ABSTRACT
This study aims to determine whether adding an additional modality (ultrasound tongue imaging) improves the inter-rater reliability of phonetic transcription in childhood speech sound disorders (SSDs) and whether it enables the identification of different or additional errors in children’s speech. Twenty-three English speaking children aged 5–13 years with SSDs of unknown origin were recorded producing repetitions of /aCa/ for all places of articulation with simultaneous audio and ultrasound. Two types of transcriptions were undertaken off-line: (1) ultrasound-aided transcription by two ultrasound-trained speech-language pathologists (SLPs) and (2) traditional phonetic transcription from audio recordings, completed by the same two SLPs and additionally by two different SSD specialist SLPs. We classified transcriptions and errors into ten different subcategories and compared: the number of consonants identified as in error by each transcriber; the inter-rater reliability; and the relative frequencies of error types identified by the different types of transcriber. Results showed that error-detection rates were different across the transcription types, with the ultrasound-aided transcribers identifying more errors than were identified using traditional audio-only transcription. Analysis revealed that these additional errors were identified on the dynamic ultrasound image despite being transcribed as correct, suggestive of subtle motor speech differences. Interrater reliability for classifying the type of error was substantial (κ = 0.72) for the ultrasound-aided transcribers and ranged from fair to moderate for the audio-only transcribers (κ = 0.38 to 0.52). Ultrasound-aided transcribers identified more instances of increased variability and abnormal timing errors than the audio-only transcribers.
Introduction
A speech sound disorder (SSD) is a delay or difficulty in acquiring intelligible or acceptable speech in a child’s native language. SSDs – especially of unknown origin – are common, affecting 3.6% of eight-year-old children (Wren et al., Citation2016). SSD is an umbrella term, likely comprising several distinct disorders with different causes, yet there is no universally agreed classification system (Waring & Knight, Citation2013). However, most classification systems acknowledge subtypes involving substitutions (for example, in phonological disorders), distortions (for example, in residual speech sound disorders) and inconsistencies (for example, in Childhood Apraxia of Speech [CAS] or Inconsistent Phonological Disorder). These, and other types of errors, are usually identified using phonetic transcription of the child’s speech collected during a face-to-face assessment. Such transcriptions, and the subsequent error analysis, support the speech-language pathologist (SLP) in diagnosing the subtype (or subtypes) of SSD present and in guiding intervention choices. Although phonetic transcription and the subsequent error analysis is insufficient for differential diagnosis of SSDs, it is nonetheless a crucial step in the clinical management of the disorder.
Phonetic transcription is a complicated task. It is a representation of the child’s speech as perceived by a trained listener and as such it is subject to the experiences and bias of that listener (for example, categorical perception; e.g., Liberman et al., Citation1957). Narrow phonetic transcription is particularly important when working with disordered speech (Ball et al., Citation2009); yet the inter-rater reliability of phonetic transcription – and in particular narrow transcription – is known to be problematic (Shriberg & Lof, Citation1991). Point-by point inter-rater reliability of transcription of disordered speech lowers as the severity of the speech disorder increases (Shriberg & Lof, Citation1991). To be most useful clinically and appropriate for research, phonetic transcriptions must be both valid and reliable. Although other measures of reliability exist, such as weighted reliability or consensus methods (e.g., Oller & Ramsdell, Citation2006; Smit et al., Citation2018), point-by-point agreement is frequently used within the literature (Seifert et al., Citation2020). These issues of reliability, or using binary correct/incorrect judgements instead of transcription, can lead to missing distortion errors or the under-reporting of covert contrasts (productions which are perceived to be identical but have subtle articulatory differences; Munson et al., Citation2010). Distortions and covert contrast errors are more common when a speech disorder is severe or a child’s errors are unusual or atypical, which likely further impacts the reliability of phonetic transcription for these populations. This problem is compounded when trying to transcribe the speech of children with motor speech disorders, such as CAS or Speech Motor Delay, as the speaker may simultaneously make several different error types, including distorting phones, being inconsistent, or producing non-native speech sounds (for example, in CAS [ASHA, Citation2007]).
Given the importance of transcription as a first step in diagnosis and intervention planning, several studies have explored ways to improve the reliability of transcription. One such strategy, which has been shown to improve the inter- and intra-rater reliability of the transcription of disordered speech, is to add additional modalities. Typically, in speech-language pathology practice this means audio or video recording any speech assessment and playing it back, perhaps several times, while transcribing. Although time-consuming, such practice is common in the cleft palate clinic and incorporating video – rather than audio alone – has been shown to increase inter-rater reliability of transcriptions and to increase the number of errors identified (Klinto & Lohmander, Citation2017). Other modalities that may support phonetic transcription include the addition of instrumental articulatory approaches.
Instrumental approaches to phonetic transcription
The predominant instrumental articulatory approach to transcription over the last few decades has been electropalatography (EPG). In this technique, tongue-palate contact is recorded using a pseudo-palate into which electrodes are embedded. Many small-N studies have used EPG to show that errors such as double articulations, increased contact and covert contrasts – which are visible when using the technique – are missed by perceptual phonetic transcription (for example, Gibbon, Citation1999, Citation2004; Hardcastle et al., Citation1987). These subtle unexpected articulations might suggest underlying motor difficulties, even if they do not lead to a loss of phonemic contrast. Given their subtle differences, these errors are likely to be missed when transcribing speech if only broad phonetic transcription or correct/incorrect judgements are used by the listener. In light of the underlying suspected motoric basis of these errors, the implications of incorrect transcription for differential diagnosis are clear. However, EPG is not routinely used as a tool to supplement phonetic transcription due to cost and logistical barriers. In contrast, a different instrumental approach – ultrasound tongue imaging (UTI) – allows low cost data acquisition from a larger number of speakers.
Ultrasound tongue imaging
In this technique, an ultrasound probe is placed under the chin to show a dynamic image of the tongue in either a mid-sagittal or coronal view. The technique has been used in several studies to analyse the speech of children with SSDs and it can also be used as a biofeedback tool in intervention (Sugden et al., Citation2019). In their UTI study of persistent velar fronting, Cleland et al. (Citation2017) describe a detailed case study of “Rachel”, a nine-year-old with persistent velar fronting. Ultrasound revealed several covert errors and the analysis also identified that she produced all oral stops, not just target velars, with a variety of different tongue shapes including retroflexes, undifferentiated gestures, and correct alveolar gestures. These errors were random in nature, suggestive of a motor speech disorder. This information is important because it indicates the need for a different intervention approach for Rachel than the phonologically-based approaches that would usually be used to treat velar fronting. Other researchers have also used UTI to identify covert contrasts or errors in the speech of children with speech delay and cleft palate (Bressmann et al., Citation2011; McAllister Byun et al., Citation2016). However, all of these studies used very small numbers of speakers: thus, their conclusions that UTI can reveal diagnostically useful information about the speech of children with SSDs may have limited wider applications.
One exception to this is a recent study in which the authors compared ultrasound-aided with perception-based transcription and classification of errors in the speech of 39 children with cleft lip and/or palate (Cleland et al., Citation2020). In this study, the transcribers identified all of the eight speech error types summarised in a review paper by Gibbon (Citation2004) which sought to provide a taxonomy of errors found in EPG studies of children with cleft lip and palate. The eight error types are: increased contact; retraction; fronted placement; complete closure; open pattern (undershoot or post-velar productions); double articulations; increased variability; and abnormal timing. Although derived from studies with children with cleft lip and/or palate, none of these error types are unique to cleft palate speech and in fact some of them may be more likely to indicate a motor-based or articulatory error. For example, increased contact may be due to an undifferentiated lingual gesture where the speaker is unable to achieve adequate tongue tip/blade and dorsum differentiation (Gibbon, Citation1999). Likewise, increased variability and abnormal timing may be articulatory hallmarks of CAS (Barry, Citation1995). lists and describes these eight error types and includes references to studies which have reported these types of errors in SSD of unknown origin. shows an example ultrasound image of each error type, with the tongue tip to the right for all panels except for Panel D (complete closure) which is in the coronal view. The green line on the images indicates the position of the palate. Two additional error types are included: retroflexion (Cleland et al., Citation2017) and coronal or dorsal production. Given that the error types listed in can be both identified by ultrasound (Cleland et al., Citation2020) and are indicative of other types of SSD, it therefore seems plausible that UTI could be a viable tool for identifying these types of errors in children with SSD of unknown origin. Such information could be useful for providing important diagnostic information about the potential SSD subtype or subtypes present.
Table 1. Error types, descriptions, example references and expected IPA transcriptions.
In addition to demonstrating that UTI can be used to reveal subtle speech errors, the Cleland et al. (Citation2020) study also demonstrated that adding UTI to perception-based phonetic transcription resulted in increased inter-rater reliability. Contrary to expectations, the use of ultrasound to aid transcriptions did not change the overall number of errors identified by transcribers; however, it did change how errors were classified into the categories listed in . Such classifications may be diagnostically important. Of course, the results of a study of cleft palate speech cannot be assumed to be the same for children with other types of speech disorders given the different types of errors typically associated with each disorder. Thus, despite evidence that the additional visual information provided by ultrasound can increase the reliability of phonetic transcription of cleft speech, it is unknown whether this holds true for other types of speech disorders. If it does, ultrasound could be a useful tool for supplementing traditional phonetic transcription for a wider group of children. Given that ultrasound also shows promise as a biofeedback tool for intervention (Sugden et al., Citation2019), the dual applicability of ultrasound as an assessment and intervention tool makes it worth investigating whether the findings of Cleland et al. (Citation2020) replicate with this group of children.
Purpose
The purpose of this preliminary study was two-fold. Firstly, we wanted to explore whether the finding of Cleland et al. (Citation2020) that UTI improves the reliability of phonetic transcription of cleft palate speech extended to children with SSD of unknown origin by replicating the method with a different population of children. Secondly, we wanted to explore whether ultrasound could be used to identify subtle, likely motor-based, errors in a larger group of children with SSD of unknown origin. We therefore conducted a preliminary study investigating whether the addition of ultrasound images of the tongue to perception-based phonetic transcription impacts: (1) The overall number of errors identified in the speech of children with SSD of unknown origin; (2) the inter-rater reliability; and (3) the types of errors identified.
Methods
Participants/Speakers
Speakers were attendees of speech-language pathology services in rural and urban areas in the east of Scotland. Children met two inclusion criteria: (1) aged 5 to 16 years, and; (2) have a current diagnosis of any type of SSD of unknown origin made by their usual SLP. Participants were excluded if they had: (1) a current or repaired syndromic or non-syndromic cleft lip and/or palate of any type, (2) no spoken English, (3) evidence of severe/profound hearing loss, or (4) evidence of severe/profound learning disability. Participants were not excluded based on the presence of other concomitant communication disorders. Determination of participant eligibility was made by their SLP.
Data was collected as part of a larger project aiming to record ultrasound data from children with a variety of SSD subtypes from community clinical contexts (ULTRAX2020; Cleland et al., Citation2018), This meant that we did not have full diagnostic information available for the children. Although UTI is potentially most useful for identifying errors associated with motor speech disorders we chose not to select children with only these diagnoses at the outset. Given the focus of this study on inter-rater reliability and the previous research which suggests that even children with diagnoses of phonological disorders can present with subtle motor impairments (Gibbon, Citation1999), inclusion of a wide variety of SSDs was both convenient and desirable
Twenty-six children and their parent/guardian consented to participate in the project. Of these, three datasets were unusable due to poor quality of the ultrasound image (n = 1) or insufficient data collection to enable transcription (n = 2). Thus, data from 23 children (18 male, 5 female) aged 5;2 to 12;11 (mean = 8.23 years; SD = 2.11) were included. shows participants’ demographic information. The study received ethical approval from the South East Scotland Research Ethics Committee 01 (reference 18/SS/0072).
Table 2. Speakers’ demographic information.
Speech recording materials
Speech materials included those used in the study by Cleland et al. (Citation2020). That is, ten repetitions of all English sonorants and voiceless obstruents in /aCa/ context (with the exception of the velar nasal which was produced in the word “bang”), in the following order: /t, n, ɹ, θ, s, ʃ, l, j, k, ʧ, ŋ, p, m, f, w/. These recordings were a subset of a longer dataset which is fully described in Cleland et al. (Citation2020).
Ultrasound speech recording
High-speed UTI data were acquired using a Micro machine controlled via a laptop running Sonospeech (version 2.17.10, version 2.18.01, version 2.18.02, or version 2.18.04; Articulate Instruments Ltd, Citation2019), a specialised software developed to collect ultrasound and audio data for use in speech-language pathology assessment and intervention. The echo return data were recorded at ~100 fps over a field of view of 162°. This field of view allowed the greatest view of the tongue, including both the hyoid and mandible shadows. A 5–8 MHz 10 mm radius microconvex ultrasound probe was stabilized with an Ultrafit lightweight plastic headset (Pucher et al., Citation2020). An Audio Technica3350 microphone was attached to the headset to record the audio data. All data was collected by community-based SLPs who were trained in the data collection and assessment process by both authors. Data was collected in a quiet clinic room at the SLP’s place of work.
Transcriptions
Two different types of transcriptions were performed: (1) Ultrasound-aided phonetic transcription (UA), and (2) traditional phonetic transcription using audio-only data (AO). Unlike the original study by Cleland et al. (Citation2020), we did not ask the SLPs collecting the data to provide on-line transcriptions of the children’s speech. This decision was made in response to a recommendation from Cleland et al. (Citation2020) that clinicians should not attempt live phonetic transcription while completing an ultrasound assessment due to the need to manage multiple tasks during a recording session.
Ultrasound-aided phonetic transcriptions
Transcriptions were made using an adaptation of the assessment form by Cleland et al. (Citation2020). This form includes space to record a phonetic transcription using IPA and extIPA symbols and allows the transcriber to note the presence of various descriptive error types based on the aforementioned work by Gibbon (Citation2004). We excluded complete closure from the original taxonomy by Gibbon (Citation2004) as this error is only visible in the coronal view (which was not used in this study) and added a category of coronal or dorsal production for labial targets produced with either coronal or dorsal articulations (e.g., /m/ to [n], as this error was observed in the original Cleland et al., Citation2020 study). This gave us nine ultrasound-imageable error types plus a tenth category for errors which are not imageable using ultrasound, such as voicing errors. A previous version of the form and further instructions for use are available in an open access manual (Cleland et al., Citation2018). Descriptions of each error type are provided in .
Ultrasound-aided transcriptions were performed offline by two ultrasound-trained SLPs (the authors, JC [Transcriber 1, T1] and ES [Transcriber 2, T2]). Both authors are academic SLPs, hold PhDs, and at the time had between 7 and 15 years’ experience researching and working clinically with SSDs. These transcribers also acted as the ultrasound-aided transcribers in the Cleland et al. (Citation2020) study. To perform the transcriptions, the two transcribers sat in the same quiet room. They first watched and listened to a recording of each child counting from 1 to 10 (to familiarise themselves with the child’s speech), then systematically worked through the consonant repetitions. Each recording was played once in real time, after which the transcribers noted down the phonetic transcription of the child’s productions and also selected any present error types from the nine categories on the assessment sheet. We chose to allow only one viewing of the ultrasound recording to replicate Cleland et al. (Citation2020) where one purpose was to design a qualitative ultrasound analysis tool which would be quick and easy for clinicians to use in the clinic. Multiple sessions of approximately 1–2 hours were needed to transcribe all of the data. Although the two transcribers completed the transcriptions in the same room, they worked independently to transcribe each recording.
Audio-only traditional phonetic transcriptions
The audio signal was extracted from the recordings of the children producing /aCa/. MP3s of the audio data were provided to the transcribers and they did not have access to the ultrasound images. They were instructed to listen to each recording (containing a participant’s repetitions of the target consonant in /aCa/ context) only once, to note their transcription using IPA and extIPA symbols (including diacritics where necessary), and to then move onto the next recording. Transcribers were asked to transcribe only the target consonant in the /aCa/ recording (that is, to ignore the vowels), and to include all different productions made by the child or to include only one transcription if all of the repetitions sounded the same. After completing this process for all target consonants, the transcribers were permitted to listen to each recording up to a maximum of three additional times and to note any further transcriptions in a separate column. These additional transcriptions were not included in the analysis for the purposes of this paper, and the transcribers were not permitted to change their original transcription.
The audio-only data was transcribed by two pairs of transcribers. The first pair comprised the authors (JC [T1] and ES [T2]) who completed the audio-only transcriptions at least 12 months after completing the ultrasound-aided transcriptions. The second pair comprised two SLPs (Transcriber 3 [T3] and Transcriber 4 [T4]). These clinical-academic SLPs were also experts in transcribing disordered speech: one held a PhD in biofeedback approaches to treating SSD and the other was a university senior lecturer (US associate professor) in phonetic transcription. One of the transcribers (T3) had been involved in collecting some of the data; however, the data (sound files of /aCa/ repetitions) were de-identified and this transcriber did not complete the transcriptions until at least six months after the data were collected. Note, these were not the same audio-only transcribers as in the original Cleland et al. (Citation2020) study. The transcribers were asked to wear headphones when completing their transcriptions. The inclusion of two pairs of audio-only transcribers allowed for a comparison between raters completing the same transcription task.
Coding of audio-only phonetic transcriptions
Following the procedure by Cleland et al. (Citation2020), the audio-only phonetic transcriptions from all four transcribers were then coded by the research team as either correct or incorrect. That is, if only one transcription was given that matched the adult target this was scored as “correct”. If a transcription did not match the adult target, or multiple correct and incorrect transcriptions were given this was marked as “incorrect”. Note, this is somewhat different to traditional percentage consonant correct measures as the child was required to produce all 10 repetitions of the consonant correctly for it to be classified as “correct”. Variable productions where some were transcribed as correct and some were not were classified as “incorrect”. Incorrect productions were then classified into one or more of the nine ultrasound-imageable error types described in , or as a non-imageable error. This classification process was similar to traditional phonetic/phonological analysis, for example, “retraction” () was chosen for any alveolar target that was transcribed using a palatal or velar symbol; “open pattern” was chosen for any consonant that was transcribed using a uvular or pharyngeal symbol, or contained the “lowered” diacritic (see ). Non-imageable errors were any error which does not involve a tongue-shape or place error and were taken from a list by McLeod and Baker (Citation2017), for example, de/voicing. These codes (correct, incorrect, and error categories) were used in subsequent analyses.
Analyses
Statistical analyses
The non-parametric Wilcoxon signed-rank test was used to compare the percent of each speaker’s consonant repetitions coded as correct by the different types and pairs of transcribers. Due to violations in assumptions of independence, we were unable to statistically compare differences between the ultrasound-aided and audio-only transcriptions completed by the same pair of transcribers (T1 and T2).
To determine whether ultrasound-aided transcriptions led to changes in point-by-point interrater reliability, we calculated Cohen’s kappa with a 95% confidence interval between transcribers for three different subclasses of errors: (1) correct versus incorrect productions, (2) imageable versus non-imageable errors (a lingual error from any one of the nine ultrasound-visible error types in versus any non-lingual error such as voicing or change in resonance), and (3) interrater reliability across all nine error types plus a tenth category of any non-imageable error (that is, the classification of the type of error). As Cohen’s kappa is limited to one error categorisation, if multiple errors were identified in a single recording (for example, retracted and variable) the first relevant error from the list in was used in calculations. Note that the IPA transcriptions provided by both types of transcribers were not directly used in analyses; rather, analysis focussed on the error categories identified as present in a child’s production.
Descriptive analysis of error types
As we wanted to explore whether the addition of ultrasound imaging to phonetic transcription resulted in the identification of different types of errors and/or subtle speech errors, we compared the total number of different error types across all participants identified by type of transcription (using an average of the transcribers from each group/pair). Visual inspection of the data was carried out due to the small number of raters and speakers.
Results
There were a total of 345 recordings of consonant repetitions produced by the participants. Due to poor quality, four recordings were unable to be used so a total of 341 recordings were transcribed.
Identification of errors across transcription types
Overall, for the ultrasound-aided transcriptions, an average of 191/341 (56.0%) of all recordings were classified as always being produced correctly. For the audio-only transcriptions, the first pair of transcribers (who also completed the ultrasound-aided transcriptions) identified 214.5/341 (62.9%) recordings as correct and the second pair of transcribers identified 194.5/341 (57.0%) as correct. That is, the transcribers who completed both types of transcription had a higher error identification rate when completing ultrasound-aided compared to audio-only transcription.
Looking at ratings for each participant, the average number of productions rated as having all consonant repetitions produced correctly (i.e. a proxy measure of percentage consonants correct per child) by the ultrasound-aided transcriptions was 56.1% (range 6.7% to 86.7% per child), by the first pair of audio-only transcriptions was 63.1% (range 26.7% to 88.7%), and by the second pair was 56.7% (range 23.3% to 84.6%). These results are shown in . The difference in error detection by the two pairs of raters who completed the audio-only transcriptions was significant (Z = −3.554, p < .001), whereas there was no significant difference between the ultrasound-aided and audio-only transcriptions provided by the two different pairs of raters (Z = −0.563, p = .573). We were interested in the descriptive finding that the same transcribers identified more errors when completing ultrasound-aided transcriptions than when completing audio-only transcriptions. Further investigation revealed that the additional incorrect productions identified by the ultrasound-aided raters had been phonetically transcribed as “always correct” but that the raters had identified one or more imageable error types from , such as abnormal timing or variability (and thus, due to the presence of an error, these productions were coded as incorrect – albeit within the phonemic boundary of an acceptable production).
Figure 2. Percent of children’s productions rated as always correct by the different types/pairs of transcribers (average of transcriber pair).
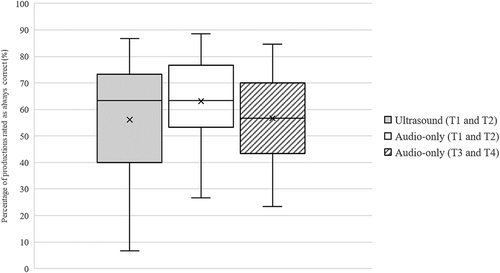
Considering the overall number of types of errors identified, the ultrasound-aided transcribers identified a total of 333 errors in the data (those listed in and those classed as non-UTI errors), equating to an average of 2.22 error types per incorrect production. The first pair of audio-only transcribers identified a total of 269 errors, representing an average 2.13 error types per incorrect production. The second pair of audio-only transcribers identified 305.5 errors (an average of 2.09 errors per incorrect production).
Interrater reliability of transcriptions
The results of interrater reliability calculations are shown in . When classifying participants’ productions as either correct or incorrect (that is, all consonant repetitions within the recording produced correctly or incorrectly), the two ultrasound-aided transcribers had almost perfect interrater reliability (κ = 0.86). The first pair of audio-only transcribers had substantial interrater reliability (κ = 0.62) and the second pair had moderate reliability (κ = 0.56). When comparing across transcriber category (that is, comparing the rating of correctness of an ultrasound-aided transcriber with an audio-only transcriber), interrater reliability was generally moderate, ranging from κ = 0.42 to κ = 0.62, with reliability lower for audio transcriber T4.
Table 3. Agreement between transcribers for rating (1) production as always correct or incorrect, (2) errors as imageable or non-imageable, and (3) classification of error types.
When classifying the participants’ errored productions as either an imageable or a non-imageable error, the ultrasound-aided transcribers had almost perfect interrater reliability (κ = 0.86) and both pairs of audio-only transcribers had moderate reliability (κ = 0.59 and κ = 0.53). Interrater reliability was moderate when compared across transcriber category (κ = 0.43 to 0.58), with lower reliability seen with T4.
A similar relationship was found with interrater reliability of the classification of errored productions into the error categories identified in or as a non-ultrasound error. That is, the two ultrasound-aided transcribers had substantial interrater reliability (κ = 0.72), both pairs of audio-only transcribers having lower interrater reliability (moderate, κ = 0.46–0.48) and the across-transcriber category interrater reliability was moderate to fair (κ = 0.32 to 0.51). Overall, interrater reliability was lowest for error classification and highest for judgements of correctness.
Descriptive analysis of error types identified by transcribers
shows the total number of different error types identified by each group. An average of the two transcribers from each group/pair is shown. The ultrasound-aided transcribers identified more instances of increased variability and abnormal timing than the audio-only transcribers, perhaps reflecting that these error types may be covert or difficult to detect when listening to speech. They also identified more non-UTI errors than the audio-only transcribers. The audio-only transcribers identified more instances of retraction than the ultrasound-aided transcribers.
Discussion
In this study we replicated the method of Cleland et al. (Citation2020) with data collected from children with SSD of unknown origin. Findings regarding error detection differ from the original study, but findings regarding inter-rater reliability and identification of error-types are similar. Implications, limitations, and directions for future research are considered below.
Error detection and transcription reliability
In this study we found that, when completed by the same transcribers, ultrasound-aided transcription resulted in the identification of more errors in children’s speech than audio-only phonetic transcription. Further analysis revealed that these ‘additional’ errors were typically categorised as increased variability or abnormal timing errors identified in the dynamic ultrasound image in productions that had been transcribed perceptually as falling into the correct phonemic category. Several possible explanations for this finding exist. For example, the identification of visual differences in perceptually-correct productions could be due to natural variation in articulation and in the development of mature motor control of the speech system. Numerous studies have shown that typically-developing children demonstrate increased articulatory and acoustic variation in their speech which reduces as they age (e.g., Cheng et al., Citation2007; Murdoch et al., Citation2012). It could be that the ultrasound-aided transcribers were categorising articulatory variation as an error when in fact it represents natural variability in articulatory production which is within the correct acoustic and perceptual boundaries of the target phone. However, previous ultrasound research with children with typically developing speech has shown that, although children do present with low levels of variability on repeated productions of target consonant sequences, this variability is likely below the level able to be identified using visual inspection of ultrasound images in real-time (Zharkova et al., Citation2012). Further, the ultrasound-aided transcribers in the original study by Cleland et al. did not identify increased instances of variability or abnormal timing in disordered speech compared to the audio-only transcribers, which suggests that these additional errors are less likely to be due to transcribers identifying natural variability. Future research on the level of articulatory variability in the speech of typically-developing children is needed, but based on these two small studies it appears that the ‘additional’ variability and timing errors identified by the ultrasound-aided transcribers in the present study most likely represent errors that may be indicative of subtle motor speech difficulties (Vick et al., Citation2014). Indeed, previous research has identified errors or differences in tongue shape and/or movement between productions in this population (Cleland & Scobbie, Citation2021). This point will be expanded upon below.
Although our finding about increased error-detection rates when using ultrasound to support transcription is contrary to the original study by Cleland et al. (Citation2020) – who found no difference in the number of errors identified by the two transcription types – it is consistent with the hypotheses from the original study. The difference in error detection rates between the two studies can likely be explained by expected differences in the clinical samples from which the speech samples were drawn. Children with cleft lip and/or palate typically present with predictable speech errors such as backing and incorrect resonance which are either clearly visible on ultrasound and/or are perceptually salient, whereas children with SSDs of unknown origin may present with a wider range of subtle articulatory differences that indicate potential motor-based problems despite surface-level phonetic transcriptions indicating that none exist. Thus, it appears that the use of ultrasound to supplement phonetic transcription of the speech of children with SSDs of unknown origin may support the identification of subtle underlying articulatory errors.
The error identification rates between the two pairs of audio-only transcribers were significantly different in this study, with the transcribers who also completed the ultrasound-aided transcriptions identifying 62.9% of all recordings as correct and the transcribers who completed only the audio transcriptions identifying 57.0% as correct. The individual differences in classifying a production as correct or incorrect could be explained by clinical experience, training in phonetic transcription, perceptual bias, or personal phonemic category boundaries and acceptability and subsequent classification of intermediate productions (e.g., Munson et al., Citation2010; Pye et al., Citation1988). It is also possible that training using instrumental techniques may influence an individual’s approach to audio-only traditional transcription tasks; however, due to the low number of raters in this study, we are unable to draw any conclusions in this regard. While the difference in error detection rates seen in this study is unlikely to be clinically significant, the fact that these four experienced SLPs had different error detection rates when completing the same transcription task has implications for clinical practice, particularly in regards to decisions in severity classification, target selection and progress monitoring. Despite identifying significant differences in the error detection rates between the two types of transcribers, interrater reliability ranged from moderate to almost perfect.
In line with the original study, we found that the addition of ultrasound imaging to transcription improves inter-rater reliability compared to audio-only transcriptions. In all cases the inter-rater reliability was higher when comparing the two ultrasound-aided transcribers versus the audio-only phonetic transcriptions. Improving the reliability of phonetic transcription is important because it supports the SLP in having confidence about the nature and type of speech errors they identify during assessment, which can facilitate differential diagnosis and thus lead to more accurate intervention planning.
Taken together, both the previous (Cleland et al., Citation2020) and current study suggest that ultrasound might be a useful addition to the SLPs’ assessment toolkit for certain children with SSDs of unknown origin. It is important to emphasise that ultrasound can only support – not replace – traditional perceptual phonetic transcription. The technique records the movements of the tongue only and as such no information is given about voicing, nasal resonance, or labial articulations. Thus it is unlikely that ultrasound will be widely adopted by SLPs as a tool for only improving transcription reliability. Instead, we intend the current findings to inform clinicians about the dual possibility of using ultrasound in assessment as well as in intervention. Specifically, ultrasound may be useful for identifying subtle articulatory errors in children where motor speech difficulties are suspected. Confirming the presence of such errors is important for differential diagnosis, treatment planning and progress monitoring (Namasivayam et al., Citation2013).
Error types in children’s speech
The second goal of this study was to determine whether ultrasound can be used to identify subtle articulatory errors in children with SSD of unknown origin. In the current study the transcribers using ultrasound identified more instances of increased contact, variability, abnormal timing compared to the audio-only transcribers and the transcriptions of cleft speech from the Cleland et al. (Citation2020) study. All of these errors have potential motor origins, and identification of them may lead to a change in treatment focus.
The first of these errors, increased contact, was first described in EPG studies of children with “functional articulation disorders” (a now outdated term equivalent to SSD of unknown origin) by Gibbon (Citation1999) as indicating an undifferentiated lingual gesture. In this type of error the child raises the whole tongue body during stop closures, rather than differentiating the tip/blade from the tongue dorsum. Often these errors are transcribed as alveolar/velar mergers or inconsistent productions and in fact our audio-only transcribers recorded more instances of retracted and similar instances of fronted productions than the ultrasound-aided transcribers. The second of these errors, increased variability (or articulatory instability), is reported in studies of children and adults with motor speech disorders. An early study using EPG showed that children with suspected CAS present with increased variability in productions of the same consonant across multiple repetitions (Hardcastle et al., Citation1987). More recently, Grigos et al. (Citation2015) demonstrated that movement variability of the jaw and lips is significantly higher in children with CAS compared to children with other types of SSD or to typical children. Increased variability may therefore be an important indicator of reduced motor control. Finally, our ultrasound transcribers also reported increased instances of abnormal timing. This was not reported at all in the original study of children with cleft lip and palate (Cleland et al., Citation2020), who we would not necessarily expect to have motor control difficulties. Abnormal timing is also reported in the speech of both children and adults with motor speech disorders. A recent study by Kopera and Grigos (Citation2020) demonstrated that children with CAS show poor temporal control of articulatory movements during a lexical stress task. This, and other studies suggest that abnormal timing is a diagnostically important error type which is unlikely to be identified using audio-based transcription alone.
The increased identification of these subtle errors by the ultrasound-aided transcribers is not to say that all children that present with errors revealed by ultrasound will meet criteria for severe SSDs such as CAS. Rather, some may have less severe diagnoses such as Speech Motor Delay (Shriberg et al., Citation2019; Vick et al., Citation2014) that may still require a motor-based intervention approach. Ultrasound visual biofeedback is an intervention based on the principles of motor learning and therefore has the potential to be a useful technique for both the diagnosis and remediation of such errors (Sugden et al., Citation2019). The current study provides further evidence that assessment supplemented with ultrasound imaging might be an important precursor to ultrasound visual biofeedback therapy. Moreover, the qualitative method used here – rather than time-consuming lab-based analysis – has the potential to be used by trained clinicians.
Limitations and future directions
These preliminary findings are limited by the raters used in this experiment, who were experts in SSD, rather than community clinicians. It is possible that results would be different with raters of different levels of experience. The age of the children in the dataset (mean age 8.23 years) may also have influenced the type of errors identified by the transcribers, as older children may be more likely to have persistent SSDs involving disordered motor speech processes (Wren et al., Citation2016). The results are also limited by the classification into ultrasound error types, rather than, for example, calculation of inter-rater reliability on the IPA/ExtIPA transcriptions and further studies should consider both. Indeed, there were some limitations in using the taxonomy of error types described by Gibbon (Citation2004). While all of these errors are applicable to children with SSD of unknown origin, the error types were developed through Gibbon’s work with EPG and are therefore not all relevant for ultrasound. In particular, the error open pattern which in EPG means no tongue-palate contact but in ultrasound can mean undershoot, uvular, or pharyngeal productions. Furthermore, we also noted instances of lowering of an articulation, for example, a fricative lowered to an approximant which was difficult to categorise within the taxonomy. We would recommend that future studies add additional descriptions as further errors are identified in different datasets.
The decision to use the qualitative rating sheet when completing the ultrasound-aided transcriptions but not when completing the audio-only transcriptions was pragmatic and based on the methods of the original study by Cleland et al. (Citation2020). This decision may have limited the types of errors able to be identified by the audio-only transcribers, which could have influenced our findings.
Finally, the type of ultrasound analysis used in the current study was qualitative in nature and SLPs with experience using ultrasound rated the children’s productions by watching them only once. This methodological decision was made to replicate Cleland et al. (Citation2020) who had the additional aim of designing an ultrasound assessment that would be quick and easy to use in clinical settings. However, this did limit us to analysing speech in only very limited contexts (/aCa/ repeated 10 times). Moreover, it necessitated scoring items as “correct” only when all 10 productions were correct. Watching the ultrasound multiple times (or listening to the recording multiple times) and transcribing each production individually would doubtless have revealed further information, though this would have been time consuming. Furthermore, if we had analysed more complex contexts or connected speech different error types might have been identifiable. However, this would need a different and more complex approach, either playing back short sections of speech in slow motion/ frame by frame or performing analysis using ultrasound metrics (see Cleland, Citation2021 for a list of ultrasound metrics applied in clinical studies). Ongoing work seeks to automate the process of ultrasound analysis to allow us to identify errors in a quick and objective manner.
Conclusions
This study adds to the evidence around the use of additional modalities to support phonetic transcription. Moreover, it adds to the instrumental articulatory literature which suggests that measuring the articulators directly can affect the number of errors identified in children’s speech as well as the types of errors that transcribers identify. Transcribers who used ultrasound identified more instances of increased variability, abnormal timing, and increased contact in the children’s speech, which may be diagnostically important in children with SSDs. These preliminary findings are intended to inform SLPs of the dual potential of ultrasound as a targeted assessment and intervention tool.
Acknowledgments
We would like to thank the children and parents who took part in the project and the SLPs who provided the data. Thank you to Dr Zoe Roxburgh and Sarah White for performing the audio-only transcriptions.
Disclosure statement
There are no relevant conflicts of interest to declare.
Additional information
Funding
References
- American Speech-Language-Hearing Association. (2007). Childhood apraxia of speech [Technical report]. Retrieved from www.asha.org/policy/
- Articulate Instruments Ltd. (2019). SonoSpeech user guide: Version 2.18_04. (Articulate Instruments. Ltd. Ed.).
- Ball, M., Muller, N., Klopfenstein, M., & Rutter, B. (2009). The importance of narrow phonetic transcription for highly unintelligible speech: Some examples. Logopedics Phoniatrics Vocology, 34(2), 84–90. https://doi.org/10.1080/14015430902913535
- Barry, R. M. (1995). A comparative study of the relationship between dysarthria and verbal dyspraxia in adults and children. Clinical Linguistics & Phonetics, 9(4), 311–332. https://doi.org/10.3109/02699209508985339
- Bressmann, T., Radovanovic, B., Kulkarni, G. V., Klaiman, P., & Fisher, D. (2011). An ultrasonographic investigation of cleft-type compensatory articulations of voiceless velar stops. Clinical Linguistics & Phonetics, 25(11–12), 1028–1033. https://doi.org/10.3109/02699206.2011.599472
- Case, J., & Grigos, M. I. (2016). Articulatory control in childhood apraxia of speech in a novel word-learning task. Journal of Speech, Language, and Hearing Research, 59(6), 1253–1268. https://doi.org/10.1044/2016_JSLHR-S–14–0261
- Cheng, H.-Y., Murdoch, B. E., Goozée, J. V., & Dion, S. (2007). Electropalatographic assessment of tongue-to-palate contact patterns and variability in children, adolescents, and adults. Journal of Speech, Language, and Hearing Research, 50(2), 375–392. https://doi.org/10.1044/1092-4388(2007/027)
- Cleland, J. (2021). Ultrasound tongue imaging. In M. J. Ball (Ed.), Manual of clinical phonetics(pp. 399–416). Routledge.
- Cleland, J., Lloyd, S., Campbell, L., Crampin, L., Palo, J. P., Sugden, E., … Zharkova, N. (2020). The impact of real-time articulatory information on phonetic transcription: Ultrasound-aided transcription in cleft lip and palate speech. Folia Phoniatrica Et Logopaedica, 72(2), 120–130. https://doi.org/10.1159/000499753
- Cleland, J., & Scobbie, J. M. (2021). The dorsal differentiation of velar from alveolar stops in typically developing children and children with persistant velar fronting. Journal of Speech, Language and Hearing Research, 64(6S), 2347–2362. https://doi.org/10.1044/2020_JSLHR-20-00373
- Cleland, J., Scobbie, J. M., Heyde, C., Roxburgh, Z., & Wrench, A. A. (2017). Covert contrast and covert errors in persistent velar fronting. Clinical Linguistics & Phonetics, 31(1), 35–55. https://doi.org/10.1080/02699206.2016.1209788
- Cleland, J., Scobbie, J. M., & Wrench, A. A. (2015). Using ultrasound visual biofeedback to treat persistent primary speech sound disorders. Clinical Linguistics & Phonetics, 29(8–10), 575–597. https://doi.org/10.3109/02699206.2015
- Cleland, J., Wrench, A., Lloyd, S., & Sugden, E. (2018). ULTRAX2020: Ultrasound technology for optimising the treatment of speech disorders: Clinicians’ resource manual. University of Strathclyde. https://doi.org/10.15129/63372
- Dodd, B., & Lacano, T. (1989). Phonological disorders in children: Changes in phonological process use during treatment. International Journal of Language & Communication Disorders, 24(3), 333–352. https://doi.org/10.3109/13682828909019894
- Gibbon, F. E. (1999). Undifferentiated lingual gestures in children with articulation/phonological disorders. Journal of Speech, Language, and Hearing Research, 42(2), 382–397. https://doi.org/10.1044/jslhr.4202.382
- Gibbon, F. E. (2004). Abnormal patterns of tongue‐palate contact in the speech of individuals with cleft palate. Clinical Linguistics & Phonetics, 18(4–5), 285–311. https://doi.org/10.1080/02699200410001663362
- Grigos, M. I., Moss, A., & Lu, Y. (2015). Oral articulatory control in childhood apraxia of speech. Journal of Speech, Language, and Hearing Research, 58(4), 1103–1118. https://doi.org/10.1044/2015_JSLHR-S-13-0221
- Hardcastle, W. J., Barry, R. M., & Clark, C. (1987). An instrumental phonetic study of lingual activity in articulation-disordered children. Journal of Speech, Language, and Hearing Research, 30(2), 171–184. https://doi.org/10.1044/jshr.3002.171
- Klintö, K. & Lohmander, A. (2017). Does the recording medium influence phonetic transcription of cleft palate speech? International Journal of Language & Communication Disorders, 52(4), 440–449. https://doi.org/10.1111/1460–6984.12282
- Kopera, H. C., & Grigos, M. I. (2020). Lexical stress in childhood apraxia of speech: Acoustic and kinematic findings. International Journal of Speech-Language Pathology, 22(1), 12–23. https://doi.org/10.1080/17549507.2019.1568571
- Liberman, A. M., Harris, K. S., Hoffman, H. S., & Griffith, B. C. (1957). The discrimination of speech sounds within and across phoneme boundaries. Journal of Experimental Psychology, 54(5), 358–368. https://doi.org/10.1037/h0044417
- Lundeborg, I., & McAllister, A. (2007). Treatment with a combination of intra-oral sensory stimulation and electropalatography in a child with severe developmental dyspraxia. Logopedics, Phoniatrics, Vocology, 32(2), 71–79. https://doi.org/10.1080/14015430600852035
- McAllister Byun, T., Buchwald, A., & Mizoguchi, A. (2016). Covert contrast in velar fronting: An acoustic and ultrasound study. Clinical Linguistics & Phonetics, 30(3–5), 249–276. https://doi.org/10.3109/02699206.2015.1056884
- McLeod, S., & Baker, E. (2017). Children’s speech:An evidence-based approach to assessment and intervention. Pearson.
- Munson, B., Edwards, J., Schellinger, S. K., Beckman, M. E., & Meyer, M. K. (2010). Deconstructing phonetic transcription: Covert contrast, perceptual bias, and an extraterrestrial view of Vox Humana. Clinical Linguistics & Phonetics, 24(4–5), 245–260. https://doi.org/10.3109/02699200903532524
- Murdoch, B. E., Cheng, H.-Y., & Goozée, J. V. (2012). Developmental changes in the variability of tongue and lip movements during speech from childhood to adulthood: An EMA study. Clinical Linguistics & Phonetics, 26(3), 216–231. https://doi.org/10.3109/02699206.2011.604459
- Namasivayam, A. K., Pukonen, M., Goshulak, D., Yu, V. Y., Kadis, D. S., Kroll, R., Pang, E. W., & De Nil, L. F. (2013). Relationship between speech motor control and speech intelligibility in children with speech sound disorders. Journal of Communication Disorders, 46(3), 264–280. https://doi.org/10.1016/j.jcomdis.2013.02.003
- Oller, D. K., & Ramsdell, H. L. (2006). A weighted reliability measure for phonetic transcription. Journal of Speech, Language, & Hearing Research, 49(6), 1391–1411. https://doi.org/10.1044/1092-4388(2006/100)
- Pucher, M., Klingler, N., Luttenberger, J., & Spreafico, L. (2020). Accuracy, recording interference, and articulatory quality of headsets for ultrasound recordings. Speech Communication, 123, 83–97. https://doi.org/10.1016/j.specom.2020.07.001
- Pye, C., Wilcox, K. A., & Siren, K. (1988). Refining transcriptions: The significance of transcriber ‘errors’. Journal of Child Language, 15(1), 17–37. https://doi.org/10.1017/S0305000900012034
- Seifert, M., Morgan, L., Gibbin, S., & Wren, Y. (2020). An alternative approach to measuring reliability of transcription in children’s speech samples: Extending the concept of near functional equivalence. Folia Phoniatrica Et Logopaedica, 72(Suppl. 2), 84–91. https://doi.org/10.1159/000502324
- Shriberg, L., Kwiatkowski, J., & Mabie, H. L. (2019). Estimates of the prevalence of motor speech disorders in children with idiopathic speech delay. Clinical Linguistics & Phonetics, 33(8), 679–706. https://doi.org/10.1080/02699206.2019.1595731
- Shriberg, L., & Lof, G. (1991). Reliability studies in broad and narrow phonetic transcription. Clinical Linguistics & Phonetics, 5(3), 225–279. https://doi.org/10.3109/02699209108986113
- Smit, A. B., Brumbaugh, K. M., Weltsch, B. & Hilgers, M. (2018). Treatment of phonological disorder: A feasibility study with focus on outcome measures. American Journal of Speech-Language Pathology, 27(2), 536–552. https://doi.org/10.1044/2017_AJSLP-16-0225
- Sugden, E., Lloyd, S., Lam, J., & Cleland, J. (2019). Systematic review of ultrasound visual biofeedback in intervention for speech sound disorders. International Journal of Language & Communication Disorders, 54(5), 705–728. https://doi.org/10.1111/1460-6984.12478
- Vick, J. C., Campbell, T. F., Shriberg, L. D., Green, J. R., Truemper, K., Rusiewicz, H. L., & Moore, C. A. (2014). Data-driven subclassification of speech sound disorders in preschool children. Journal of Speech, Language, and Hearing Research, 57(6), 2033–2050. https://doi.org/10.1044/2014_JSLHR-S-12-0193
- Viera, A. J., & Garrett, J. M. (2005). Understanding interobserver agreement: The Kappa statistic. Family Medicine, 37(5), 360–363.
- Waring, R., & Knight, R. (2013). How should children with speech sound disorders be classified? A review and critical evaluation of current classification systems. International Journal of Language & Communication Disorders, 48(1), 25–40. https://doi.org/10.1111/j.1460-6984.2012.00195.x
- Wren, Y., Miller, L. L., Peters, T. J., Emond, A., & Roulstone, S. (2016). Prevalence and predictors of persistent speech sound disorder at eight years old: Findings from a population cohort study. Journal of Speech, Language, and Hearing Research, 59(4), 647–673. https://doi.org/10.1044/2015_JSLHR-S-14-0282
- Zharkova, N., Hewlett, N., & Hardcastle, W. (2012). An ultrasound study of lingual coarticulation in /sV/ syllables produced by adults and typically developing children. Journal of the International Phonetic Association, 42(2), 193–208. https://doi.org/10.1017/S0025100312000060