Abstract
Logwood and pellet stoves are popular heating sources around the world. The particulate matter emitted from such stoves contains organic particulate matter (OM), soot, and ash, each of which may have significant effects on climate and health. In this study, the primary OM (POM) emitted from a wood stove and a pellet stove operated according to standard Swiss testing protocols were characterized using aerosol mass spectrometry. The POM mass spectra were found to be highly reproducible, and contained CO+ as the dominant ion. Because the POM emitted by such stoves is typically enhanced by the condensation of gaseous organics following atmospheric aging, the secondary OM (SOM) formation potential of these stoves was simulated using the Micro Smog Chamber (MSC) designed by Keller and Burtscher in Citation2012. In general, OM emission factors from MSC-aged aerosols were comparable to lower-time-resolution results from the literature, although the MSC exposed aerosols to much higher concentrations of oxidants and therefore produced OM that was more oxidized than expected for atmospheric samples. In addition, the logwood-stove particles remained highly aspherical even after oxidation, indicating that mixing with an external aerosol is required for these particles to become spherical. The one exception to this observation occurred when the wood failed to ignite and appeared to generate tar-ball OM particles.
1. INTRODUCTION
Biofuel combustion is a major global source of primary organic aerosol as well as light-absorbing black carbon (BC) (Bond et al. Citation2013). The initially emitted particulate organic matter (OM) can be more than doubled in mass by the condensation of secondary OM (SOM) during subsequent oxidation chemistry (Grieshop et al. Citation2009b; Hennigan et al. Citation2011; Heringa et al. Citation2011; Ortega et al. Citation2013), although plume dilution may counteract this increase in some cases, as discussed by Hennigan et al. Citation(2011). In addition, this SOM can increase overall particle hygroscopicity (Dinar et al. Citation2007; Martin et al. Citation2012), light-scattering (Saathoff et al. Citation2003), and light-absorption (Saathoff et al. Citation2003), yet it remains inadequately represented in climate models (Bond et al. Citation2013). While not all source-apportionment techniques can distinguish between different sources of SOM, radiocarbon studies by Szidat et al. (Citation2006, Citation2007) have attributed up to 80% of total wintertime OM loadings in Swiss villages to wood combustion, and 40% in Swiss cities. Although the health impacts of this SOM are uncertain, the corresponding primary OM (POM) may contain significantly more harmful reactive oxygenated species than cigarette smoke (Miljevic et al. Citation2010). The regulation of both POM and SOM emissions is therefore important from both a health and climate perspective.
In Europe, where wood stoves are commonly used for space heating and contribute a large fraction to total PM concentrations on average (Fountoukis et al. Citation2014), emissions regulations include only POM and ash. The amount of SOM that may form when such emissions are aged in the atmosphere may be estimated by oxidizing fresh emissions in smog chambers or flow reactors. The amount of SOM formation observed in previous studies has been highly variable, but generally comparable to or greater than POM (Grieshop et al. Citation2009a, Citation2009b; Hennigan et al. Citation2011; Heringa et al. Citation2011; Ortega et al. Citation2013). Predicting such SOM formation is challenging due to the complex mixture of Volatile Organic Compounds (VOCs) present in the gas phase of combustion aerosols (Donahue et al. Citation2012; Ortega et al. Citation2013). Each constituent of this VOC mixture may condense as SOM if its vapour pressure is lowered by oxidation-initiated reactions. Mechanistically predicting the production of SOM by aerosol oxidation requires knowledge of the reactivity, vapour pressure, and concentration of the emitted VOCs (May et al. Citation2013) as well as their oxidation products (Donahue et al. Citation2012). Consequently, bulk VOC concentrations cannot be used to effectively predict SOM formation (Keller and Burtscher Citation2012) and SOM regulation is better achieved by simulated atmospheric aging.
Keller and Burtscher Citation(2012) have recently designed a continuous-flow photoreactor, the Micro Smog Chamber (MSC), for this purpose. The MSC employs a compact reaction volume (<300 cm3) to initiate SOM production by OH oxidation, and aims to provide a simple, real-time approach for the estimation of potential SOM.
This work aims to (i) provide a detailed characterization of the organic phase of a modern wood stove and a pellet stove, and (ii) evaluate the efficacy of the MSC as a tool for the online monitoring of SOM formation potential during the emissions testing of such stoves. Towards these ends, the OM produced by these stoves was measured by an Aerodyne High-Resolution Aerosol Mass Spectrometer (HR-AMS) for three cases: fresh (“POM”), oxidized (“OOM”), or filtered and oxidized (“SOM”) emissions. The focus is on the wood stove, which produced higher emissions of a more variable composition than the pellet stove. Rather than attempt to cover all possible combustion conditions and fuel types, the stoves were operated in the efficient modes that are defined by Swiss stove-testing protocols to maximize experimental reproducibility. The results are compared to previous atmospheric observations in terms of elemental ratios (O:C and H:C), OM density, and particle morphology.
2. METHODS
2.1. Experiment Setup and Instrumentation
Experiments were carried out at the wood-combustion test facility of the University of Applied Sciences and Arts Northwestern Switzerland (FHNW), an officially certified testing facility (Swiss register STS 396, European register NB 2113) using the setup shown in Figure . The stoves were operated by experienced test-cell personnel. Beech wood (Fagus sylvatica) logs were burnt in a Rüegg Mars 9 kW stove, according to Swiss federal type-approval protocols. Stove exhaust was directed through an indoor chimney and monitored for Non-Methane Hydrocarbons (NMHCs) and selected gases [O2, CO, CO2, and NO; details in Keller and Burtscher Citation(2012)]. Aerosols were sampled from the chimney, immediately diluted by a factor of eight with air heated to 160°C, then transported at 2 slpm via lines also heated to 160°C to the MSC, which samples 1 slpm.
FIG. 1. Experimental setup. Experiments were performed both with and without the particle filter and UV reactor, respectively. Note that the line to the UV reactor was diluted whereas the lower heated line (to the gas analyzers) was not. The rotating-disc dilutor was adjusted to reach varying dilution ratios, but was typically set to dilute by a factor of ∼20. The TEOM sampled through the bypass flow of the rotating-disc dilutor.
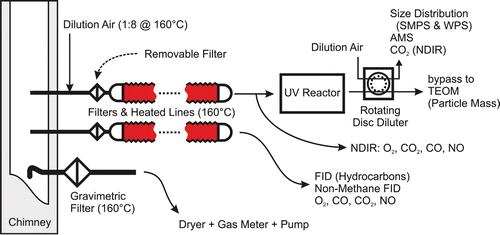
Following dilution, the above-mentioned gas analysis was repeated before aerosols passed through the MSC (Section 2.2) to a rotating-disc dilutor (Matter Aerosol, Switzerland). The bypass flow of the dilutor was sent to a Tapered Element Oscillating Microbalance (TEOM, Thermo Scientific, model 1400AB) for measurement of total particulate mass. Particle-size-distribution measurements (WPS, Model 1000XP, MSP Inc., providing optical-equivalent diameter measurements at 360–9500 nm) indicated that the TEOM was effectively sampling submicron PM (PM1). The TEOM data are considered lower limits for periods following spikes in OM concentrations, as the mass loading of its filter was observed to decrease significantly at these times, especially in the SOM experiment, indicating the volatilization of material (likely SOM) from the sampled particles.
The output of the rotating-disc dilutor (relative humidity [RH] <3%) was monitored for CO2 and H2O by a LICOR LI-840 gas analyzer, which was used to determine dilution ratios. In parallel, aerosol particulate composition was characterized by aerosol mass spectrometry (Section 2.5) and a scanning mobility particle sizer (SMPS, TSI Inc., model 3080/3081/3022) measured particle mobility diameters from 17–760 nm every 2 min. The mobility-sizing accuracy of the SMPS was verified to within 3% accuracy for 305 nm polystyrene latex spheres (BS-Partikel, Wiesbaden, Germany). The SMPS mobility distributions were used to estimate total mass for wood-stove SOM as detailed in the online supplementary information (SI; Section S1.3). This was not possible for the OOM- or POM-containing particles since these particles were highly aspherical (Section 6). It was also not possible for the pellet stove, the emissions of which fluctuated on a timescale of seconds. An aethalometer was also deployed during this study; however, its data were found to suffer from major artifacts due to the high PM loadings sampled in this study. BC concentration data were therefore not available.
2.2. Experimental Protocol
Three different cases were explored for the wood-stove and pellet burner with one day for each case. First, the fresh emissions were measured (POM case). Second, the entire primary aerosol was passed through the flow reactor for OOM experiments. Third, the primary aerosol was filtered such that only its gas phase was oxidized, nucleating to form SOM. In each case, stove emissions were sampled in real time. The aerosol composition therefore changed too rapidly to switch between POM, OOM, and SOM for a single burn; instead, the experiments were performed on different burns that were carried out as reproducibly as possible (see below).
Each wood-stove experiment consisted of adding beech wood to the stove in sequential batches of ∼3 kg, arranged in a consistent manner (Figure S1). The first logwood burns of each day were ignited using tinder and kindling and are not considered representative for this reason, and because of the lack of thermal equilibrium in the stove and chimney. Subsequent batches were allowed to self-ignite on the embers of the previous batch (Figure S1A) so that no kindling was required, with one exception: when the second “OOM” burn failed to ignite, a small amount of kindling was used and manually ignited.
The pellet stove was operated in its automatic configuration, using commercial (EN 14961-2 / A1 standard) pellets. Pellets were added continuously and automatically to a conical combustion crucible. A software-triggered 5-min cleaning cycle was entered approximately once per hour, removing ash from the crucible. Emissions therefore reflected a mixture of aerosols from freshly added and older pellets.
2.3. Micro Smog Chamber (MSC)
2.3.1. MSC Principles of Operation
The Micro Smog Chamber (MSC) consists of three identical UV-grade quartz tubes in series and is detailed by Keller and Burtscher Citation(2012). Each tube has an effective length of 25 cm, a volume of only 76 cm3, and a wall thickness of 1.5 mm. The connections and sealing between each tube were designed so that aerosols are exposed only to quartz or PTFE surfaces. The aerosol residence time in the MSC was on the order of seconds (flow: 1 L min−1 at 273 K and 1 atm), and the temperature approximately ∼90°C (due largely to the UV lamps).
As an aerosol enters the first MSC tube, it is exposed to 20 W of UVC (254 nm and 185 nm emission lines) generated by five low-pressure mercury lamps (Heraeus, type GPH212T5VH/2), forming O3 (∼60 ppm) from O2 and subsequently OH radicals from background water vapour. The room-temperature-equivalent RH from this background water was on average 18% (interquartile range: 8–25%), depending on the stage of combustion. In the second tube, 30 W of UVA radiates from a high-pressure halogen lamp (Panacol-Elosol, type UV-H 255) to drive further OH-radical chemistry. Based on follow-up experiments (Bruns et al. Citation2014) OH exposures during this study were estimated as roughly . This amount of oxidant exposure exceeds the expected upper limit of atmospheric exposures, and resulted in a highly oxidized aerosol.
Some amount of NOx or SO2 chemistry may also have occurred, depending on the combustion. The last tube allows the aerosol to cool slightly while reaction continues. In the present study, the second tube was removed for the final burn of each MSC experiment. No difference was observed in this case, so it is not discussed further.
The MSC does not aim to simulate atmospheric chemistry as accurately as possible, as in a smog chamber, but rather aims to rapidly oxidize VOCs to such a degree that any potential SOM will condense into the particulate phase. This approach may cause SOM yields to be underestimated due to fragmentation reactions (Lim and Ziemann Citation2009). Conversely, the increased density of highly oxidized SOM may have enhanced the measured SOM mass (Kroll et al. Citation2009). The MSC was designed in this way to allow transient emissions to be resolved during emission-regulation testing.
2.3.2. MSC Wall Losses
A crucial difference between the MSC and both smog chambers and the atmosphere is that the MSC oxidizes aerosols at extremely high concentrations. Combustion emissions are only slightly diluted (as stated above, by a factor of eight) before oxidation, such that some mg PM m−3 are typically present within the MSC. These extreme PM concentrations mean that diffusional losses to the particulate surface area (condensation sink; CS) are normally much higher than similar losses to the chamber-wall surface area during aerosol oxidation. Condensing gases are much more likely to condense onto aerosol particles than onto the walls, in contrast to smog chambers (Zhang et al. Citation2014), and larger flow reactors operated with lower PM concentrations (Lambe et al. Citation2011).
Figure illustrates this relationship between CS and wall (diffusional) losses for the MSC in terms of the lifetimes against 50% loss, , and
. Figure a shows these losses as a function of particle size, for a fixed CS. Figure b shows these losses as a function of CS, for the loss of 1 nm particles. The calculations for 1 nm particles also represent a loss estimate for the large, Extremely-Low Volatility Organic Compounds (ELVOCs), which have been implicated in SOM formation (Ehn et al. Citation2014), and which are expected to be slightly larger than 1 nm in mobility diameter (Kulmala et al. Citation2013).
FIG. 2. (a) Lifetime of particles (or large molecules) against diffusive loss on the MSC walls or on the PM condensation sink
for a typical combustion aerosol (after 10 × dilution). Number-weighted geometric mean diameters (GMD) and standard deviations (GSD) are shown. (b) Ratio of
to
for a 1 nm particle for varying CS. Thermophoretic forces and new-particle formation not included. The “concentration during MSC experiments” range was calculated for the unfiltered wood-burning experiments in this study (cf. Figures S6 and S7).
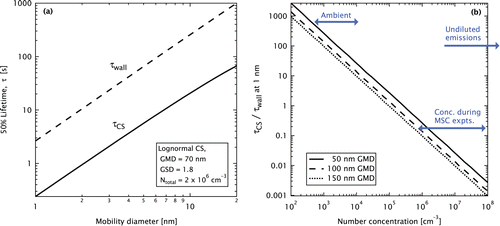
In Figure , diffusional losses were calculated for particles with diffusion coefficient D in a tube of length L with laminar volumetric flow Q. Such losses are a function only of the parameter ξ = πDL/Q, and not the tube diameter (Brockmann Citation2011), so it is the tube L/Q ratio and not the surface-area-to-volume ratio that governs wall losses in a cylindrical laminar-flow reactor.
The CS losses in Figure were calculated according to the Brownian coagulation equation (Friedlander Citation2000), assuming a negligible influence of coagulation on the reference size distribution (the reference being the x axis in Figure b).
The reference CS in Figure a, a lognormal distribution of GMD 70 nm, GSD 1.8, and total number , corresponds to a mass loading of 2 mg m−3 in the MSC, with an assumed density of 1200 kg m−3. This mass loading accounts for a ten-fold MSC dilution and conservatively represents an extreme minimum for a real-world, manually operated 9kW logwood stove. Emissions from such a stove typically exceed 50 mg m−3 at 13% O2; modern, well-operated appliances may emit several times this concentration. Note that these calculations have not considered thermophoretic forces, which would act to minimize wall losses since the MSC walls are heated by its UV lamps. Also, we have conservatively neglected the increase of particle surface area that occurs during gas-to-particle condensation or new-particle formation, as discussed further below. A more detailed model of the MSC will be presented in future work.
For 1 nm particles (representing ELVOCs), Figure a shows that a of 2.6 s is estimated. The corresponding
is 0.24 s, an order of magnitude smaller. Thus, under typical MSC operating conditions, the majority of ELVOCs are expected to be lost to the PM surface rather than to the MSC walls. These lifetimes may be compared with those recently reported by Ehn et al. Citation(2014), for ELVOCs important to the formation of α-pinene SOM, who estimated a wall-loss lifetime for ELVOCs of
s in their chamber.
Figure a shows that a changing diffusion coefficient (changing particle size) affects and
similarly, such that the ratio between the two remains practically unchanged. We therefore plotted this ratio in Figure b as a function of CS. The range of CS plotted represents the expected range for ambient to combustion sources, as labeled. Based on these calculations, less than 10% of 1 nm particles/molecules are expected to be lost to the MSC walls when operating at the concentrations for which it is designed. As noted above, this estimate is intended to represent a worst-case scenario where neither thermophoretic reductions in wall losses, nor CS enhancement via nucleation have been considered.
The above calculations did not address potential particulate losses in the sampling lines leading to and from the MSC (representing some meters of tubing). However, better than 50% penetration of 10 nm particles was observed in separate experiments when only 0.3 slpm were sampled through twice the length of tubing. In addition, the nucleation and growth of new particles was not considered. Such new particle formation was at times observed during the OOM experiments, as an additional mode of small particles (nucleation mode). The existence of this mode indicates that the new-particle-formation rate was much greater than the CS-loss rate (which in turn was much greater than the wall-loss rate). The above calculations conservatively neglected the formation of PM via nucleation and the consequent increase in CS. However, freshly nucleated particles were also too small for AMS quantification (see below), so that the emission factors reported below are lower limits.
2.4. Aerodyne Aerosol Mass Spectrometer
The HR-ToF-AMS (hereafter shortened to “AMS”) has been described in detail elsewhere (Jayne et al. Citation2000; DeCarlo et al. Citation2006). In brief, the instrument samples aerosol through an aerodynamic lens, which efficiently focusses particles with aerodynamic diameters between approximately 60 nm and 600 nm into a narrow beam. The lens opens into a vacuum-pumped chamber, where gas expansion accelerates particles to their size-dependent terminal velocity. Particles then transit a sizing chamber to be flash-vaporized by impaction upon a porous-tungsten thermal vaporizer held at 600°C. Only Non-Refractory Particulate Matter is vaporized (NR-PM; operationally defined, DeCarlo et al. Citation2006). The vapor is ionized by electron impact; the resulting ions are mass analyzed by pulsed extraction into an ion-time-of-flight chamber.
The AMS provides free-molecular regime aerodynamic size measurements by modulating the sampled particle beam with a mechanical “chopper” and acquiring time-resolved measurements. However, in normal operation the chopper is removed entirely (except when measuring background) to enhance throughput (“Mass Spectrum” mode). Data reported here are the mean of two 5-s-measurement, 5-s-background cycles. The AMS was operated in the shorter of its two mass spectral modes (“V” mode) with one reflectron pass. This mode was sufficient for the unambiguous distinction between the major mass-spectral ions, which had mass-to-charge ratios (m/z) below 100.
2.5. AMS Data Analysis
The AMS data were analyzed using the community software package SQUIRREL (version 1.51H) and PIKA (version 1.10H) as well as custom code written in Igor Pro (version 6, WaveMetrics Inc., OR, USA). High-resolution analysis (resolution 4200 at m/z 91) was used to identify the elemental composition of each peak. These elemental compositions were then used to estimate the overall elemental composition of the analyzed OM (Aiken et al. Citation2007, Citation2008).
Three modifications were made to the standard AMS high-resolution fragmentation table (Allan et al. Citation2004; Aiken et al. Citation2008), which is used to estimate the signal of OM-related ions that cannot be resolved from background or mass-spectral interferences based on the expected composition of atmospheric OM (Jimenez et al. Citation2009). First, gas-phase interferences at CO+2 were corrected for using a particle filter placed centimeters upstream of the AMS inlet. This filter was employed over brief intervals throughout each day to derive a time-resolved CO2(g) correction factor in combination with the CO2(g) measurements described above. Second, the OM-related signal at H2O+ was estimated using an H2O+:CO+2 ratio of unity, reflecting laboratory observations (Alfarra et al. Citation2004; Kiendler-Scharr et al. Citation2009; Sun et al. Citation2010; Chen et al. Citation2011). This change was reversed for the literature comparisons in Section 5.2, with minor impact, to the default 0.225.
Third, we measured CO+ directly using high-resolution PIKA analysis and removed the corresponding fragmentation-table entry. As CO+ was poorly resolved from the nearby N+2 peak from air at low mass loadings but varied linearly with CO+2 otherwise, linear regression was used to obtain CO+:CO+2 ratios of 3.92 ± 0.01, 1.22 ± 0.01, and 0.86 ± 0.02 for POM, OOM, and SOM, respectively (slope ± fit uncertainty). As discussed in the SI (Section S1.1), no other ions overlapped with the N+2 peak. The OOM and SOM ratios are within the range 0.9–1.25 expected for atmospheric OM (Aiken et al. Citation2008).
Even if particles are within the AMS lens-transmission range, particle bounce at the AMS vaporizer may reduce the observed signals. A composition-dependent correction factor, the bounce-related Collection Efficiency (CEb), must be derived to account for this effect (Crosier et al. Citation2007; Matthew et al. Citation2008; Mensah et al. Citation2012; Middlebrook et al. Citation2012). A CEb of 1.0 was used for wood-stove POM based on a re-evaluation of the literature in the context of our CO+ measurements (detailed in the SI). For the oxidized aerosols, a failed-start burn that produced OM-dominated particles (Section 6) was used to constrain the wood-stove OOM CEb to > 0.7 (Figure S3). Given previous evidence that the CEb of wood-combustion particles does not change upon oxidation (Hennigan et al. Citation2011), this observation suggested that a fixed CEb of 1.0 was appropriate in all wood-stove cases. The same CEb was applied to the pellet-stove data for lack of additional information. However, the reduced transmission of very small and/or irregular particles through the AMS lens meant that AMS SOM measurements were not quantitative except during spikes in SOM concentration. Therefore, all SOM and all pellet-stove measurements made with the AMS represent lower limits. In all cases, the CE (bounce-related or otherwise) represents the largest uncertainty in the AMS measurements.
3. WOOD-STOVE EMISSIONS
3.1. Reproducibility and Evolution of Burns
During the wood-stove experiments, burn-to-burn variability was minimized wherever possible. Burns were carried out indoors by a technician familiar with the Swiss protocol. The wood used was taken from a single batch, cut into equally sized pieces, and loaded into the stove in a consistent manner (Figure S1) between burns. The resulting modified combustion efficiency (MCE; the ratio of CO2 to CO2 plus CO emitted by the stove) was consistently ∼0.9 at the start of a burn and >0.99 during flaming combustion (Figure ).
FIG. 3. Time-resolved emission factors from the wood stove. (a–d) are: total PM for the POM experiment (TEOM data, 1–5), POM (AMS data, 1–5), OOM (AMS, 6–9), and SOM (SMPS, a–d, and AMS). SOM results include both SMPS (letters) and AMS (circles) data. Marker symbols (numbers and letters) indicate the burn sequence, with shading indicating modified combustion efficiency (MCE). The anomalously high OOM signals show an experiment where the second batch of wood failed to ignite. Also shown are the smog chamber data of Heringa et al. Citation(2011) with error bars described in the text.
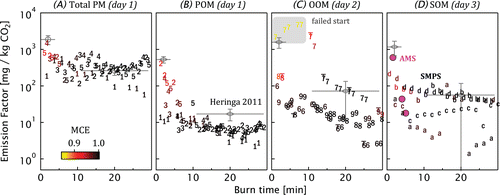
For the first wood-stove burns of each day (“cold start”) combustion was less vigorous and resulted in an aerosol of different composition to normal, consistent with previous studies (Miljevic et al. Citation2010; Lamberg et al. Citation2011). For the second and subsequent burns, the particle composition observed by the AMS was similar, as discussed below.
For each burn, OM loadings spiked with the addition of each batch of wood, reflecting pyrolysis and lower combustion efficiencies. During this “starting phase” the wood was only partially aflame (Figure S1A) so that pyrolysis may have dominated OM emissions. Once flames had engulfed the entire batch of wood (Figure S1B), low and stable OM emissions were observed for 10–20 min. This “flaming phase” and the testing protocol, ended when flames died out. Measurements during the subsequent “burnout phase” (Figure S1C) showed negligible emissions. Time series of the burns are included in the SI (Figures S6–S8).
3.2. Wood-Stove Emission Factors
Figure shows the emission factors observed during each burn in the three experiments. The emissions are normalized to CO2(g) to account for a changing combustion rate. Panels a–d represent the emission factors of total unoxidized PM (TEOM data), POM (AMS data), OOM (AMS), and SOM (SMPS and AMS), respectively. Of the available measurements, these data were specifically selected to minimize the possible influences of PM volatilization (TEOM), particle-transmission limits (AMS), and particle asphericity (SMPS), on the data, as described in Section 2.
In general, the emissions were lower for the first burn of each day, as shown by the numbered data symbols in the figure. This is likely related to the top-down combustion of that burn (Section 2) and suggests that separate wood-stove emission factors should be considered when modeling single burns (e.g., recreational burning [Fine et al. Citation2001]) or long-term combustion (e.g., home heating). The emission factors in Figure a are much higher than those in Figure b–d, indicating that most of the wood-stove PM consisted of refractory BC and ash (Lamberg et al. Citation2011; Heringa et al. Citation2012; Torvela et al. Citation2014) rather than OM. Refractory PM emission factors were relatively constant across the starting and flaming phases.
The data in Figure are colored by the Modified Combustion Efficiency (MCE): the molecular ratio of CO2(g) with the sum of CO(g) plus CO2(g). Starting-phase emissions were associated with poorer combustion efficiencies (∼0.9). The failed-start burn (Figure c, burn 7) showed the lowest MCE, reaching 0.8. Otherwise, trends in combustion efficiency were reproducible.
Heringa et al. Citation(2011) have reported emission factors from a smog-chamber study using a similar wood stove and fuel. Their results are included in Figure for context (gray symbols). Vertical and horizontal error bars represent measurement uncertainty and time-of-burn, respectively. The POM emission factors of Heringa et al. Citation(2011) shown in Figure b are generally higher than ours, and would be higher still if their CO+ signals (not measured) had been similar to ours (which represented 30% of the AMS OM signals).
The similarity between the MSC-derived emission factors and the smog-chamber derived emission factors of Heringa et al. Citation(2012) suggests that the MSC may be useful as a tool for the online estimation of SOM formation potential during regulatory testing. This similarity stands in contrast to the distinctly different conditions within the MSC and a smog chamber (Section 2.3), in particular, the much smaller size and corresponding much higher reactant and oxidant concentrations in the MSC. Future studies will directly compare the MSC to smog-chamber results to account for variability between combustion conditions.
Previous studies have reported “OM enhancements” as the ratio of OOM to POM for a given sample (Grieshop et al. Citation2009a; Hennigan et al. Citation2011; Heringa et al. Citation2011; Ortega et al. Citation2013). This was not possible in the present study, where the goal was to monitor SOM formation potential online rather than using a reservoir (smog chamber or otherwise). As noted above, such online monitoring is desirable for emissions regulation.
OM enhancements may be estimated from the OOM and POM observed in the two separate experiments, given their high reproducibility. Substantially more OOM (Figure c) than POM (Figure b) was observed, with an enhancement factor of 2–4 across all phases. This enhancement is similar to that observed in previous smog-chamber studies (Grieshop et al. Citation2009a; Heringa et al. Citation2011). However, it is important to note that these OM enhancements are dependent on the amount of dilution and on the dilution temperature of the aerosol (Ortega et al. Citation2013) since wood-combustion POM consists of a significant fraction of semivolatile material (Lipsky and Robinson Citation2006; May et al. Citation2013).
At the extreme oxidant exposures used in the present study, fragmentation reactions (C‒C bond cleavage, Ng et al. Citation2011a) may begin to form lower-volatility products, reducing the observed emission factors (Lambe et al. Citation2012). The importance of fragmentation reactions may be lower for cyclic organics such as levoglucosan (for which C‒C bond cleavage does not split the molecule), which are contained in large amounts in wood-burning OM (Shafizadeh Citation1985; Rogge et al. Citation1998; Simoneit et al. Citation1999; Fine et al. Citation2001; Graham et al. Citation2002). Nevertheless, fragmentation may play a significant role in the oxidation of combustion aerosols even at atmospherically realistic oxidant levels (Donahue et al. Citation2012).
If fragmentation played a more significant role in the MSC than in the atmosphere, the reported OM enhancements would be underestimated.
It is also important to note that a significant fraction of OOM partitioned into particles that were well below the minimum size for transmission into the AMS with unit efficiency (∼60 nm aerodynamic diameter; Liu et al. Citation2007) similarly to Bruns et al. Citation(2014). Assuming spherical particles, the SMPS measurements (Figures S5–S7) suggested that less than 5% of the total PM volume (which, besides OM, includes BC and ash) resided in such particles. This is a slight overestimation as the larger particles were aspherical. The fraction of OM that was not observed by the AMS cannot be accurately quantified without knowledge of the OM fraction in the larger mode. Based on the minimum OM content of 10% observed in an AMS for flaming-phase combustion by Heringa et al. Citation(2012), the present emission factors may have been underestimated by up to 50%. This represents the worst-case scenario where OM in the larger and smaller size modes are at their minimum and maximum, respectively (the nucleation mode did not always contribute significantly to particulate mass), and may have contributed to the enhanced apparent variability in the OOM emission factors in Figure c relative to Figure b. While this variability was already taken into account in making the conclusions above, the importance of the nucleation mode indicates that care must be taken when using an AMS to quantify samples where a significant fraction of NR-PM is found in particles with an aerodynamic diameter below 60 nm.
FIG. 4. Time-resolved emission factors for the pellet stove from the TEOM (black squares) and AMS (green circles). The shaded periods show the self-cleaning periods of the stove. AMS error bars represent the uncertainty due to unquantified . All data are lower limits (see text).
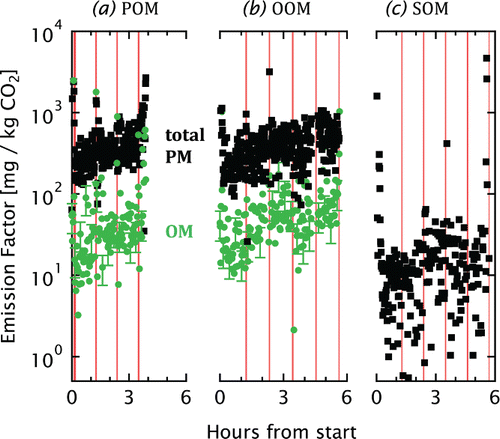
Figure d shows the SOM emission factors, which have been estimated from the SMPS data because most particles were too small for AMS quantification: the estimated mode diameter of the volume-weighted SMPS distributions was 35–62 nm (interquartile range). The SMPS distributions were fitted, integrated, and converted to mass using a measured SOM density, as described in the SI (Section S1). Figure d also includes three AMS measurements taken when SOM particles were largest (to minimize the impacts of AMS lens transmission), which corroborate the SMPS analysis. The SOM emission factors are comparable to those of Heringa et al. Citation(2011) and Keller and Burtscher Citation(2012), similar to the above-discussed OOM emission factors.
4. PELLET-STOVE EMISSIONS
The pellet stove produced a much more complete combustion than the logwood stove, and thus produced much less OM. Consequently, all CO+ signals (the major wood-stove signals) were below detection limit and were estimated as a factor of 2.5 ± 1.5 greater than CO+2 (the mean of the wood-stove POM and OOM cases).
4.1. Pellet-Stove Emission Factors
Time-resolved emission factors for the pellet burner are shown in Figure . In contrast to the wood-stove emission factors, TEOM measurements are included for all cases (black squares) since inspection of the data indicated that volatilization artifacts were negligible. The high time resolution of the MSC allows spikes to be resolved during self-cleaning periods (red bars). Given their short duration, these spikes made a relatively small contribution to the integrated emissions.
OM emission factors from the AMS for the pellet burner are shown in Figure for POM and OOM (green circles). The pellet-stove OOM emissions were a factor of 1.3 ± 0.1 higher than the pellet-stove POM (geometric mean ± geometric standard deviation, including self-cleaning periods and not accounting for changes in CO+ signals or the CE). This factor, which represents steady-state conditions, is lower than the factor of 2.3 ± 1.0 enhancement reported by Heringa et al. Citation(2011) for the starting phase of the exact same pellet stove. The pellet-stove OOM represented only about 15% of the total emissions (Figure ) from the stove.
Pellet-stove SOM could not be quantified by AMS, as the particles were again too small. An SMPS analysis as performed for the wood stove was not possible because the continuous addition of pellets to the combustion generated fluctuations in SOM on a timescale much shorter than that of an SMPS scan. Therefore, only the TEOM emission factors are presented in Figure . These data are in agreement with previous MSC results (Keller and Burtscher Citation2012).
5. WOOD-STOVE PM COMPOSITION AND PROPERTIES
In the subsections below the mass spectrum and elemental composition of the wood-stove OM are discussed in detail. Atmospherically relevant characteristics of the primary wood-combustion emissions are highlighted and the efficacy of the MSC as an online SOM-formation monitoring tool is simultaneously discussed. The pellet-stove emissions are not discussed in similar detail since neither CO+ (the major ion in wood-stove OM) nor AMS sizing data (required to calculate effective densities) were above their detection limits.
5.1. OM Mass Spectrum
OM comprised the majority of the NR-PM emitted by the wood stove. All OM mass spectra were dominated by low-mass (m/z < 80), oxygenated organic ions, as shown in Figure .
FIG. 5. AMS mass spectra of fresh wood-stove emissions (POM, a), oxidized emissions (OOM, b), and filtered and oxidized emissions (SOM, c). Bars for and related ions are drawn thinner since these ions were not directly measured.
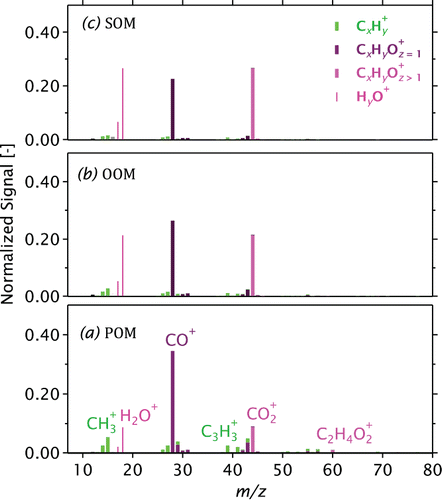
The POM mass spectrum (Figure a) was dominated by CO+ and CO+2 (and the H2O+ signals predicted from CO+2, Section 2.6). The major ion was CO+, in contrast to typical atmospheric OM where the CO+ and CO+2 signals are similar (Aiken et al. Citation2008). Overall, this highly oxygenated POM mass spectrum is consistent with previous AMS studies on beech wood combusted in logwood stoves (Weimer et al. Citation2008; Heringa et al. Citation2011; Elsasser et al. Citation2013). Although this study is the first wood-stove study to identify and quantify CO+, two others (Grieshop et al. Citation2009b; Eriksson et al. Citation2014) have reported elevated signals at m/z 28 (most likely CO+ or C2H+4) and other biomass-burning studies (Ortega et al. Citation2013) have observed similar patterns. As discussed in the SI (Section S1.1), negligible amounts of C2H+4 were produced by our sample. It is worth emphasizing that overloaded stoves or poor combustion conditions may create considerably different POM to that observed here, with less oxygenation and significant PAH content (Elsasser et al. Citation2013; Eriksson et al. Citation2014).
Figure b shows the OOM mass spectrum, which contained less CO+ and more CO+2 than the POM spectrum. While the OOM mass spectrum may have reflected a mixture of SOM and oxidized POM (Hennigan et al. Citation2011), the MSC residence time of seconds, the much slower rate of heterogeneous versus gas-phase oxidation of organics (Lambe et al. Citation2009; Donahue et al. Citation2013), and the elevated temperatures within the MSC (Section 2.3) suggest that SOM condensed from gas-phase reaction products may have dominated the OOM mass spectrum in this study. This is consistent with the qualitative similarity between the OOM and SOM mass spectra (Figure b and c), which differ mainly in a slightly lower CO+:CO+2 ratio of SOM (Section 2.6). For comparison to the wood-stove results, the pellet-stove mass spectra are shown in Figure S4.
5.2. H:C and O:C ratios
Figure plots hydrogen- and oxygen-to-carbon ratios (H:C and O:C) for POM, OOM, and SOM. To place the data in an atmospheric context, Mexico City measurements from Heald et al. Citation(2010) are also shown. Each Mexico City data point reflects either bulk or factor-analysis result from a given measurement site (Aiken et al. Citation2008, Citation2009) as defined in the legend.
FIG. 6. H:C and O:C ratios for wood-stove OOM and SOM in comparison to ambient observations from Mexico City (Heald et al. Citation2010). The two biomass-burning cases identified by Heald et al. Citation(2010) are highlighted. The numbered diagonal lines illustrate different average carbon oxidation states Kroll et al. (Citation2011) for reference.
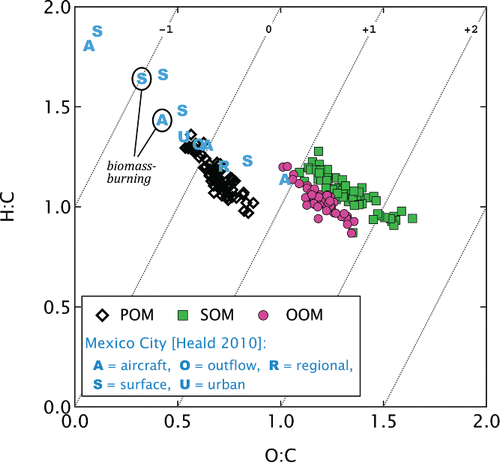
Figure shows that the POM data were shifted downwards and to the right relative to the Heald et al. Citation(2010) data, including for the two biomass-burning examples highlighted in the figure. This shift, indicative of a more-oxygenated aerosol, is unlikely to be an artifact of the different CO+:CO+2 ratio used by Heald et al. Citation(2010) relative to the current study, as the mass spectra from which the Heald et al. Citation(2010) elemental ratios were derived contained less oxygenated ions overall.
In Figure , the O:C ratios of OOM and SOM elemental ratios (approximately 1.2) are significantly higher than those of POM (approximately 0.7) and of the most-aged sample observed by Heald et al. Citation(2010) (approximately 1.0). These O:C ratios correspond to average carbon oxidation states () of 0.3, 1.4, and 1.4 for POM, OOM, and SOM, respectively, for the median O:C and H:C measurements. These
values are more robust to the uncertainty due to the unquantified organic H2O+ signals (Canagaratna et al. Citation2015) discussed in Section 2.6. The highly oxidized OOM and SOM is consistent with the high oxidant levels in the MSC, and suggests that the degree of MSC oxidation used in future studies might be reduced to achieve a degree of oxidation closer to atmospheric observations.
5.3. f44 – f43 Plot
As wood-stove combustion POM is a major component of European wintertime aerosols (Szidat et al. Citation2007; Fountoukis et al. Citation2014), the location of the current POM sample in f44 – f43 space (Ng et al. Citation2010) is of interest for the interpretation of future atmospheric measurements. This space is frequently used to characterize the age of unit-mass-resolution (UMR) AMS mass spectra, from which H:C and O:C ratios cannot be accurately derived.
Figure shows the f44 – f43 plot that would be obtained with a UMR AMS from the present data (filled symbols) together with the triangle of Ng et al. Citation(2010), which shows the location of aged atmospheric OM in f44 – f43 space. As CO+ would likely have been below the detection limit in an atmospheric study, the data are shown after calculation with the default fragmentation table (CO+:CO+2 =1) and with the updated fragmentation table used in all other plots (CO+:CO+2 = 3.92, as measured). Only the primary emissions (POM) are shown, since the OOM and SOM samples were established as lying outside of the atmospheric range in Section 5.2.
FIG. 7. The POM f44 vs. f43 triangle plot (Ng et al. Citation2010, Citation2011a), for both the measured data (brown symbols) and the result that would be obtained using the default fragmentation-table entry (green symbols) for CO+. The filled symbols represent the plot that would be obtained with a unit-mass-resolution (UMR) instrument; the open symbols a high-resolution (HR) instrument. The shaded area reflects ambient observations (Ng et al. Citation2011a). Only POM results are shown as the OOM and SOM samples were more oxidized than would be observed in the atmosphere (Figure ).
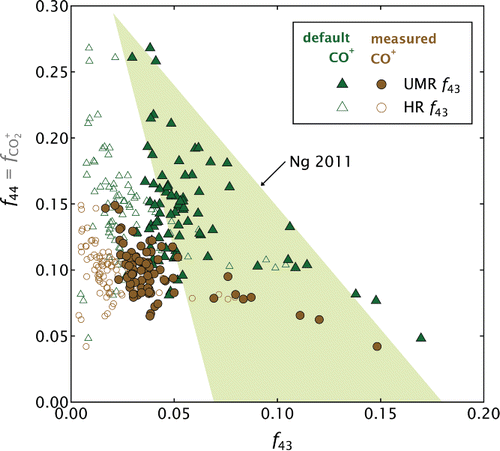
The open circles in Figure provide the most meaningful comparison with the Ng triangle, as f43 in this data set contained a significant contribution from C3+7 in addition to the C2H3O+ ion, which it is intended to represent (Ng et al. Citation2011a). These circles illustrate that the present sample was not compositionally similar to aged atmospheric OM. However, f43 is much higher when it is defined by including both ions at m/z 43 (filled circles), as would be the case if a UMR instrument such as the Aerosol Chemical Speciation Monitor (ACSM, Ng et al. Citation2011b) had been used. In addition, f44 would have been much higher had CO+ not been measurable (open triangles), as is the case for most atmospheric or smog-chamber measurements. This increase reflects the removal of the major contribution of CO+ to the mass spectrum, which leads CO+2 to be overemphasized.
Together, these two common limitations cause the wood-combustion OM to lie within the Ng triangle (filled triangles), which may lead to the misleading interpretation of fresh wood-combustion OM as secondary atmospheric OM. Air masses impacted by wood-combustion from efficient logwood stoves, as found in Europe (Alfarra et al. Citation2004), should therefore not be summarized in f44 – f43 space. For such air masses, the biomass-burning OM (BBOM) f44-O:C parameterization proposed by Ortega et al. Citation(2013) may provide a better metric than f44 – f43 ratios. Our data are in good agreement with the Ortega et al. Citation(2013) parameterization when the measured CO+:CO+2 ratio is used.
6. WOOD-STOVE PARTICLE PROPERTIES
The AMS-SMPS data provided information on three basic particle properties: the OM/OC ratios required to convert filter-based carbon measurements to OM mass (Turpin and Lim Citation2001; Aiken et al. Citation2008), OM density, and particle effective density (reflecting particle morphology).
Our wood-stove POM, OOM, and SOM samples had average OM/OC ratios of 2.3 ± 0.2, 2.8 ± 0.3, and 3.9 ± 0.3, respectively (mean ± standard deviation). This POM ratio is within the range 2.2–2.6 reported by Turpin and Lim Citation(2001) and was derived using the measured CO+ and updated H2O+ discussed in Section 2.6. If CO+ had not been measured, the POM OM/OC would have been significantly different: 1.8 ± 0.1 with the default fragmentation table, 2.0 ± 0.1 with the adjustment to the H2O+ signal attributed to OM (Section 2.6). Using these values to estimate POM from filter measurements would have significantly underestimated OM.
To investigate particle morphology, we calculated effective densities () from the AMS- and SMPS-measured aerodynamic and mobility sizes (DeCarlo et al. Citation2004; Bahreini et al. Citation2005), as detailed in the SI (Section S1.4). As an effective density is the density that an observed particle would have if it was spherical, the calculated
are by definition smaller than the particle material density. However, it is worth noting that the AMS-SMPS effective density is not equivalent to the effective density frequently reported by mass-mobility studies (e.g., Park et al. Citation2004). Neglecting slip corrections, the current
is the particle density divided by the product of the free-molecular (from the AMS) and transition-regime (from the SMPS) shape factors (DeCarlo et al. Citation2004), whereas the mass-mobility effective density is the particle density divided by the cubed transition-regime shape factor. The AMS-SMPS
will therefore be closer to the particle density than the mass-mobility effective density. The relationships described by DeCarlo et al. Citation(2004) can be used to verify that this conclusion remains true even when slip corrections are included. In both cases, the effective density is the density a particle would have if it was spherical, and is therefore smaller than the particle density for irregularly shaped particles.
The measured are shown in Figure . For the SOM sample, which often consisted of very small particles, the data represent a single period during which large particles (aerodynamic diameter >100 nm) were observed. The representativeness of this period is discussed below. The freshly nucleated SOM particles are known to be spherical, therefore
. This SOM density is much higher than that observed from other secondary OM studies, exceeding the values given by Turpin and Lim Citation(2001) and Hallquist et al. Citation(2009), and may be related to the abnormally high degree of oxidation (Kroll et al. Citation2009) of the MSC-produced SOM.
FIG. 8. Effective densities measured by AMS-SMPS (gray) and OM densities (green) parameterized from H:C and O:C ratios (Kuwata et al. Citation2012). As well as the POM, OOM, and SOM cases, the special failed-start OOM burn is included. Horizontal dashed lines show the OM densities reported by Hallquist et al. Citation(2009) for typical SOM and by Turpin and Lim Citation(2001) for urban OM.
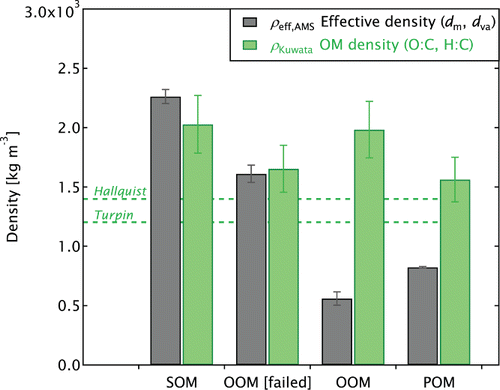
The OM density was also independently determined using the approach of Kuwata et al. Citation(2012). This method parameterizes the density of OM using only O:C and H:C ratios and is therefore independent of particle shape (and ). This estimate of OM density is referred to as the Kuwata density,
. The valid range of the Kuwata density has previously determined as 750–1900 kg m−3 (12% accuracy, Kuwata et al. Citation2012); however, the parameterization provided a density estimate for the SOM that was not significantly different from the measurement-based approach described above (Figure S5).
is a useful quantity since
could only be determined when particles and mass loadings were large enough. A time-resolved evaluation of
verified that the SOM density was similar (within 15% of
) across all stages of the burn (Figure S5).
For the failed-start OOM case, and
were also in agreement. This indicates that particles were near-spherical and comprised mostly of OM. These particles may be comparable to the “tar balls” reported by a number of microscopy studies for wildfires (Li et al. Citation2003; Pósfai et al. Citation2003, Citation2004; China et al. Citation2013). As these particles were only observed for this anomalous failed-start burn, and wood-stove tar balls have been reported as absent by previous microscopy studies (Kocbach et al. Citation2005; Vernooij et al. Citation2009; Leskinen et al. Citation2014), we infer that tar balls are not normally produced by well-operated wood stoves.
For the OOM and POM cases, was well below 900 kg m−3. As this
is below the density of the major particle components [
(Figure ),
, Park et al. Citation(2004)], it shows that particles were significantly aspherical. Such asphericity is expected for particles from flaming combustion, which produces fractal aggregates of black carbon spherules (Kocbach et al. Citation2005; Gwaze et al. Citation2006; Leskinen et al. Citation2014). However, asphericity is also expected to decrease when OM coatings are formed (Ghazi and Olfert Citation2013). The fact that our OOM particles (after being coated with a similar mass of OM as observed in previous studies; Section 3.2) remained highly aspherical after aging indicates that the particles produced by flaming wood combustion in such a stove may also remain highly aspherical, unless mixed with aerosols from other sources. This conclusion is consistent with atmospheric observations suggesting that such external mixing is often insufficient for BC-containing particles to appear spherical (Johnson et al. Citation2005; Adachi et al. Citation2010). Models that represent aged soot as coated spheres (Jacobson Citation2001) may therefore obtain biased estimates of light absorption by BC for well-operated wood stoves and other BC sources producing aerosols with similar fractions of organic mass.
7. CONCLUSIONS
Beech wood combustion in a modern, well-operated wood stove may produce highly oxygenated primary OM. During efficient combustion, this POM contributes only a minor mass fraction of total stove emissions, but may contribute much more if wood is added to the stove but fails to ignite. In contrast, modern pellet stoves produce negligible amounts of OM relative to their total PM emissions.
The AMS mass spectrum of such POM yielded CO+ as the major ion. This CO+ signal impacts estimates of O:C and H:C ratios, OM/OC ratios, f44 and f43, and instrument collection efficiency. These impacts highlight the importance of quantifying CO+ in wood-combustion AMS studies, and in other AMS studies where oxygenated primary OM is measured.
Oxidation of the wood-stove OM in the MSC generated OM that was more oxidized than expected for atmospheric SOM. This level of oxidation may be reduced in future studies. Nevertheless, SOM yields derived from the MSC were within the range expected from a previous smog-chamber study of beech-wood combustion in a logwood stove (Heringa et al. Citation2011). This consistency supports the intended use of the MSC as a simple and online tool for SOM-formation-potential estimation. Additional studies are needed (Bruns et al. Citation2014) and have been planned to determine the desirable level of oxidant concentrations in the MSC for this task. These studies will focus on achieving realistic SOA yields, since an atmospherically realistic SOA composition is not required for the MSC to achieve its goal of producing a simple, online estimate of SOA formation for the purposes of stove regulation and intercomparison.
ACKNOWLEDGMENTS
The authors gratefully acknowledge Josef Wüest, Erich Wildhaber, and Martin Büchler for their support during the measurements.
FUNDING
This work was supported by the Swiss National Science Foundation, the Swiss Federal Offices for Energy (SFOE) and Environment (FOEN), and the OPTIWARES project of the Competence Centers for Environment and Sustainability (CCES) and Energy and Mobility (CCEM) of the ETH Zürich.
SUPPLEMENTARY MATERIALS
Supplemental data for this article can be accessed on the publisher’s website. It includes extended data analysis discussion: CO+ quantification, AMS collection efficiency, emission factor calculations, and particle effective densities. Supplemental figures include: experimental setup, additional mass spectra, and additional time series.
UAST_1079586_supplementary_file.PDF
Download PDF (2.7 MB)REFERENCES
- Adachi, K., Chung, S. H., and Buseck, P. R. (2010). Shapes of Soot Aerosol Particles and Implications for Their Effects on Climate. J. Geophys. Res. Atmos., 115, doi:10.1029/2009JD012868.
- Aiken, A., Salcedo, D., Cubison, M. J., Huffman, J., DeCarlo, P., Ulbrich, I. M., Docherty, K. S., Sueper, D., Kimmel, J., Worsnop, D. R., Trimborn, A., Northway, M., Stone, E., Schauer, J. V. R., Fortner, E., de Foy, B., Wang, J., Laskin, A., Shutthanandan, V., Zheng, J., Zhang, R., Gaffney, J., Marley, N. A., Paredes-Miranda, G., Arnott, W., Molina, L., Sosa, G., and Jimenez, J. (2009). Mexico City Aerosol Analysis during MILAGRO Using High Resolution Aerosol Mass Spectrometry at the Urban Supersite (T0)–Part 1: Fine Particle Composition and Organic Source Apportionment. Atmos. Chem. Phys., 9:6633–6653, doi:10.5194/acp-9-6633-2009.
- Aiken, A. C., DeCarlo, P. F., and Jimenez, J. L. (2007). Elemental Analysis of Organic Species with Electron Ionization High-Resolution Mass Spectrometry. Anal. Chem., 79:8350–8358, doi:10.1021/ac071150w.
- Aiken, A. C., DeCarlo, P. F., Kroll, J. H., Worsnop, D. R., Huffman, J. A., Docherty, K. S., Ulbrich, I. M., Mohr, C., Kimmel, J. R., and Sueper, D. (2008). O/C and OM/OC Ratios of Primary, Secondary, and Ambient Organic Aerosols with High-Resolution Time-of-Flight Aerosol Mass Spectrometry. Environ. Sci. Technol., 42:4478–4485, doi:10.1021/es703009q.
- Alfarra, M. R., Coe, H., Allan, J. D., Bower, K. N., Boudries, H., Canagaratna, M. R., Jimenez, J. L., Jayne, J. T., Garforth, A. A., and Li, S.-M. (2004). Characterization of Urban and Rural Organic Particulate in the Lower Fraser Valley Using Two Aerodyne Aerosol Mass Spectrometers. Atmos. Environ., 38:5745–5758, doi:10.1016/j.atmosenv.2004.01.054.
- Allan, J. D., Delia, A. E., Coe, H., Bower, K. N., Alfarra, M. R., Jimenez, J. L., Middlebrook, A. M., Drewnick, F., Onasch, T. B., and Canagaratna, M. R. (2004). A Generalised Method for the Extraction of Chemically Resolved Mass Spectra from Aerodyne Aerosol Mass Spectrometer Data. J. Aerosol Sci., 35:909–922, doi:10.1016/j.jaerosci.2004.02.007.
- Bahreini, R., Keywood, M., Ng, N., Varutbangkul, V., Gao, S., Flagan, R., Seinfeld, J., Worsnop, D., and Jimenez, J. (2005). Measurements of Secondary Organic Aerosol from Oxidation of Cycloalkenes, Terpenes, and m-Xylene Using an Aerodyne Aerosol Mass Spectrometer. Environ. Sci. Technol., 39:5674–5688, doi:10.1021/es048061a.
- Bond, T. C., Doherty, S. J., Fahey, D. W., Forster, P. M., Berntsen, T., DeAngelo, B. J., Flanner, M. G., Ghan, S., Kärcher, B., Koch, D., Kinne, S., Kondo, Y., Quinn, P. K., Sarofim, M. C., Schultz, M. G., Schulz, M., Venkataraman, C., Zhang, H., Zhang, S., Bellouin, N., Guttikunda, S. K., Hopke, P. K., Jacobson, M. Z., Kaiser, J. W., Klimont, Z., Lohmann, U., Schwarz, J. P., Shindell, D., Storelvmo, T., Warren, S. G., and Zender, C. S. (2013). Bounding the Role of Black Carbon in the Climate System: A Scientific Assessment. J. Geophys. Res. Atmos., 118:5380–5552, doi:10.1002/jgrd.50171.
- Brockmann, J. (2011). Aerosol Transport in Sampling Lines and Inlets, in Aerosol Measurement: Principles, Techniques, and Applications, 3rd ed., P. Kulkarni, P. A. Baron, and K. Willeke, eds., John Wiley, Hoboken, NJ, pp. 69–105.
- Bruns, E. A., El Haddad, I., Keller, A., Klein, F., Kumar, N. K., Pieber, S. M., Corbin, J. C., Slowik, J. G., Brune, W. H., Baltensperger, U., and Prévôt, A. S. H. (2014). Inter-Comparison of Laboratory Smog Chamber and Flow Reactor Systems on Organic Aerosol Yield and Composition. Atmos. Meas. Tech. Discuss., 8:309–352, doi:10.5194/amtd-8-309-2015.
- Canagaratna, M., Jimenez, J., Kroll, J., Chen, Q., Kessler, S., Massoli, P., Hildebrandt Ruiz, L., Fortner, E., Williams, L., Wilson, K., Surratt, J., Donahue, N., Jayne, J., and Worsnop, D. R. (2015). Elemental Ratio Measurements of Organic Compounds Using Aerosol Mass Spectrometry: Characterization, Improved Calibration, and Implications. Atmos. Chem. Phys., 15:253–272, doi:10.5194/acp-15-253-2015.
- Chen, Q., Liu, Y., Donahue, N. M., Shilling, J. E., and Martin, S. T. (2011). Particle-Phase Chemistry of Secondary Organic Material: Modeled Compared to Measured O:C and H:C Elemental Ratios Provide Constraints. Environ. Sci. Technol., 45:4763–4770, doi:10.1021/es104398s.
- China, S., Mazzoleni, C., Gorkowski, K., Aiken, A. C., and Dubey, M. K. (2013). Morphology and Mixing State of Individual Freshly Emitted Wildfire Carbonaceous Particles. Nat. Comm., 4, doi:10.1038/ncomms3122.
- Crosier, J., Allan, J., Coe, H., Bower, K., Formenti, P., and Williams, P. (2007). Chemical Composition of Summertime Aerosol in the Po Valley (Italy), Northern Adriatic and Black Sea. Quart. J. Roy. Meteor. Soc., 133:61–75, doi:10.1002/qj.88.
- DeCarlo, P., Slowik, J., Worsnop, D., Davidovits, P., and Jimenez, J. (2004). Particle Morphology and Density Characterization by Combined Mobility and Aerodynamic Diameter Measurements. Part 1: Theory. Aerosol Sci. Technol., 38:1185–1205, doi:10.1080/027868290903907.
- DeCarlo, P. F., Kimmel, J. R., Trimborn, A., Northway, M. J., Jayne, J. T., Aiken, A. C., Gonin, M., Fuhrer, K., Horvath, T., and Docherty, K. S. (2006). Field-Deployable, High-Resolution, Time-of-Flight Aerosol Mass Spectrometer. Anal. Chem., 78:8281–8289, doi:10.1021/ac061249n.
- Dinar, E., Taraniuk, I., Graber, E., Anttila, T., Mentel, T. F., and Rudich, Y. (2007). Hygroscopic Growth of Atmospheric and Model Humic-Like Substances. J. Geophys. Res. Atmos., 112, doi:10.1029/2006JD007442.
- Donahue, N., Chuang, W., Epstein, S., Kroll, J., Worsnop, D., Robinson, A., Adams, P., and Pandis, S. (2013). Why Do Organic Aerosols Exist? Understanding Aerosol Lifetimes Using the Two-Dimensional Volatility Basis Set. Environ. Chem., 10:151–157, doi:10.1071/EN13022.
- Donahue, N., Kroll, J., Pandis, S., and Robinson, A. (2012). A Two-Dimensional Volatility Basis Set–Part 2: Diagnostics of Organic-Aerosol Evolution. Atmos. Chem. Phys., 12:615–634, doi:10.5194/acp-12-615-2012.
- Ehn, M., Thornton, J. A., Kleist, E., Sipilä, M., Junninen, H., Pullinen, I., Springer, M., Rubach, F., Tillmann, R., Lee, B., et al. (2014). A Large Source of Low-Volatility Secondary Organic Aerosol. Nature, 506:476–479, doi:10.1038/nature13032.
- Elsasser, M., Busch, C., Orasche, J., Schön, C., Hartmann, H., Schnelle-Kreis, J., and Zimmermann, R. (2013). Dynamic Changes of the Aerosol Composition and Concentration during Different Burning Phases of Wood Combustion. Energy Fuels, 27:4959–4968, doi:10.1021/ef400684f.
- Eriksson, A., Nordin, E., Nyström, R., Pettersson, E., Swietlicki, E., Bergvall, C., Westerholm, R., Boman, C., and Pagels, J. H. (2014). Particulate PAH Emissions from Residential Biomass Combustion: Time-Resolved Analysis with Aerosol Mass Spectrometry. Environ. Sci. Technol., 48(12):7143–7150, doi:10.1021/es500486j.
- Fine, P. M., Cass, G. R., and Simoneit, B. R. (2001). Chemical Characterization of Fine Particle Emissions from Fireplace Combustion of Woods Grown in the Northeastern United States. Environ. Sci. Technol., 35:2665–2675, doi:10.1021/es001466k.
- Fountoukis, C., Butler, T., Lawrence, M., Denier van der Gon, H., Visschedijk, A., Charalampidis, P., Pilinis, C., and Pandis, S. (2014). Impacts of Controlling Biomass Burning Emissions on Wintertime Carbonaceous Aerosol in Europe. Atmos. Environ., 87:175–182, doi:10.1016/j.atmosenv.2014.01.016.
- Friedlander, S. (2000). Smoke, Dust, and Haze: Fundamentals of Aerosol Dynamics. Oxford University Press, Oxford, UK.
- Ghazi, R., and Olfert, J. (2013). Coating Mass Dependence of Soot Aggregate Restructuring Due to Coatings of Oleic Acid and Dioctyl Sebacate. Aerosol Sci. Technol., 47:192–200, doi:10.1080/02786826.2012.741273.
- Graham, B., Mayol-Bracero, O. L., Guyon, P., Roberts, G. C., Decesari, S., Facchini, M. C., Artaxo, P., Maenhaut, W., Köll, P., and Andreae, M. O. (2002). Water-Soluble Organic Compounds in Biomass Burning Aerosols Over Amazonia 1. Characterization by NMR and GC-MS. J. Geophys. Res. Atmos., 107:LBA–14, doi:10.1029/2001JD000336.
- Grieshop, A., Donahue, N., and Robinson, A. (2009a). Laboratory Investigation of Photochemical Oxidation of Organic Aerosol from Wood Fires 2: Analysis of Aerosol Mass Spectrometer Data. Atmos. Chem. Phys., 9:2227–2240, doi:10.5194/acp-9-2227-2009.
- Grieshop, A., Logue, J., Donahue, N., and Robinson, A. (2009b). Laboratory Investigation of Photochemical Oxidation of Organic Aerosol from Wood Fires 1: Measurement and Simulation of Organic Aerosol Evolution. Atmos. Chem. Phys., 9:1263–1277, doi:10.5194/acp-9-1263-2009.
- Gwaze, P., Schmid, O., Annegarn, H. J., Andreae, M. O., Huth, J., and Helas, G. (2006). Comparison of Three Methods of Fractal Analysis Applied to Soot Aggregates from Wood Combustion. J. Aerosol Sci., 37:820–838, doi:10.1016/j.jaerosci.2005.06.007.
- Hallquist, M., Wenger, J., Baltensperger, U., Rudich, Y., Simpson, D., Claeys, M., Dommen, J., Donahue, N., George, C., and Goldstein, A. (2009). The Formation, Properties and Impact of Secondary Organic Aerosol: Current and Emerging Issues. Atmos. Chem. Phys., 9:5155–5236, doi:10.5194/acp-9-5155-2009.
- Heald, C., Kroll, J., Jimenez, J., Docherty, K., DeCarlo, P., Aiken, A., Chen, Q., Martin, S., Farmer, D., and Artaxo, P. (2010). A Simplified Description of the Evolution of Organic Aerosol Composition in the Atmosphere. Geophys. Res. Lett., 37, doi:10.1029/2010GL042737.
- Hennigan, C., Miracolo, M., Engelhart, G., May, A., Presto, A., Lee, T., Sullivan, A., McMeeking, G., Coe, H., and Wold, C. (2011). Chemical and Physical Transformations of Organic Aerosol from the Photo-Oxidation of Open Biomass Burning Emissions in an Environmental Chamber. Atmos. Chem. Phys., 11:7669–7686, doi:10.5194/acpd-11-11995-2011.
- Heringa, M., DeCarlo, P., Chirico, R., Lauber, A., Doberer, A., Good, J., Nussbaumer, T., Keller, A., Burtscher, H., and Richard, A. (2012). Time-Resolved Characterization of Primary Emissions from Residential Wood Combustion Appliances. Environ. Sci. Technol., 46:11418–11425, doi:10.1021/es301654w.
- Heringa, M., DeCarlo, P., Chirico, R., Tritscher, T., Dommen, J., Weingartner, E., Richter, R., Wehrle, G., Prévôt, A., and Baltensperger, U. (2011). Investigations of Primary and Secondary Particulate Matter of Different Wood Combustion Appliances with a High-Resolution Time-of-Flight Aerosol Mass Spectrometer. Atmos. Chem. Phys., 11:5945–5957, doi:10.5194/acp-11-5945-2011.
- Jacobson, M. Z. (2001). Strong Radiative Heating Due to the Mixing State of Black Carbon in Atmospheric Aerosols. Nature, 409:695–697, doi:10.1038/35055518.
- Jayne, J. T., Leard, D. C., Zhang, X., Davidovits, P., Smith, K. A., Kolb, C. E., and Worsnop, D. R. (2000). Development of an Aerosol Mass Spectrometer for Size and Composition Analysis of Submicron Particles. Aerosol Sci. Technol., 33:49–70, doi:10.1080/027868200410840.
- Jimenez, J. L., Canagaratna, M. R., Donahue, N. M., Prévôt, A. S. H., Zhang, Q., Kroll, J. H., DeCarlo, P. F., Allan, J. D., Coe, H., Ng, N. L., Aiken, A. C., Docherty, K. S., Ulbrich, I. M., Grieshop, A. P., Robinson, A. L., Duplissy, J., Smith, J. D., Wilson, K. R., Lanz, V. A., Hueglin, C., Sun, Y. L., Tian, J., Laaksonen, A., Raatikainen, T., Rautiainen, J., Vaattovaara, P., Ehn, M., Kulmala, M., Tomlinson, J. M., Collins, D. R., Cubison, M. J. E., Dunlea, J., Huffman, J. A., Onasch, T. B., Alfarra, M. R., Williams, P. I., Bower, K., Kondo, Y., Schneider, J., Drewnick, F., Borrmann, S., Weimer, S., Demerjian, K., Salcedo, D., Cottrell, L., Griffin, R., Takami, A., Miyoshi, T., Hatakeyama, S., Shimono, A., Sun, J. Y., Zhang, Y. M., Dzepina, K., Kimmel, J. R., Sueper, D., Jayne, J. T., Herndon, S. C., Trimborn, A. M., Williams, L. R., Wood, E. C., Middlebrook, A. M., Kolb, C. E., Baltensperger, U., and Worsnop, D. R. (2009). Evolution of Organic Aerosols in the Atmosphere. Science, 326:1525–1529, doi:10.1126/science.1180353.
- Johnson, K. S., Zuberi, B., Molina, L., Molina, M. J., Iedema, M. J., Cowin, J. P., Gaspar, D. J., Wang, C., and Laskin, A. (2005). Processing of Soot in an Urban Environment: Case Study from the Mexico City Metropolitan Area. Atmos. Chem. Phys., 5:3033–3043, doi:10.5194/acp-5-3033-2005.
- Keller, A., and Burtscher, H. (2012). A Continuous Photo-Oxidation Flow Reactor for a Defined Measurement of the SOA Formation Potential of Wood Burning Emissions. J. Aerosol Sci., 49:9–20, doi:10.1016/j.jaerosci.2012.02.007.
- Kiendler-Scharr, A., Zhang, Q., Hohaus, T., Kleist, E., Mensah, A., Mentel, T. F., Spindler, C., Uerlings, R., Tillmann, R., and Wildt, J. (2009). Aerosol Mass Spectrometric Features of Biogenic SOA: Observations from a Plant Chamber and in Rural Atmospheric Environments. Environ. Sci. Technol., 43:8166–8172, doi:10.1021/es901420b.
- Kocbach, A., Johansen, B., Schwarze, P., and Namork, E. (2005). Analytical Electron Microscopy of Combustion Particles: A Comparison of Vehicle Exhaust and Residential Wood Smoke. Sci. Total Environ., 346:231–243, doi:10.1016/j.scitotenv.2004.10.025.
- Kroll, J. H., Donahue, N. M., Jimenez, J. L., Kessler, S. H., Canagaratna, M. R., Wilson, K. R., Altieri, K. E., Mazzoleni, L. R., Wozniak, A. S., Bluhm, H., et al. (2011). Carbon Oxidation State as a Metric for Describing the Chemistry of Atmospheric Organic Aerosol. Nature Chem., 3:133–139, doi:10.1038/nchem.948.
- Kroll, J. H., Smith, J. D., Che, D. L., Kessler, S. H., Worsnop, D. R., and Wilson, K. R. (2009). Measurement of Fragmentation and Functionalization Pathways in the Heterogeneous Oxidation of Oxidized Organic Aerosol. Phys. Chem. Chem. Phys., 11:8005–8014, doi:10.1039/B905289E.
- Kulmala, M., Kontkanen, J., Junninen, H., Lehtipalo, K., Manninen, H. E., Nieminen, T., Petäjä, T., Sipilä, M., Schobesberger, S., Rantala, P., Franchin, A., Jokinen, T., Järvinen, E., Äijälä, M., Kangasluoma, J., Hakala, J., Aalto, P. P., Paasonen, P., Mikkilä, J., Vanhanen, J., Aalto, J., Hakola, H., Makkonen, U., Ruuskanen, T., Mauldin III, R. L., Duplissy, J., Vehkamäki1, H., Bäck, J., Kortelainen, A., Riipinen, I., Kurtén, T., Johnston, M. V., Smith, J. N., Ehn, M., Mentel, T. F., Lehtinen, K. E. J., Laaksonen, A., Kerminen, V.-M., and Worsnop, D. R. (2013). Direct Observations of Atmospheric Aerosol Nucleation. Science, 339:943–946, doi:10.1126/science.1227385.
- Kuwata, M., Zorn, S. R., and Martin, S. T. (2012). Using Elemental Ratios to Predict the Density of Organic Material Composed of Carbon, Hydrogen, and Oxygen. Environ. Sci. Technol., 46:787–794, doi:10.1021/es202525q.
- Lambe, A., Ahern, A., Williams, L., Slowik, J., Wong, J., Abbatt, J., Brune, W., Ng, N., Wright, J., Croasdale, D., Worsnop, D., Davidovits, P., and Onasch, T. (2011). Characterization of Aerosol Photooxidation Flow Reactors: Heterogeneous Oxidation, Secondary Organic Aerosol Formation and Cloud Condensation Nuclei Activity Measurements. Atmos. Meas. Tech., 4:445–461, doi:10.5194/amt-4-445-2011.
- Lambe, A. T., Miracolo, M. A., Hennigan, C. J., Robinson, A. L., and Donahue, N. M. (2009). Effective Rate Constants and Uptake Coefficients for the Reactions of Organic Molecular Markers (n-Alkanes, Hopanes, and Steranes) in Motor Oil and Diesel Primary Organic Aerosols with Hydroxyl Radicals. Environ. Sci. Technol., 43:8794–8800, doi:10.1021/es901745h.
- Lambe, A. T., Onasch, T. B., Croasdale, D. R., Wright, J. P., Martin, A. T., Franklin, J. P., Massoli, P., Kroll, J. H., Canagaratna, M. R., and Brune, W. H. (2012). Transitions from Functionalization to Fragmentation Reactions of Laboratory Secondary Organic Aerosol (SOA) Generated from the OH Oxidation of Alkane Precursors. Environ. Sci. Technol., 46:5430–5437, doi:10.1021/es300274t.
- Lamberg, H., Nuutinen, K., Tissari, J., Ruusunen, J., Yli-Pirilä, P., Sippula, O., Tapanainen, M., Jalava, P., Makkonen, U., and Teinilä, K. (2011). Physicochemical Characterization of Fine Particles from Small-Scale Wood Combustion. Atmos. Environ., 45:7635–7643, doi:10.1016/j.atmosenv.2011.02.072.
- Leskinen, J., Ihalainen, M., Torvela, T., Kortelainen, M., Lamberg, H., Tiitta, P., Jakobi, G., Grigonyte, J., Joutsensaari, J., Sippula, O., Tissari, J., Virtanen, A., Zimmermann, R., and Jokiniemi, J. (2014). Effective Density and Morphology of Particles Emitted from Small-Scale Combustion of Various Wood Fuels. Environ. Sci. Technol., 48:13 298–13 306, doi:10.1021/es502214a.
- Li, J., Pósfai, M., Hobbs, P. V., and Buseck, P. R. (2003). Individual Aerosol Particles from Biomass Burning in Southern Africa: 2. Compositions and Aging of Inorganic Particles. J. Geophys. Res. Atmos., 108, doi:10.1029/2002JD002310.
- Lim, Y. B., and Ziemann, P. J. (2009). Effects of Molecular Structure on Aerosol Yields from OH Radical-Initiated Reactions of Linear, Branched, and Cyclic Alkanes in the Presence of NOx. Environ. Sci. Technol., 43:2328–2334, doi:10.1021/es803389s.
- Lipsky, E. M., and Robinson, A. L. (2006). Effects of Dilution on Fine Particle Mass and Partitioning of Semivolatile Organics in Diesel Exhaust and Wood Smoke. Environ. Sci. Technol., 40:155–162, doi:10.1021/es050319p.
- Liu, P. S. K., Deng, R., Smith, K. A., Williams, L. R., Jayne, J. T., Canagaratna, M. R., Moore, K., Onasch, T. B., Worsnop, D. R., and Deshler, T. (2007). Transmission Efficiency of an Aerodynamic Focusing Lens System: Comparison of Model Calculations and Laboratory Measurements for the Aerodyne Aerosol Mass Spectrometer. Aerosol Sci. Technol., 41:721–733, doi:10.1080/02786820701422278.
- Martin, M., Tritscher, T., Jurányi, Z., Heringa, M. F., Sierau, B., Weingartner, E., Chirico, R., Gysel, M., Prévôt, A. S. H., and Baltensperger, U. (2012). Hygroscopic Properties of Fresh and Aged Wood Burning Particles. J. Aerosol Sci., 56:15–29, doi:10.1016/j.jaerosci.2012.08.006.
- Matthew, B. M., Middlebrook, A. M., and Onasch, T. B. (2008). Collection Efficiencies in an Aerodyne Aerosol Mass Spectrometer as a Function of Particle Phase for Laboratory Generated Aerosols. Aerosol Sci. Technol., 42:884–898, doi:10.1080/02786820802356797.
- May, A. A., Levin, E. J. T., Hennigan, C. J., Riipinen, I., Lee, T., Collett, J. L., Jimenez, J. L., Kreidenweis, S. M., and Robinson, A. L. (2013). Gas-Particle Partitioning of Primary Organic Aerosol Emissions: 3. Biomass Burning. J. Geophys. Res., 118:11,327–11,338, doi:10.1002/jgrd.50828.
- Mensah, A., Holzinger, R., Otjes, R., Trimborn, A., Mentel, T. F., ten Brink, H., Henzing, B., and Kiendler-Scharr, A. (2012). Aerosol Chemical Composition at Cabauw, The Netherlands as Observed in Two Intensive Periods in May 2008 and March 2009. Atmos. Chem. Phys., 12:4723–4742, doi:10.5194/acp-12-4723-2012.
- Middlebrook, A. M., Bahreini, R., Jimenez, J. L., and Canagaratna, M. R. (2012). Evaluation of Composition-Dependent Collection Efficiencies for the Aerodyne Aerosol Mass Spectrometer Using Field Data. Aerosol Sci. Technol., 46:258–271, doi:10.1080/02786826.2011.620041.
- Miljevic, B., Heringa, M., Keller, A., Meyer, N., Good, J., Lauber, A., Decarlo, P., Fairfull-Smith, K., Nussbaumer, T., and Burtscher, H. (2010). Oxidative Potential of Logwood and Pellet Burning Particles Assessed by a Novel Profluorescent Nitroxide Probe. Environ. Sci. Technol., 44:6601–6607, doi:10.1021/es100963y.
- Ng, N., Canagaratna, M., Jimenez, J., Chhabra, P., Seinfeld, J., and Worsnop, D. (2011a). Changes in Organic Aerosol Composition with Aging Inferred from Aerosol Mass Spectra. Atmos. Chem. Phys., 11:6465–6474, doi:10.5194/acp-11-6465-2011.
- Ng, N., Canagaratna, M., Zhang, Q., Jimenez, J., Tian, J., Ulbrich, I., Kroll, J., Docherty, K., Chhabra, P., and Bahreini, R. (2010). Organic Aerosol Components Observed in Northern Hemispheric Datasets from Aerosol Mass Spectrometry. Atmos. Chem. Phys., 10:4625–4641, doi:10.5194/acp-10-4625-2010.
- Ng, N. L., Herndon, S. C., Trimborn, A., Canagaratna, M. R., Croteau, P., Onasch, T. B., Sueper, D., Worsnop, D. R., Zhang, Q., and Sun, Y. (2011b). An Aerosol Chemical Speciation Monitor (ACSM) for Routine Monitoring of the Composition and Mass Concentrations of Ambient Aerosol. Aerosol Sci. Technol., 45:780–794, doi:10.1080/02786826.2011.560211.
- Ortega, A., Day, D., Cubison, M., Brune, W., Bon, D., de Gouw, J., and Jimenez, J. (2013). Secondary Organic Aerosol Formation and Primary Organic Aerosol Oxidation from Biomass-Burning Smoke in a Flow Reactor During FLAME-3. Atmos. Chem. Phys., 13:11 551–11 571, doi:10.5194/acp-13-11551-2013.
- Park, K., Kittelson, D. B., Zachariah, M. R., and McMurry, P. H. (2004). Measurement of Inherent Material Density of Nanoparticle Agglomerates. J. Nanopart. Res., 6:267–272, doi:10.1023/B:NANO.0000034657.71309.e6.
- Pósfai, M., Gelencsér, A., Simonics, R., Arató, K., Li, J., Hobbs, P. V., and Buseck, P. R. (2004). Atmospheric Tar Balls: Particles from Biomass and Biofuel Burning. J. Geophys. Res. Atmos., 109, doi:10.1029/2003JD004169.
- Pósfai, M., Simonics, R., Li, J., Hobbs, P. V., and Buseck, P. R. (2003). Individual Aerosol Particles from Biomass Burning in Southern Africa: 1. Compositions and Size Distributions of Carbonaceous Particles. J. Geophys. Res. Atmos., 108, doi:10.1029/2002JD002291.
- Rogge, W. F., Hildemann, L. M., Mazurek, M. A., Cass, G. R., and Simoneit, B. R. (1998). Sources of Fine Organic Aerosol. 9. Pine, Oak, and Synthetic Log Combustion in Residential Fireplaces. Environ. Sci. Technol., 32:13–22, doi:10.1021/es960930b.
- Saathoff, H., Naumann, K.-H., Schnaiter, M., Schöck, W., Möhler, O., Schurath, U., Weingartner, E., Gysel, M., and Baltensperger, U. (2003). Coating of Soot and (NH4)2SO4 Particles by Ozonolysis Products of a-Pinene. J. Aerosol Sci., 34:1297–1321, doi:10.1016/S0021-8502(03)00364-1.
- Shafizadeh, F. (1985). Pyrolytic Reactions and Products of Biomass, in Fundamentals of Thermochemical Biomass Conversion, R. Overend, T. Milne, and L. Mudge, eds., Springer, Dordrecht, the Netherlands, pp. 183–217, doi:10.1007/978-94-009-4932-4_11.
- Simoneit, B., Schauer, J., Nolte, C., Oros, D., Elias, V., Fraser, M., Rogge, W., and Cass, G. (1999). Levoglucosan, a Tracer for Cellulose in Biomass Burning and Atmospheric Particles. Atmos. Environ., 33:173–182, doi:10.1016/s1352-2310(98)00145-9.
- Sun, Y., Zhang, Q., Anastasio, C., and Sun, J. (2010). Insights into Secondary Organic Aerosol Formed via Aqueous-Phase Reactions of Phenolic Compounds Based on High Resolution Mass Spectrometry. Atmos. Chem. Phys., 10:4809–4822, doi:10.5194/acp-10-4809-2010.
- Szidat, S., Jenk, T. M., Synal, H.-A., Kalberer, M., Wacker, L., Hajdas, I., Kasper-Giebl, A., and Baltensperger, U. (2006). Contributions of Fossil Fuel, Biomass-Burning, and Biogenic Emissions to Carbonaceous Aerosols in Zurich as Traced by 14C. J. Geophys. Res. Atmos., 111, doi:10.1029/2005JD006590.
- Szidat, S., Prévôt, A. S. H., Sandradewi, J., Alfarra, M. R., Synal, H.-A., Wacker, L., and Baltensperger, U. (2007). Dominant Impact of Residential Wood Burning on Particulate Matter in Alpine Valleys during Winter. Geophys. Res. Lett., 34:L05 820, doi:10.1029/2006GL028325.
- Torvela, T., Tissari, J., Sippula, O., Kaivosoja, T., Leskinen, J., Virén, A., Lähde, A., and Jokiniemi, J. (2014). Effect of Wood Combustion Conditions on the Morphology of Freshly Emitted Fine Particles. Atmos. Environ., 87:65–76, doi:10.1016/j.atmosenv.2014.01.028.
- Turpin, B. J., and Lim, H.-J. (2001). Species Contributions to PM2.5 Mass Concentrations: Revisiting Common Assumptions for Estimating Organic Mass. Aerosol Sci. Technol., 35:602–610, doi:10.1080/02786820119445.
- Vernooij, M. G. C., Mohr, M., Tzvetkov, G., Zelenay, V., Huthwelker, T., Kaegi, R., Gehrig, R., and Grobéty, B. (2009). On Source Identification and Alteration of Single Diesel and Wood Smoke Soot Particles in the Atmosphere: An X-Ray Microspectroscopy Study. Environ. Sci. Technol., 43:5339–5344, doi:10.1021/es800773h.
- Weimer, S., Alfarra, M. R., Schreiber, D., Mohr, M., Prévôt, A. S. H., and Baltensperger, U. (2008). Organic Aerosol Mass Spectral Signatures from Wood-Burning Emissions: Influence of Burning Conditions and Wood Type. J. Geophys. Res. Atmos., 113, doi:10.1029/2007JD009309.
- Zhang, X., Cappa, C. D., Jathar, S. H., McVay, R. C., Ensberg, J. J., Kleeman, M. J., and Seinfeld, J. H. (2014). Influence of Vapor Wall Loss in Laboratory Chambers on Yields of Secondary Organic Aerosol. Proc. Natl. Acad. Sci., 111:5802–5807, doi:10.1073/pnas.1404727111, 2014.