ABSTRACT
Correlations between O/C and cloud condensation nucleus activity, represented by κ, are a computationally efficient approach to estimate the impact of aerosol aging on cloud formation and climate; however, previously reported correlations between these two variables are empirical and vary widely in their slopes and extrapolations to high O/C values. This study proposes a theoretical framework that bridges elemental ratios, volatility, solubility, and κ. The framework estimates intrinsic κ based on molecular formulas of organics composed of carbon, oxygen, and hydrogen that partition to condensed phase, and then it estimates apparent κ considering solubility distribution parameterized by O/C. This article applied the new framework to the two-dimensional volatility basis set (2D-VBS) and found that distribution of O/C and molecular size play key roles in determining apparent κ. For highly soluble organics, κ is dictated by gas-particle partitioning and it is unlikely for κ of organics to go beyond 0.3 in typical ambient organic material loadings. Sensitivity analysis showed that surface-active compounds are not likely to profoundly alter the overall trend within a reasonable range of surface activity. This framework provides a simple yet plausible explanation of why κ would and would not correlate with O/C; distributions of OA in the 2D-VBS as well as the presence of inorganic salts determine the trend. The framework opens up new opportunities to evaluate two-dimensional representations of organic aerosol aging using κ, a significant advancement from the current empirical linear fits to κ and O/C.
Copyright © 2017 American Association for Aerosol Research
EDITOR:
1. Introduction
Organic aerosols (OA) are metastable intermediates in the atmosphere whose physical and chemical properties continuously evolve during its lifetime (Donahue et al. Citation2013).
The ability of OA to serve as cloud condensation nuclei (CCN) is of interest in understanding OA lifetime in the atmosphere and its impact on the cloud microphysics (McFiggans et al. Citation2006; Andreae and Rosenfeld Citation2008). The effect of oxidative processing, or aging, of OA on CCN activity has been a subject of debate in recent years. Some studies have reported that the single hygroscopicity parameter κ of OA linearly increases with O/C (Jimenez et al. Citation2009; Massoli et al. Citation2010; Lambe et al. Citation2011; Wong et al. Citation2011; Mei et al. Citation2013a,Citationb); other studies have found only weak or non-existent correlations (Chang et al. Citation2010; Frosch et al. Citation2011; Alfarra et al. Citation2013; Lathem et al. Citation2013; Rickards et al. Citation2013; Suda et al. Citation2014).
It is not surprising that O/C fails to fully capture variability in κ. Clearly, O/C can have an impact on κ through solubility (Kuwata et al. Citation2013), i.e., oxidized organics tend to be water soluble, and hence contribute to the solute effects in Köhler theory (Shulman et al. Citation1996; Pruppacher and Klett Citation1997; Petters and Kreidenweis Citation2008; Suda et al. Citation2014). Recently, Riipinen et al. (Citation2015) reported that the solubility distribution of organics in the range of 0.1–100 g L−1 is critical for capturing the CCN activation. However, O/C or solubility has no information on molecular size that can be a controlling factor in cloud droplet activation of water soluble molecules (Padro et al. Citation2007; Asa-Awuku et al. Citation2008; Petters et al. Citation2009).
Furthermore, surface-active compounds may impact CCN activity although its significant remains uncertain. A number of studies concluded that surface-active compounds enhance apparent CCN activity by lowering the surface tension of growing droplets (Shulman et al. Citation1996; Facchini et al. Citation1999; Ruehl et al. Citation2012; Giordano et al. Citation2013). Other studies suggested that the effects of surface-activity might be smaller than previously thought, and in some case negligible, due to canceling effects of surface tension depression and bulk solute depletion by partitioning to the droplet surface (Prisle et al. Citation2010; Topping Citation2010; Raatikainen and Laaksonen Citation2011; Petters and Kreidenweis Citation2013; Raatikainen and Laaksonen Citation2014; Lowe et al. Citation2016). An emerging alternative view is that cloud droplet activation occurs at the point where individual molecules of a surface organic monolayer begin to separate, i.e., the point of a two-dimensional phase transition between compressed and gaseous surface states (Ruehl et al. Citation2016). This study focuses on the conventional bulk-surface partitioning (Sorjamaa et al. Citation2004; Topping Citation2010; Raatikainen and Laaksonen Citation2011) as the initial step of the model development.
This study aims at answering a simple question: Why would (and would not) apparent CCN activity linearly change with O/C? Although a number of qualitative explanations exist, and in some aspects quantitative models are available (e.g., solubility, volatility, surface activity), a plausible explanation that accounts for the interplay of various parameters as a whole is yet to be presented. This article presents a new framework that couples both solubility effects and molecular size effects in a manner readily applicable to existing modeling approaches of OA aging. The key assumptions are that (1) the CCN activity of sufficiently soluble compounds can be estimated by Raoult's law (Rosenørn et al. Citation2006; Suda et al. Citation2014), (2) solubility can be parameterized by O/C (Kuwata et al. Citation2013), (3) therefore O/C distributions can be converted into solubility distributions (Riipinen et al. Citation2015), and (4) O/C and molecular size determine volatility of organic compounds (Donahue et al. Citation2011; Daumit et al. Citation2013). The uncertainties of key assumptions and surface activity are evaluated through Monte Carlo simulations. The new framework reasonably reproduces previously observed trends between κ and extent of oxidation, and thus provides new opportunities to evaluate models of OA aging in terms of κ.
2. Model description
The first step of the model development is to formulate the relationship between volatility, O/C, and intrinsic values of CCN activity assuming entirely soluble solutes. The next step is to estimate apparent values of CCN activity considering solubility distribution parameterized by O/C. Finally, Monte Carlo simulations evaluate the impact of a reasonable range of surface activity on apparent CCN activity. The online supplementary information (SI) include MATLAB codes that implement the algorithms discussed below.
2.1. Estimation of intrinsic CCN activity using molecular formulas
CCN activity of individual water-soluble species can be estimated based on dissociation, non-ideality of solution, molecular weight, and density (Rose et al. Citation2008; Kreidenweis et al. Citation2009; Petters et al. Citation2009):[1] where ν is the stoichiometric dissociation number for the solute, Φ is the molal or practical osmotic coefficient that accounts for solution non-idealities, ρs is the density of solute (ρorg when the solute is organic), ρw is the density of water, Ms is the molecular weight of solute (Morg when the solute is organic), and Mw is the molecular weight of water. κ of organic materials is denoted by κorg. νΦ is approximately equivalent to the van't Hoff factor (Pruppacher and Klett Citation1997; Kreidenweis et al. Citation2005). The list of symbols is shown in the SI (Table S1).
Experimentally, κ can be determined by measuring the diameter of dry particles that activate into cloud droplets at known critical saturation ratio (Sc) (Petters and Kreidenweis Citation2007):[2] where Dd is the dry particle diameter, Sc is the critical saturation ratio, σs/a is the surface tension of the solution/air interface, R is the universal gas constant, and T is the temperature. The values of κ are conventionally reported by assuming the dilute solution surface tension is close to that of water, σs/a = σw = 0.072 J m−2 (Petters and Kreidenweis Citation2007). The effects of surface-active compounds are discussed in detail in Section 2.4. The value of κ determined by Sc and Dd assuming σs/a = σw is denoted as κapp, to clarify the difference from Equation (Equation1
[1] ) based on solute properties and solution non-ideality.
For an ideal solution of non-dissociating organics, νΦ equals to 1, i.e., Raoult's law holds. The applicability of the assumption to atmospheric OA is uncertain. Rosenørn et al. (Citation2006) reported that cloud droplet activation of saccharides and levoglucosan could be estimated assuming a van't Hoff factor of 1. Since oxidized OA may be significantly comprised of carboxylic acids, dissociation of acid is possible. Frosch et al. (Citation2010) suggests that the van't Hoff factor for monovalent acids with pKa > 4 is close to 1 at the critical droplet conditions (assuming Morg = 150 g mol−1, ρorg = 1.5 g cm−3, Dd = 30–100 nm; their Figure 8). If νΦ can be assumed to be 1, the remaining unknown in Equation (Equation1[1] ) is ρs for a given molecular formula.
Assuming that OA is only comprised of carbon, hydrogen, and oxygen, the density of atmospherically relevant organic materials, ρorg (g cm−3), can be estimated from O/C and H/C (Kuwata et al. Citation2012):[3]
According to Kuwata et al. (Citation2012), Equation (Equation3[3] ) has an accuracy of 12% for more than 90% of atmospherically relevant compounds used in their training set as well as biogenic secondary OA (SOA). Nakao et al. (Citation2013) confirmed the accuracy based on an extensive dataset of SOA produced from biogenic hydrocarbons, aromatic hydrocarbons, and phenolic compounds.
From EquationEquations (1[1] ) and (Equation3
[3] ), κorg can be estimated as follows:
[4] where nC is the number of carbon. νΦ is assumed to be one (ideal, no dissociation). As is evident from Equation (Equation4
[4] ), κorg can be estimated from a molecular formula (CxHyOz) assuming water-soluble organics and ideal solutions, which is a reasonable approximation for cloud droplet activation (dilute solution) of highly oxidized (water-soluble) organic aerosol. Although organic nitrates (-ONO2) may be significant at high NOx conditions, this study neglects them for simplicity. In order to include the effect of nitrates, the density parameterization by Kuwata et al. (Citation2012) needs to be updated to include N/C, and the low solubility of nitrates needs to be considered (Suda et al. Citation2014). Note that O/C in this article is only for organic carbon. It does not include inorganic carbons (e.g., CaCO3).
The accuracy of Equation (Equation4[4] ) is evaluated in . Model predictions are compared against observed κ of various atmospherically relevant compounds including carboxylic acids, polyols, and saccharides (Petters et al. Citation2016). For comparison, also includes the model prediction based on functional group contribution methods (Petters et al. Citation2016), as well as a simple treatment of solubility and surface activity discussed in Sections 2.3 and 2.4. Raoult's law overestimates apparent κ of low solubility compounds as expected. For highly soluble compounds, the model predictions by all the three models generally agree with observations within a factor of two. In other words, Raoult's law can predict intrinsic κ within a factor of two. The uncertainties in individual compounds are expected to cancel out to some extent when Equation (Equation4
[4] ) is applied to a mixture in the 2D-VBS framework (Donahue et al. Citation2011). The impact of the factor of two uncertainty in the intrinsic κ is evaluated via Monte Carlo simulations in Section 3.
Figure 1. Comparison of predicted vs. experimentally observed κorg. Petters et al. (Citation2016) (red circle) is based on a group contribution method (UNIFAC) that accounts for solution non-ideality. The entirely soluble case (blue triangle) assumes water-solubility, no dissociation (ν = 1), and ideal solution (Φ = 1) (Equation (Equation1[1] )). Density is estimated using Equation (Equation3
[3] ). The third case (yellow square) is based on estimated solubility (Equation (Equation10
[10] )) and surface activity where available (Section 2.4). The dotted lines correspond to two-fold differences. κ values less than 10−3 are shown as 10−3. Table S2 provides the individual compound names and corresponding values.
![Figure 1. Comparison of predicted vs. experimentally observed κorg. Petters et al. (Citation2016) (red circle) is based on a group contribution method (UNIFAC) that accounts for solution non-ideality. The entirely soluble case (blue triangle) assumes water-solubility, no dissociation (ν = 1), and ideal solution (Φ = 1) (Equation (Equation1[1] )). Density is estimated using Equation (Equation3[3] ). The third case (yellow square) is based on estimated solubility (Equation (Equation10[10] )) and surface activity where available (Section 2.4). The dotted lines correspond to two-fold differences. κ values less than 10−3 are shown as 10−3. Table S2 provides the individual compound names and corresponding values.](/cms/asset/a50048e8-dbef-4cbb-ab5c-68dd17f53e66/uast_a_1352082_f0001_oc.gif)
Equation (Equation4[4] ) can be further constrained by assuming (Heald et al. Citation2010; Donahue et al. Citation2011)
[5] which is based on van Krevelen analysis of a large set of field measurements. EquationEquations (4
[4] ) and (Equation5
[5] ) suggest that nc and O/C are sufficient for predicting intrinsic κ. Although direct measurement of nc is generally not available, previous studies estimated nc based on the relationship between volatility and assumed functionality (Donahue et al. Citation2011). Therefore, the next step is to consider the relationship between volatility, O/C, and nc of OA.
2.2. Effects of gas-particle partitioning on intrinsic CCN activity
This study builds upon recent work that established simplified estimates of volatility (i.e., vapor pressures) from average molecular structures (Pankow and Asher Citation2008; Donahue et al. Citation2011; Daumit et al. Citation2013). The first assumption is that oxygen exists either as carbonyl or hydroxyl groups:[6] where nO, nC, n-OH, and n=O are the number of oxygen, carbon, hydroxyl groups, and carbonyl groups, respectively. Donahue et al. (Citation2011) assume that there is an equal number of carbonyls and alcohols (n=O = n-OH) and that carboxylic acids can be treated as a combination of an alcohol and a carbonyl; this assumption is reasonably consistent with previous observations based on van Krevelen diagrams, with an approximate slope of −1 in H/C vs. O/C space (Heald et al. Citation2010). As a result, the relationship between volatilities, nC and O/C can be derived using the SIMPOL.1 group contribution method (Pankow and Asher Citation2008; Daumit et al. Citation2013):
[7] where Psat is the vapor pressure (atm), α is the conversion factor from Psat to C*, and the b terms are the group contribution terms representing the impact of each moiety: b0 is the zero order term, bC is the carbon number term, b=O is the ketone term, and b-OH is the hydroxyl term. The values of b0, bC, b=O, and b-OH at 293.15 K are 1.79, −0.438, −0.935, and −2.23, respectively. The unit conversion factor α is calculated by α = 106 (Morg)/RT, where Morg is the average molecular weight (assumed to be 200 g mol−1, the assumed value only has a minor effect), and R is the ideal gas constant (8.21 × 10−5 atm m3 mol−1 K−1) (Daumit et al. Citation2013). In Equation (Equation7
[7] ), the ideality of organics is assumed (Daumit et al. Citation2013), i.e., γ = 1 in C* = γCo, where C*, Co, and γ are volatility in mixture, volatility in pure species, and activity coefficient, respectively. The assumption is reasonable when the mixture is comprised of organics with similar values of O/C (Donahue et al. Citation2011). Therefore, caution must be taken when hydrocarbon-like OA and oxidized OA are internally mixed, in which case the activity coefficient must be estimated (Donahue et al. Citation2011). Using Equations (Equation4
[4] ), (Equation5
[5] ), and (Equation7
[7] ), intrinsic κ can be calculated for each bin in the 2D-VBS space as shown in Table S3 and (solid contour lines). Since intrinsic κ is the upper limit of κ, strongly suggest that κ of OA is unlikely to be over 0.3 even in the heavily polluted atmosphere with organic aerosol mass loading ∼103 μg m−3, where compounds with C* ∼ 103 μg m−3 or less partition to the condensed phase. The likely upper limit of κ ∼0.3 is consistent with Suda et al. (Citation2014) who quantified κ of numerous pure compounds. provides the first quantitative explanation that the upper limit of κ is a result of volatility and gas-particle partitioning. A possible exception is organic salts such as neutralization products of ammonia and dicarboxylic acids, which can lower vapor pressure of organics dramatically (Paciga et al. Citation2014).
Figure 2. Simulation results of α-pinene SOA without inorganic seed. The 2D-VBS distribution is taken from Donahue et al. (Citation2012). Ddry = 100 nm. (a) Distribution of organic aerosol volume in terms of O/C and logC*. The solid lines correspond to intrinsic κ. The dotted lines correspond to volume-based solubility C. (b) Köhler curves considering only solubility (black) and entire non-aqueous, aqueous, and surface partitioning (full partition). (c) Assumed/estimated surface tension. Solubility only case assumes surface tension of water. Full partition case assumes Γimax' = 1.45 × 10−6 mol m−2 and K'i = 3.5 × 102. (d) Estimated non-aqueous, aqueous, and surface phase partitioning. (e) Estimated non-aqueous and aqueous phase partitioning.
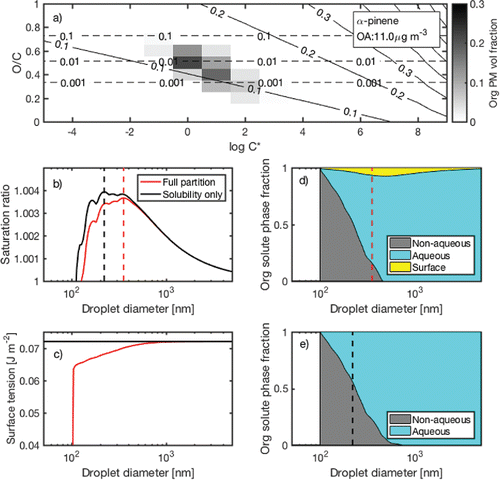
At this point, incorporation of intrinsic κ into the 2D-VBS is relatively straightforward since intrinsic κ of a mixture can be calculated by the simple mixing rule (Petters and Kreidenweis Citation2007):[8] where κIntr is the intrinsic κ, i and j are the index for O/C and C* in the 2D-VBS space, respectively, ϵi,j is the volume fraction of OA, and κi,j is the intrinsic κ of the corresponding bin (Table S3). Previous studies on the development of the volatility basis set (VBS) have discussed the procedure for calculating gas-particle partitioning in the framework (Donahue et al. Citation2006, Citation2011). Briefly, the gas-particle partitioning is described by the following partitioning coefficient ξi (Donahue et al. Citation2006):
[9]
Equation (Equation9[9] ) is solved iteratively to find the array of partition coefficients. The partition coefficients and the distribution of total organic material (gas + particle) in the 2D-VBS determine the mass concentrations of OA in each bin. Then, ϵi,j can be obtained by converting the mass fractions into volume fractions using the density parameterization (Equation (Equation3
[3] )).
Again, all the discussion so far is based on entirely soluble organics, which may be directly applied to highly oxidized OA such as those produced by aqueous reactions (aqSOA) (Ervens et al. Citation2011). For entirely soluble organics, previous studies have established that molecular size is the controlling parameter (Rosenørn et al. Citation2006; Petters et al. Citation2009). This study provides a new quantitative insight that the gas-particle partitioning determines the upper limit of κ for a given concentration of OA (). The next step is account for solubility limitation.
2.3. Effects of solubility distribution on apparent CCN activity
A recent modeling study by Riipinen et al. (Citation2015) concluded that the solubility distribution in the range of 0.1–100 g L−1 determines the CCN activation. Assuming a unit density (1 g cm−3), the mass-based solubility range is equivalent to the volume-based solubility range, C(v/v) = 10−4 ∼ 10−1. Kuwata et al. (Citation2013) proposed the following parameterization of C(v/v) by O/C:[10]
Based on Equation (Equation10[10] ), the solubility range corresponds to O/C range 0.2–0.7. The majority of OA except for freshly emitted hydrocarbon-like OA falls into this range (Jimenez et al. Citation2009). Therefore, it is crucial to consider the distribution of O/C, not just the average. By default, the 2D-VBS includes the O/C distribution, and hence the solubility distribution ( dotted lines; Table S3).
The effects of solubility on κ can be implemented in the 2D-VBS by applying the method described in Petters and Kreidenweis (Citation2008). Briefly, OA in the 2D-VBS is treated as a mixture of m × n components, where m and n are the number of O/C and C* bins, respectively. Based on the discussion above, solubility Ci(v/v), volume fraction ϵi,j, and intrinsic κi,j are already known. Unlike the simple mixing rule in Equation (Equation8[8] ), an additional term of dissolved fraction is necessary for partially soluble mixtures (Petters and Kreidenweis Citation2008):
[11] where xi,j is the dissolved fraction of the bin (i,j), g is the diameter growth factor g = D/Dd, and D is the diameter of the droplet. κ of the dissolved fraction determines the saturation ratio, S, as shown in the κ-Κöhler equation (Petters and Kreidenweis Citation2008):
[12]
The representation of solubility is applied to pure compounds shown in (yellow square: w/ solubility and surface). Surface activity did not significantly affect the results as discussed in the following section. illustrates the challenges of modeling partially soluble organics. In some cases, κ is significantly underpredicted when experimental observations show moderate κ. Petters et al. (Citation2016) made the same observation and hypothesized that trace contamination might affect observed κ. Overall, despite the simplicity of Equation (Equation10[10] ), the prediction in this study is only slightly inferior to the more sophisticated model developed by Petters et al. (Citation2016). The uncertainty in solubility prediction by Equation (Equation10
[10] ) is evaluated through Monte Carlo simulation in Section 3.
As an example, the framework discussed so far is applied to the 2D-VBS reaction stoichiometry of α-pinene ozonolysis provided in Donahue et al. (Citation2012). shows the distribution of OA in 2D-VBS for 100 μg m−3 of α-pinene reacted as well as intrinsic κ and solubility isolines. Intrinsic κ in this case is 0.114, which can be intuitively seen by comparing the 2D-VBS distribution and κ isolines (). If solubility and surface activity do not play any role, intrinsic κ equals apparent κ. Apparent κ of this case is calculated using Sc (, maximum of solubility only case, 1.0039), surface tension of water, and Ddry = 100 nm. shows the total dissolved volume fraction (aqueous) and the remaining fraction (non-aqueous). Apparent κ in this case is calculated to be 0.088. The lower καππ value (0.088) compared to the intrinsic κ (0.114) is due to solubility limitation (Equation (Equation11[11] ) and ).
2.4. Effects of surface-active compounds on apparent CCN activity
Although surface-active compounds may significantly affect the Köhler curve, it is currently not possible to introduce a simple relationship between surface activity and average molecular composition as was done for volatility (Equation (Equation7[7] )) and solubility (Equation (Equation10
[10] )). Further studies are needed in development of simple structure–property relationships for surfactants (Hu et al. Citation2010). As the first step, this study neglects structure-dependence of surface activity, and instead evaluates the sensitivity of apparent κ on surface activity parameters within a plausible parameter range as discussed below.
Previous studies derived simple quadratic equations that describe partitioning of a surfactant between the bulk solution and the droplet surface via adsorption (Topping Citation2010; Raatikainen and Laaksonen Citation2011):[13] where xiB is the mole fraction of i in the bulk aqueous solution, Γimax′ is the saturated surface excess, K′i is the adsorption equilibrium constant, nwT is the total mole of water, and niT is the total mole of solute i. Derivation of Equation (Equation13
[13] ) is discussed in the SI. Since this study assumes all the organic solutes have the same surface activity, the solution is treated as a binary mixture of solute i and water. nwT and niT are determined by the initial particle size, molar volume, and droplet size (Topping Citation2010). The fraction of solute remaining in the bulk phase, ξi, is given as
[14]
Depletion of the bulk-phase solute results in a lower κ in a similar fashion to the solubility limitation (Equation (Equation11[11] )) (Petters and Kreidenweis Citation2013):
[15]
Also, surface tension depression is described by the Langmuir–Szyskowski equation (Langmuir Citation1917; Topping Citation2010):[16]
Therefore, one can account for the impact of surface-active compounds on Raoult's effect through κ modification (Equation (Equation15[15] )) and Kelvin effect through surface tension depression (Equation (Equation16
[16] )) for given Γimax′ and K′i.
In order to assess the sensitivity of apparent κ on Γimax′ and K′i, a reasonable range of the parameters must be determined. summarizes previously observed surface activity parameters in terms of Γimax′ and K′i. The majority of Γimax′ is within 3 × 10−7 to 7 × 10−6 mol m−2, and K' is within 2–6 × 104, shown as the gray rectangle in . The relatively tight distribution of Γimax′ compared to K′i. is reasonable since the inverse of Γimax′ is equivalent to the area occupied by a molecule in the surface monolayer (Petters and Petters Citation2016; Ruehl et al. Citation2016), which depends on the overall size and shape of the molecule, rather than its chemical moiety. In this study, the gray area is assumed to encompass 95% of normally distributed values of logΓmax′ and logK′, i.e., ±2 standard deviations of normal distributions. Using a random number generator for a normal distribution (“randn” in Matlab), randomly sampled logΓmax′ and logK' values are introduced to Köhler curve calculation.
Figure 3. Summary of surface activity parameters for various organic compounds. Table S4 provides individual compound names and corresponding values.
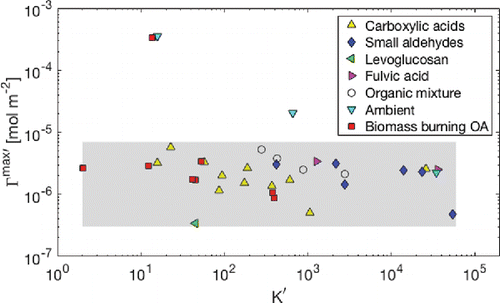
Figure S1 shows the results of 1000 simulations at Ddry = 100 nm, intrinsic κ = 0.10, and randomly chosen logΓmax' and logK'. Although the variability in Γimax' and K'i. results in a wide range of bulk solute fraction and surface tension, apparent κ was approximately the same as the intrinsic κ. Figure S1 is consistent with recent studies showing that the effects of bulk-surface partitioning and surface tension depression could cancel out in terms of apparent κ (Prisle et al. Citation2012; Raatikainen and Laaksonen Citation2014; Lowe et al. Citation2016).
2.5. Coupling the effects of solubility and surface activity
A significant challenge, however, in incorporating the surface-activity in the 2D-VBS is that bulk-surface partitioning may coexist with solubility-limitation. Since this study applies the same surface activity parameters to the entire dissolved organic, the bulk solution is expected to remain saturated as long as an undissolved non-aqueous core remains. The following iterative procedure is used at each droplet diameter (D):
1. | Calculate the initial dissolved fraction (Fbulk) for each 2D-VBS bin (i, j) based on the solubility (EquationEquations (10 | ||||
2. | Sum up all the dissolved solutes to obtain the total dissolved mole (bulk + surface). | ||||
3. | Calculate bulk-surface partitioning treating all the dissolved organics as one species (EquationEquations (13 | ||||
4. | Calculate the surface solute fraction (Fsurf) for each 2D-VBS bin (i, j). | ||||
5. | Offset the increase in Fsurf by decreasing the undissolved core fraction (Fcore) if Fcore > 0. | ||||
6. | Repeat 2–5 until dissolved solute mole converges. | ||||
7. | Calculate surface tension using the bulk-phase solution mole fraction (Equation (Equation17 |
κ at each D is determined in an analogous manner as the solubility-only case (Equation (Equation11[11] )) and the surface activity-only case (Equation (Equation15
[15] )):
[17]
Finally, the approach is applied to the example of α-pinene ozonolysis and results are shown in (full partition case). Γimax′ and K′i are assumed to be 1.45 × 10−6 mol m−2 and 3.5 × 102, respectively, which are the midpoints of the assumed 95% bounds (). suggests that in case of α-pinene SOA, solubility limitation and bulk-surface partitioning may co-exist at the point of activation. Since undissolved solutes replenish the lost bulk solute due to bulk-surface partitioning, canceling effects of surface tension depression and bulk-solute depletion are expected to be not as pronounced as the entirely soluble case. This suggests that surface tension depression might have a significant impact even after considering bulk-surface partitioning if bulk solute depletion is compensated by additional dissolution of partially soluble solutes. More studies are needed in understanding the implication of the core-bulk-surface partitioning ().
Note that the simulation shown in assumes no inorganic seeds. Inorganic salts may alter bulk-surface partitioning of organics through salting-out effects (Long and McDevit Citation1952; Shulman et al. Citation1996). The framework in this study does not include the effects of inorganic salts on surface activity. However, due to high solubility of inorganic salts, a small amount of inorganic seeds in OA would significantly change the Köhler curves of solubility-limited OA even without considering surface activity (Abbatt et al. Citation2005; Petters and Kreidenweis Citation2008). As an example, Figure S2 shows the results of a simulation assuming 10% ammonium sulfate internally mixed with α-pinene SOA. The rest of the conditions are identical to . The small amount of ammonium sulfate removes the cusps of Köhler curve observed in and increases the critical droplet diameter. As a result, more organic salutes are dissolved at the point of activation because more water is available (Figure S2e). Within the framework developed in this study, internally mixed salts generally minimize the gaps between intrinsic κ and apparent κ. This suggests that when comparing results of CCN activities of OA from different studies, one must pay attention to the presence of inorganic salts that tend to effectively enhance apparent κ of OA by removing the cusps in Köhler curves ( vs. Figure S2b).
3. Discussions
In this section, κ vs. O/C relationship is modeled by performing simulations over a wide range of OA mass loadings and is compared against the previously observed trends (Jimenez et al. Citation2009; Rickards et al. Citation2013 and references therein). In order to evaluate the effects of key assumptions made in the model, some of the key parameters are allowed to vary randomly within reasonable ranges. Specifically, the following analysis varied the surface activity parameters (Γimax′ and K′i), O/C, volatility (C*), intrinsic κ, and solubility (C) as discussed below. Note that the proposed bounds are not fully established ranges; rather this is an attempt to approximately evaluate the uncertainty of the framework using plausible ranges of parameters.
The range of surface activity parameters (Γimax′ and K′i) is chosen to be the gray area in as discussed in Section 2.4. The range is assumed to encompass ± 2 standard deviations of normally distributed logΓmax′ and logK′ (e.g., Figures S1e and S1f). For the rest of parameters, a logarithm of the ratio of randomized parameters to the default parameters (e.g., log[XRandom/XDefault]) is assumed to follow a normal distribution, and plausible bounds are chosen as ±2 standard deviations. For instance, O/C is assumed to vary by 30% for individual species (Aiken et al. Citation2007, Citation2008). In order to apply the 30% variability in O/C to normally distributed random numbers (“randn” in Matlab), the logarithm of the ratio of randomized O/C to the default O/C (log[(O/C)Random/(O/C)Default]) is assumed to follow a normal distribution, and log (1.3) equals two standard deviations. Similarly, prediction of C* is assumed to vary by two orders of magnitude (Donahue et al. Citation2011). Prediction of intrinsic κ from a given molecular formula is assumed to vary by a factor of two (). Note that this variability in intrinsic κ includes uncertainties in density (Equation (Equation3[3] )), νΦ = 1 (Equation (Equation1
[1] )), and experimental CCN measurements. Prediction of solubility (C) using O/C (Equation (Equation10
[10] )) is assumed to vary by a factor of 10 (Kuwata et al. Citation2013).
shows comparisons of intrinsic κ, apparent κ in both solubility-only case (Section 2.3) and the full core-bulk-surface partitioning case (Section 2.5) as a function of O/C. assumes no inorganic salts; assumes 10% ammonium sulfate internally mixed with OA. The dataset contains the 2D-VBS reaction stoichiometry of α-pinene ozonolysis provided in Donahue et al. (Citation2012). In addition, includes 2D-VBS stoichiometry reported in the work of Murphy et al. (Citation2012), which is based on reaction products of organic vapor with O/C = 0.4 and C* = 104 μg m−3. This case is relevant to highly oxidized OA with O/C ∼ 0.7. An example set of simulation results based on Murphy et al. (Citation2012) is also shown in Figure S3. The model shows that highly oxidized OA in Murphy et al. (Citation2012) is fully dissolved at the point of activation (Figures S3 d and S3 e). The trend in the predicted κ vs. O/C is compared against the extensive survey of this relationship by Rickards et al. (Citation2013). Their parameterization for supersaturated relative humidity (RH) data is shown as the shaded area in .
Figure 4. Predicted κ vs. O/C relationships for (a) no inorganic seed case and (b) 10% ammonium sulfate seed case. The solid line corresponds to the median values of 1000 Monte Carlo simulations. The dotted lines correspond to 25 and 75 percentile. The simulations are performed at 10, 100, and 1000 μg m−3 of reacted precursors: α-pinene (Donahue et al. Citation2012), ambient reactive organic gas (Murphy et al. Citation2012).
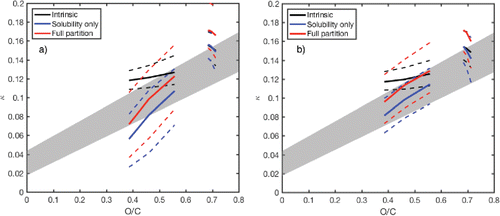
Although uncertainties shown as 25 and 75 percentiles are significant, the overall trend of all three cases (intrinsic, solubility only, and full partition) reasonably agree with the empirical fit, despite the simplicity of the theoretical framework developed in this study. Generally, solubility limitation is predicted to lower the apparent κ of α-pinene SOA when there are no inorganic seeds (). shows that the small amount of inorganic seeds reduces the solubility limitation, and therefore apparent κ approaches intrinsic κ. Therefore, this analysis shows that it is important to consider the presence of inorganic seeds especially when comparing κ of SOA in different chamber studies. In addition, shows potential impact of surface activity within a reasonable range of Γimax′ and K′i. The model predicts slight enhancement of apparent κ, approximately 5%–25%. However, it is unclear if surface activity plays a significant role considering the uncertainties. At least shows that surface activity is unlikely to profoundly change the overall trend of κ vs. O/C for the range of Γimax′ and K′i parameters considered in this study. Finally, suggests that κ can also decrease as O/C increases depending on the distribution of OA in 2D-VBS; in case of Murphy et al. (Citation2012) (near O/C 0.7), κ decreased as O/C increased. Ultimately, distribution of OA relative to the isolines of κ as well as solubility limitation determines apparent κ in this framework. This could explain why in some cases κ of OA does not linearly increase with O/C (Alfarra et al. Citation2013). Further studies are needed in estimating 2D-VBS distributions of those systems that exhibit different trends of κ vs. O/C.
The model calculation provides a plausible explanation on why O/C can have an impact on κ. The mechanism is likely to be not just the solubility (Kuwata et al. Citation2013; Riipinen et al. Citation2015), but a combination of the solubility and volatility that determines the molecular size of dissolved solutes. Also, it is clear that the distribution of O/C throughout the 2D-VBS space, not just the average, is crucial in modeling CCN activation, consistent with Riipinen et al. (Citation2015). The insights obtained by this framework can aid interpretation of complex behavior of CCN activity in response to various processes such as volatilization. As an example, the SI includes discussions on the interpretation of ambient CCN in the southeastern United States by Cerully et al. (Citation2015).
It is of course possible that the model results can match experimental observations for wrong reasons. However, development of a plausible explanation that accounts for the interplay of volatility, solubility, and surface activity is a significant progress from the current state of entirely empirical fit of the observed κ vs. O/C trend. Although this study focused on the 2D-VBS, in principle, the same approach is readily applicable to other equivalent two-dimensional representations of OA aging (Kroll et al. Citation2011; Shiraiwa et al. Citation2014). The simplicity of the framework opens up new opportunities to evaluate model performance of OA aging in terms of κ. Further studies are needed to account for the effects of nitrogen species in terms of volatility (Chuang and Donahue Citation2016), solubility (Suda et al. Citation2014), and density (Kuwata et al. Citation2012). Treatment of surface activity can be improved by developing structure–property relationship (Hu et al. Citation2010) and accounting for mixture effects (Li and Lu Citation2001; Topping Citation2010). A recently developed alternative approach based on the compressed film equation of state may better represent the shape of the Köhler curve (Ruehl et al. Citation2016): Its implication in various conditions of ambient OA must be investigated.
4. Conclusions
This study proposes a simple framework that presents plausible answers to the question: “Why would apparent κ linearly change with O/C?” The answer would depend on the distribution of OA in the 2D-VBS space relative to the isolines of κ and solubility, as well as the presence of inorganic salts. For instance, a distribution of OA in the 2D-VBS parallel to an isoline for κ () would result in no change in apparent κ as a function of O/C if solubility limitation were not exhibited (e.g., highly oxidized OA, significant inorganic salt fraction). In that case, volatilization of OA by a thermodenuder would also result in no change in κ. In case of no inorganic salts and moderate O/C, solubility may significantly limit apparent κ of OA, and hence apparent κ can increase up to the intrinsic κ value as O/C increases (). However, the intrinsic κ value is determined by combination of O/C and C* (EquationEquations (4[4] ), (Equation5
[5] ), and (Equation7
[7] )). Furthermore, the framework suggests that κ of OA is unlikely to be above 0.3 no matter how high the O/C is under atmospherically relevant mass loadings of OA. The framework reasonably reproduces observed relationships between κ and O/C when applied to previously reported 2D-VBS reaction stoichiometry datasets. Monte Carlo simulations show that surface activity is not likely to profoundly affect modeled κ considering uncertainties of various parameters in this study. This study presents the first prediction of the κ – O/C relationship, based only on the product distribution in the 2D-VBS space. The framework enables future studies to use κ as an additional constraint on models of OA aging.
UAST_1352082_Supplementary_Files.zip
Download Zip (1 MB)Acknowledgments
The author thanks Prof. Sonia Kreidenweis and Prof. Barbara Turpin for valuable discussions.
Funding
This material is partly based upon work supported by the National Science Foundation under an Atmospheric and Geospace Sciences Postdoctoral Research Fellowship (AGS-PRF, 1230395). Any opinions, findings, and conclusions or recommendations expressed in this material are those of the author and do not necessarily reflect the views of the National Science Foundation. The author also acknowledges additional support from US Department of Energy, under DESC0006633, and the Department of Chemical and Biomolecular Engineering, Clarkson University.
References
- Abbatt, J. P. D., Broekhuizen, K., and Kumar, P. (2005). Cloud Condensation Nucleus Activity of Internally Mixed Ammonium Sulfate/Organic Acid Aerosol Particles. Atmos. Environ., 39:4767–4778.
- Aiken, A. C., DeCarlo, P. F., and Jimenez, J. L. (2007). Elemental Analysis of Organic Species with Electron Ionization High-Resolution Mass Spectrometry. Anal. Chem., 79:8350–8358.
- Aiken, A. C., DeCarlo, P. F., Kroll, J. H., Worsnop, D. R., Huffman, J. A., Docherty, K., Ulbrich, I., Mohr, C., Kimmenl, J. R., Sun, Y., Zhang, Q., Trimborn, A. M., Northway, M., Ziemann, P. J., Canagaratna, M. R., Onasch, T. B., Alfarra, M. R., Prevot, A. S., Dommen, J., Duplissy, J., Metzger, A., Baltensperger, U., and Jimenez, J. L. (2008). O/C and OM/OC Ratios of Primary, Secondary, and Ambient Organic Aerosols with High-Resolution Time-of-Flight Aerosol Mass Spectrometry. Environ. Sci. Technol., 42:4487–4485.
- Alfarra, M. R., Good, N., Wyche, K. P., Hamilton, J. F., Monks, P. S., Lewis, A. C., and McFiggans, G. (2013). Water Uptake is Independent of the Inferred Composition of Secondary Aerosols Derived from Multiple Biogenic VOCs. Atmos. Chem. Phys., 13:11769–11789.
- Andreae, M. O., and Rosenfeld, D. (2008). Aerosol–Cloud–Precipitation Interactions. Part 1. The Nature and Sources of Cloud-Active Aerosols. Earth-Sci. Rev., 89:13–41.
- Asa-Awuku, A., Sullivan, A. P., Hennigan, C. J., Weber, R. J., and Nenes, A. (2008). Investigation of Molar Volume and Surfactant Characteristics of Water-Soluble Organic Compounds in Biomass Burning Aerosol. Atmos. Chem. Phys., 8:799–812.
- Cerully, K. M., Bougiatioti, A., Hite Jr, J. R., Guo, H., Xu, L., Ng, N. L., Weber, R., and Nenes, A. (2015). On the Link Between Hygroscopicity, Volatility, and Oxidation State of Ambient and Water-Soluble Aerosols in the Southeastern United States. Atmos. Chem. Phys., 15:8679–8694.
- Chang, R. Y. W., Slowik, J. G., Shantz, N. C., Vlasenko, A., Liggio, J., Sjostedt, S. J., Leaitch, W. R., and Abbatt, J. P. D. (2010). The Hygroscopicity Parameter (κ) of Ambient Organic Aerosol at a Field Site Subject to Biogenic and Anthropogenic Influences: Relationship to Degree of Aerosol Oxidation. Atmos. Chem. Phys., 10:5047–5064.
- Chuang, W. K., and Donahue, N. M. (2016). A Two-Dimensional Volatility Basis Set – Part 3: Prognostic Modeling and NOx Dependence. Atmos. Chem. Phys., 16:123–134.
- Daumit, K. E., Kessler, S. H., and Kroll, J. H. (2013). Average Chemical Properties and Potential Formation Pathways of Highly Oxidized Organic Aerosol. Faraday Discuss., 165:181–202.
- Donahue, N. M., Chuang, W., Epstein, S. A., Kroll, J. H., Worsnop, D. R., Robinson, A. L., Adams, P. J., and Pandis, S. N. (2013). Why do Organic Aerosols Exist? Understanding Aerosol Lifetimes Using the Two-Dimensional Volatility Basis Set. Environ. Chem., 10:151–157.
- Donahue, N. M., Epstein, S. A., Pandis, S. N., and Robinson, A. L. (2011). A Two-Dimensional Volatility Basis Set: 1. Organic-Aerosol Mixing Thermodynamics. Atmos. Chem. Phys., 11:3303–3318.
- Donahue, N. M., Kroll, J. H., Pandis, S. N., and Robinson, A. L. (2012). A Two-Dimensional Volatility Basis Set - Part 2: Diagnostics of Organic-Aerosol Evolution. Atmos. Chem. Phys., 12:615–634.
- Donahue, N. M., Robinson, A. L., Stanier, C. O., and Pandis, S. N. (2006). Coupled Partitioning, Dilution, and Chemical Aging of Semivolatile Organics. Environ. Sci. Technol., 40:2635–2643.
- Ervens, B., Turpin, B. J., and Weber, R. J. (2011). Secondary Organic Aerosol Formation in Cloud Droplets and Aqueous Particles (aqSOA): A Review of Laboratory, Field and Model Studies. Atmos. Chem. Phys., 11:11069–11102.
- Facchini, M. C., Mircea, M., Fuzzi, S., and Charlson, R. J. (1999). Cloud Albedo Enhancement by Surface-Active Organic Solutes in Growing Droplets. Nature, 401:257–259.
- Frosch, M., Bilde, M., DeCarlo, P. F., Jurányi, Z., Tritscher, T., Dommen, J., Donahue, N. M., Gysel, M., Weingartner, E., and Baltensperger, U. (2011). Relating Cloud Condensation Nuclei Activity and Oxidation Level of α-Pinene Secondary Organic Aerosols. J. Geophys. Res., 116:D22212.
- Frosch, M., Zardini, A. A., Platt, S. M., Müller, L., Reinnig, M. C., Hoffmann, T., and Bilde, M. (2010). Thermodynamic Properties and Cloud Droplet Activation of a Series of Oxo-Acids. Atmos. Chem. Phys., 10:5873–5890.
- Giordano, M. R., Short, D. Z., Hosseini, S., Lichtenberg, W., and Asa-Awuku, A. A. (2013). Changes in Droplet Surface Tension Affect the Observed Hygroscopicity of Photochemically Aged Biomass Burning Aerosol. Environ. Sci. Technol., 47:10980–10986.
- Heald, C. L., Kroll, J. H., Jimenez, J. L., Docherty, K., DeCarlo, P. F., Aiken, A. C., Chen, Q., Martin, S. T., Farmer, D. K., and Artaxo, P. (2010). A Simplified Description of the Evolution of Organic Aerosol Composition in the Atmosphere. Geophys. Res. Lett., 37:L08803.
- Hu, J., Zhang, X., and Wang, Z. (2010). A Review on Progress in QSPR Studies for Surfactants. Int. J. Mol. Sci., 11:1020–1047.
- Jimenez, J. L., Canagaratna, M. R., Donahue, N. M., Prevot, A. S. H., Zhang, Q., Kroll, J. H., DeCarlo, P. F., Allan, J. D., Coe, H., Ng, N. L., Aiken, A. C., Docherty, K. S., Ulbrich, I. M., Grieshop, A. P., Robinson, A. L., Duplissy, J., Smith, J. D., Wilson, K. R., Lanz, V. A., Hueglin, C., Sun, Y. L., Tian, J., Laaksonen, A., Raatikainen, T., Vaattovaara, P., Ehn, M., Kulmala, M., Tomlinson, J. M., Collins, D. R., Cubison, M. J., Dunlea, E. J., Huffman, J. A., Onasch, T. B., Alfarra, M. R., Williams, P. I., Bower, K., Kondo, Y., Schneider, J., Drewnick, F., Borrmann, S., Weimer, S., Demerjian, K., Salcedo, D., Cottrell, L., Griffin, R. J., Takami, A., Miyoshi, T., Hatakeyama, S., Shimono, A., Sun, J. Y., Zhang, Y. M., Dzepina, K., Kimmel, J. R., Sueper, D., Jayne, J. T., Herndon, S. C., Trimborn, A. M., Williams, L. R., Wood, E. C., Middlebrook, A. M., Kolb, C. E., Baltensperger, U., and Worsnop, D. R. (2009). Evolution of Organic Aerosols in the Atmosphere. Science, 326:1525–1529.
- Kreidenweis, S. M., Koehler, K., DeMott, P. J., Prenni, A. J., Carrico, C., and Ervens, B. (2005). Water Activity and Activation Diameters from Hygroscopicity Data - Part I: Theory and Application to Inorganic Salts. Atmos. Chem. Phys., 5:1357–1370.
- Kreidenweis, S. M., Petters, M. D., and Chuang, P. Y. (2009). Cloud Particle Precursors. The MIT Press, Cambridge, MA.
- Kroll, J. H., Donahue, N. M., Jimenez, J. L., Kessler, S. H., Canagaratna, M., Wilson, K. R., Altieri, K. E., Mazzoleni, C., Wozniak, A. S., Bluhm, H., Mysak, E. R., Smith, J. D., Kolb, C. E., and Worsnop, D. R. (2011). Carbon Oxidation State as a Metric for Describing the Chemistry of Atmospheric Organic Aerosol. Nat. Chem., 3:133–139.
- Kuwata, M., Shao, W., Lebouteiller, R., and Martin, S. T. (2013). Classifying Organic Materials by Oxygen-to-Carbon Elemental Ratio to Predict the Activation Regime of Cloud Condensation Nuclei (CCN). Atmos. Chem. Phys., 13:5309–5324.
- Kuwata, M., Zorn, S. R., and Martin, S. T. (2012). Using Elemental Ratios to Predict the Density of Organic Material Composed of Carbon, Hydrogen, and Oxygen. Environ. Sci. Technol., 46:787–794.
- Lambe, A. T., Onasch, T. B., Massoli, P., Croasdale, D. R., Wright, J. P., Ahern, A. T., Williams, L. R., Worsnop, D. R., Brune, W. H., and Davidovits, P. (2011). Laboratory Studies of the Chemical Composition and Cloud Condensation Nuclei (CCN) Activity of Secondary Organic Aerosol (SOA) and Oxidized Primary Organic Aerosol (OPOA). Atmos. Chem. Phys., 11:8913–8928.
- Langmuir, I. (1917). The Constitution and Fundamental Properties of Solids and Liquids. II. Liquids. J. Am. Chem. Soc., 39:1848–1906.
- Lathem, T. L., Beyersdorf, A. J., Thornhill, K. L., Winstead, E. L., Cubison, M. J., Hecobian, A., Jimenez, J. L., Weber, R. J., Anderson, B. E., and Nenes, A. (2013). Analysis of CCN Activity of Arctic Aerosol and Canadian Biomass Burning During Summer 2008. Atmos. Chem. Phys., 13:2735–2756.
- Li, Z., and Lu, B. C. Y. (2001). Surface Tension of Aqueous Electrolyte Solutions at High Concentrations — Representation and Prediction. Chem. Eng. Sci., 56:2879–2888.
- Long, F. A., and McDevit, W. F. (1952). Activity Coefficients of Nonelectrolyte Solutes in Aqueous Salt Solutions. Chem. Rev., 51:119–169.
- Lowe, S., Partridge, D. G., Topping, D., and Stier, P. (2016). Inverse Modelling of Köhler Theory – Part 1: A Response Surface Analysis of CCN Spectra with Respect to Surface-Active Organic Species. Atmos. Chem. Phys., 16:10941–10963.
- Massoli, P., Lambe, A. T., Ahern, A. T., Williams, L. R., Ehn, M., Mikkilä, J., Canagaratna, M. R., Brune, W. H., Onasch, T. B., Jayne, J. T., Petäjä, T., Kulmala, M., Laaksonen, A., Kolb, C. E., Davidovits, P., and Worsnop, D. R. (2010). Relationship Between Aerosol Oxidation Level and Hygroscopic Properties of Laboratory Generated Secondary Organic Aerosol (SOA) Particles. Geophys. Res. Lett., 37:L24801.
- McFiggans, G., Artaxo, P., Baltensperger, U., Coe, H., Facchini, M. C., Feingold, G., Fuzzi, S., Gysel, M., Laaksonen, A., Lohmann, U., Mentel, T. F., Murphy, D. M., O'Dowd, C. D., Snider, J. R., and Weingartner, E. (2006). The Effect of Physical and Chemical Aerosol Properties on Warm Cloud Droplet Activation. Atmos. Chem. Phys., 6:2593–2649.
- Mei, F., Hayes, P. L., Ortega, A., Taylor, J. W., Allan, J. D., Gilman, J., Kuster, W., de Gouw, J., Jimenez, J. L., and Wang, J. (2013a). Droplet Activation Properties of Organic Aerosols Observed at an Urban Site During CalNex-LA. J. Geophys. Res.: Atmos., 118:2903–2917.
- Mei, F., Setyan, A., Zhang, Q., and Wang, J. (2013b). CCN Activity of Organic Aerosols Observed Downwind of Urban Emissions During CARES. Atmos. Chem. Phys., 13:12155–12169.
- Murphy, B. N., Donahue, N. M., Fountoukis, C., Dall'Osto, M., O'Dowd, C., Kiendler-Scharr, A., and Pandis, S. N. (2012). Functionalization and Fragmentation During Ambient Organic Aerosol Aging: Application of the 2-D Volatility Basis Set to Field Studies. Atmos. Chem. Phys., 12:10797–10816.
- Nakao, S., Tang, P., Tang, X., Clark, C. H., Qi, L., Seo, E., Asa-Awuku, A., and Cocker III, D. (2013). Density and Elemental Ratios of Secondary Organic Aerosol: Application of a Density Prediction Method. Atmos. Environ., 68:273–277.
- Paciga, A. L., Riipinen, I., and Pandis, S. N. (2014). Effect of Ammonia on the Volatility of Organic Diacids. Environ. Sci. Technol., 48:13769–13775.
- Padro, L. T., Asa-Awuku, A., Morrison, R., and Nenes, A. (2007). Inferring Thermodynamic Properties from CCN Activation Experiments: Single-Component and Binary Aerosols. Atmos. Chem. Phys., 7:5263–5274.
- Pankow, J. F., and Asher, W. E. (2008). SIMPOL.1: A Simple Group Contribution Method for Predicting Vapor Pressures and Enthalpies of Vaporization of Multifunctional Organic Compounds. Atmos. Chem. Phys., 8:2773–2796.
- Petters, M. D., and Kreidenweis, S. M. (2007). A Single Parameter Representation of Hygroscopic Growth and Cloud Condensation Nucleus Activity. Atmos. Chem. Phys., 7:1961–1971.
- Petters, M. D., and Kreidenweis, S. M. (2008). A Single Parameter Representation of Hygroscopic Growth and Cloud Condensation Nucleus Activity - Part 2: Including Solubility. Atmos. Chem. Phys., 8:6273–6279.
- Petters, M. D., and Kreidenweis, S. M. (2013). A Single Parameter Representation of Hygroscopic Growth and Cloud Condensation Nucleus Activity - Part 3: Including Surfactant Partitioning. Atmos. Chem. Phys., 13:1081–1091.
- Petters, M. D., Kreidenweis, S. M., Prenni, A. J., Sullivan, A., Carrico, C. M., Koehler, K. A., and Ziemann, P. J. (2009). Role of Molecular Size in Cloud Droplet Activation. Geophys. Res. Lett., 36:L22801.
- Petters, M. D., Kreidenweis, S. M., and Ziemann, P. J. (2016). Prediction of Cloud Condensation Nuclei Activity for Organic Compounds Using Functional Group Contribution Methods. Geosci. Model Dev., 9:111–124.
- Petters, S. S., and Petters, M. D. (2016). Surfactant Effect on Cloud Condensation Nuclei for Two-Component Internally Mixed Aerosols. J. Geophys. Res.: Atmos., 121:1878–1895.
- Prisle, N. L., Asmi, A., Topping, D., Partanen, A. I., Romakkaniemi, S., Dal Maso, M., Kulmala, M., Laaksonen, A., Lehtinen, K. E. J., McFiggans, G., and Kokkola, H. (2012). Surfactant Effects in Global Simulations of Cloud Droplet Activation. Geophys. Res. Lett., 39:L05802.
- Prisle, N. L., Raatikainen, T., Laaksonen, A., and Bilde, M. (2010). Surfactants in Cloud Droplet Activation: Mixed Organic-Inorganic Particles. Atmos. Chem. Phys., 10:5663–5683.
- Pruppacher, H. R., and Klett, J. D. (1997). Microphysics of Clouds and Precipitation. Kluwer Academic Publishers, Dordrecht.
- Raatikainen, T., and Laaksonen, A. (2011). A Simplified Treatment of Surfactant Effects on Cloud Drop Activation. Geosci. Model Dev., 4:107–116.
- Raatikainen, T., and Laaksonen, A. (2014). Comment on “Changes in Droplet Surface Tension Affect the Observed Hygroscopicity of Photochemically Aged Biomass Burning Aerosol.” Environ. Sci. Technol., 48:2082–2083.
- Rickards, A. M. J., Miles, R. E. H., Davies, J. F., Marshall, F. H., and Reid, J. P. (2013). Measurements of the Sensitivity of Aerosol Hygroscopicity and the κ Parameter to the O/C Ratio. J. Phys. Chem. A, 117:14120–14131.
- Riipinen, I., Rastak, N., and Pandis, S. N. (2015). Connecting the Solubility and CCN Activation of Complex Organic Aerosols: A Theoretical Study Using Solubility Distributions. Atmos. Chem. Phys., 15:6305–6322.
- Rose, D., Gunthe, S. S., Mikhailov, E., Frank, G. P., Dusek, U., Andreae, M. O., and Pöschl, U. (2008). Calibration and Measurement Uncertainties of a Continuous-Flow Cloud Condensation Nuclei Counter (DMT-CCNC): CCN Activation of Ammonium Sulfate and Sodium Chloride Aerosol Particles in Theory and Experiment. Atmos. Chem. Phys., 8:1153–1179.
- Rosenørn, T., Kiss, G., and Bilde, M. (2006). Cloud Droplet Activation of Saccharides and Levoglucosan Particles. Atmos. Environ., 40:1794–1802.
- Ruehl, C. R., Chuang, P. Y., Nenes, A., Cappa, C. D., Kolesar, K. R., and Goldstein, A. H. (2012). Strong Evidence of Surface Tension Reduction in Microscopic Aqueous Droplets. Geophys. Res. Lett., 39:L23801, doi: 23810.21029/22012GL053706.
- Ruehl, C. R., Davies, J. F., and Wilson, K. R. (2016). An Interfacial Mechanism for Cloud Droplet Formation on Organic Aerosols. Science 351:1447–1450.
- Shiraiwa, M., Berkemeier, T., Schilling-Fahnestock, K. A., Seinfeld, J. H., and Pöschl, U. (2014). Molecular corridors and kinetic regimes in the multiphase chemical evolution of secondary organic aerosol. Atmos. Chem. Phys., 14:8323–8341.
- Shulman, M. L., Jacobson, M. C., Carlson, R. J., Synovec, R. E., and Young, T. E. (1996). Dissolution Behavior and Surface Tension Effects of Organic Compounds in Nucleating Cloud Droplets. Geophys. Res. Lett., 23:277–280.
- Sorjamaa, R., Svenningsson, B., Raatikainen, T., Henning, S., Bilde, M., and Laaksonen, A. (2004). The Role of Surfactants in Köhler Theory Reconsidered. Atmos. Chem. Phys., 4:2107–2117.
- Suda, S. R., Petters, M. D., Yeh, G. K., Strollo, C., Matsunaga, A., Faulhaber, A., Ziemann, P. J., Prenni, A. J., Carrico, C. M., Sullivan, R. C., and Kreidenweis, S. M. (2014). Influence of Functional Groups on Organic Aerosol Cloud Condensation Nucleus Activity. Environ. Sci. Technol., 48:10182–10190.
- Topping, D. (2010). An Analytical Solution to Calculate Bulk Mole Fractions for Any Number of Components in Aerosol Droplets After Considering Partitioning to a Surface Layer. Geosci. Model Dev., 3:635.
- Wong, J. P. S., Lee, A. K. Y., Slowik, J. G., Cziczo, D. J., Leaitch, W. R., Macdonald, A., and Abbatt, J. P. D. (2011). Oxidation of Ambient Biogenic Secondary Organic Aerosol by Hydroxyl Radicals: Effects on Cloud Condensation Nuclei Activity. Geophys. Res. Lett., 38:L22805.