Abstract
Waterborne pathogens in urban rivers of estuarine areas pose a great threat to human health. In this study, both bacterial and fungal community structures of four urban rivers in the Pearl River estuarine area were investigated using high-throughput sequencing. Results showed that the diversity and richness indices of the bacterial community were higher than that of fungi. Taxonomic analysis indicated that the most abundant bacterial phylum was Actinobacteria, while the most abundant fungal phylum was Ascomycota. In addition, 35 major pathogens were found at the level of genus with Mycobacterium being the most abundant. Specifically, pathogens of Burkholderiales, Bartonella, Rhizobiales, Bordetella and Brucella were first detected in this area, although with relative low abundances. Spearman correlation analysis revealed that temperature, TOC and TDP were significant factors shaping both total microbial community and pathogenic microbial community structure. TDP was positively correlated with almost all the potential pathogenic genera. PICRUSt analysis further predicted that bacterial infectious disease was the most abundant pathway associated with microbial functions of human disease in all the studied rivers. This work provides useful information not only for in-depth understanding of bacterial and fungal communities in urban rivers of estuarine areas under anthropogenic disturbances but also for environmental risk management of potential waterborne pathogens.
Introduction
Estuarine ecosystems, connecting the terrestrial and coastal areas, play a crucial role in biogeochemical cycling and global energy flows. Rivers, lakes, reservoirs, ponds, sewers and sewage treatment systems are important habitats for microorganism populations, which participate in biogeochemical processes including the nitrogen, carbon, sulfur and phosphorus cycles, as well as the degradation of organic pollutants. However, the increasing pace and extent of urbanization alters the physicochemical parameters of surface waters, which leads to changes in the microbial community. Thus, microbial analysis has become an essential procedure for evaluating water quality and its impact on human health.
Although it has been reported that the microbial communities in freshwater are composed of bacteria belonging to Proteobacteria (mostly Betaproteobacteria), Actinobacteria and Cyanobacteria (Sun et al. Citation2014; Mohiuddin et al. Citation2019; Xue et al. Citation2020), the community structure is sensitively affected by the water environment, especially in areas with intensive human activities. For example, antibiotic pollution from human activities has greatly changed the microbial community structure of the Urumqi River, resulting in the increase of unknown bacteria taxa (Xue et al. Citation2020). Ammonia nitrogen and ORP (oxidation-reduction potential) were identified as the main factors that determine bacterial community composition in a heavily polluted urban river (Zhang et al. Citation2019). Total nitrogen (TN), pH, dissolved oxygen (DO), and heavy metals were the main drivers that affected the diversity of the bacterial community in Maozhou River under anthropogenic disturbances (Ouyang et al. Citation2020). On the other hand, principal health risks are associated with waterborne pathogenic microorganisms from anthropogenic discharges, including domestic sewage, industrial wastes, hospital effluents, and urban/agricultural runoffs (Cui et al. Citation2019; Wu et al. Citation2019; Al Salah et al. Citation2020; Zhang et al. Citation2021). For instance, the bacteria Vibrio cholerae found in domestic sewage can result in severe gastroenteritis, which causes dehydration and death (Moehling et al. Citation2020). Opportunistic fungal pathogens such as Penicillium, Paracoccidioides and Coccidioides from urban effluents are involved in respiratory tract infections (Yu et al. Citation2005; Gade et al. Citation2020; Singulani et al. Citation2020). The inputs of agricultural waste water with high phosphorus concentrations are responsible for the presence and abundance of both fungal and bacterial pathogens (Duarte et al. Citation2015; Wu et al. Citation2019). However, the pollution profile of pathogens and the driving environmental factors in typical urban rivers in estuarine areas have not been reported.
The Pearl River is the second largest (annual discharge of 3.30 × 1011 m3·yr−1) and the third longest (2.32 × 103 km) river in China (Mai et al. Citation2018). As the estuary of the Pearl River, Wanqingsha district has the most complex estuarine system located in the center of the "Golden Triangle" of Guangzhou, Hong Kong and Macao, which has become an economically developed and densely populated area in China. Recently, increased industrialization and urbanization have brought the discharge of industrial and sewage waste to the Pearl River estuary causing environmental pollution problems, such as severe eutrophication (Liu et al. Citation2020), pollution from heavy metals (Ye et al. Citation2012; Xie and Wang Citation2020), and organic pollutant pollution (Li et al. Citation2014) derived from riverine sewage and waste disposal (Mai et al. Citation2018). Additionally, many airborne opportunistic pathogenic bacteria and fungi were found and 21 ARG (antibiotic resistance gene) subtypes were detected in The Pearl River area according to our previous report (Liang et al. Citation2020). However, the waterborne pathogenic microbial community composition and its corresponding driving factors in this area have not been studied. It is difficult to directly detect the pathogens in water due to the wide variety of types and difficulties in cultivation. Microbial indicators, such as total coliforms and fecal coliforms are closely associated with pathogenic bacteria and, therefore, are widely used to assess the pollution profile of waterborne pathogens. However, owing to their nonpathogenic characteristics, these indicators are not sufficient to accurately evaluate the microbial safety. Therefore, direct examination of the pathogenic microbial community structure is essential to assess the threats to human health.
In this work, the microbial community structure of both fungi and bacteria in Wanqingsha urban rivers affected by human activities in the Pearl River estuarine area were analyzed using ITS and 16S rRNA high-throughput sequencing. Meanwhile, the characteristics of potential pathogens were also investigated in this area. The main objective was to clarify the key factors that shape the microbial and pathogen communities and to assess the microbial threats to human health. This work provides useful information for in-depth understanding of estuarine microbial communities for risk management of potential waterborne pathogens.
Methods
Water sampling
The sampling region was located on the main branch of Pearl River Estuary and a total of 11 samples were collected in August 2019 across Wanqingsha Island (22°35′17″–22°43′4″ N, 113°33′92″-113°37′52″ E) in the Nansha district, Guangzhou, China. The Wanqingsha Island has a total 19 human-made urban rivers that connect to the estuary (). Urban rivers 4, 8, 16 and 19 were selected as sampling sites. Urban river 4 was located in an industrial area which was influenced by industrial production activities. Urban river 8 was located in a residential area which was influenced by residential activities. Urban river 16 was an ecological area which had a small human population and thus was less affected by anthropogenic activities. Urban river 19 was considered as the inlet area at the mouth of the sea. As shown in , the water sampling sites were located in the industrial-influenced area (upper reach (R4U), middle reach (R4M), and lower reach of urban river 4 (R4D)), the residential-influenced area (upper reach (R8U), middle reach (R8M), and lower reach of urban river 8 (R8D)) , the ecological area (upper reach (R16U), middle reach (R16M), and lower reach of urban river 16 (R16D), and the estuary area (middle reach (R19M), and lower reach of urban river 19 (R19D)), respectively.
Figure 1. Map of sampling sites for different areas influenced from human activities in the urban rivers of Pearl River Estuary.
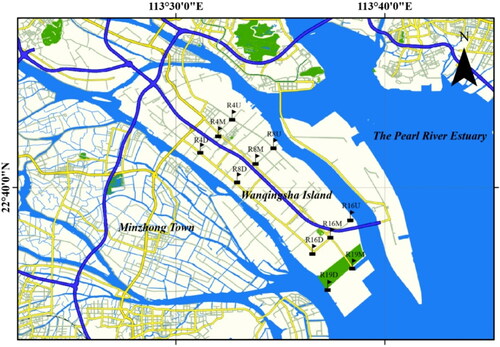
Surface water samples from approximately 0.5 m depth were collected using an ethanol-disinfected water sampler and stored in sterile plastic sampling bottles. The collected waters were immediately transported to the lab and filtered through 0.45 μm glass fiber filters. The filtered water samples were stored at 4 °C in darkness until water quality analysis was performed. Microorganisms were collected by filtering 150 mL of the collected water through a membrane filter (0.22 μm, Feiteng, China) and then stored at −80 °C until DNA extraction.
Water quality analysis
Water quality indicators including dissolved oxygen (DO), water temperature (T) and pH were immediately measured after sampling using a portable DO meter (ST300D/B, Ohaus, US), while chemical oxygen demand (COD), total dissolved phosphorus (TDP), nitrate (NO3-N) and nitrite (NO2-N) were analyzed in the laboratory according to standard methods (GB 5749-2006) (Wu et al. Citation2016). Total organic carbon (TOC) was measured using a total organic carbon analyzer (TOC-L CPH, Shimadzu, Japan). The UV254 absorbance of the samples was measured using a UV-vis spectrophotometer (Agilent, Cary 100, US) with a 1 cm quartz cuvette, and ultrapure water was used as both a reference and a blank.
EEM measurements and PARAFAC modeling
Organic matter profiles were investigated by three-dimension excitation emission matrix (3 D-EEM) fluorescence spectroscopy. The 3 D-EEM spectra of the samples with excitation (Ex) wavelengths of 240-400 nm and emission (Em) wavelengths of 290-550 nm were measured using a fluorescence spectrometer (FS5, Edinburgh, UK). The PARAFAC models were generated using the DOMFluor toolbox of the Matlab (2.0, Eigenvector Research Inc.) software package, which contains all of the tools used to identify outliers and can decompose the EEM spectrum into independent fluorescent components as well as perform residual errors and split-half diagnostics according to the tutorial described by Murphy et al. Citation2008. EEMs were corrected for instrument bias and inner filtering effects, a blank subtracted and normalized to the area under the water Raman peak of the blank at 350 nm.
DNA extraction, PCR amplification and sequencing
High-quality DNA was extracted from the filter membranes with MP FastDNA® SPIN Kit for Soil (MP Biomedicals, USA) according to the previous study (Liang et al. Citation2020). The details of DNA extraction and quality control procedures can be found in Supplemental Information. PCR amplification was conducted using the primers 338 F:5′-ACTCCTACGGGAGGCAGCAG-3′ and 806 R:5′-GGACTACHVGGGTWTCTAAT-3′ targeting V3-V4 hyper-variable region of the bacterial 16S rRNA gene, and ITS3F: 5′-GCATCGATGAAGAACGCAGC-3′ and ITS4R: 5′-TCCTCCGCTTATTGATATGC-3′ targeting the internal transcribed spacer (ITS) region of the fungi, respectively. PCR amplification was performed in triplicate for one sample at each site. PCR reactions were performed using 20 μL mixtures containing 5 μL of 5 × FastPuf Buffer, 2 μL of 2.5 mM dNTPs, 0.8 μL of each primer (5 μM), 0.4 μL of FastPuf polymerase, 0.2 μL of BSA, 10 ng of template DNA, and sterilized ultrapure water to bring volume up to 20 μL. PCR conditions were as follows: initial denaturation at 95 °C for 3 min; followed by 35 cycles of 95 °C for 30 s, annealing at 55 °C for 30 s, and extension at 72 °C for 45 seconds; and a final extension at 72 °C for 10 min. PCR products were extracted from 2% agarose gels and purified using the AxyPrep DNA Gel Extraction Kit (Axygen Biosciences, USA) according to the manufacturer’s instructions and quantified using QuantiFluorTM-ST (Promega, USA). All PCR products were sequenced using the Illumina MiSeq platform (Majorbio Bio-pharm Technology).
Data analysis
Data were processed using the Quantitative Insights Into Microbial Ecology (Qiime 1.9.1) pipeline for 16S rRNA and ITS data sets (http://qiime.org/tutorials/index.html). Low quality sequences were identified by denoising and filtered out of the dataset. Chimeric sequences were removed using the Uchime function with the sequence collection as its own database in the Mothur program 1.30.2 (https://www.mothur.org/wiki/Download_mothur) (Schloss et al. Citation2009). The effective sequences were then clustered into operational taxonomic units (OTU) at 97% sequence similarity with a standard Uparse method (http://www.drive5.com/uparse/). The taxonomy of bacteria, fungi and opportunistic pathogens were analyzed by RDP Classifier algorithm aligned and compared with the SILVA version 132 (https://www.arb-silva.de/), UNITE version 8.0 (https://unite.ut.ee/) and human pathogenic bacteria (HPB) database constructed by the taxonomic list derived from the HPB virulence factor database (http://www.mgc.ac.cn/VFs/) (Chen et al. Citation2012) and other references (Fang et al. Citation2015; Liang et al. Citation2020), respectively. The details of fungal community analyses can be found in Supplemental Information. Axis lengths of DCA1 of bacterial and fungal sequences were 0.70 and 0.74, respectively. Therefore, RDA using R-vegan was most suitable for comparing species-environment correlations. Spearman correlation between the physicochemical parameters and the relative abundance of bacterial communities was performed using R package (version 3.5.1). Phylogenetic Investigation of Communities by Reconstruction of Unobserved States (PICRUSt) (http://picrust.github.io/picrust/) was used to predict the bacterial community function. The prediction genes were compared with the Kyoto Encyclopedia of Genes and Genomes (KEGG) database.
Results and discussion
Water parameters and 3 D-EEM analysis
Table S1 lists the temporal physicochemical parameters of collected water samples, including Temperature, pH, TDP, DO, COD, TOC, NO3-N, NO2-N, and UV254. The water temperature was in the range of 28.4 °C ∼ 32.3 °C with an average of 30.8 °C, and pH ranged from 7.86 to 8.3 with an average of 8.07. The water temperature of studied rivers displayed a decreasing trend from upstream to downstream, and the pH value displayed a gradually increasing trend from river 4 to river 19. Notably, the concentrations of TDP in urban river 4 were higher than in the others with the highest value observed at site R4U (1.98 mg/L), which indicates the industrial-influenced river may be mainly contaminated by phosphorus-polluted effluents. Meanwhile, the highest COD (147.2 mg/L) and TOC (4.6 mg/L) concentrations were found at site R8U (residential area), suggesting organic polluted wastewater discharge was even worse in the residential area than in the industrial area. Irregular changes in DO (ranging from 3.70 mg/L to 7.08 mg/L), NO3-N (ranging from 7.06 mg/L to 45.9 mg/L) and NO2-N (ranging from 0.1 mg/L to 0.43 mg/L) were observed in all the sites, and the highest values were found at urban river 16, which was the area least affected by human activities.
To study the pollution profile, 3 D-EEM was used to characterize the features of organic pollutants. A total of 6 components were determined by PARAFAC modeling from the residuals and split-half analysis. As shown in , the spectral characteristics of these 6 components were matched with the OpenFluor database (similarity > 0.95) (Murphy et al. Citation2014). Specifically, Component 2 (C2) with the Ex/Em maximum at (250)335/450 nm could be assigned to typical humic-like substances according to the references (Lambert et al. Citation2016; Derrien et al. Citation2018). The components C1 (<240/475 nm) and C3 (<240/385 nm) were attributable to terrestrial humic-like substances (Murphy et al. Citation2011; Kowalczuk et al. Citation2013) and microbial humic-like substances (Yamashita et al. Citation2011; Retelletti Brogi et al. Citation2019), respectively. The components C4 (<240/360(450) nm) and C6 (275/325 nm) were indicators for the presence of tyrosine-like and tryptophan-like proteins and were presumably derived from plankton-derived organics or wastewater-impacted waters according to the references (Harjung et al. Citation2019; Wang et al. Citation2019). Overall, it was found that humic-like components C1, C2, C3, and C5 represented a total of 81 ± 3% (mean ± SD), while the two protein-like components (C4 and C6) accounted for 19 ± 3% of the six components, suggesting the dissolved organic matter is dominated by humic-like substances. Besides, the highest relative abundances of C1 were found at site R4U, which may be ascribed to the industrial production increasing the dissolved organic matter load.
3.2. The abundance and diversity of waterborne microorganisms
A total of 485,900 high quality 16S rRNA gene sequences and 635,875 ITS2 sequences were optimized for classification with dominant length distributions of 414 and 334 bp, respectively. After randomizing the sequences according to the minimum sample sequence number, 26,354 16S rRNA gene sequences and 28,771 ITS2 sequences of each sample were conducted for further statistical analyses.
Alpha diversity of the samples was investigated by analyzing Sobs, Shannon, Simpson, ACE, Chao, and coverage indices at the same sequence depth (). Overall, the Good’s coverage index of the bacteria and fungi was all above 99.0% and the rarefaction curves according to Sobs index on OTU level tended to approach the saturation plateaus (Figure S1), indicating that the obtained sequences could well represent the waterborne microbial communities in these samples. The Venn diagram showed that there were 766 bacterial OTUs shared by all sites, while R4 had the largest number of unique OTUs (195), followed by R8 (74 OTUs), R16 (26 OTUs) and R19 (23 OTUs) (Figure S2). The Shannon diversity and Chao1 richness index of the bacteria ranged from 4.38 to 5.20 and from 789.97 to 1344.81, respectively, and was higher than that of fungi which ranged from 2.82 to 4.85 and from 375.76 to 1090.67, respectively, suggesting the alpha diversity and richness of the bacterial community was higher than that of the fungal community. For different urban rivers, the Shannon diversity index suggests that R4D (industrial area) had the highest bacterial diversity, while R8D (residential area) had the highest fungal diversity. In addition, the Sobs number and Chao1 estimator of the bacteria and fungi in R4 was much higher than in the other three rivers (R8, R16, R19). This may due to the enrichment of phosphorus and terrestrial humic-like substances in the industrial area promoting the population growth of bacteria and fungi, while the enrichment of organic matter in the residential area favored the growth and reproduction of fungi.
Table 1. Richness and abundance of bacteria and fungi estimated by diversity index.
To study the similarity of the microbial community structures of the samples, redundancy analysis (RDA) was performed (). The sites influenced by human activity (R4, R8, R19) were well separated from upstream to downstream, indicating the structure of microbial communities was significantly changed under anthropogenic disturbances. The sites in the ecological area (R16) with less human activity were clustered together, suggesting that they had similar community structure composition. However, for fungi, the separation of sites R16U and R16M from the other sites shows a more obvious difference than the bacterial community. These results further demonstrate that the bacterial and fungal community structures are changed under the influence of human activities. The specific bacterial and fungal taxon differences are discussed in the next section.
3.3. Taxonomic assignment and community structure
The taxonomic distributions for 16S rRNA/ITS2 sequences of all the water samples are demonstrated in Figure S3. A total of 1,612 bacterial OTUs and 1,602 fungal OTUs were detected at a similarity threshold of 0.97, which can be classified into 35 phyla, 83 classes, 217 orders, 355 families, 587 genera, 929 species and 9 phyla, 32 classes, 67 orders, 116 families, 147 genera, and 172 species, respectively.
For bacterial sequences at the phylum level, the largest bacterial sequences (89,327 sequences) were assigned to Actinobacteria, and the second largest groups were Proteobacteria (75,794) and Cyanobacteria (68,944) followed by Bacteroidetes (29,805). Among them, the most OTUs belonged to Proteobacteria (41.27%), followed by Bacteroidetes (19.46%) and Cyanobacteria (10.14%), suggesting that there were more species of Proteobacteria. Based on relative abundances, the major bacterial groups (>1% abundance) were further studied and the results were displayed in . It was found that Actinobacteria, Proteobacteria, Cyanobacteria and Bacteroidetes were the most dominant groups in all the samples, which occupied more than 80% of all the species. However, the community structure obviously varied from upstream to downstream of the studied rivers, except for ecological area (R16). For instance, the relative abundance of Proteobacteria was increased from upstream to downstream in R4 and R8. The proportion of Cyanobacteria in downstream sites was lower than midstream/upstream in R4, R8 and R19, while it remained uniform in R16. In addition, the site R8U had the highest percentage of Actinobacteria (39.23%), the sample R4D had the highest percentage of Proteobacteria (38.8%), and the sample R8M contained the highest percentage of Cyanobacteria (34.68%).
Among the fungi that can be classified, the most predominant fungi were Ascomycota (134,282 sequences) which occupied 24.4% of all the fungal OTUs, while Chytridiomycota (9,317) occupied 4.11% and Basidiomycota (1,467) occupied 4.99% with relatively lower proportion. To be specific, shows that urban river 16 exhibited higher relative abundance of Ascomycota with the highest value obtained at R16M (70.36%). It was reduced obviously in the other rivers which have intense human activity, indicating that human activity may limit the growth of Ascomycota. In addition, the highest abundance of Chytridiomycota and Basidiomycota was found in sample R8D (occupied 9.20% and 2.05%, respectively), followed by sample R4D (occupied 8.82% and 0.68%, respectively). These results showed that the bacterial and fungal community structure at the phylum level were different in different urban rivers, with Proteobacteria, Cyanobacteria and Ascomycota phyla more susceptible to human activities.
3.4. Detection of waterborne pathogens
The waterborne pathogen community was identified by a total of 8 phyla, 13 classes, 19 orders, 32 families, 35 genera, 47 species according to the standard of USEPA. At the genus level, Mycobacterium was found to be the most dominant pathogen in all samples (>20%), which belongs to the phyla of Actinobacteria (). The second abundant was Burkholderia-Paraburkholderia (>5%), while Burkholderiales, Bartonella, Rhizobiales, Bordetella, Brucella were detected with relative low abundance (>1%). Coincidentally, they all belonged to the phyla of Proteobacteria and the abundance increased from upstream to downstream in human activity-influenced rivers (i.e., R4 and R19). To be specific, high abundances of Bartonella, Rhizobiales and Brucella were found in R4D, which accounted for 6.33%, 4.81% and 1.23%, respectively. High abundances of Burkholderiales and unclassified phyla proteobacteria were found in R19D, which accounted for 5.77% and 4.91%, respectively. In addition, potential waterborne pathogens with relatively low abundance (<1%) were also investigated. It was found that Mycoplasma was rich in R8U and R8M, while Helicobacter, Legionella, Pseudomonas, Salmonella, Enterococcus, Streptococcus, Escherichia-Shigella and Staphylococcus were rich in R4D and R8D. In particular, Escherichia-Shigella, Streptococcus, and Enterococcus which are considered to be human-associated enteric pathogens were detected in all the studied rivers except the non-human-activity-influenced river (i.e., R16). This result indicated that potential human contamination would favor the community of pathogens.
3.5. Correlations of environmental factors with waterborne pathogens
The relationship between microbial taxa and environmental factors was investigated by RDA analysis (), and the correlation coefficient (r2) values were shown in Table S2. Temperature (r2 = 0.58, p < 0.50) and TOC (r2 = 0.66, p < 0.50) were found to be the most significant environmental factors that shaped the bacterial community, while temperature (r2 = 0.77, p < 0.01) and nitrite (r2 = 0.54, p < 0.50) were significantly influential factors driving the fungal community. Spearman correlation was further used to examine the correlations between the specific phylum and environmental factors (). For bacteria, the factors of nitrite, temperature and TOC had a correlation with 5, 7 and 9 phyla, respectively. For instance, the relative abundance of Cyanobacteria was positively correlated with temperature and TOC, while the relative abundances of Dependentiae, Acidobacteria, and Nitrospirae displayed the opposite pattern. In addition, the relative abundance of Proteobacteria had a strong negative connection with temperature and TOC, while the relative abundance of Spirochaetes showed the opposite pattern. Notably, TDP was the only factor that had a positive correlation with all six phyla (i.e., Epsilonbacteraeota, Acidobacteria, Nitrospirae, Verrucomicrobia, Armatimonadetes, unclassified). For fungi, TDP was also the environmental factor that had the greatest impact on fungal communities, exhibiting a positive correlation with the abundance of Basidiomycota, Chytridiomycota, Mortierellomycota, Rozellomycota, and Zoopagomycota, and a negative correlation with Ascomycota (the predominant fungi in these samples). The abundance of Chytridiomycota had a negative correlation with 4 water indices (temperature, pH, UV254, and nitrite). Furthermore, pathogens at the genus level were also investigated. As shown in the spearman correlation heatmap in Figure S4, 21 of the 30 top genera were significantly correlated with these environmental factors. TDP was found to be the most important factor, which was positively correlated with 9 genera (Acinetobacter, Burkholderia-Paraburkholderia, Bacteroides, Pseudomonas, Escherichia-Shigella, Brucella, Helicobacter, Gammaproteobacteria and Enterobacteriaceae). Further, all 9 genera were identified to be negatively correlated with TOC and UV254, while 7 and 6 genera were positively related to temperature and nitrite, respectively. These results illustrated that the discharge of organic matters is closely related to the growth of different bacteria and fungi species.
Figure 6. The Spearman correlation heatmap between physicochemical factors and the top 20 abundant bacteria (a) and the top 9 abundant fungi (b) at the phylum level. A single (*), double asterisks (**), treble asterisks (***) represent statistically significant differences at p values < 0.05, 0.01 and 0.001 with t-test, respectively.
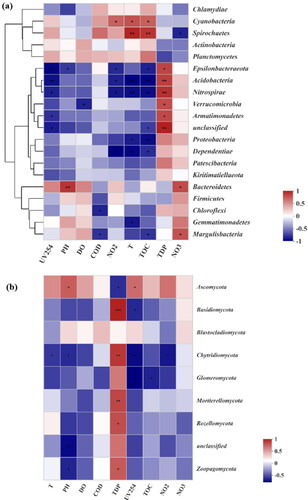
3.6. Functional predictions of potential pathogenic communities
The functional profiles were predicted according to PICRUSt analysis. The PICRUSt gene function prediction results were used to enrich the Kyoto Encyclopedia of Genes and Genomes (KEGG) pathway annotation information. In our study, a total of 6 KEGG level 1 pathways and 40 KEGG level 2 pathways were annotated in all samples, while the differences among the studied urban rivers were not significant (Figure S5(a)). Among the level 1 pathways, metabolism was the function most enriched (60%), including metabolism of carbohydrate, amino acids, lipid, cofactors and vitamins. Environmental information processing was the second most abundant function (16.83%), including the process of signal transduction, membrane transport, signaling molecules and interaction. Genetic information processing (12.13%) was the function containing the process of translation, transcription, replication and repair, folding, sorting and degradation. Cellular processes (3.15%) were the function containing the process of cell growth and death, transport and catabolism, cell motility. Organismal systems (1.14%) were the function containing immune system, excretory system, endocrine system, circulatory system. The KEGG level 2 pathways associated with human diseases were involved in infectious disease (bacterial, parasitic and viral), neurodegenerative disease, cancer, immune disease, endocrine and metabolic disease and cardiovascular disease (Figure S5(b)). It was noted that bacterial infectious disease was shown to be the most abundant pathway in all the studied rivers. The highest abundance of bacterial infectious disease was found at site R4D, suggesting the health risk of pathogenic microorganism contamination was more associated with human industrial activities.
3.7. Association with human activity
Human activities have raised great threats to coastal and estuary ecosystems worldwide, such as the input of nutrients, sewage, and industrial wastes. In this study, a great impact on the microbial community influenced by discharged chemicals from human activities was found. On the one hand, the concentrations of TDP (ranged from 0.76 mg/L to 1.98 mg/L) and COD (ranged from 32.2 mg/L to 147.2 mg/L) all exceeded the national surface water quality criteria IV (TDP < 0.3 mg/L; COD < 30 mg/L), indicating that the studied urban rivers suffered from severe phosphorus and organic matter load. Specially, R4 (industrial area) contained much higher concentration of TDP than the other three rivers, indicating that the river was richer in phosphorus nutrition which leads to the variation of microbial diversity, especially the multiplication of Proteobacteria and Chytridiomycota. On the other hand, previous research had revealed that Fluorescent Dissolved Organic Matter (FDOM), a type of soluble organic matter in water that contains humic acid, fulvic acid and aromatic polymers (Rodriguez-Avella et al. Citation2020), were more sensitive to anthropogenic sources, such as effluents of aquaculture, treated domestic and industrial wastewater (Du et al. Citation2021). In this study, the EEMs data with PARAFAC analysis showed that the terrestrial humic-like substances C1 had a higher maximum fluorescence intensities (FImax) level at R4 (Figure S6), which mainly existed in high nutrient and wastewater-impacted environments, indicating the direct influence by human activities through exporting anthropogenic organic matters. In addition, higher concentration of TOC and COD were found at site R8U (4.60 mg/L and 147.20 mg/L, respectively), followed by R4U (3.87 mg/L and 113.20 mg/L, respectively), further indicating that the urban rivers influenced by industrial and residential waste were suffered from organic pollutant contamination from human activity.
For all the studied rivers, the diversity and richness of bacterial community were higher than that of fungal community, which is consistent with the results of airborne microorganisms according to our previous report (Liang et al. Citation2020). At the phylum level, Actinobacteria (31.09%), Proteobacteria (26.38%) and Cyanobacteria (24.00%) were found to be the three most dominant bacteria, followed by Bacteroidetes (10.37%) (Figure S3). However, Mai et al. (Citation2018) demonstrated that Proteobacteria was the most abundant (63.97%) phyla in surface waters from Pearl River estuaries, followed by Actinobacteria (17.01%) and particularly low abundance of Cyanobacteria (1.82%). The results may be attributed to shorter distance from urban area and overall higher nutrient content in our studies, which likely promotes the growth of Actinobacteria and Cyanobacteria (Harris et al. Citation2016). Previous studies also found that the dominant phyla Actinobacteria and Proteobacteria in the Ganjiang River and Maozhou River under anthropogenic disturbances (Wang et al. Citation2016; Ouyang et al. Citation2020). Higher relative abundance of Actinobacteria and Proteobacteria indicated higher health risk, because they were the most abundant phylum in wastewater and hospital effluents, which contains the opportunistic pathogens, including Mycobacterium spp., Legionella spp., and M. avium (Marti et al. Citation2013). For fungi, Ascomycota was found to be the most abundant phylum occupying ∼42.49% of all the fungal sequences, while Chytridiomycota occupied 2.95%. Li et al. (Citation2016) also reported the dominant role of Ascomycota in the intertidal region, which was highly influenced by anthropogenic activities.
Furthermore, the potential waterborne pathogen community composition was analyzed at the genus level, indicating that Mycobacterium was the major genera in The Pearl River estuaries, followed by Burkholderia-Paraburkholderia (Figure S7). A previous study reported that Mycobacterium was also the dominant pathogens from the water supply network and typical urban surface water (Wolf-Baca and Piekarska Citation2020), which was positively related to autochthonous organisms (Leight et al. Citation2018). Several species of Mycobacterium were pathogenic to both animals and human beings, causing pulmonary and cutaneous disease, like tuberculosis (Jin et al. Citation2018; Tao et al. Citation2020). In contrast, Liang et al. (Citation2020) found the higher abundance of Burkholderia-Paraburkholderia, Bacillales and Staphylococcus in the bioaerosols samples at the same area. Jin et al. (Citation2018) has investigated 16 typical surface water samples and found that the most frequently identified genera were Pseudomonas and Aeromonas, while Ouyang et al. (Citation2020) found Vibrio and Arcobacter were the dominant genera in Maozhou River with anthropogenic disturbances. Except for these abundant pathogens, Burkholderiales, Bartonella, Rhizobiales, Bordetella, Brucella were detected with relative low abundances in this study, which has not been reported in previous research of this area. It should be noted that these species were highly related to human health. For instance, Burkholderia-pseudomallei, a Gram-negative pathogen, can cause melioidosis infected through wounds, mucous membranes and respiratory routes, often fatal infectious disease for humans. Bartonella is responsible for Oroya fever and Verruca Peruana. Bordetella can cause human pertussis, which is a highly contagious respiratory disease. Moreover, PICRUSt analysis predicted that the high relative abundance of functions related to human disease was bacterial infectious disease. Overall, the high abundance of pathogens in industrial-influenced and residential-influenced urban rivers suggests that more attention should be paid to these areas for risk management.
Spearman correlation showed that TDP was the main factor that driven both bacterial and fungal community compositions in this study. The concentration of TDP was positively correlated with six bacterial and five fungal phyla, which was consistent with the previous research that the increased nutrient loading from urban lands would stimulate the growth of bacteria (Shao et al. Citation2013; Du et al. Citation2021). In addition, UV254, Nitrate, TOC and Temperature were also shown to be significant factors to determine bacterial community structures. It was noted that TDP had positive correlation with almost all the potential pathogenic genera (Figure S4), while TOC, UV254 and Temperature were negatively correlated with some of them. This result further suggested that the surface water with high nutrient concentration (indicated by TDP) would provide a favorable environment for the growth of potential pathogens.
4. Conclusions
In this study, the pollution profile of waterborne bacterial and fungal community along with potential pathogens was investigated in urban rivers of Pearl River estuarine area. It was found that the microbial pollution was strongly related to the physiochemical characteristics and chemicals in the water samples. Anthropogenic activities, such as discharge of industrial waste, domestic sewage, agriculture and fishery pollution, changed the physicochemical parameters of the water and then increased the diversity of bacterial and fungal community, while the abundance and diversity of bacterial community were higher than that of fungi. Temperature, TOC and TDP were the major factors that drive both total microbial and pathogenic microbial community compositions. Besides, 35 major pathogens were detected in these samples at the level of genera while the most abundant was Mycobacterium, rising potential human health risk and a high risk of waterborne disease outbreak. Overall, the information provided in this study is valuable not only for in-depth understanding of the changes of microbial community driven by discharged chemicals from anthropogenic activities in estuary reservoirs, but also for assessment of microbial safety caused by the identified pathogens.
Supplemental Material
Download MS Word (1.2 MB)Disclosure statement
The authors declare no conflict of interest.
Data availability statement
High-throughput sequencing raw data of bacteria and fungi were submitted to the NCBI Sequence Read Archive (SRA) database with accession number PRJNA664612.
Additional information
Funding
Notes on contributors
Qiyan Gu
Qiyan Gu is a Postgraduate at School of Environmental Science and Engineering, Guangdong University of Technology, Guangzhou, China. Her work explores Data Curation, Formal analysis, Visualization, Writing - Original Draft;
Wanjun Wang
Wanjun Wang is a Professor at School of Environmental Science and Engineering, Guangdong University of Technology, Guangzhou, China. His work explores Conceptualization, Resources, Funding acquisition, Supervision;
Guiying Li
Guiying Li is a Professor at School of Environmental Science and Engineering, Guangdong University of Technology, Guangzhou, China. Her work explores Supervision, Resources;
Yan Liu
Yan Liu is a Postgraduate at School of Environmental Science and Engineering, Guangdong University of Technology, Guangzhou, China. Her work explores Software, Formal analysis;
Po Keung Wong
Po Keung Wong is a Professor at School of Environmental Science and Engineering, Guangdong University of Technology, Guangzhou, China. His work explores Validation, Visualization.
Taicheng An
Taicheng An is a Professor at School of Environmental Science and Engineering, Guangdong University of Technology, Guangzhou, China. His work explores Conceptualization, Supervision.
References
- Al Salah DMM, Ngweme GN, Laffite A, Otamonga J-P, Mulaji C, Poté J. 2020. Hospital wastewaters: A reservoir and source of clinically relevant bacteria and antibiotic resistant genes dissemination in urban river under tropical conditions. Ecotoxicol Environ Safety. 200:110767.
- Chen L, Xiong Z, Sun L, Yang J, Jin Q. 2012. VFDB 2012 update: toward the genetic diversity and molecular evolution of bacterial virulence factors. Nucleic Acids Res. 40(Database issue):D641–D645.
- Cui Q, Huang Y, Wang H, Fang T. 2019. Diversity and abundance of bacterial pathogens in urban rivers impacted by domestic sewage. Environ Pollut. 249:24–35.
- Derrien M, Kim MS, Ock G, Hong S, Cho J, Shin KH, Hur J. 2018. Estimation of different source contributions to sediment organic matter in an agricultural-forested watershed using end member mixing analyses based on stable isotope ratios and fluorescence spectroscopy. Sci Total Environ. 618:569–578.
- Du Y, Lu Y, Roebuck JA, Jr., Liu D, Chen F, Zeng Q, Xiao K, He H, Liu Z, Zhang Y, et al. 2021. Direct versus indirect effects of human activities on dissolved organic matter in highly impacted lakes. Sci Total Environ. 752:141839–141839.
- Duarte S, Bärlocher F, Trabulo J, Cássio F, Pascoal C. 2015. Stream-dwelling fungal decomposer communities along a gradient of eutrophication unraveled by 454 pyrosequencing. Fungal Diver. 70(1):127–148.
- Fang H, Wang H, Cai L, Yu Y. 2015. Prevalence of antibiotic resistance genes and bacterial pathogens in long-term manured greenhouse soils as revealed by metagenomic survey. Environ Sci Technol. 49(2):1095–1104.
- Gade L, McCotter OZ, Bowers JR, Waddell V, Brady S, Carvajal JA, Sunenshine R, Komatsu KK, Engelthaler DM, Chiller T, et al. 2020. The detection of Coccidioides from ambient air in Phoenix, Arizona: Evidence of uneven distribution and seasonality. Med Mycol. 58(4):552–559.
- Harjung A, Ejarque E, Battin T, Butturini A, Sabater F, Stadler M, Schelker J. 2019. Experimental evidence reveals impact of drought periods on dissolved organic matter quality and ecosystem metabolism in subalpine streams. Limnol Oceanogr. 64(1):46–60.
- Harris JM, Vinobaba P, Kularatne RKA, Kankanamge CE. 2016. Spatial and temporal distribution of cyanobacteria in Batticaloa Lagoon. J Environ Sci (China). 47:211–218.
- Jin D, Kong X, Cui B, Jin S, Xie Y, Wang X, Deng Y. 2018. Bacterial communities and potential waterborne pathogens within the typical urban surface waters. Sci Rep. 8(1):13368.
- Kowalczuk P, Tilstone GH, Zabłocka M, Röttgers R, Thomas R. 2013. Composition of dissolved organic matter along an Atlantic meridional transect from fluorescence spectroscopy and parallel factor analysis. Mar Chem. 157:170–184.
- Lambert T, Bouillon S, Darchambeau F, Massicotte P, Borges AV. 2016. Shift in the chemical composition of dissolved organic matter in the Congo River network. Biogeosciences. 13(18):5405–5420.
- Leight AK, Crump BC, Hood RR. 2018. Assessment of fecal indicator bacteria and potential pathogen co-occurrence at a shellfish growing area. Front Microbiol. 9:384.
- Li H, Lu L, Huang W, Yang J, Ran Y. 2014. In-situ partitioning and bioconcentration of polycyclic aromatic hydrocarbons among water, suspended particulate matter, and fish in the Dongjiang and Pearl Rivers and the Pearl River Estuary, China. Mar Pollut Bull. 83(1):306–316.
- Li W, Wang M, Bian X, Guo J, Cai L. 2016. A high-level fungal diversity in the intertidal sediment of Chinese seas presents the spatial variation of community composition. Front Microbiol. 7(2098):2098.
- Liang Z, Yu Y, Ye Z, Li G, Wang W, An T. 2020. Pollution profiles of antibiotic resistance genes associated with airborne opportunistic pathogens from typical area, Pearl River Estuary and their exposure risk to human. Environ Int. 143:105934.
- Liu Y, Lin Q, Feng J, Yang F, Du H, Hu Z, Wang H. 2020. Differences in metabolic potential between particle-associated and free-living bacteria along Pearl River Estuary. Sci Total Environ. 728:138856.
- Mai YZ, Lai ZN, Li XH, Peng SY, Wang C. 2018. Structural and functional shifts of bacterioplanktonic communities associated with spatiotemporal gradients in river outlets of the subtropical Pearl River Estuary, South China. Mar Pollut Bull. 136:309–321.
- Marti E, Jofre J, Luis Balcazar J. 2013. Prevalence of antibiotic resistance genes and bacterial community composition in a river influenced by a wastewater treatment plant. PLoS One. 8(10):e78906.
- Moehling TJ, Lee DH, Henderson ME, McDonald MK, Tsang PH, Kaakeh S, Kim ES, Wereley ST, Kinzer-Ursem TL, Clayton KN, et al. 2020. A smartphone-based particle diffusometry platform for sub-attomolar detection of Vibrio cholerae in environmental water. Biosens Bioelectron. 167:112497–112497.
- Mohiuddin MM, Botts SR, Paschos A, Schellhorn HE. 2019. Temporal and spatial changes in bacterial diversity in mixed use watersheds of the Great Lakes region. J Great Lakes Res . 45(1):109–118.
- Murphy KR, Hambly A, Singh S, Henderson RK, Baker A, Stuetz R, Khan SJ. 2011. Organic matter fluorescence in municipal water recycling schemes: toward a unified PARAFAC model. Environ Sci Technol. 45(7):2909–2916.
- Murphy KR, Stedmon CA, Waite TD, Ruiz GM. 2008. Distinguishing between terrestrial and autochthonous organic matter sources in marine environments using fluorescence spectroscopy. Mar Chem . 108(1-2):40–58.
- Murphy KR, Stedmon CA, Wenig P, Bro R. 2014. OpenFluor- an online spectral library of auto-fluorescence by organic compounds in the environment. Anal Methods . 6(3):658–661.
- Ouyang L, Chen H, Liu X, Wong MH, Xu F, Yang X, Xu W, Zeng Q, Wang W, Li S. 2020. Characteristics of spatial and seasonal bacterial community structures in a river under anthropogenic disturbances. Environ Pollut. 264:114818.
- Retelletti Brogi S, Kim J-H, Ryu J-S, Jin YK, Lee YK, Hur J. 2019. Exploring sediment porewater dissolved organic matter (DOM) in a mud volcano: Clues of a thermogenic DOM source from fluorescence spectroscopy. Mar Chem. 211:15–24.
- Rodriguez-Avella KA, Baraer M, Mark B, McKenzie J, Somers L. 2020. Comparing the performance of three methods to assess DOM dynamics within two distinct glacierized watersheds of the tropical Andes. Environ Pollut. 265(Pt A):115052–115052.
- Schloss PD, Westcott SL, Ryabin T, Hall JR, Hartmann M, Hollister EB, Lesniewski RA, Oakley BB, Parks DH, Robinson CJ, et al. 2009. Introducing mothur: open-source, platform-independent, community-supported software for describing and comparing microbial communities. Appl Environ Microbiol. 75(23):7537–7541.
- Shao K, Gao G, Wang Y, Tang X, Qin B. 2013. Vertical diversity of sediment bacterial communities in two different trophic states of the eutrophic Lake Taihu, China. J Environ Sci . 25(6):1186–1194.
- Singulani J. d L, da Silva J. d F, Gullo FP, Costa MC, Fusco-Almeida AM, Enguita FJ, Soares Mendes-Giannini MJ. 2020. Fungal-host interactions: insights into microRNA in response to Paracoccidioides species. Mem Inst Oswaldo Cruz. 115:e200238.
- Sun Z, Li G, Wang C, Jing Y, Zhu Y, Zhang S, Liu Y. 2014. Community dynamics of prokaryotic and eukaryotic microbes in an estuary reservoir. Sci Rep. 4:6966.
- Tao Y, Yun J, Wang J, Xu P, Li C, Liu H, Lan Y, Pan J, Du W. 2020. High-performance detection of Mycobacterium bovis in milk using digital LAMP. Food Chem. 327:126945.
- Wang P, Chen B, Yuan R, Li C, Li Y. 2016. Characteristics of aquatic bacterial community and the influencing factors in an urban river. Sci Total Environ. 569-570:382–389.
- Wang Y, Liu Y, Wang J, Luo T, Zhang R, Sun J, Zheng Q, Jiao N. 2019. Seasonal dynamics of bacterial communities in the surface seawater around subtropical Xiamen Island, China, as determined by 16S rRNA gene profiling. Mar Pollut Bull. 142:135–144.
- Wolf-Baca M, Piekarska K. 2020. Biodiversity of organisms inhabiting the water supply network of Wroclaw. Detection of pathogenic organisms constituting a threat for drinking water recipients. Sci Total Environ. 715:136732.
- Wu D, Su Y, Xi H, Chen X, Xie B. 2019. Urban and agriculturally influenced water contribute differently to the spread of antibiotic resistance genes in a mega-city river network. Water Res. 158:11–21.
- Wu J, Hong Y, Guan F, Wang Y, Tan Y, Yue W, Wu M, Bin L, Wang J, Wen J. 2016. A rapid and high-throughput microplate spectrophotometric method for field measurement of nitrate in seawater and freshwater. Sci Rep. 6:20165.
- Xie M, Wang W-X. 2020. Contrasting temporal dynamics of dissolved and colloidal trace metals in the Pearl River Estuary. Environ Pollut. 265(Pt B):114955.
- Xue N, Wang L, Li W, Wang S, Pan X, Zhang D. 2020. Increased inheritance of structure and function of bacterial communities and pathogen propagation in plastisphere along a river with increasing antibiotics pollution gradient. Environ Pollut. 265(Pt A):114641.
- Yamashita Y, Panton A, Mahaffey C, Jaffé R. 2011. Assessing the spatial and temporal variability of dissolved organic matter in Liverpool Bay using excitation–emission matrix fluorescence and parallel factor analysis. Ocean Dyn. 61(5):569–579.
- Ye F, Huang X, Zhang D, Tian L, Zeng Y. 2012. Distribution of heavy metals in sediments of the Pearl River Estuary, Southern China: Implications for sources and historical changes. J Environ Sci . 24(4):579–588.
- Yu J, Cleveland TE, Nierman WC, Bennett JW. 2005. Aspergillus flavus genomics: gateway to human and animal health, food safety, and crop resistance to diseases. Rev Iberoam Micol. 22(4):194–202.
- Zhang L, Zhou Y, Cheng Y, Lu W, Liang Y. 2021. Effect of different types of industrial wastewater on the bacterial community of urban rivers. J Freshwater Ecol. 36(1):31–48.
- Zhang W, Lei M, Li Y, Wang P, Wang C, Gao Y, Wu H, Xu C, Niu L, Wang L, et al. 2019. Determination of vertical and horizontal assemblage drivers of bacterial community in a heavily polluted urban river. Water Res. 161:98–107.