legal is analogous to the “superordinate computation” that is common across the tasks highlighted in . One could further analogize the different contexts (cards, roulette, sports, etc.) in which “gambling” is implemented as analogues to the different tasks that activate the supramarginal gyrus. Now let’s run the overlap experiment on the analogy: There could be two ways in which gambling is implemented by task (i.e., by cards, roulette, sports) geographically across Nevada. In one world, only a single type of gambling (cards OR roulette OR sports games) occurs at any given physical establishment or business. In the other world, any given establishment, at any given physical location, has all types of gambling occurring under one roof. Both worlds, to our intuition, are compatible with the idea that a superordinate computation (the Nevada law) is the reason why all of the different forms of gambling (cards, sports, roulette) can occur in Nevada (and not in nearby states).
The point of the gambling analogy is illustrative (as opposed to demonstrative) about what granular physical overlap, or lack thereof, might mean for a theory of how processing unfolds across different tasks. In the end, the core issue reduces to specifying the relevant spatial granularity at which overlap is expected – “relevant”, because this begs the hard problem of specifying what parts of the biology match up with which parts of a computational theory of how the process works (the “granularity mismatch problem”; Krakauer et al., Citation2017; Poeppel, Citation2012). Perhaps “brain region”, as identified functionally using BOLD fMRI, picks out areas that for which a condition is met that allows a certain type of computation to occur (the way the laws of Nevada are the condition for gambling, in all its forms, to co-localize in Nevada).
One difference between our interpretation of “overlap”, compared to the “motor simulationist” examples discussed above, is that our proposal posits a “superordinate” computation that applies to all tasks. By contrast, motor simulationist theories started with the assumption that motor-relevant areas implement motor processes; thus, any task that activates those areas is assumed to involve a motor process. That assumption was licensed, within the framework of reverse inference, because the effects were observed in motor- or peri-motor areas (localized by a “motor task”). The common computation that we have proposed is not perfectly aligned with any one task. Indeed, one way in which the view that we have proposed can be further challenged and tested is to consider other tasks that may also drive activity in the same regions/network.
Notably, there is a well-attested role of the supramarginal gyrus in phonological processing (for review and empirical investigation, see Oberhuber et al., Citation2016). Is this a counterfactual to our proposal? Or, does it further triangulate a computational refrain common to the tasks that engage the supramarginal gyrus? Phonemes are perceptual categories (in speech perception) and action categories (in production). As high-level action categories, phonemes must be implemented and linearized, with accommodation (e.g., co-articulation) to the current and future states of the speech system. Hickok and Poeppel (Citation2007) argued that the dorsal language pathway, which is supported by the long fibres of the arcuate fasciculus, maps sound categories to motor categories – and the supramarginal gyrus is a key hub integrating the “long” segment of the arcuate fasciculus (connected to frontal speech motor areas) with the descending segment of the arcuate (connecting to temporal lobe perceptual representations). The expectation on our proposal would be that what the supramarginal gyrus is “doing” in the context of phoneme processing is fundamentally about prediction.
To conclude this discussion about “overlap”, we return to another potential take-away from the analogy to gambling. The Nevada law that makes gambling possible need not describe every form that gambling may take. We have emphasized the idea of a common superordinate computation across tasks that seeks to explain why there is neural overlap. The neural overlap does not necessarily tell us what the computation is (although we have suggested it can be triangulated by studying the different tasks and contexts that lead to activity in that region). Perhaps what makes the supramarginal gyrus play host to such a diverse set of tasks is not a computation, but a condition that makes certain types of computations possible. Each of those computations might be inherently tasks specific (like different forms of gambling), but they share (like gambling) a common local condition that allows them to colocalize there (while other brain regions do not have such conditions). One intriguing possibility is that such 'conditions' can be quantified in terms of patterns of structural connectivity.
Next steps
Why do the representation of manipulable objects, action planning, and intuitive physics co-localize in fronto-parietal areas? The premise of this review has been that it is because of a common computation: Physical reasoning about the future state of the world in a first-person perspective. Actions are first person; perception is also first person. Thus, by arguing for a computational stance that is inherently first person, we are not arguing that the relevant superordinate computation is necessarily motor- or action-based. Both perceptual and motor constraints shape the inferences that are generated by the brain’s first-person physics engine.
Of the regions that emerge across studies, the supramarginal gyrus is a structure that we would (speculatively) propose as the basis for such predictive first-person inferences. The supramarginal gyrus is a structure that anatomically and functionally integrates perception (vision, auditory, proprioceptive, somatosensory) with actions (by the hands, mouth, limbs, and eyes). Presumably, the conditions (or computations) that drive co-localization of those tasks to the supramarginal gyrus and its network are innately specified. When thinking about why there are innate constraints in the brain, it is easy to gin up stories of selective pressures operating to encourage the system toward its current organization. Such “just so” stories offer no hard constraints on theories. This is because the pressures that led to the current universal organization may not be the same as current use, and the pressures may not have even had to do with current function (Dehaene & Cohen, Citation2007). “Innate constraints” does not imply “selected for current use” – it could have been a spandrel of other constraints (Gould & Lewontin, Citation1979).
Actions, manipulable object representation, and intuitive physics reasoning are tasks and groupings of stimuli. Those tasks and groupings of stimuli neatly capture variance in neural responses in the common fronto-parietal network shown in because each of those tasks, by hypothesis, engages a computation that is enabled by that network. The merit of the approach we have proposed will be weighed in whether it generates expectations that organize available evidence and future studies.
Disclosure statement
No potential conflict of interest was reported by the author(s).
Additional information
Funding
Notes
1 We are very grateful to Alex Martin for raising the conceptual issues, and some of the empirical examples, to be discussed in this section.
2 It should be noted that the original Motor Theory of Speech Perception predates all forms of functional brain imaging.
References
- Aglioti, S., DeSouza, J. F., & Goodale, M. A. (1995). Size-contrast illusions deceive the eye but not the hand. Current Biology, 5(6), 679–685. https://doi.org/10.1016/S0960-9822(95)00133-3
- Battaglia, P. W., Hamrick, J. B., & Tenenbaum, J. B. (2013). Simulation as an engine of physical scene understanding. Proceedings of the National Academy of Sciences, 110(45), 18327–18332. https://doi.org/10.1073/pnas.1306572110
- Beauchamp, M., Lee, K., Haxby, J., & Martin, A. (2003). FMRI responses to video and point-light displays of moving humans and manipulable objects. Journal of Cognitive Neuroscience, 15(7), 991–1001. https://doi.org/10.1162/089892903770007380
- Beauchamp, M. S., Lee, K. E., Haxby, J. V., & Martin, A. (2002). Parallel visual motion processing streams for manipulable objects and human movements. Neuron, 24(1), 149–159. https://doi.org/10.1016/S0896-6273(02)00642-6
- Binkofski, F., Dohle, C., Posse, S., Stephan, K. M., Hefter, H., Seitz, R. J., & Freund, H. J. (1998). Human anterior intraparietal area subserves prehension: A combined lesion and functional MRI activation study. Neurology, 50(5), 1253–1259. https://doi.org/10.1212/WNL.50.5.1253. https://www.ncbi.nlm.nih.gov/pubmed/9595971
- Caramazza, A., McCloskey, M., & Green, B. (1981). Naive beliefs in “sophisticated” subjects: Misconceptions about trajectories of objects. Cognition, 9(2), 117–123. https://doi.org/10.1016/0010-0277(81)90007-X
- Chao, L. L., & Martin, A. (2000). Representation of manipulable man-made objects in the dorsal stream. Neuroimage, 12(4), 478–484. https://doi.org/10.1006/nimg.2000.0635
- Culham, J. C., Danckert, S. L., DeSouza, J. F., Gati, J. S., Menon, R. S., & Goodale, M. A. (2003). Visually guided grasping produces fMRI activation in dorsal but not ventral stream brain areas. Experimental Brain Research, 153(2), 180–189. https://doi.org/10.1007/s00221-003-1591-5
- D'Ausilio, A., Pulvermuller, F., Salmas, P., Bufalari, I., Begliomini, C., & Fadiga, L. (2009). The motor somatotopy of speech perception. Current Biology, 19(5), 381–385. https://doi.org/10.1016/j.cub.2009.01.017
- Dehaene, S., & Cohen, L. (2007). Cultural recycling of cortical maps. Neuron, 56(2), 384–398. https://doi.org/10.1016/j.neuron.2007.10.004
- Dinstein, I., Thomas, C., Behrmann, M., & Heeger, D. J. (2008). A mirror up to nature. Current Biology, 18(1), R13–R18. https://doi.org/10.1016/j.cub.2007.11.004
- di Pellegrino, G., Fadiga, L., Fogassi, L., Gallese, V., & Rizzolatti, G. (1992). Understanding motor events: A neurophysiological study. Experimental Brain Research, 91(1), 176–180. https://doi.org/10.1007/BF00230027
- Fischer, J. (2020). Naive physics: Building a mental model of how the world behaves. In M. Gazzaniga, G. R. Mangun, & D. Poeppel (Eds.), The cognitive neurosciences (VI) (pp. 779–785). MIT Press.
- Fischer, J., Mikhael, J. G., Tenenbaum, J. B., & Kanwisher, N. (2016). Functional neuroanatomy of intuitive physical inference. Proceedings of the National Academy of Sciences, 113(34), E5072–E5081. https://doi.org/10.1073/pnas.1610344113
- Freud, E., Behrmann, M., & Snow, J. C. (2020). What does dorsal cortex contribute to perception? Open Mind, 4, 40–56. https://doi.org/10.1162/opmi_a_00033
- Galantucci, B., Fowler, C. A., & Turvey, M. T. (2006). The motor theory of speech perception reviewed. Psychonomic Bulletin & Review, 13(3), 361–377. https://doi.org/10.3758/bf03193857
- Gallese, V., & Lakoff, G. (2005). The brain's concepts: The role of the sensory-motor system in conceptual knowledge. Cognitive Neuropsychology, 22(3), 455–479. https://doi.org/10.1080/02643290442000310
- Gallivan, J. P., & Culham, J. C. (2015). Neural coding within human brain areas involved in actions. Current Opinion in Neurobiology, 33, 141–149. https://doi.org/10.1016/j.conb.2015.03.012
- Gilden, D. L., & Proffitt, D. R. (1994). Heuristic judgment of mass ratio in two-body collisions. Perception & Psychophysics, 56(6), 708–720. https://doi.org/10.3758/BF03208364
- Glenberg, A. M. (2015). Few believe the world is flat: How embodiment is changing the scientific understanding of cognition. Canadian Journal of Experimental Psychology/Revue Canadienne de Psychologie Expérimentale, 69(2), 165–171. https://doi.org/10.1037/cep0000056
- Goldenberg, G. (2014). Apraxia – the cognitive side of motor control. Cortex, 57, 270–274. https://doi.org/10.1016/j.cortex.2013.07.016
- Goldenberg, G., & Spatt, J. (2009). The neural basis of tool use. Brain, 132(6), 1645–1655. https://doi.org/10.1093/brain/awp080
- Gonzalez-Rothi, L., & Heilman, K. (1996). Liepmann (1900 and 1905): A definition of apraxia and a model of praxis. In C. Code, Y. Joanette, A. R. Lecours, & C.-W. Wallesch (Eds.), Classic cases in neuropsychology (pp. 118–128). Psychology Press.
- Goodale, M. A. (2011). Transforming vision into action. Vision Research, 51(13), 1567–1587. https://doi.org/10.1016/j.visres.2010.07.027
- Goodale, M. A., & Milner, A. D. (1992). Separate visual pathways for perception and action. Trends in Neurosciences, 15(1), 20–25. https://doi.org/10.1016/0166-2236(92)90344-8. https://www.ncbi.nlm.nih.gov/pubmed/1374953
- Goodale, M. A., Milner, A. D., Jakobson, L. S., & Carey, D. P. (1991). A neurological dissociation between perceiving objects and grasping them. Nature, 349(6305), 154–156. https://doi.org/10.1038/349154a0
- Gould, S., & Lewontin, R. (1979). The spandrels of San Marco and the Panglossian paradigm: A critique of the adaptationist programme. Proceedings of the Royal Society B: Biological Sciences, 205(1161), 581–598. https://doi.org/10.1098/rspb.1979.0086
- Hauk, O. (2016). Only time will tell – why temporal information is essential for our neuroscientific understanding of semantics. Psychonomic Bulletin & Review, 23(4), 1072–1079. https://doi.org/10.3758/s13423-015-0873-9
- Hauk, O., Johnsrude, I., & Pulvermuller, F. (2004). Somatotopic representation of action words in human motor and premotor cortex. Neuron, 41(2), 301–307. https://doi.org/10.1016/s0896-6273(03)00838-9
- Hetu, S., Gregoire, M., Saimpont, A., Coll, M. P., Eugene, F., Michon, P. E., & Jackson, P. L. (2013). The neural network of motor imagery: An ALE meta-analysis. Neuroscience & Biobehavioral Reviews, 37(5), 930–949. https://doi.org/10.1016/j.neubiorev.2013.03.017
- Hickok, G. (2009). Eight problems for the mirror neuron theory of action understanding in monkeys and humans. Journal of Cognitive Neuroscience, 21(7), 1229–1243. https://doi.org/10.1162/jocn.2009.21189
- Hickok, G., & Poeppel, D. (2007). The cortical organization of speech processing. Nature Reviews Neuroscience, 8(5), 393–402.
- Huber, L., Handwerker, D. A., Jangraw, D. C., Chen, G., Hall, A., Stüber, C., Gonzalez-Castillo, J., Ivanov, D., Marrett, S., Guidi, M., Goense, J., Poser, B. A., & Bandettini, P. A. (2017). High-resolution CBV-fMRI allows mapping of laminar activity and connectivity of cortical input and output in human M1. Neuron, 96(6), 1253–1263.e7. https://doi.org/10.1016/j.neuron.2017.11.005
- Krakauer, J. W., Ghazanfar, A. A., Gomez-Marin, A., MacIver, M. A., & Poeppel, D. (2017). Neuroscience needs behavior: Correcting a reductionist bias. Neuron, 93(3), 480–490. https://doi.org/10.1016/j.neuron.2016.12.041
- Kravitz, D. J., Saleem, K. S., Baker, C. I., Ungerleider, L. G., & Mishkin, M. (2013). The ventral visual pathway: An expanded neural framework for the processing of object quality. Trends in Cognitive Sciences, 17(1), 26–49. https://doi.org/10.1016/j.tics.2012.10.011
- Kristensen, S., Garcea, F. E., Mahon, B. Z., & Almeida, J. (2016). Temporal frequency tuning reveals interactions between the dorsal and ventral visual streams. Journal of Cognitive Neuroscience, 28(9), 1295–1302. https://doi.org/10.1162/jocn_a_00969
- Liberman, A., Cooper, F., Shankweiler, D., & Studdert-Kennedy, M. (1967). Perception of the speech code. Psychological Review, 74(6), 431–461. https://doi.org/10.1037/h0020279
- Livingstone, M., & Hubel, D. (1988). Segregation of form, color, movement, and depth: Anatomy, physiology, and perception. Science, 240(4853), 740–749. https://doi.org/10.1126/science.3283936. https://www.ncbi.nlm.nih.gov/pubmed/3283936
- Lotto, A. J., Hickok, G. S., & Holt, L. L. (2009). Reflections on mirror neurons and speech perception. Trends in Cognitive Sciences, 13(3), 110–114. https://doi.org/10.1016/j.tics.2008.11.008
- Ludwin-Peery, E., Bramley, N. R., Davis, E., & Gureckis, T. M. (2020). Broken physics: A conjunction-fallacy effect in intuitive physical reasoning. Psychological Science, 31(12), 1602–1611. https://doi.org/10.1177/0956797620957610
- Ludwin-Peery, E., Bramley, N. R., Davis, E., & Gureckis, T. M. (2021). Limits on simulation approaches in intuitive physics. Cognitive Psychology, 127, Article 101396. https://doi.org/10.1016/j.cogpsych.2021.101396
- Mahon, B. (2020). The representation of tools in the human brain. In D. Poeppel, G. R. Mangun, & M. S. Gazzaniga (Eds.), The New cognitive neurosciences (pp. 764–776). MIT Press.
- Mahon, B. (in press). Higher-order visual object representations: A functional analysis of their role in perception and action. In G. G. B. K. Haaland, B. Crosson, & T. King (Eds.), APA handbook of neuropsychology.
- Mahon, B., Milleville, S., Negri, G., Rumiati, R., Caramazza, A., & Martin, A. (2007). Action-related properties shape object representations in the ventral stream. Neuron, 55(3), 507–520. https://doi.org/10.1016/j.neuron.2007.07.011
- Mahon, B., & Wu, W. (2015). Cognitive penetration of the dorsal visual stream? In J. Zeimbekis & A. Raftopoulos (Eds.), The cognitive penetrability of perception: New philosophical perspectives (pp. 200–217). Oxford University Press.
- Mahon, B. Z. (2020). Brain mapping: Understanding the ins and outs of brain regions. Current Biology, 30(9), R414–R416. https://doi.org/10.1016/j.cub.2020.03.061
- Martin, A. (2016). GRAPES-Grounding representations in action, perception, and emotion systems: How object properties and categories are represented in the human brain. Psychonomic Bulletin & Review, 23(4), 979–990. https://doi.org/10.3758/s13423-015-0842-3
- Martin, A., & Weisberg, J. (2003). Neural foundations for understanding social and mechanical concepts. Cognitive Neuropsychology, 20(3–6), 575–587. https://doi.org/10.1080/02643290342000005
- McCloskey, M., Caramazza, A., & Green, B. (1980). Curvilinear motion in the absence of external forces: Naive beliefs about the motion of objects. Science, 210(4474), 1139–1141. https://doi.org/10.1126/science.210.4474.1139
- McCloskey, M., & Kohl, D. (1983). Naive physics: The curvilinear impetus principle and its role in interactions with moving objects. Journal of Experimental Psychology: Learning, Memory, and Cognition, 9(1), 146–156. https://doi.org/10.1037/0278-7393.9.1.146
- McCloskey, M., Washburn, A., & Felch, L. (1983). Intuitive physics: The straight-down belief and its origin. Journal of Experimental Psychology: Learning, Memory, and Cognition, 9(4), 636–649. https://doi.org/10.1037/0278-7393.9.4.636
- Merigan, W. H., & Maunsell, J. H. (1993). How parallel are the primate visual pathways? Annual Review of Neuroscience, 16(1), 369–402. https://doi.org/10.1146/annurev.ne.16.030193.002101
- Mitko, A., & Fischer, J. (2020). When it all falls down: The relationship between intuitive physics and spatial cognition. Cognitive Research: Principles & Implications, 5. https://doi.org/10.1186/s41235-020-00224-7
- Navarro-Cebrian, A., & Fischer, J. (2022). Precise functional connections from the dorsal anterior cingulate cortex to the intuitive physics network in the human brain. European Journal of Neuroscience. https://doi.org/10.1111/ejn.15670
- Negri, G. A., Rumiati, R. I., Zadini, A., Ukmar, M., Mahon, B. Z., & Caramazza, A. (2007). What is the role of motor simulation in action and object recognition? Evidence from apraxia. Cognitive Neuropsychology, 24(8), 795–816. https://doi.org/10.1080/02643290701707412
- Neupärtl, N., Tatai, F., & Rothkopf, C. (2022). Naturalistic embodied interactions elicit intuitive physical behavior in accordance with Newtonian physics. Cognitive Neuropsychology. https://doi.org/10.1080/02643294.2021.2008890
- Noppeney, U., Price, C. J., Penny, W. D., & Friston, K. J. (2006). Two distinct neural mechanisms for category-selective responses. Cerebral Cortex, 16(3), 437–445. https://doi.org/10.1093/cercor/bhi123
- Oberhuber, M., Hope, T. M. H., Seghier, M. L., Parker Jones, O., Prejawa, S., Green, D. W., & Price, C. J. (2016). Four functionally distinct regions in the left supramarginal gyrus support word processing. Cerebral Cortex, 26(11), 4212–4226. https://doi.org/10.1093/cercor/bhw251
- Pazzaglia, M., Pizzamiglio, L., Pes, E., & Aglioti, S. M. (2008). The sound of actions in apraxia. Current Biology, 18(22), 1766–1772. https://doi.org/10.1016/j.cub.2008.09.061
- Persichetti, A. S., Avery, J. A., Huber, L., Merriam, E. P., & Martin, A. (2020). Layer-specific contributions to imagined and executed hand movements in human primary motor cortex. Current Biology, 30(9), 1721–1725.e3. https://doi.org/10.1016/j.cub.2020.02.046
- Pisella, L., Binkofski, F., Lasek, K., Toni, I., & Rossetti, Y. (2006). No double-dissociation between optic ataxia and visual agnosia: Multiple sub-streams for multiple visuo-manual integrations. Neuropsychologia, 44(13), 2734–2748. https://doi.org/10.1016/j.neuropsychologia.2006.03.027
- Poeppel, D. (2012). The maps problem and the mapping problem: Two challenges for a cognitive neuroscience of speech and language. Cognitive Neuropsychology, 29(1–2), 34–55. https://doi.org/10.1080/02643294.2012.710600
- Poldrack, R. A. (2011). Inferring mental states from neuroimaging data: From reverse inference to large-scale decoding. Neuron, 72(5), 692–697. https://doi.org/10.1016/j.neuron.2011.11.001
- Pramod, R. T., Cohen, M. A., Tenenbaum, J. B., & Kanwisher, N. (2022). Invariant representation of physical stability in the human brain. Elife, 11. https://doi.org/10.7554/eLife.71736
- Pulvermuller, F. (2013). Semantic embodiment, disembodiment or misembodiment? In search of meaning in modules and neuron circuits. Brain and Language, 127(1), 86–103. https://doi.org/10.1016/j.bandl.2013.05.015
- Rizzolatti, G., & Fogassi, L. (2014). The mirror mechanism: Recent findings and perspectives. Philosophical Transactions of the Royal Society B: Biological Sciences, 369(1644), 20130420. https://doi.org/10.1098/rstb.2013.0420
- Rogalsky, C., Love, T., Driscoll, D., Anderson, S. W., & Hickok, G. (2011). Are mirror neurons the basis of speech perception? Evidence from five cases with damage to the purported human mirror system. Neurocase, 17(2), 178–187. https://doi.org/10.1080/13554794.2010.509318
- Rothi, L. J. G., Ochipa, C., & Heilman, K. M. (1991). A cognitive neuropsychological model of limb praxis. Cognitive Neuropsychology, 8(6), 443–458. https://doi.org/10.1080/02643299108253382. Go to ISI://A1991GW56100003
- Rumiati, R. I., Zanini, S., Vorano, L., & Shallice, T. (2001). A form of ideational apraxia as a delective deficit of contention scheduling. Cognitive Neuropsychology, 18(7), 617–642. https://doi.org/10.1080/02643290126375
- Schenk, T. (2006). An allocentric rather than perceptual deficit in patient D.F. Nature Neuroscience, 9(11), 1369–1370. https://doi.org/10.1038/nn1784
- Schneider, G. (1969). Two visual systems. Science, 163(3870), 895–902. https://doi.org/10.1126/science.163.3870.895
- Schwartz, D. L., & Black, T. (1999). Inferences through imagined actions: Knowing by simulated doing. Journal of Experimental Psychology: Learning, Memory, and Cognition, 25(1), 116–136. https://doi.org/10.1037/0278-7393.25.1.116
- Schwettmann, S., Tenenbaum, J. B., & Kanwisher, N. (2019). Invariant representations of mass in the human brain. Elife, 8. https://doi.org/10.7554/eLife.46619
- Smith, K., Battaglia, P., & Vul, E. (2013). Consistent physics underlying ballistic motion prediction. Proceedings of the 35th Conference of the Cognitive Science Society (pp. 3426–3431).
- Smith, K. A., Battaglia, P. W., & Vul, E. (2018). Different physical intuitions exist between tasks, not domains. Computational Brain and Behavior, 1–18.
- Stasenko, A., Bonn, C., Teghipco, A., Garcea, F. E., Sweet, C., Dombovy, M., McDonough, J., & Mahon, B. Z. (2015). A causal test of the motor theory of speech perception: A case of impaired speech production and spared speech perception. Cognitive Neuropsychology, 32(2), 38–57. https://doi.org/10.1080/02643294.2015.1035702
- Stasenko, A., Garcea, F. E., & Mahon, B. Z. (2013). What happens to the motor theory of perception when the motor system is damaged? Language and Cognition, 5(2–3), 225–238. https://doi.org/10.1515/langcog-2013-0016
- Titchener, E. B. (1901). Experimental psychology: A manual of laboratory practice, vol. I: Qualitative experiments. Macmillan.
- Ungerleider, L., & Mishkin, M. (1982). Two cortical visual systems. In D. J. Ingle, M. A. Goodale, & R. J. W. Mansfield (Eds.), Analysis of visual behavior (pp. 549–586). MIT Press.
- Washington, T., & Fischer, J. (2021). Intuitive physics does not rely on visual imagery. Journal of Vision, 21(9), 2894–2894. https://doi.org/10.1167/jov.21.9.2894
- Weisberg, J., van Turennout, M., & Martin, A. (2007). A neural system for learning about object function. Cerebral Cortex, 17(3), 513–521. https://doi.org/10.1093/cercor/bhj176
- Wurm, M. F., Caramazza, A., & Lingnau, A. (2017). Action categories in lateral occipitotemporal cortex are organized along sociality and transitivity. The Journal of Neuroscience, 37(3), 562–575. https://doi.org/10.1523/JNEUROSCI.1717-16.2016
- Xu, Y. (2018). A tale of two visual systems: Invariant and adaptive visual information representations in the primate brain. Annual Review of Vision Science, 4(1), 311–336. https://doi.org/10.1146/annurev-vision-091517-033954
- Yeatman, J. D., Weiner, K. S., Pestilli, F., Rokem, A., Mezer, A., & Wandell, B. A. (2014). The vertical occipital fasciculus: A century of controversy resolved by in vivo measurements. Proceedings of the National Academy of Sciences, 111(48), E5214–E5223. https://doi.org/10.1073/pnas.1418503111