Abstract
Copyright © American Association for Aerosol Research
EDITOR:
Dear Editor,
Recently a manuscript was published in Aerosol Science and Technology (50:2, 118–125, 2016; doi: 10.1080/02786826.2015.1136403) by D. M. Murphy, entitled, “The effects of molecular weight and thermal decomposition on the sensitivity of a thermal desorption aerosol mass spectrometer.” That manuscript presents a simple model (hereafter “M16 model”) for the vaporization and ionization of molecules in a vacuum system, similar to the processes occurring in the Aerodyne Aerosol Mass Spectrometer (AMS). The idealized model is based on the dispersion of molecules in the free molecular regime and predicts an additional square root dependence of the ionization efficiency on the molecular weight (MW) of the molecule of interest. This model correctly accounts for only one of the many complex processes that affect AMS sensitivity to aerosol chemical components.
The AMS instrument is widely used in the international atmospheric sciences community. As experienced AMS users, we find the Murphy (Citation2016) conclusions regarding AMS data quality to be inconsistent with our understanding of and findings on AMS performance. This inconsistency and the seriousness of the conclusions make it imperative to us to present a response providing a more balanced perspective to our atmospheric science colleagues inside and outside the AMS user community.
We present here a variety of evidence and reasoning demonstrating the inadequacy of the M16 model to represent important aspects of AMS performance. In the following, we use previously published results to address key issues raised in Murphy (Citation2016) associated with basic AMS features (vaporization temperature, single-particle timescales, and ionization efficiencies), AMS calibration (relative ionization efficiency), and evaluation of possible biases on organic aerosol field data. Rather than being an exhaustive review, the evidence put forth in this letter is representative of the AMS performance experienced by the large and varied AMS users' community. We conclude that the generalizations and assertions based on the M16 model are not consistent with many experimental AMS results and, hence, this model cannot be used to draw conclusions about the sensitivity of the AMS as a function of MW and the accuracy of ambient AMS measurements.
1. Evidence that the M16 model is inconsistent with AMS measurements
1.1. The M16 model is not consistent with measured AMS vaporizer temperature dependences
AMS detection is based on impaction of the particles on a heated vaporizer (typically operating at a temperature, Tv, of 600°C) under vacuum followed by vaporization, electron ionization of the resulting gas-phase molecules, and mass spectrometric detection of the ions (Jayne et al. Citation2000; Jimenez et al. Citation2003; Canagaratna et al. Citation2007). Murphy (Citation2016) proposes that the intensity of the ion signal (I) produced from an individual chemical species vaporizing from an aerosol particle in the AMS should follow the following equation (under a steady state assumption):[1] where C is a proportionality constant representing the efficiency of the analysis system, σ is the electron ionization cross section of the molecule,
is the vaporizing mass flux, A is the area of the holes in the ionizer through which molecules can escape to the pumping system, k is the Boltzmann constant, Tv is the absolute temperature of the AMS vaporizer, and MW is the molecular weight of the vaporizing molecules.
According to Equation (Equation1[1] ), the measured ion signal should follow a
dependence as the vaporizer temperature is varied. However, published evidence shows that this dependence is not observed. shows a direct comparison of AMS data from two different field studies (Docherty et al. Citation2015) with the expected dependence from the M16 model for nitrate, sulfate, and ammonium. Data are only shown for the Tv range when the species size distribution and instrument background data indicate that vaporization was as fast as at 600°C (Docherty et al. Citation2015), consistent with the temperature range and the timescales in Drewnick et al. (Citation2015) discussed later (). The signals of the three species stay constant or decrease slightly with decreasing Tv, in sharp contrast with the M16 model that predicts a signal increase of 20% over the range shown.
Figure 1. Examples of evidence that the M16 model does not capture important aspects of AMS detection. (a) Measured signals versus vaporizer temperature (relative to signals at Tv = 600°C) for ambient aerosols at Blodgett Forest, CA during BEARPEX-2007, and at an urban site in Riverside, CA during SOAR-1 in 2005 (Docherty et al. Citation2015). NO3, SO4, and NH4 refer to total nitrate, sulfate, and ammonium as quantified by the AMS by adding all species-related ion signals (Allan et al. Citation2004a; Canagaratna et al. Citation2007). Vaporizer temperature was automatically cycled every 30 min for multiple days across the range shown. Data are only shown for the Tv range where the species size distribution data indicate that vaporization was as fast as at Tv = 600°C (see Figure 3 of Docherty et al. Citation2015). These Tv ranges are consistent with the ranges for fast single-particle vaporization timescales shown in panels b and c of this figure. Relative variation of timescales of detection of (b) ammonium nitrate and (c) ammonium sulfate ion fragments from single particles versus Tv, compared to the Murphy (Citation2016) model predictions. Data from Drewnick et al. (Citation2015). The very long timescales at lower temperatures are due to slower vaporization. (d) Comparison of absolute timescales of single-particle detection for ammonium nitrate (Drewnick et al. Citation2015), compared with the Murphy (Citation2016) model results. Model results were interpolated from Figure 2 in Murphy's paper, assuming vaporization of NH4NO3 (MW = 80) as suggested by Murphy (Citation2016), and for vaporization of HNO3 thermal decomposition products such as NO2 (MW = 46) (Drewnick et al. Citation2015). Digitization was also not simulated in the model and may account for approximately 6 μs in the Drewnick et al. (Citation2015) results, which is much smaller than the observed model-measurement differences. (e) Timescales of single-particle vaporization for ammonium nitrate and (f) ammonium sulfate fragments vs. m/z, for the data of Drewnick et al. (Citation2015), and the Murphy (Citation2016) model using the intact salt MWs. Data points are sized so the area of each point is proportional to the fraction of the total signal at that ion. (g) Measured ammonium concentration during the SOAR-1 study compared to that predicted for cation/anion balance from the measured anions. Small corrections have been applied based on estimated organonitrate, organosulfate, and amine concentrations. See Figure 12 of Docherty et al. (Citation2011) and associated discussion for further details. (h) Ratio of measured to predicted ammonium versus the ambient nitrate to sulfate mass ratio in the same study.
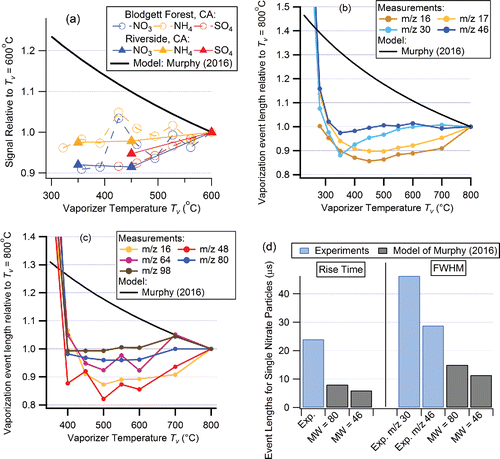
Experimental single-particle vaporization event lengths (Drewnick et al. Citation2015) also show a very different dependence on temperature than the predicted by the model (). Thus, through c indicate that other Tv-independent effects not accounted for in the M16 model must play a major role in the conversion of aerosol mass to ion signal in the AMS detection process. In particular, it appears that the vaporization process, and not the distribution of molecular speeds at Tv, controls the observed single-particle timescales.
One possible explanation of the discrepancy between the modeled and measured temperature dependence of the ion signal is that the effective translational temperature of a species after vaporization (Teff) differs from Tv. These results suggest that Teff < Tv and that Teff is not proportional to Tv over very wide ranges of Tv. Murphy (Citation2016) uses 500°C as the vapor temperature in the model to compare with data taken with Tv = 420°C. The use of (approximately) Tv in the model is justified based on limited evidence from a different instrument (Sykes et al. Citation2002), and is supported by the model result that many vaporizing molecules will experience one collision with the vaporizer before ionization (Murphy Citation2016). However, the data in Sykes et al. (Citation2002) do not conclusively demonstrate that the molecules reached Tv in their instrument, as their measured pulses were longer than the modeled ones, and their model was tuned to the observations. In addition, more than one collision is needed to thermalize gas molecules to a surface temperature (Lahaye et al. Citation1995; Butler et al. Citation1997). Since boiling point increases with MW, it is possible that Teff also increases with MW and this effect partially compensates the free molecular flow effect. The question arises whether quantifying and incorporating a species-dependent Teff into the M16 model could result in better agreement with the observations. However, a Teff < −200°C would be needed to explain the high ionization efficiencies for NH4 and H2O observed in the AMS and discussed later.
1.2. The M16 model is not consistent with measured single-particle timescales
The M16 model assumes that the single-particle vaporization pulse lengths measured with the AMS are solely determined by a species-independent particle vaporization time (4 μs in the model) and the time spent by vaporized molecules in the ionization region, assuming a Boltzmann velocity distribution at Tv. The only use of AMS data to validate the M16 model in Murphy (Citation2016) is a comparison of calculated single-particle pulse length with measured single-particle pulse length from the experiments of Jayne et al. (Citation2000). As reported by Jayne et al., the geometry of their vaporizer was a closed-tube furnace approximately 1 cm long with a 0.3 cm ID opening. Note that this geometry is not the same as the inverted cone vaporizer, which has been incorporated into all AMS instruments since 2000 and was used for the M16 model. The Jayne et al. (Citation2000) ionizer was also smaller than the one in all current AMS instruments. These differences in geometry resulted in significantly shorter pulses measured in the Jayne et al. (Citation2000) experiments compared to those from Drewnick et al. (Citation2015). Hence, the timing and duration of single-particle pulses from Jayne et al. (Citation2000) cannot be used to validate the M16 model.
Single-particle ammonium nitrate vaporization pulse lengths for the inverted-cone vaporizer from the Drewnick et al. (Citation2015) experiments are compared directly with those calculated using the M16 model in , showing the measured pulses are at least twice as long as those predicted by the model, and three to four times in the case of m/z 30. In addition, the measured ion pulse at m/z 30 is substantially longer than that at m/z 46, which is inconsistent with both the predicted MW dependence in M16 (which predicts the opposite trend) as well as the proposed ionization of intact ammonium nitrate molecules (which predicts equal values, as described later in the article). This indicates that additional processes (e.g., vaporization at lower effective temperatures, vaporization and decomposition over times longer than 4 μs, adsorption and subsequent desorption onto and from ionizer surfaces, and/or other effects) have a significant influence on the measured particle vaporization timescales, none of which are included in the M16 model.
1.3. There is a large discrepancy between the observed sensitivity to ammonium and water and the M16 model predictions
1.3.1. Ammonium ionization efficiency
The M16 model predicts an AMS ionization efficiency for ammonia (relative to nitrate, RIENH3) of ∼0.75 compared to values of 3.5–6.2 that are observed for ammonium in the AMS (Salcedo et al. Citation2006; Canagaratna et al. Citation2007). In an attempt to explain this large discrepancy, Murphy (Citation2016) proposes that ammonium nitrate and ammonium sulfate vaporize as molecular salt species, as follows:
where (p) and (g) indicate particle and gas-phase species, respectively, and a, b, c, and d indicate fractional yields in the respective reactions. Subsequent fragmentation of the ions shown would not change the ratios of total ions observed from each species. Mixed fragments (e.g., containing parts of both the NH3 and H2SO4 molecules) are not observed in AMS mass spectra (Hogrefe et al. Citation2004a). In the M16 model, a vaporized molecular salt [e.g., NH4NO3(g)] would have a specific distribution of velocities in the gas phase, leading to the same detection timescales for different ion fragments of each pure salt (e.g., NHx+ and NOx+).
compares the measured single particle pulse widths for ammonium nitrate (sulfate)-related ions from Drewnick et al. (Citation2015) with the results from the M16 model. Most apparent are the longer pulse widths for NO+ (m/z 30), SO+ (m/z 48), and SO2+ (m/z 64) than for the other ions. The main ammonium and anion fragments produced from each salt do not have the same pulse widths, confirming that the ion fragments observed for ammonium nitrate and ammonium sulfate do not arise from complete neutral salt molecules reaching the ionization region. Rather, other processes such as ammonium nitrate decomposing into NH3(g) and HNO3(g) (and potentially other molecules such as NO and NO2) at the vaporizer surface must be occurring in the AMS that causes the observed fragments to have different pulse lengths arising from separate decomposition products.
The robust fragmentation patterns for AMS ammonium detection (Allan et al. Citation2004a) are independent of the type of ammonium salt and very similar to the NIST EI spectrum for NH3(g) (NIST, Citation2016). Moreover, AMS measurements consistently show that the sensitivity for ammonium from ammonium nitrate is the same as from ammonium sulfate. For example, field data such as those shown in show that the average ratio of the measured-to-predicted (based on ion balance) ammonium is constant within 5%, despite variations on the ambient sulfate/nitrate ratio of over a factor of 20 as well as substantial changes in the concentrations of other internally mixed species (Docherty et al. Citation2011). This would be very unlikely if the salts vaporized as molecular species. It is implausible that the result of the intact salt vaporization processes for pure and mixed salts would always produce the same ionization efficiency and fragmentation pattern for ammonium, independent of wide variations in mixture composition. These arguments strongly support the vaporization of NH3(g) from the sampled ammonium salts.
Given the strong evidence for vaporization of NH3(g) from ammonium nitrate and sulfate, not molecular salt vaporization, the large discrepancy with M16 model predictions of RIENH4 and single particle vaporization times indicate a major incompleteness in the representation of the AMS vaporization/ionization processes in the M16 model.
1.3.1.1. Sensitivity to ammonium in ammonium oxalate
In Murphy (Citation2016), the understanding of AMS RIENH4 is questioned based on a report of lower than expected signal for ammonium from ammonium oxalate (AmOx) by Takegawa et al. (Citation2007). These authors suggested that one of several possible interpretations of their results was that RIENH4 from AmOx was one third of the RIENH4 for NH4NO3, while noting that “the RIENH4 issue remains uncertain and requires additional laboratory experiments in future studies.” Mensah et al. (Citation2011) performed similar laboratory experiments and report the same RIENH4 from AmOx and from NH4NO3, in contrast with the Takegawa et al. results. We note that the Mensah et al. (Citation2011) results cannot be explained if RIENH4 from ammonium oxalate was lower than for ammonium nitrate, as it is implausible that their particles contained an amount of ammonium three times larger than required to neutralize oxalic acid. One possible explanation for the low ammonium signal in the Takegawa et al. experiments is preferential evaporation of NH3(g) from the ammonium oxalate particles, likely in the aerosol sample lines or size classifiers. Indeed, thermodynamic model calculations using the extended aerosol inorganics model (E-AIM; Clegg and Seinfeld Citation2006a,Citationb; Clegg et al. Citation2016) show that the saturation concentration of NH3(g) over a solution of ammonium oxalate is of the order of 40,000 μg m−3, while that of oxalic acid is negligible (∼1 ng m−3), creating conditions that would favor preferential evaporation of NH3(g) from AmOx. In contrast, the estimated vapor pressures of NH3(g) and HNO3(g) over NH4NO3 are between these values, which lead to evaporation of both NH3(g) and HNO3(g) in that case. Evaporation of NH4NO3 in laboratory setups has been reported (Zelenyuk et al. Citation2006) although it is not common during AMS calibrations (Canagaratna et al. Citation2007). The differences in estimated vapor pressures indicate that preferential evaporation of NH3(g) is likely to be much more important for AmOx. Evaporation of AmOx in laboratory setups has been observed using size measurements (M. Petters, pers. comm., 2016). We thus hypothesize that the different experimental conditions in the two AmOx studies led to differences in NH3(g) evaporation and thus detected ammonium. Further experimental study of this system is recommended, but taken together, these results suggest that the low ammonium signal in the Takegawa et al. experiments is most likely unrelated to RIENH4.
1.3.1.2. Potential conversion of ammonium to NO+ on the AMS vaporizer
The possibility of ammonium being converted to NO+ on the AMS vaporizer is discussed in Murphy (Citation2016). While not explicitly stated in the table of sulfate ion fragments, early experiments of ammonium sulfate particles did not show evidence of conversion of ammonium to NO+ (Hogrefe et al. Citation2004a). Ammonium sulfate calibrations on several instruments in our groups show an NO+ signal of ∼1/2000 of the ammonium signal. This is likely due to cross contamination from ammonium nitrate because of alternating use of the same atomizer. Thus, we conclude that conversion of ammonium to NO+ is unlikely to play a role for AMS detection.
1.3.2. Water ionization efficiency
There is consensus on a high relative sensitivity to water from particles as they are sampled by the AMS (Matthew et al. Citation2008; Engelhart et al. Citation2011; Mensah et al., Citation2011). The first two studies did not directly report RIE for particulate water due to evaporation in the instrument. Mensah et al. (Citation2011) used dried particles with known hydrates to estimate RIEH2O = 2. Engelhart et al. (Citation2011) reported that the product of RIEH2O and the evaporated fraction of water in their system was close to unity during their ambient air study, and their data can be used to estimate RIEH2O. There is substantial evaporation of water from ambient particles upon entering the AMS aerodynamic lens system, as supported from typical evaporation rates of water under ambient conditions (∼150 monolayers in ∼15 ms residence time in the aerodynamic lens). Zelenyuk et al. (Citation2006) studied ammonium sulfate particles (similar in composition to those in Engelhart et al.) and estimated that ∼80% of the particle water evaporated after sampling. Even larger fractional evaporation has been published for ammonium sulfate by Matthew et al. (Citation2008). Using an estimated 80% loss of water for the Engelhart results, we estimate RIEH2O ∼5, similar to the values observed for RIENH4. Thus, the range of RIEH2O estimated from experiments is 2–5, in strong disagreement with the value of 0.60 predicted by the M16 model.
2. Quantification of organic aerosol mass
2.1. Calibration of relative ionization efficiencies
In Murphy (Citation2016), the following inaccurate and misleading statement is made about quantification of organic aerosol (OA) mass by the AMS: “… the calibration for ammonium nitrate is transferred to organic species using approximate electron ionization cross section (Canagaratna et al., Citation2007).” The connection between AMS sensitivities and electron ionization (EI) cross sections was originally made in Jimenez et al. (Citation2003) in order to highlight that (a) the ion signals are approximately proportional to mass instead of to molar concentrations, providing the basis for mass being the primary data product of the AMS; and (b) sensitivities are of the same order for different species, so that differences of several orders-of-magnitude as seen in other techniques (e.g., single-particle laser ablation or chemical-ionization mass spectrometry) are not expected. However, Jimenez et al. (Citation2003) included a species-specific response factor (later specified as CEs × RIEs) in the quantification equation to account for differences in ionization efficiency and other effects, and stated the need for calibration. In the standard AMS analysis, no aerosol species (including OA) is quantified based on relative EI cross sections.
AMS quantification of the mass concentration of a species s within a multicomponent aerosol particle is based on the following equation (Alfarra et al. Citation2004a; Canagaratna et al. Citation2007):[2] where Cs is the mass concentration of species s, MWNO3 is the molecular weight of nitrate, CEs is the collection efficiency of species s, RIEs is the relative ionization efficiency of species s, IENO3 is the ionization efficiency of nitrate (from calibrations), Q is the volume flow rate into the AMS, NA is Avogadro's number, Is,i is the ion signal from ion i produced by species s, and the 1012 factor accounts for unit conversions. We note that almost all ambient and laboratory experiments, including the calibrations discussed here, are carried out with a standard Tv ∼ 550–600°C.
Equation (Equation2[2] ) relies on a calibrated relative ionization efficiency (RIEs) and not on a single fundamental molecular property (such as the electron ionization cross section). The ionization efficiency of a species (IEs) has an expected linear MW dependence since electron ionization cross sections increase approximately linearly with MW (Canagaratna et al. Citation2007):
[3]
Murphy (Citation2016) argues that there is an additional secondary dependence on for each component species that is not taken into account explicitly in Equation (Equation3
[3] ) and, based exclusively on theoretical arguments, asserts that substantial errors arise from its omission. While we agree with an expected theoretical dependence of RIEs on the time spent by the vaporized molecules within the ionization region, we assert that this dependence (as well as other effects not explicitly included in Equations (Equation2
[2] ) and (Equation3
[3] )) is implicitly included in the experimentally determined RIEs.
Since ambient OA consists of thousands of different molecules, an average RIEOA must necessarily be used to calculate OA mass concentrations. As stated in Canagaratna et al. (Citation2007), the average RIEOA of 1.4 that is recommended for calculating ambient OA mass concentrations was obtained from laboratory calibrations. shows the data used to derive this value. RIEOA is obtained from the slope of the linear regression of ionization efficiency (IE) versus MW for a calibration dataset obtained from a variety of commercially available, oxygenated organic compounds analogous to those observed in ambient aerosol particles (Zhang et al. Citation2007). The relatively large error bars in reflect the difficulties in accounting for factors, such as particle bounce, multiply charged particles, particle shape factors, and particle volatilization during these calibrations. Although has not been previously published in the peer-reviewed literature, it has been presented at major conferences in the United States and Europe (e.g., Silva et al. Citation2001; Jayne et al. Citation2002; Worsnop et al. Citation2002, Citation2004Citationa,Citationb) and has been publicly available for over a decade at the University of Colorado website archive of AMS Users' Meetings presentations (Worsnop Citation2007).
Figure 2. Calibration data for different species in the AMS. (a) Calibration data used to derive RIEOA = 1.4 for ambient organic aerosol mass calculations (Canagaratna et al. Citation2007). The calibration dataset includes several types of organic species including methanesulfonic acid, malonic acid, maleic acid, succinic acid, glutaric acid, malic acid, nitrophenol, levoglucosan, phthalic acid, and azelaic acid (P. Silva, Aerodyne Research and Boston College, unpublished work). Particles were generated by atomization of aqueous or organic solutions of the species, size selected with a differential mobility analyzer and counted with a condensation particle counter. (b) Scatter plot of measured RIEs vs. species MW for the organic species in Figure 2a as well as published RIEs values for hydrocarbons (lubricating oil, decane, and 4 PAHs) from Slowik et al. (Citation2004) and Dzepina et al. (Citation2007), and unpublished values for diesel, oleic acid and hexadecanol (P. Silva, Aerodyne Research and Boston College). Values for inorganic species are also shown. The horizontal (green) line and light gray region denote RIEOA = 1.4 with 2σ uncertainty of 20%. The region between the dashed green lines shows the range of estimated dependence for intact species resistant to thermal decomposition in Murphy (Citation2016). Note that PAHs and lubricating oil would be expected to lie in between these lines according to the M16 model. “EB to 6.2” (error bar) is added to mark that RIENH4 up to 6.2 are often measured (Salcedo et al. Citation2006; Canagaratna et al. Citation2007).
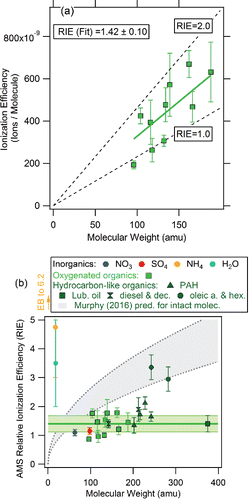
The overwhelming majority of the signal from OA in ambient aerosol is detected at m/z < 100 even in urban areas (e.g., > 90%; DeCarlo et al. Citation2008). This is due to both thermal decomposition during/after vaporization and fragmentation after ionization with 70 eV electrons. Previous work utilizing AMS systems coupled to “soft” ionization sources indicates that an important cause for the dominance of low m/z ions in AMS spectra is thermal decomposition of organic species (both oxidized and hydrocarbon-like) prior to ionization (Northway et al. Citation2007; Kroll et al. Citation2009; Robinson et al. Citation2011; Canagaratna et al. Citation2015). Thus, for most organic molecules, the actual MW of the molecules being vaporized and travelling through the ionization region is smaller than the parent MW due to thermal decomposition on the AMS vaporizer (Canagaratna et al. Citation2015). Any additional MW-dependence of IE beyond that shown in is expected to be further reduced by thermal decomposition during vaporization.
shows published RIEs values (in addition to those in ) measured for organic and inorganic species as a function of MW. The organic data points in the figure are colored depending on whether they are more likely to thermally decompose (light green) or to vaporize intact or with limited thermal decomposition (dark green). The gray dashed lines show the dependence of RIE predicted for thermally stable intact molecules using the M16 model (see of Murphy [Citation2016]). In Murphy (Citation2016) thermal decomposition was also included as a potentially important process and decomposing molecules were shown to have RIEs in the region below the lower dashed line. The possibility of formulating an RIEs parameterization with an explicit
dependence was originally considered with respect to high AMS RIE values () measured for organic species, such as oleic acid (MW 282) and hexadecanol (MW 242). However, as shown in measured RIE values of several other hydrocarbon species that are expected to vaporize with limited thermal decomposition do not follow an obvious
dependence. Lubricating oil, a high MW species (average MW of ∼375; Worton et al. Citation2014) that is representative of ambient primary organic aerosol (POA) from traffic emissions (Canagaratna et al. Citation2004) and is expected to be stable with respect to thermal decomposition, has an RIE value of 1.4 (Slowik et al. Citation2004) compared to the ∼4 predicted using the M16 model. Similarly, PAHs, which are good test cases for
dependence of RIE since they tend to survive vaporization intact in the AMS (Dzepina et al. Citation2007), have measured RIE values of ∼1.7 (for PAHs for MW 202–248; Slowik et al. Citation2004; Dzepina et al. Citation2007) instead of ∼3.2 as predicted by the M16 model. Finally, RIE values of some inorganic species deviate strongly from the M16 model prediction, as discussed earlier. Taken together, there is significant variability in measured organic and inorganic RIEs, but it cannot be explained with a simple
term.
RIEOA from the linear regression slope in is 1.42, while the average of all organic RIEs in is ∼1.6 (13% higher). A 2σ uncertainty of 20% for RIEOA was estimated previously (Bahreini et al. Citation2009), consistent with this variability. The complexity of ambient multicomponent OA mixtures will lead to significant averaging and hence it is unlikely that using an average RIEOA value for ambient AMS analysis will generate large biases in OA concentration. Note that some of the higher RIEs values shown in belong to species that may not be highly representative of ambient OA constituents. For example, PAHs are known to make insignificant contributions to ambient OA mass (Dzepina et al. Citation2007). Ambient OA is dominated by contributions from oxidized organic species similar to those shown in , and hydrocarbon-like organic species such as lubricating oil (Canagaratna et al. Citation2004; Zhang et al. Citation2007). The applicability of a single RIEOA = 1.4 for ambient aerosols over a range of conditions (including with higher POA) is discussed further in Section 2.2.
For the record, the data referenced in Murphy (Citation2016) from Alfarra's doctoral thesis work (Citation2004b) is not included in because the experiments were primarily performed for the purpose of exploring qualitative trends in detection of organics as a function of vaporizer temperature and not for absolute mass quantification. The absolute accuracy of the ions-per-particle values, needed to derive a quantitative trend with MW, is highly uncertain in this specific instance, as certain aspects of the experiment, such as how particle collection efficiency and multiply charged particles were accounted for, were not optimal at that early stage of AMS development.
It is important to note that RIEOA = 1.4 is not recommended for laboratory data (or when studying a specific OA source), other than to obtain an estimate of the mass concentration. It has been known since the first environmental chamber experiments using an AMS that there is significant variability of collection efficiency (CE) for laboratory organic particles (Bahreini et al. Citation2005; Docherty et al. Citation2013; Ye et al. Citation2016). There is also real variability in RIE for different species () that is more important for laboratory experiments due to the use of single species or simpler mixtures. For these reasons, measurements of the response factor (RIE × CE) relevant to the OA type under investigation should be obtained empirically, for example by comparison with aerosol concentrations from Scanning Mobility Particle Sizer or other measurements (Li et al. Citation2013; Docherty et al. Citation2013). Alternatively, in lieu of determining specific RIE × CE values for various types of laboratory OA, some AMS users have chosen to use ratios such as OA/SO4 to track relative changes in OA abundance, for example, in chamber experiments (Hildebrandt et al. Citation2009; Wong et al. Citation2015).
2.2. Accuracy of ambient OA mass concentration quantification with AMS
In Murphy (Citation2016), it is claimed that the AMS sensitivity to OA may vary by more than a factor of 3, depending on the fraction of species thermally decomposing at the AMS vaporizer and those being thermally stable, and that, as a consequence, AMS OA mass concentration measurements may have several-fold biases. We dispute these claims as they are made with no compelling evidence while previously published work has already shown evidence to the contrary. OA uncertainty for ambient measurements was estimated to be significantly smaller (±38%) with the largest source of uncertainty being attributed to collection efficiency (Bahreini et al. Citation2009; Middlebrook et al. Citation2012). There are many published intercomparisons involving high quality measurements of ambient aerosols that show that the AMS OA mass concentrations are consistent with independent measurements within the stated uncertainty (e.g., Takegawa et al. Citation2005; DeCarlo et al. Citation2008; Timonen et al. Citation2010; Docherty et al. Citation2011; Middlebrook et al. Citation2012; Setyan et al. Citation2012; Washenfelder et al. Citation2015).
An example of evaluating the accuracy of AMS mass concentration measurements and how it might vary as different types of aerosols are encountered is shown in . The ratio of total aerosol mass concentration from the sum of AMS species mass concentrations and black carbon mass concentration (not detected by the AMS) to the total mass concentration determined by independent measurements of the accumulation mode (Mfine) was found to distribute around a value of unity for two airborne campaigns, during which OA comprised about one half of the fine aerosol mass. The distributions of measured ratio values are not wider than the distributions expected based on the combined uncertainties of the measurements and, hence, support the current operational AMS uncertainty estimates. For , the observed distribution is narrower than expected, suggesting that actual AMS uncertainties are lower than estimated for that case.
Figure 3. Examples of evidence that the OA concentrations reported by the AMS are correct within the stated uncertainties. (a) Ratio of the AMS total mass (using the standard RIEOA) plus black carbon mass to the mass calculated from the fine particle volume for airborne campaigns over Alaska with aged biomass burning influence and (b) over Texas with aged urban and biogenic influences, with the combined experimental uncertainties depicted as dotted lines (adapted from Middlebrook et al. Citation2012). OA comprised 43% and 47% of total submicron nonrefractory mass in these campaigns, respectively. (c) Values of the OA hygroscopicity parameter (Petters and Kreidenweis Citation2007) needed for hygroscopicity closure in the OA-dominated southeastern United States and a forest site in the Rocky Mountains under different assumptions. Black: values expected for pure laboratory SOA from multiple precursors for the O/C measured in the ambient studies (Lambe et al. Citation2011). Gray: values reported in the literature assuming the AMS composition is correct (Brock et al. Citation2016; Cerully et al. Citation2015; Levin et al. Citation2014). Other colors: values needed to explain the measured hygroscopicity if AMS OA was overestimated by factors of 2 (orange), 3 (red), and +38% (green; 2σ upper limit of the OA accuracy stated in the literature; Bahreini et al. Citation2009). (d) Ratio of OC measurements from the AMS and a Sunset Laboratories OC instrument vs. the fraction of primary OC (POC) in total OC, for a campaign in Riverside, CA (Docherty et al. Citation2011). Also shown is the combined instrumental uncertainty (gray area, ±38% for AMS OA, ±15% for Sunset OC) and two lines representing assumed primary OA detection twice and three times more sensitively than SOA, as suggested by Murphy (Citation2016).
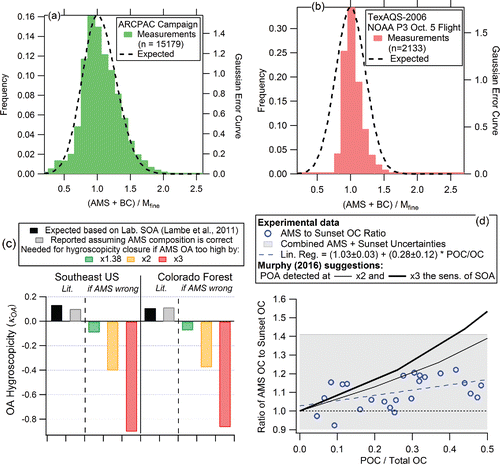
Constraints on the accuracy of AMS ambient OA mass concentration measurements can also be obtained from aerosol hygroscopicity data, where comparisons between AMS organic concentrations and growth factors under subsaturated conditions have long been found to be consistent (Gysel et al. Citation2007). Values of the hygroscopicity parameter (κOA, Petters and Kreidenweis Citation2007) obtained from laboratory and field studies for oxidized OA are ∼0.05–0.3 (Gunthe et al. Citation2009; Lambe et al. Citation2011; Brock et al. Citation2016; Cheung et al. Citation2015; Petters et al. Citation2016). Values of κOA needed for hygroscopicity closure and assuming that AMS OA mass concentrations were erroneously high have been estimated with the method described in the appendix. shows that unphysical negative values of κOA would be necessary to explain the bulk hygroscopicity if OA mass was overestimated by a factor of 2–3. In fact, negative values of κOA are needed if OA is overestimated by just +38%, the upper limit of the reported AMS accuracy, much less than a factor of 2–3, while reasonable κOA are obtained for the actually reported OA mass concentrations. Combined with the volume closure as, for example, shown in , this further demonstrates that the biases in OA mass concentrations suggested in Murphy (Citation2016) are inconsistent with independent measurements. We note that some of the κOA measurements and submicron aerosol volume measurements used here as well as the assumption that the latter are correct, were performed in publications by Murphy and coworkers (Froyd et al. Citation2010; Liao et al. Citation2015; Brock et al. Citation2016). When only those two previous results and assumptions are combined with the Murphy (Citation2016) suggestion of a large overestimate of OA, a strong inconsistency emerges as illustrated in .
Ambient AMS data also do not support the assumption of a several-fold bias on the quantification of POA (consisting of more thermally stable molecules) relative to secondary organic aerosol (SOA; consisting of less thermally stable molecules), as suggested in Murphy (Citation2016). For example, Docherty et al. (Citation2011) evaluated the possible difference in AMS sensitivity to primary and secondary organics. Their data replotted in show that although the data are always in agreement within the combined instrumental uncertainties, it is possible that the response factor for POA (=RIEPOA × CEPOA) might be larger than that for SOA as already published by Docherty et al. (Citation2011). The possibility of using a different response factor for POA compared to SOA has been under active discussion in the AMS community for many years in the context of reducing uncertainties in both CE and RIE. Jimenez et al. (Citation2003) showed that EI cross sections (molecular properties, unrelated to the MW effect suggested in Murphy [2016]) of hydrocarbon molecules are about 50% higher than those of O and N containing organics. However, as shown earlier, the ratios of AMS response factors for two species can be different from ratios of their EI cross sections. CE factors may also differ between SOA and POA. Different response factors have not been widely adopted in standard AMS analyses of ambient OA as laboratory calibrations () do not show consistent evidence for them, and as intercomparisons such as in (which encompass further effects such as CE) typically do not show large deviations beyond the estimated uncertainties as POA fractions vary. Work is in progress to better characterize the AMS response factor for different types of POA, but as indicated by the example in , ambient data are not consistent with changes in response factors that would result in biases in the range of 100%–200% and larger as implied in Murphy (Citation2016).
In summary, we find no evidence of very large systematic biases in AMS ambient OA mass concentrations contrary to what is suggested in Murphy (Citation2016). If biases in ambient OA mass concentration measurements were as large as a factor of 2–3, they would have been identified over a decade ago through comparisons, such as those just discussed. We note that systematic biases in sulfate and nitrate mass quantification of about a factor of 2 were identified in the very first intercomparisons of AMS with other instruments, and ultimately led to CE quantification (Drewnick et al. Citation2003, Hogrefe et al. Citation2004b; Allan et al. Citation2004b; Quinn et al. Citation2006; Matthew et al. Citation2008; Middlebrook et al. Citation2012).
Conclusions
While the M16 model correctly reflects certain physical features of some processes in the AMS, it is unable to predict multiple observed dependencies and other detailed features of AMS measurements. This is likely because it is too simple and it does not include important processes within the AMS. Furthermore, there is ample evidence from ambient analyses that AMS reported uncertainties are not underestimated, and large systematic biases in OA mass determination are generally not observed, contrary to what is predicted by the M16 model. Thus, the M16 model is not suitable to predict or evaluate the uncertainty or other performance metrics of AMS instruments used for ambient OA analysis, or to conclude that salts vaporize as complete molecules in the AMS.
Given the complexity of the sampling, vaporization and ionization processes in the AMS, the AMS community decided early on to base mass quantification on empirical calibrations rather than explicit modeling of these processes. Empirical calibrations underlie the quantification of the vast majority of atmospheric chemistry instruments (and also of broader analytical chemistry instruments), and the chemical composition of atmospheric aerosols is difficult to measure accurately by many methods. In the vast majority of ambient field measurements where the AMS was properly calibrated, operated without problems, and analyzed using standard procedures, the results have been overwhelmingly consistent with other colocated measurements of high quality. This, we believe, provides confidence in the robustness of the methods developed by the AMS community over the past 15 years.
We agree with Murphy (Citation2016) that additional experimental and theoretical characterization studies are important for a fuller understanding of the physical processes in the AMS. The M16 model that describes free molecular flow within the ion source would have to be a component of any future larger model that successfully represents the full complexity of aerosol analysis by the AMS. We believe that a more important effect in practice is the uncertainty in the collection efficiency due to particle bounce, rather than the effect described in Murphy (Citation2016). Work is ongoing in our laboratories, including the development and characterization of a novel capture vaporizer to address particle bounce, as well as studies of the detection of organic compounds in the AMS. Finally, we reiterate the need for direct calibration in laboratory experiments utilizing specific organic compounds or mixtures.
Acknowledgments
The authors are grateful to Simon Clegg for his support on running and interpreting the output of the E-AIM model, and to Markus Petters and Chuck Brock for useful discussions on constraints on composition from hygroscopicity measurements. The authors thank Kenneth Docherty, Philip Silva, Pedro Campuzano-Jost, Douglas Day, and Weiwei Hu for useful comments and discussions. The authors thank the many Aerodyne scientists, particularly in the Center for Aerosol & Cloud Chemistry (CACC), who have contributed to the development of the AMS and this work. The authors also thank the AMS community of users for their input on this topic as well as for many past and ongoing contributions to the development, characterization, and application of the different AMS instruments.
Funding
The authors thank NASA, EPA, and NSF, for supporting the collection and analysis of some of the data used here. JLJ acknowledges support from a College Scholar Award from the University of Colorado.
References
- Alfarra, M. R., Coe, H., Allan, J. D., Bower, K. N., Boudries, H., Canagaratna, M. R., Jimenez, J. L., Jayne, J. T., Garforth, A., Li, S.-M., and Worsnop, D. R. (2004a). Characterization of Urban and Rural Organic Particulate in the Lower Fraser Valley using two Aerodyne aerosol Mass Spectrometers. Atmos. Environ., 38:5745–5758.
- Alfarra, R. (2004b). Insights Into Atmospheric Organic Aerosols Using An Aerosol Mass Spectrometer. Ph.D. Thesis at the University of Manchester.
- Allan, J. D., Bower, K. N., Coe, H., Boudries, H., Jayne, J. T., Canagaratna, M. R., Millet, D. B., Goldstein, A. H., Quinn, P. K., Weber, R. J., and Worsnop, D. R. (2004b). Submicron Aerosol Composition at Trinidad Head, CA During ITCT 2K2, its Relationship with Gas Phase Volatile Organic Carbon and Assessment of Instrument Performance. J. Geophys. Res., 109:D23S24, doi:10.1029/2003JD004208.
- Allan, J. D., Delia, A. E., Coe, H., Bower, K. N., Alfarra, M. R., Jimenez, J. L., Middlebrook, A. M., Drewnick, F., Onasch, T. B., Canagaratna, M. R., Jayne, J. T., and Worsnop, D. R. (2004a). A Generalised Method for the Extraction of Chemically Resolved Mass Spectra from Aerodyne Aerosol Mass Spectrometer Data. J. Aerosol. Sci., 35:909–922. 10.1016/j.jaerosci.2004.02.007.
- Bahreini, R., Ervens, B., Middlebrook, A. M., Warneke, C., de Gouw, J. A., DeCarlo, P. F., Jimenez, J. L., Brock, C. A., Neuman, J. A., Ryerson, T. B., Stark, H., Atlas, E., Brioude, J., Fried, A., Holloway, J. S., Peischl, J., Richter, D., Walega, J., Weibring, P., Wollny, A. G., and Fehsenfeld, F. C. (2009). Organic Aerosol Formation in Urban and Industrial Plumes Near Houston and Dallas, Texas. J. Geophys. Res., 114:D00F16. 10.1029/2008JD011493.
- Bahreini, R., Keywood, M. D., Ng, N. L., Varutbangkul, V., Gao, S., Flagan, R. C., Seinfeld, J. H., Worsnop, D. R., and Jimenez, J. L. (2005). Measurements of Secondary Organic Aerosol (SOA) from Oxidation of Cycloalkenes, Terpenes, and m-Xylene Using an Aerodyne Aerosol Mass Spectrometer. Environ. Sci. Technol., 39:5674–5688, doi: 10.1021/es048061a.
- Brock, C. A., Wagner, N. L., Anderson, B. E., Attwood, A. R., Beyersdorf, A., Campuzano-Jost, P., Carlton, A. G., Day, D. A., Diskin, G. S., Gordon, T. D., Jimenez, J. L., Lack, D. A., Liao, J., Markovic, M. Z., Middlebrook, A. M., Ng, N. L., Perring, A. E., Richardson, M. S., Schwarz, J. P., Washenfelder, R. A., Welti, A., Xu, L., Ziemba, L. D., and Murphy, D. M. (2016). Aerosol Optical Properties in the Southeastern United States in Summer-Part 1: Hygroscopic Growth. Atmos. Chem. Phys., 16:4987–5007, doi:10.5194/acp-4987-2016.
- Butler, D. A., Berenbak, B., Stolte, S., and Kleyn, A. W. (1997). Elastic Scattering in a Reactive Environment: NO on Ru(0001)-(1×1)H. Phys. Rev. Lett., 78:4653–4656.
- Canagaratna, M. R., Jayne, J. T., Ghertner, D. A., Herndon, S., Shi, Q., Jimenez, J. L., Silva, P. J., Williams, P., Lanni, T., Drewnick, F., Demerjian, K. L., Kolb, C. E., and Worsnop, D. R. (2004). Chase Studies of Particulate Emissions from in-use New York City Vehicles. Aerosol Sci. Technol., 38:555–573.
- Canagaratna, M. R., Jayne, J. T., Jimenez, J. L., Allan, J. D., Alfarra, M. R., Zhang, Q., Onasch, T. B., Drewnick, F., Coe, H., Middlebrook, A., Delia, A., Williams, L. R., Trimborn, A. M., Northway, M. J., DeCarlo, P. F., Kolb, C. E., Davidovits, P., and Worsnop, D. R. (2007). Chemical and Microphysical Characterization of Ambient Aerosols with the Aerodyne Aerosol Mass Spectrometer. Mass Spectrometry Reviews, 26:185–222.
- Canagaratna, M. R., Jimenez, J. L., Kroll, J. H., Chen, Q., Kessler, S. H., Massoli, P., Hildebrandt Ruiz, L., Fortner, E., Williams, L. R., Wilson, K. R., Surratt, J. D., Donahue, N. M., Jayne, J. T., and Worsnop, D. R. (2015). Elemental Ratio Measurements of Organic Compounds Using Aerosol Mass Spectrometry: Characterization, Improved Calibration, and Implications. Atmos. Chem. Phys., 15:253–272, doi:10.5194/acp-15-253-2015.
- Cerully, K. M., Bougiatioti, A., Hite, Jr., J. R., Guo, H., Xu, L., Ng, N. L., Weber, R., and Nenes, A. (2015). On the Link Between Hygroscopicity, Volatility, and Oxidation State of Ambient and Water Soluble Aerosols in the Southeastern United States. Atmos. Chem. Phys., 15:8679–8694, doi:10.5194/acp-15-8679-2015.
- Cheung, H. H. Y., Yeung, M. C., Li, Y. J., Lee, B. P., and Chan, C. K. (2015). Relative Humidity-Dependent HTDMA Measurements of Ambient Aerosols at the HKUST Supersite in Hong Kong, China. Aerosol Sci. Technol., 49:643–654, doi:10.1080/02786826.2015.1058482.
- Clegg, S. L., Brimblecombe, P., and Wexler, A. S. (2016). Extended AIM Aerosol Thermodynamics Model Website. http://www.aim.env.uea.ac.uk/aim/aim.php, accessed 22-Jan-2016.
- Clegg, S. L., and Seinfeld, J. H. (2006a). Thermodynamic Models of Aqueous Solutions Containing Inorganic Electrolytes and Dicarboxylic Acids at 298.15 K. I. The Acids as Non-Dissociating Components. J. Phys. Chem. A, 110:5692–5717.
- Clegg, S. L., and Seinfeld, J. H. (2006b). Thermodynamic Models of Aqueous Solutions Containing Inorganic Electrolytes and Dicarboxylic Acids at 298.15 K. II. Systems Including Dissociation Equilibria. J. Phys. Chem. A, 110:5718–5734.
- DeCarlo, P. F., Dunlea, E. J., Kimmel, J. R., Aiken, A. C., Sueper, D., Crounse, J., Wennberg, P. O., Emmons, L., Shinozuka, Y., Clarke, A., Zhou, J., Tomlinson, J., Collins, D. R., Knapp, D., Weinheimer, A. J., Montzka, D. D., Campos, T., and Jimenez, J. L. (2008). Fast Airborne Aerosol Size and Chemistry Measurements Above Mexico City and Central Mexico During the MILAGRO Campaign. Atmos. Chem. Phys., 8:4027–4048, doi:10.5194/acp-8-4027-2008.
- Docherty, K. S., Aiken, A. C., Huffman, J. A., Ulbrich, I. M., DeCarlo, P. F., Sueper, D., Worsnop, D. R., Snyder, D. C., Peltier, R. E., Weber, R. J., Grover, B. D., Eatough, D. J., Williams, B. J., Goldstein, A. H., Ziemann, P. J., and Jimenez, J. L. (2011). The 2005 Study of Organic Aerosols at Riverside (SOAR-1): Instrumental Intercomparisons and Fine Particle Composition. Atmos. Chem. Phys., 11:12387–12420, doi:10.5194/acp-11-12387-2011.
- Docherty, K. S., Jaoui, M., Corse, E., Jimenez, J. L., Offenberg, J. H., Lewandowski, M., and Kleindienst, T. E. (2013). Collection Efficiency of the Aerosol Mass Spectrometer for Chamber-Generated Secondary Organic Aerosols. Aerosol Sci. Technol., 47:294–309, doi:10.1080/02786826.2012.752572.
- Docherty, K. S., Lewandowski, M., and Jimenez, J. L. (2015). Effect of Vaporizer Temperature on Ambient Non-Refractory Submicron Aerosol Composition and Mass Spectra Measured by the Aerosol Mass Spectrometer. Aerosol Sci. Technol., 49:485–494, doi:10.1080/02786826.2015.1042100.
- Drewnick, F., Diesch, J.-M., Faber, P., and Borrmann, S. (2015). Aerosol Mass Spectrometry: Particle-Vaporizer Interactions and Their Consequences for the Measurements. Atmos. Meas. Tech., 8:3811–3830, doi:10.5194/amt-8-3811-2015.
- Drewnick, F., Schwab, J. J., Hogrefe, O., Peters, S., Husain, L., Diamond, D., Weber, R., and Demerjian, K. L. (2003). Intercomparison and Evaluation of Four Semi-Continuous PM2.5 Sulfate Instruments. Atm. Env., 37:3335–3350.
- Dzepina, K., Arey, J., Marr, L. C., Worsnop, D. R., Salcedo, D., Zhang, Q., Onasch, T. B., Molina, L. T., Molina, M. J., and Jimenez, J. L. (2007). Detection of Particle-Phase Polycyclic Aromatic Hydrocarbons in Mexico City using an Aerosol Mass Spectrometer. Int. J. Mass Spec., 263:152–170.
- Engelhart, G. J., Hildebrandt, L., Kostenidou, E., Mihalopoulos, N., Donahue, N. M., and Pandis, S. N. (2011). Water Content of Aged Aerosol. Atmos. Chem. Phys., 11:911–920, doi:10.5194/acp-11-911-2011.
- Froyd, K. D., Murphy, S. M., Murphy, D. M., de Gouw, J. A., Eddingsaas, N. C., and Wennberg, P. O. (2010). Contribution of Isoprene-Derived Organosulfates to Free Tropospheric Aerosol Mass. Proc. Nat. Acad. Sci. USA, 107:21360–21365, doi:10.1073/pnas.1012561107.
- Fry, J. L., Draper, D. C., Zarzana, K. J., Campuzano-Jost, P., Day, D. A., Jimenez, J. L., Brown, S. S., Cohen, R. C., Kaser, L., Hansel, A., Cappellin, L., Karl, T., Hodzic Roux, A., Turnipseed, A., Cantrell, C., Lefer, B. L., and Grossberg, N. (2013). Observations of Gas-and Aerosol-Phase Organic Nitrates at BEACHON-RoMBAS 2011. Atmos. Chem. Phys., 13:8585–8605, doi:10.5194/acp-13-8585-2013.
- Gunthe, S. S., King, S. M., Rose, D., Chen, Q., Roldin, P., Farmer, D. K., Jimenez, J. L., Artaxo, P., Andreae, M. O., Martin, S. T., and Pöschl, U. (2009). Cloud Condensation Nuclei in Pristine Tropical Tainforest air of Amazonia: Size-Resolved Measurements and Modeling of Atmospheric Aerosol Composition and CCN Activity. Atmos. Chem. Phys., 9:7551–7575, doi:10.5194/acp-9-7551-2009.
- Gysel, M., Crosier, J., Topping, D. O., Whitehead, J. D., Bower, K. N., Cubison, M. J., Williams, P. I., Flynn, M. J., McFiggans, G. B., and Coe, H. (2007). Closure Study Between Chemical Composition and Hygroscopic Growth of Aerosol Particles During TORCH2. Atmos. Chem. Phys., 7:6131–6144.
- Hildebrandt, L., Donahue, N. M., and Pandis, S. N. (2009). High Formation of Secondary Organic Aerosol from the Photo-Oxidation of Toluene. Atmos. Chem. Phys., 9:2973–2986, doi:10.5194/acp-9-2973-2009.
- Hogrefe, O., Drewnick, F., Lala, G. G., Schwab, J. J., and Demerjian, K. L. (2004a). Development, Operation and Applications of an Aerosol Generation, Calibration and Research Facility. Aerosol Sci. Technol., 38:196–214.
- Hogrefe, O., Schwab, J. J., Drewnick, F., Lala, G. G., Peters, S., Demerjian, K. L., Rhoads, K., Felton, H. D., Rattigan, O. V., Husain, L., and Dutkiewicz, V. A. (2004b). Semicontinuous PM2.5 Sulfate and Nitrate Measurements at an Urban and a Rural Location in New York: PMTACS-NY Summer 2001 and 2002 Campaigns. J. Air & Waste Manage. Assoc., 54:1040–1060.
- Jayne, J. T., Canagaratna, M. R., Boudries, H., Jimenez, J. L., Worsnop, D. R., Allan, J., Alfarra, R., Williams, P., Bower, K., and Coe, H. (2002). Atmospheric Aerosol Chemistry Measured with an Aerosol Mass Spectrometer. 223rd American Chemical Society National Meeting, Environmental Award Symposium for Roger Atkinson, Paper ENVI-111, Orlando, FL, April 2002.
- Jayne, J. T., Leard, D. C., Zhang, X., Davidovits, P., Smith, K. A., Kolb, C. E., and Worsnop, D. R. (2000). Development of an Aerosol Mass Spectrometer for Size and Composition Analysis of Submicron Particles. Aerosol Sci. Technol., 33:49–70, 10.1080/027868200410840.
- Jimenez, J. L., Jayne, J. T., Shi, Q., Kolb, C. E., Worsnop, D. R., Yourshaw, I., Seinfeld, J. H., Flagan, R. C., Zhang, X., Smith, K. A., Morris, J., and Davidovits, P. (2003). Ambient Aerosol Sampling Using the Aerodyne Aerosol Mass Spectrometer. J. Geophys. Res., 108:8425, doi:10.1029/2001JD001213.
- Kroll, J. H., Smith, J. D., Che, D. L., Kessler, S. H., Worsnop, D. R., and Wilson, K. R. (2009). Measurement of Fragmentation and Functionalization Pathways in the Heterogeneous Oxidation of Oxidized Organic Aerosol. Phys. Chem. Chem. Phys, 11:8005–8014, doi: 10.1039/b905289e.
- Kuwata, M., Zorn, S. R., and Martin, S. T. (2012). Using Elemental Ratios to Predict the Density of Organic Material Composed of Carbon, Hydrogen, and Oxygen. Environ. Sci. Technol., 46(2):787–794, doi:10.1021/es202525q.
- Lahaye, R. J. W. E., Kleyn, A. W., Stolte, S., and Holloway, S. (1995). The Scattering of Ar from Ag(111): A Molecular Dynamics Study. Surface Science, 338:169–182.
- Lambe, A. T., Onasch, T. B., Massoli, P., Croasdale, D. R., Wright, J. P., Ahern, A. T., Williams, L. R., Worsnop, D. R., Brune, W. H., and Davidovits, P. (2011). Laboratory Studies of the Chemical Composition and Cloud Condensation Nuclei (CCN) Activity of Secondary Organic Aerosol (SOA) and Oxidized Primary Organic Aerosol (OPOA). Atmos. Chem. Phys., 11:8913–8928, doi:10.5194/acp-11-8913-2011.
- Levin, E. J. T., Prenni, A. J., Palm, B. B., Day, D. A., Campuzano-Jost, P., Winkler, P. M., Kreidenweis, S. M., DeMott, P. J., Jimenez, J. L., and Smith, J. N. (2014). Size-Resolved Aerosol Composition and Its Link to Hygroscopicity at a Forested Site in Colorado. Atmos. Chem. Phys., 14:2657–2667, doi:10.5194/acp-14-2657-2014.
- Li, R., Palm, B. B., Borbon, A., Graus, M., Warneke, C., Ortega, A. M., Day, D. A., Brune, W. H., Jimenez, J. L., and de Gouw, J. A. (2013). Laboratory Studies on Secondary Organic Aerosol Formation from Crude Oil Vapors. Environ. Sci. Technol., 47:12566–12574, doi:10.1021/es402265y.
- Liao, J., Froyd, K. D., Murphy, D. M., Keutsch, F. N., Yu, G., Wennberg, P. O., Clair, J. M.St., Crounse, J. D., Wisthaler, A., Mikoviny, T., Jimenez, J. L., Campuzano-Jost, P., Day, D. A., Hu, W., Ryerson, T. B., Pollack, I. B., Peischl, J., Anderson, B. E., Ziemba, L. D., Blake, D. R., Meinardi, S., and Diskin, G. (2015). Airborne Measurements of Organosulfates Over the Continental U.S.. J. Geophys. Res. Atmos., 120:2990–3005, doi: 10.1002/2014JD022378.
- Lide, D. R. (1991). CRC Handbook of Chemistry and Physics. CRC Press, USA.
- Matthew, B. M., Middlebrook, A. M., and Onasch, T. B. (2008). Collection efficiencies in an Aerodyne aerosol mass spectrometer as a function of particle phase for laboratory generated aerosols. Aerosol Sci. Technol., 42:884–898, doi: 10.1080/02786820802356797.
- Mei, F., Hayes, P. L., Ortega, A., Taylor, J. W., Allan, J. D., Gilman, J., Kuster, W., de Gouw, J., Jimenez, J. L., and Wang, J. (2013). Droplet Activation Properties of Organic Aerosols Observed at an Urban site During CalNex-LA. J. Geophys. Res. Atmos., 118:2903–2917, doi:10.1002/jgrd.50285.
- Mensah, A. A., Buchholz, A., Mentel, T., Tillmann, R., and Kiendler-Scharr, A. (2011). Aerosol Mass Spectrometric Measurements of Stable Crystal Hydrates of Oxalates and Inferred Relative Ionization Efficiency of Water. J. Aerosol Sci., 42:11–19, doi:10.1016/j.jaerosci.2010.10.003.
- Middlebrook, A. M., Bahreini, R., Jimenez, J. L., and Canagaratna, M. R. (2012). Evaluation of Composition-Dependent Collection Efficiencies for the Aerodyne Aerosol Mass Spectrometer using Field Data. Aerosol Sci. Technol., 46:258–271.doi:10.1080/02786826.2011.620041.
- Murphy, D. M. (2016). The Effects of Molecular Weight and Thermal Decomposition on the Sensitivity of a Thermal Desorption Aerosol Mass Spectrometer. Aerosol Sci. Technol., 50:118–125. doi: 10.1080/02786826.2015.1136403.
- NIST Chemistry WebBook. (2016). http://webbook.nist.gov/chemistry/ accessed 15-Jan-2016.
- Northway, M. J., Jayne, J. T., Toohey, D. W., Canagaratna, M. R., Trimborn, A., Akiyama, K.-I., Shimono, A., Jimenez, J. L., DeCarlo, P. F., Wilson, K. R., and Worsnop, D. R. (2007). Demonstration of a VUV Lamp Photoionization Source for Improved Organic Speciation in an Aerosol Mass Spectrometer. Aerosol Sci. Technol., 41:829–839.
- Petters, M. D., and Kreidenweis, S. M. (2007). A Single Parameter Representation of Hygroscopic Growth and Cloud Condensation Nucleus Activity. Atmos. Chem. Phys., 7:1961–1971, doi:10.5194/acp-7-1961-2007.
- Petters, M. D., Kreidenweis, S. M., and Ziemann, P. J. (2016). Prediction of Cloud Condensation Nuclei Activity for Organic Compounds Using Functional Group Contribution Methods. Geosci. Model Dev., 9:111–124, doi:10.5194/gmd-9-111-2016.
- Quinn, P. K., Bates, T. S., Coffman, D., Onasch, T. B., Worsnop, D., Baynard, T., de Gouw, J. A., Goldan, P. D., Kuster, W. C., Williams, E., Roberts, J. M., Lerner, B., Stohl, A., Pettersson, A., and Lovejoy, E. R. (2006). Impacts of sources and aging on submicrometer aerosol properties in the marine boundary layer across the Gulf of Maine. J. Geophys. Res., 111:D23S36, 10.1029/2006JD007582.
- Robinson, C. B., Kimmel, J. R., David, D. E., Jayne, J. T., Trimborn, A., Worsnop, D. R., and Jimenez, J. L. (2011). Thermal Desorption Metastable Atom Bombardment Ionization Aerosol Mass Spectrometer. Int. J. Mass Spec., 303:164–172, doi:10.1016/j.ijms.2011.01.027.
- Salcedo, D., Onasch, T. B., Dzepina, K., Canagaratna, M. R., Zhang, Q., Huffman, J. A., DeCarlo, P. F., Jayne, J. T., Mortimer, P., Worsnop, D. R., Kolb, C. E., Johnson, K. S., Zuberi, B., Marr, L. C., Volkamer, R., Molina, L. T., Molina, M. J., Cardenas, B., Bernabé, R. M., Márquez, C., Gaffney, J. S., Marley, N. A., Laskin, A., Shutthanandan, V., Xie, Y., Brune, W., Lesher, R., Shirley, T., and Jimenez, J. L. (2006). Characterization of Ambient Aerosols in Mexico City During the MCMA-2003 Campaign with Aerosol Mass Spectrometry: Results from the CENICA Supersite. Atmos. Chem. Phys., 6:925–946, doi:10.5194/acp-6-925-2006.
- Setyan, A., Zhang, Q., Merkel, M., Knighton, W. B., Sun, Y. L., Song, C., Shilling, J. E., Onasch, T. B., Herndon, S. C., Worsnop, D. R., Fast, J. D., Zaveri, R. A., Berg, L. K., Wiedensohler, A., Flowers, B. A., Dubey, M. K., and Subramanian, R. (2012). Characterization of Submicron Particles Influenced by Mixed Biogenic and Anthropogenic Emissions Using High-Resolution Aerosol Mass Spectrometry: Results from CARES. Atmos. Chem. Phys., 12:8131–8156, doi:10.5194/acp-12-8131-2012.
- Silva, P. J., Davidovits, P., Jayne, J. T., Canagaratna, M. R., Worsnop, D. R., and Kolb, C. E. (2001). Quantitative Analysis of Submicron Aerosol Particles Using a Real-Time Aerosol Mass Spectrometer. Pittcon Conference, New Orleans, LA.
- Slowik, J. G., Stainken, K., Davidovits, P., Williams, L. R., Jayne, J. T., Kolb, C. E., Worsnop, D. R., Rudich, Y., DeCarlo, P., and Jimenez, J. L. (2004). Particle Morphology and Density Characterization by Combined Mobility and Aerodynamic Diameter Measurements. Part 2: Application to Combustion Generated Soot Particles as a Function of Fuel Equivalence Ratio. Aerosol Sci. Technol., 38:1206–1222, doi:10.1080/027868290903916.
- Sykes, D. C., Woods, III, E., Smith, G. D., Baer, T., and Miller, R. E. (2002). Thermal Vaporization-Vacuum Ultraviolet Laser Ionization Time-of-Flight Mass Spectrometry of Single Aerosol Particles. Anal. Chem., 74:2048–2952.
- Takegawa, N., Miyakawa, T., Kawamura, K., and Kondo, Y. (2007). Contribution of Selected Dicarboxylic and ω-Oxocarboxylic acids in Ambient Aerosol to the m/z 44 Signal of an Aerodyne Aerosol Mass Spectrometer. Aerosol Sci. Technol., 41:418–437.
- Takegawa, N., Miyazaki, Y., Kondo, Y., Komazaki, Y., Miyakawa, T., Jimenez, J. L., Jayne, J. T., Worsnop, D. R., Allan, J., and Weber, R. J. (2005). Characterization of an Aerodyne Aerosol Mass Spectrometer (AMS): Intercomparison with other Aerosol Instruments. Aerosol Sci. Technol., 39:760–770.
- Timonen, H., Aurela, M., Carbone, S., Saarnio, K., Saarikoski, S., Mäkelä, T., Kulmala, M., Kerminen, V.-M., Worsnop, D. R., and Hillamo, R. (2010). High Time-Resolution Chemical Characterization of the Water-Soluble Fraction of Ambient Aerosols with PILS-TOC-IC and AMS. Atmos. Meas. Tech., 3:1063–1074, doi:10.5194/amt-3-1063-2010.
- Washenfelder, R. A., Attwood, A. R., Brock, C. A., Guo, H., Xu, L., Weber, R. J., Ng, N. L., Allen, H. M., Ayres, B. R., Baumann, K., Cohen, R. C., Draper, D. C., Duffey, K. C., Edgerton, E., Fry, J. L., Hu, W. W., Jimenez, J. L., Palm, B. B., Romer, P., Stone, E. A., Wooldridge, P. J., and Brown, S. S. (2015). Biomass Burning Dominates Brown Carbon Absorption in the Rural Southeastern United States. Geophys. Res. Lett., 42:653–664, doi:10.1002/2014GL062444.
- Wong, J. P. S., Zhou, S., and Abbatt, J. P. D. (2015). Changes in Secondary Organic Aerosol Composition and Mass due to Photolysis: Relative Humidity Dependence. J. Phys. Chem. A, 119:4309–4316, doi:10.1021/jp506898c.
- Worsnop, D. R. (2007). AMS Users Meeting presentations in 2003, 2005. http://cires1.colorado.edu/jimenez-group/UsrMtgs/Doug_Boulder_March03.pdf, http://cires1.colorado.edu/jimenez-group/UsrMtgs/UsersMtg4/AMSusersIntro_Worsnop.pdf, http://cires1.colorado.edu/jimenez-group/UsrMtgs/UsersMtg8/DRW.intro.AMSuser.pdf (accessed 1 February 2016).
- Worsnop, D. R., Jayne, J. T., Canagaratna, M. R., Boudries, H., Onasch, T., Mortimer, P., Williams, L., Jimenez, J., Zhang, Q., Coe, H., Allan, J., and Alfarra, R. (2004a). Field Applications of an Aerosol Mass Spectrometer: What are we Learning about Aerosol Organics? Geophys. Res. Abstracts, 6:07659 SRef-ID: 1607-7962/gra/EGU04-A-07659.
- Worsnop, D. R., Jayne, J. T., Canagaratna, M. R., Boudries, H., Onasch, T., Mortimer, P., Williams, L., Jimenez, J., Zhang, Q., Coe, H., Allan, J., Alfarra, R., Slowik, J., and Davidovits, P. (2004b). Field Applications of an Aerosol Mass Spectrometer: What are we Learning about Atmospheric Aerosol Chemical and Microphysical Properties? Proceedings of the 52nd ASMS Conference on Mass Spectrometry and Allied Topics, Nashville, Tennessee, May 2004b.
- Worsnop, D., Jayne, J., Canagaratna, M. R., Boudries, H., Kolb, C., Jimenez, J. L. (2002). Aerosol Chemical and Microphysics Measured With an Aerosol Mass Spectrometer. Eos Trans. AGU, 83(47): Fall Meet. Suppl., Abstract A11F-02.
- Worton, D. R., Isaacman, G., Gentner, D. R., Dallmann, T. R., Chan, A. W. H., Ruehl, C., Kirchstetter, T. W., Wilson, K. R., Harley, R. A., and Goldstein, A. H. (2014). Lubricating Oil Dominates Primary Organic Aerosol Emissions from Motor Vehicles. Environ. Sci. Technol., 48:3698–3706, doi:10.1021/es405375j.
- Ye, P., Ding, X., Hakala, J., Hofbauer, V., Robinson, E. S., and Donahue, N. M. (2016). Vapor Wall Loss of Semi-Volatile Organic Compounds in Teflon Chamber. Aerosol Sci. Technol., 50(8):822–834.
- Zelenyuk, A., Imre, D., and Cuadra-Rodriguez, L. (2006). Evaporation of Water from Particles in the Aerodynamic Lens inlet: An Experimental Study. Anal Chem., 78:6942–6947.
- Zhang, Q., Jimenez, J. L., Canagaratna, M. R., Allan, J. D., Coe, H., Ulbrich, I., Alfarra, M. R., Takami, A., Middlebrook, A. M., Sun, Y. L., Dzepina, K., Dunlea, E., Docherty, K., DeCarlo, P. F., Salcedo, D., Onasch, T., Jayne, J. T., Miyoshi, T., Shimono, A., Hatakeyama, S., Takegawa, N., Kondo, Y., Schneider, J., Drewnick, F., Borrmann, S., Weimer, S., Demerjian, K., Williams, P., Bower, K., Bahreini, R., Cottrell, L., Griffin, R. J., Rautiainen, J., Sun, J. Y., Zhang, Y. M., and Worsnop, D. R. (2007). Ubiquity and Dominance of Oxygenated Species in Organic Aerosols in Anthropogenically—Influenced Northern Hemisphere Mid-latitudes. Geophys. Res. Lett., 34:L13801, doi:10.1029/2007GL029979.
Appendix
Estimation of κOA values that provide hygroscopicity closure if OA was overestimated
The hygroscopicity parameter for particles containing an internal mixture of different chemical species can be estimated with the Zdanovskii, Stokes, and Robinson (ZSR) volume-mixing rule (Petters and Kreidenweis Citation2007):[A1] where ϵi is the volume fraction of species i in the dry particles, and κi is the hygroscopicity parameter for species i. For submicron particles dominated by ammonium-sulfate salts (AS) and OA, Equation (EquationA1
[A1] ) can be written explicitly as:
[A2]
Since κAS is well known (∼0.6; Petters and Kreidenweis Citation2007), and measurements of the mass fractions of AS and OA are available from, for example, AMS measurements, Equation (EquationA2[A2] ) can be solved to allow the estimation of κOA as:
[A3]
In practice, these estimates are reliable only if the OA mass fraction is large (see, e.g., Figure 5 of Mei et al. Citation2013, and associated discussion). The volume mass fractions can be estimated from composition data as:[A4] where fi and ρi are the mass fraction and density of component i, respectively. We use a density of 1.78 g cm−3 for ammonium-sulfate salts (Lide Citation1991), and we estimate the OA density from the parameterization of Kuwata et al. (Citation2012).
While multiple published results could be used as examples, we chose recently published studies in two different regions of the United States. κOA was estimated with this method using AMS data in three studies with high OA mass fractions in the Southeast US during Summer 2013 from aircraft and ground platforms, as part of the SOAS, SENEX, and SEAC4RS field studies. Cerully et al. (Citation2015) report κOA ∼0.15 for the cloud activation regime, while Brock et al. (Citation2016) report κOA ∼0.05 for the subsaturated regime, and we use the average of 0.10 here. The same method was applied to data from a forested site in Colorado during the summer of 2011, yielding κOA ∼0.13 for the BEACHON-RoMBAS field study (Levin et al. Citation2014). Submicron nitrate concentrations were very small and submicron chloride was negligible at both locations (Fry et al. Citation2013; Brock et al. Citation2016).
Here we recalculated κOA using Equation (EquationA3[A3] ) and reducing the OA concentration reported from the AMS by factors of 2 and 3, both of which are quoted by Murphy (Citation2016) as possible overestimation factors. We assume that the measured hygroscopicity is correct, as well as that the volume concentration reported by collocated instruments is correct as routinely done in many papers including those by Murphy's group (Froyd et al. Citation2010; Liao et al. Citation2015), and thus any decrease in the OA mass concentration has to be compensated by an error of equal magnitude and opposite sign in the ammonium-sulfate salt concentration.
Results for these calculations are shown in and discussed in the main article text.