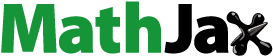
ABSTRACT
Self-relevance has been demonstrated to impair instrumental learning. Compared to unfamiliar symbols associated with a friend, analogous stimuli linked with the self are learned more slowly. What is not yet understood, however, is whether this effect extends beyond arbitrary stimuli to material with intrinsically meaningful properties. Take, for example, stimulus valence an established moderator of self-bias. Does the desirability of to-be-learned material influence self-learning? Here, in conjunction with computational modelling (i.e. Reinforcement Learning Drift Diffusion Model analysis), a probabilistic selection task was used to establish if and how stimulus valence (i.e. desirable/undesirable posters) impacts the acquisition of knowledge relating to object-ownership (i.e. owned-by-self vs. owned-by-friend). Several interesting results were observed. First, undesirable posters were learned more rapidly for self compared to friend, an effect that was reversed for desirable posters. Second, learning rates were accompanied by associated differences in reward sensitivity toward desirable and undesirable choice selections as a function of ownership. Third, decisional caution was greater for self-relevant (vs. friend relevant) responses. Collectively, these findings inform understanding of self-function and how valence and stimulus relevance mutually influence probabilistic learning.
As a basic psychological construct, the self provides stability, continuity, and meaning in a complex world (Baars, Citation1988; Baumeister, Citation1998; Conway, Citation2005; Conway & Pleydell-Pearce, Citation2000; Gallagher, Citation2000; James, Citation1890; Markus & Nurius, Citation1986; Markus & Wurf, Citation1987; Oakley & Halligan, Citation2017). Pervading all aspects of social-cognitive functioning, it shapes thinking and doing, serving as a processing hub through which stimulus appraisal and action initiation unfold (Humphreys & Sui, Citation2016; Sui & Humphreys, Citation2015, Citation2017). As Markus and Wurf (Citation1987, p. 300) reported, the self “ … is a dynamic interpretative structure that mediates most significant intrapersonal processes (including information processing, affect, and motivation) and a wide variety of interpersonal processes”.
Over several decades, extensive empirical efforts have focused on exploring how manifestations of self-bias can inform theoretical understanding of self-function (Sui & Humphreys, Citation2017; Symons & Johnson, Citation1997). These endeavours have been productive. Regardless of the domain of interest (e.g. memory, attention, decision-making), personally significant material biases information processing and response selection in emphatically self-enhancing and self-prioritising ways (e.g. Conway, Citation2005; Mezulis et al., Citation2004; Sedikides & Alicke, Citation2012; Sui & Rotshtein, Citation2019; Symons & Johnson, Citation1997). For example, compared to stimuli associated with other people (e.g. friend, parent, stranger), items linked with the self are easier to detect, evaluate, and remember (Alexopoulos et al., Citation2012; Bargh & Pratto, Citation1986; Gray et al., Citation2004; Kuiper & Rogers, Citation1979; Shapiro et al., Citation1997; Symons & Johnson, Citation1997). Moreover, reflecting the potency of self-relevance, these facilitatory effects emerge not only for highly familiar stimuli (e.g. one’s face or forename), but also arbitrary items (e.g. shapes, colours, objects) that have been associated with the self (Constable et al., Citation2019; Falbén, Golubickis, Tamulaitis, et al., Citation2020; Golubickis et al., Citation2018, Citation2020; Macrae et al., Citation2017, Citation2018; Schäfer et al., Citation2015, Citation2016; Sui et al., Citation2012; Woźniak & Knoblich, Citation2019; Yin et al., Citation2019). For some, these findings have suggested that self-bias may be an inescapable facet of mental life (Sui & Humphreys, Citation2017; but see Golubickis & Macrae, Citation2023).
The self and learning
Motivating the current inquiry, a recent line of investigation has considered the extent to which self-relevance influences another fundamental psychological process; learning (Golubickis & Macrae, Citation2022; Liao et al., Citation2021; Lockwood et al., Citation2018). Just as the personal significance of material facilitates information processing (Humphreys & Sui, Citation2016; Sui & Humphreys, Citation2015, Citation2017), then so too it may enhance the rate at which knowledge is acquired. At least in the context of probabilistic learning, however, Golubickis and Macrae (Citation2022) demonstrated that this was not in fact the case. Using a probabilistic selection task (PST), participants were presented with three different stimulus pairs – comprising Japanese Hiragana characters – with an item in each pairing representing either self or a friend. Their objective was to learn which of the characters was most likely to denote self and friend based on probabilistic feedback that was provided following repeated choice selections (Frank et al., Citation2004, Citation2007).
The PST is useful as it taps into reward sensitivity (i.e. how effectively expected values are integrated into the process of evidence accumulation during decision-making) which is related to the fundamental exploration-exploitation dilemma that underpins learning (Cohen et al., Citation2007; Daw et al., Citation2006; Sutton & Barto, Citation1998). The quandary is straightforward. When competing options are readily available, is it better to make choice selections that have typically been rewarding in the past (i.e. exploitation) or instead choose novel options of unknown utility (i.e. exploration)? Although optimal learning calls for a balance between these rival strategies, over time, exploration facilitates the acquisition of knowledge. The benefits of exploitation, in contrast, derive from the provision of immediate decisional rewards (Sutton & Barto, Citation1998).
Adopting a computational approach to probe the characteristics of self-learning (Reinforcement Drift Diffusion Model [RL-DDM] analysis; Fontanesi et al., Citation2019; Pedersen et al., Citation2017; Pedersen & Frank, Citation2020), Golubickis and Macrae (Citation2022) reported several interesting findings. Most notably, learning rates were slower for self-relevant compared to friend-relevant Hiragana characters and self-learning (vs. friend-learning) was characterised by increased reward sensitivity, indicating that knowledge acquisition favoured the exploitation of previously successful choice selections. That is, formerly rewarded self-related characters were chosen more often than novel (i.e. potentially riskier) choice selections, a strategy that ultimately reduced the rate of learning (Sutton & Barto, Citation1998). Thus, deviating from extant research underscoring the benefits of self-relevance (Humphreys & Sui, Citation2016; Sui & Humphreys, Citation2015, Citation2017), personally relevant stimuli slowed rather than speeded probabilistic learning.
To account for their findings, Golubickis and Macrae (Citation2022) highlighted the role of reward-related processing during learning. According to a popular theoretical account, self-referential cognition is underpinned by the inherently rewarding properties of self-associated (vs. other-associated) stimuli (Northoff & Hayes, Citation2011). Indeed, research has demonstrated considerable overlap in the mechanisms that support self-referential and emotional processing, particularly with regard to positive emotions (Gutchess & Kensinger, Citation2018; Yankouskaya & Sui, Citation2022). Given the fundamental contribution of reward value (i.e. dopamine uptake) during learning (Dayan & Balleine, Citation2002; Schultz, Citation1998; Sutton & Barto, Citation1998), it is therefore understandable that the selection of previously successful self-related (vs. friend-related) stimuli was preferred to novel options. Albeit at the cost of slowed learning, selecting formerly successful outcomes yielded the most potent choice-related rewards, even when the stimuli in question were not intrinsically rewarding, but rather acquired their positivity through self-association.
In keeping with contemporary research on self-prioritisation, the to-be-learned material in Golubickis and Macrae’s (Citation2022) experiments comprised arbitrary stimuli that held no prior significance for participants (Golubickis et al., Citation2018; Sui et al., Citation2012). It therefore remains to be seen whether comparable effects would emerge with items that are intrinsically meaningful and have implications for self-function. Take, for example, stimulus valence, a dimension of obvious importance outside the laboratory. A developing literature has shown item desirability to be an important determinant of self-prioritisation. Across a range of tasks (e.g. shape-label matching, object categorisation), self-association typically benefits only desirable (vs. undesirable) stimuli, thereby supplying further evidence for the positivity biases that typify self-referential processing (Constable et al., Citation2021; Golubickis et al., Citation2021; Sedikides & Strube, Citation1997; Vicovaro et al., Citation2022; Ye & Gawronski, Citation2016). As a case in point, using an ownership-categorisation task, Golubickis et al. (Citation2021) demonstrated facilitated (i.e. speeded) responding when desirable posters were owned-by-self (vs. owned-by-a-friend) and undesirable posters were owned-by-a-friend (vs. owned-by-self). In other words, as a function of ownership (i.e. self vs. other), both positivity and negativity effects were observed (Unkelbach et al., Citation2020).
Given the need to maintain positive self-regard (Taylor & Brown, Citation1988), the prioritisation of desirable stimuli serves as a cognitive pathway through which this objective can be accomplished (Constable et al., Citation2021; Golubickis et al., Citation2021; Ye & Gawronski, Citation2016). In the context of instrumental learning, of course, this raises several interesting issues. As has been observed for arbitrary items, does self-relevance similarly impair (i.e. slow) learning when the stimuli under consideration vary in valence? Specifically, to what extent does stimulus desirability influence reward sensitivity, as manifest in people’s tendency to select previously successful self-relevant compared to friend-relevant options during decision-making? Given the lure of immediate decisional rewards (Golubickis & Macrae, Citation2022), it is possible that reward sensitivity may be elevated when individuals are tasked with establishing which positive items are associated with the self compared to a friend. This is unlikely to be the case, however, when negative items comprise the correct choice selections (i.e. decisional rewards are offset when undesirable stimuli are associated with the self – Golubickis et al., Citation2021; Greenwald et al., Citation2002; Ye & Gawronski, Citation2016). If indeed the case, then this would suggest that self-relevance may impair the rate of learning for positive compared to negative material, a possibility we explored in the current investigation.
The current research
To establish if and how the valence of material influences the rate of self-related (vs. friend-related) learning, here we adopted a modified PST (Frank et al., Citation2004, Citation2007). Participants were presented with six different pairs of desirable or undesirable posters and, on a trial-by-trial basis, were required to learn which poster in each pairing was most likely to be owned by either self or friend (i.e. three pairings positively reinforced self-ownership and three pairings positively reinforced friend-ownership). Critically, the feedback provided after each selection was probabilistic and varied across the stimulus pairs. For example, on a third of trials (i.e. trials including one stimulus pair), choosing one poster led to positive feedback (i.e. correct) on 80% of trials, whereas selecting the other poster led to positive reinforcement on only 20% of the trials. These probabilities were 70%−30%, and 60%−40% for each of the other pairs of posters. As such, over numerous choice selections (i.e. self-learning trials & friend-learning trials), by gathering information on which choices were rewarded most frequently, participants learned which posters were likely to be owned by each of the targets.
Following Golubickis and Macrae (Citation2022), to identify the processes underpinning learning a RL-DDM analysis was undertaken on the data (Fontanesi et al., Citation2019; Pedersen et al., Citation2017; Pedersen & Frank, Citation2020). Integrating sequential sampling and reinforcement learning models, the RL-DDM elucidates the decisional processes that support learning and how these evolve as learning progresses (Miletić et al., Citation2020; Ratcliff et al., Citation2016). This is achieved through the simultaneous hierarchical Bayesian modelling of response time and choice data. A process central to the model’s operation is the implementation of the delta learning rule which updates expected values about the stimulus on each trial it appears. Through this rule, the RL-DDM effectively captures the dynamic nature of learning, reflecting how participants assimilate and adjust their beliefs based on feedback received. It is this continual updating process that allows for the computation of an overall learning rate, encapsulating the cumulative speed at which participants modify their expectations in response to new information (i.e. feedback/reward). The learning rate parameter (η) then quantifies the efficacy of this updating process. Larger η values denote a faster acquisition of knowledge based on feedback, indicative of rapid learning, whereas smaller η values indicate expected value integration at a reduced rate, characterising slow learning. More formally, the learning rate governs the extent to which prediction error – the discrepancy between predicted and actual rewards received – influences the updating of the expected Q-value for a chosen option at a trial t (Rescorla & Wagner, Citation1972; Watkins & Dayan, Citation1992, see Eq. 1).
(1)
(1) This equation succinctly encapsulates how each encounter with a stimulus pair leads to an adjusted expectation, shaping future decision-making in light of the newly acquired information. Additionally, to account for two competing stimuli being presented in each trial that yield different informational value (i.e. probability to be correct), the evidence accumulation process is formulated and adjusted by how efficiently the expected beliefs of both positively (Q+) and negatively reinforced (Q−) stimuli are utilised. A drift rate scaling parameter (vscaling), therefore, effectively measures the sensitivity and reliance on the acquired knowledge about stimulus probabilities. See Eq. (2):
(2)
(2) In this way, vscaling is similar to the inverse temperature parameter (β) in classic RL models which scales the difference between the expected values of positively and negatively reinforced options. In other words, this parameter reflects the degree to which the subjective expected values of choices influence the evidence accumulation process, such that higher values indicate the efficient and more confident integration of knowledge during decision-making and often can be associated with the exploitation (vs. exploration) of choice selections (Pedersen & Frank, Citation2020). Specifically, in the context of the current PST in which participants were presented with two stimuli on each trial and learned which one was most likely to be correct, exploration refers to the tendency to sample and consider both options (lower sensitivity to previously learned rewards), rather than consistently selecting the option that signals higher expected value (i.e. exploitation; higher sensitivity to previously learned rewards). The RL-DDM also establishes how much evidence is needed before a decision is made (i.e. threshold separation, a), with larger (vs. smaller) values indicating greater response caution. Finally, the efficiency of non-decisional processes (e.g. stimulus encoding, response execution, t0) is also estimated. Based on previous research (Golubickis et al., Citation2021; Golubickis & Macrae, Citation2022) we expected that, for positive posters, learning rates would be faster for friend compared to self, whereas for negative posters this effect would be reversed, such that learning rates would be faster for self than friend.
Method
Participants and design
Sixty participants (41 females, 16 males, 3 others; Mage = 22.04, SD = 2.32), with normal or corrected-to-normal visual acuity, took part in the experiment. Data collection was conducted online using Prolific Academic (www.prolific.co), with each participant receiving compensation at the rate of £7.50 (∼$10) per hour. Eight participants (6 females, 2 males) were excluded due to failures in the attention checks (Oppenheimer et al., Citation2009). Informed consent was obtained from participants prior to the commencement of the experiment and the protocol was reviewed and approved by the Ethics Committee at the School of Psychology, University of Aberdeen. The experiment had a 2 (Owner: self or friend) × 2 (Valence: positive or negative) mixed design with repeated measures on the first factor. To detect a significant effect (i.e. Owner × Valence interaction) with a large effect size (i.e. d = .80; Golubickis et al., Citation2021), a sample of fifty-two participants afforded 93% power (PANGEA, v .0.2).
Stimulus materials and procedure
Participants performed a PST (Frank et al., Citation2004, Citation2007) comprising a learning phase in which six pairs of stimuli were presented consisting of either positive or negative posters. Of these, three pairs were designated for self-learning trials, where an item in each pair belonged to the participant, and the other three pairs were allocated for friend-learning trials, where an item in each pair was associated with a friend. Participants were informed their task was to establish which posters were most likely to be owned by each of the targets (i.e. positively reinforced), whereas the remaining item (i.e. negatively reinforced) was not associated with any target. Following previous research, participants were requested to bring their best friend (i.e. the owner of the posters) to mind prior to performing the task (Golubickis et al., Citation2018). Half of the participants were randomly assigned to perform a version of the PST in which desirable posters were presented on both self-learning and friend-learning trials, whereas the others performed a PST during which undesirable posters were presented. On a trial-by-trial basis (Golubickis & Macrae, Citation2022), before the appearance of each stimulus pair, a cue (i.e. “YOU” or “FRIEND”) appeared on the screen indicating the target to which the posters referred (see ). Thus, overall, there were three stimulus pairs (i.e. AB, CD, EF) within each of the four learning environments (i.e. self/positive, self/negative, friend/positive, friend/negative). Based on feedback provided (i.e. onscreen information indicating whether the response was correct or incorrect), participants were told their task was to learn which poster in each pair was most likely to be owned-by-self (on self-learning trials) or owned-by-friend (on friend-learning trials).
The probabilities indicating which poster in each pairing (i.e. AB, CD, EF) was more likely to be correct followed the standard version of the PST (Frank et al., Citation2004, Citation2007). The letters AB, CD, and EF indicate two types of important information about the stimuli in the task: the winning (i.e. more likely to be correct) poster in each pairing, and the likelihood of each poster to be correct. Specifically, for the AB pair, A was 80% likely to be correct (20% for B), for the CD pair, C was 70% likely to be correct (30% for D), and finally, for the EF pair, E was 60% likely to be correct (40% for F). Over numerous choice selections, participants learned which item in each pairing was more likely to be correct based on the feedback provided. The task was completed when participants reached sufficient levels of accuracy for each pairing (i.e. AB, 60% or above; CD, 55% or above; EF, 50% or above; Frank et al., Citation2004, Citation2007). The posters (see Supplemental Material) comprised 12 desirable and 12 undesirable colour images taken from the OASIS database (Kurdi et al., Citation2017). Of the 12 posters in each of the positive and negative conditions, six were randomly assigned to self-learning while the other six were assigned to friend-learning. Based on the ratings in the database, the images differed significantly in valence (Ms: positive = 5.41, negative = 2.82, t(22) = 11.13, p < .001); but were equivalent in terms of arousal (Ms: positive = 3.42, negative = 3.37, t(22) = 0.08, p = .935).
Each trial began with the presentation of an ownership cue above a pair of posters that remained on the screen until the participant made a response. After the participant selected one of the posters, feedback (i.e. the word “Correct” in green or “Incorrect” in red) was presented for 1000 ms, followed by a blank screen for 500 ms, after which the next trial commenced (i.e. “correct” feedback = positive reinforcement, “incorrect” feedback = negative reinforcement). Participants had to select a poster by pressing the appropriate button on the keyboard (i.e. “A” for the poster on the left side of the screen, “L” for the poster on the right side of the screen). The posters in each pair were equally likely to be presented on the left or right side of the screen and assignment to each stimulus pairing (i.e. AB, CD, EF) was randomised. The experiment was conducted using Inquisit Web. Participants completed blocks of 60 trials in which each of the three stimulus pairs appeared randomly, equally often, until accuracy reached a satisfactory level. The maximum number of learning blocks was set to six (i.e. 360 trials in total) if the participant had not reached satisfactory levels of accuracy earlier in the task (Frank et al., Citation2007). On completion of the experiment, participants were debriefed and thanked.
Results
Behavioural analysis
Responses faster than 200 ms were excluded from the analysis, eliminating approximately 1% of the trials. A 2 (Owner: self or friend) × 2 (Valence: positive or negative) mixed model analysis of variance (ANOVA) was conducted on the response time and learning performance data (see for treatment means). These analyses revealed no significant effects on either measure.
Table 1. Mean response times (ms) and learning performance (%) as a function of Owner and Valence.
Additionally, using generalised estimating equations (GEE), a trial-by-trial analysis was conducted on the first 20 responses to explore the effects of Trial, Stimulus Pair, Owner, and Valence on learning performance (see ). A main effect of Trial (Wald χ2(1) = 10.85, p = .001), and a significant Trial X Stimulus Pair interaction (Wald χ2(1) = 8.30, p = .004) were observed. Follow-up analyses revealed that, for the AB stimulus pair, learning performance increased as a function of trial number (Wald χ2(1) = 16.10, p < .001). For both the CD (Wald χ2(1) = 3.48, p = .062) and EF (Wald χ2(1) = 0.08, p = .774) stimulus pairs, this effect was not significant.
Figure 2. Based on ceiling performance during the PST, Learning curves over the first 20 trials as a function of Owner, Valence, and Stimulus Pair. The raw data points represent the observed learning outcomes, and the modelled curves reflect the results of the generalised estimating equations (GEE) analysis. The GEE analysis examines differences in learning trajectories across trials and experimental conditions.
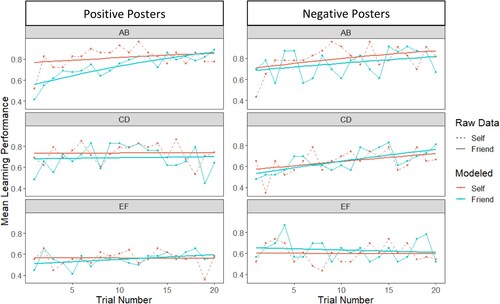
Modeling analysis
To estimate model parameters, the data were submitted to an extension of the Bayesian hierarchical drift diffusion toolbox (Pedersen & Frank, Citation2020; Wiecki et al., Citation2013). Models were response-coded, such that the upper threshold corresponded to responses to stimuli that were positively reinforced (i.e. “correct” – posters corresponding to the letters A, C, & E) and the lower threshold to stimuli that were negatively reinforced (i.e. “incorrect” – posters corresponding to the letters B, D, & F; Pedersen & Frank, Citation2020). Bayesian posterior distributions were modelled using a Markov chain Monte Carlo (MCMC) with 10,000 samples (including 1,000 burn), with outliers (5% of the trials) removed by the HDDM software (Ratcliff & Tuerlinckx, Citation2002; Wiecki et al., Citation2013). Model comparison was assessed using the Deviance Information Criterion (DIC) as this method is routinely adopted when comparing hierarchical Bayesian models (Spiegelhalter et al., Citation1998, Citation2002). Lower DIC values favour models with the highest likelihood and least number of parameters.
Several models were tested for comparison. Based on prior research (Golubickis et al., Citation2021; Golubickis & Macrae, Citation2022), Model 1 allowed drift rate scaling (vscaling), boundary separation (a) and learning rate (η) to vary as a function of Owner and Valence (DIC = 51,535). In Model 2, an additional starting point parameter (z) was also estimated (DIC = 51,202). Finally, in Model 3, intertrial variability for drift rate scaling (sv), non-decision time (st) and starting point (sz) were also estimated (DIC = 49,341). Model 3 yielded the best fit. This model also converged well across three chains of 10,000 samples and 1000 burn (Ȓ = 1.004; Gelman & Rubin, Citation1992; Pedersen et al., Citation2017). To further evaluate the best fitting model, a Posterior Predictive Check (PPC) was performed (Pedersen & Frank, Citation2020; Wiecki et al., Citation2013). Using the posterior distributions from Model 3, data sets were estimated. The quality of model fit was then gauged by comparing the simulated and observed data for choice proportions and decision times for each stimulus pair (AB, CD, EF; Pedersen et al., Citation2017; Pedersen & Frank, Citation2020). A visual review of the PPC suggested a good model fit (see Supplemental Material for plots). Examination of the posterior distributions for the best fitting model revealed differences in learning rate (η), drift rate scaling (vscaling), and threshold separation (a). The parameter estimates are listed in the Supplemental Material.
Learning rate (η)
The analysis yielded evidence that, for positive posters, the learning rate was faster for friend-owned compared to self-owned items (pBayes (friend > self) < .001, BF10 > 1000). For negative posters, in contrast, this effect was reversed, such that learning was faster for self-owned than friend-owned stimuli (pBayes (friend > self) = .23, BF10 = 3; see ).
Drift rate scaling (v)
The analysis yielded strong evidence that, for positive posters, drift rate scaling (i.e. reward sensitivity) was larger for self-owned compared to friend-owned items (pBayes (friend < self) < .001, BF10 > 1000; see ). For negative posters, in contrast, drift rate scaling was larger for friend-owned than self-owned stimuli (pBayes (friend > self) = .004, BF10 > 249; see ).
Boundary separation (a)
The analysis revealed strong evidence that the evidential requirements of response selection were higher for negative compared to positive items (pBayes (positive < negative) < .001, BF10 > 1000, see ). For negative posters, evidence for a difference in the evidential requirements of response selection was not observed.
Discussion
Extending prior research, here we explored the effects of stimulus valence on self-learning (Liao et al., Citation2021; Lockwood et al., Citation2018; Golubickis & Macrae, Citation2022). Using a PST, over the course of numerous choice selections, participants were required to learn which positive or negative posters were associated with self and a friend. To identify the processes underpinning learning, a RL-DDM analysis was conducted on the data (Fontanesi et al., Citation2019; Pedersen et al., Citation2017; Pedersen & Frank, Citation2020). In the context of self-learning, several interesting results were observed. First, learning was accelerated when negative posters were owned-by-self (vs. friend) but slowed when positive posters were self-relevant (vs. friend-relevant). Second, these effects were accompanied by associated differences in reward sensitivity during the PST. Whereas sensitivity to expected reward was low when self-relevant posters were negative in valence, sensitivity was high when the posters were positive. In other words, when learning which desirable posters were owned-by-self, previously rewarded stimuli were selected more often than novel (i.e. riskier) alternatives. In contrast, when undesirable posters were self-relevant, novel choice selections were chosen most frequently. Third, for desirable posters, the evidential requirements of choice selection were elevated for self-owned compared to friend-owned items, indicating greater response caution during the former trials (Golubickis & Macrae, Citation2022).
Dynamics of self-bias
Inspection of the existing literature confirms the complex character of self-bias (Golubickis & Macrae, Citation2023). Whether self-relevance enhances or impedes information processing – or indeed impacts task performance at all – is determined by multiple factors; including people’s goal states, how self-object associations are created and measured, and the demands of the immediate task context (e.g. Caughey et al., Citation2021; Constable et al., Citation2019; Falbén et al., Citation2019, Falbén, Golubickis, Tamulaitis, et al., Citation2020; Golubickis et al., Citation2017, Citation2020, Citation2021; Macrae et al., Citation2017, Citation2018; Siebold et al., Citation2015; Stein et al., Citation2016; Svensson et al., Citation2022; Wang et al., Citation2016; Woźniak & Knoblich, Citation2021). Rather than reflecting an immutable aspect of social cognition, self-prioritisation is inherently malleable. As demonstrated herein, the effects of self-relevance on probabilistic learning were similarly nuanced, with the valence of material impacting the rate at which knowledge was acquired, with corresponding differences in sensitivity to reward following choice selections during decision-making.
That stimulus valence has the capacity to moderate the rate of self-learning likely derives from the multifaceted character and demands of self-function. Crucial to effective social-cognitive functioning is the possession of a stable and positive self-concept (Baumeister, Citation1998; Greenwald, Citation1980; Markus, Citation1977). In this regard, selecting desirable options that formerly were correctly associated with the self (i.e. rewarding) is clearly a strategy that would enhance the positivity of the self-concept (Dayan & Balleine, Citation2002; Northoff & Hayes, Citation2011; Schultz, Citation1998; Sutton & Barto, Citation1998). Although adoption of this approach for undesirable choice selections should have a similar effect (i.e. these selections are also rewarding), the problem in such a situation is that connections are forged between the self and negative stimuli. That is, a rewarding response has unwelcome consequences for the status of the self-concept (Baumeister, Citation1998). Given their reticence to associate undesirable material with the self, participants instead favoured novel choice selections, a strategy that ultimately increased the rate of learning for negative (vs. positive) stimuli (Sutton & Barto, Citation1998). Thus, unlike arbitrary (e.g. Hiragana characters) and positive material that trigger the exploitation of choice selections (Golubickis & Macrae, Citation2022), negative stimuli generate a preference for the exploration of response options.
The intricacies of early learning dynamics, particularly in the context of rapid information assimilation associated with positive self-owned posters, warrants nuanced consideration. Initial observations, as depicted in , suggest a pronounced acceleration in learning rates during the early trials. This finding raises an intriguing hypothesis: the swift learning curve in these preliminary stages might not be entirely encapsulated within the traditional learning rate parameter. Instead, it may reflect the significant influence of other model components, such as the starting-point, variability parameters, or drift rate scaling. Such modulation could ostensibly lead to a perceived diminution in the learning rate, as a plateauing effect – a near-approach to a learning ceiling – is potentially reached early in the process. This conjecture implies a complex interplay between the initial preference-based choice (akin to exploitation) and the evolving learning rate, a relationship that is possibly reflected in the vscaling parameter. The implication of this scenario is that the observed differences in learning rates between conditions may be partly attributable to an early saturation of learning in specific scenarios. In light of these considerations, it becomes apparent that the theoretical interpretations and conclusions presented herein could be intrinsically linked to these underlying model dynamics.
Additionally, it is worth noting that differences in reward sensitivity (i.e. drift rate scaling) may derive from motivational factors that influence self-referential cognition when people are confronted with desirable and undesirable options (Mezulis et al., Citation2004; Sedikides & Alicke, Citation2012; Sedikides & Strube, Citation1997). For example, the desire to forge positive (vs. negative) object-related associations with self compared to friend may motivate the rapid integration of rewarding choice selections into the process of evidence accumulation during decision-making (Ziegler et al., Citation2016). In contrast, absent such influence, friend-learning is characterised by the incremental acquisition of expected values (in accordance with the delta learning rule – Pedersen et al., Citation2017) resulting in higher learning rates (Golubickis & Macrae, Citation2022). In other words, in combination with the valence of to-be-learned stimuli, motivational factors shape the decisional strategies through which the self-concept can be maintained and/or bolstered. In this way, given the higher motivational salience of self-relevant stimuli, motivational accounts may provide an alternative explanation for why reward sensitivity was high for positive and low for negative self-owned posters. When previous feedback had indicated an association between the self and negative posters, there would be higher motivation to explore alternative choices that, while risky, could provide higher reward than before. On the other hand, when self-associated posters were positive, reliance on previously reinforced associations would be higher. Although not the focus of the current investigation, the broader issue of how motivation impacts self-learning merits further scrutiny.
In tandem with motivational accounts of self-enhancement during decisional processing (Sedikides & Strube, Citation1997), associative models of ownership provide a complimentary theoretical framework within which the current findings can be located (Beggan, Citation1992; Gawronski et al., Citation2007; Greenwald et al., Citation2002; Ye & Gawronski, Citation2016). According to Balanced-Identity Theory, associations between objects (e.g. posters) and the self are constrained by basic principles of associative networks (Cvencek et al., Citation2012; Greenwald et al., Citation2002). First, the principle of balance-congruity asserts that if two nodes are linked to the same third node, the two nodes should have a shared property that facilitates their association. Second, the principle of imbalance-dissonance states that the associative network should resist forming a new association if that connection links bipolar-opposite nodes. In the context of ownership and learning, application of these principles informs understanding of how stimulus valence potentially influences task performance (Golubickis et al., Citation2021; Ye & Gawronski, Citation2016). Specifically, the associative network’s preference for self-object congruity increases reward sensitivity toward desirable – and decreases reward sensitivity toward undesirable – choice selections.
In recent years, evidence accumulation models of decision-making (e.g. DDM) have been an important tool in establishing process-based accounts of social-cognitive biases (e.g. Correll et al., Citation2015; Golubickis et al., Citation2018, Citation2020; Hackel & Amodio, Citation2018; Johnson et al., Citation2018; Persson et al., Citation2021; Tsamadi et al., Citation2020). Based on response time and accuracy data, these models estimate a set of latent parameters that represent associated cognitive processes, while controlling for basic decisional contaminants (e.g. speed-accuracy trade-off) that otherwise muddle the interpretation of the observed effects (Ratcliff et al., Citation2016). To date, the DDM has been the most widely adopted sequential sampling model to investigate how self-relevance impacts decisional processing (Falbén, Golubickis, Tamulaitis, et al., Citation2020, Falbén, Golubickis, Wischerath, et al., Citation2020; Golubickis et al., Citation2017, Citation2021; Macrae et al., Citation2017; Reuther & Chakravarthi, Citation2017; Yankouskaya et al., Citation2020). Based on the application of this approach, prior research has established that, depending on the characteristics of the task context, personal relevance selectively influences the stimulus- and/or response-driven operations through which self-bias arises (e.g. Falbén, Golubickis, Tamulaitis, et al., Citation2020, Falbén, Golubickis, Wischerath, et al., Citation2020; Golubickis et al., Citation2018, Citation2020; Macrae et al., Citation2017). Interestingly, recent computational developments that combine decision-making with aspects of learning or attention have also successfully elucidated the dynamics of self-bias (Golubickis & Macrae, Citation2021, Citation2022; Svensson et al., Citation2022). Adopting just such an approach, here we further demonstrated the utility of computational approaches in the investigation of core social-cognitive topics (Hackel & Amodio, Citation2018).
Limitations and future research
Given the malleability of self-bias, it is unlikely that the effects observed here extend to the processing of evaluatively tinged material for all types of people (Golubickis & Macrae, Citation2022). Driving the interpretation of the current findings was the assumption that, through association with desirable material, individuals strive to maintain a positive conception of the self (Baumeister, Citation1998; Greenwald, Citation1980; Markus, Citation1977; Taylor & Brown, Citation1988). Although self-positivity has been demonstrated across cultures (Sedikides et al., Citation2015), there are nevertheless persons for whom self-construal takes a quite different course. For example, depression, low self-esteem, and possession of a negative self-concept all trigger what could be termed the dark side of self-referential processing, whereby individuals display enhanced sensitivity to undesirable (vs. desirable) stimuli (e.g. Krompinger & Simons, Citation2009; Kuiper & Derry, Citation1982; McCabe & Gotlib, Citation1995; Segal et al., Citation1995). Whether through chronic negative appraisals of the self, or the effects of experimental manipulations that temporarily impair feelings of self-worth (e.g. priming, memory retrieval, rejection), of interest would be to explore how differences in self-construal influence probabilistic learning. For example, when learning which valence-related stimuli are associated with self and a friend, do individuals with a negative self-concept exploit display enhanced reward sensitivity toward previously reinforced undesirable choice selections?
Also of potential importance are well-documented changes in the character of self-referential and emotional processing across the lifespan. Generally speaking, compared to their younger peers, older individuals display attentional and memorial biases toward material that is personally relevant and/or emotionally charged, with the latter effects most prominent for positive emotions (Carstensen & Mikels, Citation2005; Carstensen & Turk-Charles, Citation1994; Mather & Carstensen, Citation2005). These biases have obvious implications for how stimulus valence may influence instrumental learning. If, as has been noted, aging increases the prevalence and intensity of positivity biases, then elderly (vs. younger) adults should exhibit a higher sensitivity to reward following desirable (vs. undesirable) choice selections that previously were correctly associated with the self (Frank et al., Citation2004, Citation2007). That is, for positively-valenced material, immediate decisional rewards may be especially appealing for older adults. As the current investigation considered only younger individuals, this matter merits empirical attention as work of the kind will inform understanding of the characteristics of self-learning as a function of aging.
To extend the scope of the current investigation, research should also explore the neural mechanisms that support the learning of material relating to the self and others (Golubickis & Macrae, Citation2022; Lockwood et al., Citation2018), particularly evaluatively laden stimuli. Given the pivotal contribution of the pre-frontal cortex during self-referential processing (Kelley et al., Citation2002; Mitchell et al., Citation2002, Citation2006; Sui et al., Citation2013), it is interesting to note that activity in this region is also associated with the exploration-exploitation dilemma and sensitivity to reward during decision-making (Blanchard & Gershman, Citation2018; Domenech et al., Citation2020). Whereas activity in the ventromedial PFC (vmPFC) indexes the subjective value of outcomes given the response-related strategy that is currently in place, activity in dorsomedial PFC (dmPFC) tracks reductions in these values and the implementation of new action plans (Donoso et al., Citation2014). How these patterns of activity are modulated by both the target (i.e. self vs. friend) and stimuli (i.e. positive vs. negative) under consideration warrant empirical scrutiny. Likewise, attention should be directed to the combined effects of self-relevance and valence on reward-related processing (e.g. ventral striatum [VS], dorsal anterior cingulate cortex [dACC] during instrumental learning) (Gershman & Daw, Citation2017; Schultz, Citation1998). In particular, how rewarding are choice selections for correctly identified valence-related stimuli (i.e. positive vs. negative) pertaining to the self and others? In conjunction with computational modelling (Fontanesi et al., Citation2019; Pedersen & Frank, Citation2020), work of this kind will advance understanding of the pathways through which self-relevance and valence shape social cognition.
Using a PST in combination with a RL-DDM analysis, here we considered how self-relevance and valence mutually influence instrumental learning. The results revealed faster learning for negative and slower learning for positive stimuli, effects that were associated with differences in reward sensitivity during decisional processing. Additionally, for positive stimuli, self-relevant (vs. friend-relevant) responses were characterised by greater caution. Extending previous work, these findings underscore the benefits of computational approaches when exploring the dynamics of self-learning (Golubickis & Macrae, Citation2022; Lockwood et al., Citation2018).
Supplemental Material.docx
Download MS Word (1.7 MB)Disclosure statement
No potential conflict of interest was reported by the author(s).
Data availability statement
The data and stimulus materials are available at https://osf.io/5bswv/.
References
- Alexopoulos, T., Muller, D., Ric, F., & Marendaz, C. (2012). I, me, mine: Automatic attentional capture by self-related stimuli. European Journal of Social Psychology, 42(6), 770–779. https://doi.org/10.1002/ejsp.1882
- Baars, B. J. (1988). A cognitive theory of consciousness. Cambridge University Press.
- Bargh, J. A., & Pratto, F. (1986). Individual construct accessibility and perceptual selection. Journal of Experimental Social Psychology, 22(4), 293–311. https://doi.org/10.1016/0022-1031(86)90016-8
- Baumeister, R. F. (1998). The self. In D. T. Gilbert, S. T. Fiske, & G. Lindzey (Eds.), Handbook of social psychology (4th ed., pp. 680–740). McGraw-Hill.
- Beggan, J. K. (1992). On the social nature of nonsocial perception: The mere ownership effect. Journal of Personality and Social Psychology, 62(2), 229–237. https://doi.org/10.1037/0022-3514.62.2.229
- Blanchard, T. C., & Gershman, S. J. (2018). Pure correlates of exploration and exploitation in the human brain. Cognitive, Affective, & Behavioral Neuroscience, 18(1), 117–126. https://doi.org/10.3758/s13415-017-0556-2
- Carstensen, L. L., & Mikels, J. A. (2005). At the intersection of emotion and cognition: Aging and the positivity effect. Current Directions in Psychological Science, 14(3), 117–121. https://doi.org/10.1111/j.0963-7214.2005.00348.x
- Carstensen, L. L., & Turk-Charles, S. (1994). The salience of emotion across the adult life span. Psychology and Aging, 9(2), 259–264. https://doi.org/10.1037/0882-7974.9.2.259
- Caughey, S., Falbén, J. K., Tsamadi, D., Persson, L. M., Golubickis, M., & Macrae, C. N. (2021). Self-prioritization during stimulus processing is not obligatory. Psychological Research, 85(2), 503–508. https://doi.org/10.1007/s00426-019-01283-2
- Cohen, J. D., McClure, S. M., & Yu, A. J. (2007). Should I stay or should I go? How the human brain manages the trade-off between exploitation and exploration. Philosophical Transactions of the Royal Society B: Biological Sciences, 362(1481), 933–942. https://doi.org/10.1098/rstb.2007.2098
- Constable, M. D., Becker, M. L., Oh, Y.-I., & Knoblich, G. (2021). Affective compatability with the self modulates the self-prioritization effect. Cognition and Emotion, 35(2), 291–304. https://doi.org/10.1080/02699931.2020.1839383
- Constable, M. D., Welsh, T. N., Huffman, G., & Pratt, J. (2019). I before U: Temporal order judgements reveal bias for self-owned objects. Quarterly Journal of Experimental Psychology, 72(3), 589–598. https://doi.org/10.1177/1747021818762010
- Conway, M. A. (2005). Memory and the self. Journal of Memory and Language, 53(4), 594–628. https://doi.org/10.1016/j.jml.2005.08.005
- Conway, M. A., & Pleydell-Pearce, C. W. (2000). The construction of autobiographical memories in the self-memory system. Psychological Review, 107(2), 261–288. https://doi.org/10.1037/0033-295X.107.2.261
- Correll, J., Wittenbrink, B., Crawford, M. T., & Sadler, M. S. (2015). Stereotypic vision: How stereotypes disambiguate visual stimuli. Journal of Personality and Social Psychology, 108(2), 219–233. https://doi.org/10.1037/pspa0000015
- Cvencek, D., Greenwald, A. G., & Meltzoff, A. N. (2012). Balanced identity theory: Evidence for implicit consistency in social cognition. In B. Gawronski & F. Strack (Eds.), Cognitive consistency: A unifying concept in social psychology (pp. 157–177). Guilford Press.
- Daw, N. D., O’Doherty, J. P., Dayan, P., Seymour, B., & Dolan, R. J. (2006). Cortical substrates for exploratory decisions in humans. Nature, 441(7095), 876–879. https://doi.org/10.1038/nature04766
- Dayan, P., & Balleine, B. W. (2002). Reward, motivation, and reinforcement learning. Neuron, 36(2), 285–298. https://doi.org/10.1016/S0896-6273(02)00963-7
- Domenech, P., Rheims, S., & Koechlin, E. (2020). Neural mechanisms resolving exploitation-exploration dilemmas in the medial prefrontal cortex. Science, 369(6507), eabb0184. https://doi.org/10.1126/science.abb0184
- Donoso, M., Collins, A. G., & Koechlin, E. (2014). Foundations of human reasoning in the prefrontal cortex. Science, 344(6191), 1481–1486. https://doi.org/10.1126/science.1252254
- Falbén, J. K., Golubickis, M., Balseryte, R., Persson, L. M., Tsamadi, D., Caughey, S., & Macrae, C. N. (2019). How prioritized is self-prioritization during stimulus processing? Visual Cognition, 27(1), 46–51. https://doi.org/10.1080/13506285.2019.1583708
- Falbén, J. K., Golubickis, M., Tamulaitis, S., Caughey, S., Tsamadi, D., Persson, D., Svensson, L. M., Sahraie, S. L., & Macrae, C. N. (2020). Self-relevance enhances evidence gathering during decision-making. Acta Psychologica, 209, 103122. https://doi.org/10.1016/j.actpsy.2020.103122
- Falbén, J. K., Golubickis, M., Wischerath, D., Tsamadi, D., Persson, L. M., Caughey, S., Svensson, S., & Macrae, C. N. (2020). It’s not always about me: The effects of prior beliefs and stimulus prevalence on self-other prioritization. Quarterly Journal of Experimental Psychology, 73(9), 1466–1480. https://doi.org/10.1177/1747021820913016
- Fontanesi, L., Gluth, S., Spektor, M. S., & Rieskamp, J. (2019). A reinforcement learning diffusion decision model for value-based decisions. Psychonomic Bulletin & Review, 26(4), 1099–1121. https://doi.org/10.3758/s13423-018-1554-2
- Frank, M. J., Moustafa, A. A., Haughey, H. M., Curran, T., & Hutchinson, K. E. (2007). Genetic triple dissociation reveals multiple roles for dopamine in reinforcement learning. Proceedings of the National Academy of Sciences, 104(41), 16311–16316. https://doi.org/10.1073/pnas.0706111104
- Frank, M. J., Seeberger, L. C., & O’Reilly, R. C. (2004). By carrot or by stick: Cognitive reinforcement learning in Parkinsonism. Science, 306(5703), 1940–1943. https://doi.org/10.1126/science.1102941
- Gallagher, S. (2000). Philosophical conceptions of the self: Implications for cognitive science. Trends in Cognitive Sciences, 4(1), 14–21. https://doi.org/10.1016/S1364-6613(99)01417-5
- Gawronski, B., Bodenhausen, G. V., & Becker, A. P. (2007). I like it, because I like myself: Associative self-anchoring and post-decisional change of implicit evaluations. Journal of Experimental Social Psychology, 43(2), 221–232. https://doi.org/10.1016/j.jesp.2006.04.001
- Gelman, A., & Rubin, D. B. (1992). Inference from iterative simulation using multiple sequences. Statistical Science, 7, 457–472.
- Gershman, S. J., & Daw, N. D. (2017). Reinforcement learning and episodic memory in humans and animals: An integrative framework. Annual Review of Psychology, 68(1), 101–128. https://doi.org/10.1146/annurev-psych-122414-033625
- Golubickis, M., Falbén, J. K., Cunningham, W. A., & Macrae, C. N. (2018). Exploring the self-ownership effect: Separating stimulus and response biases. Journal of Experimental Psychology: Learning, Memory, and Cognition, 44(2), 295–306. https://doi.org/10.1037/xlm0000455
- Golubickis, M., Falbén, J. K., Ho, N. S., Sui, J., Cunningham, W. A., & Macrae, C. N. (2020). Parts of me: Identity-relevance moderates self-prioritization. Consciousness and Cognition, 77, 102848. https://doi.org/10.1016/j.concog.2019.102848
- Golubickis, M., Falbén, J. K., Sahraie, A., Visokomogilski, A., Cunningham, W. A., Sui, J., & Macrae, C. N. (2017). Self-prioritization and perceptual matching: The effects of temporal construal. Memory & Cognition, 45(7), 1223–1239. https://doi.org/10.3758/s13421-017-0722-3
- Golubickis, M., Ho, N. S. P., Falbén, J. K., Schwertel, C. L., Maiuri, A., Dublas, D., Cunningham, W. A., & Macrae, C. N. (2021). Valence and ownership: Object desirability influences self-prioritization. Psychological Research, 85(1), 91–100. https://doi.org/10.1007/s00426-019-01235-w
- Golubickis, M., & Macrae, C. N. (2021). That’s me in the spotlight: Self-relevance modulates attentional breadth. Psychonomic Bulletin & Review, 28(6), 1915–1922. https://doi.org/10.3758/s13423-021-01964-3
- Golubickis, M., & Macrae, C. N. (2022). Sticky me: Self-relevance slows reinforcement learning. Cognition, 227, 105207. https://doi.org/10.1016/j.cognition.2022.105207
- Golubickis, M., & Macrae, C. N. (2023). Self-prioritization reconsidered: Scrutinizing three claims. Perspectives on Psychological Science, 18(4), 876–886. https://doi.org/10.1177/17456916221131273
- Gray, H. M., Ambady, N., Lowenthal, W. T., & Deldin, P. (2004). P300 as an index of attention to self-relevant stimuli. Journal of Experimental Social Psychology, 40(2), 216–224. https://doi.org/10.1016/S0022-1031(03)00092-1
- Greenwald, A. G. (1980). The totalitarian ego: Fabrication and revision of personal identity. American Psychologist, 35(7), 603–618. https://doi.org/10.1037/0003-066X.35.7.603
- Greenwald, A. G., Banaji, M. R., Dudman, L. A., Farnham, S. D., Nosek, B. A., & Mellot, D. S. (2002). A unified theory of implicit attitudes, stereotypes, self-esteem, and self-concept. Psychological Review, 109(1), 3–25. https://doi.org/10.1037/0033-295X.109.1.3
- Gutchess, A., & Kensinger, E. A. (2018). Shared mechanisms may support mnemonic benefits from self-referencing and emotion. Trends in Cognitive Sciences, 22(8), 712–724. https://doi.org/10.1016/j.tics.2018.05.001
- Hackel, L. M., & Amodio, D. M. (2018). Computational neuroscience approaches to social cognition. Current Opinion in Psychology, 24, 92–97. https://doi.org/10.1016/j.copsyc.2018.09.001
- Humphreys, G. W., & Sui, J. (2016). Attentional control and the self: The self-attention network (SAN). Cognitive Neuroscience, 7(1-4), 5–17. https://doi.org/10.1080/17588928.2015.1044427
- James, W. (1890). The principles of psychology. Henry-Holt & Co.
- Johnson, D. J., Cesario, J., & Pleskac, T. J. (2018). How prior information and police experience impact decisions to shoot. Journal of Personality and Social Psychology, 115(4), 601–623. https://doi.org/10.1037/pspa0000130
- Kelley, W. M., Macrae, C. N., Wyland, C. L., Caglar, S., Inati, S., & Heatherton, T. F. (2002). Finding the self? An event-related fMRI study. Journal of Cognitive Neuroscience, 14(5), 785–794. https://doi.org/10.1162/08989290260138672
- Krompinger, J. W., & Simons, R. F. (2009). Electrophysiological indicators of emotional processing biases in depressed undergraduates. Biological Psychology, 81(3), 153–163. https://doi.org/10.1016/j.biopsycho.2009.03.007
- Kuiper, N. A., & Derry, P. A. (1982). Depressed and nondepressed content self-references in mild depressives. Journal of Personality, 50, 67–80. https://doi.org/10.1111/j.1467-6494.1982.tb00746.x
- Kuiper, N. A., & Rogers, T. B. (1979). Encoding of personal information: Self-other differences. Journal of Personality and Social Psychology, 37(4), 499–514. https://doi.org/10.1037/0022-3514.37.4.499
- Kurdi, B., Lozano, S., & Banaji, M. R. (2017). Introducing the open affective standardized image set (OASIS). Behavior Research Methods, 49(2), 457–470. https://doi.org/10.3758/s13428-016-0715-3
- Liao, Z., Huang, L., & Luo, S. (2021). Intranasal oxytocin decreases self-oriented learning. Psychopharmacology, 238(2), 461–474. https://doi.org/10.1007/s00213-020-05694-7
- Lockwood, P. L., Wittmann, M. K., Apps, M. A., Klein-Flügge, M. C., Crockett, M. J., Humphreys, G. W., & Rushworth, M. F. (2018). Neural mechanisms for learning self and other ownership. Nature Communications, 9(1), 1–11. https://doi.org/10.1038/s41467-018-07231-9
- Macrae, C. N., Visokomogilski, A., Golubickis, M., Cunningham, W. A., & Sahraie, A. (2017). Self-relevance prioritizes access to visual awareness. Journal of Experimental Psychology: Human Perception and Performance, 43(3), 438–443. https://doi.org/10.1037/xhp0000361
- Macrae, C. N., Visokomogilski, A., Golubickis, M., & Sahraie, A. (2018). Self-relevance enhances the benefits of attention on perception. Visual Cognition, 26(7), 475–481. https://doi.org/10.1080/13506285.2018.1498421
- Markus, H. (1977). Self-schemata and processing information about the self. Journal of Personality and Social Psychology, 35(2), 63–78. https://doi.org/10.1037/0022-3514.35.2.63
- Markus, H., & Wurf, E. (1987). The dynamic self-concept: A social psychological perspective. Annual Review of Psychology, 38(1), 299–337. https://doi.org/10.1146/annurev.ps.38.020187.001503
- Markus, H. R., & Nurius, P. (1986). Possible selves. American Psychologist, 41(9), 954–969. https://doi.org/10.1037/0003-066X.41.9.954
- Mather, M., & Carstensen, L. L. (2005). Aging and motivated cognition: The positivity effect in attention and memory. Trends in Cognitive Sciences, 9, 469–502.
- McCabe, S. B., & Gotlib, I. H. (1995). Selective attention and clinical depression: Performance on a deployment-of-attention task. Journal of Abnormal Psychology, 104(1), 241–245. https://doi.org/10.1037/0021-843X.104.1.241
- Mezulis, A. H., Abramson, L. Y., Hyde, J. S., & Hankin, B. L. (2004). Is there a universal positivity bias in attributions? A meta-analytic review of individual, developmental, and cultural differences in the self-serving attributional bias. Psychological Bulletin, 130(5), 711–747. https://doi.org/10.1037/0033-2909.130.5.711
- Miletić, S., Boag, R. J., & Forstmann, B. U. (2020). Mutual benefits: Combining reinforcement learning with sequential sampling models. Neuropsychologia, 136, 107261. https://doi.org/10.1016/j.neuropsychologia.2019.107261
- Mitchell, J. P., Heatherton, T. F., & Macrae, C. N. (2002). Distinct neural systems subserve person and object knowledge. Proceedings of the National Academy of Sciences, 99(23), 15238–15243. https://doi.org/10.1073/pnas.232395699
- Mitchell, J. P., Macrae, C. N., & Banaji, M. R. (2006). Dissociable medial prefrontal contributions to judgments of similar and dissimilar others. Neuron, 50(4), 655–663. https://doi.org/10.1016/j.neuron.2006.03.040
- Northoff, G., & Hayes, D. J. (2011). Is our self nothing but reward? Biological Psychiatry, 69(11), 1019–1025. https://doi.org/10.1016/j.biopsych.2010.12.014
- Oakley, D. A., & Halligan, P. W. (2017). Chasing the rainbow: The non-conscious nature of being. Frontiers in Psychology, 8, 1924. https://doi.org/10.3389/fpsyg.2017.01924
- Oppenheimer, D. M., Meyvis, T., & Davidenko, N. (2009). Instructional manipulation checks: Detecting satisficing to increase statistical power. Journal of Experimental Social Psychology, 45(4), 867–872. https://doi.org/10.1016/j.jesp.2009.03.009
- Pedersen, M. L., & Frank, M. J. (2020). Simultaneous hierarchical Bayesian parameter estimation for reinforcement learning and drift diffusion models: A tutorial and links to neural data. Computational Brain & Behavior, 3(4), 458–471. https://doi.org/10.1007/s42113-020-00084-w
- Pedersen, M. L., Frank, M. J., & Biele, G. (2017). The drift diffusion model as the choice rule in reinforcement learning. Psychonomic Bulletin & Review, 24(4), 1234–1251. https://doi.org/10.3758/s13423-016-1199-y
- Persson, L., Golubickis, M., Dublas, D., Mastnak, N., Falbén, J. K., Tsamadi, D., Caughey, S., Svensson, S., & Macrae, C. N. (2021). Comparing person and people perception: Multiple group members do not increase stereotype priming. Quarterly Journal of Experimental Psychology, 74(8), 1418–1431. https://doi.org/10.1177/17470218211012852
- Ratcliff, R., Smith, P. L., Brown, S. D., & McKoon, G. (2016). Diffusion decision model: Current issues and history. Trends in Cognitive Sciences, 20(4), 260–281. https://doi.org/10.1016/j.tics.2016.01.007
- Ratcliff, R., & Tuerlinckx, F. (2002). Estimating parameters of the diffusion model: Approaches dealing with contaminant reaction times and parameter variability. Psychonomic Bulletin & Review, 9(3), 438–481. https://doi.org/10.3758/BF03196302
- Rescorla, R. A., & Wagner, A. R. (1972). A theory of pavlovian conditioning: Variations in the effectiveness of reinforcement and nonreinforcement. In A. H. Black & W. F. Prokasy (Eds.), Classical conditioning II: Current research and theory (pp. 64–99). Appleton- Century-Crofts.
- Reuther, J., & Chakravarthi, R. (2017). Does self-prioritization affect perceptual processes? Visual Cognition, 25(1–3), 381–398. https://doi.org/10.1080/13506285.2017.1323813
- Schäfer, S., Frings, C., & Wentura, D. (2016). About the composition of self-relevance: Conjunctions not features are bound to the self. Psychonomic Bulletin & Review, 23(3), 887–892. https://doi.org/10.3758/s13423-015-0953-x
- Schäfer, S., Wentura, D., & Frings, C. (2015). Self-prioritization beyond perception. Experimental Psychology, 62(6), 415–425. https://doi.org/10.1027/1618-3169/a000307
- Schultz, W. (1998). Predictive reward signal of dopamine neurons. Journal of Neurophysiology, 80(1), 1–27. https://doi.org/10.1152/jn.1998.80.1.1
- Sedikides, C., & Alicke, M. D. (2012). Self-enhancement and self-protection motives. In R. M. Ryan (Ed.), The Oxford handbook of human motivation (pp. 303–322). Oxford University Press.
- Sedikides, C., Gaertner, L., & Cai, H. (2015). On the panculturality of self-enhancement and self-protection motivation: The case for the universality of self-esteem. Advances in Motivation Science, 2, 184–241.
- Sedikides, C., & Strube, M. J. (1997). Self-evaluation: To thine own self be good, to thine own self be sure, to thine own self be true, and to thine own self be better. Advances in Experimental Social Psychology, 29, 209–269. https://doi.org/10.1016/S0065-2601(08)60018-0
- Segal, Z. V., Gemar, M., Truchon, C., Guirguis, M., & Horowitz, L. M. (1995). A priming methodology for studying self-representation in major depressive disorder. Journal of Abnormal Psychology, 104(1), 205–213. https://doi.org/10.1037/0021-843X.104.1.205
- Shapiro, K. L., Caldwell, J., & Sorensen, R. E. (1997). Personal names and the attentional blink: A visual “cocktail party” effect. Journal of Experimental Psychology: Human Perception and Performance, 23(2), 504–514. https://doi.org/10.1037/0096-1523.23.2.504
- Siebold, A., Weaver, M. D., Donk, M., & van Zoest, W. (2015). Social salience does not transfer to oculomotor visual search. Visual Cognition, 23(8), 989–1019. https://doi.org/10.1080/13506285.2015.1121946
- Spiegelhalter, D. J., Best, N. G., Carlin, B. P., & van der Linde, A. (1998). Bayesian deviance, the effective number of parameters, and the comparison of arbitrarily complex models. Research Report, 98-1009.
- Spiegelhalter, D. J., Best, N. G., Carlin, B. P., & van der Linde, A. (2002). Bayesian measures of model complexity and fit. Journal of the Royal Statistical Society: Series B, 64, 583–639. https://doi.org/10.1111/1467-9868.00353
- Stein, T., Siebold, A., & van Zoest, W. (2016). Testing the idea of privileged awareness of self-relevant information. Journal of Experimental Psychology: Human Perception and Performance, 42(3), 303–307. https://doi.org/10.1037/xhp0000197
- Sui, J., He, X., & Humphreys, G. W. (2012). Perceptual effects of social salience: Evidence from self-prioritization effects on perceptual matching. Journal of Experimental Psychology: Human Perception and Performance, 38(5), 1105–1117. https://doi.org/10.1037/a0029792
- Sui, J., & Humphreys, G. W. (2015). The integrative self: How self-reference integrates perception and memory. Trends in Cognitive Sciences, 19(12), 719–728. https://doi.org/10.1016/j.tics.2015.08.015
- Sui, J., & Humphreys, G. W. (2017). The ubiquitous self: What the properties of self-bias tell us about the self. Annals of the New York Academy of Sciences, 1396(1), 222–235. https://doi.org/10.1111/nyas.13197
- Sui, J., & Rotshtein, P. (2019). Self-prioritization and the attentional systems. Current Opinion in Psychology, 29, 148–152. https://doi.org/10.1016/j.copsyc.2019.02.010
- Sui, J., Rotshtein, P., & Humphreys, G. W. (2013). Coupling social attention to the self forms a network for personal significance. Proceedings of the National Academy of Sciences, 110(19), 7607–7612. https://doi.org/10.1073/pnas.1221862110
- Sutton, R. S., & Barto, A. G. (1998). Reinforcement learning: An introduction (Vol. 135). MIT Press.
- Svensson, S. L., Golubickis, M., Maclean, H., Falbén, J. K., Persson, L. M., Tsamadi, D., Caughey, S., Sahraie, A., & Neil Macrae, C. (2022). More or less of me and you: Self-relevance augments the effects of item probability on stimulus prioritization. Psychological Research, 86(4), 1145–1164. https://doi.org/10.1007/s00426-021-01562-x
- Symons, C. S., & Johnson, B. T. (1997). The self-reference effect in memory: A meta-analysis. Psychological Bulletin, 121(3), 371–394. https://doi.org/10.1037/0033-2909.121.3.371
- Taylor, S. E., & Brown, J. D. (1988). Illusion and well-being: A social psychological perspective on mental health. Psychological Bulletin, 103(2), 193–210. https://doi.org/10.1037/0033-2909.103.2.193
- Tsamadi, D., Falbén, J. K., Persson, L. M., Golubickis, M., Caughey, S., Sahin, B., & macrae, C. N. (2020). Stereotype-based priming without stereotype activation: A tale of two priming tasks. Quarterly Journal of Experimental Psychology, 73(11), 1939–1948. https://doi.org/10.1177/1747021820925396
- Unkelbach, C., Alves, H., & Koch, A. (2020). Negativity bias, positivity bias, and valence asymmetries: Explaining the differential processing of positive and negative information. Advances in Experimental Social Psychology, 62, 115–187. https://doi.org/10.1016/bs.aesp.2020.04.005
- Vicovaro, M., Delmaso, M., & Bertamini, M. (2022). Towards the boundaries of self-prioritization: Associating the self with asymmetric shapes disrupts the self-prioritization effect. Journal of Experimental Psychology: Human Perception and Performance, 48(9), 972–986. https://doi.org/10.1037/xhp0001036
- Wang, H., Humphreys, G., & Sui, J. (2016). Expanding and retracting from the self: Gains and costs in switching self-associations. Journal of Experimental Psychology: Human Perception and Performance, 42(2), 247–256. https://doi.org/10.1037/xhp0000125
- Watkins, C., & Dayan, P. (1992). Q-learning. Machine Learning, 8, 279–292.
- Wiecki, T. V., Sofer, I., & Frank, M. J. (2013). HDDM: Hierarchical Bayesian estimation of the drift-diffusion model in Python. Frontiers in Neuroinformatics, 7, 1–10. https://doi.org/10.3389/fninf.2013.00014
- Woźniak, M., & Knoblich, G. (2021). Self-prioritization depends on assumed task-relevance of self-association. Psychological Research, 86(5), 1599–1614. https://doi.org/10.1007/s00426-021-01584-5
- Woźniak, M., & Knoblich, G. (2019). Self-prioritization of fully unfamiliar stimuli. Quarterly Journal of Experimental Psychology, 72(8), 2110–2120. https://doi.org/10.1177/1747021819832981
- Yankouskaya, A., Bührle, R., Lugt, E., Stolte, M., & Sui, J. (2020). Intertwining personal and reward relevance: Evidence from the drift-diffusion model. Psychological Research, 84(1), 32–50. https://doi.org/10.1007/s00426-018-0979-6
- Yankouskaya, A., & Sui, J. (2022). Self-prioritization is supported by interactions between large- scale brain networks. European Journal of Neuroscience, 55(5), 1244–1261. https://doi.org/10.1111/ejn.15612
- Ye, Y., & Gawronski, B. (2016). When possessions become part of the self: Ownership and implicit self-object liking. Journal of Experimental Social Psychology, 64, 72–87. https://doi.org/10.1016/j.jesp.2016.01.012
- Yin, S., Sui, J., Chiu, Y.-C., Chen, A., & Egner, T. (2019). Automatic prioritization of self-referential stimuli in working memory. Psychological Science, 30(3), 415–423. https://doi.org/10.1177/0956797618818483
- Ziegler, S., Pedersen, M. L., Mowinckel, A. M., & Biele, G. (2016). Modelling ADHD: A review of ADHD theories through their predictions for computational models of decision-making and reinforcement learning. Neuroscience & Biobehavioral Reviews, 71, 633–656. https://doi.org/10.1016/j.neubiorev.2016.09.002