ABSTRACT
Professional skills have long been perceived as lacking in junior engineers. Adopting a social realist theoretical framework of knowledge in practice, a hypothesis-based survey study of early career engineers’ perceptions of engineering expertise was conducted. It investigated a professional skills readiness difference between initial career trajectories (hypothesis 1) through an analysis of engineering expertise perception, and whether this difference decreases over time as engineers mature (hypothesis 2). Both hypotheses were supported by three statistical tests which established the specific nature and size of this difference. Three themes were identified: Academic bias, Technical competence bias, and Rationality bias. Thematic analysis through the framework of these three themes indicates how context and complexity (Semantic dimension) and Knowledge and Knower (Specialisation dimension) were understood in practice. The three themes expressed challenges over these two dimensions in understanding Technical knowledge, Collaboration, and the Legitimate basis for practice, leading to recommendations for education and practice.
1. Introduction
The insistence that engineering practice immediately requires professional skills has become, if not universal, then at least uncontroversial (Itani and Srour Citation2016, 1; Winberg et al. Citation2018, 167). The difficulty for new engineers transitioning to engineering practice and industry’s concern about their often lacking in professional skills are well documented (Brunhaver et al. Citation2018; Jesiek, Trellinger, and Nittala Citation2017; Trevelyan Citation2014, chapter 3; Trevelyan Citation2019). However, despite decades-long efforts of educational research and accrediting bodies such as ABETFootnote1 and ENAEE,Footnote2 calls for teaching these skills largely remain as loud as they are vague in what it means to require effective problem-solving, teamwork, communication, and coordination. A recent exception is the extensive review and detailed competency elaboration of Honor J. Passow and Passow (Citation2017, Table 1), who also point out an important reason for this vagueness, finding technical skills inseparable from effective collaboration (491) and ‘non-technical’ skills inseparable from its technical context in practice (501). The mitigation of these two inseparability-views is central to curricular innovations that enable stepping into contexts, such as CDIO and PBL (Edström and Kolmos Citation2014, 539–540). In contrast, Wolff (Citation2018) has addressed the consequence of these two views by urging educators to step out of real-world contexts and help students understand the need for the ability to consciously shift between significantly different ways of thinking and meaning-making (192) in engineering problem-solving. Lack of instruction that respects these two views (Winberg et al. Citation2018, 177) and fails to inculcate this shifting-ability in students creates an education to practice misalignment (EPM) which seems to stand behind general exhortations of the need for professional skills. All engineers thus seem to need professional skills immediately after graduation, but little is known of the relative perceived importance of these skills during the early career in different engineering practice contexts (Brunhaver et al. Citation2018). Identifying the nature of such differences in early practice would be helpful for understanding and providing nuance to this EPM. It could also help to sensitise educators to the need to present a more contextualised view of the role of professional skills to students, who (we think rightly) often express doubt toward universalised and isolated views of ‘non-technical’ skills (Downey Citation2015; Pascail Citation2011). Therefore, this study focuses on measuring practicing early engineers’ perceptions of engineering expertise as a whole rather than on measuring students’ perceptions of separate skills for practice (c.f. Byrne, Weston, and Cave Citation2018; Shuman, Besterfield-Sacre, and McGourty Citation2005). It aims to investigate differences in how early engineers in different practice-roles but from the same educational background understand engineering expertise.
There is a seeming consensus on seeing Professional or ‘non-technical’ skills as a generic competency category important for all practicing engineers from the start (hereafter called the universality-view) and that such skills cannot be understood independently from technical skills (hereafter called the integrative-view) (Anderson et al. Citation2010; Bucciarelli Citation1994; Downey Citation1998; Trevelyan Citation2007, Citation2014; Vinck Citation2003). Studies are needed in this context (Brunhaver et al. Citation2018) and furthermore there is a dearth of studies measuring skills-perceptions in such early practice while taking this integrative-view into methodological account. Hence the purpose of this study. This study proposes to put the division between the ‘technical’ and ‘non-technical’ is on the analytical level rather than on the level of data collection by using engineering expertise as a skill-integrated measurement construct. The study uses a hypothesis-testing approach to investigate differences in the relative importance attributed to professional skills by early-career engineers of differing career trajectories.
Section 2 starts with a review of the thematic difference between engineering education and work. This difference is viewed from the integrative-view of professional skills in engineering work, which is used to question the universality-view through the presented social realist theoretical framework. Based on this framework, Section 2 concludes with posing two hypotheses: the first (H1) being that early engineers value professional skills differently depending on discipline, and the second (H2) being that such difference lessens over time as the engineer matures. Section 3 develops the methodology for the statistical testing of the hypotheses using a survey. The results are presented in Section 4. The theoretical framework is used to analyse the results in Section 5. In the discussion of Section 6 the analysis is connected back to the purpose presented above, together with the discussion of limitations and implications for education and practice. Section 7 shortly summarises and ends the paper.
2. An integrative view of skill in early engineering practice
This section reviews the engineering education literature on differences between engineering work and education, professional skills and these skills’ misalignment between education and practice. A social realist theoretical framework is adopted, and two hypotheses are formulated.
2.1. The nature of misalignment between engineering work and education
Anderson et al. (Citation2010, 154–155) argued that the nature of engineering work and design is often represented by themes that concern the messiness of the work, the primacy of interdisciplinary collaboration, and practical problem solving (See also Bucciarelli Citation1994; Michael Citation1998; Vinck Citation2003; Walter Citation1990). Trevelyan (Citation2008b, Citation2014, Citation2019) emphasised that the messiness of the work and the need for collaborative performance frame the nature of problem-solving in engineering work. Considering these overarching themes, what specifically is it that an effective engineer does? Passow and Passow (Citation2017) synthesised a portrait of generic engineering practice (Figure 5, 492) from their findings of systematically reviewing the literature on generic competencies for engineering practice: This portrait focuses on engineers as creating, implementing, and maintaining solutions under multidimensional constraints, many of which are ‘non-technical’. Practice is inherently collaborative because technological endeavours are too complex for any single person to grasp. Effective engineers make decisions based on data that they independently gather in their projects. They manage and collaborate in these projects (often) without formal authority, taking initiative on what to do and which functional, horizontal, and vertical boundaries to cross to achieve desired performance and function in the target system.
However, students generally find themselves in a thematically opposite position to this portrait and the overarching themes. Most student work is predefined in scope and outcome, oriented toward measurable individual performance, and focused on theoretical problem solving (c.f. the discussion of technical rationality by Cosgrove and O’Reilly (Citation2018, 40–42) and the student norms of Leonardi, Jackson, and Diwan (Citation2002)). This partly explains why engineering education and engineering work are two fundamentally different realms of social practice, with distinct communities of practice in universities and firms (Korte, Brunhaver, and Sheppard Citation2015). These communities perceive different skills and competencies as important (Passow Citation2012, 98).
These thematic differences seem to generate significant misalignment between education and practice, driving numerous attempts at engineering education reform. Problem/project-based learning (PBL, both complementary but different approaches) and conceive-design-implement-operate (CDIO) (Edström and Kolmos Citation2014) are two major efforts at such reform. The notion of ‘T-Shaped’ engineers (Rogers and Freuler Citation2015) is another associated curricular reaction to EPM. The ‘T’ is a metaphor describing a depth of expertise in a single field and a breadth in several others, strengthening the ability to collaborate across disciplines with experts in many areas (See Figure 2 in Rogers and Freuler Citation2015). More specifically, design and capstone project courses are common features of EPM-minimising efforts in engineering education, attempting to simulate engineering practice in various ways.
However, despite the widespread use and long-established benefit of such curricular innovations, simulating practice inside of an engineering education context can be seen as problematic for several reasons: Engineering problems cannot be simulated to afford an understanding of ‘real world’ contexts (Wolff Citation2018, 192). As found by Leonardi et al. (Citation2002) in their interview study of how students act on their understanding of ‘good engineer(s/ing)’, being stuck in the same education-thematic context can engender counter-productive design practices in engineering students. While several attempts exist within PBL and CDIO to address problems of educational contexts being divorced from practice, such as by integrating and assessing student’s artistry, self-reflection, and design process (Cosgrove and O’Reilly Citation2018), engineering education must still be structured to allow learning to be predicted using curricula and learning goals, and repeatably assessed through the uniform examination of learning performances, which are time-bounded. Poor (non-failing) performance in a course,Footnote3 however defined and measured, does not affectFootnote4 the next course. However, this is not the case in professional practice since poor performance in past work affects the conditions of future work.
The paradox embodied here has several underlying assumptions that deserve to be problematised: It is assumed that an engineering degree guarantees ‘basic technical skill’, which Grimson and Murphy (Ch. 9 in Hyldgaard Christensen Citation2015) call the Foundation Layer of engineering knowledge; It is assumed that such a degree does not afford a guarantee of ‘professional skill’; It is assumed that all engineering practice has context-dependent skill/competency requirements; It is assumed that ‘professional skills’ are generic, immediately-needed, and transferable over these contexts (representing a universality-view); It is assumed that this must imply that higher education institutions cannot fully mitigate EPM, but should still try. Behind these assumptions stands a core view shared by several studies in engineering education: That engineering education in both its research and practice serves as ‘R&D departments’ and ‘production facilities’ of engineering competency delivered to private companies in the form of graduate engineers. Such a view is by no means held by all engineering education researchers, or most universities themselves.Footnote5 Indeed, many writings in this journal stand (implicitly or explicitly) ideologically opposed to such positions held by other prominent engineering education journals. Pascail (Citation2011) described and reflected on this view as being a consequence of engineering education’s adherence to a ‘skills approach’ by industry. In Brunhaver et al. (Citation2018)’s critique, professional skills are seen as inherently general skills that are transferable over engineering practice contexts and do not directly address learning contextual rulesets of one’s firm, department, or group: Professional skills (if understood as general and transferable) might thus help in learning such rulesets, but do not constitute them. In contrast, each firm will demand competency profiles that benefit their organisation’s specific needs, often based on the primary types of technology and engineering disciplines they deal with. Cappelli (Citation2008) argued that over time market pressures have motivated firms to offload the needed internal training of new hires unto engineering education by demanding curricular focus on professional skills (i.e. a ‘skills approach’). These needs could easily be shortsighted, only reflecting current needs of the organisation and job market. Recent empirical research findings also support such opposition, such as the recommendations of Wolff (Citation2018, 192–193) that educators step out of ‘real world’ contexts, thereby affording a more conceptual view by explicating and teaching what Wolff calls code-shifting between ways of thinking in engineering practice problem-solving: This is a stream of educational innovation that takes a direction different from that of CDIO and PBL, but in a manner that complements rather than oppose them. The point is not that maintaining a fundamental ‘employability’ view on engineering education is good or bad, but rather that this lens is a major underlying viewpoint to which one must relate when problematising skill-concepts in education, engineering or otherwise. For example, what might be opposed to is not the idea that higher education institutions should produce ‘viable’ graduates, but rather the way to go about it, a move which the code-shifting argument in the Wolff-article is a good example. Indeed, Wolff’s introduction motivates their research through the current state of the engineering job market, which is a common and arguably reasonable move.
Furthermore, standardised basic technical curricular elements (the foundational layer) do not necessarily form a paradox against context-specific engineering practice. These elements, such as math and basic science, deliver desirable fundamental technical competencies that are general to all engineering students (also described as the competency ‘Apply Knowledge’ by Passow and Passow (Citation2017, 493)). The paradox stems from the fact that such standardisation cannot be maintained in the same way for professional skills since such must be integrated with the relevant engineering context to be effectively taught, learned, and practised. Writing from an Anglo-Saxon perspective based on a system of chartering engineers, Grimson and Murphy (Ch. 9 in Hyldgaard Christensen, Citation2015, 171–172) argued that learning professional skills (in the top layer of their engineering knowledge model) is done in two parts: competence formed during the engineering degree programme and competence formed over minimum eight years of initial professional engineering practice. In short, a universality-view of what skills are (generic) and when they are needed (always) can be maintained for the foundation layer, but not the top layer. The results of this study came to reflect this view, although in a more nuanced fashion; seeing that such professional skills might be expressed differently in early practice over varying disciplinary and institutional contexts. However, over time perspectives around the importance and role of professional skills will align over contexts and disciplines for the early career. Indeed, the eight-year time span of the present study covers the second part of Grimson and Murphy’s top layer.
In short, standardised curricular elements do deliver fundamental technical skills necessary for all engineers, but cannot fully deliver competence in so-called ‘non-technical’ skills that are dependent on the engineering practice context in which they are deployed: the universality-view holds for the former, but not the latter. This paradox of standardised elements against context-dependent practice is a central reason why engineering education cannot take on the responsibility of eliminating EPM by itself. This paradox is a central driver for EPM. Therefore, we choose to investigate the nature of these ‘non-technical’ skills or competencies for early engineering practice. The next section discusses and problematises the concept of professional skills further.
2.2. Professional skills as an integrated part of practice
There is no consensus on a strict definition of professional skills, even though a common way is to refer to the third criteria of ABET (Passow Citation2007; Shuman et al. Citation2005) or other accreditation schemes. Colwell (Citation2010, 3) pointed out that if one were to ask educators in … engineering … what is meant by the term ‘soft skills’, there would likely be some consensus on the list, but each educator asked would probably have a different list. Referencing previous studies of engineering programme alumni, practitioners, and educators (Passow Citation2007; Shuman et al. Citation2005), Gilbuena and colleagues (Citation2015) argue that such limited consensus will include communication (written and oral), teamwork, project management, and self-awareness.
However, looking at synonyms for these skills affords an understanding of how the concept is discursively bounded: professional (in the sense of general), soft, social, transferrable, or non-technical skills. Thus, the concept of a skill set of these terms is necessarily understood in relation to their opposites. The discourse around engineering skills has often distinguished between technical or professional skills, even though this has long been argued to constitute a false dichotomy (Bucciarelli Citation1994; Downey Citation1998; Trevelyan Citation2007, Citation2014; Vinck Citation2003). Shuman et al. (Citation2005) argued that this dichotomised view can be seen in the ABET criteria, stating that while the ABET professional skills can be taught, such teaching must be cognizant of teaching engineering in its appropriate context (51). This principle was observed in student behaviour by Gilbuena and colleagues (Citation2015), who while investigating professional skills in capstone design projects found an interplay between professional skills activities and technical activities. While conducting an interview study on the preparedness of students for practice, Martin and colleagues (Citation2005) also found that a clear link existed between technical competency, and communication and teamwork (177); they concluded that The implications for curriculum development are that the non-technical skills can not be taught in isolation from the technical context in which they will be used (179). Despite this, as Jesiek et al. (Citation2017, 1) argued, engineering education still keeps technical and non-technical learning separate, which they see as artificial and arguably counterproductive. Passow and Passow (Citation2017) provide strong indirect empirical support to this inseparability of the technical and social in their thorough review of engineering education research on needed competencies, stating that the most striking finding in this systematic review is that technical competence is inseparably intertwined with effective collaboration (see finding 2, 491).
This is where the study arrives at accepting the integrative-view of skill, where Professional Skills do not refer to a single well-defined skill set, but rather to a wide range of value-adding social performances (Trevelyan and Williams Citation2018). Establishing an operating definition for this study, these performances constitute an ability to enact technical knowledge, through social networks of professional actors, by continually recognising the present legitimate basis for practice. These three parts build on each other: Seeing the legitimate basis enables collaboration in networks, which in turn enables the enactment of technical knowledge (see ).
This view, together with needed technical knowledge, constitutes Engineering Expertise. This relates closely to Passow and Passow’s (Citation2017) portrait of engineering practice from the previous section. Discussing whether professional skills are important or not might thus miss the point of engineering expertise: Different aspects or types of professional skills might vary in importance depending on the work context of engineers (Winberg et al. Citation2018, 168), who for instance belong to different engineering disciplines and career-phases. The integrative-view and operating definition motivate a questioning of the assumption of the universality-view of professional skills. Especially for the early career (Brunhaver et al. Citation2018).
Indeed, the phenomena of professional/soft/social/non-technical skills seem to be both integrated and integrating, in that it is an integrated skill set with a context-dependent composition that in turn integrates technical knowledge with other practice dimensions. The last part of the operating definition (legitimate basis) is only indirectly present in the engineering practice portrait but is central to a social realist view of knowledge of-and-in practice. Therefore, theoretical support is needed to frame how professional skills might be clearly understood as a part of engineering expertise, which this view can afford.
2.3. Theoretical frame and hypothesis
The operating definition necessitates the recognition of what legitimate practice is based on in each situation for the engineer. The idea of different bases for legitimate engineering practice is close to Wolff’s (Citation2018) writing on ‘code-shifting’ between knowledge and practice contexts. Wolff (Citation2018) investigated the engineering theory-practice divide (embodied in the EPM paradox) in industrial problem-solving of mechatronics engineers by employing a social realist framework called Legitimation Code Theory (LCT) (Maton Citation2014). This is a multidimensional conceptual toolkit for researching knowledge-building. While problem-solving is not in itself the focus of the present work, LCT is particularly interesting for the integrative-view, more specifically Maton’s Specialisation Dimension and its visualisation in the specialisation plane (Maton Citation2014, 30): this dimension explores the basis of achievement underlying practices, dispositions and contexts, seeing knowledge-knower structures as constitutive of practices. The organising principles of these structures can be seen in relations along the two dimensions: epistemic relations (ER) and social relations (SR), which are combined to constitute a number of specialisation codes: knowledge codes focus on what is known, knower codes focus on who knows, élite codes focus on both, and relativist codes focus on neither (See ).
Figure 2. The specialisation plane (Maton Citation2014, 30).
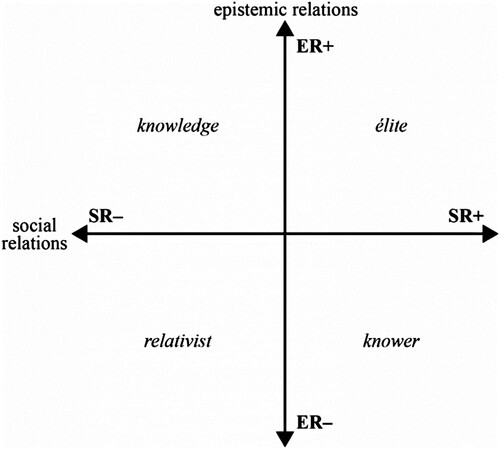
Specifically related to our aim of framing integrative understandings of professional skills, Winberg et al. (Citation2018, Figure 1) used this plane to map out engineering knowledge as knowledge codes and Professional Skills as knower codes when reviewing the EER literature on curricular elements promoting employability. Their findings (Winberg et al. Citation2018, Figure 2) of ‘Hard skills in soft contexts’ parallel the integrative-view of this study: that engineering knowledge (knowledge codes) and professional skills (knower codes) must be integrated when taught because these are integrated in practice (Èlite codes).
The Semantic dimension of LCT can serve to theoretically frame the universality-view. This dimension represents how context-dependent and complex legitimate meanings are in any given practice (Maton Citation2020). Specifically, the organising principles of the semantic structures are semantic gravity (SG) and semantic density (SD), denoting degrees of context-dependency and complexity, respectively (Maton Citation2016, 15). Relative strengths along SG and SD combine to constitute a number of semantic codes (see Citation2016, 16): rhizomatic codes (SG−, SD+) see meanings as complex but independent of context; prosaic codes (SG+, SD−) are context-dependent but have a simple view of meanings; rarefied codes (SG−, SD−) reduce meanings to context-independent and simple forms; and worldly codes (SG+, SD+), where meanings are context-dependent and complex (See ).
Figure 3. The semantic plane (Maton Citation2014, 131).
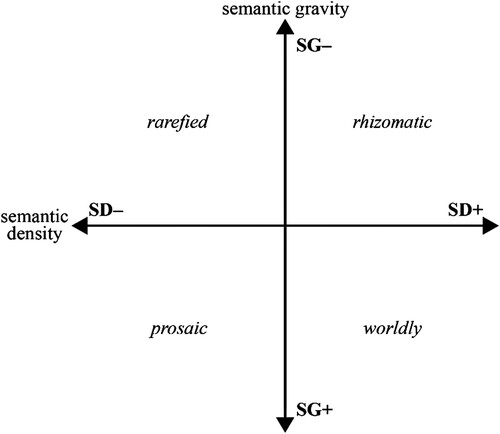
Maton (Citation2016, 17; Citation2020, 66) directly addressed the theory/practice-divide using semantic codes to describe and question the false dichotomy of the universality-view, stating that the theory/practice-divide … represent[s] rhizomatic codes (SG−, SD+) [i.e. Technical Skills] and prosaic codes (SG+, SD−) [i.e. Professional skills], respectively, and exclude the possibility of rarefied codes (SG−, SD−) and worldly codes (SG+, SD+) (Citation2016, 17). Here we can see engineering expertise as embedded with worldly codes that see expertise as a semantically … context-dependent stance that condense[s] manifold meanings (Citation2016, 16).
Seeing how specialization codes and semantic codes can be used together to analyse the same empirical data and offer complementary insights into the same phenonema (sic) (Maton Citation2016, 18), we apply the two LCT dimensions of Specialisation and Semantics to the understanding developed above on the nature of Professional Skills. From this application, two hypotheses can now be formulated with the purpose to nuance the assumptions of the universality-view while accepting the assumptions of the integrative-view, necessitating understanding professional skills in relation to engineering expertise. As Wolff (Citation2018, 193) found, we expect that early-career engineers will employ different specialisation codes to understand the legitimate basis of their practice, and what they perceive as constitutive of expertise. However, because students are taught a universality-view (SG–) of professional skills, despite their contextual-dependent (SG+) nature, various degrees of code-clash or code-match (Maton Citation2016, 13) on both the specialisation plane and the semantic plane should be expected. Such clashes are expected to arise between how early engineers understand their practice and the legitimate basis for that practice, depending on initial career direction. This can be embodied in perceived disciplinary role of the early engineer. We expect that the understanding and value placed on professional skills will differ over perceived disciplinary roles.
Hypothesis 1 – Early engineers acting in different engineering disciplines can ascribe a significantly different value to professional skills.
Given that we know from previous research that professional skills are important for engineering careers as a whole, we would expect that the code-clash between legitimation codes used should drift (See Maton Citation2016, 13) toward increasing code-match over time, evening out discursive differences on the issue of the perceived importance of the ‘non-technical’.
Hypothesis 2 – This difference in ascribed value will decrease over time in the initial career.
3. Methodology
This section starts with a description of the research process. It goes on to explains the choice of study population and methods for data collection and analysis, ending with a discussion of validity.
3.1. Study design process
This study employs the statistical testing and analysis of a two-part survey on early-career engineers from one graduate-degree programme. See for a visual representation of how the theory and methodology of the study relate to each other. The italicised arrow text boxes show how the study’s core concepts (square boxes) are connected. The rounded boxes show where in the text these are explained. The Theory-part of concertises concepts from Early-career misalignment down to Engineering Expertise, which is operationalised in the Methodology-part through the questionnaire. The Methodology-part details how the instrument, empirical context, collection, and analysis method relate to each other.
The study has two core design requirements that deserve explicit description, the first concerns the empirical context and sample, the second concerns how skills relate to expertise.
Design requirement #1: H1 and H2 motivated us to sample early-career engineers with identical and multidisciplinary educational backgrounds. Identical educational backgrounds are important because any differences found might otherwise be attributed to differences in formal educational background, and Multidisciplinary backgrounds are important because we want a sample of engineers acting in a broad range of professional engineering contexts. The empirical context and sample are further discussed in Section 3.2 below.
Design requirement #2: the literature review motivated us to think that, in both engineering education and practice, technical and non-technical (i.e. professional) skills are empirically inseparable and holistically blended into the construct of engineering expertise. Therefore, to enable an analysis of professional skills in an engineering practice context, we needed an instrument that measures expertise. Otherwise, any data received would be based on a manufactured divide of these two inseparable concepts. This would have seriously weakened the quality of our study, further discussed in Section 3.5.
Since it is better to use an existing instrument if it measures the thing in question, rather than develop our own instrumentation, we first searched for existing questionnaires and inventories that aimed to capture engineering expertise perception. Most available instruments were related to assessment using lists of competency-criteria such as ABET (c.f. Passow Citation2012; Passow and Passow Citation2017; Shuman et al. Citation2005), but in reading the work of James Trevelyan (‘The making of an expert engineer’, Citation2014, 17–18) we found a 12-item questionnaire probing how engineering expertise is understood. This questionnaire came with a grading scheme that awarded up to 10 points to each question, for a total score of 120 points to assess how much the respondent’s expertise-perception agreed with the book’s research on the topic. The questionnaire and grading scheme, which can be found in the appendix (Table A4), is further discussed in Section 3.3.
Trevelyan’s writings on engineering work (Trevelyan Citation2008b, Citation2014, Citation2019) and skill (Trevelyan Citation2008a, Citation2014; Trevelyan and Williams Citation2018) form a significant part of our position on how engineering expertise depends on the intertwining of technical and professional skills. Furthermore, his questionnaire and its grading scheme fit with the second design requirement. Therefore, we chose to implement it (Study Survey Part II in ) in a survey administered to a sample in our empirical context: junior mechatronics engineers. Study Survey Part I in probes career path and self-perceived roles of the respondent. In Part I, we address the first design requirement of situating the measurement in the early-career of engineers with similar educational backgrounds. Before developing the questions for Part I, we screened our entire sample of early-career mechatronics engineers manually through public information available on LinkedIn to assess what career path questions and role-alternatives seemed relevant to offer as answer options.
Subsequently, we assembled the items for the two parts into an electronic survey, pre-tested it for face validity, and sent it out to our sample (see Section 3.3). We divided the received data into two independent groups (see Section 3.4) and tested H1 by conducting an ANCOVA on the Part II-score, controlled for career length, between the groups. An independent U-test was conducted to understand what questions in Part II were driving the difference from the ANCOVA. We tested H2 using a Pearson’s correlation test between the groups to see if the differences identified for H1 decreased over time. This concludes the process description of the methodology.
3.2. Empirical context
To test the hypotheses, the study investigates a purposive sample of early-career engineers, consisting of alumni from a two-year Master’s programme in Mechatronics at KTH Royal Institute of Technology in Stockholm, Sweden. Mechatronics engineering is a half-century old (Bradley Citation2010, 828, Ch. 2) multidisciplinary engineering field of industrial practice consisting of mechanical, electronics, control, and software engineering. A central concept in mechatronics is the integration of its constituent engineering disciplines (Auslander Citation1996; Harashima, Tomizuka, and Fukuda Citation1996; Janschek Citation2012). However, few of our alumni practice as pure mechatronics engineers. Most transition into work as engineers within one of the constituent disciplines in a broad range of industrial contexts. Although students recruited to the Master’s programme have backgrounds in various Bachelor’s programmes, Mechanical Engineering is the most common. Since the BSc and MSc programmes were integrated into five-year programmes before the Bologna Process streamlined programme structures amongst its signatories (most of Europe), the Bachelor’s programmes (still) mainly prepare for the Master’s programme teaching basic math, science and the relevant prerequisite applied technical subjects. This purposive sample is thus ideal for testing the two hypotheses, as these alumni have the same formal educational background but ended up practicing in different engineering communities.
The mechatronics, mechanics, electronics, and control engineering roles filled by our alumni have remained relatively stable over time. However, the associated software-oriented roles have drastically changed and proliferated during the last decade, in general due to digitalisation. In our empirical context of mechatronics engineering design, this is due to the introduction of increasingly complex and software-intensive cyber-physical systems (Törngren et al. Citation2017). Indeed, in researching how mechatronics engineers engage in problem-solving, Wolff (Citation2018, 192) found that software-oriented (marked as ‘logic’, or ‘computer control’ (Figure 1 in Wolff Citation2018, 184)) participants preferred practice contexts where they could decide how to go about solving a static what. This indicated challenges in code-shifting, especially in environments where they were under constraints as to how to go about solving a problem. This reflects the view of our mechatronics alumni and poses an interesting avenue for grouping: self-perceived software-oriented roles and those who identify otherwise.
3.3. Data collection method
All 346 graduates from the mechatronics programme between 2011 and 2018 were sampled. While there are other somewhat similar university programmes in Sweden, the first design requirement of the research design necessitated a sample with the same formal educational background. As such, these 346 alumni constitute the total relevant population. Therefore, while statistical generalisations to all early-career engineers might not be easy to make based on this purposive sampling approach, theoretical/logical generalisations can be made (Patton Citation2002, 237).
The two-part electronic survey was pre-tested to ensure face validity. It was sent out via e-mail, which detailed the structure and purpose of the study. After the stated deadline expired, reminders were sent out. An acceptable response rate of 46% (n = 158, out of 346) was achieved. See Table A1: response rates in the appendix for a breakdown of the sample and response rates.
Part I of the survey measured career path, perceived work role, and competency profile through eight questions.Footnote6 These questions were selected based on a manual pre-screening of the sample to ensure relevance and content validity. Common roles in the pre-screening, together with the core disciplines of mechatronics engineering, constituted the available roles to choose from in Part I.
Part II uses the 12-itemFootnote7 questionnaire developed by James Trevelyan (Citation2014, 17–18). The engineering expertise questionnaire in Part II assesses to what degree that the respondent’s understanding of engineering expertise aligns with the skill-integrated view of expertise the study uses. Trevelyan’s questionnaire asks the respondent to what degree, on a five-point Likert scale, they agree with twelve statements about engineering practice. Each statement represents one or several frequent misconceptions that paint engineering practice as more objective, solitary, and hands-on than is typically the case. The misconceptions in Trevelyan’s book on engineering expertise are supportedFootnote8 by research such as the recent thorough review of competencies for engineering work by Passow and Passow (Citation2017). This is especially true in their findings that technical competence is inseparably integrated with social interactions (Finding 2), where engineers spend the majority of their time communicating (Finding 3), and that what is actually coordinated in these communications are the necessary multiple competencies (carried by different people) to enable engineering practice (i.e. problem-solving) to function (Finding 4). These findings by Passow & Passow match well with Trevelyan’s misconceptions of engineering expertise. All three see problem-solving as a fundamentally multidisciplinary exercise in collaboration that coordinates heterogenous skill sets at different phases of product development. It is around this view that the twelve statements in the Engineering Expertise questionnaire revolve. For each answer to the twelve statements, the grading schemeFootnote9 developed by Trevelyan assigns a point value that reflects the correctness of the position relating to the above view. Each answer can score up to 10 points, up to a max total of 120. The distribution of points over the five-point Likert-scale for each statement represents the approximately correct position to take on that statement according to the research that the book is based on. Several positions on the Likert-scale can afford full points for some statements, while for others the research takes a more polarised view (such as on statement 9: You can only learn communication skills by practice; they cannot be taught, which is a seen as a mostly false statement).
3.4. Data analysis method
The data was split into two independent groups. Group 1 (n = 62) consisted of those respondents who see their work-content as best described through the role of software developers, operationalised as the respondent ranking Software Developer (SW) as the first (n = 40) or second (n = 22) most important role ‘ … that best capture[s] what you actually do currently at work’ (PI-6 in Table A3). The reason for including those who ranked SW as the second most important role (see below) was primarily that none of these respondents ranked another core technical discipline (Mechanical/Electronic/Control) first. Conversely, the technical roles that were chosen above SW in importance describe a category that is related to SW: Embedded Systems Developer (n = 10), Mechatronics Engineer (n = 4), Systems Architect (n = 4), Test Engineer (n = 2). None of these represent a different dominant primary technical field from the perspective of the empirical context of mechatronics engineering.
Table 1. Breakdown of roles in group 1.
Group 2 (n = 97) consisted of those who rather see their work content best described with either the other core technical fields or more directly described as managerial. This group was operationalised as the remainder of the respondents who were not part of Group 1 (See ).
Table 2. Breakdown of roles in group 2.
To test Hypothesis 1 an ANCOVA was conducted between the two groups on the Engineering Expertise perception score from Part II, controlling for career length in line with the argument for Hypothesis 2. To understand the nature of the difference in detail, a Mann–Whitney U-test was performed to identify which individual questions in Part II drove the overall difference found by the ANCOVA test.
A correlation test between test score and career length was conducted to test Hypothesis 2: If a significant correlation could be observed for Group 1 but not Group 2, it would constitute supporting evidence for Hypothesis 2. This test is also needed as it shows the direction of any influence by career length. As discussed at the end of Section 2.3, such a correlation could then be analysed through the theoretical frame and understood as going from code clash to code match over the early career (see Section 5.4).
3.5. Validity concerns
The operating definition of professional skills in Section 2.2 argued for an integrated view of the technical and non-technical in engineering practice, leading to the use of the engineering expertise construct, on which the survey instrument measured. Using this construct instead of a dichotomised and itemised view of skills strengthens the content validity of the study. However, there remains the question of how to analytically distinguish between these two non-separable skill-dimensions when comparing the two groups on the engineering expertise construct. We argue that this construct can be used to validate the two hypotheses, specifically because we focus on early-career engineers. Due to the focus of engineering education on technical skills, the respondents are likely to be more mature regarding technical skill when transitioning into early-career engineers. Thus, the likelihood that the two groups would differ widely on technical skill by chance is low. Moreover, the fact that a candidate for a junior job position has graduated from an established engineering programme is an important factor for recruiting companies (Lang et al. Citation1999). In short, an engineering degree is accepted as a basic guarantee of technical skill, but not professional skill, for recent graduates. Indeed, this has been found to be the case in numerous surveys on the readiness of engineering graduates that systematically identified communication and teamwork as ‘competency gaps’ (c.f. Itani and Srour Citation2016; Meier, Williams, and Humphreys Citation2000; Passow Citation2012; Passow and Passow Citation2017), the implication of which Sageev and Romanowski (Citation2001, 690) boiled down to: Technical abilities are a given, communication and leadership differentiate.
All respondents were chosen from the same multidisciplinary engineering programme, mechatronics engineering, and as such share both basic technical (e.g. math/science) and advanced applied (e.g. electronics, embedded systems development) standardised curricular elements. Therefore, the observed differences should not stem from differences in their engineering education background, which strengthens internal validity. This is intentional, since what we wanted to investigate is whether differences in perceptions on professional skills can be found in the early career between different initial career paths. It is the existence, direction, and size of these differences themselves which are the focus, rather than the nature of the disciplines that the difference is found between. The nature of practice disciplines is influenced by so much more than its members’ educational backgrounds, which is what we control for in this study.
Another validity concern relates to the fact that the engineering expertise construct is based upon the assumption that real engineering work, contrary to common misconceptions (Trevelyan Citation2014, 48–54), contains little solitary design work and that the majority of worktime is spent in social interaction, especially forms of technical collaboration (Passow and Passow Citation2017, 491; Trevelyan Citation2007, Citation2014). Seeing how this is the nature of most engineering practice and a core part of the integrated view of engineering expertise taken in this study, there is a need to validate that the assumption of social interaction constituting the majority of meaningful work and work time holds for our empirical context. Otherwise, any difference found in the subsequent tests could be attributed to a potential difference in this assumption between groups, weakening internal validity. Therefore, we included a question that asked the respondent to estimate the amount of time spent in any kind of social interaction to solve a task or problem. The result in below supports this assumption, in line with, for example, Finding 3 of Passow and Passow (Citation2017), and therefore also taking the integration-viewpoint and using the engineering expertise construct. The distribution in is largely the same across the groups.
4. Results
The three tests are conducted to establish the existence of an overall difference in test score (the ANCOVA test), the nature of that difference (the U-test), and finally the direction of the difference (the correlation test). Table A2 in the appendix gives the mean score and standard deviation for each question in Part II for each group, and the figure below for the whole sample ().
4.1. Testing hypothesis 1
4.1.1. All questions
The ANCOVA compared the two groups on the Engineering Expertise perception score while controlling for career length. There was a linear relationship between test score and career length, as assessed by visual inspection of a scatterplot (see appendix “Scatterplot”). Standardised residuals were normally distributed, as assessed by Shapiro-Wilk’s test (p > .05). There was homoscedasticity and homogeneity of variances, as assessed by visual inspection of a scatterplot and Levene’s test of homogeneity of variance (p = .223). Since no cases in the data had standardised residuals outside of ±3 standard deviations, no outliers were found. After adjustment for career length, there was a statistically significant difference in test scores between the two groups, F(156, 1) = 5.4, p < .05, partial η2 = 0.033. Test scores were significantly smaller in Group 1 compared to Group 2, with a mean difference of 6.0 points (95% CI, 0.89–11), p < .05 ().
Since the purpose of this first test is to test the existence of a difference in total score, and the size of the difference is not the most important aspect here, the significant difference identified by the ANCOVA test is considered to support the first hypothesis. The next test drills down into the nature and extent of this difference between the groups.
Table 3. Comparisons between the two groups.
4.1.2. Individual questions
To determine which questions in part II contributed most to the observed test score difference, a Mann–Whitney U test was conducted on each question. Distributions of the scores for the groups were similarly shaped, as assessed by visual inspection. Question P2-1, P2-5, and P2-6 proved to be statistically significantly (p < .05) lower for the first group.
The size of the mean differences in should be understood in relation to the mean size of the scores of the two groups (see Table A2 in appendix): For question P2-1, Group 1 scored 55% lower than Group 2; For question P2-5, Group 1 scored 17% lower than Group 2; For question P2-6, Group 1 scored 55% lower than Group 2. Note the large differences for P2-1 and 6, which are not scored linearly and as such could be in danger of under-representing ‘close-to-right-answer’. The distributions of the answers (not scores of answers) over the two groups were visually inspected to ensure that this was not the case.
Table 4. Significant U-test result for three questions.
It is at this level, where it is known which questions drive the general difference, that the relative size of the results is relevant for the first hypothesis. Supported by the existence of a general difference in total score, and the relative size of this difference on the level of significantly distinct questions between groups, the first hypothesis is accepted.
4.2. Testing hypothesis 2
A Pearson’s correlation was conducted to test Hypothesis 2. The results are detailed in . A statistically significant (p < .05) correlation of 0.3 was found between test score and career length for Group 1, but not for Group 2.
Table 5. Correlations between career length and test score.
Interpretations of effect size must be related to the relevant context of both the variables in question as well as the research. There is a paucity of literature on effect sizes in engineering education, but a common benchmark often used in many research fields (outside its home of behavioural science) is that of Cohen (Citation1988) which presents a framework recommending Pearson’s r = .10, .30, and .50 to indicate small, medium, and large effects, respectively. However, recent critiques (Gignac and Szodorai Citation2016, 74) point out that Cohen’s labels were not developed from a systematic, quantitative analysis of data, such as in Hemphill (Citation2003). Some even go so far as to argue that this decontextualised framing of effect size is ‘nonsensical’ (Funder and Ozer Citation2020, 157–158). Funder and Ozer (Citation2020) suggest, based on Gignac and Szodorai (Citation2016)’s findings of 708 meta-reviewed correlations in social and personality psychology (of which this study is an application), considering an effect size r = .30 to indicate an effect that is large and potentially powerful in both the short and the long run in this context. Therefore, based on this guidance, we choose to interpret our effect size in personal difference on the engineering expertise construct of r = .30 as medium to large. The reason for being conservative here is the distance in research contexts and the preliminary nature of our results: This is the first quantitative study of a holistic measure of this kind in engineering education research.
Seeing the statistically significant and meaningful size of our results, hypothesis 2 is accepted.
5. Clashing views of the legitimate basis for expertise in practice
5.1. Analysis process
The previous section established the existence (H1: ANCOVA), nature (H1: U-test), direction (H1: ANCOVA & U-test), change (H2: Correlation), and size (H1: U-test, H2: Correlation) of how engineering expertise is perceived between the two groups. Thereby both hypotheses were supported. We now turn to the adopted theoretical frame of LCT, in terms of a number of legitimation codes, to interpret the nature and change of the identified difference. Having coded the nature of the difference, that is the three questions identified in Section 4.1.2, these codes will then be related back up to the overall construct of engineering expertise by relating them to the three parts of operating definition (Section 2.2): enacting technical knowledge, collaborating in social networks, and seeing the legitimate basis of practice (see ). In doing so, we have closed a circle starting from the general, down to particulars, and then relating those particulars back up again. The change of the difference will then be coded as a shift from code clash to code match to explain the hypothesised decrease of EPM over the early career.
5.2. Three themes
The three significant statements found driving the overall difference in total test score represent three distinct themesFootnote10: The first theme is academic performance bias (P2-1), which states that university grades have little to no effect on career performance. The second theme is technical expertise bias (P2-5), which states that while technical expertise is what distinguishes an engineer, success in engineering primarily depends on effective collaboration. The third theme is rationality bias (P2-6), which states that numbers are meaningless without words to describe what they signify, a description in words lies behind every number and assumptions about a physical process lie behind every measurement. The remaining Part 2 questions, which together constitute the overall difference, should not be understood as unimportant or identical in their perception over the two groups. Rather, that no relation could be found on these particular data with these particular methods. However, since it was established that together they matter (H1: ANCOVA), it is prudent to point out that no difference was found on the most prominent theme: collaboration. However, the second theme of technical expertise bias indirectly implicates a position toward collaboration, and as such is indirectly but weakly represented.
The three themes point to positions on both the specialisation plane and semantic plane. The former position denotes a code clash on how epistemic relations and social relations of engineering expertise are understood, and the latter represents a code clash on how the complexity and context-dependency of the technical and professional skills (which are constitutive of expertise) are understood.
In the first theme of Academic bias, Group 1’s semantic view of performance in practice has rhizomatic codes. They (correctly) see the importance of having learned technical skills in university, due to the technical complex nature of engineering problems (SD+). However, they fail to account for the context-dependent (SG+) nature of that importance for practice, that is, the requirements for enacting technical knowledge (), which imply worldly codes. Therefore, Group 1 is at risk of code-clash, mistakenly seeing the legitimate basis for practice through knowledge codes (‘if you know the right thing you will succeed’) even when other codes form that basis. This could also pose a challenge for required code-shifting behaviours in such cases.
For the second theme of technical expertise bias, Group 1 strongly positions the legitimate basis for practice on knowledge codes (ER+/SR-), seeing technical expertise as constitutive of stronger epistemic relations (ER+). However, since technical expertise is what distinguishes an engineer, indicating knower codes that focus on the importance of social relations and identity for professional legitimacy (SR+). In short, to be an engineer at all technical expertise is needed. However, to be a successful engineer (i.e. engineering expertise), collaboration is needed, indicating elité codes requiring both identity (SR+) and knowledge (ER+).
The last theme, rationality bias, is indicated weakly in the Specialisation dimension, but strongly in the Semantic dimension: Group 1 clearly displays rarefied codes in seeing numbers as more objective and unbiased than words. Maintaining rarefied codes, implying a simplified (SD−) and context-independent (SG−) semantic stance toward facts, should not be interpreted as incompetence in the related practice, but rather as a misunderstanding of the semantic structure of that practice. In engineering work, the semantic structure of facts about systems and processes is commonly context-dependent (SG+) and complex (SD+), indicating worldly codes. In other words, the engineer might implicitly understand both the complexities and dependencies inherent in a numerical measurement or data-point, without realising that such understanding cannot be taken for granted in others. This is usually not a problem in contexts where the legitimate basis for practice also rests on rarefied codes; for example, interacting with engineering colleagues that have the same implicit intimate knowledge about the current relevant fact(s). However, in contexts that require code-shifting behaviour (Wolff Citation2018) to other codes, the engineer would find it challenging to recognise the legitimate basis for practice, making collaborating to enact technical knowledge more difficult. This parallels, on the semantic plane, similar findings of Wolff (Citation2018, 192) on the epistemic plane.
5.3. Code clashes in the operating definition
In terms of the operating definition (), these three themes and their codes point to specific kinds of EPM problems for early practice in Group 1 compared to Group 2. The first theme indicates misalignment of the ability to dynamically recognise the ever-changing legitimate basis for practice. The second theme shows a gap for EPM on the role of collaboration. The third theme connects to problematic views on both the nature of collaboration and what constitutes legitimate practice. Unsurprisingly, no code clash was directly indicated toward understandings of the enactment of technical knowledge (the last box in ) in any of the three themes: This matches both the assumptions of initial basic technical competency (the universality-view) and the rhizomatic codes identified for the first theme as well as argued for by Maton (Citation2016, 17). This matches the view developed in Section 2 on the inseparability and contextuality of skill in engineering practice. The sources of possible EPM seem to be in the prerequisites of collaboration and legitimate basis for practice, and especially evident in the possibility for code-shifting challenges. summarises the code clashes that represent the specific nature of this EPM.
Table 6. Semantic and specialisation code clashes of themes in the operating definition.
It seems that Group 1 does not explicitly clash in understanding the importance of EPM on collaboration. However, the themes of technical bias and rationality bias indirectly indicate EPM on collaboration: The former express code clash in social relations in engineering practice and the legitimising function of an engineering identity based on having technical knowledge; the latter expresses a semantic code clash on the meanings of facts-in-practice, indicating possible rigidity regarding the code-shifting behaviour required for effective collaboration.
When it comes to misalignment in the ability to consciously perceive the legitimate basis for practice, the themes of academic bias and rationality bias seem to play into the difference between the two groups. The first theme indicates connected code clashes in both the Semantic and the Specialisation dimensions. In semantically holding rhizomatic codes in their understanding of the role of academic achievement, Group 1 seems to be at (relative) risk of a code clash in practice if maintaining knowledge codes in contexts where other codes form the legitimate basis for practice. Code-shifting challenges would probably ensue, which is also indicated by the code clash of the third theme. Here rarefied codes are deployed by Group 1 when understanding facts as representable in a simplified (SD-) and context-independent (SG-) manner in engineering practice, which goes against the understanding developed in this study of engineering expertise and how Group 2 answered.
Thus, Code-shifting challenges seem to be core to the identified code clashes in the operating definition.
5.4. Clash to match over the early career
One way to understand the code-shifting challenges is to relate them to the correlation test results that supported Hypothesis 2. In the medium-strong effect size of the result, a shift over time can be seen where both semantic perspectives on engineering skills and understandings of epistemic relations and social relations in engineering practice tend toward an integrative-view, resulting in increasing code match between the groups. This indicates a lessening of EPM on professional skills, as the concept is defined here, for Group 1. In the Semantic dimension, Group 1 seems to shift from rarefied codes (non-understanding or non-conscious understanding) or Rhizomatic codes (understanding complexity aspects, but not the context-dependency of that complexity) to Worldly codes. In the Specialisation dimension, a shift from knowledge codes (where knowing the right thing is seen as enough) toward Elité codes (where how you know it, being the right kind of knower, is equally important for expertise) is indicated for Group 1 relative to Group 2.
6. Discussion
The study starts from an integrative-view while questioning a universalised-view of engineering skill for the early career, leading to an operating definition of professional skills which integrates technical knowledge into engineering expertise. The purpose is to see if differing views of expertise could be found between initial career trajectories, indicating EPM on professional skills. Supported by the framework of two dimensions of LCT that theorise knowledge-knowers relations and context-complexity aspects of meaning, a survey methodology tests and finds support for two hypotheses of an initial (H1) but decreasing (H2) difference.
6.1. Elaboration on key findings
As expected, the primary difference/code-clash is not in the direct perception of technical knowledge, but in perceptions of collaboration and legitimate basis for practice. Surprisingly, collaboration was only indirectly indicated as clashing in two dimensions, technical and rationality bias. One way to interpret this is that when directly asked whether it is important to collaborate in engineering work, no one would say ‘no’. This parallels the established view in the literature that the issue is not whether or not ‘non-technical’ skills are important (Winberg et al. Citation2018, 167), but in what way and when. This salient context-dependency can be seen when asking about aspects that strongly relate to collaboration, such as rigid semantic perspectives on the nature of facts or when explicitly seeing the importance of knowledge but not the way it is important. In our results, the former can be seen in the rarefied codes VS worldly codes-clash for the rationality bias theme. Such simplified and context-independent views on the nature of facts in engineering work would challenge effective collaboration, paralleling work on the importance of empathy in engineering by Walther, Miller, and Sochacka (Citation2017). The latter can be seen in the relatively weaker social relations of the code-clash in the technical bias theme. The operating definition uses elité codes to understand the role of the technical knowledge for the engineer as comprising strong epistemic and social relations. The clash in this context imply that technical knowledge is only understood as having an internal and isolated value (ER+), missing the crucial identity-value (SR+) that technical knowledge constitutes for an engineer. Thus, an indirect misapprehension of collaboration is indicated.
This makes for a more detailed portrait of skill perceptions in early practice which can offer a preliminary explanation of the non-universality of professional skills in the early career: Knowing that collaboration is important and that the legitimate basis for practice does shift might be the same over early career trajectories, which was found to be the case in Passow and Passow (Citation2017, 487), but knowing how might differ. It might be argued that this is just a matter of gaining professional experience, as posited by Grimson and Murphy (Ch. 9 in Hyldgaard Christensen Citation2015, 170) in presenting a view of acquiring professional competence (top layer).
However, while professional formation crucially takes place after transitioning to engineering work and cannot be pushed on or fully translated into the curriculum, students should be prepared in ways that recognise the non-universal, situated, and integrative nature of professional skills. One way of doing this in the curriculum is through directed feedback on professional skills in the context of design work (Gilbuena et al. Citation2015), and another would be the direction recommended by Wolff (Citation2018, 192) to accept that non-universality of professional skills and contexts for problem-solving and instead focus on developing code-shifting behaviour by making the codes explicit. Indeed, Wolff’s suggestion is supported by our findings that code-clashes in both collaboration and legitimate basis for practice indicate code-shifting challenges in early practice. However, the question remains as to how to make the codes explicit. Wolff finishes by making a preliminary suggestion of issuing the same project brief to several student groups with varying affordances and constraints. This is an interesting practical point of departure to enact this principle, but to step out of context and into a more conceptual perspective, educators need an understanding of the codes and impart them to students (192). It is here that the operating definition can serve to motivate students as to why they should care about the codes: without an explicit understanding of codes, it is hard to explicitly perceive the legitimate basis for practice, in turn hampering collaboration and making the enactment of technical knowledge itself difficult. The need for students to learn about codes and the requisite shifting behaviour can be situated in terms of ‘solving the problem’ and as a way of ‘using your engineering know-how’.
While in need of further investigation, one way of achieving this would be to focus on showing the students (or letting them experience) the consequences of maintaining rarefied codes in the context of rationality bias. Not understanding the context-dependence of engineering data or their complexity severely affect the visibility of the legitimate basis of practice, which can be seen in the importance assigned to the concept of interiority as discussed by Cosgrove and O’Reilly (Citation2018, 45–46), arguing that a reflexive stance to one’s own understanding can mitigate this risk. Another way to understand the results in terms of enabling this code-shifting behaviour would be to explicate the consequences of Academic bias. This might seem counterintuitive at first to educators and students alike, but the importance here is not in stating that grades do not matter (they do), but in what way. The probability of finding real instances of clashes between knowledge codes and other specialisation codes during education is unlikely since most students and educators match specialisation codes in the context of academic bias. Therefore, it is important to impart a nuanced understanding of the role of grades to ensure that students know how to use them, as they will often stand as legitimising social relations and consequently indicating elite codes or knower codes. A practical, but possibly sensitive, way of exemplifying this to students is to discuss deeply embedded counter-productive student group work practices of ‘excluding inferiority’ (See Work Practice 8 in Figure 1, Leonardi et al. Citation2002). While being a difficult subject, this could provide a semantic basis for discussing perceptions of ‘incompetence’. A further example in the context of work placement of students can be seen in how the general development of professional skills are seen as unrelated to grading and other assessments (Bennett, Richardson, and MacKinnon Citation2016; Edwards et al. Citation2015, 35–51). The reasons for this seem to often relate to not maintaining an integrative-perspective, either from lack of industrial context knowledge on the part of educators or a view that students professional skills development are their own responsibility (Amiet et al. Citation2020).
The implication of the shift found in Hypothesis 2, where social relations (understanding identity-legitimacy) and semantic gravity (understanding context-dependency) first clash but strengthen over time, can also be used to motivate the importance of code-shifting to educators and students. The importance of foregrounding social relations and semantic gravity as a possible differentiating factor for early practice can be motivated by Finding 6 in Passow and Passow’s review (Citation2017, 498–499) which states that, while problem-solving is the core overarching task of engineers, what separates ‘ordinary’ from ‘outstanding’ engineers is effective coordination of other skills (499). This requires understanding and dynamically shifting along strengths of social relations as well as semantic gravity. Thus, at the tail end of a master’s programme, the engineering student is expected to be nominally capable in problem-solving but needs to understand (even if phrased differently pedagogically) that this is the general base layer for practice and that dimensions of Specialisation and Semantics must be understood for proper shifting in practice, especially over strengths of SR and SG. This is significant in the context of, for example, establishing a functional mentoring relationship during work placement between a student and a senior engineer, which is important for working efficiently (Davis, Vinson, and Stevens Citation2017). Such relationships are most common where responsibility is shared and hierarchy is weak (Davis et al. Citation2017).
6.2. Limitations
The preliminary nature of this work cautions against making broad claims about the nature of professional skills in general, and for any specific engineering discipline, including software-oriented ones (such as in Group 1). The reason for this is the same as the departure of this study in the two views of integration and non-universality: The nature of each discipline and its knowledge base must be considered together with the practice context in which these are deployed, including such diverse considerations as industry-type, firm size, department type, kind of engineering work, level of ‘projectification’, and (inter)national contexts. This is less of a problem for the study itself since the intention is to focus on the nature of difference itself, rather than the disciplinary fields. With that said, some comments can tentatively be made on the relation of the software-oriented roles of Group 1 to the results. Regarding the lower initial score, it is unlikely that early software engineers see engineering expertise differently than other engineers do because their professional practice is more bound to good grades, blind faith in numbers, or that they see technical expertise as the be-all-end-all of engineering (Bjørnson and Dingsøyr Citation2008): that it is not a question of misalignment of professional skills as such, but that software engineer communities of practice do not require as much, or the same kind of, emphasis on professional skills for legitimate peripheral participation (which would reflect the non-universality principle). In other words, the legitimate basis for practice might (initially) correctly be seen through knowledge codes. Nevertheless, the longer one is a member of such a community, the greater the increase in the benefits of these skills (Lee, Citation1994), which is supported by Hypothesis 2.
6.3. Implications for engineering educators and industrial actors
Engineering educators should be mindful to motivate the need for professional skills to students, as they can end up in different practice contexts. It might be discouraging if the emphasis on professional skills seems not to be reflected in one’s first workplace. However, practice-oriented parts of curricula can never fully represent actual practice, but can be valuable in making own attitudes visible to students and changing them (Paretti Citation2008). Gilbuena et al. (Citation2015) exemplified how this enculturation into communities of practice can start already during education through feedback on professional skills situated in senior design capstone courses. As such, effective attitude change strategies must be built on an understanding of the more frequent initial career tracks of students in any programme because attitude is essential to learning professional skills (Byrne et al. Citation2018).
Industrial actors should not only consider that ‘students have not been taught what they need’ when looking at the curricula of higher education institutions. Early-career engineers might be entirely forgiven for pushing back on suggestions that they lack in skill if neither their work context nor their social context challenges them on these skills. Newcomers cannot simply be told what constitutes expertise in their new practice context but must participate in the doings that constitute and necessitate such expertise.
Future research could dive into developing a deeper understanding of the semantic codes and specialisation codes of the three themes. An interview study between the two groups could achieve this. Another interesting avenue would be to further investigate the nature of this variability in early career misalignment: Does it differ for other fields? Are they predisposed to certain trajectories for initial misalignment, as is indicated for software developers in this work? Does it differ in other possible measurements of misalignment than skills, such as empathy (Walther et al. Citation2017)?
7. Concluding comments
The transition from education to practice is still jarring for many students. This study shows that misalignment might exist along different themes depending on the engineering practice context that new engineers join, and as such makes a theoretical contribution in questioning an isolated and universal view of non-technical skills for early practice. The practical contribution thus becomes an invitation to educators to strengthen attitude change strategies of professional skills, through an understanding of codes and code-shifting behaviours in and for students. Finding ways to show and motivate understanding engineering practice through legitimation codes might give early engineers an edge in their core activity of problem-solving, as was found by Wolff (Citation2018, 192) in practicing engineers, if they can leverage this conceptual understanding into their practice.
Disclosure statement
No potential conflict of interest was reported by the author(s).
Correction Statement
This article has been corrected with minor changes. These changes do not impact the academic content of the article.
Additional information
Notes on contributors
Elias Flening
Elias Flening is currently pursuing his PhD at the Division of Mechatronics at KTH Royal Institute of Technology. He achieved a MSc in Industrial Engineering and Management from KTH in 2014, and has worked as a research assistant at the Stockholm School of Economics. Research interests include engineering design processes, engineering education and project management in the mechatronics domain.
Fredrik Asplund
Fredrik Asplund received his PhD at the KTH Royal Institute of Technology in 2014, has been a Postdoctoral Researcher within the Mobility for Growth programme, and was employed as an Assistant Professor at KTH Royal Institute of Technology in 2020. Throughout his academic positions he has taught courses in research methodology and supervised students at the master's level. Research interests include system safety, empirical software engineering and engineering education in the Cyber-Physical Systems domains. Dr Asplund is a Marie Curie Fellow and a VINNMER Fellow since his postdoctoral research at Rolls-Royce plc in Derby, UK.
Martin Edin Grimheden
Martin Edin Grimheden is associate professor and dean at the Machine Design department at KTH Royal Institute of Technology. Dr Grimheden has a combination of degrees in Engineering and Education. He has been heavily involved in the transition into the BSc/MSc-system at KTH. His current research interest includes studies of learning in higher education, of learning in Mechatronics, and of internationalization of higher education.
Notes
3 Here we mean “course” in the sense of a self-contained unit of learning, a structured number of which constitute a degree program, rather than in the UK sense where “course” means the degree program itself.
4 The student might have less stable required knowledge going into the next course, which obviously can worsen performance, but the requirements put on the student does not change: Courses are (by design) independent environments of educational practice. However, development projects in engineering firms are not.
5 See the broad base of international signatories of the Magna Charta Universitatum, proclaiming academic freedom and institutional autonomy principles for universities: http://www.magna-charta.org/magna-charta-universitatum.
6 The pre-screening resulted in the inclusion of questions 1-4, and 8 into Part I. These were not used in the final analysis, but they have been included in the appendix for transparency.
7 We originally included four extra questions on top of the original twelve in Part II, but these are not used in the final analysis and are not a part of the scoring system which was designed by Trevelyan. They have been included in the appendix for transparency.
8 Specifically misconceptions #1-5, #8, #10-12, and #15 in Trevelyan’s book.
9 See the rightmost column-matrix of table 10 in the appendix for point-distributions for each statement. Trevelyan has made the grading scheme, together with a discussion of its point-distributions, available as an online appendix to his book The making of an expert engineer.
It is downloadable from the publisher under the file name App12-1-Practice Quiz 2 Self-assessment 140807 in a compressed folder found here: https://www.routledge.com/downloads/K24392/K24392_Appendices.zip.
10 The italicized explanations of each theme are directly cited from Trevelyan’s discussion motivating the grading of each of these three statements (P2-1, 5, and 6 in table 10), again found under the file name App12-1-Practice Quiz 2 Self-assessment 140807 at the download-link: https://www.routledge.com/downloads/K24392/K24392_Appendices.zip.
References
- Amiet, D., J. Choate, J. Hoskin, and J. Dart. 2020. “Exploring Attitudes, Beliefs and Practices of Academic Staff Towards Undergraduate Career Development in Non-vocational Courses.” Higher Education Research & Development, 40: 1–16.
- Anderson, K. J. B., S. S. Courter, T. McGlamery, T. M. Nathans-Kelly, and C. G. Nicometo. 2010. “Understanding Engineering Work and Identity: A Cross-Case Analysis of Engineers Within Six Firms.” Engineering Studies 2 (3): 153–174. doi:10.1080/19378629.2010.519772.
- Auslander, D. M. 1996. "What is mechatronics?" IEEE/ASME Transactions on Mechatronics 1: 5–9.
- Bennett, D., S. Richardson, and P. MacKinnon. 2016. Enacting Strategies for Graduate Employability: How Universities Can Best Support Students to Develop Generic Skills. Sydney: Australian Government Office for Learning and Teaching.
- Bjørnson, F. O., and T. Dingsøyr. 2008. “Knowledge Management in Software Engineering: A Systematic Review of Studied Concepts, Findings and Research Methods Used.” Information and Software Technology 50 (11): 1055–1068. doi:10.1016/j.infsof.2008.03.006.
- Bradley, D. 2010. “Mechatronics – More Questions Than Answers.” Mechatronics 20 (8): 827–841. doi:10.1016/j.mechatronics.2010.07.011.
- Brunhaver, S. R., R. F. Korte, S. R. Barley, and S. D. Sheppard. 2018. “Bridging the Gaps Between Engineering Education and Practice.” In U.S. Engineering in a Global Economy, edited by R. B. Freeman and H. Salzman, 129–163. University of Chicago Press.
- Bucciarelli, L. L. 1994. Designing Engineers. Cambridge, MA: MIT Press.
- Byrne, Z. S., J. W. Weston, and K. Cave. 2018. “Development of a Scale for Measuring Students’ Attitudes Towards Learning Professional (i.e., Soft) Skills.” Research in Science Education 50 (4): 1417–1433. doi:10.1007/s11165-018-9738-3.
- Cappelli, P. 2008. Talent on Demand: Managing Talent in an Age of Uncertainty. Boston: Harvard Business Press.
- Cohen, J. 1988. Statistical Power Analysis for the Behavioral Sciences. 2nd ed. New York: Taylor & Francis.
- Colwell, J. 2010. “Soft Skills for the New Economy: Their Place in Graduate Education in Engineering and Engineering Technology.” Paper Presented at the ASEE Conference and Exposition, Louisville.
- Cosgrove, T., and J. O’Reilly. 2018. “Theory, Practice and Interiority: An Extended Epistemology for Engineering Education.” European Journal of Engineering Education 45 (1): 38–54. doi:10.1080/03043797.2018.1544226.
- Davis, P., A. Vinson, and R. Stevens. 2017. “Informal Mentorship of New Engineers in the Workplace.” Paper presented at 2017 ASEE Annual Conference & Exposition, Columbus, Ohio. doi:10.18260/1-2–28527.
- Downey, G. L. 1998. The Machine in Me: An Anthropologist Sits Among Computer Engineers. New York: Routledge.
- Downey, G. L. 2015. “The Normative Contents of Engineering Formation: Engineering Studies.” In Cambridge Handbook of Engineering Education Research, edited by A. Johri and B. M. Olds, 693–712. Cambridge University Press.
- Edström, K., and A. Kolmos. 2014. “PBL and CDIO: Complementary Models for Engineering Education Development.” European Journal of Engineering Education 39 (10): 539–555.
- Edwards, D., K. Perkins, J. Pearce, and J. Hong. 2015. “Work Integrated Learning in STEM in Australian Universities.” Final Report: Submitted to the Office of the Chief Scientist.
- Funder, D. C., and D. J. Ozer. 2020. “Evaluating Effect Size in Psychological Research: Sense and Nonsense.” Advances in Methods and Practices in Psychological Science 3 (4): 509–509. doi:10.1177/2515245920979282.
- Gignac, G. E., and E. T. Szodorai. 2016. “Effect Size Guidelines for Individual Differences Researchers.” Personality and Individual Differences 102: 74–78. doi:10.1016/j.paid.2016.06.069.
- Gilbuena, D. M., B. U. Sherrett, E. S. Gummer, A. B. Champagne, and M. D. Koretsky. 2015. “Feedback on Professional Skills as Enculturation into Communities of Practice.” Journal of Engineering Education 104 (1): 7–34. doi:10.1002/jee.20061.
- Harashima, F., M. Tomizuka, and T. Fukuda. 1996. "Mechatronics - 'What Is It, Why, and How?'" An editorial. IEEE/ASME Transactions on Mechatronics 1: 1–4.
- Hemphill, J. F. 2003. “Interpreting the Magnitudes of Correlation Coefficients.” American Psychologist 58 (1): 78–79. doi:10.1037/0003-066x.58.1.78.
- Hyldgaard Christensen, S. 2015. Engineering Identities, Epistemologies and Values: Engineering Education and Practice in Context: Vol. 2. Springer.
- Itani, M., and I. Srour. 2016. “Engineering Students’ Perceptions of Soft Skills, Industry Expectations, and Career Aspirations.” Journal of Professional Issues in Engineering Education and Practice 142 (1): 1–12. doi:10.1061/(asce)ei.1943-5541.0000247.
- Janschek, K. 2012. "Mechatronic Systems Design." Computer Applications in Engineering Education 4.
- Jesiek, B. K., N. Trellinger, and S. Nittala. 2017. “Closing the Practice Gap: Studying Boundary Spanning in Engineering Practice to Inform Educational Practice.” Proceedings – Frontiers in Education Conference, FIE, 1–9, October. doi:10.1109/FIE.2017.8190503.
- Korte, R., S. Brunhaver, and S. Sheppard. 2015. “(Mis)Interpretations of Organizational Socialization: The Expectations and Experiences of Newcomers and Managers.” Human Resource Development Quarterly 26 (2): 185–208. doi:10.1002/hrdq.21206.
- Lang, J. D., S. Cruse, F. D. McVey, and J. McMaster. 1999. “Industry Expectations of New Engineers: A Survey to Assist Curriculum Designers.” Journal of Engineering Education 88 (1): 43–51.
- Lee, D. M. S. 1994. “Social Ties, Task-Related Communication and First job Performance of Young Engineers.” Journal of Engineering and Technology Management 11 (3–4): 203–228. doi:10.1016/0923-4748(94)90010-8.
- Leonardi, P. M., M. H. Jackson, and A. Diwan. 2002. “Rationalization and the Persistence of Counter-Productive Technology Design Practices in Student Engineering.” Academy of Management Journal 52: 211–248.
- Martin, R., B. Maytham, J. Case, and D. Fraser. 2005. “Engineering Graduates’ Perceptions of How Well They Were Prepared for Work in Industry.” European Journal of Engineering Education 30 (2): 167–180. doi:10.1080/03043790500087571.
- Maton, K. 2014. Knowledge and Knowers: Towards a Realist Sociology of Education. London: Routledge.
- Maton, K. 2016. “Legitimation Code Theory: Building Knowledge About Knowledge-Building.” In Knowledge-building Educational Studies in Legitimation Code Theory, 1–255. London: Routledge.
- Maton, K. 2020. “Semantic Waves: Context, Complexity and Academic Discourse.” In Accessing Academic Discourse Systemic Functional Linguistics and Legitimation Code Theory, edited by J. R. Martin, K. Maton, and Y. J. Doran, 59–85. London: Routledge.
- Meier, R. L., M. R. Williams, and M. A. Humphreys. 2000. “Refocusing Our Efforts: Assessing Non-technical Competency Gaps.” Journal of Engineering Education 89 (3): 377–385. doi:10.1002/j.2168-9830.2000.tb00539.x.
- Michael, D. 1998. Thinking Like an Engineer.
- Paretti, M. C. 2008. “Teaching Communication in Capstone Design: The Role of the Instructor in Situated Learning.” Journal of Engineering Education 97 (4): 491–503. doi:10.1002/j.2168-9830.2008.tb00995.x.
- Pascail, L. 2011. “The Emergence of the Skills Approach in Industry and its Consequences for the Training of Engineers.” European Journal of Engineering Education 31 (1): 55–61. doi:10.1080/03043790500428965.
- Passow, H. J. 2007. “What Competencies Should Engineering Programs Emphasize? A Metaanalysis of Practitioners’ Opinions Informs Curricular Design.” Paper Presented at the 3rd International CDIO Conference. http://www.cdio.org/knowledge-library/documents/what-competencies-should-engineering-programs-emphasize-meta-analysis–0.
- Passow, H. J. 2012. “Which ABET Competencies Do Engineering Graduates Find Most Important in Their Work?” Journal of Engineering Education 101 (1): 95–118. doi:10.1002/j.2168-9830.2012.tb00043.x.
- Passow, H. J., and C. H. Passow. 2017. “What Competencies Should Undergraduate Engineering Programs Emphasize? A Systematic Review.” Journal of Engineering Education 106 (3): 475–526.
- Patton, M. Q. 2002. Qualitative Research and Evaluation Methods. 3rd ed. Thousand Oaks: SAGE Publications.
- Rogers, P., and R. Freuler. 2015. “The ‘T-Shaped’ Engineer.” Paper Presented at the 122nd ASEE Annual Conference & Exposition, Seattle, June 14–17.
- Sageev, P., and C. Romanowski. 2001. “A Message from Recent Engineering Graduates in the Workplace: Results of a Survey on Technical Communication Skills.” Journal of Engineering Education 90: 685–693.
- Shuman, L. J., M. Besterfield-Sacre, and J. McGourty. 2005. “The ABET “Professional Skills” – Can They Be Taught? Can They Be Assessed?” Journal of Engineering Education 94 (1): 41–55. doi:10.1002/j.2168-9830.2005.tb00828.x.
- Törngren, M., F. Asplund, S. Bensalem, J. McDermid, R. Passerone, H. Pfeifer, … B. Schätz. 2017. “Characterization, Analysis, and Recommendations for Exploiting the Opportunities of Cyber-Physical Systems.” In Cyber-Physical Systems, edited by Houbing Song, Danda Rawat, Sabina Jeschke, and Christian Brecher, 3–14. Academic Press.
- Trevelyan, J. 2007. “Technical Coordination in Engineering Practice.” Journal of Engineering Education 96 (3): 191–204. DOI 10.1002/j.2168-9830.2007.tb00929.x.
- Trevelyan, J. 2008a. “Coordination in Mechatronic Engineering Work.” In Mechatronics and Machine Vision in Practice, edited by J. Billingsley, and R. Bradbeer, 51–61. Springer.
- Trevelyan, J. 2008b. “The Intertwined Threads of Work.” Engineers Australia 80 (2): 38–39.
- Trevelyan, J. 2014. The Making of an Expert Engineer, 574. CRC Press.
- Trevelyan, J. 2019. “Transitioning to Engineering Practice.” European Journal of Engineering Education 44 (6): 821–837. doi:10.1080/03043797.2019.1681631.
- Trevelyan, J., and B. Williams. 2018. “Value Creation in the Engineering Enterprise: An Educational Perspective.” European Journal of Engineering Education 3797, 1–23. Taylor & Francis.
- Vinck, D. 2003. Everyday Engineering: An Ethnography of Design and Innovation. Cambridge, MA: MIT Press.
- Walter, V. 1990. What Engineers Know and How They Know It. The Johns Hopkins University Press.
- Walther, J., S. E. Miller, and N. W. Sochacka. 2017. “A Model of Empathy in Engineering as a Core Skill, Practice Orientation, and Professional Way of Being.” Journal of Engineering Education 106 (1): 123–148. doi:10.1002/jee.20159.
- Winberg, C., M. Bramhall, D. Greenfield, P. Johnson, P. Rowlett, O. Lewis, … K. Wolff. 2018. “Developing Employability in Engineering Education: A Systematic Review of the Literature.” European Journal of Engineering Education 45 (2): 165–180. doi:10.1080/03043797.2018.1534086.
- Wolff, K. 2018. “Researching the Engineering Theory-Practice Divide in Industrial Problem Solving.” European Journal of Engineering Education 45 (2): 181–195. doi:10.1080/03043797.2018.1516738.
Appendix
Two-part survey on engineering career and perceptions of expertise
Response rates for the whole sample and the two groups
Table A1. Response rates.
Table A2. Test score results for the two groups.
Part 1: questions regarding career path and work-content
Table A3. Survey part I – career path and role.
Part 2: statements probing opinions about engineering expertise in practice
Part 2 uses a five-point Likert scale with the options strongly disagree, disagree, neutral, agree, strongly agree for the respondent to take a position on each of the twelve statements. In the scoring table column, the grading schemes assigns a point-value for each answer on the Likert scale.
The following four questions were added to the original twelve from the questionnaire. In this article only the original twelve were used in the analysis. They are included here for completeness sake: Skill in building collaborative relationships with other engineers to solve technical problems are more important than skill in solving the same problems yourself; If you give a concise, rational argument based on quantified facts you will convince others; Understanding the reason for investment and budget decisions in a product development project is important for the engineer working in that project; Expert engineers solve technical problems by independently developing their technical competence without help so they can address the problem by themselves.
Table A4. Survey part II – Trevelyan’s (Citation2014) engineering expertise questionnaire.