ABSTRACT
Speaking involves the preparation of the linguistic content of an utterance and of the motor programs leading to articulation. The temporal dynamics of linguistic versus motor-speech (phonetic) encoding is highly debated: phonetic encoding has been associated either to the last quarter of an utterance preparation time (∼150ms before articulation), or to virtually the entire planning time, simultaneously with linguistic encoding. We (i) review the evidence on the time-course of motor-speech encoding based on EEG/MEG event-related (ERP) studies and (ii) strive to replicate the early effects of phonological-phonetic factors in referential word production by reanalysing a large EEG/ERP dataset. The review indicates that motor-speech encoding is engaged during at least the last 300ms preceding articulation (about half of a word planning lag). By contrast, the very early involvement of phonological-phonetic factors could be replicated only partially and is not as robust as in the second half of the utterance planning time-window.
Introduction
It takes several hundred milliseconds to plan and initiate an utterance that conveys the message the speaker wishes to express. Planning an utterance involves both linguistic (conceptual, lexical-semantic, syntactic, phonological) and motor speech encoding processes. Motor speech encoding refers to the transformation of a linguistic code into motor programs that guide articulation, a process that has been called in multiple ways in the different research fields: “phonetic encoding” (Levelt, Citation1989; Levelt et al., Citation1999), “motor phonological system” (Hickok, Citation2012), “higher order speech motor control” (Brendel et al., Citation2010), “speech motor planning” (Van der Merwe, Citation2008), “planning frame with speech sound maps’ (Guenther, Citation2002, Citation2006; see Parrell et al., Citation2019 for a comprehensive review). The different proposals stem from different frameworks, all seeking to explain how a linguistic input is transformed via cognitive-motor processes into motor commands, but they refer to different sets of postulated processes involved in the transformation of the linguistic code into articulated speech. Here we will use the general terms “motor speech encoding” and “phonetic encoding” synonymously and without distinction of the specific sub-processes involved in the transformation of the linguistic code into muscular commands. Motor speech encoding is thus part of utterance planning and is thought to contribute to the utterance preparation time. The amount of time necessary to encode motor speech programs and its dynamics relative to linguistic encoding is far from being clear and is currently debated. Actually, whereas some insight into the dynamics of language (linguistic) planning has been achieved in the last 20 years (see for instance de Zubicaray & Piai, Citation2019; Indefrey, Citation2011; Kemmerer, Citation2019; Munding et al., Citation2016; Nozari & Pinet, Citation2020), the time-course of motor speech planning is much less clear. Motor speech encoding has been attributed to very different time-windows of the utterance planning lag. In one view phonetic encoding is engaged in a small proportion of the utterance planning time, corresponding to the very final processes in utterance preparation (e.g., the last 150 ms representing about ¼ of the total utterance planning time in Indefrey, Citation2011). In an opposite view, motor speech planning has been claimed to be involved in virtually the whole utterance encoding time-period, concomitant with linguistic planning (Strijkers et al., Citation2017; Strijkers & Costa, Citation2011). In the following, we will first review the empirical evidence suggesting that motor speech encoding is engaged in a time-window that is larger than the last 150 ms preceding articulation. We will then address the view holding that phonetic encoding is involved very early in the utterance (word) planning process, simultaneously with lexical-semantic processes, and strive for a replication of the empirical evidence.
Review of the current evidence on the time-course of motor speech (phonetic) encoding
The time course of the planning processes involved in utterance production has been investigated extensively mainly with single word production tasks with electrophysiological (EEG) or magnetophysiological (MEG) event related potentials (ERP), as well as with electrocorticographic (ECoG) recordings. In the very influential meta-analysis estimating the time-course of word planning by Indefrey and Levelt (Citation2004; see also Indefrey, Citation2011), the largest proportion of the time taken to encode a word in referential production paradigms has been associated with linguistic (semantic, lexical, morpho-phonological) encoding, while phonetic encoding is engaged in the last 150 ms preceding articulation, which represent about a quarter of the total word planning time. The serial processes in the spatio-temporal decomposition of word encoding processes proposed by Indefrey and Levelt has been questioned in the framework of models integrating interactive or cascading activations within the language production system at the lexical-semantic and lexical-phonological levels (Dell, Citation1985, Citation1986), or between phonological and phonetic processes (Alderete et al., Citation2021; Goldrick et al., Citation2011). In particular, based on the results of EEG/MEG studies, some authors (Munding et al., Citation2016; Strijkers et al., Citation2017) proposed that linguistic and motor speech encoding processes are engaged in parallel rather than sequentially. These proposals also involve that motor speech encoding processes are involved earlier and take longer (virtually the whole utterance preparation time) than suggested in the serial models. We will come back to the issue of serial versus parallel activation of linguistic and phonetic processes in the final section. Before that, it seems important to clarify whether and how motor speech encoding can be targeted separately from linguistic encoding. Different approaches have been devised to specifically target motor speech planning, including seeking common activations between different elicitation modes, analyzing the time-course of speech (phonetic) errors and that of factors known to affect motor speech encoding. Each of these approaches will be reviewed below. The converge of results across different approaches will possibly represent the best approximation to the timing of motor speech encoding.
Immediate versus delayed production and other comparisons across tasks
Typical (immediate, overt) utterance production tasks are bound to involve all (linguistic and motor speech) encoding processes. To target motor speech encoding, delayed production tasks, i.e., the production of an utterance prompted by a cue that appears after a short delay, are usually used as they are thought to allow the preparation of at least the linguistic code, leaving only/mainly motor speech encoding processes to be executed after the delay. This assumption is based on the observation that linguistic effects (e.g., lexical frequency effects) that are observed in the immediate production disappear or decrease in the delayed production (Jescheniak & Levelt, Citation1994; Savage et al., Citation1990). It has also been argued that phonetic plans (motor speech plans) may also be prepared and kept in memory in standard delayed production tasks, leaving only motor speech programming/parametrization of muscle commands to be computed after the cue. To ensure that all motor speech encoding processes have to be prepared after the cue, the delay is sometimes filled with an articulatory suppression task (see rationale in Laganaro & Alario, Citation2006). Delayed production tasks have been used to target phonetic/motor speech preparation in behavioral (Kawamoto et al., Citation2008; Laganaro & Alario, Citation2006) and in neuroimaging studies (Bohland & Guenther, Citation2006; Chang et al., Citation2009; Herman et al., Citation2013; Tilsen et al., Citation2016) and the direct comparison between immediate and delayed production thus provides some insight into the time-window of motor speech encoding relative to linguistic encoding in conceptually driven utterance production. In a comparison of immediate and delayed picture naming, Laganaro and Perret (Citation2011) observed that EEG/ERP amplitudes and microstates diverge between these two conditions at about 300 ms after picture presentation, i.e., around less than half of the latencies in immediate production (about 700 ms in that study). The meta-analysis of MEG studies on single word production by Munding et al. (Citation2016), including both, immediate and delayed production tasks, identified activation of brain areas compatible with “motor functions” from about 300 ms after stimulus presentation (either pictures or words).
Hence, a very first conclusion from the comparison of immediate and delayed production points to a time-window of motor speech encoding processes that covers about half of the single word utterance planning lag. Converging evidence can also be found in studies comparing overt and silent/covert word production (Liljeström et al., Citation2015) or contrasting the production of the same words elicited with different production paradigms (Fargier & Laganaro, Citation2017). In the MEG study by Liljeström et al. (Citation2015), beta-band coherence increased in a brain network compatible with motor speech encoding (sensorimotor and premotor cortex) after 300 ms in overt relative to covert/silent word production, also suggesting that motor speech encoding is engaged in overt production around 300 ms. Finally, the production of the same words elicited with a referential (picture naming) and an inferential (naming from definition) task, thought to involve different linguistic encoding processes but similar motor speech encoding, yielded common EEG/ERP waveform amplitudes and microstates only in the 300 ms preceding the vocal onset (Fargier & Laganaro, Citation2017). While in the studies mentioned above the EEG or MEG signals have been analysed aligned to the eliciting stimuli, in the latter study the ERP signal has also been analysed aligned to the vocal onset (response-locked backwards), thus more clearly pointing to an engagement of motor speech encoding in the last 300 ms preceding articulation.
Phonemic-phonetic errors
In addition to the comparison across tasks, the analysis of the time-window underlying the production of phonemic-phonetic errors can also inform the time-course of phonological-phonetic encoding. Two studies conducted on phonemic errors elicited in neurotypical speakers reported that ERP waveform amplitudes diverge between correct and erroneous production around 350–370 ms following the presentation of the eliciting stimuli (written words in Möller et al., Citation2007; pictures in Monaco et al., Citation2017). Given the tasks used in those studies (Spoonerisms of Laboratory Induced Predisposition and tongue-twisters respectively), these results are interpreted as the-time-window of conflict during phonetic encoding, which again corresponds to approximately the half of the mean utterance encoding time. The same rationale can be applied to the results of a study comparing EEG/ERPs between left-hemisphere stroke patients producing phonemic and phonetic errors and neurotypical controls, yielding diverging amplitudes and microstates from around 380–400 ms following stimulus onset and lasting until the vocal onset in the response-locked analysis (Laganaro et al., Citation2013). Hence, studies on errors in neurotypical speakers and in brain-damaged speakers also point to phonetic encoding being involved in the last half time-window of utterance planning.
Factors affecting motor speech encoding
Finally, a last approach to the time-window of motor speech encoding consists in tracking the temporal dynamics of specific factors related to the speech stimuli. The main factors that have been associated to motor speech encoding relate to the frequency of speech sequences (syllables in Bürki et al., Citation2015; Citation2020; biphones in Fairs et al., Citation2021), to their length (Miozzo et al., Citation2015) or to the phonemic properties of the word onset phonemes (Strijkers et al., Citation2017). Two ERP studies manipulating the frequency of use of the produced syllables have reported that ERPs diverge between the production of frequent and infrequent speech sequences in the last 170–150 ms (Bürki et al., Citation2015) or in the last 350–300 ms (Bürki et al., Citation2020) preceding the vocal onset. The latter result converges with the results of the studies from the other approaches summarized in the two previous sections, although with different types of speech stimuli (pseudo-words). By contrast, other investigations that manipulated speech-related factors in picture naming tasks reported effects on the EEG/MEG signals in a much earlier time-window of the word planning process: biphone frequency effects have been reported starting at 74–145 ms in the study by Fairs et al. (Citation2021), length effects around 150 ms in Miozzo et al. (Citation2015) and effects of place of articulation of the first phoneme (lip versus tongue) in the 160–240 ms in Strijkers et al. (Citation2017). These results rather suggest that motor speech encoding is engaged very early in utterance planning, meaning that linguistic and motor speech processes are engaged quasi simultaneously. The observation that effects of lexical-semantic and phonological/ phonetic factors in picture naming tasks emerged in the same early time window, around 150–200 ms after the presentation of the picture (Fairs et al., Citation2021; Miozzo et al., Citation2015; Strijkers et al., Citation2010, Citation2017), is interpreted against serial and sequential encoding processes and rather favors interactive or cascading activation of lexical-semantic and phonological-phonetic representations. While interactive word encoding processing is also suggested in a study reporting concurrent activation of various left hemisphere areas during word production with intracranial electro-corticographic (ECoG) recordings (Conner et al., Citation2019), results favoring rather limited temporal overlap between regions have also been reported with intracranial EEG (Dubarry et al., Citation2017).
In sum, whereas the evidence reviewed in the two previous sections on the comparison of word production across tasks and on phonemic-phonetic errors suggest that motor speech encoding is involved in a time-window preceding the vocal onset, corresponding to approximately half of the utterance planning time, several results on the effects of phonological/phonetic factors on the EEG/MEG signals rather suggest that speech encoding is engaged much earlier, simultaneously with linguistic encoding processes. Before any further discussion of this issue, in the next section we will try to replicate the results of the early EEG/ERP modulation by speech-related factors, that have been reported by some authors.
(Re)-analyses of EEG/ERP data to strive for a replication of the early effect of phonological-phonetic factors in picture naming
To replicate the effects reported by Strijkers et al. (Citation2017) and Fairs et al. (Citation2021) on the simultaneous or quasi-simultaneous effects of lexical and phonemic-phonetic properties of the words in picture naming tasks, we conducted new analyses on a large EEG/ERP dataset available from previous studies.
Dataset
Te data from three studies (all the participants from Valente et al., Citation2014, and the adult groups from Atanasova et al., Citation2020 and from Krethlow et al., submitted) in which the participants performed the exact same picture naming task procedure with the same 120 stimuli under the same EEG recording procedure (128 electrodes with the Active-Two Biosemi EEG system, acquired at 512 Hz in the same lab) was combined. The total number of participants (66, mean age: 29.1, 19 males) allowed us to reach a minimum of 45 artefact free epochs per item/word (see below).
The EEG/ERP data was analyzed by contrasting the effects of lexical frequency to those of phonological-phonetic factors as done in previous studies by Strijkers et al and Fairs et al. Note that although an orthogonal design is described in the material section in those two previous studies, the EEG/ERP analyses were carried out separately for lexical and phonological-phonetic factors; here we therefore used three subsets of stimuli, one for each factor (one lexical factor—lexical frequency—and two phonological-phonetic factors: length and onset phoneme properties), and controlled all the pertinent (psycho-)linguistic factors through careful matching across conditions. Each subset was composed of 18 items per condition extracted from the original 120 items. The lexical subset contained 18 high- and 18 low-frequency words; the length subset contained 18 monosyllabic (short) and 18 three-syllabic (long) words and the onset phoneme set contained 18 labial unvoiced, 18 labial voiced and 18 velar word onset phonemes. In each subset, words were matched across conditions on all the psycholinguistic factors known to affect word production in picture naming tasks (Perret & Bonin, Citation2019). The values were extracted from the original databases from which the stimuli were issued (Alario & Ferrand, Citation1999; Bonin et al., Citation2003) or newly extracted from the updated French database Lexique (New et al., Citation2004). The psycholinguistic properties of each of the three subsets of stimuli are presented in .
Table 1. Psycholinguistic properties (mean and SD) of the stimuli in the three subsets contrasting lexical frequency, length and onset phoneme properties and latencies (in ms) for the analysed dataset.
Pre-processing and analyses
All epochs corresponding to correct response and artefact-free EEG signal (visually checked using Cartool, Brunet et al., Citation2011) were extracted aligned to the picture onset (stimulus-locked, 500 ms) and to 100 ms before the vocal onset (response-locked, from −600 to −100 ms before RTs), filtered 0.2–30 Hz. Bad channels were interpolated at single trial/epoch level with a 3-D spline interpolation (Perrin et al., Citation1987). For the factorial analysis, ERPs epochs were averaged per item across participants. The minimum of artefact-free epochs per item across the 66 participants was 45.
It was not straightforward to determine temporal time-windows or regions of interest (ROIs) based on previous literature as they differed across studiesFootnote1 (see also Pernet et al., Citation2020 for issues and recommendations on EEG/MEG analysed based on ROIs); waveform amplitudes were thus compared across conditions using massed approaches on each electrode and each time point. Two different approaches were used: a less conservative approach applying time point by time-point t-tests with limited correction for multiple tests (alpha set to .01 on a minimum of 10 consecutive time-points), and cluster-mass statistics with a better control for Type 1 family-wise errors. The cluster-mass statistics were run with the permuco4brain R package by Frossard and Renaud (Citation2021; Citation2022) based on 5000 permutations (alpha set to 0.05).
The second analysis was run on the global field power (GFP) of single trials/epochs, based on the time-windows yielding different amplitudes across conditions in the previous analysis. The exact time-periods for the GFP analyses were determined with a spatio-temporal segmentation dividing the ERP signal into periods of stable or quasi-stable global electric field at scalp. The map templates that best explained the averaged ERP signal were fitted in each single trial/epoch (following the standard procedure described Brunet et al., Citation2011; Michel & Koenig, Citation2018). The mean GFP of each segment was used as dependent variables in mixed model analyses with participants and items as random structure and each of the conditions of interest as fixed factors. Three separate models were run on each map/time-window, one on each set of items (frequency, length and first phoneme property) with alpha set to .01 due to the multiple models on the same data.
Results
All ERP results are presented in . On stimulus-locked ERPs, the less conservative statistical approach indicates different amplitudes between high- and low-frequency words in a time-window between 190 and 210 ms after picture onset on central and posterior left electrodes (see , A.a.). Amplitudes also differ around 240 ms across onset phonemes on clusters of central anterior and right posterior electrodes for labial unvoiced versus voiced onsets and on a few sparse electrodes for the labial versus velar contrast (, C.a). Word length did not affect the stimulus-locked amplitudes and neither frequency nor onset phoneme effects survived the cluster-mass approach (see Figure1, A.b. and C.b.). In the response-locked signal, length and first phoneme properties modulate amplitudes from around 250–230 ms before the vocal onset (, B.a. and C.a.), a result that is confirmed with the cluster-mass approach for both, the length and the first phoneme contrasts (, B.b. and C.b.).
Figure 1. Results of the ERP analyses on each of the three data sub-sets. A. High versus low lexical frequency. a. Exemplar waveforms on Fz, Cz and POz for each condition in stimulus-locked ERPs in the left hand-side and response-locked on the right. The pink bar represents the time-period of significant difference between conditions in the (uncorrected) t-test analysis run on each electrode and time-point; on top, all the electrodes yielding the difference within this time-window are highlighted in pink. b. Results of the ERP cluster mass waveform analyses on the stimulus-locked ERPs on electrodes (Y axes) and time points (X axes) for high and low lexical frequency. The analysis did not display any period of significant p values (in white and gray, p ≥ 0.05). B. Monosyllabic (short) versus three-syllabic (long) words. a. Exemplar waveforms on Fz, Cz and POz for each condition in stimulus-locked ERPs in the left hand-side and response-locked on the right. The pink bar represents the time-period of significant difference between conditions in the (uncorrected) t-test analysis run on each electrode and time-point; on top, all the electrodes yielding the difference within this time-window are highlighted in pink. b. Results of the ERP cluster mass waveform analyses on electrodes (Y axes) and time points (X axes) on the stimulus-locked and response-locked ERPs (in red p < 0.01 and in yellow p < 0.05, in white and gray, p ≥ 0.05). C. First phoneme properties: unvoiced labial, voiced labial and velar. a. Exemplar waveforms on Fz, Cz and POz for each condition in stimulus-locked ERPs in the left hand-side and response-locked on the right. The pink bar represents the time-periods of significant difference between conditions in the (uncorrected) t-test analysis run on each electrode and time-point; on top, all the electrodes yielding the difference within this time-window are highlighted in pink (the different distributions represent different time-windows and contrasts). b. Results of the ERP cluster mass waveform analyses on electrodes (Y axes) and time points (X axes) on the stimulus-locked and response-locked ERPs for each contrast of interest (in red p < 0.01 and in yellow p < 0.05, in white and gray, p ≥ 0.05). D. Results of the ERP microstate (spatio-temporal segmentation) on stimulus-locked ERPs in the left hand-side and response-locked ERPs on the right with time-periods corresponding to each map summarized under the mean GFP of the averaged data across all epochs. The corresponding map templates (I to VIII) are displayed on top. The table presents the mean GFP (and SD) for the maps observed in the time-windows yielding significant differences across conditions in the results on waveform amplitudes (.A, B and C) and summarizes the effects on GFP of the three (psycho-)linguistic factors in the single trial analyses. * In italics: results of the analyses run with all 120 items and all continuous psycholinguistic factors (see Footnote 2).
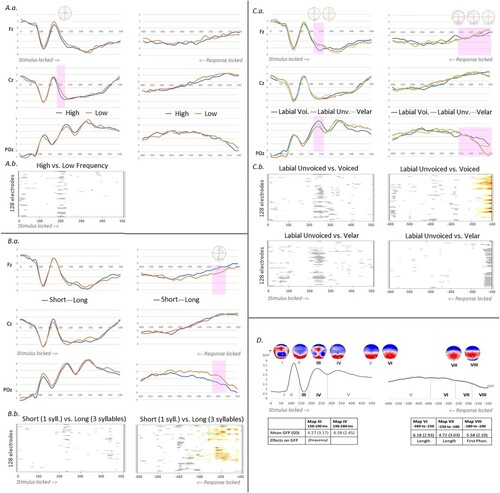
The eight map templates issued from the spatio-temporal segmentation of the stimulus-locked and the response-locked ERP average signal are shown in .D. along with their time-period illustrated on the mean GFP of stimulus-locked and response-locked ERPs. The five template maps corresponding to the time-periods that have shown significant results in one or more contrasts in the analyses on waveform amplitudes (whether corrected or not) were fitted in all single epochs (N = 4,516), namely Maps III and IV in the stimulus-locked ERPs and Maps VI, VII and VIII in the response-locked signals. The mean GFP of each segment in each single epoch was the dependent variable in the mixed models (one for each map, formula: MeanGFP[Map] ∼ [Factor] + (1 | Subject) + (1 | Item)).
In the stimulus-locked ERPs there were no significant effects of the three factors on the GFP of the microstates III or IV (the first phoneme contrast between velar and labial unvoiced was the closest to significance on Period-Map IV : t(51.2) = −2.18, p = .034, and frequency did not reach significance on the GFP of Map III: t(31.8) = 1.3, p = .19, all other ts < 1).Footnote2
In the response-locked ERPs, length modulated the GFP of Maps VI and VII (respectively t(39.4) = 2.74, Estimate = 0.36, SE = 0.13, p = .009 and t(37.4) = 2.76, Estimate = 0.37, SE = 0.135, p = .009), with higher GFP for three-syllabic words. The first phoneme properties modulated the GFP of Map VIII, with lower GFP for Labial voiced and Velar as compared to Labial Unvoiced (respectively t(51.7) = −4.39, Estimate = −0.383, SE = 0.09, p < .0001; t(49.8) = −3.07, Estimate = −0.267, SE = 0.09, p = .003).
Discussion
The analyses carried out on the picture naming ERP data from 66 participants were aimed at replicating the early effects of phonemic-phonetic factors on the ERP signal reported in some previous studies on picture naming tasks. A factorial design was used as in Strijkers et al. (Citation2017) and Fairs et al. (Citation2021), with three sub-sets of stimuli corresponding to a lexical factor (lexical frequency) and to two phonemic factors (length in syllables and properties of the word onset phoneme). With the less conservative statistical analyses, early modulation of ERPs by the properties of the first phoneme is observed, with amplitudes differing around 240 ms between labial unvoiced and labial voiced onset phonemes and between labial and velar onset phonemes. ERP amplitudes were also modulated by lexical frequency in a slightly earlier time-window (190–210 ms). At first sight these results replicate the ones reported by Strijkers et al. (Citation2017), who found effects of both lexical frequency and place of articulation of the first phoneme (lip versus tongue) in the 160–240 ms time-window following picture onset. However, these early effects are no longer observed when more conservative statistical approaches that follow current recommendations on the massed analyses of high density EEG or MEG data are applied (Maris & Oostenveld, Citation2007; Pernet et al., Citation2020), nor when the GFP of single trials is analysed with mixed-models. On the contrary, the effects of word length and of the properties of the first phoneme are very consistent across all analyses in the response-locked ERPs: word length (one versus three syllabic words) systematically modulates ERPs in the 250 ms preceding the vocal onset and the effect of the first phoneme properties is consistent across the analyses in the last 180 ms.
We will further discuss the present results in the general discussion by integrating them with the literature review carried out in the previous sections.
General discussion
The review of the empirical evidence on the time-course of motor speech encoding based on EEG/MEG ERP results included studies using three different approaches: (i) comparison of different speech elicitation tasks, (ii) investigation of the temporal-dynamics associated with the production of phonemic-phonetic errors in neurotypical and brain damaged speakers and (iii) time-window of ERP modulation by phonemic-phonetic factors associated to the produced utterances. Altogether the results of the first two approaches point to a time-window of motor speech encoding covering the last 300 ms preceding the vocal onset. This time-window is larger than the 150 ms attributed to motor speech encoding in the model by Indefrey (Citation2011), and it rather corresponds to approximately half of the utterance encoding time. The results of studies carried out on the effects of factors related to motor speech encoding (frequency of speech sequences or phonemic properties of the onset phoneme) do not contradict the main conclusion that phonetic encoding is engaged during a large portion of the utterance planning time, but some results rather suggest that speech encoding is involved in virtually the entire utterance preparation lag. In such proposals, language and speech encoding processes run simultaneously from the very beginning of the utterance planning time, i.e., from around 150–200 ms in picture naming tasks (Miozzo et al., Citation2015; Strijkers et al., Citation2010, Citation2017, and even earlier in Fairs et al., Citation2021). Here we strived to replicate those results on the simultaneous early engagement of lexical and phonological-phonetic factors by re-analyzing a large ERP dataset with the lexical and phonemic-phonetic contrasts used in previous studies. The present results indeed replicate the quasi-simultaneous modulations of ERP amplitudes by lexical frequency and by first phoneme properties (but not by word length) in early time-windows (respectively 190–210 ms and around 240 ms following picture presentation in the picture naming task). However, those effects are observed only with the less conservative statistical approaches, i.e., with weak control of family-wise type I error. These early effects disappear when cluster analyses are applied to control the family-wise error rate in massive tests. In particular, the properties of the word onset phoneme consistently modulate ERPs across analyses only in the signal locked to the vocal onset. As for the word length effect, it is only observed in the response-locked signal across all analyses, meaning that the early effect reported around 150 ms in the MEG study by Miozzo et al. (Citation2015), although with a composite measure of word length and neighborhood density, is not replicated here.
The issue then is whether the present results on phonemic properties of the word onset do represent a replication of the previously reported early engagement of motor speech encoding; in other words, do the results obtained with uncorrected massed analyses add evidence in favor of early concurrent activation of lexical-semantic and phonological-phonetic processes ? A closer look into previous results highlights that the “early” effects of phonological-phonetic factors vary up to 100 ms across studies using the same picture naming paradigm (around or before 150 ms in Fairs et al., Citation2021 and in Miozzo et al., Citation2015; in the time-period 160–240 ms in Strijkers et al., Citation2017). These variations may be partly attributed to a different operationalization of the phonological-phonetic factors across the studies : the time-period of the effect of the onset phonemic properties observed here around 240 ms corresponds to the end of the 160–240 ms time-window reported with a similar manipulation by Strijkers et al., but is about 100 ms later than the effects on other phonological-phonetic factors in Miozzo et al. (Citation2015) and Fairs et al. (Citation2021). The time-periods seem to converge better across studies for lexical frequency effects: 190- 210 ms after picture onset on amplitudes (and between 150 and 190 ms on the GFP with frequency as continuous variable on all the 120 items) in the present results, also corresponding to the time-period of lexical frequency effect in the MEG study by Strijkers et al. (Citation2017) and in the EEG study by Strijkers et al. (Citation2010), although not on the same electrodes. It should be noticed however that important variations have also been reported for the time-window of lexical frequency effects, for instance up to 500 ms later in the ECoG study by Conner et al. (Citation2019).
Hence, if the quasi-simultaneous early lexical and phonological-phonetic effects observed with the less conservative statistical approach was to be taken as a replication of previous results favoring early concurrent or quasi concurrent activation or ignition of all processes/representations involved in utterance production (Strijkers & Costa, Citation2011), there are still important incongruences across studies on the absolute and relative timing that merits further investigation. Part of those incongruencies may be due to the fact that most studies computed only stimulus-locked analyses on data averaged across trials that have very variable processing times. When analyses are carried out on single trials (Dubarry et al., Citation2017), very limited temporal overlap between active brain regions has been observed, although it was not clearly serial either.
Overall, the review of the results from the different approaches along with the results of the analyses presented here strongly converge towards a major engagement of motor speech encoding in the last 300–200 ms preceding utterance production. Although this may seem a quite long motor speech computation time, it is consistent with production latencies in delayed production tasks (usually longer than 400 ms), in which the linguistic code of the utterance can be prepared in advance, and only motor speech planning and/or programming have to be completed. It is also in line with the report of consistent activation of frontal and sensorimotor brain regions from around 300 ms in picture naming tasks (Ala-Salomäki et al., Citation2021), and in particular with the involvement of the precentral gyrus from about 320 ms (Hassan et al., Citation2015), a region involved in motor speech encoding (Guenther, Citation2016; see also Silva et al., Citation2022 for a recent review on the precentral gyrus).
Conclusion
The literature review on the time-course of motor speech encoding points to a rather long involvement of motor speech planning in the overall utterance planning process. Motor speech encoding being engaged in about half of the utterance planning time still leaves place for at least partial sequential linguistic and motor speech encoding, as linguistic effects have been reported mostly in earlier time-windows. There is however also some evidence of much earlier involvement of factors related to motor speech encoding that supports interactive or cascading dynamics rather than strictly sequential/successive activation of linguistic and speech encoding processes. Here the early involvement of phonemic-phonetic factors in utterance planning could be replicated only partially, and those early effects seem less robust than the results in the time-window preceding speech articulation, an issue that merits further investigation.
Disclosure statement
No potential conflict of interest was reported by the author(s).
Additional information
Funding
Notes
1 The analysed time-windows (TW) in previous studies were: 74–145 ms, 186–287 ms, and 316–369 ms in Fairs et al. (Citation2021) and 100–160 ms, 160–240 ms, 260–340 ms in Strijkers et al. (Citation2017). The peak and TW of concurrent lexical-semantic and phonological-phonetic activation also differ across studies: 150 ms in Miozzo et al. (Citation2015), 160–240 ms in Strijkers et al. (Citation2017), 186–287 ms in Fairs et al. (Citation2021).
2 An analysis run on the mean GFP of each time-period/map on the entire set of 120 items with all the continuous psycholinguistic factors (Image Complexity, Name Agreement, Lexical Frequency, Length in syllables, Word Age of Acquisition, First Phoneme Sonority) yielded an effect of frequency on Period-Map III (t(104) = −3.32, Estimate = −.003, SE = −.0009, p = .001), of Complexity on Map IV (t(115) = 3.32, p = .001 and of Age of Acquisition on Map V (t(115) = −4.58, p < .001).
References
- Alario, F., & Ferrand, L. (1999). A set of 400 pictures standardized for French: Norms for name agreement, image agreement, familiarity, visual complexity, image variability, and age of acquisition. Behavior Research Methods, Instruments, & Computers, 31(3), 531–552. https://doi.org/10.3758/BF03200732
- Ala-Salomäki, H., Kujala, J., Liljeström, M., & Salmelin, R. (2021). Picture naming yields highly consistent cortical activation patterns: Test–retest reliability of magnetoencephalography recordings. NeuroImage, 227, 117651. https://doi.org/10.1016/j.neuroimage.2020.117651
- Alderete, J., Baese-Berk, M., Leung, K., & Goldrick, M. (2021). Cascading activation in phonological planning and articulation: Evidence from spontaneous speech errors. Cognition, 210, 104577. https://doi.org/10.1016/j.cognition.2020.104577
- Atanasova, T., Fargier, R., Zesiger, P., & Laganaro, M. (2020). Dynamics of word production in the transition from adolescence toAdulthood. Neurobiology of Language, 2(1), 1–21. https://doi.org/10.1162/nol_a_00024
- Bohland, J. W., & Guenther, F. H. (2006). An fMRI investigation of syllable sequence production. NeuroImage, 32(2), 821–841. https://doi.org/10.1016/j.neuroimage.2006.04.173
- Bonin, P., Peereman, R., Malardier, N., Méot, A., & Chalard, M. (2003). A new set of 299 pictures for psycholinguistic studies: French norms for name agreement, image agreement, conceptual familiarity, visual complexity, image variability, age of acquisition, and naming latencies. Behavior Research Methods, Instruments, & Computers, 35(1), 158–167. https://doi.org/10.3758/BF03195507
- Brendel, B., Hertrich, I., Erb, M., Lindner, A., Riecker, A., Grodd, W., & Ackermann, H. (2010). The contribution of mesiofrontal cortex to the preparation and execution of repetitive syllable productions: An fMRI study. Neuroimage, 50(3), 1219–1230. https://doi.org/10.1016/j.neuroimage.2010.01.039
- Brunet, D., Murray, M. M., & Michel, C. M. (2011). Spatiotemporal analysis of multichannel EEG: CARTOOL. Computational Intelligence and Neuroscience, 2011.
- Bürki, A., Pellet, P., & Laganaro, M. (2015). Do speakers have access to a mental syllabary? ERP comparison of high frequency and novel syllable production. Brain and Language, 150, 90–102. https://doi.org/10.1016/j.bandl.2015.08.006
- Bürki, A., Viebahn, M., & Gafos, A. (2020). Plasticity and transfer in the sound system: exposure to syllables in production or perception changes their subsequent production. Language, Cognition and Neuroscience, 35(10), 1371–1393. https://doi.org/10.1080/23273798.2020.1782445
- Chang, S.-E., Kenney, M. K., Loucks, T. M. J., Poletto, C. J., & Ludlow, C. L. (2009). Common neural substrates support speech and non-speech vocal tract gestures. NeuroImage, 47(1), 314–325. https://doi.org/10.1016/j.neuroimage.2009.03.032
- Conner, C. R., Kadipasaoglu, C. M., Shouval, H. Z., Hickok, G., & Tandon, N. (2019). Network dynamics of Broca’s area during word selection. PLoS One, 14(12), e0225756. https://doi.org/10.1371/journal.pone.0225756
- Dell, G. S. (1985). Positive feedback in hierarchical connectionistmodels: Application to language production. Cognitive Science, 9, 3–23.
- Dell, G. S. (1986). A spreading-activation theory of retrieval in sentence production. Psychological Review, 93(3), 283–321. https://doi.org/10.1037/0033-295X.93.3.283
- de Zubicaray, G. I., & Piai, V. (2019). Investigating the spatial and temporal components of speech production. In The Oxford handbook of neurolinguistics (pp. 471–497). Oxford University Press.
- Dubarry, A. S., Llorens, A., Trébuchon, A., Carron, R., Liégeois-Chauvel, C., Bénar, C. G., & Alario, F. X. (2017). Estimating parallel processing in a language task using single-trial intracerebral electroencephalography. Psychological Science, 28(4), 414–426. https://doi.org/10.1177/0956797616681296
- Fairs, A., Michelas, A., Dufour, S., & Strijkers, K. (2021). The same ultra-rapid parallel brain dynamics underpin the production and perception of speech. Cerebral Cortex Communications, 2(3), tgab040. https://doi.org/10.1093/texcom/tgab040
- Fargier, R., & Laganaro, M. (2017). Spatio-temporal dynamics of referential and inferential naming: Different brain and cognitive operations to lexical selection. Brain Topography, 30(2), 182–197. https://doi.org/10.1007/s10548-016-0504-4
- Frossard, J., & Renaud, O. (2021). Permutation tests for regression, ANOVA, and comparison of signals: the permuco package. Journal of Statistical Software, 99(15), 1–32. https://doi.org/10.18637/jss.v099.i15
- Frossard, J., & Renaud, O. (2022). The cluster depth tests: Toward point-wise strong control of the family-wise error rate in massively univariate tests with application to M/EEG. NeuroImage, 247, 118824. https://doi.org/10.1016/j.neuroimage.2021.118824
- Goldrick, M., Baker, H. R., Murphy, A., & Baese-Berk, M. (2011). Interaction and representational integration: Evidence from speech errors. Cognition, 121(1), 58–72. https://doi.org/10.1016/j.cognition.2011.05.006
- Guenther, F. H. (2002). Neural control of speech movements. In A. Meyer, & N. Schiller (Eds.), Phonetics and phonology in language comprehension and production: Differences and similarities (pp. 209–240). Mouton de Gruyter.
- Guenther, F. H. (2016). Neural control of speech. Mit Press.
- Guenther, F. H., Ghosh, S. S., & Tourville, J. A. (2006). Neural modeling and imaging of the cortical interactions underlying syllable production. Brain and Language, 96(3), 280–301. https://doi.org/10.1016/j.bandl.2005.06.001
- Hassan, M., Benquet, P., Biraben, A., Berrou, C., Dufor, O., & Wendling, F. (2015). Dynamic reorganization of functional brain networks during picture naming. Cortex, 73, 276–288. https://doi.org/10.1016/j.cortex.2015.08.019
- Herman, A. B., Houde, J. F., Vinogradov, S., & Nagarajan, S. S. (2013). Parsing the phonological loop: Activation timing in the dorsal speech stream determines accuracy in speech reproduction. The Journal of Neuroscience, 33(13), 5439–5453. https://doi.org/10.1523/JNEUROSCI.1472-12.2013
- Hickok, G. (2012). Computational neuroanatomy of speech production. Nature Reviews Neuroscience, 13(2), 135–145. https://doi.org/10.1038/nrn3158
- Indefrey, P. (2011). The spatial and temporal signatures of word production components: A critical update. Language Sciences, 2, 255. http://doi.org/10.3389/fpsyg.2011.00255
- Indefrey, P., & Levelt, W. J. M. (2004). The spatial and temporal signatures of word production components. Cognition, 92(1-2), 101–144. https://doi.org/10.1016/j.cognition.2002.06.001
- Jescheniak, J. D., & Levelt, W. J. (1994). Word frequency effects in speech production: Retrieval of syntactic information and of phonological form. Journal of Experimental Psychology: Learning, Memory, and Cognition, 20(4), 824–843. https://doi.org/10.1037/0278-7393.20.4.824
- Kawamoto, A. H., Liu, Q., Mura, K., & Sanchez, A. (2008). Articulatory preparation in the delayed naming task. Journal of Memory and Language, 58(2), 347–365. https://doi.org/10.1016/j.jml.2007.06.002
- Kemmerer, D. (2019). From blueprints to brain maps: The status of the Lemma Model in cognitive neuroscience. Language, Cognition and Neuroscience, 34(9), 1085–1116. https://doi.org/10.1080/23273798.2018.1537498
- Laganaro, M., & Alario, F. X. (2006). On the locus of the syllable frequency effect in speech production. Journal of Memory and Language, 55(2), 178–196. https://doi.org/10.1016/j.jml.2006.05.001
- Laganaro, M., & Perret, C. (2011). Comparing electrophysiological correlates of word production in immediate and delayed naming through the analysis of word age of acquisition effects. Brain Topography, 24(1), 19–29. https://doi.org/10.1007/s10548-010-0162-x
- Laganaro, M., Python, G., & Toepel, U. (2013). Dynamics of phonological-phonetic encoding in word production: Evidence from diverging ERPs between stroke patients and controls. Brain and Language, 126(2), 123–132. https://doi.org/10.1016/j.bandl.2013.03.004
- Levelt, W. (1989). Speaking: From intention to articulation. MIT Press.
- Levelt, W. J. M., Roelofs, A., & Meyer, A. S. (1999). A theory of lexical access in speech production. Behavioral and Brain Sciences, 22, 1–75.
- Liljeström, M., Kujala, J., Stevenson, C., & Salmelin, R. (2015). Dynamic reconfiguration of the language network preceding onset of speech in picture naming. Human Brain Mapping, 36(3), 1202–1216. https://doi.org/10.1002/hbm.22697
- Maris, E., & Oostenveld, R. (2007). Nonparametric statistical testing of EEG- and MEG-data. Journal of Neuroscience Methods, 164(1), 177–190. https://doi.org/10.1016/j.jneumeth.2007.03.024
- Michel, C. M., & Koenig, T. (2018). EEG microstates as a tool for studying the temporal dynamics of whole-brain neuronal networks: A review. Neuroimage, 180, 577–593. https://doi.org/10.1016/j.neuroimage.2017.11.062
- Miozzo, M., Pulvermuller, F., & Hauk, O. (2015). Early parallel activation of semantics and phonology in picture naming: Evidence from a multiple linear regression MEG study. Cerebral Cortex, 25(10), 3343–3355. https://doi.org/10.1093/cercor/bhu137
- Möller, J., Jansma, B. M., Rodriguez-Fornells, A., & Münte, T. F. (2007). What the brain does before the tongue slips. Cerebral Cortex, 17(5), 1173–1178. https://doi.org/10.1093/cercor/bhl028
- Monaco, E., Pellet, P., & Laganaro, M. (2017). Facilitation and interference of phoneme repetition and phoneme similarity in speech production. Language, Cognition and Neuroscience, 32(5), 650–660. https://doi.org/10.1080/23273798.2016.1257730
- Munding, D., Dubarry, A.-S., & Alario, F.-X. (2016). On the cortical dynamics of word production: A review of the MEG evidence. Language, Cognition and Neuroscience, 31(4), 441–462. https://doi.org/10.1080/23273798.2015.1071857
- New, B., Pallier, C., Brysbaert, M., & Ferrand, L. (2004). Lexique 2 : A new French lexical database. Behavior Research Methods, Instruments, & Computers, 36(3), 516–524. https://doi.org/10.3758/BF03195598
- Nozari, N., & Pinet, S. (2020). A critical review of the behavioral, neuroimaging, and electrophysiological studies of co-activation of representations during word production. Journal of Neurolinguistics, 53, 100875. https://doi.org/10.1016/j.jneuroling.2019.100875
- Parrell, B., Lammert, A. C., Ciccarelli, G., & Quatieri, T. F. (2019). Current models of speech motor control: A control-theoretic overview of architectures and properties. The Journal of the Acoustical Society of America, 145(3), 1456–1481. https://doi.org/10.1121/1.5092807
- Pernet, C., Garrido, M. I., Gramfort, A., Maurits, N., Michel, C. M., Pang, E., … Puce, A. (2020). Issues and recommendations from the OHBM COBIDAS MEEG committee for reproducible EEG and MEG research. Nature Neuroscience, 23(12), 1473–1483. https://doi.org/10.1038/s41593-020-00709-0
- Perret, C., & Bonin, P. (2019). Which variables should be controlled for to investigate picture naming in adults? A Bayesian meta-analysis. Behavior Research Methods, 51(6), 2533–2545. https://doi.org/10.3758/s13428-018-1100-1
- Perrin, F., Pernier, J., Bertnard, O., Giard, M.-H., & Echallier, J. (1987). Mapping of scalp potentials by surface spline interpolation. Electroencephalography and Clinical Neurophysiology, 66(1), 75–81. https://doi.org/10.1016/0013-4694(87)90141-6
- Savage, G. R., Bradley, D. C., & Forster, K. I. (1990). Word frequency and the pronunciation task: The contribution of articulatory fluency. Language and Cognitive Processes, 5(3), 203–236. https://doi.org/10.1080/01690969008402105
- Silva, A. B., Liu, J. R., Zhao, L., Levy, D. F., Scott, T. L., & Chang, E. F. (2022). A neurosurgical functional dissection of the middle precentral gyrus during speech production. The Journal of Neuroscience, 42(45), 8416–8426. https://doi.org/10.1523/JNEUROSCI.1614-22.2022
- Strijkers, K., & Costa, A. (2011). Riding the lexical speedway: A critical review on the time course of lexical selection in speech production. Frontiers in Psychology, 2, 356.
- Strijkers, K., Costa, A., & Pulvermüller, F. (2017). The cortical dynamics of speaking: Lexical and phonological knowledge simultaneously recruit the frontal and temporal cortex within 200 ms. NeuroImage, 163, 206–219. https://doi.org/10.1016/j.neuroimage.2017.09.041
- Strijkers, K., Costa, A., & Thierry, G. (2010). Tracking lexical access in speech production: electrophysiological correlates of word frequency and cognate effects. Cerebral Cortex, 20(4), 912–928. https://doi.org/10.1093/cercor/bhp153
- Tilsen, S., Spincemaille, P., Xu, B., Doerschuk, P., Luh, W.-M., Feldman, E., & Wang, Y. (2016). Anticipatory posturing of the vocal tract reveals dissociation of speech movement plans from linguistic units. PLoS One, 11(1), e0146813. https://doi.org/10.1371/journal.pone.0146813
- Valente, A., Bürki, A., & Laganaro, M. (2014). ERP correlates of word production predictors in picture naming: A trial by trial multiple regression analysis from stimulus onset to response. Frontiers in Neuroscience, 8, 390. https://doi.org/10.3389/fnins.2014.00390
- Van der Merwe, A. (2008). A theoretical framework for the characterization of pathological speech sensorimotor control. In M. R. McNeil (Ed.), Clinical management of sensorimotor speech disorders ( 2nd ed., pp. 407–409). Thieme Medical Publishers.