ABSTRACT
A number of prominent theories have linked tendencies to mimick others’ facial movements to empathy and facial emotion recognition, but evidence for such links is uneven. We conducted a meta-analysis of correlations of facial mimicry with empathy and facial emotion recognition skills. Other factors were also examined for moderating influence, e.g. facets of empathy measured, facial muscles recorded, and facial emotions being mimicked. Summary effects were estimated with a random-effects model and a meta-regression analysis was used to identify factors moderating these effects. 162 effects from 28 studies were submitted. The summary effect size indicated a significant weak positive relationship between facial mimicry and empathy, but not facial emotion recognition. The moderator analysis revealed that stronger correlations between facial mimicry and empathy were observed for static vs. dynamic facial stimuli, and for implicit vs. explicit instances of facial emotion processing. No differences were seen between facial emotions, facial muscles, emotional and cognitive facets of empathy, or state and trait measures of empathy. The results support the claim that stronger facial mimicry responses are positively related to higher dispositions for empathy, but the weakness and variability of this effect suggest that this relationship is conditional on not-fully understood factors.
Facial expressions convey essential information for guiding social interactions. The sensitivity of humans to faces can be demonstrated behaviourally in our automatic tendency to imitate expressions of facial emotions, called facial mimicry. Research over the last 30 years has flagged many possible social functions and correlates of facial mimicry (e.g. Dimberg et al., Citation2000; Hess & Fischer, Citation2013; Meltzoff & Moore, Citation1997). One example, which has been formulated in different ways, is a relationship between facial mimicry and the sharing and understanding of the emotions of others; a capacity often referred to as empathy. In a similar manner, mimicking other people’s faces has been further linked to the ability to infer the emotions of others from faces, called facial emotion recognition (FER). Through these routes, facial mimicry has been broadly considered to capture a person’s sensitivity to the emotional meaning of faces. In this report, we tested this idea by conducting a meta-analysis of findings relating to variability in facial mimicry responses and capacities of empathy and FER across individuals.
Facial mimicry responses are subtle and difficult to observe visually, but can be sensitively indexed by electromyographically (EMG)-recorded activity in facial muscles, or by scoring of facial movements from video recordings using the Facial Action Coding System (FACS) (Ekman & Rosenberg, Citation2005; for further information see https://www.paulekman.com/facial-action-coding-system/). Two common examples of facial mimicry are smiling when seeing a happy face, and frowning when seeing an angry face, measured using EMG by placing sensors over smiling and frowning muscles respectively. As with imitation of other body parts (Chartrand & Bargh, Citation1999), facial mimicry has seen to be particularly elicited when interacting with people who are perceived positively, for whom there is a desire for social affiliation (Leighton et al., Citation2010; Likowski et al., Citation2008; *Sims et al., Citation2012).
A neural explanation for why faces and actions are automatically imitated comes from work on the mirror-neuron system (MNS; Rizzolatti et al., Citation1996); a set of brain regions activated both by the enactment and observation of motor actions. Evidence for this system in humans came from findings of spatially overlapping regions of increased brain activation during action execution and observation (Buccino et al., Citation2001; Decety et al., Citation1997). This bi-directional pathway between observation and action is argued to activate a representation of the motor action in the observer, potentiating its physical movement in the form of imitation. Some have argued that the imitation outputs of the MNS go beyond motor representations; activating a motor action’s representation also elicits some of the experiential states connected with that action, e.g. the intention of a hand movement, the emotion of a facial expression (Iacoboni et al., Citation2005; Preston & de Waal, Citation2002). The MNS accordingly provides a mechanism for how observing a person’s motor action can lead to the sharing of subjective states between individuals, and in turn, a useful reference for decoding the meaning of another person’s actions. This subjective insight into others offers a channel for motor imitation to provide inputs into empathy and FER.
As empathy has been defined in many ways, at this point it is helpful to make clear how it’s defined and segmented in this paper. First, its important to point out that researchers have tested links between facial mimicry and empathy using definitions of empathy that vary in their precision, some referring to specific constructs, others testing more general links. To reflect this variability, in this meta-analysis, we aimed to accommodate both broad and granular definitions. We use the term “compound empathy” as an umbrella term for all the subcomponents covered here. We also test links to specific components of empathy to allow for more granular interpretations. In segmenting these components, we take the recommendation of Hall and Schwartz (Citation2019) and use both measure-focused segmentations (state empathy versus trait empathy), as well as traditional conceptual segments (cognitive empathy versus emotional empathy).
The empathy component most often put forward as linked to facial mimicry is the automatic sharing of observed emotions, referred to as “emotional contagion” or emotional empathy, where the observer’s emotions increase in similarity to the expressor’s (Hatfield et al., Citation1994). Emotional empathy has been measured in two ways: using task-based “state” measures that track stimuli-induced emotional sharing responses, or questionnaire-based “trait” measures that index an individual’s self-reported tendencies to engage in everyday emotional sharing. Although state emotional empathy most directly traces the emotional sharing responses thought to be relevant to facial mimicry, trait empathy has also been shown to successfully capture inter-individual variation in emotional sharing responses (*Drimalla et al., Citation2019), suggesting emotional state and trait measures might overlap in the underlying empathy processes they track.
Emotional empathy is usually distinguished in theories from cognitive empathy, which involves the conscious understanding of others’ emotions or thoughts. This understanding does not necessitate but can co-occur with emotional contagion effects (Shamay-Tsoory, Citation2011). A typical questionnaire item measuring cognitive empathy is “I am good at understanding the feelings of others”. Note further that cognitive empathy has sometimes been used to refer to abilities of theory-of-mind or perspective taking, as measured by accuracy in performance-based lab tasks (Baron-Cohen & Wheelwright, Citation2004). Unlike trait measures, these performance-based constructs have not been explicitly claimed to involve spontaneous facial mimicry, and were therefore considered outside the current scope.
Facial emotion recognition (FER) differs from empathy in that it does not involve a subjective understanding of others’ emotional states, but only the perceptual ability categorise faces according to their emotional expressions. We decided to bring FER into the meta-analysis because it is thought to overlap extensively with emotional empathy in terms of its perceptual inputs (i.e. visual facial emotional stimuli), and cognitive empathy in terms of its inferential processes. Echoing this distinction of perceptual and inferential processes, FER has been conceptually segmented into corresponding automatic implicit and explicit facial processing routes. In the implicit route, emotional information is processed outside of awareness, and in the explicit route, conscious efforts are made to recognise the displayed emotions (Schirmer & Adolphs, Citation2017). While there is clear overlap in cognitive empathy and FER skills, these constructs have usually been kept separate due to the exclusive focus of FER on visual face perception, as opposed to cognitive empathy, where emotions are inferred based on a variety of cues, including e.g. speech and situational cues.
To outline the motivation for the current study, the following sections contain a brief description of the theory landscape relating facial mimicry, empathy, and FER: Perception-Action model, social context model, and SIMS model. The current study was not a formal test of any of these theories, but only addressed a specific assumption that is common to them.
A useful way to delineate these theories is how they differ in their claims of the causal direction, e.g. do facial mimicry responses affect empathy and FER, or vice-versa. In one version, often referred to as the “facial feedback” hypothesis (Buck, Citation1980), learned repeated associations between facial muscle configuration and affective states lead to the feedback from facial muscles when mimicking to re-activate that emotion in the observer (Hatfield et al., Citation2009). One popular method of testing the facial feedback hypothesis is via “facial blocking” tasks, where attempts are made to block the expression of facial emotions physically by asking participants to hold a pen in their mouth. Such blocking interventions are reported to reduce the recognition of facial emotions (Niedenthal et al., Citation2001), indicating a causal effect of the kind of facial movements elicited in facial mimicry on FER.
In contrast, the opposite causal relationship from social skills to facial movements has been argued. For instance, in Hess and Fischer (Citation2013), the meaning of facial expressions is first decoded, then its cognitive representation leads to its facial expression, which assuming a one-to-one matching of observed and interpreted emotion, a facial mimicry response is elicited. Supportive evidence from multiple findings that facial responses to emotional cues depend on their interpretation, not on their visual perception, as seen in reports that facial mimicry responses can be elicited by emotional auditory sounds and vocalisations (Hawk et al., Citation2012; Hietanen et al., Citation1998; Magnée et al., Citation2007).
Despite differing in causal direction, theories linking facial movements to the understanding of others, to varying degrees, share the assumption of a positive relationship between these constructs. The earliest such theory was proposed by the German philosopher Theodor Lipps (Citation1907), which was a formulation of the facial feedback hypothesis mentioned above. Next, the perception action model (PAM) claims that imitation responses, such as facial mimicry, can motivate altruism by enhancing personal investment in the emotions of others (de Waal & Preston, Citation2017; Preston & de Waal, Citation2002). In this account, imitation facilitates the reciprocal sharing of emotions between self and others via the MNS, and this contingency of one’s own emotions on those of others enhances concern for others’ well-being, motivating altruism for them. In the PAM, a positive relationship between emotional empathy and facial mimicry is directly posited. In line with the PAM’s view for the role of the MNS in empathy, a resent fMRI study by Oosterwijk et al. (Citation2017), observed that patterns of neural activity that coded for participants’ own emotional experiences (e.g. emotional actions, interoceptive sensations and situations) also coded for the understanding of others’ emotions.
Other models focus less on empathy and more on the modulation of facial mimicry by contextual cues for social affiliation, but these also imply a positive relationship between facial mimicry and empathy. The social context theory (as referred to here) argues that facial mimicry is regulated by affiliative signals, such as contexts that encourage adopting an affiliative “stance”, and serves to provide subtle signs of mutual intentions and emotions; an indicator of the potential quality of a future relationship (Hess & Fischer, Citation2014). Evidence for the importance of affiliative goals to regulating facial mimicry can be seen in a report from Likowski et al. (Citation2008), in which participants mimicked the faces of others more when experimentally manipulated to form positive vs. negative attitudes about them. The authors of the social context theory claim that facial mimicry does not itself induce emotional sharing, but often can co-occur with empathy when social affiliation goals are activated, as these motivate both imitation and empathy to engender social bonding. This view would therefore readily account for findings that stronger facial mimicry responses correspond with more intense demonstrations of emotional empathy, not causally, but incidentally.
Lastly, the simulation of smiles (SIMS) model (Niedenthal et al., Citation2010) deals specifically with mimicry of smiles, and whether they are interpreted as signals of an affiliative stance (e.g. mutual joy) or not (e.g. Schadenfreude). The SIMS claims that facial movements facilitates automatic empathic emotion sharing, and this sharing mechanism enhances understanding of others’ emotions (in the form of FER and perception of affiliative stance). The SIMS goes further by claiming that facial mimicry triggers the matching of facial movements, which through facial feedback, then leads to greater similarity in internal emotions. Like the facial feedback hypothesis, evidence for the SIMS comes from findings that blocking facial expressions of smiles disrupts the recognition of happy faces (Niedenthal et al., Citation2001; Oberman et al., Citation2007), and impairs the ability to assess the authenticity of smiles (Korb et al., Citation2014; Maringer et al., Citation2011; Rychlowska et al., Citation2014). It should be noted that there is currently some debate as to the reliability of facial blocking effects. Seventeen labs have failed to replicate a basic instance of this effect (Wagenmakers et al., Citation2016), but a recent meta-analysis from 138 studies reports evidence that small but stable effects do exist (Coles et al., Citation2019).
The above list of theories on social roles of facial mimicry is not intended to be exhaustive, but we used it to highlight the common thread of the assumption that facial mimicry responses have a positive relationship with empathy and FER. However, contrary to the pervasiveness of this claim, Heyes (Citation2011) has argued that empirical support for a link between imitation and empathy is lacking. A close inspection of the literature suggests that the evidence is indeed mixed (e.g. studies failing to find support for a relationship between facial mimicry and empathy include *Hess & Blairy, Citation2001; *Sims et al., Citation2012; *Sun et al., Citation2015), and requires synthesis into a coherent picture for accurately assessing support. To do this, we examined studies investigating co-variance between individuals’ facial mimicry responses to facial stimuli, and their scores on measures of empathy and FER. Summarising all these findings together would allow us to detect if true effects exist that might be too weak for detection in available sample sizes, or are only elicited under specific experimental conditions, e.g. choice of facial stimuli or instrument to measure empathy.
The current study conducted a meta-analysis to assess the available evidence for a correspondence between facial mimicry and empathy, both as a unitary construct (“compound empathy”) and by task-based and common conceptual segmentations, and FER. The inputs to the meta-analysis were effect sizes relating individual variability in these constructs. We also conducted a moderator analysis to identify any experimental factors that affect correlation effect sizes, including gender, muscle recorded, emotion label, how empathy was indexed (e.g. state vs. trait, emotional vs. cognitive subscales), and various stimuli properties (e.g. photos vs. videos, short vs. long stimulus exposures).
Methods and materials
Study search and inclusion criteria
The workflow of the meta-analysis followed the useful practical recommendations provided by Lakens et al. (Citation2016) and Quintana (Citation2015). Studies were searched for using the terms “facial” AND “mimicry” OR “imitation” AND “EMG” on Google Scholar and cross-checking references of reviews and studies. All studies reporting on facial mimicry data were inspected for suitability according to the following inclusion criteria. In addition, appeals for data were made on social networking platforms (Twitter, Facebook), and by email via listservs of psychological journals and organisations (e.g. ESSAN). First, studies had to involve EMG measurements of facial mimicry responses (as defined in Fridlund & Cacioppo, Citation1986), or observational protocols (e.g. FACS; https://imotions.com/blog/facial-action-coding-system/). Second, studies had to include one of the following measures:
Questionnaire measure of trait empathy (e.g. Interpersonal Reactivity Index, IRI; Davis, Citation1983).
State empathy measure, consisting either of a rating of the participant’s current state of valence/emotion in response to viewing a facial emotion, with higher empathy scores for ratings in the direction of the facial stimuli, or a rating of “how much did you enter into the other’s feelings?”.
FER accuracy measure for the correct labelling of emotions of facial stimuli, based either on selecting correct labels or matching stimuli, or making correct label-contingent responses e.g. happy vs. angry faces in Go-Nogo task.
Only data from non-clinical samples were included. In studies where pharmacological or brain stimulation intervention was used, effects were included from control comparison groups unless only effects that did not separate by group were reported, but the active condition did not differ significantly in terms of facial EMG or empathy/FER. If studies did not report a useable test statistic, it was requested by email from the author. Such unpublished findings from the so-called “grey literature” were only included if they were collected from studies that were peer-reviewed in published papers. If t or F statistics were reported for differences in facial mimicry between median split of high vs. low empathy groups were converted to r correlation coefficients. However, studies in which differences between empathy groups were artificially inflated by excluding participants in the mid-range were excluded. If possible, means and standard deviations were extracted from text or gleaned from figures to calculate missing test statistics. If Beta values were reported, these were converted to r using the formula from Peterson and Brown (Citation2005).
Effects were included that indexed facial mimicry as EMG changes when observing a target emotion relative to when observing neutral faces or a non-face baseline (e.g. fixation cross), or compared to facial emotions of the opposite valence (e.g. increased corrugator supercilli (CS) activity for angry compared to happy faces). Data from studies involving facial emotions that changed within trials (e.g. Offset task of *Korb et al., Citation2015) were excluded. Studies in which multiple emotion conditions (usually negative types) were collapsed into a single analysis were excluded to maximise sensitivity in the moderator analysis for separating these effects. For consistency with the majority of facial mimicry study designs, we opted to exclude some effects related to very rapid presentations of facial stimuli, with a minimum duration of 100 ms required for inclusion to ensure participants could reasonably recognise the facial emotion, based on finding that presentations longer than 100 ms do not hinder FER (Matsumoto et al., Citation2000). Stimuli presented during recording of facial mimicry that did not have a continuous display of a consistent facial emotion for repeated trials were excluded, e.g. single-trial affective movie clips where facial emotions were not constantly in focus. Since the focus of the meta-analysis was to test the assumption that natural occurrences of empathy are related to facial mimicry, which are generalisable to real-life behaviour, studies in participants were instructed by the experimenter to empathise were excluded (e.g. Balconi & Canavesio, Citation2013; Lamm et al., Citation2008).
Coding of moderators
Below are descriptions of the selected moderators, the rationale for their selection, and how they were coded and analysed. Since operationalisations of empathy widely differ, we considered the possibility that relationships with facial mimicry might be selective among them. However, since the results indicated no evidence of differences between them in correlations with facial mimicry responses, we included all of them (i.e. “compound empathy”) when examining all moderators excluding those where the different operationalisations was the focus of the moderator analysis.
The first moderator concerned which component was targeted by trait empathy questionnaires, emotional or cognitive. This included the “empathic concern” and “perspective taking” subscales of the IRI, and “emotional” and “cognitive” subscales of the Empathy Quotient (EQ; Baron-Cohen & Wheelwright, Citation2004), have been used to capture emotional and cognitive components of empathy respectively. Note that the Fantasy subscale of the IRI was excluded from all analyses due to consensus that this scale is not relevant to real-life empathic dispositions (Nomura & Akai, Citation2012). The Questionnaire Measure of Emotional Empathy (QMEE; Mehrabian & Epstein, Citation1972), and the Balanced Emotional Empathy Scale (BEES; Mehrabian, Citation1996) also target the emotional sharing aspect of emotional empathy.
Another moderator concerned the operationalisation of empathy as either a trait, in terms of personal everyday dispositions, or a state, in terms of specific momentary instances of emotional sharing. This question was examined by comparing emotional empathy trait measures with state empathy measures, thereby matching measures on the target empathy component of emotional empathy. Note: state measures track matching of emotional states, and are therefore considered to target emotional empathy.
Another way of separating facial mimicry paradigms is in terms of whether they relatively engage implicit vs. explicit routes of facial emotion processing. These routes can be selectively engaged in two ways, which were examined by separated moderators. First, shorter exposures of stimuli tend to depend on faster implicit routes of processing. This was coded in the moderator analysis as whether facial stimuli were shown for shorter or longer than 1 s durations. Second, designs of facial mimicry paradigms involve either passive viewing of faces during facial mimicry recording, without participants needing to respond to or infer any emotional information, or alternatively, participants were required to make some judgment on the emotional quality of the face e.g. label, valence, intensity. These passive vs. active conditions map, in relative terms, onto distinct routes of implicit vs. explicit of facial emotion processing.
In sum, the following nine categorical variables were chosen, coded for, and analysed in their moderating influence on effect sizes relating facial mimicry and empathy:
from which facial muscle were responses recorded;
which facial emotion were displayed;
whether visual facial stimuli were dynamic videos or static photos;
whether emotional empathy was measured as a trait or state;
whether the empathy measure was related to the emotional or cognitive component;
whether samples were exclusively female, male, or included both genders;
which specific instrument was used to index empathy (see for a list);
whether stimuli were presented for short (< 1 s) or long (> 1 s) durations.
whether emotional processing of faces was passive or active.
Table 1. Summary of studies submitted to meta-analysis.
Summary effect size analysis
The meta-analysis was conducted using the “MAc” and “metafor” packages, as implemented in R-project. We conducted our meta-analysis according to the steps outlined in Del Re (Citation2015) and Viechtbauer (Citation2010). All effect sizes that were not correlation coefficients were converted using functions in the “MAc” package. This package aggregates effect sizes within studies to eliminate the influence of dependencies between effects from the same study, e.g. between effect sizes involving total scores of questionnaires and scores of subscales. A summary effect size was then computed via a random-effects omnibus test (“mareg” function), as variability in effect sizes were assumed to be sensitive to differences in designs and sensitivity of instruments and not just due to sample variability as assumed by fixed-effects models (Borenstein et al., Citation2010).
To measure the amount of heterogeneity in our studies, we calculated the I2 (Higgins et al., Citation2003) and Tau-squared (τ2) statistics (Higgins, Citation2008). Meta-analyses comprising of heterogeneous studies indicate less shared effect (Quintana, Citation2015). To identify which studies excessively contribute to study heterogeneity in the summary effect size, we created a forest plot (Lewis & Clarke, Citation2001). Publication bias for reporting stronger effect sizes was examined visually using a funnel plot and then tested for asymmetry using Egger’s Regression Test (Egger et al., Citation1997).
Moderator analysis
Moderator analyses were conducted to identify factors that influenced effect sizes. In the moderator analysis we separately examined relationships between facial mimicy and compound empathy, and between facial mimicry and state empathy (the specific component/measure most often linked to facial mimicry). The numbers of studies reporting on effect sizes involving FER were deemed to be too few for the power required for a moderator analysis. Moderators of correlations between facial mimicry and the relevant operationalisation of empathy were analyzed in two ways. First, we tested if summary effect sizes were significant in each level separately by fitting a single mixed-effects model to each moderator level. For example, our moderator examining the effect of the emotion expressed by facial stimuli contained the levels angry, happy, etc. In this separate-level analysis, a significant p value for the model indicates that among effect size at a given level (e.g. happy), a significant relationship between facial mimicry and compound empathy was observed. Next, in a combined-level analysis, the difference between levels was tested, indicating whether the moderator had an overall influence on the summary effect size (e.g. differences between effect sizes of happy vs. angry), as indicated by the QM-statistic (QM) and its significance level (QMp).
Power analysis
Power analyses for meta-analysis are pivotal to accurate interpretation of results, particularly due to challenges of comparing effects from different studies, which can be highly heterogeneous, therefore requiring more studies for reliable comparison. Under conditions of low power (e.g. < 80%), the conclusion that there is absence or presence of an effect in a test should be avoided. Power calculations for the analysis of summary effect sizes were calculated using the macro for random-effects in meta-analysis from Quintana (Citation2015) (available at osf.io/5c7uz/). For the meta-analysis involving compound empathy (i.e. all operationalisations included), for an average sample size of 40 and 25 effects from different studies, and moderate heterogeneity between effects, there was 88% power to detect a small effect of r = 0.2, indicating adequate power. For the meta-analysis involving FER, for an average sample size of 40, 10 effects, and high heterogeneity, there was 29% power to detect a small effect of r = 0.2. Hence, given the smaller number of available studies on FER, a much larger effect was required to be reliably detected. As noted in the discussion section, this low power seriously limits the interpretability of results from the meta-analysis involving FER. However, we decided to proceed with the analysis for completeness of reporting.
Post-hoc power estimates for categorical moderator analyses using mixed-effects meta-regressions were calculated using a macro developed by Cafri et al. (Citation2009) implementing the formulae of Hedges and Pigott (Citation2004). The parameters for these calculations used the same average sample size as above (n = 40), a constant τ2 of .03 (a conservative approximation given the observed results), and the observed effect sizes. These resulted in similar power estimates for all moderators in the range of 52–59%.
Results
Descriptive summary of included studies
The literature search (finished on March, 2019) returned 44 studies. After exclusion criteria were applied, 28 studies reporting 162 effect sizes (k) remained (see for a brief summary and for an overview of the workflow for data collect, in line with PRISMA guidelines, Moher et al. Citation2009; for a detailed summary see the database in the online repository). The compound empathy set contained 128 effect sizes from 23 studies (average sample size = 38) and the FER set contained 33 effect sizes from 9 studies (average sample size = 50). None of the studies using FACS measurements of facial mimicry met inclusion criteria.
Summary effect size results
The random-effects omnibus test of effect sizes relating facial mimicry and compound empathy indicated a moderate significant effect (r = .188; 95% CI [.11, .26], p < .0001). The effect estimates per study are depicted visually with a forest plot in (separated for emotional trait and state empathy in ). The I2 estimate of heterogeneity was 24.8% (95% CI [0, 61.3]), representing a moderate but acceptable amount of true heterogeneity among studies (Higgins et al., Citation2003), and a τ2 of .008 (95% CI [0, .04]).
Figure 3. Forest plot of aggregated effect sizes between facial mimicry and emotional empathy from trait (A) and state (B) measures.
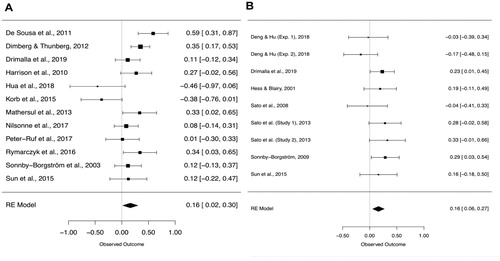
In the funnel plot of (A), the horizontal axis represents effect sizes from studies, the vertical axis shows the standard error of these effect sizes, and a triangular shaded area in which 95% of effects should be symmetrically distributed if no publication bias is present (Egger et al., Citation1997). Visual inspection of the funnel plot and the Egger’s Regression Test for asymmetry indicated no publication bias was present on reporting correlations between facial mimicry and compound empathy, although this was trending towards significance (z = −1.7, p = .09). On the vertical axis of the Baujat plot in (B) the standardised difference of the overall summary effect with and without each study is plotted, and the contribution of each study to the overall heterogeneity on the horizontal axis. Studies falling away from the mass of observations in a Baujat graph should be further investigated to understand and address the reasons of such simultaneously outstanding heterogeneity and influence. Visual inspection of the Baujat plot indicated that Study 4 (*de Sousa et al., Citation2011) had a major and possibly biasing contribution to the overall summary effect size. To investigate this, we conducted a sensitivity analysis by estimating the summary effect size without this study, and found it was still highly significant, r = .173, p < .0001. Close inspection of this study suggested its design was not unusual compared to other included studies, except that skin conductance was also recorded, but this was not considered problematic. For these reasons, the study was not removed.
Figure 4. A. Funnel plot of aggregated effect sizes between facial mimicry and compound empathy. B. Baujat plot of numbered effect sizes aggregated within studies.
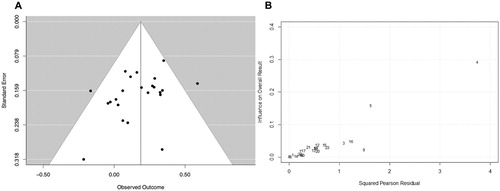
The random-effects omnibus test for effect sizes relating facial mimicry and FER indicated a non-significant effect (r = -.06; 95% CI [-.25, .13], p = .54). The I2 estimate of heterogeneity was 77.6% (95% CI [50, 93.7]), representing a very high amount of true heterogeneity among studies (Higgins et al., Citation2003), and a τ2 of .06 (95% CI [.02, .28]), as can be seen in the forest plot in . The Egger’s Regression Test was non-significant (z = −1.7, p = .09), which indicates that a publication bias was not present.
Moderator analysis results for facial mimicry and compound empathy
In the following results, n denotes number of included studies, k denotes number of included effect sizes. For the moderator analysis of muscle site, the separate-level analysis showed that the correlation between facial mimicry and compound empathy was significant for levels of CS (n = 18, k = 60, r = .12, p < .0001), LL (n = 3, k = 5, r = .2, p = .05) and ZM (n = 19, k = 49, r = .12, p = .0005), with no significant difference between them (QM = 1.62, p = .9).
For the moderator analysis of the displayed emotion of facial stimuli, the separate-level analysis indicated that the correlation between facial mimicry and compound empathy was significant for levels of anger (n = 15, k = 32, r = .12, p = .003), fear (n = 4, k = 13, r = .18, p = .006), happiness (n = 21, k = 51, r = .12, p = .0004), and sadness (n = 4, k = 10, r = .18, p = .009), but was non-significant for pain (n = 1, k = 8, r = .02, p = .86) or disgust (n = 3, k = 10, r = .1, p = .17). The combined-level analysis found no significant effect for this moderator (QM = 3.3, p = .65).
For the moderator analysis of whether facial stimuli were dynamic or static, the separate-level analysis showed that significant effect sizes were observed for both levels of dynamic (n = 14, k = 85, r = .08, p = .0007) and static stimuli types (n = 13, k = 42, r = ..2, p < .0001). The combined-level analysis indicated static facial stimuli have a significantly higher average effect size than dynamic stimuli (QM = 7.9, p = .005).
For the moderator analysis of cognitive empathy (measured by IRI and EQ subscales) vs. emotional empathy (measured by IRI and EQ subscales and sum scores of QMEE and BEES), the separate-level analysis showed that the correlation between facial mimicry and empathy was significant for both emotional empathy (n = 12, k = 36, r = .13, p = .001) and cognitive empathy (n = 8, k = 26, r = .16, p = .004). The combined-level analysis indicated no significant difference between these levels (QM = .22, p = .6).
For the analysis comparing state and trait measures of emotional empathy, a significant relationship was observed for both trait (n = 12, k = 36, r = .13, p = .001) and state empathy (n = 8, k = 26, r = .16, p = .0008), with no significant difference between these levels (QM = .14, p = .7).
For the moderator of participant gender on correlations between facial mimicry and compound empathy, the separate-level analysis showed a significant effect for female (n = 2, k = 8, r = .14, p = .03) but no significant effect for male participants (n = 2, k = 10, r = .06, p = .2), with no significant difference between these levels (QM = 1.13, p = .29).
For the moderator analysis of the specific empathy measure used, the separate-level analysis showed that significant effect sizes were observed for a number of both state and trait empathy measures (see for list of measures). The combined-level analysis indicated a significant difference between measures of trait empathy (QM = 26.1, p = .001).
Table 2. Results of moderator analysis between facial mimicry and selected empathy measures (QM indicates overall effect, r indicates effect size at each moderator level).
For the comparison of exposure times of facial stimuli, the separate-level analysis showed a significant effect size for studies using short exposure times below 1 s (n = 4, k = 17, r = .25, p < .0001), and for studies using longer exposure times of equal or longer than 1 s (n = 18, k = 109, r = .1, p < .0001), with shorter exposures times related to stronger correlations than longer exposure times (QM = 5.23, p = .02).
Effect sizes were compared from studies in which facial mimicry was passively recorded without requiring responses contingent on facial stimuli emotion, versus when they were (e.g. FER, valence/intensity ratings). The separate-level analysis showed a significant effect size for studies with passive task procedures (n = 15, k = 65, r = .18, p < .0001), as well as for studies requiring explicit responses to facial emotions (n = 8, k = 63, r = .06, p < .03), and this difference was significant (QM = 8.25, p = .004).
Moderator analysis results for facial mimicry and state empathy
For the moderator analysis of muscle site, the separate-level analysis showed that the correlation between facial mimicry and state empathy was significant for levels of CS (n = 6, k = 16, r = .16, p < .05), LL (n = 1, k = 1, r = .38, p < .05), and ZM (n = 6, k = 10, r = .15, p < .05), with no significant difference between them (QM = 2.5, p = .47).
For the moderator analysis of the displayed emotion of facial stimuli, the separate-level analysis indicated that the correlation between facial mimicry and state empathy was significant for levels of anger (n = 5, k = 9, r = .14, p = .047), disgust (n = 1, k = 1, r = .38, p = .045), happiness (n = 7, k = 11, r = .13, p = .037), and sadness (n = 2, k = 3, r = .23, p = .034), with no significant difference between them (QM = 2, p = .72).
For the moderator analysis of whether facial stimuli were dynamic or static, the separate-level analysis showed that significant effect sizes were observed for both levels of dynamic (n = 7, k = 14, r = .14, p = .0005) and static stimuli types (n = 4, k = 12, r = .18, p = .001), but no significant differences between these (QM = .3, p = .58).
Discussion
The current meta-analysis aimed to assess the empirical support for a link between individual dispositions for spontaneous facial mimicry to empathy and FER. For compound empathy (including all operationalisations), a significant but weak relationship was found, but no relationship was observed for FER, although fewer studies were available to robustly assess this relationship. Positive correlations between facial mimicry and compound empathy appeared to be driven equally by trait measures (of both emotional and cognitive empathy components) and state measures. Correlations varied between facial emotion displayed and recorded muscle, but these factors were not found to be influential overall. The sum finding is that people who exhibited stronger facial mimicry responses tended to have higher dispositions of trait empathy, and reported to share the emotional state of others to a greater degree in state empathy. This strengthens the position of several prominent theories of facial mimicry, all of which posit such a link. However, the summary effect size for this relationship was small and sensitive to numerous moderating factors, suggesting this relationship should not be considered as direct. Below we discuss the moderating factors identified in the meta-analysis, and outline some limitations and wider implications of the results.
Methodological limitations need to be considered for accurate interpretation of meta-analytic results, where data are aggregated from highly diverse sources. Our heterogeneity results showed a moderate amount of variance between studies reporting correlations between facial mimicry and compound empathy, suggesting some reliability in our estimate of summary effect size. However, there was a large degree of uncertainty in our estimate, which is expected given the relatively small sample of available studies and diversity of study designs (Ioannidis et al., Citation2007). Compared to the results from the main analysis offacial mimicry and compound empathy correlations which included a larger number of effect sizes, caution is encouraged for interpreting the results of the meta-analysis of FER, and also in some of the empathy moderator results, where fewer effect sizes were available. It should also be noted that the samples submitted to the meta-analysis included control samples from clinical studies, which are often recruited very selectively to fit within narrow ranges of “normal” (Schwartz & Susser, Citation2011). These samples are less likely to match the distributions of social and cognitive abilities in the wider popular, and could have biased the resulting estimation of the strength of relationship between facial mimicry and empathy.
The finding that FER accuracy was not significantly related to facial mimicry is contrary to claims of the SIMS model that feedback from facial muscles facilitates the decoding of facial emotions (e.g. Oberman et al., Citation2007), and is consistent with previous reports of no detected associations (e.g. Blairy, Herrera, & Hess, Citation1999; *Hess & Blairy, Citation2001). However, the restricted sample size of 9 studies warrants caution in interpreting this null result. A further possibility is that FER paradigms, as they stand, suffer from poor sensitivity to detect variability between individuals. Test-retest reliability of a standard FER task indicated moderate reliability (Adams et al., Citation2016). However, as a relatively simple task, it is susceptible to ceiling effects that compress ranges of individual variance. For example, error rates in *McDonald et al. (Citation2011) were lower than 8%. Lastly, this null finding could also reflect the complexity of FER as a skill that relies on the interplay of more distributed cognitive capacities that build on and go beyond those involved in emotional empathy (e.g. higher-level inferences), leaving facial mimicry to play a less central role.
In the following sections we explore the results of the moderator analyses between facial mimicry and empathy (total and specific operationalisations), beginning with the most basic moderators for facial mimicry data; the specific muscle recorded and facial emotion displayed. No difference was found between the recorded muscle sites, suggesting that facial mimicry is not related to compound empathy in a clear emotion-specific way. For the moderator of facial emotion, the summary effect size of correlations with compound empathy were strongest for sad and fearful faces, followed by the most commonly studied emotions of happiness and anger, but no significant difference was observed across emotions. Note that the number of effect sizes involving fear and sadness was far fewer than those relating to happiness or anger, and thus these summary effect sizes are less likely to be as reliable. In light of this, the effect of facial emotion could be considered small and an indicator that facial mimicry of anger, happiness, fear, and sadness, are all equally associated with empathy, with non-significant correlations for pain and disgust.
The weak effect of pain is perhaps surprising, as responses to observing pain in others (i.e. the potentiation of motor threat reflexes) is considered a gold standard for indexing the empathic sharing of the states of others (Avenanti et al., Citation2005). However, it has been argued that empathic responses to others’ pain can be clouded on the affective level by the simultaneous responses of personal distress to seeing an upsetting aversive scene of someone in pain (Batson et al., Citation1983; Hein & Singer, Citation2008), interfering with the measurement of empathic responses to others’ pain.
It has been suggested that facial mimicry of angry faces might not necessarily indicate an imitation or sharing of emotions, but instead be a reaction of irritation to the threatening angry face (Hess & Fischer, Citation2014). In addition, angry faces are less likely to be mimicked, which is taken to indicate that facial emotions that do not have social affiliative intent are not mimicked (Bourgeois & Hess, Citation2008). Since the level of facial mimicry was not examined, our analysis cannot speak to this latter point. We did observe that facial mimicry responses to angry faces were at least as strongly related to both total and emotional state empathy as those related to happy faces. This indicates that any facial mimicry present did appear to coincide with empathic responses. However, because of the way state empathy is usually measured (rating the current experience of the emotion recently observed in others), a person indicating feeling angry in response to seeing an angry face might not be due to feelings of the anger of the other (proper empathic sharing), but feeling anger to the other (a threat response).
Another moderator, and one that bears directly on theories of facial mimicry’s social functions, was the examination of which subcomponent of empathy was most strongly related to facial mimicry. Significant correlations with facial mimicry were found for both emotional and cognitive empathy components, with no differences in the strength of these correlations. This null finding has a number of possible interpretations, two of which are: (a) links between facial mimicry and empathy are not limited to emotional sharing (Hatfield et al., Citation2009), as thought to occur via activation of the MNS, but extent to higher cognitive empathy abilities also, (b) facial mimicry is primarily related to emotional sharing, but these also provide inputs to higher cognitive abilities, leading to a correspondence between facial mimicry and cognitive empathy abilities.
In addition, tendencies to share the emotions of others, as captured by trait emotional empathy and state empathy, were both highly significantly related to facial mimicry, with neither more highly related than the other. This suggests some equivalence in trait and state approaches to measuring emotional empathy in terms of their ability to track emotional sharing via automatic imitative responses.
A major consideration when designing a facial mimicry experiment is whether to use static photos or dynamic video-clips of facial emotions. Here, both stimulus types were found to significantly elicit correlations between facial mimicry and compound empathy. Surprisingly, the current results indicated that static faces elicit stronger correlations with compound empathy than dynamic ones. No difference between static and dynamic was found among state empathy measures. The interpretation of the stronger correlations with compound empathy is made difficult by many confounding factors. For example, the peak of emotional intensity of facial stimuli is more likely to be displayed longer for static stimuli, as the peak is presented from stimulus onset, whereas for dynamic stimuli faces usually begin from a neutral standpoint. Studies are needed to control for these factors to systematically disentangle effects caused by the numerous differences between static and dynamic stimuli, besides just ecological validity. For instance, it is possible that because of dynamic stimuli’s documented ability to elicited stronger facial mimicry responses (Rymarczyk et al., Citation2011; *Rymarczyk et al., Citation2016; *Sato et al., Citation2008; Weyers et al., Citation2006), this high level of responding might lead to ceiling levels in facial mimicry responses that suppress individual variability, and the ability to detect correlations with compound empathy.
The moderator analyses of differences among empathy measures indicated that correlations with facial mimicry were significantly stronger for some measures of trait empathy compared to others. Correlations were strongest for the BEES, EQ-affective, and QMEE, notably all measures of emotional empathy. However, since the number of instances of other empathy measures were far fewer, this analysis needs to be repeated in the future with balanced samples of empathy measures to accurately infer their relative sensitivities to facial mimicry. From this moderator analysis of empathy measures, we could also observe trends on the specificity or redundancy of empathy measure subscales. For instance, for the EQ, we could see that the subscale of emotional empathy correlated more strongly with facial mimicry than either the cognitive empathy subscale, or the total EQ score. This indicates that for indexing aspects of empathy related to facial mimicry, the emotional empathy subscale of the EQ outperforms any and all of the other subscales in this instrument.
The moderating influence of gender was examined on correlations between facial mimicry and compound empathy. As summarised by *Korb et al. (Citation2015),
Women are more emotionally expressive and more empathic than men (Eisenberg & Lennon, Citation1983; Kring & Gordon, Citation1998); more accurate and/or efficient in processing facial expressions of emotion in reduced and context rich settings (Hall, Citation1978; Hall & Matsumoto, Citation2004; Wacker et al., Citation2017; Hoffmann et al., Citation2010); show more facial mimicry than men (Dimberg & Lundquist, Citation1990); and are more susceptible to emotional contagion, as revealed both in self-report and dyadic interaction (Doherty et al., Citation1995)
The moderator analysis revealed that stronger correlations between facial mimicry and empathy were observed for study designs involving both briefer exposures of facial stimuli, and in which participants were not required to make explicit responses to facial emotions. This latter finding that explicit inference demands attenuate automatic imitation responses is, to our knowledge, novel, and warrants investigation by future studies. Taken together, these findings suggest that implicit routes are not only sufficient to instantiate the link between facial mimicry and compound empathy, but increased engagement of explicit routes appears to weaken this link. As a “spontaneous” response, it is well documented that automatic processing is sufficient to elicit facial mimicry responses (Dimberg et al., Citation2000). Directly corroborating evidence that more implicit routes are involved in links between facial mimicry and empathy come from one of the earliest reports of a correlation between these constructs. Sonnby-Borgström (Citation2002) rapidly presented facial emotions on the threshold of attention and found that compared to when viewing longer presentations, the relationship between facial mimicry and compound empathy was stronger, fitting with the current set of results. However, the question of why this is so has yet to be explored.
One key issue not addressed so far is the extent to which facial mimicry responses can be actually characterised as individual disposition that vary reliably across people; an implicit assumption of the idea that they relate to other dispositions, such as empathy. The test-retest reliability of EMG-measured facial mimicry was examined by Hess et al. (Citation2017) by estimating its stability in a large group of people across a 15 and 24 month period. Indications of good temporal stability was reported. Interestingly, stability varied depending on study design factors such as mode of presentation, more so than on the type of emotion eliciting the facial reaction. These results support the basic premise that facial mimicry responses are a reliable personal trait, and highlight the additional importance of factors that have not yet been sufficiently considered, including some that are not intrinsically emotional or social.
The primary aim of the current meta-analysis was to examine the relationship between facial mimicry and the sharing and understanding of others’ emotional states in terms of empathy and FER. The results showed a weak relationship between facial mimicry and all considered operationalisations of empathy, providing key support for a number of prominent theories claiming mechanistic links between facial mimicry and empathy, but the evidence for such a link with FER is inconclusive. While a relationship was detected, its low strength and inconsistency warrant caution in over-assuming an inseparable coupling of facial mimicry responses and empathy capacities, and encourage further work in this area to disentangle how this relationship is moderated by social and experimental contexts. By summarising the empirical evidence and moderating effects of design factors, and making available data from both the published and unpublished literature, we hope this work provides a useful resource for future investigations and theoretical formulations of the social functions of facial mimicry.
Acknowledgements
We thank all the authors whose work contributed to the meta-analysis, and especially those who took the time to respond to our requests for data.
Disclosure statement
No potential conflict of interest was reported by the author(s).
References
- Adams, T., Pounder, Z., Preston, S., Hanson, A., Gallagher, P., Harmer, C. J., & McAllister-Williams, R. H. (2016). Test-retest reliability and task order effects of emotional cognitive tests in healthy subjects. Cognition and Emotion, 30(7), 1247–1259. https://doi.org/10.1080/02699931.2015.1055713
- *Argaud, S., Delplanque, S., Houvenaghel, J. F., Auffret, M., Duprez, J., Vérin, M., Sauleau, P., Grandjean, D., & Sauleau, P. (2016). Does facial amimia impact the recognition of facial emotions? An EMG study in Parkinson’s Disease. PLoS ONE, 11(7), e0160329. https://doi.org/10.1371/journal.pone.0160329
- Avenanti, A., Bueti, D., Galati, G., & Aglioti, S. M. (2005). Transcranial magnetic stimulation highlights the sensorimotor side of empathy for pain. Nature Neuroscience, 8(7), 955–960. https://doi.org/10.1038/nn1481
- *Bailey, P. E., Henry, J. D., & Nangle, M. R. (2009). Electromyographic evidence for age-related differences in the mimicry of anger. Psychology and Aging, 24(1), 224–229. https://doi.org/10.1037/a0014112
- Balconi, M., & Canavesio, Y. (2013). Emotional contagion and trait empathy in prosocial behavior in young people: The contribution of autonomic (facial feedback) and Balanced emotional empathy Scale (BEES) measures. Journal of Clinical and Experimental Neuropsychology, 35(1), 41–48. https://doi.org/10.1080/13803395.2012.742492
- Baron-Cohen, S., & Wheelwright, S. (2004). The empathy Quotient: An investigation of adults with Asperger Syndrome or high functioning Autism, and normal sex differences. Journal of Autism and Developmental Disorders, 34(2), 163–175. https://doi.org/10.1023/B:JADD.0000022607.19833.00
- Batson, C. D., O'Quin, K., Fultz, J., Vanderplas, M., & Isen, A. M. (1983). Influence of self-reported distress and empathy on egoistic versus altruistic motivation to help. Journal of Personality and Social Psychology, 45(3), 706–718. https://doi.org/10.1037/0022-3514.45.3.706.
- Blairy, S, Herrera, P, & Hess, U. (1999). Mimicry and the judgment of emotional facial expressions. Journal of Nonverbal Behavior, 23(1), 5–41. doi: 10.1023/A:1021370825283
- Borenstein, M., Hedges, L. V., Higgins, J. P. T., & Rothstein, H. R. (2010). A basic introduction to fixed-effect and random-effects models for meta-analysis. Research Synthesis Methods, 1(2), 97–111. https://doi.org/10.1002/jrsm.12
- Bourgeois, P., & Hess, U. (2008). The impact of social context on mimicry. Biological Psychology, 77(3), 343–352. https://doi.org/10.1016/j.biopsycho.2007.11.008
- Buccino, G., Binkofski, F., Fink, G. R., Fadiga, L., Fogassi, L., Gallese, V., & Freund, H. J. (2001). Action observation activates premotor and parietal areas in a somatotopic manner: An fMRI study. The European Journal of Neuroscience, 13(2), 400–404. https://doi.org/10.1111/j.1460-9568.2001.01385.x.
- Buck, R. (1980). Nonverbal behavior and the theory of emotion: The facial feedback hypothesis. Journal of Personality and Social Psychology, 38(5), 811–824. https://doi.org/10.1037/0022-3514.38.5.811
- Cafri, G., Kromrey, J. D., & Brannick, M. T. (2009). A SAS macro for statistical power calculations in meta-analysis. Behavior Research Methods, 41(1), 35–46. https://doi.org/10.3758/BRM.41.1.35
- *Chakraborty, A., & Chakrabarti, B. (2015). Is it me? Self-recognition bias across sensory modalities and its relationship to autistic traits. Molecular Autism, 6(1), https://doi.org/10.1186/s13229-015-0016-1
- Chartrand, T. L., & Bargh, J. A. (1999). The chameleon effect: The perception-behavior link and social interaction. Journal of Personality and Social Psychology, 76(6), 893–910. https://doi.org/10.1037/0022-3514.76.6.893
- Codispoti, M., Surcinelli, P., & Baldaro, B. (2008). Watching emotional movies: Affective reactions and gender differences. International Journal of Psychophysiology, 69(2), 90–95. https://doi.org/10.1016/j.ijpsycho.2008.03.004
- Coles, N. A., Larsen, J. T., & Lench, H. C. (2019). A meta-analysis of the facial feedback literature: Effects of facial feedback on emotional experience are small and variable. Psychological Bulletin. [Advance online publication] https://doi.org/10.1037/bul0000194
- Davis, M. H. (1983). Measuring individual differences in empathy: Evidence for a multidimensional approach. Journal of Personality and Social Psychology, 44(1), 113–126. https://doi.org/10.1037/0022-3514.44.1.113
- Decety, J., Grèzes, J., Costes, N., Perani, D., Jeannerod, M., Procyk, E., … Fazio, F. (1997). Brain activity during observation of actions. Influence of action content and subject’s strategy. Brain, 120(Pt 10), 1763–1777. https://doi.org/10.1093/brain/120.10.1763
- Del Re, A. C. (2015). A practical tutorial on conducting meta-analysis in R. The Quantitative Methods for Psychology, 11(1), 37–50. https://doi.org/10.20982/tqmp.11.1.p037
- *Deng, Y., Chang, L., Yang, M., Huo, M., & Zhou, R. (2016). Gender differences in emotional response: Inconsistency between experience and expressivity. PLoS ONE, 11(6), e0158666. https://doi.org/10.1371/journal.pone.0158666
- *Deng, Y., & Hu, P. (2017). Matching your face or appraising the situation: Two paths to emotional contagion. Frontiers in Psychology, 8, 2278. https://doi.org/10.3389/fpsyg.2017.02278
- *de Sousa, A., McDonald, S., Rushby, J., Li, S., Dimoska, A., & James, C. (2011). Understanding deficits in empathy after traumatic brain injury: The role of affective responsivity. Cortex, 47(5), 526–535. https://doi.org/10.1016/j.cortex.2010.02.004
- de Waal, F. B. M., & Preston, S. D. (2017). Mammalian empathy: Behavioural manifestations and neural basis. Nature Reviews Neuroscience, 18(8), 498–509. https://doi.org/10.1038/nrn.2017.72
- Dimberg, U., & Lundquist, L.-O. (1990). Gender differences in facial reactions to facial expressions. Biological Psychology, 30(2), 151–159. https://doi.org/10.1016/0301-0511(90)90024-Q
- Dimberg, U., Thunberg, M., & Elmehed, K. (2000). Unconscious facial reactions to emotional facial expressions. Psychological Science, 11(1), 86–89. https://doi.org/10.1111/1467-9280.00221
- *Dimberg, U., & Thunberg, M. (2012). Empathy, emotional contagion, and rapid facial reactions to angry and happy facial expressions: Empathy and rapid facial reactions. PsyCh Journal, 1(2), 118–127. https://doi.org/10.1002/pchj.4
- Doherty, R. W., Orimoto, L., Singelis, T. M., Hatfield, E., & Hebb, J. (1995). Emotional contagion: Gender and occupational differences. Psychology of Women Quarterly, 19(3), 355–371. https://doi.org/10.1111/j.1471-6402.1995.tb00080.x
- *Drimalla, H., Landwehr, N., Hess, U., & Dziobek, I. (2019). From face to face: The contribution of facial mimicry to cognitive and emotional empathy. Cognition and Emotion, https://doi.org/10.1080/02699931.2019.1596068
- Egger, M., Davey Smith, G., Schneider, M., & Minder, C. (1997). Bias in meta-analysis detected by a simple, graphical test. BMJ, 315(7109), 629–634. https://doi.org/10.1136/bmj.315.7109.629
- Eisenberg, N., & Lennon, R. (1983). Sex differences in empathy and related capacities. Psychological Bulletin, 94(1), 100–131. https://doi.org/10.1037/0033-2909.94.1.100
- Ekman, P., & Rosenberg, E. L. (2005). What the face RevealsBasic and applied studies of spontaneous expression using the facial action coding system (FACS). Oxford University Press.
- Fridlund, A. J., & Cacioppo, J. T. (1986). Guidelines for human electromyographic research. Psychophysiology, 23(5), 567–589. https://doi.org/10.1111/j.1469-8986.1986.tb00676.x
- Hall, J. A. (1978). Gender effects in decoding nonverbal cues. Psychological Bulletin, 85(4), 845–857. https://doi.org/10.1037/0033-2909.85.4.845
- Hall, J. A., & Matsumoto, D. (2004). Gender differences in judgments of multiple emotions from facial expressions. Emotion, 4(2), 201–206. https://doi.org/10.1037/1528-3542.4.2.201
- Hall, J. A., & Schwartz, R. (2019). Empathy present and future. The Journal of Social Psychology, 159(3), 225–243. https://doi.org/10.1080/00224545.2018.1477442
- *Harrison, N. A., Morgan, R., & Critchley, H. D. (2010). From facial mimicry to emotional empathy: A role for norepinephrine? Social Neuroscience, 5(4), 393–400. https://doi.org/10.1080/17470911003656330
- Hatfield, E., Cacioppo, J. T., & Rapson, R. L. (1994). Emotional contagion. Cambridge University Press . Editions de la Maison des sciences de l’homme.
- Hatfield, E., Rapson, R. L., & Yen-Chi, L. (2009). Emotional contagion and empathy. MIT Press.
- Hawk, S. T., Fischer, A. H., & Van Kleef, G. A. (2012). Face the noise: Embodied responses to nonverbal vocalizations of discrete emotions. Journal of Personality and Social Psychology, 102(4), 796–814. https://doi.org/10.1037/a0026234
- Hedges, L. V., & Pigott, T. D. (2004). The power of statistical tests for moderators in meta-analysis. Psychological Methods, 9(4), 426–445. https://doi.org/10.1037/1082-989X.9.4.426
- Hein, G., & Singer, T. (2008). I feel how you feel but not always: The empathic brain and its modulation. Current Opinion in Neurobiology, 18(2), 153–158. https://doi.org/10.1016/j.conb.2008.07.012
- Hess, U., Arslan, R., Mauersberger, H., Blaison, C., Dufner, M., Denissen, J. J. A., & Ziegler, M. (2017). Reliability of surface facial electromyography. Psychophysiology, 54(1), 12–23. https://doi.org/10.1111/psyp.12676
- Hess, U., & Fischer, A. (2013). Emotional mimicry as social regulation. Personality and Social Psychology Review, 17(2), 142–157. https://doi.org/10.1177/1088868312472607
- Hess, U., & Fischer, A. (2014). Emotional mimicry: Why and when we mimic emotions: Emotional mimicry. Social and Personality Psychology Compass, 8(2), 45–57. https://doi.org/10.1111/spc3.12083
- *Hess, U., & Blairy, S. (2001). Facial mimicry and emotional contagion to dynamic emotional facial expressions and their influence on decoding accuracy. International Journal of Psychophysiology, 40(2), 129–141. https://doi.org/10.1016/S0167-8760(00)00161-6
- Heyes, C. (2011). Automatic imitation. Psychological Bulletin, 137(3), 463–483. https://doi.org/10.1037/a0022288
- Hietanen, J. K., Surakka, V., & Linnankoski, I. (1998). Facial electromyographic responses to vocal affect expressions. Psychophysiology, 35(5), 530–536. https://doi.org/10.1017/S0048577298970445
- Higgins, J. P. T. (2008). Commentary: Heterogeneity in meta-analysis should be expected and appropriately quantified. International Journal of Epidemiology, 37(5), 1158–1160. https://doi.org/10.1093/ije/dyn204
- Higgins, J. P. T., Thompson, S. G., Deeks, J. J., & Altman, D. G. (2003). Measuring inconsistency in meta-analyses. BMJ, 327(7414), 557–560. https://doi.org/10.1136/bmj.327.7414.557
- Hoffmann, H., Kessler, H., Eppel, T., Rukavina, S., & Traue, H. C. (2010). Expression intensity, gender and facial emotion recognition: Women recognize only subtle facial emotions better than men. Acta Psychologica, 135(3), 278–283. https://doi.org/10.1016/j.actpsy.2010.07.012
- *Hua, A. Y., Sible, I. J., Perry, D. C., Rankin, K. P., Kramer, J. H., Miller, B. L., Rosen H. J., & Sturm, V. E. (2018). Enhanced positive emotional Reactivity Undermines empathy in Behavioral Variant Frontotemporal Dementia. Frontiers in Neurology, 9, 402. https://doi.org/10.3389/fneur.2018.00402
- *Hühnel, I., Fölster, M., Werheid, K., & Hess, U. (2014). Empathic reactions of younger and older adults: No age related decline in affective responding. Journal of Experimental Social Psychology, 50, 136–143. https://doi.org/10.1016/j.jesp.2013.09.011
- Iacoboni, M., Molnar-Szakacs, I., Gallese, V., Buccino, G., Mazziotta, J. C., & Rizzolatti, G. (2005). Grasping the intentions of others with one’s own mirror neuron system. PLoS Biology, 3(3), e79. https://doi.org/10.1371/journal.pbio.0030079
- Ioannidis, J. P. A., Patsopoulos, N. A., & Evangelou, E. (2007). Uncertainty in heterogeneity estimates in meta-analyses. BMJ, 335(7626), 914–916. https://doi.org/10.1136/bmj.39343.408449.80
- Korb, S., With, S., Niedenthal, P., Kaiser, S., & Grandjean, D. (2014). The perception and mimicry of facial movements Predict Judgments of Smile authenticity. PLoS ONE, 9(6), e99194. https://doi.org/10.1371/journal.pone.0099194
- *Korb, S., Malsert, J., Rochas, V., Rihs, T. A., Rieger, S. W., Schwab, S., Niedenthal P. M., & Grandjean, D. (2015). Gender differences in the neural network of facial mimicry of smiles–An rTMS study. Cortex, 70, 101–114. https://doi.org/10.1016/j.cortex.2015.06.025
- Kring, A. M., & Gordon, A. H. (1998). Sex differences in emotion: Expression, experience, and physiology. Journal of Personality and Social Psychology, 74(3), 686–703. https://doi.org/10.1037/0022-3514.74.3.686
- *Künecke, J., Hildebrandt, A., Recio, G., Sommer, W., & Wilhelm, O. (2014). Facial EMG responses to emotional expressions are related to emotion perception ability. PLoS ONE, 9(1), e84053. https://doi.org/10.1371/journal.pone.0084053
- Lakens, D., Hilgard, J., & Staaks, J. (2016). On the reproducibility of meta-analyses: Six practical recommendations. BMC Psychology, 4(1), https://doi.org/10.1186/s40359-016-0126-3
- Lamm, C., Portes, E. C., Cacioppo, J., & Decety, J. (2008). Perspective taking is associated with specific facial responses during empathy for pain. Brain Research, 1227, 153–161. https://doi.org/10.1016/j.brainres.2008.06.066
- Leighton, J., Bird, G., Orsini, C., & Heyes, C. (2010). Social attitudes modulate automatic imitation. Journal of Experimental Social Psychology, 46(6), 905–910. https://doi.org/10.1016/j.jesp.2010.07.001
- Lewis, S., & Clarke, M. (2001). Forest plots: Trying to see the wood and the trees. BMJ, 322(7300), 1479–1480. https://doi.org/10.1136/bmj.322.7300.1479
- Likowski, K. U., Mühlberger, A., Seibt, B., Pauli, P., & Weyers, P. (2008). Modulation of facial mimicry by attitudes. Journal of Experimental Social Psychology, 44(4), 1065–1072. https://doi.org/10.1016/j.jesp.2007.10.007
- Lipps, T. (1907). Das wissen von fremden ichen. Psychologischen Untersuchungen, 1, 694–722.
- Magnée, M. J. C. M., Stekelenburg, J. J., Kemner, C., & de Gelder, B. (2007). Similar facial electromyographic responses to faces, voices, and body expressions. Neuroreport, 18(4), 369–372. https://doi.org/10.1097/WNR.0b013e32801776e6
- Maringer, M., Krumhuber, E. G., Fischer, A. H., & Niedenthal, P. M. (2011). Beyond smile dynamics: Mimicry and beliefs in judgments of smiles. Emotion, 11(1), 181–187. https://doi.org/10.1037/a0022596
- *Mathersul, D., McDonald, S., & Rushby, J. A. (2013). Automatic facial responses to briefly presented emotional stimuli in autism spectrum disorder. Biological Psychology, 94(2), 397–407. https://doi.org/10.1016/j.biopsycho.2013.08.004
- Matsumoto, D., LeRoux, J., Wilson-Cohn, C., Raroque, J., Kooken, K., Ekman, P., Yrizarry N., Loewinger S., Uchida H., Yee A., Amo L., & Goh, A. (2000). A new test to measure emotion recognition ability: Matsumoto and Ekman’s Japanese and Caucasian brief affect recognition test (JACBART). Journal of Nonverbal Behavior, 24(3), 179–209. https://doi.org/10.1023/A:1006668120583
- *McDonald, S., Li, S., De Sousa, A., Rushby, J., Dimoska, A., James, C., & Tate, R. L. (2011). Impaired mimicry response to angry faces following severe traumatic brain injury. Journal of Clinical and Experimental Neuropsychology, 33(1), 17–29. https://doi.org/10.1080/13803391003761967
- Mehrabian, A. (1996). Manual for the balanced emotional empathy scale (BEES).
- Mehrabian, A., & Epstein, N. (1972). A measure of emotional empathy. Journal of Personality, 40(4), 525–543. https://doi.org/10.1111/j.1467-6494.1972.tb00078.x
- Meltzoff, A. N., & Moore, M. K. (1997). Explaining facial imitation: A theoretical model. Early Development and Parenting, 6(3–4), 179–192. https://doi.org/10.1002/(SICI)1099-0917(199709/12)6:3/4<179::AID-EDP157>3.0.CO;2-R doi: 10.1002/(SICI)1099-0917(199709/12)6:3/4<179::AID-EDP157>3.0.CO;2-R
- Moher, D., Liberati, A., Tetzlaff, J., Altman, D. G., & The, P. G. (2009). Preferred reporting items for systematic reviews and meta-analyses: The PRISMA Statement. PLoS Medicine, 6(7), e1000097. https://doi.org/10.1371/journal.pmed.1000097
- Niedenthal, P. M., Brauer, M., Halberstadt, J. B., & Innes-Ker, ÅH. (2001). When did her smile drop? Facial mimicry and the influences of emotional state on the detection of change in emotional expression. Cognition & Emotion, 15(6), 853–864. https://doi.org/10.1080/02699930143000194
- Niedenthal, P. M., Mermillod, M., Maringer, M., & Hess, U. (2010). The simulation of smiles (SIMS) model: Embodied simulation and the meaning of facial expression. Behavioral and Brain Sciences, 33(6), 417–433. https://doi.org/10.1017/S0140525X10000865
- *Nilsonne, G., Tamm, S., Golkar, A., Sörman, K., Howner, K., Kristiansson, M., Olsson A., Ingvar M., & Petrovic, P. (2017). Effects of 25 mg oxazepam on emotional mimicry and empathy for pain: A randomized controlled experiment. Royal Society Open Science, 4(3), 160607. https://doi.org/10.1098/rsos.160607
- Nomura, K., & Akai, S. (2012). Empathy with fictional stories: Reconsideration of the Fantasy scale of the Interpersonal Reactivity index. Psychological Reports, 110(1), 304–314. https://doi.org/10.2466/02.07.09.11.PR0.110.1.304-314
- Oberman, L. M., Winkielman, P., & Ramachandran, V. S. (2007). Face to face: Blocking facial mimicry can selectively impair recognition of emotional expressions. Social Neuroscience, 2(3–4), 167–178. https://doi.org/10.1080/17470910701391943
- Oosterwijk, S., Snoek, L., Rotteveel, M., Barrett, L. F., & Scholte, H. S. (2017). Shared states: Using MVPA to test neural overlap between self-focused emotion imagery and other-focused emotion understanding. Social Cognitive and Affective Neuroscience, 12(7), 1025–1035. https://doi.org/10.1093/scan/nsx037
- *Peter-Ruf, C., Kirmse, U., Pfeiffer, S., Schmid, M., Wilhelm, F. H., & In-Albon, T. (2017). Emotion Regulation in high and Low Socially Anxious individuals: An experimental study investigating emotional mimicry, emotion recognition, and self-reported emotion Regulation. Journal of Depression and Anxiety Disorders, 1(1), 17–26.
- Peterson, R. A., & Brown, S. P. (2005). On the use of Beta coefficients in meta-analysis. Journal of Applied Psychology, 90(1), 175–181. https://doi.org/10.1037/0021-9010.90.1.175
- Preston, S. D., & de Waal, F. B. M. (2002). Empathy: Its ultimate and proximate bases. Behavioral and Brain Sciences, 25(1), 1–20. -71. https://doi.org/10.1017/S0140525X02000018
- Quintana, D. S. (2015). From pre-registration to publication: A non-technical primer for conducting a meta-analysis to synthesize correlational data. Frontiers in Psychology, 6), https://doi.org/10.3389/fpsyg.2015.01549
- *Rehberger, C. (2014). Examining the relationships between empathy, mood, and facial mimicry. DePaul Discoveries, 3(1), 5.
- Rizzolatti, G., Fadiga, L., Gallese, V., & Fogassi, L. (1996). Premotor cortex and the recognition of motor actions. Cognitive Brain Research, 3(2), 131–141. https://doi.org/10.1016/0926-6410(95)00038-0
- Rychlowska, M., Cañadas, E., Wood, A., Krumhuber, E. G., Fischer, A., & Niedenthal, P. M. (2014). Blocking mimicry makes true and false smiles look the same. PLoS ONE, 9(3), e90876. https://doi.org/10.1371/journal.pone.0090876
- Rymarczyk, K., Biele, C., Grabowska, A., & Majczynski, H. (2011). EMG activity in response to static and dynamic facial expressions. International Journal of Psychophysiology, 79(2), 330–333. https://doi.org/10.1016/j.ijpsycho.2010.11.001
- *Rymarczyk, K., Żurawski, Ł, Jankowiak-Siuda, K., & Szatkowska, I. (2016). Do dynamic compared to static facial expressions of happiness and anger reveal enhanced facial mimicry? PLoS ONE, 11(7), e0158534. https://doi.org/10.1371/journal.pone.0158534
- *Sato, W., Fujimura, T., Kochiyama, T., & Suzuki, N. (2013). Relationships among facial mimicry, emotional experience, and emotion recognition. PLoS ONE, 8(3), e57889. https://doi.org/10.1371/journal.pone.0057889
- *Sato, W., Fujimura, T., & Suzuki, N. (2008). Enhanced facial EMG activity in response to dynamic facial expressions. International Journal of Psychophysiology, 70(1), 70–74. https://doi.org/10.1016/j.ijpsycho.2008.06.001
- *Scarpazza, C., Làdavas, E., & Cattaneo, L. (2018). Invisible side of emotions: Somato-motor responses to affective facial displays in alexithymia. Experimental Brain Research, 236(1), 195–206. https://doi.org/10.1007/s00221-017-5118-x
- Schirmer, A., & Adolphs, R. (2017). Emotion perception from face, Voice, and Touch: Comparisons and Convergence. Trends in Cognitive Sciences, 21(3), 216–228. https://doi.org/10.1016/j.tics.2017.01.001
- Schwartz, S., & Susser, E. (2011). The use of well controls: An unhealthy practice in psychiatric research. Psychological Medicine, 41(6), 1127–1131. https://doi.org/10.1017/S0033291710001595
- Shamay-Tsoory, S. G. (2011). The neural Bases for empathy. The Neuroscientist, 17(1), 18–24. https://doi.org/10.1177/1073858410379268
- *Sims, T. B., Van Reekum, C. M., Johnstone, T., & Chakrabarti, B. (2012). How reward modulates mimicry: EMG evidence of greater facial mimicry of more rewarding happy faces. Psychophysiology, 49(7), 998–1004. https://doi.org/10.1111/j.1469-8986.2012.01377.x
- Sonnby-Borgström, M. (2002). Automatic mimicry reactions as related to differences in emotional empathy. Scandinavian Journal of Psychology, 43(5), 433–443. https://doi.org/10.1111/1467-9450.00312
- *Sonnby-Borgström, M. (2009). Alexithymia as related to facial imitation, mentalization, empathy, and internal working models-of-self and-others. Neuropsychoanalysis, 11(1), 111–128. https://doi.org/10.1080/15294145.2009.10773602
- *Sonnby-Borgström, M., Jönsson, P., & Svensson, O. (2003). Emotional empathy as related to mimicry reactions at different levels of information processing. Journal of Nonverbal Behavior, 27(1), 3–23. https://doi.org/10.1023/A:1023608506243
- *Sun, Y.-B., Wang, Y.-Z., Wang, J.-Y., & Luo, F. (2015). Emotional mimicry signals pain empathy as evidenced by facial electromyography. Scientific Reports, 5(1), 16988. https://doi.org/10.1038/srep16988
- Viechtbauer, W. (2010). Conducting meta-analyses in R with the metafor package. Journal of Statistical Software, 36(3), https://doi.org/10.18637/jss.v036.i03
- Wacker, R., Bölte, S., & Dziobek, I. (2017). Women know better what other women think and Feel: Gender effects on mindreading across the adult life span. Frontiers in Psychology, 8. https://doi.org/10.3389/fpsyg.2017.01324
- Wagenmakers, E.-J., Beek, T., Dijkhoff, L., Gronau, Q. F., Acosta, A., Adams, R. B., Albohn D. N., Allard E. S., Benning S. D., Blouin-Hudon E.-M., Bulnes L. C., Caldwell T. L., Calin-Jageman R. J., Capaldi C. A., Carfagno N. S., Chasten K. T., Cleeremans A., Connell L., DeCicco J. M., … Zwaan, R. A. (2016). Registered replication report: Strack, Martin, & Stepper (1988). Perspectives on Psychological Science, 11(6), 917–928. https://doi.org/10.1177/1745691616674458
- Weyers, P., Mühlberger, A., Hefele, C., & Pauli, P. (2006). Electromyographic responses to static and dynamic avatar emotional facial expressions. Psychophysiology, 43(5), 450–453. https://doi.org/10.1111/j.1469-8986.2006.00451.x
- *Papers included in the meta-analysis