ABSTRACT
Attentional control theory (ACT) was proposed to account for trait anxiety’s effects on cognitive performance. According to ACT, impaired processing efficiency in high anxiety is mediated through inefficient executive processes that are needed for effective attentional control. Here we review the central assumptions and predictions of ACT within the context of more recent empirical evidence from neuroimaging studies. We then attempt to provide an account of ACT within a framework of the relevant cognitive processes and their associated neural mechanisms and networks, particularly the fronto-parietal, cingular-opercula, and default mode networks. Future research directions, including whether a neuroscience-informed model of ACT can provide a platform for novel neurocognitive intervention for anxiety, are also discussed.
1. Introduction
Anxiety disorders are the most prevalent class of psychological disorders (Remes et al., Citation2016) and the sixth leading cause of disability worldwide (Baxter et al., Citation2014), causing significant disruption to everyday life and placing a considerable burden on healthcare systems (Wittchen et al., Citation2011). An established risk factor for anxiety disorders is trait anxiety, which is a normally distributed personality dimension (Sandi & Richter-Levin, Citation2009) characterised by intrusive thoughts, worry, and difficulty in disengaging from negative material (Heeren et al., Citation2018), as well physiological manifestations (Engels et al., Citation2007; Nitschke et al., Citation2001). Trait anxiety has been associated with functional consequences including increased distractibility and attention problems (Bishop, Citation2009; Bishop et al., Citation2004; Eysenck et al., Citation2007). Indeed, the effects of trait anxiety on cognitive function have long been recognised (Berggren & Derakshan, Citation2013) and can be accounted for by attentional control theory (ACT; Eysenck et al., Citation2007; Eysenck & Derakshan, Citation2011). Furthermore, experimental work has shown that impaired attentional control can maintain and exacerbate the distracting effects of anxiety (Borkovec & Ruscio, Citation2001; Leigh & Hirsch, Citation2011; Stefanopoulou et al., Citation2014). Thus, it is important that we advance our current theoretical models, so that we better understand how trait anxiety affects cognitive performance, and the neural substrates involved. Such an approach is likely to be useful if we wish to develop and test novel mechanistically targeted interventions for anxiety.
Moreover, from a theoretical perspective, models that focus on brain mechanisms plus cognitive processes (e.g. Braver, Citation2012; Eysenck et al., Citation2007) can combine information at behavioural and neural levels, thus providing a more substantial and holistic evidential basis for evaluating theories. This is important because many behavioural studies have reported non-significant effects of trait anxiety on cognitive performance (Eysenck et al., Citation2007). Such findings have been typically regarded as uninformative but often camouflage important differences in brain processes that may provide information regarding inefficient and compensatory neural processing. In this manuscript we will first outline ACT and its assumptions. We then attempt to derive a series of neurofunctional predictions from ACT and examine them in the context of existing neuroimaging finding in trait-anxious samples. First, we outline the predictions of ACT before examining how these might fit with, or be adapted within, our current understanding of the brain’s neurofunctional architecture. We then discuss neurofunctional evidence for ACT with specific focus on neuroimaging studies of susceptibility to distraction, compensatory mechanisms, and the associated role of neurofunctional network. Based on a synthesis of ACT and existing neuroimaging findings, we then consider implications for future research directions and examine if a neurofunctional account of ACT might aid in the development of novel neurocognitive interventions for anxiety disorders.
2. Attentional Control Theory (ACT)
First, we will discuss the major assumptions of Attentional Control Theory (ACT; Eysenck et al., Citation2007; Eysenck & Derakshan, Citation2011). Of key importance in ACT is the theoretical distinction between performance effectiveness and processing efficiency.
Importantly, neuroimaging data may also be useful in quantifying processing efficiency, as behavioural performance can be considered in the context of levels of neural activity and connectivity using techniques such as functional Magnetic Resonance Imaging (fMRI) and electroencephalography (EEG).
A second key prediction of ACT is that high levels of trait anxiety impair processing efficiency more than performance effectiveness, because individuals who experience high levels of anxiety often utilise compensatory strategies or mechanisms to overcome inefficiency caused by non-task processing, without concomitant performance improvement. Such compensatory strategies or processes may be measured by longer correct antisaccade latencies (Derakshan, Ansari, et al., Citation2009), reaction times during a task-shifting paradigm (Derakshan, Smyth, et al., Citation2009), greater CNV (Contingent Negative Variation) amplitude of the ERP response, a well-known index for cognitive effort utilisation for an upcoming task demand involving inhibitory responses (Ansari & Derakshan, Citation2011) or fMRI measures such as greater bold activity in the DLPFC on tasks such as the Stroop demanding efficient selective attention and response inhibition (Basten et al., Citation2011, Citation2012) . However, the precise measures of interest will depend on the task paradigm being used as well as the task instructions given (see Berggren & Derakshan, Citation2013, for a review).
Specifically, ACT predicts that trait anxiety impairs three executive functions: (i) the inhibition function (using attentional control to inhibit focus on task-irrelevant stimuli and prepotent but incorrect responses); (ii) the shifting function (using attentional control to redirect attention in response to changing demands within and between tasks); and (iii) the updating function (updating and monitoring information within working memory). Although a full review of behavioural evidence is outside the remit of this manuscript, there is considerable experimental support for ACT’s prediction that trait anxiety affects these executive functions (Berggren & Derakshan, Citation2013).
ACT assumes that impaired efficiency of these executive functions is mediated by interactions between two attentional systems (Corbetta & Shulman, Citation2002): (1) a top-down, “goal-directed attentional system influenced by expectation, knowledge, and current goals” and (2) a bottom-up “stimulus-driven attentional system responding maximally to salient or conspicuous stimuli”. fMRI and EEG studies are ideally suited to examine this prediction. Experimentally, it has been shown that when tasks require attentional control, anxiety can reduce processing efficiency, usually indexed by increased functional activity in top-down and reactive attentional networks (Barker et al., Citation2018; Basten et al., Citation2011; Sylvester et al., Citation2012) and/or a late correction process known as Error Related Negativity (ERN) (Hsieh et al., Citation2021; Moser et al., Citation2013); both believed to be compensatory mechanisms that maintain task effectiveness, albeit inefficiently (Berggren & Derakshan, Citation2013). However, whilst some neuroimaging findings are consistent with the processing inefficiency prediction of ACT, the model provides little neurocognitive conceptualisation of the mechanisms through which anxiety competes for limited processing resources.
3. How might the predictions of ACT fit with our current understanding of the brain’s functional architecture?
The original conceptualisation of ACT emphasised dysfunctional integrations between top-down and bottom-up attentional systems that loosely mapped onto the dorsal and ventral attention systems (Corbetta & Shulman, Citation2002), respectively. More recently, a number of functional brain networks have been identified. Goal-driven or top-down attentional control is known to be supported by activation in the DLPFC and a wider network of superior parietal regions known as the fronto-parietal network (FPN; Dosenbach et al., Citation2008; Dosenbach Nico et al., Citation2007; Hopfinger et al., Citation2000). In addition to the FPN, a second compensatory or ‘reactive’ attentional control system (Braver, Citation2012) is thought to be utilised when task-elated errors and conflict are detected. The Cingular Opercula Network (CON; Dosenbach et al., Citation2006; Dosenbach et al., Citation2008), sometimes referred to as the salience network (Seeley et al., Citation2007), is considered to be a late correction mechanism that maintains and facilitates optimal task performance. CON activity, particularly the dorsal anterior cingulate (dACC), is thought to signal to the DLPFC to update task demands – so proactive control can be maintained and/or reoriented to reduce the subsequent need for compensatory effort (Carter & van Veen, Citation2007). Such a mechanism would likely rely on efficient and causally directed coupling between the dACC and DLPFC. An analogous electrophysiological mechanism is error-related negativity (ERN), a component of the event-related potential (ERP) reflecting error-monitoring functions of the dACC (described in more detail below).
The default mode network (DMN; Gusnard & Raichle, Citation2001; Raichle et al., Citation2001), a network of regions active during stimulus-independent/self-referential thought (Fox et al., Citation2005; Fox et al., Citation2015), may also play an important role in attentional control. This is because fMRI studies have shown that brain regions within the DMN are associated with perseverative cognition such as worry (e.g. Makovac et al., Citation2020; Servaas et al., Citation2014; Weber-Goericke & Muehlhan, Citation2019) and rumination (Zhou et al., Citation2020), i.e. difficult to control negative thoughts that are a key cognitive component of anxiety (Borkovec et al., Citation1983).
In particular, the posterior cingulate gyrus (PCC; part of the medial DMN) appears to be strongly associated with worry (Makovac et al., Citation2020) and lapses in attentional control (Cieslik et al., Citation2015; Weissman et al., Citation2006), potentially leading to impaired processing under certain task demands. A neurocognitive extension of ACT is now needed that provides an account of the theory’s predictions within the context of our current understanding of these functional brain networks, and their interactions. Of importance to ACT would appear to be the interactions between three major functional brain networks discussed above (FPN, CON, DMN) during task processing, and how these interactions are affected by anxiety (See and ).
Figure 1. A neurofunctional account of ACT emphasises the differential involvement of three brain networks (DMN; CON; FPN)* during task performance by individuals with high and low trait anxiety or worry. Neural activity in the FPN is anti-correlated with activity in the DMN, where the activity in the FPN increases due to attentional demands, activity in the DMN decreases (or deactivates) over the duration of a task requiring some attentional control. During high load, where compensatory processes are required, activity in CON regions increases to maintain effective task performance by signalling the need for increased attentional control. CON activity is required to compensate for altered FPN activity. Note the temporal relationship between FPN and CON activity/connectivity is not well understood in this context alternative temporal relationship are possible. *ROIs are from Shirer et al. (Citation2012).
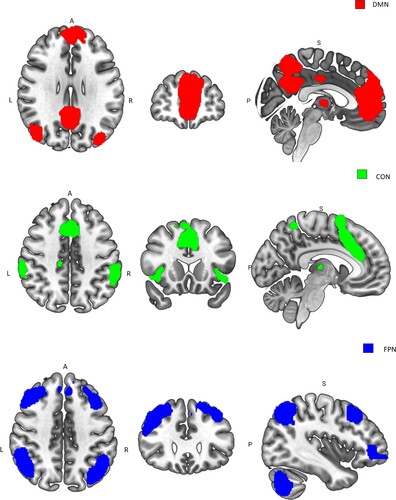
Figure 2. In anxious individuals worry competes for cognitive resources. This impairs attentional control and in turn increases distractibility and maintains worry i.e. a bi-directional influence of worry on attentional control and of attentional control on worry.

First, activity in the FPN is crucial during tasks requiring attentional control, to maintain effective and efficient task performance (Sylvester et al., Citation2012). Second, there is now a large body of fMRI studies showing that neural activity in the FPN is anti-correlated with activity in the DMN (Fox et al., Citation2005), such that over the duration of a task requiring some attentional control (usually arranged into TASK ON/OFF blocks), as activity in the FPN increases due to attentional demands, activity in the DMN decreases (or deactivates) (e.g. Hugdahl et al., Citation2019; Raichle, Citation2015). Third, during periods of increasing task demands, when compensatory mechanisms are required, activity in CON regions increases to maintain effective task performance (Sylvester et al., Citation2012) by signalling the need for increased attentional control (Moran et al., Citation2015; Zanto & Gazzaley, Citation2013). ACT predicts that anxiety competes for processing resources. In the context of these functional brain network interactions, a neurofunctional account of ACT would predict that trait anxiety, would perturb these network interactions, leading to reduced processing efficiency. An ERP study reported that during a flanker task, high worriers shown an enhanced N2 component during distractor trials, in the absence of errors (Owens et al., Citation2015). This may be because anxiety (particularly negative and worrisome thoughts), is associated with increased activity (or reduced deactivation) in the DMN regions, such as the PCC (Fox et al., Citation2015; Makovac et al., Citation2020; Servaas et al., Citation2014; Weber-Goericke & Muehlhan, Citation2019). Failure to deactivate (or reduce activation) in the DMN due to anxiety would then disrupt the ‘normative’ anti-correlation between the DMN and FPN activity that is crucial for efficient network switching during attentional control. Although this needs to be confirmed experimentally, individuals with high levels of worry may be less able to deactivate the DMN and allow activity in the FPN to be up regulated when attentional control is required, results in CON and N2 activity.
To compensate for perturbed DMN/FPN interactions and to maintain effective task processing (albeit inefficiently), anxious individuals are then more reliant upon activity in CON regions, particularly the anterior cingulate cortex (ACC) (Paulus et al., Citation2004; Sylvester et al., Citation2012), especially when task demands are high (Wheaton et al., Citation2014).
First, the conceptualisation of processing efficiency differs from that in ACT. Within ACT, the emphasis was on a nebulously defined global processing efficiency based on very general measures of resource utilisation such as self-reported mental effort or activation within a single brain area (typically the DLPFC) (e.g. Bishop, Citation2009). It is often assumed optimal processing efficiency during task performance is associated with a high level of attentional control involving relatively increased activation in the prefrontal cortex (especially the DLPFC) (Chrysikou et al., Citation2014). However, performance of implicit and largely ‘automatic’ tasks is often associated with a low level of attentional control (Chrysikou et al., Citation2014), and the DLPFC activation (Jansma et al., Citation2001; Poldrack et al., Citation2005), so this assumption may not always apply in certain task contexts. A neurofunctional account of ACT defines processing efficiency in more definite terms of optimal, task context-dependent, interactions and connectivity between three functional brain networks, i.e. the FPN, CON and DMN. It should be noted, however, that such conceptualisations of ‘processing efficiency’ may de-emphasise task differences in optimal processing strategies. And, as already discussed, effectiveness/efficiency trade-offs can be dependent on the task instructions that are provided to participants. How these attentional brain networks interact under such conditions, and their associated temporal dynamics, is still unclear. For example, greater FPN/DLPFC activation in high-anxious individuals is not always reported (Bishop, Citation2009; Forster et al., Citation2015). This is probably because several factors determine the level of FPN activation in high-anxious individuals. For example, individuals with high levels of anxiety have greater problems than those with low levels of anxiety with goal-directed attentional processes involved in goal maintenance (Moser et al., Citation2013). This may occur, in part, because they have experienced increased anxious apprehension. As a consequence, their initial FPN activation during task processing may be lower than that of low-anxious individuals due to worry competing for neural resources and perturbing FPN/DMN anti-correlations. Thus, if a task requires minimal top-down attentional control and goal maintenance, overall FPN activation could be lower in high-anxious than low-anxious individuals, whilst task performance levels are unaffected (e.g. Bishop, Citation2009). However, FPN/DLPFC activity would likely need to increase, as a task becomes more attentionally demanding. Again, if FPN/DMN anti-correlations are perturbed by worrisome thoughts that compete for limited neural resources, high-anxious individuals may have less FPN activation than low-anxious ones shortly after task presentation or in a low task demand condition, but subsequently have higher FPN activation when tasks require more attentional control. It is also difficult to assess processing efficiency from brain activity. Researchers have often focused on activity within a single brain area (e.g. the relationship between trait anxiety and ‘inefficient’ DLPFC activity; (Basten et al., Citation2011; Bishop, Citation2009). This is oversimplified because many tasks involve sequential processing stages associated with different brain regions (e.g. Silton et al., Citation2010; Silton et al., Citation2011). There are several reasons why high-anxious individuals might have greater (less efficient) brain activation than low-anxious individuals despite comparable performance effectiveness. For example, the use of more complex processing strategies, and/or impaired connectivity between brain networks could lead to over-activity in local hubs and disrupted temporal dynamics between networks. Indeed, temporal dynamics relating to processing efficiency are poorly understood using fMRI. Techniques such as event-related potentials (ERPs) and time series analysis such as finite-impulse response function for fMRI are approaches that can provide a superior assessment of such dynamics (see later).
Second, ACT assumes high anxiety is associated with increased involvement of the Ventral Attentional Network (a stimulus-driven attentional system). However, little empirical evidence supports that assumption. A neurofunctional account of ACT places greater emphasis on the CON, encompassing the anterior cingulate cortex (ACC) and bilateral insula (discussed below). Moreover, within ACT, the compensatory strategies used by high-anxious individuals are not explicit. Within a neurofunctional account, processes relating to error monitoring, conflict, and ‘reactive’ control (Braver, Citation2012) often involving the engagement and coupling of the DLPFC and dorsal ACC are posited to instantiate such compensatory functions.
4. Neurofunctional evidence for ACT
To summarise, a neurofunctional account of ACT emphasises the differential involvement of three brain networks (FPN; CON; DMN; see ) during task performance by individuals with high and low trait anxiety or worry. We now examine evidence relating to the proposed neurofunctional account of ACT.
4.1. ACT prediction: anxiety increases susceptibility to distraction
4.1.1. ERPs and the role of the DMN
ACT predicts, high-anxious individuals are more susceptible to distraction from neutral task-irrelevant stimuli than low-anxious ones because they have impaired attentional control making them more responsive to task-irrelevant stimuli. These theoretical assumptions are supported by a large body of behavioural studies (Berggren & Derakshan, Citation2013) and additional evidence has been obtained in studies using ERPs to assess attention to neutral external distractors (Gaspar & McDonald, Citation2018; Moran & Moser, Citation2015; Moser et al., Citation2012; Tsai et al., Citation2017). In all four ERP studies, high-anxious individuals had a larger N2pc (reflecting attentional selectivity) than low-anxious individuals indicating they were more likely to misallocate attention to the external distractors. Studies using fMRI generally support the finding of these ERP studies regarding external distractors during task processing and are discussed in more detail below. However, fMRI studies have also allowed us to gain a better understanding of how internal/endogenous distraction might affect attentional control.
Whilst ACT emphasises the effects of trait anxiety on limited capacity cognitive systems, a number of studies propose a more refined role for worry and its effects on limited attentional/executive resources (e.g. Processing Efficiency Theory; Eysenck & Calvo, Citation1992; Stefanopoulou et al., Citation2014). Furthermore, high-anxious individuals exhibit more mind-wandering than low-anxious ones. Robison et al. (Citation2017) found individuals high in neuroticism (highly correlated with trait anxiety) reported more mind-wandering than low neuroticism individuals during cognitive tasks. fMRI research in this area is relatively limited. However, as already discussed, there is increasing evidence that worry, perseverative cognitions, and mind-wandering involve the DMN, and that high anxiety is associated with increased DMN activation (Fox et al., Citation2015; Makovac et al., Citation2020; Servaas et al., Citation2014; Weber-Goericke & Muehlhan, Citation2019). Whilst networks known to support language and memory processing have also been linked to worry (Makovac et al., Citation2020), as discussed earlier, fMRI studies also report that worry is linked to altered activity and connectivity in the brain’s DMN. The most direct link between DMN activity and worry has been demonstrated by Servaas and colleagues (Servaas et al., Citation2014). During this fMRI study, participants were requested to worry about a topic, which led to significantly increased DMN activation. Gentili et al. (Citation2015) found DMN activity was correlated positively with social anxiety scores and Maresh et al. (Citation2014) found higher levels of social anxiety were positively associated with DMN activity during task performance. However, according to a recent review by Northoff (Citation2020), increased deactivation of DMN in high-anxious individuals across various tasks have also been reported. One reason for the apparently inconsistent findings is that task-related deactivation of DMN may depend on the type of task and its reliance upon executive processes (Weissman et al., Citation2006). It is also important to consider the temporal sequencing of processes. According to the anti-correlation principle described by Fox et al. (Citation2005), increased activity in one brain network is usually associated with decreased activity in another network. It is possible that high-anxious individuals initially allocate attentional resources to mind-wandering and other processes involving the DMN (e.g. precuneus and posterior cingulate), thereby reducing attentional resources (involving DLPFC and ACC) available for task performance. However, it is also possible that later in task processing, high-anxious individuals’ enhanced attentional control may produce reduced DMN activation through an anti-correlational relationship. Currently, our limited understanding of network dynamics during task processing (using fMRI) is an obstacle to fully understanding these temporal relationships. Further, although a different construct to worry, a meta-analysis of mind-wandering studies using fMRI has also indicated that mind-wandering and spontaneous thoughts are associated with activation in several key areas within the DMN (Fox et al., Citation2015).
4.2. ACT prediction: anxiety reduces goal-directed attentional control
4.2.1. Role of the FPN
Because anxiety competes for limited cognitive resources, ACT predicts that anxiety can impair goal-directed attentional control, thus impacting task performance and outcomes. fMRI studies have generally indicated that trait-anxious individuals having greater DLPFC activation than low-anxious ones when tasks require attentional and/or executive control (i.e. inhibition; shifting; updating). Basten et al. (Citation2011) assessed right DLPFC activity during colour Stroop Task performance. High-anxious participants showed a greater increase in DLPFC activation than low-anxious ones on incongruent trials only. Since the effects of trait anxiety on task performance were non-significant, it could be argued that anxiety impaired processing efficiency rather than performance effectiveness, i.e. a greater level of DLPFC activation was required to achieve the sample level of Stroop Task performance than low trait anxious subjects. Barker and colleagues (Barker et al., Citation2018), assessed levels of worry (anticipatory anxiety) and reported similar results to those reported by Basten and colleagues, i.e. higher levels of worry were associated with greater activation in DLPFC (and ACC) regions during an emotional interference task without associated improvements in behavioural performance. Whilst more difficult to assess the effects on task performance, Basten et al. (Citation2012) observed a greater increase in activation in DLPFC in high-anxious than low-anxious participants only when updating was required during a working memory task. Fales et al. (Citation2008) also used a task requiring the updating function (the n-back task). High-anxious individuals showed reduced sustained activation within the FPN network (possibly reflecting impaired engagement of the FPN for sustained goal-directed attentional control) but increased transient FPN activation reflecting use of compensatory processes. By using the same task, Liu et al. (Citation2021) showed higher FPN activity in high trait-anxious individuals under long-term stress. Whilst it is not always possible to distinguish between processing effectives and efficiency, these neurofunctional data suggest that, in anxious individuals, there is a transient need to engage greater levels of cognitive control to maintain task performance because of a failure in sustaining goal-directed control throughout the task.
Some other fMRI findings appear less consistent with the prediction of ACT, that anxiety is associated with the use of compensatory mechanisms. Bishop (Citation2009) used two tasks: one involving minimal perceptual load and a harder task involving a much greater perceptual load (with congruent and incongruent distractors being used in both tasks). Trait anxiety was linked to impoverished/reduced recruitment of prefrontal attentional control mechanisms (DLPFC) to inhibit distractor processing when the task involved minimal perceptual load. However, in the minimal perceptual load condition, it is possible that the task required no, or low levels of executive function, so enhanced DLPFC activation in high-anxious individuals associated with compensatory control processes were not required. Forster et al. (Citation2015) found on no-go trials of a go/no-go task that anxiety was also associated with reduced DLPFC, as well as ACC activation – interpreted as reflecting “impoverished frontal control of attention”. However, using a similar go/no-go paradigm, Karch et al. (Citation2008) compared brain activity using fMRI in high and low-anxious individuals. Trait anxiety did not affect performance, but when the task required inhibitory control (i.e. no-go trials), anxious individuals had greater activity than low-anxious ones in the DLPFC and temporo-parietal brain regions, a finding again suggesting the use of compensatory mechanisms to maintain task performance.
It should be noted however there are several limitations when using fMRI to assess attentional control. First, many tasks described above do not adequately dissociate goal-directed attentional control (involving maintenance of goal-relevant information) and compensatory attentional control mechanisms (transient goal-reactivation; i.e. Braver, Citation2012), which may involve different brain networks, i.e. the FPN and CON respectively. Second, as mentioned, fMRI has poor temporal resolution, which limits inferences about DLPFC’s temporal dynamics in the context of early goal-directed attentional/cognitive control and subsequent compensatory or reactive mechanisms, or a complex combination of the two.
4.3. ACT prediction: anxiety is associated with greater use compensatory mechanisms during attentional control
4.3.1. Compensatory strategies, conflict and error monitoring: role of the CON
ACT predicts that anxiety is associated with the use of compensatory strategies. This is because studies requiring executive function, i.e. using the anti-saccade task (e.g. Derakshan, Ansari, et al., Citation2009; Hepsomali et al., Citation2017; Hepsomali et al., Citation2019; Wright et al., Citation2014), the Stroop Task, and the go/no-go paradigm (Berggren et al., Citation2013; Hepsomali et al., Citation2019) have all provided experimental evidence linking trait-anxiety to impaired inhibition function.
The investigation of compensatory strategies during conflict tasks is particularly suited to ERP research. Previous research has established ERP components closely associated with inhibitory control, permitting more precise examination of associations between anxiety and inhibition. Much ERP research has used the go/no-go paradigm where participants must infrequently inhibit a motor response (no-go trials). The N2 ERP component is typically much larger on no-go than go trials, strongly suggesting it reflects the use of inhibitory processes. If top-down or goal-directed attentional control, supported by the FPN, is impaired in high-anxious individuals they will exhibit impaired goal focus and reduced ability to discriminate between task-relevant and task-irrelevant, affecting performance outcomes. As a consequence, compensatory strategies deployed by individuals with anxiety should be reflected in a larger N2 on no-go trials.
ERP studies have provided evidence of this compensatory mechanism. Righi et al. (Citation2009) used the same go/no-go task as Forster et al. (Citation2015) while recording ERPs. High-anxious participants had significantly larger N2 responses (reflecting inhibitory processes) than low-anxious ones on no-go trials (but no differences in other ERP components). These enhanced N2 responses most likely reflect inhibitory processes occurring within the frontal cortex. Since there were no effects of trait anxiety on performance (RTs and/or accuracy), anxiety appears to be associated with compensatory mechanisms that maintain performance effectiveness. Similar findings were reported by Sehlmeyer et al. (Citation2010) in high-anxious and by (Owens et al., Citation2015) in high-worrier participants, only when the task demands were high. Converging evidence came from studies that utilised Contingent Negative Variation (CNV) and showed increased frontal CNVs (a marker of effort) in high (vs. low) trait anxious individuals in the anti-saccade task (Ansari & Derakshan, Citation2011), especially under high load conditions (Hepsomali et al., Citation2019). Similar findings were also reported in individuals with high levels of perceived chronic stress (Shi & Wu, Citation2020). However, some studies utilising the Go/No-Go task have reported attenuated N2 responses (Hepsomali et al., Citation2019; Xia et al., Citation2020; Yang & Li, Citation2014), a finding harder to reconcile with the use on compensatory mechanism by anxious individuals.
Savostyanov et al. (Citation2009) and Neo et al. (Citation2011) extended the ERP findings by measuring EEG desynchronisation (event-related spectral perturbations, reflecting effort or use of processing resources in a go/no-go task). High-anxious participants showed greater EEG desynchronisation than low-anxious ones only in the no-go condition (Neo et al., Citation2011; Savostyanov et al., Citation2009). Thus, as predicted by ACT, findings from these studies suggest that the requirement to utilise inhibitory processes causes high-anxious individuals to use more effort and processing resources than those individuals with low anxiety.
Arguably less well suited to the study of compensatory mechanisms and their temporal dynamics, due to the sluggish hemodynamic response, fMRI studies have examined the effects of trait anxiety on ACC activity. The dorsal ACC (dACC), part of the CON, is important for detecting conflict (e.g. mismatch between prepotent and correct responses) and signalling to the DLPFC the need for increased cognitive control (Botvinick et al., Citation2001). As ACT predicts that high-anxious individuals have less efficient goal-directed control making them susceptible to internal and external distractors, the theory would consequently predict that anxious individuals would need to devote more resources to compensatory conflict detection and so should exhibit greater dACC on conflict tasks.
Silton et al. (Citation2011) showed that at higher levels of anxious apprehension, increased dorsal ACC activity was related to greater Stroop interference (worse performance). In a different conflict task, Comte et al. (Citation2015) presented participants simultaneously with a face and a scene having congruent or incongruent emotional valence and required them to determine the emotion of the face or scene. Task performance was unaffected by trait anxiety. However, high trait anxiety was associated with increased dACC activity on incongruent trials compared to congruent ones, suggesting the use of greater compensatory processing. In addition, functional connectivity between the ACC and lateral PFC was reduced in high-anxious relative to low-anxious individuals suggesting disrupted coupling and communication between these attentional network hubs. Although, unclear, this finding could be interpreted as reduced neural efficiency, resulting the need for compensatory processing to maintain task performance.
Eisenberger et al. (Citation2005) used an oddball task where a different response was required on infrequent oddball trials. The extent to which dACC activity was greater on oddball trials than non-oddball trials correlated positively with neuroticism (highly correlated with trait anxiety), again, probably reflecting compensatory control in anxious individuals. Decreased functional connectivity between the dACC (perhaps signalling the need for increased cognitive control) and DLPFC (perhaps involved in implementing that increased control) has also been reported in high-anxious individuals compared to low-anxious ones during conflict tasks (Barker et al., Citation2018; Basten et al., Citation2011; Comte et al., Citation2015). Silton et al. (Citation2010) assessed brain activation, and, importantly, the timing of such activation using both fMRI and ERPs with the Stroop Task. During incongruent trials, dACC activity followed DLPFC activity. These findings suggest dACC activity works as a compensatory mechanism during attentional control, presumably when DLPFC-mediated top-down control is inefficient.
Other related research has focused on dACC’s role in error monitoring and detection. A neurofunctional account of ACT would predict that high-anxious individuals require greater error monitoring than low-anxious ones as a compensatory strategy to maintain performance because of impaired goal-directed control (Moser et al., Citation2013). Moser et al. (Citation2013) focused on error-related negativity (ERN). ERN has been consistently localised to the ACC and may reflect the activity of a co-ordinated network involving communication between ACC and DLPFC engaged within 100 ms of an erroneous response (Gehring et al., Citation2012). In a meta-analysis of studies using standard conflict tasks (e.g. the Stroop Task; the go/no-go task; the flanker task), Moser et al. (Citation2013) found anxiety was associated with a larger ERN. Moser et al. (Citation2013) concluded, “Enhanced ERN in anxiety may index a compensatory effort signal aimed at maintaining a standard level of performance.” Anxiety typically had no effect on performance, suggesting such compensatory efforts are generally successful. In a recent systematic review, heightened ERN response has also been proposed as a neurocognitive marker of anxiety-related disorders (Sagalakova et al., Citation2021). It is important to note that enhanced ERN has also been proposed as a risk marker for anxiety (Banica et al., Citation2020; Olvet & Hajcak, Citation2008), as the ERN amplitude showed considerable heritability (47% of the phenotypic variance being accounted for by genetic factors) (Anokhin et al., Citation2008), and enhanced ERN in childhood and adolescence may predict the onset of anxiety disorders (Meyer, Citation2017; Meyer et al., Citation2018), although the ERN’s role in risk for anxiety is a matter of debate (Moser, Citation2017; Moser et al., Citation2014).
Moran et al. (Citation2015) further found anxiety was not only associated with enlarged ERN, but also with reduced coupling between dACC and lateral DLPFC recording sites on error trials using advanced EEG signal processing analysis. This finding is consistent with fMRI findings mentioned above indicating reduced ACC-DLPFC coupling during high conflict trials in trait-anxious individuals. Using path analysis accounting for effects of anxiety, ERN and ACC-DLPFC coupling on post-error performance, Moran et al. (Citation2015) found enhanced ERN in anxious individuals served the proposed compensatory function of counteracting reduced ACC-DLPFC coupling, thereby stabilising post-error performance relative to lower anxious individuals.
Finally, error monitoring is likely facilitated by inhibitory functions. Research by Hoffmann et al. (Citation2014) suggests ERN reflects inhibition of the error response to replace it with the correct response. Thus, enhanced ERN in anxiety is consistent with the ACT predictions that anxiety impairs the inhibition function of executive control, requiring a greater ERN for successful inhibition.
To summarise, anxious individuals often compensate for impaired or inefficient top-down control involving the DLPFC via the ERN (localised to the dACC). This compensatory strategy is generally effective in part because the ERN reflects inhibition of error responses. However, during conflict and error monitoring, high-anxious individuals appear to have weaker coupling between the dACC and DLPFC, which may result in additional use of neural resources (possibly involving both the DLPFC and dACC) to maintain task performance, although it is not clear how this might affect task performance.
It is also important to note that bigger N2 and ERN responses observed in anxious individuals might be attributable to increased generic need-for-control signal of the medial frontal cortex known as frontal midline theta (FMT) (Cavanagh & Frank, Citation2014), as FMT has been shown to be heightened in individuals with high anxiety (Cavanagh et al., Citation2017; Cavanagh & Shackman, Citation2015; Osinsky et al., Citation2017; Schmidt et al., Citation2018), and might reflect a higher general control-related resource recruitment employed by high anxious individuals.
5. A neurofunctional account of ACT: further factors to consider
5.1. Trait anxiety vs. worry
According to ACT, performance effectiveness and processing efficiency deficits during tasks requiring attentional control depend primarily on individual differences in trait anxiety. However, trait anxiety is a multifaceted construct (Endler & Kocovski, Citation2001), involving cognitive and physiological dimensions. Worry, considered the cognitive component of anxiety, is characterised by negative and repetitive thoughts that are attention-demanding and consume limited attentional resources, impacting adversely on top-down attentional control. Moreover, experimental work has shown that reduced or inefficient attentional control can maintain and exacerbate the distracting effects of worry (Armstrong et al., Citation2011; Hotton et al., Citation2018; Stefanopoulou et al., Citation2014). Of relevance to a neurofunctional account of ACT, worry is often associated with increased neural activity in brain areas important for attentional control (Silton et al., Citation2010; Spielberg et al., Citation2013), and associated with DMN activity (Steinfurth et al., Citation2017) which, as explained above, is an important network within the framework of a neurofunctional account of ACT. Barker et al. (Citation2018) tested more directly the effect of worry on activity and connectivity in prefrontal and cingulate cortices during an attentional control task. Worry (but not trait anxiety) was associated with increased activity across CON regions in the ACC and insula, and FPN regions in the DLPFC and parietal cortex, findings consistent with ACT. Moreover, reduced functional connectivity between ACC and DLPFC was also observed during an emotional interference task, reflecting the effect of worry on attentional network coupling. However, neither worry nor trait anxiety predicted task performance. This fMRI finding is also consistent with ERP studies. Moser et al. (Citation2013) in a meta-analysis discussed earlier, found anxiety positively correlated with enhanced ERN. However, this effect was threefold greater when anxious apprehension or worry was the measure of anxiety rather than anxious arousal. Schroder et al. (Citation2017) studied the error-preceding positivity (EPP; an ERP component larger on pre-error trials than pre-correct trials), which reflects reduced attentional focus immediately preceding errors. The EPP was reduced in females with high worry, suggesting they attempt to compensate for reduced task-focused attention by engaging in increased target processing. Moreover, Schroder et al. also found that the reduced EPP mediated the relationship between worry and enlarged ERN, further confirming that the ERN reflects compensatory processing to increase task-focused attention following mistakes.
5.2. Processing efficiency
Cognitive neuroscience provides compelling evidence that impaired processing efficiency associated with high trait anxiety (or worry) depends on several factors. Here we consider some of the main complexities involved. Global conceptualisations of processing efficiency (i.e. based on notions of resource utilisation or mental effort) are oversimplified and should mostly be replaced by more local or specific conceptualisations (Poldrack, Citation2015). An especially promising approach involves distinguishing between efficiency involving task-relevant processing and efficiency involving task-irrelevant processing. According to this approach, we should assess task-relevant and task-irrelevant processing efficiency in high and low worriers (or high – and low-anxious individuals) taking account of the temporal sequencing of processes during task performance. For example, it is possible that early in task processing, high-anxious individuals typically engage in more task-irrelevant processing than low-anxious ones due to impaired attentional control. According to a neurofunctional account of ACT, such task-irrelevant processing of internal stimuli, i.e. worry, and mind-wandering, may be associated with activation within the DMN that perturbs network interactions during the temporal course of a task. In addition, high-anxious individuals are also more likely than low-anxious ones to engage in processing of task-irrelevant external stimuli (e.g. distractors). Thus, high-anxious individuals exhibit greater task-irrelevant local inefficiency than low-anxious ones. Later in task processing, high-anxious individuals typically engage in more task-relevant processing than low-anxious ones through their enhanced use of compensatory strategies to re-direct processing resources to tasks requiring the use of executive functions. Enhanced brain activation in high-anxious individuals associated with such strategies includes the N2 ERP component, the EPP, the ERN, dACC/CON, and DLPFC/FPN.
Tentative evidence to support this mechanistic account comes from fMRI studies reporting that high-anxious individuals generally have greater DLPFC activation than low-anxious individuals only when the current task is attentionally demanding (e.g. Barker et al., Citation2018; Basten et al., Citation2011, Citation2012; Bishop, Citation2009; Santos et al., Citation2006). Similar findings have been reported with respect to dACC activation (Comte et al., Citation2015; Eisenberger et al., Citation2005). Whilst these fMRI studies tell us little about the temporal dynamics of this process, as the averaging of the BOLD signal over task ON/OFF blocks is likely to obscure temporal information, it may be that on average, high-anxious individuals are able to recruit these compensatory mechanisms relatively early during task processing, resulting in a relatively increased task related BOLD signal in FPN and CON regions during task ON blocks or events. In fact, some behavioural and ERP studies have already addressed the presence of dynamic, trial-to-trial variations of resource recruitment in anxiety that are responsive to task demands and/or characteristics (Booth & Peker, Citation2017; Jeong & Cho, Citation2021; Larson et al., Citation2013; Osinsky et al., Citation2010; Osinsky et al., Citation2012; Suárez-Pellicioni et al., Citation2014; Yu et al., Citation2018). Hence, more sophisticated analytical approaches to BOLD time series analysis are needed to truly test this prediction.
5.3. Connectivity
Another factor of relevance to assessing efficiency in high – and low-anxious individuals is functional connectivity between brain areas involved in attentional control and task performance. There is increasing evidence high anxiety is associated with reduced functional connectivity between ACC and DLPFC (or CON and DLPFC) (Barker et al., Citation2018; Basten et al., Citation2011; Comte et al., Citation2015). Indeed, a recent fMRI study using real-time neurofeedback shows that training participants to increase functional connectivity between attentional networks reduces anxiety levels in anxious individuals (Morgenroth et al., Citation2020). Servaas et al. (Citation2015) found individuals high in neuroticism (highly correlated with trait anxiety) also had impaired connectivity within brain networks (the default mode network; the fronto-parietal network).
Dysfunctional connectivity is also found in high worriers. Weber-Goericke and Muehlhan (Citation2019) reported a meta-analysis of fMRI studies. High worriers had strong activation in brain areas associated with language processing (e.g. middle frontal gyrus; inferior frontal gyrus; anterior insula), and there were strong functional connections between these areas and DMN. Thus, high worriers may have strong connections among brain areas associated with ongoing internally oriented task-irrelevant processing. These strong connections may affect network switching and interactions during task processing and reflect their impaired attentional control and processing inefficiency. However, more research is needed to better understand the complex network interactions and dynamics, e.g. anticorrelations between the FPN and DMN and how these interactions are affected by worry.
6. Future research directions and implications for novel intervention for anxiety
Better fMRI task designs are required to examine how FPN, CON and DMN activity is modulated interactively by trait anxiety and temporally changing task demands. Neurofunctionally, ACT predicts a positive association between trait anxiety and FPN activation during tasks requiring high levels of executive processes. An alternative, more targeted approach to assessing the effects of anxiety on FPN activity, connectivity and temporal dynamics will be important for understanding the processing inefficiency associated with high anxiety. Indeed, establishing a better understanding of the temporal dynamics of FPN, CON and DMN and how these networks interact during task processing and attentional control are important. Recently, a number of analytic approaches have been developed that can be applied to the understanding of network temporal dynamic and interaction. For example, finite-impulse response function (FIR) had been used to quantify the dynamic regulation of DMN and FPN networks (Hugdahl et al., Citation2019). This and similar fMRI analyses approaches are likely to be suitable for fMRI studies that aim to understand how these (and other) networks interact during different task conditions, and, how these interactions are affected by worry and trait anxiety.
Better mechanistic models will ultimately provide critical insights into the development of new neurocognitive interventions for anxiety. Behaviourally, experimental work has shown that reduced or inefficient attentional control can maintain and exacerbate the distracting effects of anxiety (Borkovec & Ruscio, Citation2001; Leigh & Hirsch, Citation2011; Stefanopoulou et al., Citation2014). Poor attentional control may also limit the efficacy of psychological interventions for anxiety (e.g. CBT) in many individuals (Covin et al., Citation2008; Hanrahan et al., Citation2013), because they do not have the attentional resources required to implement effortful psychological techniques at times of heightened anxiety – when they are most required. Thus, enhancing attentional control in anxious people and helping them learn to utilise this capacity may reduce anxiety, and also be useful as an augmentation strategy to improve the efficacy of existing psychological interventions that require attentional resources (Hirsch et al., Citation2020). Indeed, training to enhance attentional control and/or executive functions can reduce anxiety. Sari et al. (Citation2016) trained working memory to enhance attentional control in high trait-anxious individuals. Hotton et al. (Citation2018) found improvement during working memory training correlated with reductions in worry in high worriers. Hadwin and Richards (Citation2016) found working memory training increased inhibitory control and reduced anxiety symptoms. Moser et al. (Citation2015) found attentional training reduced state anxiety. Recent studies have also shown similar patterns of results (i.e. reduced anxiety and improved task performance following training) (Lotfi et al., Citation2021; Lukasik et al., Citation2019; Zhao et al., Citation2020).
Morgenroth et al. (Citation2020) used fMRI neurofeedback training to up-regulate functional connectivity in attentional networks, between the DLPFC and ACC. Relative to a sham condition, connectivity-based real time neurofeedback training significantly reduced anxiety in a group of high trait anxiety participants. New research is needed to understand the potential for brain stimulation techniques such as tDCS and tACS to enhance attentional control in anxious individuals. Recently, tACS has been used to enhance cognitive performance by non-invasively modulating intrinsic oscillatory neural activity (Klink et al., Citation2020). An advantage of tACS relative to other brain stimulation techniques is that it can target specific brain oscillations or frequencies. EEG studies of cognitive and attentional control generally report that these cognitive abilities are related to the alpha range frequency band, and there is also evidence that tACS can improve function in the brain’s attentional networks during and after the stimulation period (see Klink et al., Citation2020 for a review). In recent years, a small number of experimental medicine studies have demonstrated that tACS can reduce symptoms in clinical populations (Clancy et al., Citation2018; Grover et al., Citation2021). Therefore, ACT may provide useful mechanistic information for future interventional studies testing the efficacy of dietary interventions in large cohorts, particularly in patients with anxiety disorders and/or individuals with high trait anxiety and/or worry.
7. Limitations and conclusions
Before drawing any conclusions, it is first necessary to discuss the limitations of the neurofunctional account of ACT proposed above. First, ACT assumes that differences in task processing and performance between high and low-anxious groups are strongly influenced by individual differences in trait anxiety. Within a neurofunctional account of ACT, worry, rather than trait anxiety per se, is the central cognitive construct that affects attentional control in trait anxious individuals i.e. the cognitive dimension of trait anxiety, that co-opts available cognitive resources that would otherwise be allocated to task processing, thus leading to inefficient task processing (see ).
Whilst the physiological effects of trait anxiety may also act on attentional control (Heller et al., Citation1997), there is currently a limited understanding of its precise role in anxiety-related cognitive dysfunction. This may also be an important distinction at a neural level, as the physiological component of anxiety is reported to be associated with distinct patterns of brain activity, i.e. in parietal vigilance networks during the presentation of threat stimuli (Engels et al., Citation2007). However, to date, the vast majority of neuroimaging research has not focused directly on worry or assessed levels of worry in combination with trait anxiety measures. This limitation needs to be addressed in future neuroimaging studies of attentional control mechanisms, in particular studies that dissociate the effects of worry and trait anxiety on brain network function and interactions.
Second, at behavioural and neurofunctional levels, the effectiveness/efficiency distinction remains difficult to parse at times and is dependent on the upon the task being used and on the method of performance measurement. A more parsimonious definition of task effectiveness may be the capacity for individuals to execute attentional processes in line with attentional goals. As such, the instructions given to participants before a task may significantly affect the ‘effectiveness/efficiency trade-off’. Furthermore, the links between cognitive processes and associated patterns of brain activation are often relatively indirect are there are undoubtedly complex interactions between task-relevant and task-irrelevant processes not explicitly considered by a neurofunctional account of ACT that are important for task performance. In particular, we need to use more sophisticated techniques to assess more precisely the strength and time course of both types of processes. For example, our neurofunctional account of ACT predicts the time course of FPN activity during attentionally demanding tasks, and along with more sophisticated EEG approaches, magnetoencephalography (MEG) could be used to test these predictions more directly than fMRI.
To conclude, neuroimaging research has allowed theory to move well beyond the simplistic assumption within ACT that high-anxious individuals are globally inefficient compared to low-anxious ones because they use more processing resources (or mental effort) but achieve only comparable performance. fMRI and ERP data have enabled us to learn more about the mechanistic nature and extent of imapired or inefficient processing in high-anxious individuals, and to some extent, how this may vary over the temporal course of task processing as a function of interactions between at least three brain networks (FPN; CON; DMN). This mechanistic account, made possible by neuroimaging studies, also allows us to gain a greater understanding of how dysfunctional interactions in these brain networks, when tasks require executive functions, may affect processing efficiency. One reason for such processing inefficiency may be impaired functional connectivity among brain areas associated with attentional control in high-anxious individuals.
In sum, considerable progress has been made in identifying the different kinds of processing inefficiency exhibited by high-anxious individuals (see and ). Neuroimaging data has allowed us to construct a more complex and nuanced account of processing inefficiency within the context of ACT. It is clear the optimal research approach is both comprehensive and theoretically driven: effective testing of the new predictions that have arisen from this neurofunctional account of ACT will require assessing activity concurrently in the brain networks identified in the theory using techniques that can provide a good temporal resolution.
Disclosure statement
No potential conflict of interest was reported by the author(s).
References
- Anokhin, A. P., Golosheykin, S., & Heath, A. C. (2008). Heritability of frontal brain function related to action monitoring. Psychophysiology, 45(4), 524–534. https://doi.org/10.1111/j.1469-8986.2008.00664.x
- Ansari, T. L., & Derakshan, N. (2011). The neural correlates of impaired inhibitory control in anxiety. Neuropsychologia, 49(5), 1146–1153. https://doi.org/10.1016/j.neuropsychologia.2011.01.019
- Armstrong, T., Zald, D. H., & Olatunji, B. O. (2011). Attentional control in OCD and GAD: Specificity and associations with core cognitive symptoms. Behaviour Research and Therapy, 49(11), 756–762. https://doi.org/10.1016/j.brat.2011.08.003
- Banica, I., Sandre, A., Shields, G. S., Slavich, G. M., & Weinberg, A. (2020). The error-related negativity (ERN) moderates the association between interpersonal stress and anxiety symptoms six months later. International Journal of Psychophysiology, 153, 27–36. https://doi.org/10.1016/j.ijpsycho.2020.03.006
- Barker, H., Munro, J., Orlov, N., Morgenroth, E., Moser, J., Eysenck, M. W., & Allen, P. (2018). Worry is associated with inefficient functional activity and connectivity in prefrontal and cingulate cortices during emotional interference. Brain and Behavior, 8(12), e01137. https://doi.org/10.1002/brb3.1137
- Basten, U., Stelzel, C., & Fiebach, C. J. (2011). Trait anxiety modulates the neural efficiency of inhibitory control. Journal of Cognitive Neuroscience, 23(10), 3132–3145. https://doi.org/10.1162/jocn_a_00003
- Basten, U., Stelzel, C., & Fiebach, C. J. (2012). Trait anxiety and the neural efficiency of manipulation in working memory. Cognitive, Affective, & Behavioral Neuroscience, 12(3), 571–588. https://doi.org/10.3758/s13415-012-0100-3
- Baxter, A. J., Vos, T., Scott, K. M., Ferrari, A. J., & Whiteford, H. A. (2014). The global burden of anxiety disorders in 2010. Psychological Medicine, 44(11), 2363–2374. https://doi.org/10.1017/s0033291713003243
- Berggren, N., & Derakshan, N. (2013). Attentional control deficits in trait anxiety: Why you see them and why you don't. Biological Psychology, 92(3), 440–446. https://doi.org/10.1016/j.biopsycho.2012.03.007
- Berggren, N., Richards, A., Taylor, J., & Derakshan, N. (2013). Affective attention under cognitive load: Reduced emotional biases but emergent anxiety-related costs to inhibitory control. Frontiers in Human Neuroscience, 7, 188. https://doi.org/10.3389/fnhum.2013.00188
- Bishop, S., Duncan, J., Brett, M., & Lawrence, A. D. (2004). Prefrontal cortical function and anxiety: Controlling attention to threat-related stimuli. Nature Neuroscience, 7(2), 184–188. https://doi.org/10.1038/nn1173
- Bishop, S. J. (2009). Trait anxiety and impoverished prefrontal control of attention. Nature Neuroscience, 12(1), 92–98. https://doi.org/10.1038/nn.2242
- Booth, R. W., & Peker, M. (2017). State anxiety impairs attentional control when other sources of control are minimal. Cognition and Emotion, 31(5), 1004–1011. https://doi.org/10.1080/02699931.2016.1172474
- Borkovec, T. D., Robinson, E., Pruzinsky, T., & DePree, J. A. (1983). Preliminary exploration of worry: Some characteristics and processes. Behaviour Research and Therapy, 21(1), 9–16. https://doi.org/10.1016/0005-7967(83)90121-3
- Borkovec, T. D., & Ruscio, A. M. (2001). Psychotherapy for generalized anxiety disorder. Journal of Clinical Psychiatry, 62(Suppl 11), 37–42; discussion 43–35.
- Botvinick, M. M., Braver, T. S., Barch, D. M., Carter, C. S., & Cohen, J. D. (2001). Conflict monitoring and cognitive control. Psychological Review, 108(3), 624–652. https://doi.org/10.1037/0033-295x.108.3.624
- Braver, T. S. (2012). The variable nature of cognitive control: A dual mechanisms framework. Trends in Cognitive Sciences, 16(2), 106–113. https://doi.org/10.1016/j.tics.2011.12.010
- Carter, C. S., & van Veen, V. (2007). Anterior cingulate cortex and conflict detection: An update of theory and data. Cognitive, Affective, & Behavioral Neuroscience, 7(4), 367–379. https://doi.org/10.3758/cabn.7.4.367
- Cavanagh, J. F., & Frank, M. J. (2014). Frontal theta as a mechanism for cognitive control. Trends in Cognitive Sciences, 18(8), 414–421. https://doi.org/10.1016/j.tics.2014.04.012
- Cavanagh, J. F., Meyer, A., & Hajcak, G. (2017). Error-Specific cognitive control alterations in generalized anxiety disorder. Biological Psychiatry: Cognitive Neuroscience and Neuroimaging, 2(5), 413–420. https://doi.org/10.1016/j.bpsc.2017.01.004
- Cavanagh, J. F., & Shackman, A. J. (2015). Frontal midline theta reflects anxiety and cognitive control: Meta-analytic evidence. Journal of Physiology-Paris, 109(1–3), 3–15. https://doi.org/10.1016/j.jphysparis.2014.04.003
- Chrysikou, E. G., Weber, M. J., & Thompson-Schill, S. L. (2014). A matched filter hypothesis for cognitive control. Neuropsychologia, 62, 341–355. https://doi.org/10.1016/j.neuropsychologia.2013.10.021
- Cieslik, E. C., Mueller, V. I., Eickhoff, C. R., Langner, R., & Eickhoff, S. B. (2015). Three key regions for supervisory attentional control: Evidence from neuroimaging meta-analyses. Neuroscience & Biobehavioral Reviews, 48, 22–34. https://doi.org/10.1016/j.neubiorev.2014.11.003
- Clancy, K. J., Baisley, S. K., Albizu, A., Kartvelishvili, N., Ding, M., & Li, W. (2018). Lasting connectivity increase and anxiety reduction via transcranial alternating current stimulation. Social Cognitive and Affective Neuroscience, 13(12), 1305–1316. https://doi.org/10.1093/scan/nsy096
- Comte, M., Cancel, A., Coull, J. T., Schön, D., Reynaud, E., Boukezzi, S., & Fakra, E. (2015). Effect of trait anxiety on prefrontal control mechanisms during emotional conflict. Human Brain Mapping, 36(6), 2207–2214. https://doi.org/10.1002/hbm.22765
- Corbetta, M., & Shulman, G. L. (2002). Control of goal-directed and stimulus-driven attention in the brain. Nature Reviews Neuroscience, 3(3), 201–215. https://doi.org/10.1038/nrn755
- Covin, R., Ouimet, A. J., Seeds, P. M., & Dozois, D. J. (2008). A meta-analysis of CBT for pathological worry among clients with GAD. Journal of Anxiety Disorders, 22(1), 108–116. https://doi.org/10.1016/j.janxdis.2007.01.002
- Derakshan, N., Ansari, T. L., Hansard, M., Shoker, L., & Eysenck, M. W. (2009). Anxiety, inhibition, efficiency, and effectiveness. Experimental Psychology, 56(1), 48–55. https://doi.org/10.1027/1618-3169.56.1.48
- Derakshan, N., Smyth, S., & Eysenck, M. W. (2009). Effects of state anxiety on performance using a task-switching paradigm: An investigation of attentional control theory. Psychonomic Bulletin & Review, 16(6), 1112–1117. https://doi.org/10.3758/PBR.16.6.1112
- Dosenbach, N. U. F., Fair, D. A., Cohen, A. L., Schlaggar, B. L., & Petersen, S. E. (2008). A dual-networks architecture of top-down control. Trends in Cognitive Sciences, 12(3), 99–105. https://doi.org/10.1016/j.tics.2008.01.001
- Dosenbach, N. U. F., Visscher, K. M., Palmer, E. D., Miezin, F. M., Wenger, K. K., Kang, H. C., Burgund, E. D., Grimes, A. L., Schlaggar, B. L., & Petersen, S. E. (2006). A core system for the implementation of task sets. Neuron, 50(5), 799–812. https://doi.org/10.1016/j.neuron.2006.04.031
- Dosenbach Nico, U. F., Fair Damien, A., Miezin Francis, M., Cohen Alexander, L., Wenger Kristin, K., Dosenbach Ronny, A. T., … Petersen Steven, E. (2007). Distinct brain networks for adaptive and stable task control in humans. Proceedings of the National Academy of Sciences, 104(26), 11073–11078. https://doi.org/10.1073/pnas.0704320104
- Eisenberger, N. I., Lieberman, M. D., & Satpute, A. B. (2005). Personality from a controlled processing perspective: An fMRI study of neuroticism, extraversion, and self-consciousness. Cognitive, Affective, & Behavioral Neuroscience, 5(2), 169–181. https://doi.org/10.3758/cabn.5.2.169
- Endler, N. S., & Kocovski, N. L. (2001). State and trait anxiety revisited. Journal of Anxiety Disorders, 15(3), 231–245. https://doi.org/10.1016/S0887-6185(01)00060-3
- Engels, A. S., Heller, W., Mohanty, A., Herrington, J. D., Banich, M. T., Webb, A. G., & Miller, G. A. (2007). Specificity of regional brain activity in anxiety types during emotion processing. Psychophysiology, 44(3), 352–363. https://doi.org/10.1111/j.1469-8986.2007.00518.x
- Eysenck, M. W., & Calvo, M. G. (1992). Anxiety and performance: The processing efficiency theory. Cognition & Emotion, 6(6), 409–434. https://doi.org/10.1080/02699939208409696
- Eysenck, M. W., & Derakshan, N. (2011). New perspectives in attentional control theory. Personality and Individual Differences, 50(7), 955–960. https://doi.org/10.1016/j.paid.2010.08.019
- Eysenck, M. W., Derakshan, N., Santos, R., & Calvo, M. G. (2007). Anxiety and cognitive performance: Attentional control theory. Emotion, 7(2), 336–353. https://doi.org/10.1037/1528-3542.7.2.336
- Fales, C. L., Barch, D. M., Burgess, G. C., Schaefer, A., Mennin, D. S., Gray, J. R., & Braver, T. S. (2008). Anxiety and cognitive efficiency: Differential modulation of transient and sustained neural activity during a working memory task. Cognitive, Affective, & Behavioral Neuroscience, 8(3), 239–253. https://doi.org/10.3758/CABN.8.3.239
- Forster, S., Nunez Elizalde, A. O., Castle, E., & Bishop, S. J. (2015). Unraveling the anxious mind: Anxiety, worry, and frontal engagement in sustained attention versus Off-task processing. Cerebral Cortex, 25(3), 609–618. https://doi.org/10.1093/cercor/bht248
- Fox, K. C., Spreng, R. N., Ellamil, M., Andrews-Hanna, J. R., & Christoff, K. (2015). The wandering brain: Meta-analysis of functional neuroimaging studies of mind-wandering and related spontaneous thought processes. Neuroimage, 111, 611–621. https://doi.org/10.1016/j.neuroimage.2015.02.039
- Fox, M. D., Snyder, A. Z., Vincent, J. L., Corbetta, M., Van Essen, D. C., & Raichle, M. E. (2005). The human brain is intrinsically organized into dynamic, anticorrelated functional networks. Proceedings of the National Academy of Sciences, 102(27), 9673–9678. https://doi.org/10.1073/pnas.0504136102
- Gaspar, J. M., & McDonald, J. J. (2018). High level of trait anxiety leads to salience-driven distraction and compensation. Psychological Science, 956797618807166. https://doi.org/10.1177/0956797618807166
- Gehring, W. J., Liu, Y., Orr, J. M., & Carp, J. (2012). The error-related negativity (ERN/Ne). In S. J. Luck, & E. Kappenman (Eds.), Oxford handbook of event-related potential components. Oxford University Press.
- Gentili, C., Vanello, N., Cristea, I., David, D., Ricciardi, E., & Pietrini, P. (2015). Proneness to social anxiety modulates neural complexity in the absence of exposure: A resting state fMRI study using hurst exponent. Psychiatry Research: Neuroimaging, 232(2), 135–144. https://doi.org/10.1016/j.pscychresns.2015.03.005
- Grover, S., Nguyen, J. A., Viswanathan, V., & Reinhart, R. M. G. (2021). High-frequency neuromodulation improves obsessive-compulsive behavior. Nature Medicine, 27(2), 232–238. https://doi.org/10.1038/s41591-020-01173-w
- Gusnard, D. A., & Raichle, M. E. (2001). Searching for a baseline: Functional imaging and the resting human brain. Nature Reviews Neuroscience, 2(10), 685–694. https://doi.org/10.1038/35094500
- Hadwin, J. A., & Richards, H. J. (2016). Working memory training and CBT reduces anxiety symptoms and attentional biases to threat: A preliminary study. Frontiers in Psychology, 7, 47. https://doi.org/10.3389/fpsyg.2016.00047
- Hanrahan, F., Field, A. P., Jones, F. W., & Davey, G. C. (2013). A meta-analysis of cognitive therapy for worry in generalized anxiety disorder. Clinical Psychology Review, 33(1), 120–132. https://doi.org/10.1016/j.cpr.2012.10.008
- Heeren, A., Bernstein, E. E., & McNally, R. J. (2018). Deconstructing trait anxiety: A network perspective. Anxiety, Stress, & Coping, 31(3), 262–276. https://doi.org/10.1080/10615806.2018.1439263
- Heller, W., Nitschke, J. B., Etienne, M. A., & Miller, G. A. (1997). Patterns of regional brain activity differentiate types of anxiety. Journal of Abnormal Psychology, 106(3), 376–385. https://doi.org/10.1037/0021-843X.106.3.376
- Hepsomali, P., Hadwin, J. A., Liversedge, S. P., Degno, F., & Garner, M. (2019). The impact of cognitive load on processing efficiency and performance effectiveness in anxiety: Evidence from event-related potentials and pupillary responses. Experimental Brain Research, https://doi.org/10.1007/s00221-018-05466-y
- Hepsomali, P., Hadwin, J. A., Liversedge, S. P., & Garner, M. (2017). Pupillometric and saccadic measures of affective and executive processing in anxiety. Biological Psychology, 127, 173–179. https://doi.org/10.1016/j.biopsycho.2017.05.013
- Hirsch, C. R., Krahé, C., Whyte, J., Bridge, L., Loizou, S., Norton, S., & Mathews, A. (2020). Effects of modifying interpretation bias on transdiagnostic repetitive negative thinking. Journal of Consulting and Clinical Psychology, 88(3), 226–239. https://doi.org/10.1037/ccp0000455
- Hoffmann, S., Labrenz, F., Themann, M., Wascher, E., & Beste, C. (2014). Crosslinking EEG time-frequency decomposition and fMRI in error monitoring. Brain Structure and Function, 219(2), 595–605. https://doi.org/10.1007/s00429-013-0521-y
- Hopfinger, J. B., Buonocore, M. H., & Mangun, G. R. (2000). The neural mechanisms of top-down attentional control. Nature Neuroscience, 3(3), 284–291. https://doi.org/10.1038/72999
- Hotton, M., Derakshan, N., & Fox, E. (2018). A randomised controlled trial investigating the benefits of adaptive working memory training for working memory capacity and attentional control in high worriers. Behaviour Research and Therapy, 100, 67–77. https://doi.org/10.1016/j.brat.2017.10.011
- Hsieh, M. T., Lu, H., Lin, C. I., Sun, T. H., Chen, Y. R., & Cheng, C. H. (2021). Effects of trait anxiety on error processing and post-error adjustments: An event-related potential study With stop-signal task. Frontiers in Human Neuroscience, 15, 650838. https://doi.org/10.3389/fnhum.2021.650838
- Hugdahl, K., Kazimierczak, K., Beresniewicz, J., Kompus, K., Westerhausen, R., Ersland, L., … Specht, K. (2019). Dynamic up- and down-regulation of the default (DMN) and extrinsic (EMN) mode networks during alternating task-on and task-off periods. PLoS One, 14(9), e0218358. https://doi.org/10.1371/journal.pone.0218358
- Jansma, J. M., Ramsey, N. F., Slagter, H. A., & Kahn, R. S. (2001). Functional anatomical correlates of controlled and automatic processing. Journal of Cognitive Neuroscience, 13(6), 730–743. https://doi.org/10.1162/08989290152541403
- Jeong, H. J., & Cho, Y. S. (2021). The effects of induced and trait anxiety on the sequential modulation of emotional conflict. Psychological Research, 85(2), 618–633. https://doi.org/10.1007/s00426-020-01289-1
- Karch, S., Jäger, L., Karamatskos, E., Graz, C., Stammel, A., Flatz, W., … Mulert, C. (2008). Influence of trait anxiety on inhibitory control in alcohol-dependent patients: Simultaneous acquisition of ERPs and BOLD responses. Journal of Psychiatric Research, 42(9), 734–745. https://doi.org/10.1016/j.jpsychires.2007.07.016
- Klink, K., Paßmann, S., Kasten, F. H., & Peter, J. (2020). The modulation of cognitive performance with transcranial alternating current stimulation: A systematic review of frequency-specific effects. Brain Sciences, 10(12), https://doi.org/10.3390/brainsci10120932
- Larson, M. J., Clawson, A., Clayson, P. E., & Baldwin, S. A. (2013). Cognitive conflict adaptation in generalized anxiety disorder. Biological Psychology, 94(2), 408–418. https://doi.org/10.1016/j.biopsycho.2013.08.006
- Leigh, E., & Hirsch, C. R. (2011). Worry in imagery and verbal form: Effect on residual working memory capacity. Behaviour Research and Therapy, 49(2), 99–105. https://doi.org/10.1016/j.brat.2010.11.005
- Liu, L., Wu, J., Geng, H., Liu, C., Luo, Y., Luo, J., & Qin, S. (2021). Long-Term stress and trait anxiety affect brain network balance in dynamic cognitive computations. Cerebral Cortex, https://doi.org/10.1093/cercor/bhab393
- Lotfi, S., Ward, R. T., Ayazi, M., Bennett, K. P., Larson, C. L., & Lee, H.-J. (2021). The effects of emotional working memory training on worry symptoms and error-related negativity of individuals with high trait anxiety: A randomized controlled study. Cognitive Therapy and Research, 45(5), 969–985. https://doi.org/10.1007/s10608-020-10164-7
- Lukasik, K. M., Waris, O., Soveri, A., Lehtonen, M., & Laine, M. (2019). The relationship of anxiety and stress With working memory performance in a large Non-depressed sample. Frontiers in Psychology, 10, 4–4. https://doi.org/10.3389/fpsyg.2019.00004
- Makovac, E., Fagioli, S., Rae, C. L., Critchley, H. D., & Ottaviani, C. (2020). Can't get it off my brain: Meta-analysis of neuroimaging studies on perseverative cognition. Psychiatry Research: Neuroimaging, 295, 111020. https://doi.org/10.1016/j.pscychresns.2019.111020
- Maresh, E. L., Allen, J. P., & Coan, J. A. (2014). Increased default mode network activity in socially anxious individuals during reward processing. Biology of Mood & Anxiety Disorders, 4(1), 7. https://doi.org/10.1186/2045-5380-4-7
- Meyer, A. (2017). A biomarker of anxiety in children and adolescents: A review focusing on the error-related negativity (ERN) and anxiety across development. Developmental Cognitive Neuroscience, 27, 58–68. https://doi.org/10.1016/j.dcn.2017.08.001
- Meyer, A., Nelson, B., Perlman, G., Klein, D. N., & Kotov, R. (2018). A neural biomarker, the error-related negativity, predicts the first onset of generalized anxiety disorder in a large sample of adolescent females. Journal of Child Psychology and Psychiatry, 59(11), 1162–1170. https://doi.org/10.1111/jcpp.12922
- Moran, T. P., Bernat, E. M., Aviyente, S., Schroder, H. S., & Moser, J. S. (2015). Sending mixed signals: Worry is associated with enhanced initial error processing but reduced call for subsequent cognitive control. Social Cognitive and Affective Neuroscience, 10(11), 1548–1556. https://doi.org/10.1093/scan/nsv046
- Moran, T. P., & Moser, J. S. (2015). The color of anxiety: Neurobehavioral evidence for distraction by perceptually salient stimuli in anxiety. Cognitive, Affective, & Behavioral Neuroscience, 15(1), 169–179. https://doi.org/10.3758/s13415-014-0314-7
- Morgenroth, E., Saviola, F., Gilleen, J., Allen, B., Lührs, M., Eysenck, M. W., & Allen, P. (2020). Using connectivity-based real-time fMRI neurofeedback to modulate attentional and resting state networks in people with high trait anxiety. NeuroImage: Clinical, 25, 102191–102191. https://doi.org/10.1016/j.nicl.2020.102191
- Moser, J. S. (2017). The Nature of the Relationship between Anxiety and the Error-Related Negativity across Development. Curr Behav Neurosci Rep, 4(4), 309–321.
- Moser, J. S., Becker, M. W., & Moran, T. P. (2012). Enhanced attentional capture in trait anxiety. Emotion, 12(2), 213–216. https://doi.org/10.1037/a0026156
- Moser, J. S., Hartwig, R., Moran, T. P., Jendrusina, A. A., & Kross, E. (2014). Neural markers of positive reappraisal and their associations with trait reappraisal and worry. J Abnorm Psychol, 123(1), 91–105.
- Moser, J. S., Moran, T. P., & Leber, A. B. (2015). Manipulating attention to nonemotional distractors influences state anxiety: A proof-of-concept study in Low- and high-anxious college students. Behavior Therapy, 46(6), 834–843. https://doi.org/10.1016/j.beth.2015.07.001
- Moser, J. S., Moran, T. P., Schroder, H. S., Donnellan, M. B., & Yeung, N. (2013). On the relationship between anxiety and error monitoring: A meta-analysis and conceptual framework. Frontiers in Human Neuroscience, 7, 466–466. https://doi.org/10.3389/fnhum.2013.00466
- Neo, P. S., Thurlow, J. K., & McNaughton, N. (2011). Stopping, goal-conflict, trait anxiety and frontal rhythmic power in the stop-signal task. Cognitive, Affective, & Behavioral Neuroscience, 11(4), 485–493. https://doi.org/10.3758/s13415-011-0046-x
- Nitschke, J. B., Heller, W., Imig, J. C., McDonald, R. P., & Miller, G. A. (2001). Distinguishing dimensions of anxiety and depression. https://doi.org/10.1023/A:1026485530405
- Northoff, G. (2020). Anxiety disorders and the brain’s resting state networks: From altered spatiotemporal synchronization to psychopathological symptoms. In Y.-K. Kim (Ed.), Anxiety disorders: Rethinking and understanding recent discoveries (pp. 71–90). Springer Singapore. https://doi.org/10.1007/978-981-32-9705-0_5
- Olvet, D. M., & Hajcak, G. (2008). The error-related negativity (ERN) and psychopathology: Toward an endophenotype. Clinical Psychology Review, 28(8), 1343–1354. https://doi.org/10.1016/j.cpr.2008.07.003
- Osinsky, R., Alexander, N., Gebhardt, H., & Hennig, J. (2010). Trait anxiety and dynamic adjustments in conflict processing. Cognitive, Affective, & Behavioral Neuroscience, 10(3), 372–381. https://doi.org/10.3758/CABN.10.3.372
- Osinsky, R., Gebhardt, H., Alexander, N., & Hennig, J. (2012). Trait anxiety and the dynamics of attentional control. Biological Psychology, 89(1), 252–259. https://doi.org/10.1016/j.biopsycho.2011.10.016
- Osinsky, R., Karl, C., & Hewig, J. (2017). Dispositional anxiety and frontal-midline theta: On the modulatory influence of Sex and situational threat. Journal of Personality, 85(3), 300–312. https://doi.org/10.1111/jopy.12241
- Owens, M., Derakshan, N., & Richards, A. (2015). Trait susceptibility to worry modulates the effects of cognitive load on cognitive control: An ERP study. Emotion, 15(5), 544–549. https://doi.org/10.1037/emo0000052
- Paulus, M. P., Feinstein, J. S., Simmons, A., & Stein, M. B. (2004). Anterior cingulate activation in high trait anxious subjects is related to altered error processing during decision making. Biological Psychiatry, 55(12), 1179–1187. https://doi.org/10.1016/j.biopsych.2004.02.023
- Poldrack, R. A. (2015). Is “efficiency” a useful concept in cognitive neuroscience? Developmental Cognitive Neuroscience, 11, 12–17. https://doi.org/10.1016/j.dcn.2014.06.001
- Poldrack, R. A., Sabb, F. W., Foerde, K., Tom, S. M., Asarnow, R. F., Bookheimer, S. Y., & Knowlton, B. J. (2005). The neural correlates of motor skill automaticity. Journal of Neuroscience, 25(22), 5356. https://doi.org/10.1523/JNEUROSCI.3880-04.2005
- Raichle, M. E. (2015). The restless brain: How intrinsic activity organizes brain function. Philosophical Transactions of the Royal Society B: Biological Sciences, 370(1668), 20140172. https://doi.org/10.1098/rstb.2014.0172
- Raichle, M. E., MacLeod, A. M., Snyder, A. Z., Powers, W. J., Gusnard, D. A., & Shulman, G. L. (2001). A default mode of brain function. Proceedings of the National Academy of Sciences, 98(2), 676–682. https://doi.org/10.1073/pnas.98.2.676
- Remes, O., Brayne, C., van der Linde, R., & Lafortune, L. (2016). A systematic review of reviews on the prevalence of anxiety disorders in adult populations. Brain and Behavior, 6(7), e00497. https://doi.org/10.1002/brb3.497
- Righi, S., Mecacci, L., & Viggiano, M. P. (2009). Anxiety, cognitive self-evaluation and performance: ERP correlates. Journal of Anxiety Disorders, 23(8), 1132–1138. https://doi.org/10.1016/j.janxdis.2009.07.018
- Robison, M. K., Gath, K. I., & Unsworth, N. (2017). The neurotic wandering mind: An individual differences investigation of neuroticism, mind-wandering, and executive control. Quarterly Journal of Experimental Psychology, 70(4), 649–663. https://doi.org/10.1080/17470218.2016.1145706
- Sagalakova, O. A., Truevtsev, D. V., & Zhirnova, O. V. (2021). Error-related negativity: Neurocognitive transdiagnostic marker of anxiety disorders. Neurology Bulletin, LIII(1), 80–83. https://doi.org/10.17816/nb58800
- Sandi, C., & Richter-Levin, G. (2009). From high anxiety trait to depression: A neurocognitive hypothesis. Trends in Neurosciences, 32(6), 312–320. https://doi.org/10.1016/j.tins.2009.02.004
- Santos, R., Wall, M. B., & Eysenck, M. W. (2006). Anxiety and processing efficiency: fMRI evidence.
- Sari, B. A., Koster, E. H., Pourtois, G., & Derakshan, N. (2016). Training working memory to improve attentional control in anxiety: A proof-of-principle study using behavioral and electrophysiological measures. Biological Psychology, 121(Pt B), 203–212. https://doi.org/10.1016/j.biopsycho.2015.09.008
- Savostyanov, A. N., Tsai, A. C., Liou, M., Levin, E. A., Lee, J., Yurganov, A. V., & Knyazev, G. G. (2009). EEG-correlates of trait anxiety in the stop-signal paradigm. Neuroscience Letters, 449(2), 112–116. https://doi.org/10.1016/j.neulet.2008.10.084
- Schmidt, B., Kanis, H., Holroyd, C. B., Miltner, W. H. R., & Hewig, J. (2018). Anxious gambling: Anxiety is associated with higher frontal midline theta predicting less risky decisions. Psychophysiology, 55(10), e13210. https://doi.org/10.1111/psyp.13210
- Schroder, H. S., Glazer, J. E., Bennett, K. P., Moran, T. P., & Moser, J. S. (2017). Suppression of error-preceding brain activity explains exaggerated error monitoring in females with worry. Biological Psychology, 122, 33–41. https://doi.org/10.1016/j.biopsycho.2016.03.013
- Seeley, W. W., Menon, V., Schatzberg, A. F., Keller, J., Glover, G. H., Kenna, H., … Greicius, M. D. (2007). Dissociable intrinsic connectivity networks for salience processing and executive control. Journal of Neuroscience, 27(9), 2349. https://doi.org/10.1523/JNEUROSCI.5587-06.2007
- Sehlmeyer, C., Konrad, C., Zwitserlood, P., Arolt, V., Falkenstein, M., & Beste, C. (2010). ERP indices for response inhibition are related to anxiety-related personality traits. Neuropsychologia, 48(9), 2488–2495. https://doi.org/10.1016/j.neuropsychologia.2010.04.022
- Servaas, M. N., Geerligs, L., Renken, R. J., Marsman, J. B., Ormel, J., Riese, H., & Aleman, A. (2015). Connectomics and neuroticism: An altered functional network organization. Neuropsychopharmacology, 40(2), 296–304. https://doi.org/10.1038/npp.2014.169
- Servaas, M. N., Riese, H., Ormel, J., & Aleman, A. (2014). The neural correlates of worry in association with individual differences in neuroticism. Human Brain Mapping, 35(9), 4303–4315. https://doi.org/10.1002/hbm.22476
- Shi, X., & Wu, J. (2020). Chronic stress and anticipatory event-related potentials: The moderating role of resilience. Stress, 23(5), 607–613. https://doi.org/10.1080/10253890.2020.1766019
- Shirer, W. R., Ryali, S., Rykhlevskaia, E., Menon, V., & Greicius, M. D. (2012). Decoding subject-driven cognitive states with whole-brain connectivity patterns. Cerebral Cortex, 22(1), 158–165. https://doi.org/10.1093/cercor/bhr099
- Silton, R. L., Heller, W., Engels, A. S., Towers, D. N., Spielberg, J. M., Edgar, J. C., … Miller, G. A. (2011). Depression and anxious apprehension distinguish frontocingulate cortical activity during top-down attentional control. Journal of Abnormal Psychology, 120(2), 272–285. https://doi.org/10.1037/a0023204
- Silton, R. L., Heller, W., Towers, D. N., Engels, A. S., Spielberg, J. M., Edgar, J. C., … Miller, G. A. (2010). The time course of activity in dorsolateral prefrontal cortex and anterior cingulate cortex during top-down attentional control. Neuroimage, 50(3), 1292–1302. https://doi.org/10.1016/j.neuroimage.2009.12.061
- Spielberg, J. M., De Leon, A. A., Bredemeier, K., Heller, W., Engels, A. S., Warren, S. L., … Miller, G. A. (2013). Anxiety type modulates immediate versus delayed engagement of attention-related brain regions. Brain and Behavior, 3(5), 532–551. https://doi.org/10.1002/brb3.157
- Stefanopoulou, E., Hirsch, C. R., Hayes, S., Adlam, A., & Coker, S. (2014). Are attentional control resources reduced by worry in generalized anxiety disorder? Journal of Abnormal Psychology, 123(2), 330–335. https://doi.org/10.1037/a0036343
- Steinfurth, E. C. K., Alius, M. G., Wendt, J., & Hamm, A. O. (2017). Physiological and neural correlates of worry and rumination: Support for the contrast avoidance model of worry. Psychophysiology, 54(2), 161–171. https://doi.org/10.1111/psyp.12767
- Suárez-Pellicioni, M., Núñez-Peña, M. I., & Colomé, À. (2014). Reactive recruitment of attentional control in math anxiety: An ERP study of numeric conflict monitoring and adaptation. PloS One, 9(6), e99579–e99579. https://doi.org/10.1371/journal.pone.0099579
- Sylvester, C. M., Corbetta, M., Raichle, M. E., Rodebaugh, T. L., Schlaggar, B. L., Sheline, Y. I., … Lenze, E. J. (2012). Functional network dysfunction in anxiety and anxiety disorders. Trends in Neurosciences, 35(9), 527–535. https://doi.org/10.1016/j.tins.2012.04.012
- Tsai, Y. C., Lu, H. J., Chang, C. F., Liang, W. K., Muggleton, N. G., & Juan, C. H. (2017). Electrophysiological and behavioral evidence reveals the effects of trait anxiety on contingent attentional capture. Cognitive, Affective, & Behavioral Neuroscience, 17(5), 973–983. https://doi.org/10.3758/s13415-017-0526-8
- Weber-Goericke, F., & Muehlhan, M. (2019). A quantitative meta-analysis of fMRI studies investigating emotional processing in excessive worriers: Application of activation likelihood estimation analysis. Journal of Affective Disorders, 243, 348–359. https://doi.org/10.1016/j.jad.2018.09.049
- Weissman, D. H., Roberts, K. C., Visscher, K. M., & Woldorff, M. G. (2006). The neural bases of momentary lapses in attention. Nature Neuroscience, 9(7), 971–978. https://doi.org/10.1038/nn1727
- Wheaton, M. G., Fitzgerald, D. A., Phan, K. L., & Klumpp, H. (2014). Perceptual load modulates anterior cingulate cortex response to threat distractors in generalized social anxiety disorder. Biological Psychology, 101, 13–17. https://doi.org/10.1016/j.biopsycho.2014.06.004
- Wittchen, H. U., Jacobi, F., Rehm, J., Gustavsson, A., Svensson, M., Jönsson, B., … Steinhausen, H. C. (2011). The size and burden of mental disorders and other disorders of the brain in Europe 2010. European Neuropsychopharmacology, 21(9), 655–679. https://doi.org/10.1016/j.euroneuro.2011.07.018
- Wright, C. A., Dobson, K. S., & Sears, C. R. (2014). Does a high working memory capacity attenuate the negative impact of trait anxiety on attentional control? Evidence from the antisaccade task. Journal of Cognitive Psychology, 26(4), 400–412. https://doi.org/10.1080/20445911.2014.901331
- Xia, L., Mo, L., Wang, J., Zhang, W., & Zhang, D. (2020). Trait anxiety attenuates response inhibition: Evidence from an ERP study using the Go/NoGo task. Frontiers in Behavioral Neuroscience, 14(28).
- Yang, L., & Li, X.-B. (2014). Anxiety alters brain activity of response inhibition: Evidence from event-related potentials and source current density analysis. 160–163. https://doi.org/10.1109/icist.2014.6920355
- Yu, Y., Jiang, C., Xu, H., Yang, Q., Li, J., Xu, Y., Xiang, W., Peng, L., Liu, B., Lv, F., & Li, M. (2018). Impaired cognitive control of emotional conflict in trait anxiety: A preliminary study based on clinical and Non-clinical individuals. Frontiers in Psychiatry, 9(120).
- Zanto, T. P., & Gazzaley, A. (2013). Fronto-parietal network: Flexible hub of cognitive control. Trends in Cognitive Sciences, 17(12), 602–603. https://doi.org/10.1016/j.tics.2013.10.001
- Zhao, X., Dang, C., & Maes, J. H. R. (2020). Effects of working memory training on EEG, cognitive performance, and self-report indices potentially relevant for social anxiety. Biological Psychology, 150, 107840. https://doi.org/10.1016/j.biopsycho.2019.107840
- Zhou, H.-X., Chen, X., Shen, Y.-Q., Li, L., Chen, N.-X., Zhu, Z.-C., … Yan, C.-G. (2020). Rumination and the default mode network: Meta-analysis of brain imaging studies and implications for depression. NeuroImage, 206, 116287. https://doi.org/10.1016/j.neuroimage.2019.116287