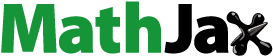
ABSTRACT
Drawing upon data on the entire population of French STEM PhD students, we explore the factors leading PhDs to pursue independent research from their supervisors during the PhD and how independence links to their career outcomes. We find that independence is significantly associated with students’ and supervisors’ characteristics. Moreover, students’ independence predicts the probability of starting an academic career and, conditional on starting an academic career, a higher number of articles published after the PhD period. However, the higher number of articles comes at the cost of receiving fewer citations and having a lower probability of obtaining an academic position outside France.
1. Introduction
When launching a collaborative project, make sure that each collaborator’s role is defined so that each can be perceived to be an independent contributor. […] Be cautious about collaborating all the time. As much as collaborative research is valued, review committees are looking for evidence that you can be an independent scholar (The University of Arizona Citation2009).
Fellows are selected on the basis of their independent research accomplishments, creativity, and potential to become leaders in the scientific community through their contribution to their field (Alfred P. Sloan Foundation Citation2022).
A common thread through the quotes reported above describing the selection criteria in hiring and grant selection committees is the candidate’s ability to demonstrate research independence (Alfred P. Sloan Foundation Citation2022; The University of Arizona Citation2009). Universities and funding agencies include independence as one of the most prominent characteristics revealing young candidates’ aptitude to make a significant scientific contribution (Daniels Citation2015; Hamilton et al. Citation2013; Heggeness et al. Citation2017; Levine Citation2007; National Research Council Citation2005). Commonly recognized as a gendered trait, independence is expected in male scientists (Schiffbaenker, Haas, and Holzinger Citation2022), while the current policy debate is looking for policy instruments to support women in reaching their independence (Nguyen et al. Citation2023).
A career step crucial in developing independence is the PhD period, during which students are trained by their supervisors to become independent researchers (Bozeman and Corley Citation2004; Campbell Citation2003; Gardner Citation2008; Horta and Santos Citation2016; Laudel and Gläser Citation2008; Stephan Citation2012). A key takeaway from the previous literature that has studied how students develop independence suggests that the student-supervisor relationship is a delicate balance between giving help and not interfering too much with the student’s work (Bastalich Citation2017; Gardner Citation2008; Lee, Dennis, and Campbell Citation2007). Moreover, previous literature suggests that independence can take different forms relating to the organizational and cognitive aspects of scientists’ work (Laudel and Gläser Citation2008; Yoshioka-Kobayashi and Shibayama Citation2020). Cognitive independence, i.e. young scientists’ capacity to autonomously define and pursue a research line, is considered the most important (Daniels Citation2015; National Research Council Citation2005).
However, despite the widespread belief that the PhD training is crucial in guiding students to become independent researchers, there is a lack of knowledge of the specific factors leading students to develop cognitive independence. Moreover, the link between cognitive independence and career achievements is often taken for granted, although only a handful of large-scale empirical studies have investigated the subject (Shibayama Citation2019; Yoshioka-Kobayashi and Shibayama Citation2020). Finally, although disciplinary and institutional cultures are expected to play a crucial role in driving independence and moderating its impact, there is a lack of comparative studies across disciplines (Gardner Citation2008).
Our study has the twofold aim of providing empirical evidence on the factors leading to cognitive independence during the PhD period and exploring the association between research independence and the likelihood of pursuing and succeeding in academia after the PhD. Our analysis leverages a large-scale dataset on the entire population of STEM students in France who graduated between 2004 and 2013 in STEM disciplines. Using a neural network algorithm to interpret the semantic meaning of words, we construct a measure of student cognitive independence by calculating the dissimilarity between 42,630 thesis abstracts and 295,596 supervisor’s publication abstracts. We focus our analysis on the student’s thesis and supervisor’s publications because these documents are good proxies for the first student’s achievement as a researcher (Gardner Citation2008) and for the supervisor’s research lines, respectively.
Looking at the factors leading to cognitive independence, we find that young French male students show high levels of independence. High levels of independence also characterize students supervised by mentors performing low-impact research, with a limited collaboration network, and not publishing with their protégé during the PhD period. When exploring the association between cognitive independence and students’ careers, we find that cognitive independence is positively associated with the probability of starting an academic career and, conditional on starting an academic career, with a higher number of publications. However, for independent students, the higher scientific productivity in terms of quantity comes at the cost of receiving fewer citations and having a lower probability of obtaining an academic position in a foreign country or the US. We find that our results concerning the determinants of independence are largely homogeneous across disciplines. On the contrary, independence is positively associated with the probability of starting an academic career in Mathematics and Medicine-biology-chemistry, but not in Engineering and Physics. Moreover, conditional on starting an academic career, we observe that independence is negatively associated with career outcomes in Engineering and Medicine-biology-chemistry, but not in Mathematics and Physics, where independence is positively associated with career outcomes.
Our paper contributes to the extant literature by studying PhD students’ cognitive independence across all STEM fields and universities in a large European country. Within this empirical context, it sheds light for the first time on the factors associated with students’ independence. Moreover, it expands what we know about the relationship between cognitive independence and career by analyzing a broad range of outcomes. Finally, differently from previous literature that relies on bibliometric indicators to measure independence (Shibayama Citation2019; van den Besselaar and Sandström Citation2019), our paper proposes the use of a neural network algorithm to compare the content of the PhD thesis text with the content of supervisors’ publications (Furman and Teodoridis Citation2020; Gentzkow, Kelly, and Taddy Citation2019).
The rest of the paper is organized as follows. Section 2 reviews the literature on independence. Section 3 presents our empirical context, data, measure of independence, and empirical strategy. Section 4 illustrates the results. Section 5 reports a discussion of our results and concludes.
2. Literature review
The PhD training is a career step crucial in developing scientists’ independence. During the PhD training, young scholars acquire knowledge and nurture their skills to become independent researchers (Bozeman and Corley Citation2004; Campbell Citation2003; Gardner Citation2008; Horta and Santos Citation2016; Laudel and Gläser Citation2008; Stephan Citation2012). The supervisor role is crucial in this transformation process from dependent to independent researchers (Bozeman and Corley Citation2004; Gardner Citation2008; Hamilton et al. Citation2013; Laudel and Gläser Citation2008; Lee Citation2008; Liénard et al. Citation2018). Supervisors contribute to students’ training by providing psychological mentoring, acting as role models, and fostering the students’ professional self-confidence (Bastalich Citation2017; Kam Citation1997). Supervisors also provide concrete career support, helping to find solutions to challenging research problems, starting collaborations on research projects, and introducing the students to relevant professional networks (Corsini, Pezzoni, and Visentin Citation2022; Hilmer and Hilmer Citation2009; Paglis, Green, and Bauer Citation2006). The relationship between supervisor and student follows an apprenticeship model in which students learn from their supervisors how to become independent researchers (Laudel and Gläser Citation2008). Specifically, the supervisors devote time and effort to students’ training activities. In exchange, students compensate their supervisors by contributing as a scientific workforce to advancing their research projects (Shibayama Citation2019; Stephan and Levin Citation2002).
The student-supervisor relationships can be of different types (Kam Citation1997; Lee Citation2008; Mainhard et al. Citation2009), and different types of relationships translate in differences in the level of students’ independence acquired during the training period (Yoshioka-Kobayashi and Shibayama Citation2020). On one extreme, some supervisors utilize the students as mere research workforce, over-guiding them rather than training them as independent researchers. This approach leads to a PhD training in which students acquire mainly technical competencies and show immediate productivity, joining the supervisor’s ongoing projects (Lee, Dennis, and Campbell Citation2007). However, over-guiding prevents students from becoming familiar with upstream research tasks, such as identifying research questions and coordinating projects (Shibayama, Baba, and Walsh Citation2015). From the supervisors’ point of view, over-guidance is an effective way to maintain or increase their productivity during the students’ training period, benefitting from the additional workforce provided by the PhD students, without incurring the costs of training the students for upstream tasks (Shibayama Citation2019). On the other extreme, avoiding students’ micro-management might force students to develop research questions and projects autonomously. However, an excessive lack of guidance might harm the PhD training success due to students’ inexperience in bearing failures, dead ends, and, in general, the uncertainty that characterizes the research enterprise (Delamont and Atkinson Citation2001; Gardner Citation2008). Indeed, students transitioning from the predictable educational setting that characterizes graduate and undergraduate education might find it challenging to face the unpredictability of outcomes that characterizes PhD training research (Aristizábal Citation2021; Austin Citation2002; Gardner Citation2008). From the supervisors’ point of view, limited guidance prevents students from contributing to their research activities during the students’ training period, but it might benefit productivity in the long term. Students trained with limited guidance are expected to become future supervisors’ collaborators with broader competencies, mastering not only technical knowledge but also upstream tasks (Shibayama Citation2019).
The main takeaway from the previous literature studying PhD students’ supervision is that the student-supervisor relationship should be ideally framed to achieve a delicate balance between providing help and not interfering too much with the students’ work (Bastalich Citation2017; Gardner Citation2008; Lee, Dennis, and Campbell Citation2007). This supervision style is expected to support the student through the uncertainty of the PhD journey and, at the same time, to nurture students’ independence.
The independence developed by students during their training period can take different forms (van den Besselaar and Sandström Citation2019). The various forms of independence can be ascribed to two main categories: organizational and cognitive independence. Organizational independence is the extent to which a scientist is not subject to organizational control by other individuals higher in the career ladder (Yoshioka-Kobayashi and Shibayama Citation2020), while cognitive independence is the extent to which a scientist autonomously defines the research agenda (Laudel and Gläser Citation2008). In this paper, we focus on the latter form of independence, and we refer to cognitive independence in relation to the choice of the student’s research topic with respect to the supervisors’ research interests. Three main reasons drive our choice. First, independence in the subject choice is often considered the most relevant form of independence, especially for young researchers (Daniels Citation2015; National Research Council Citation2005). Indeed, the choice of the research subject during the PhD can have a long-lasting impact on students’ careers due to path dependency mechanisms. This is because scientists tend to focus their research on topics previously explored during their careers (Chubin and Connolly Citation1982; Laudel and Gläser Citation2008; Mangematin Citation2000). Second, cognitive independence is more relevant than organizational independence for PhD students who just started their training as researchers and still have to climb the academic career ladder. Finally, cognitive independence is a prerequisite to organizational independence. Scientists who are able to design research projects independently can obtain their own funds and resources. Being project leaders positions these scientists higher on the career ladder, making them gain organizational independence (Laudel and Bielick Citation2018).
The choice of the research subject is a complex process mainly determined by the type of student-supervisor relationship (Campbell Citation2003; Laudel and Gläser Citation2008). On the one hand, some supervisors can exert a strong influence in orienting students’ areas of interest, disposing of considerable administrative power and scientific authority (Hilmer and Hilmer Citation2009; Laudel and Gläser Citation2008). For this reason, often, students leave to their supervisors the responsibility of identifying the subject of their first research project (Delamont and Atkinson Citation2001; Latour and Woolgar Citation2013). The prevalent role of the supervisor in determining the research subject leads young researchers to begin their careers with limited cognitive independence, mainly contributing to their advisors’ research agenda (Campbell Citation2003; Delamont and Atkinson Citation2001; Kam Citation1997; Stephan and Levin Citation1997). Setting the students’ research agenda allows the supervisor to use students as a scientific workforce and increase students’ reciprocity. For instance, those students have a high probability of staying linked to their supervisors, citing them and co-author with them also after graduation (Barres Citation2017; Shibayama Citation2019).
On the other hand, some students are autonomous in defining their research agenda (Lee, Dennis, and Campbell Citation2007; Roach and Sauermann Citation2010) and explore subjects diverging from their supervisors’ research for at least three reasons. First, students might want to develop their exploratory capabilities instead of relying on their supervisor for the choice of the research subject (Shibayama Citation2019). Second, supervisors interested in opening a new research line might encourage students to explore topics far from their competencies, asking them to invest time and effort in a risky research enterprise at their place (Lee, Dennis, and Campbell Citation2007; Mainhard et al. Citation2009). Finally, students might strive to diverge from the supervisor’s research agenda to obtain full credit for the results of their research effort. Indeed, only limited credit is attributed to students embracing their supervisor’s research agenda, most of the credit being attributed to the supervisor despite the student’s contribution (Merton Citation1968).
Considering empirical works on independence, there is a lack of studies on the factors leading students to become independent from their supervisors, while the few works attempting to relate independence to future career outcomes provide contradictory results. For example, by surveying highly reputed mentors, Blackburn et al.’s qualitative work concludes that protégés who clone their mentors succeed (Blackburn, Chapman, and Cameron Citation1981). On the contrary, Ma et al.’s work, analysing an extensive longitudinal dataset of outstanding U.S. scholars, finds that protégés who pursue their research ideas have a higher probability of excelling in academia (Ma, Mukherjee, and Uzzi Citation2020). Roach and Sauermann (Citation2010) find that, among other characteristics, students who prefer academic careers are those who prefer to choose their research subjects. Shibayama, studying 791 PhD students in life science labs in Japan, finds that subject independence increases results’ credit allocation to students and the probability of pursuing academic careers (Shibayama Citation2019). In another study based on similar data, including 188 PhD students in life sciences, Yoshioka-Kobayashi and Shibayama (Citation2020) find that a training approach encouraging exploration of original research subjects during the PhD, leads to post-graduation cognitive independence.
3. Data and methods
3.1. Empirical context
The empirical context of our study is the population of French students who obtained their PhD in the STEM field between 2004 and 2013. French universities are well-known for their excellence in the STEM disciplines such as biology, chemistry, medicine, and mathematics and enumerate notable researchers such as Laplace (mathematics), Picardet (literature and chemistry), Calmette (medicine), Curie (physics), and Pasteur (microbiology). The country ranks 4th in the world in the number of Nobel prize awards (70) after the USA, UK, and Germany.Footnote1 French universities are also well placed according to several international rankings such as the Shanghai ranking, ARWU global ranking, and Nature’s Lens score. For instance, in the 2021 edition of the Shanghai ranking, University Paris-Saclay ranked 13th in the world and third in Europe.
PhD programs are nowadays the pillars of the French educational system to train tomorrow’s researchers. Doctorates in STEM disciplines represent about 70% of the doctorates yearly granted.Footnote2 To access a PhD program, students must hold a national master’s degree or an equivalent diploma, certifying their aptitude to undertake research. A committee of professors selects students through a formal interview process. Once hired, doctoral students obtain a fixed-term working contract for the entire duration of their studies. According to the French Ministry of Higher Education Research & Innovation, 9 out of 10 theses in STEM are completed in four years.Footnote3
After completing the PhD, staying within academia is only one of the possible options. For instance, considering a large survey on PhD graduating in engineering science, Mangematin (Citation2000) found that 43.9% of the PhD graduates between 1984 and 1996 in Grenoble, obtained a tenured position in the academic sector at the time when they were surveyed, the 37.1% continued to perform research in the private sector, while the remaining moved to other functions in the private or public sector. More recent figures referring to 2018, show a similar percentage of students (44%) remaining in academia in the three years after graduation (MESRI Citation2023). However, these more recent figures include not only tenured positions but also temporary positions. The number of temporary positions for those who stayed in academia increased over time, being available in recent years only one tenure position in universities for every ten doctorates awarded (OECD Citation2021). Nowadays, career uncertainty and tough competition are making academic careers in France less attractive for PhD students in favor of the private sector (MESRI Citation2023). The trend is common in several OECD countries (OECD Citation2021). For instance, in the UK, two-thirds of PhD graduates enter non-academic employment (Hancock Citation2023).
3.2. Data
We combined different data sources to create a unique dataset of French PhD students in STEM. We obtained fine-grained data on PhD theses from the French national repository of Electronic Doctoral Theses and complemented those data with students’ and supervisors’ publication records collected from Elsevier’s SCOPUS database.
With special permission from the Agence Bibliographique de l’Enseignement Supérieur, the French public Institute in charge of maintaining the bibliographic archive of French universities, we collected the entire universe of STEM theses in France between 2004–2013. By accessing the Electronic Doctoral Theses repository, for each thesis record, we gathered information on the author, university of graduation, defense date, supervisor’s name, co-supervisor’s name (if any), field of study, and thesis abstract.
Concerning the publication data, we retrieved students’ and supervisors’ publications from Elsevier’s SCOPUS database. For each publication, we collected basic metadata such as title, abstract, journal, year of publication, citations, and keywords. In addition, publication data also provides authors’ affiliations.
Our initial sample counted 81,400 PhD students who graduated in STEM disciplines between 2004 and 2013. Among these theses, 61,037 report a thesis abstract written in English. As detailed in Section 3.3, our measure of independence is based on comparing the text of the theses and supervisors’ work. In this later comparison, we required supervisors to have at least one publication written in English during the PhD training period. We also dropped students and supervisors with common names, for whom it was unlikely to attribute publications correctly due to homonymy issues. The restrictions applied to the initial sample leave us with a study sample of 42,630 PhD students (69,84% of the theses with an abstract in English and 52.37% of all the theses defended).
3.3. A measure of independence
A crucial aspect of our empirical analysis is identifying a reliable proxy for student’s research independence from her supervisor. To construct this proxy, we compare the student’s PhD thesis and the supervisor’s research content during the training of the PhD student.Footnote4 We compute a similarity score between the thesis abstract and the abstracts of the papers published by the supervisor during the PhD period. In retrieving publications, we define the PhD period as the years ranging from t−3 to t+1, where t is the defense year. We consider the year t+1 part of the PhD period due to possible publication time lags of the supervisor’s work. To calculate the similarity score, we use a neural network algorithm for text analysis that transforms thesis and publication documents into vectors according to the semantic meaning of the words appearing in their texts (Mikolov et al. Citation2013). Appendix 1 reports a detailed description of the neural network algorithm used. Once we identify the vectorial representation of theses and publications in a 100-dimension vectorial space, we calculate the cosine similarity value (sim) for each thesis-publication pair. The cosine similarity score ranges from −1 to 1, where 1 indicates that the thesis content is identical to the paired supervisor’s publication and −1 indicates the complete dissimilarity between the two paired documents. Although −1 is theoretically the lowest possible similarity value, values smaller than 0.70 already indicate significant differences between documents. For instance, documents with a level of cosine similarity below 0.70 result from comparing documents belonging to different disciplines, e.g. a thesis in engineering and a publication in mathematics. In our sample, the similarity score of the thesis-publication pairs ranges from 0.7 to 1.
Once we obtained the similarity value between the thesis and supervisor’s publications, we proceeded to calculate the variable Independence in two steps. First, we identify the supervisor’s article most similar to the student’s thesis in terms of content, i.e. the thesis-publication pair with the maximum cosine similarity value, max(sim). Second, we calculate the variable Independence as equal to 1-max(sim).Footnote5 The variable Independence measures how much the most similar supervisor’s publication to the student’s thesis differs from the student’s thesis. In other words, high values of Independence show that the student thesis does not display significant similarity with any of the supervisor’s research subjects, while low values of Independence mean that the student thesis is similar to at least one of the supervisor’s research subjects.
Overall, we calculate Independence values by pairing the text of 42,630 thesis abstracts with 295,596 supervisors’ publication abstracts, obtaining 42,630 Independence values, one for each student-supervisor pair. We standardize the resulting values of the Independence variable by subtracting its mean and dividing by its standard deviation. In our sample, the Independence variable ranges from −2 to 5.94 standard deviations.
As an example of Independence calculation in our study sample, we consider professor A supervising two students, X and Y. Student X graduated in 2007 with a thesis proposing a mathematical model for ‘marine growth’, i.e. the growth of marine organisms in offshore structures such as boats or oil platforms. Student X shows a high level of Independence when her thesis is compared with the professor’s A work published during the thesis period, between 2004 (t−3) and 2008 (t+1). Indeed, (left panel) shows that professor A’s paper most similar to the student’s thesis, was published in 2006 (article id p.2006.82). Paper p.2006.82 models the mechanical dynamic behavior of inflatable tubes. Although X’s thesis and A’s paper have the use of mathematical modeling in common, they apply it to different physical processes (i.e. marine growth and inflatable tubes). The different applications explain the fact that paper p.2006.82 shows a relatively low similarity score of 0.848 with X’s thesis, max(sim), leading to a high value of Independence equal to 1−0.848 = 0.152 (standardized = 2.061). The second student supervised by professor A, Student Y, graduated in 2005 with a thesis presenting a model for studying the buckling of pressurized tubes. Student Y’s thesis shows the highest similarity score (0.942) with paper p.2006.82 on inflatable tubes, being both paper and thesis on the same subject and applying the same modeling technique. This latter high similarity score leads to a low level of Independence of the student from A’s work (Independence = 0.058, standardized = −0.41). Interestingly, professor A’s paper most similar to student Y’s thesis (article id p.2006.82), is not the result of a coauthorship with Y, while the only paper coauthored with Y (article id p.2006.91) shows a low level of similarity with Y’s thesis. This latter example shows that supervisor-student co-authorship does not necessarily lead to low independence scores.
Figure 1. Independence values of students X and Y. Note: The left-side radar plot reports the similarity values calculated between professor A’s five articles published between 2004 (t−3) and 2008 (t+1) and student X’s thesis defended in 2007 (t). Articles are ordered clockwise by publication date and are labeled with an id with the following format “p.publication year.article two-digit identifier”. The arrows indicate the value of student X’s Independence (0.152) from her supervisor, which is calculated as 1-max(sim). The right-side radar plot reports the similarity values calculated between professor A’s articles published between 2002 (t−3) and 2006 (t+1) and student Y’s thesis defended in 2005 (t). Articles are ordered clockwise by publication date. The arrows indicate the value of student Y’s Independence (0.058) from her supervisor, which is calculated as 1-max(sim).
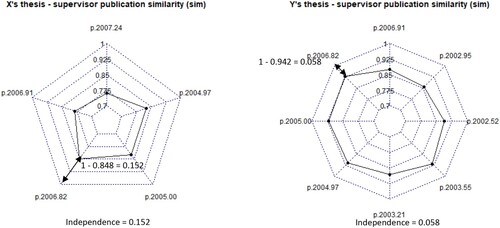
shows the distribution of our Independence variable for the 42,630 students in our sample by defense cohort (left panel) and by thesis discipline (right panel). As expected, the distributions are right-skewed since numerous theses show a high level of content similarity with supervisors’ research work (low level of independence). Nonetheless, a non-negligible part of students’ theses shows divergent content from the supervisor’s workFootnote6 (high level of independence). In , we report the average value of the variable Independence by defense cohort and discipline.
Figure 2. Standardized Independence score distribution. Note: The two figures report the Kernel density estimation of the Independence distribution by defense cohort (left panel) and thesis discipline (right panel). Values of Independence reported in the panels are standardized and range from −2 standard deviations to 6 standard deviations.
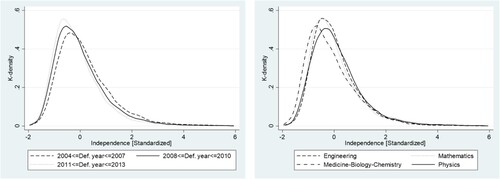
Table 1. Descriptive statistics for the variable Independence.
and show the time trend and field heterogeneity for the Independence variable values. (left panel) and show that the average value of independence has declined over time, being students’ thesis work closer to their supervisors’ research in recent years. (right panel) and the descriptive statistics in show that Medicine-biology-chemistry and Physics are the fields with the lowest and highest level of Independence, respectively.
3.4. Estimation strategy
We use the measure of independence defined above to perform three econometric exercises. First, (i) we study the factors driving research independence during the PhD period. Second, (ii) we explore how the independence level during the PhD is associated with students’ probability of starting an academic career and, finally, conditional on starting an academic career, (iii) we analyse the link between independence and an extensive set of productivity indicators.
To analyze the factors associated with research independence, we estimate an Ordinary Least Squares (OLS) regression having Independence as the dependent variable and three vectors, including student, supervisor, and thesis characteristics, as independent variables. Equation 1 represents the estimated model:
(1)
(1) Then, we explore how independence is associated with students’ career paths. We estimate a regression relating Independence to the probability of pursuing an academic career (see section 3.5 for a detailed description of the variable Academic career). Equation 2 shows the estimated model where G is a logistic function. The vectors of independent variables in Equation 2 are the same as in Equation 1.
(2)
(2) Finally, we further explore the career outcomes for those students who stay in academia. For this sub-sample of students, we explore the association between Independence and five different career outcomes (see section 3.5 for a detailed description of the five outcome variables): Number of publications, Average citations per article, Number of distinct co-authors, having a Foreign affiliation and, having a US affiliation. Equation 3 shows the estimated model. The dependent variable, Career outcome, takes, in turn, the values of the five outcomes considered, while the vectors of the controls are the same as those presented in Equation 1. We use the OLS estimator when the dependent variable is continuous, while we use the Logit estimator when the dependent variable is binary. Equation 3 reports the estimated model for a continuous dependent variable.
(3)
(3) Our study aims to obtain reliable estimates of the model parameters
,
,
,
, and
that represent the impact of student (
), supervisor (
), and thesis (
) characteristics on Independence, and the impact of Independence on the probability of starting an academic career (
) and on the academic career outcomes (
).
3.5. Variable definitions
This section provides a definition and descriptive statistics for the variables used in the regression exercises. Specifically, it defines all the dependent variables of Equations 1, 2, and 3 and the independent variables included in the vectors Student’s characteristics, Supervisor’s characteristics, and Thesis characteristics.
As described in section 3.3, we define Independence as a continuous variable that measures the student’s level of independence. The measure is standardized and based on a similarity index calculated between the student’s thesis abstract text and the abstracts of the supervisor’s articles published during the student’s PhD period, from t−3 to t+1, where t is the defense date. The variable is used as the dependent variable in Equation 1 and as independent variable in Equations 2 and 3.
We defined Academic career as the dependent variable of Equation 2. We distinguish students into two groups, those pursuing an academic career and those who exit academia. Under the assumption that those who stay in academia publish in the years after graduation (Black and Stephan Citation2010; Brischoux and Angelier Citation2015; Horta and Santos Citation2016; Sauermann and Haeussler Citation2017; van Dijk, Manor, and Carey Citation2014), we consider as students who pursue an academic career those students for whom we retrieved at least one publication with an academic affiliation in the five years after the PhD period. Specifically, we define Academic career as a dummy variable taking value one if we observe at least one article reporting an academic affiliation authored by the student during the five years after the PhD period, from t+2 to t+6, where t is the graduation year.Footnote7
As dependent variables of Equation 3, we count (1) the number of articles published by the student (Number of publications), (2) the yearly average number of citations received per article (Average citations per article) and, (3) the number of distinct coauthors appearing in the student’s articles (Number of distinct coauthors). To calculate these variables (1–3), we consider the publication in the 5 years after the PhD period, from t+2 to t+6. Moreover, we construct (4) a dummy variable, Foreign affiliation, taking value one if the student affiliates with at least one non-French institution in the 5 years after the PhD period, zero otherwise. Finally, (5) we distinguish US affiliation by calculating a dummy variable that takes value one if the student affiliates with at least one US institute in the 5 years after the PhD period, zero otherwise.
As for the independent variables included in the vector Student’s characteristics, we define At least one pub. during the PhD period as a dummy variable that takes value one if the student publishes at least one article during the PhD period, zero otherwise. To better specify the article type, we define At least one pub. coauthored with the supervisor during the PhD period as a dummy variable that takes value one if the student publishes at least one article with her supervisor during the PhD period, zero otherwise. In retrieving student’s publications, we considered the PhD period as the years ranging from t−3 to t+1, where t is the defense year. As for the student’s biographic characteristics, we calculate the variable Female studentFootnote8 defined as a dummy variable that takes value one for females, zero otherwise. The variable Age equals the student’s age in the defense year. The variable French is a dummy variable that takes value one if the student is of French nationality, zero otherwise. For some students, the information on age and nationality is missing. Therefore, we calculate the variables Missing age and Missing Nationality as dummy variables taking value one if the student’s age or nationality is missing, zero otherwise.
As for the independent variables included in the vector Supervisor’s characteristics, we define the Number of publications during the PhD period as a variable counting the articles published by the supervisor during the student’s PhD period, the Average yearly citations received per article as the average yearly citations received by those articles, and the Number of distinct coauthors during the PhD period as the number of supervisor’s distinct coauthors during the student’s PhD period. As for the supervisor’s biographic characteristics, we calculate the variable Female supervisor as a dummy variable that takes value one for a female supervisor, zero otherwise.
As for the independent variables included in the vector Thesis characteristics, we calculated four dummy variables according to the field in which the thesis is classified. Specifically, we define Mathematics as a binary variable that takes value one if the student’s thesis is classified in mathematics, zero otherwise. Similarly, we define the dummy variables Medicine-biology-chemistry, Physics, and Engineering. Moreover, we define the variable Having a co-supervisor as a binary variable that takes value one if the student is advised by a supervisor and a co-supervisor, zero otherwise. Finally, we defined a set of 10 dummy variables (Defense year dummies), one for each thesis defense year, and a set of 10 dummy variables (University dummies), one for each university where the PhD is enrolled. Specifically, we define a dummy variable for all the universities with the largest national PhD programs,Footnote9 i.e. Paris, Toulouse, Lyon, Grenoble, Marseille, Strasbourg, Bordeaux, Montpellier, Rennes, and Lille. Then, we define a residual category that includes all the remaining French universities.
3.6. Descriptive statistics
illustrates the descriptive statistics for the variables described in Section 3.5. It shows that 49% of the students in our study sample start an academic career five years after the PhD period. On average, students who start an academic career publish 5.68 articles cited 3.26 times per year; they have 26.97 distinct coauthors, a foreign affiliation in 46% of the cases, and a US affiliation in 11% of the cases.
Table 2. Descriptive statistics for dependent and independent variables.
In the overall sample, 64% of the students publish at least one paper during the PhD period, and 56% publish at least one paper with their supervisor. As expected, those percentages are higher for students who stay in academia: 85% of that sub-group of students have at least one published paper, and 76% publish at least one paper with their supervisors. About one-third of our students are females, the average student graduates when 27 years old, and the majority (70%) of the students are French. The average supervisor is a well-reputed scientist with a strong publication record (15 publications receiving about eight yearly citations) and an extensive scientific network (more than 50 co-authors). About one-quarter of the supervisors are females. Looking at the field distribution, 43% of the theses are in Medicine-biology-chemistry, 25% are in Engineering, and the remaining are in Mathematics.
4. Results
4.1. Research independence determinants
Our first set of analyses examines how PhD students’, supervisors’, and theses’ characteristics are associated with students’ independence. reports our regression analysis results and shows that students’ French nationality relates positively to students’ independence, while Age relates negatively to independence. Moreover, our estimates show heterogeneous associations between supervisor-PhD student gender pairing and students’ independence, confirming a line of previous research indicating the existence of gender disparities among researchers (Clauset, Arbesman, and Larremore Citation2015; Pezzoni et al. Citation2016). Female students tend to be less independent, especially when mentored by female supervisors. The Female supervisor – Female student pair is associated with a 0.15 standard deviations lower independence than the Male supervisor – Male student reference category. The latter category is the one associated with the highest level of independence. The lack of independence of female students observed in our study does not necessarily have a negative connotation, meaning also that female students tend to be more collaborative with their supervisors. Concerning the students’ bibliometric profile, our results indicate that students who publish at least one article during their PhD period have higher independence from their supervisors’ research work than those who do not publish (0.37 standard deviations higher Independence). However, if the published article is coauthored with the supervisor, the independence reduces by 0.48 standard deviations (0.37–0.85) Footnote10 As shown in the example reported in Section 3.3, it is important to consider that Independence and co-authorship with the supervisor are two different concepts. As a robustness check, Appendix 3 reports a set of regressions in which we calculate Independence, excluding the supervisors’ articles co-authored with the students during the PhD period, and results are consistent with the ones reported in , , and .
Table 3. Regression explaining Independence.
All supervisors’ productivity proxies (Number of publications, Average yearly citations per article, and Number of distinct co-authors) are associated with lower student independence. Specifically, one additional publication is associated with a 0.0012 standard deviations lower independence score. This result has a twofold explanation. First, comparing the PhD thesis with a large number of supervisor’s publications leads mechanically to an increased likelihood of finding a publication similar to the thesis, explaining the lower independence values. However, the result might also suggest that students align their research subjects with highly productive supervisors to benefit from their reputation and prestige within the scientific community (Petersen et al. Citation2014; Stephan and Levin Citation1997). This latter explanation is consistent with the negative signs observed for the coefficients of supervisors’ citations and network size, showing that students tend to be less independent when their supervisors are highly cited and have a large collaboration network.
Concerning the thesis characteristics, students with a co-supervisor have an independence score of 0.047 standard deviations higher than students who do not have a co-supervisor. This latter result might be explained by the fact that supervisors lacking competencies in their students’ thesis subjects tend to delegate supervision to colleagues, leading to a positive sign of the coefficient of the variable Independence.Footnote11 Overall, our findings show that student independence during the PhD correlates with the students’, supervisors’, and theses’ characteristics.
Our estimates also reveal the presence of heterogeneity across scientific fields.Footnote12 In the simple descriptive statistics reported in , mathematics appears as one of the fields with the highest level of independence, and medicine-biology-chemistry is the field with the lowest independence. However, when including control variables in our econometric exercise in , Column 1, we observe a different evidence. On average, students in mathematics are less independent from their supervisors than students in engineering (i.e. the reference category). In contrast, students in medicine-biology-chemistry and physics show higher levels of independence. Specific unobservable field norms captured by the field dummy variables might explain these results. For instance, students in mathematics might be assigned by their supervisors to a particular problem at the beginning of their PhD, reducing their independence.
4.2. Independence and academic career
Our second set of results focuses on how students’ independence level during the PhD links with students’ career paths after the PhD period.Footnote13
, Column 1, shows the regression model explaining the probability of pursuing an Academic career with student Independence, our main explanatory variable. We find that the two variables are positively associated: one standard deviation increase in Independence is associated with a one percentage point higher probability of starting an academic career. Columns 2 and 3 of investigate the non-linear relationship between the two variables. Specifically, Column 2 indicates that students belonging to the second, third, and fourth quarter of the ordered Independence values, defined using quartiles, show more than 3 percentage points higher probability of starting an academic career than students in the lowest quarter. Moreover, when comparing students from the second, third, or fourth quarter, we do not observe significant differences in the probability of pursuing a career in academia.Footnote14 In other words, there is a statistically significant gain in the likelihood of pursuing an academic career associated with moving from low values of independence (1st quarter) to slightly higher values of independence (2nd quarter), and this gain does not increase linearly when increasing the value of independence (3rd and 4th quarters). Column 3 shows estimates of a model including the Independence quadratic term. Results confirm the non-linear effect of student’s independence on the probability of starting an academic career. Indeed, we find a positive coefficient associated with the Independence linear term and a negative coefficient associated with the Independence squared term. Based on these two coefficients, plots the predicted probability of starting an academic career for an average studentFootnote15 when the Independence score ranges from −2 to +2 standard deviations. In this range, we observe a 10-percentage point variation in the probability of starting an academic career.
Figure 3. Predicted probability of starting an academic career vs. Independence, including the Independence squared term in the regression. Note: The figure represents the predicted probability of starting an academic career for an average student. We define an average student as a student having all the control variables included in the econometric model reported in Column 3 of at their average value.
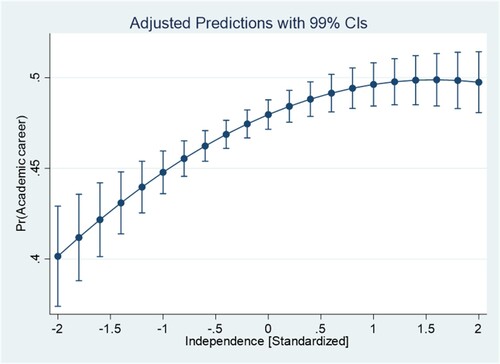
Table 4. Regression estimating the relationship between independence and the probability of starting an academic career.
Considering the controls, in line with extant empirical literature, academic productivity during the PhD increases the probability of staying in academia (Black and Stephan Citation2010; Brischoux and Angelier Citation2015; Horta and Santos Citation2016; Sauermann and Haeussler Citation2017; van Dijk, Manor, and Carey Citation2014). The regression results reported in , Column 1 show that publishing at least one paper during the PhD is associated with a 31-percentage point higher probability of starting an academic career. Co-publishing with the supervisor during the thesis further increases the probability by 6.6 percentage points. These results align with previous findings (Horta and Santos Citation2016) and suggest that coauthoring a paper with the supervisor secures students’ access to the supervisor’s academic network and community favoring academic careers. Students with French nationality show a higher probability of starting an academic career. On the contrary, the probability of starting an academic career decreases with students’ age. Our regression estimates confirm previous studies on the existence of a gender gap in academia (Bagues, Sylos-Labini, and Zinovyeva Citation2017; Lerchenmueller and Sorenson Citation2018; Sabatier Citation2010): female students show lower probabilities than male colleagues of starting an academic career regardless of the gender of their supervisor.
The effects of supervisors’ characteristics included as controls reveal how the supervisors’ scientific activity influences students’ access to academic positions. For example, a greater number of citations and a larger professional network are positively associated with the probability of starting an academic career. Indeed, supervisors’ citations and professional network size proxy the visibility of the supervisors within the scientific community and their social capital (Petersen et al. Citation2014). Such factors may favor the students’ chances of obtaining an academic position.
Finally, concerning the thesis characteristics, students in engineering have a lower probability of staying in academia than students in all the other disciplines, probably due to their high employability in alternative remunerative jobs in the private sector. Furthermore, having a co-supervisor is positively associated with the probability of starting an academic career.
4.3. Independence and early career productivity
Our final set of results explores the early career outcomes for those students who stay in academia. We define the early career period as the 5-year period after the PhD period. For the sub-sample of students remaining in academia, we explore the association between Independence and five different career outcomes, as measured by Number of publications, Average citations per article, Number of distinct coauthors, probability of having a Foreign affiliation, and probability of having a US affiliation.
The regression results in indicate that student Independence is positively associated with the number of papers published in the 5 years after the PhD period, while it is negatively associated with the number of citations received and the probability of experiencing international mobility, whether outside France or in the United States. Specifically, increasing student Independence by one standard deviation is associated with 0.13 additional articles published (2.29% of the sample average), 0.26 fewer citations (7.98% of the sample average), 1.3 lower percentage-points probability of having a foreign affiliation (2.82% of the sample average), and 1.3 percentage points lower probability of having a US-based affiliation (11.82% of the sample average). Only the Number of distinct co-authors after the PhD period is not associated with Independence. Our results point to a substantial advantage for independent students in terms of the number of articles published at the cost of fewer citations and a lower probability of obtaining a position abroad. Interpreting these results jointly with the ones reported in indicates that, on the one hand, higher values of independence raise the probability of starting an academic career and publishing after the PhD. On the other hand, independence is detrimental to the impact of the research conducted after the PhD (as measured by citations received) and the probability of experiencing international mobility.
Table 5. Regression estimating the relationship between Independence and academic career outcomes.
The estimates reported in , confirm that early productivity during the training period is likely to affect all later career outcomes (Allison, Long, and Krauze Citation1982). For example, students who publish at least one paper during the PhD period appear more productive in their follow-up academic career and are more likely to experience international mobility. French nationality students have fewer publications and a lower probability of having a foreign affiliation, while they have a higher number of coauthors and a higher probability of having a U.S. affiliation than non-French students. Older students publish more and have larger co-authorship networks, while they receive fewer citations and have a lower probability of having a U.S. affiliation. Also, we observe that not only do female students have a lower probability of pursuing an academic career, but those who stay in academia have lower publication scores, receive fewer citations, and have limited-size coauthorship networks than their male counterparts. These results hold regardless of the gender of the supervisor and confirm the difficulties of female scientists in excelling in academia, particularly in male-dominated fields like the STEM field (Hunt Citation2010). In line with previous studies (Marwell, Rosenfeld, and Spilerman Citation1979; Shauman and Xie Citation1996), female students also show a lower probability of moving abroad after the PhD period.
The supervisor’s characteristics are positively associated with all the student’s career features, with the exception of the supervisor’s number of publications that is negatively associated with the citations received by the student and the student’s co-authorship network size.
Finally, students in Medicine-biology-chemistry and Physics show the highest scores according to all the five productivity indicators considered. Moreover, correlations between co-supervision and the five productivity indicators studied indicate mixed results. For instance, having a co-supervisor is associated with a higher probability of having an affiliation with a foreign university. At the same time, co-supervised students receive fewer citations and are less likely to move to a U.S. university.
5. Discussion and conclusions
Understanding the factors promoting young scholars’ cognitive independence from their supervisors and the link between independence during the PhD training period and later career outcomes is crucial for designing appropriate science and technology policies shaping the research workforce’s career paths.
Our empirical sample covers the entire French population of PhD students who graduated in the STEM fields from 2004 to 2013. We conducted our empirical analysis by relying on detailed information on PhD students’ theses retrieved from the French national repository of Electronic Doctoral Theses. Moreover, we gather students’ and supervisors’ publication records from Elsevier’s SCOPUS database.
Considering the drivers of cognitive independence, we find that independence is significantly associated with students’ and supervisors’ characteristics. For instance, our study reveals that the gender pairing supervisor-PhD student relates to independence: female students advised by female scholars show the lowest level of independence. This latter result is in line with both psychology and educational literature. On the one hand, Western countries’ social and cultural environments favor the development of independence in men and connectedness to others in women (Cross and Madson Citation1997; Gilligan Citation1982). On the other hand, male students tend to have more conflict-ridden relationships with mentors and, therefore, be more independent than their female colleagues. Koepke and Harkins (Citation2008) studied 698 young students, finding that boys experience a more distant and adversarial relationship with their teachers than girls. Birch and Ladd (Citation1997) found similar results by analyzing 206 kindergarten children. We also find that younger students are more independent than older students. We interpret this result as evidence that younger students show a higher attitude toward exploring creative ideas, leading them to deviate from their supervisors’ work and pursue an independent research line (Jones and Weinberg Citation2011; Levin and Stephan Citation1991; Stephan and Levin Citation1992; Zuckerman and Merton Citation1972). Interestingly, French students show more independence from their supervisors than international students. This result confirms that national and international students have different relationships with their supervisors due to different learning styles, sociopolitical factors, cultural prejudices, stress due to environmental adjustment, and language barriers (Rose Citation2005). Using the theoretical lens of Hofstede’s cultural dimension theory (Hofstede Citation1980), we might interpret our result as linked to a higher level of individualism of French students with respect to the lower level of individualism of students from other countries largely represented in the PhD student population in France, such as Algeria, Morocco, Tunisia, and Lebanon (“Country Comparison,” Citation2022). Finally, our findings concerning the relationship between students’ independence and supervisors’ productivity suggest that students do not align their research subjects with their supervisors unless they perceive the opportunity to benefit from supervisors’ reputation and prestige (Petersen et al. Citation2014; Stephan and Levin Citation1997). At the beginning of their careers, PhD students lack the reputational effect needed to access funding opportunities, enter fruitful collaborations, attract citations, or publish in well-reputed scientific journals. Therefore, students might align their research interests with their supervisors to leverage their supervisor’s reputation. For instance, students aligning with a reputed supervisor’s research have privileged access to the supervisor’s network by attending prestigious conferences in the field, connecting with experts, and receiving publication guidelines.
Concerning the association between research independence and students’ careers, we find that a high level of research independence gained during the PhD training is associated with a higher probability of starting an academic career. We explain this result with the fact that independence is a requirement needed to fulfill recruiters’ and evaluators’ expectations. Indeed, evaluators expect to observe a signal of the student’s ability to launch independent research programs as a leader rather than as the supervisor’s protégé (Liénard et al. Citation2018; Stephan Citation1996; Zuckerman and Merton Citation1972). On the contrary, students avoiding the risks entailed in exploring research lines different from their supervisors give evaluators a signal of research inexperience. For this reason, PhD students are often encouraged to set up their original research agenda and explore new areas beyond their supervisors’ expertise (Kam Citation1997; Lee Citation2008; Lee, Dennis, and Campbell Citation2007; Mainhard et al. Citation2009). Our results also show that those independent students who embrace an academic career show higher publication productivity. Independent students seem to leverage the autonomy acquired during their PhD period, while those who merely acted as scientific workforce for their supervisors face more difficulties in publishing (Shibayama Citation2019). Although they have a better publication record, independent students who embrace an academic career experience the disadvantage of receiving fewer citations for the scientific work produced after the PhD. This result can be explained by the fact that not embracing the research line of their supervisors limits students’ opportunity to leverage supervisors’ reputation and visibility (Merton Citation1968). Indeed, independent students have to build their reputation and visibility in a research domain not covered by their supervisor, while students conducting research in the exact domain as their supervisors benefit from their supervisors’ research legacy. Similarly, our empirical findings show that independent students are less likely to obtain an academic appointment in a foreign university after obtaining their PhD. Accessing the international network appears more challenging for those students who do not leverage the supervisors’ reputation and visibility support. Indeed, supervisors can spend their reputation and exercise influence within the network of foreign colleagues to find positions for their PhD students. However, that network is likely to be field-specific, and students pursuing a different research line from the one of their supervisors might be excluded. Overall, our results on the impact of independence on students’ careers show the existence of a trade-off between entering academia and succeeding in academia in the first five years after the PhD period. More independent students have to pay the price for their independence. Indeed, in the short run, it seems more challenging for independent students to produce impactful research and be internationally mobile than those following the supervisor’s research avenue who leverage the supervisor’s reputation and network.
From a normative perspective, our results have implications for science, technology, and education policies oriented to design PhD programs maximizing students’ employability and productivity. Our study shows that students’ independence is not an innate characteristic of individuals, and several factors can predict it. Policymakers valuing independence can leverage these factors to increase the effectiveness of the PhD training in enhancing young scholars’ independence. For instance, university doctoral schools should incentivize students to publish at least one article of their thesis during the PhD and limit co-authoring with the supervisor. Moreover, female and international students who, according to our results, tend to have a more collaborative attitude toward their supervisors should be informed before choosing the thesis subject that the lack of independence during the PhD period could undermine their future careers. Looking at students’ careers after graduation, young scholars are usually required to show independence to be selected for an academic career and, at the same time, achieve high-impact research standards in the short run to progress in their careers and success in fundraising activities. Our study shows that for independent students, it is challenging to achieve these two clashing goals. Nonetheless, policymakers might introduce policies reducing the trade-off between independence and research impact. In France, several policies are already in place to favor the initial phases of young scholars’ careers. For instance, newly recruited professors benefit from a temporary reduction of their teaching load (Décharge d’enseignement). However, the teaching reduction is recognized regardless of the level of independence reached during the PhD period. A better policy tool should consider the level of independence as an explicit criterion to modulate the amount of teaching reduction. Another policy tool available in France is a dedicated funding scheme to support early career scholars (Jeunes Chercheuses et Jeunes Chercheurs grants). Also in this case, independence should be considered as a crucial and explicit criterion for awarding these grants. Devoting more time to research, thanks to the teaching load reduction, as well as access to financial resources would help independent young scholars to build a reputation on their research subject despite not benefitting from the supervisors’ influence. Finally, since our findings show that independent students might miss opportunities to move abroad after graduation, another concrete policy intervention could be oriented to design mobility grants targeting this category of students. Dedicated grants might compensate for the lack of support from their supervisors’ international connections. Although obtained in a French context, our results might extend to other countries characterized by a more selective tenure track process than France, like the US and UK. Assuming our results are valid in these latter countries, early career scholars who have shown independent work during their PhD should benefit from a discount when evaluated for tenured positions, taking into account that the support from their supervisors’ reputation on the selected research subject has been limited.
Our analysis is not exempt from limitations. Considering STEM fields in France limits the generalizability of our results to other fields and countries. Although STEM fields show characteristics highly valuable for our analysis, such as excellent coverage in bibliometric datasets, the prevalent use of English as the standard language for publication, and the use of univocal terms, the extension of our analysis to other disciplines like social sciences might be a fertile ground of study. Along the same line, although using France as an empirical context offers the unique opportunity to access data covering the entire population of PhD graduates over a long period, a cross-country comparison might provide interesting avenues for further research. For instance, extending our study to non-STEM fields and countries other than France, might allow us to identify field-specific and country-specific factors that influence students’ independence and independence outcomes. Another limitation of our analysis regards the way in which we identify students pursuing an academic career. Using the affiliations to academic institutions reported in students’ publications after graduation might be an imprecise way of identifying students pursuing an academic career for two main reasons. First, some publications may appear long after the thesis defense, and in the meantime, the student might have left academia. Second, we are unable to distinguish temporary from tenured positions. For instance, students obtaining a postdoc position after graduation are considered equivalent to students obtaining a tenured associate professor position after graduation. Despite the limitations associated with our way of identifying students’ academic careers, it is reassuring that our figures are in line with the official statistics. OECD (Citation2021) shows that the proportion of students who stay in academia after graduation in recent years broadly corresponds to the proportion we identified in our study, i.e. 49%. A final limitation concerns the explanation of field heterogeneity in our findings. In our econometric exercises, we attempted to control for all the observables that differ across fields to minimize biases in the estimations. Our control variables include factors such as supervisors’ productivity and teamwork characteristics. However, fields also differ by factors that are not observable in our quantitative analysis, such as norms related to students’ strategy in selecting a supervisor or advisors and students colluding to maximize the marketability of the latter for faculty positions. An in-depth analysis of disciplinary norms deserves a dedicated study, and a qualitative study with interviews with representative scientists across fields is a promising avenue for future research.
Supplemental Appendix
Download MS Word (170.1 KB)Acknowledgments
We also acknowledge the financial support of GIGA (ANR-19-CE26-0014). We thank participants at the NBER Investments in Early Career Scientists: Data and Research Gaps Workshop, the participants at the WOEPSR 2022 Workshop, and the participants at the 5th BETA-Workshop in Economics of Science and Innovation for their feedback and comments on this work’s earlier version.
Disclosure statement
No potential conflict of interest was reported by the author(s).
Additional information
Funding
Notes
1 The figure refers to 2021 data.
4 In Appendix 5, we adopt an alternative definition of Independence, not limiting the supervisor's publication to the PhD period but considering all the publications of the supervisor preceding the defense year t+1. The results of our econometric exercises are consistent across the two alternative definitions of Independence. The correlation between the two definitions of Independence equals 0.88.
5 The cosine similarity values (sim) in our study sample range from 0.7 to 1. Therefore, the values of Independence calculated as 1-max(sim) ranges from 0 (low independence) to 0.3 (high independence).
6 Appendix 2 reports a set of regressions in which we exclude from our sample students showing extremely high values of Independence, i.e., values higher than +2 standard deviations. The results of the regression exercises are consistent with those reported in the Tables 1, 2, and 3.
7 We consider the student's publications in t+1, as the result of the PhD work, therefore we exclude them from the definition of the variable Academic career.
8 We retrieved the gender information using three datasets: the official French gender-name dataset, US census Bureau gender-name dataset and WIPO gender name dataset.
9 We define the universities with the largest PhD programs by selecting the 10 universities with the largest number of enrolled PhD students in our dataset.
10 The linear combination of the coefficients of the variables At least one pub. during the PhD period and At least one pub. co-authored with the supervisor during the PhD period is statistically significant at 1% level (t-statistic = 45.28).
11 Appendix 4 reports a set of regressions in which we calculate Independence comparing both the supervisor and co-supervisor's work with the PhD student thesis's content. The results are consistent with those reported in the Tables 3, 4, and 5.
12 Appendix 6 reports a set of regressions in which we run separated estimates for each scientific field.
13 Appendix 7 reports an alternative estimation strategy relying on a propensity score matching approach that leads to similar results.
14 We test for the significance of the difference between the coefficients of the variables Independence Q2 and Independence Q3 (P-value .685), Independence Q3 and Independence Q4 (P-value .622), Independence Q2 and Independence Q4 (P-value .377).
15 We define an average student as a student having all the control variables included in the econometric model reported in Column 3 of Table 2 at their average value.
References
- Alfred P. Sloan Foundation. 2022. “Sloan Research Fellowships.” Accessed August 31, 2022. https://sloan.org/fellowships.
- Allison, P. D., J. S. Long, and T. K. Krauze. 1982. “Cumulative Advantage and Inequality in Science.” American Sociological Review 47: 615–25.
- Aristizábal, N. 2021. “I Bombed the GRE—But I’m Thriving as a Ph.D. Student,” June 3. Accessed June 17, 2021. https://www.sciencemag.org/careers/2021/06/i-bombed-gre-i-m-thriving-phd-student.
- Austin, A. E. 2002. “Preparing the Next Generation of Faculty: Graduate School as Socialization to the Academic Career.” The Journal of Higher Education 73: 94–122.
- Bagues, M., M. Sylos-Labini, and N. Zinovyeva. 2017. “Does the Gender Composition of Scientific Committees Matter?” American Economic Review 107: 1207–38. https://doi.org/10.1257/aer.20151211.
- Barres, B. A. 2017. “Stop Blocking Postdocs’ Paths to Success.” Nature 548: 517–19. https://doi.org/10.1038/548517a.
- Bastalich, W. 2017. “Content and Context in Knowledge Production: A Critical Review of Doctoral Supervision Literature.” Studies in Higher Education 42: 1145–57. https://doi.org/10.1080/03075079.2015.1079702.
- Birch, S. H., and G. W. Ladd. 1997. “The Teacher-Child Relationship and Children’s Early School Adjustment.” Journal of School Psychology 35: 61–79. https://doi.org/10.1016/S0022-4405(96)00029-5.
- Black, G. C., and P. E. Stephan. 2010. “The Economics of University Science and the Role of Foreign Graduate Students and Postdoctoral Scholars.” In American Universities in a Global Market, edited by Charles T. Clotfelter, 129–161. Chicago: University of Chicago Press.
- Blackburn, R. T., D. W. Chapman, and S. M. Cameron. 1981. “‘Cloning’ in Academe: Mentorship and Academic Careers.” Research in Higher Education 15: 315–27. https://doi.org/10.1007/BF00973512.
- Bozeman, B., and E. Corley. 2004. “Scientists’ Collaboration Strategies: Implications for Scientific and Technical Human Capital.” Research Policy 33: 599–616. https://doi.org/10.1016/j.respol.2004.01.008.
- Brischoux, F., and F. Angelier. 2015. “Academia’s Never-Ending Selection for Productivity.” Scientometrics 103: 333–36. https://doi.org/10.1007/s11192-015-1534-5.
- Campbell, R. A. 2003. “Preparing the Next Generation of Scientists: The Social Process of Managing Students.” Social Studies of Science 33: 897–927. https://doi.org/10.1177/0306312703336004.
- Chubin, D. E., and T. Connolly. 1982. “Research Trails and Science Policies: Local and Extra-Local Negotiation of Scientific Work.” In Scientific Establishments and Hierarchies, edited by N. Elias, H. Martins, and R. Whitley, 293–311. Dordrecht: Springer Netherlands. Sociology of the Sciences a Yearbook. https://doi.org/10.1007/978-94-009-7729-7_11
- Clauset, A., S. Arbesman, and D. B. Larremore. 2015. “Systematic Inequality and Hierarchy in Faculty Hiring Networks.” Science Advances 1: e1400005. https://doi.org/10.1126/sciadv.1400005.
- Corsini, A., M. Pezzoni, and F. Visentin. 2022. “What Makes a Productive Ph.D. Student?” Research Policy 51: 104561. https://doi.org/10.1016/j.respol.2022.104561.
- “Country Comparison”. 2022. Accessed September 10, 2022. https://www.hofstede-insights.com/country-comparison/.
- Cross, S. E., and L. Madson. 1997. “Models of the Self: Self-Construals and Gender.” Psychological Bulletin 122: 5–37.
- Cyranoski, D., N. Gilbert, H. Ledford, A. Nayar, and M. Yahia. 2011. “Education: The PhD factory.” Nature 472: 276–79. https://doi.org/10.1038/472276a.
- Daniels, R. J. 2015. “A Generation at Risk: Young Investigators and the Future of the Biomedical Workforce.” Proceedings of the National Academy of Sciences 112: 313–18. https://doi.org/10.1073/pnas.1418761112.
- Delamont, S., and P. Atkinson. 2001. “Doctoring Uncertainty: Mastering Craft Knowledge.” Social Studies of Science 31: 87–107. https://doi.org/10.1177/030631201031001005.
- “From Horizon 2020 to Horizon Europe”. 2018.
- Furman, J. L., and F. Teodoridis. 2020. “Automation, Research Technology, and Researchers’ Trajectories: Evidence from Computer Science and Electrical Engineering.” Organization Science 31: 330–54. https://doi.org/10.1287/orsc.2019.1308.
- Gardner, S. K. 2008. “‘What’s Too Much and What’s Too Little?’ The Process of Becoming an Independent Researcher in Doctoral Education.” The Journal of Higher Education 26: 326–350.
- Gentzkow, M., B. Kelly, and M. Taddy. 2019. “Text as Data.” Journal of Economic Literature 57: 535–74. https://doi.org/10.1257/jel.20181020.
- Gilligan, C. 1982. In a Different Voice: Psychological Theory and Women’s Development. Cambridge: Harvard University Press.
- Hamilton, J. G., W. C. Birmingham, P. Tehranifar, M. L. Irwin, W. M. P. Klein, L. Nebeling, and J. Chubak. 2013. “Transitioning to Independence and Maintaining Research Careers in a New Funding Climate: American Society of Preventive Oncology Junior Members Interest Group Report.” Cancer Epidemiology Biomarkers & Prevention 22: 2138–42. https://doi.org/10.1158/1055-9965.EPI-13-0807.
- Hancock, S. 2023. “Knowledge or Science-based Economy? The Employment of UK PhD Graduates in Research Roles beyond Academia.” Studies in Higher Education 48: 1523–37. https://doi.org/10.1080/03075079.2023.2249023.
- Heggeness, M. L., K. T. W. Gunsalus, J. Pacas, and G. McDowell. 2017. “The New Face of US Science.” Nature News 541: 21. https://doi.org/10.1038/541021a.
- Hilmer, M. J., and C. E. Hilmer. 2009. “Fishes, Ponds, and Productivity: Student-Advisor Matching and Early Career Publishing Success for Economics Phds.” Economic Inquiry 47: 290–303. https://doi.org/10.1111/j.1465-7295.2007.00108.x.
- Hofstede, G. 1980. “Culture and Organizations.” International Studies of Management & Organization 10: 15–41.
- Horta, H., and J. M. Santos. 2016. “The Impact of Publishing during PhD Studies on Career Research Publication, Visibility, and Collaborations.” Research in Higher Education 57: 28–50. https://doi.org/10.1007/s11162-015-9380-0.
- Hunt, J. 2010. “Why Do Women Leave Science and Engineering?” Working Paper 15853. Working Paper Series. National Bureau of Economic Research. doi:10.3386/w15853.
- Jones, B. F., and B. A. Weinberg. 2011. “Age Dynamics in Scientific Creativity.” Proceedings of the National Academy of Sciences 108: 18910–14. https://doi.org/10.1073/pnas.1102895108.
- Kam, B. H. 1997. “Style and Quality in Research Supervision: the Supervisor Dependency Factor.” Higher Education 34: 81–103.
- Koepke, M. F., and D. A. Harkins. 2008. “Conflict in the Classroom: Gender Differences in the Teacher–Child Relationship.” Early Education and Development 19: 843–64. https://doi.org/10.1080/10409280802516108.
- Latour, B., and S. Woolgar. 2013. Laboratory Life: The Construction of Scientific Facts. Princeton: Princeton University Press.
- Laudel, G., and J. Bielick. 2018. “The Emergence of Individual Research Programs in the Early Career Phase of Academics.” Science, Technology, & Human Values 43: 972–1010. https://doi.org/10.1177/0162243918763100.
- Laudel, G., and J. Gläser. 2008. “From Apprentice to Colleague: The Metamorphosis of Early Career Researchers.” Higher Education 55: 387–406. https://doi.org/10.1007/s10734-007-9063-7.
- Lee, A. 2008. “How Are Doctoral Students Supervised? Concepts of Doctoral Research Supervision.” Studies in Higher Education 33: 267–81. https://doi.org/10.1080/03075070802049202.
- Lee, A., C. Dennis, and P. Campbell. 2007. “Nature’s Guide for Mentors.” Nature 447: 791–97. https://doi.org/10.1038/447791a.
- Lerchenmueller, M. J., and O. Sorenson. 2018. “The Gender Gap in Early Career Transitions in the Life Sciences.” Research Policy. https://doi.org/10.1016/j.respol.2018.02.009.
- Levin, S. G., and P. E. Stephan. 1991. “Research Productivity Over the Life Cycle: Evidence for Academic Scientists.” The American Economic Review 81: 114–32.
- Levine, I. S. 2007. “Making the Leap to Independence.” Science March. https://doi.org/10.1126/science.caredit.a0700029.
- Liénard, J. F., T. Achakulvisut, D. E. Acuna, and S. V. David. 2018. “Intellectual Synthesis in Mentorship Determines Success in Academic Careers.” Nature Communications 9: 4840. https://doi.org/10.1038/s41467-018-07034-y.
- Ma, Y., S. Mukherjee, and B. Uzzi. 2020. “Mentorship and Protégé Success in STEM Fields.” Proceedings of the National Academy of Sciences 117: 14077–83. https://doi.org/10.1073/pnas.1915516117.
- Maher, B., and M. Sureda Anfres. 2016. “Young Scientists under Pressure: What the Data Show.” Nature 538: 444–45.
- Mainhard, T., R. van der Rijst, J. van Tartwijk, and T. Wubbels. 2009. “A Model for the Supervisor–Doctoral Student Relationship.” Higher Education 58: 359–73. https://doi.org/10.1007/s10734-009-9199-8.
- Mangematin, V. 2000. “PhD Job Market: Professional Trajectories and Incentives during the PhD.” Research Policy 29: 741–56. https://doi.org/10.1016/S0048-7333(99)00047-5.
- Marwell, G., R. Rosenfeld, and S. Spilerman. 1979. “Geographic Constraints on Women’s Careers in Academia.” Science 205: 1225–31.
- Merton, R. K. 1968. “The Matthew Effect in Science.” Science 159: 56–63.
- MESRI. 2023. “Le Doctorat et les Docteurs - état de l’Enseignement Supérieur, de la Recherche et de l’Innovation en France n°16.” Accessed January 20, 2024. https://publication.enseignementsup-recherche.gouv.fr/eesr/FR/T744/le_doctorat_et_les_docteurs/.
- Metcalfe, T. S. 2008. “The Production Rate and Employment of Ph.D. Astronomers.” Publications of the Astronomical Society of the Pacific 120: 229–34. https://doi.org/10.1086/528878.
- Mikolov, T., K. Chen, G. Corrado, and J. Dean. 2013. “Efficient Estimation of Word Representations in Vector Space.” arXiv:1301.3781 [Cs], January.
- National Research Council. 2005. Bridges to Independence: Fostering the Independence of New Investigators in Biomedical Research. Washington, D.C.: National Academies Press.
- Nguyen, M., A. Panyadahundi, C. Olagun-Samuel, S. I. Chaudhry, M. M. Desai, A. Dardik, and D. Boatright. 2023. “Transition From Mentored to Independent NIH Funding by Gender and Department.” JAMA 329: 2189–90. https://doi.org/10.1001/jama.2023.7693.
- “NIH Data Book - Funding Rates”. 2022.
- OECD. 2021. “Reducing the Precarity of Academic Research Careers.” OECD Science, Technology and Industry Policy Papers.
- Paglis, L. L., S. G. Green, and T. N. Bauer. 2006. “Does Adviser Mentoring Add Value? A Longitudinal Study of Mentoring and Doctoral Student Outcomes.” Research in Higher Education 47: 451–76. https://doi.org/10.1007/s11162-005-9003-2.
- Petersen, A. M., S. Fortunato, R. K. Pan, K. Kaski, O. Penner, A. Rungi, M. Riccaboni, H. E. Stanley, and F. Pammolli. 2014. “Reputation and Impact in Academic Careers.” Proceedings of the National Academy of Sciences 111: 15316–21. https://doi.org/10.1073/pnas.1323111111.
- Pezzoni, M., J. Mairesse, P. Stephan, and J. Lane. 2016. “Gender and the Publication Output of Graduate Students: A Case Study.” PLoS One 11: e0145146. https://doi.org/10.1371/journal.pone.0145146.
- Roach, M., and H. Sauermann. 2010. “A Taste for Science? PhD Scientists’ Academic Orientation and Self-selection into Research Careers in Industry.” Research Policy 39: 422–34. https://doi.org/10.1016/j.respol.2010.01.004.
- Rose, G. L. 2005. “Group Differences in Graduate Students’ Concepts of the Ideal Mentor.” Research in Higher Education 46: 53–80.
- Sabatier, M. 2010. “Do Female Researchers Face a Glass Ceiling in France? A Hazard Model of Promotions.” Applied Economics 42: 2053–62. https://doi.org/10.1080/00036840701765338.
- Sauermann, H., and C. Haeussler. 2017. “Authorship and Contribution Disclosures.” Science Advances 3: e1700404. https://doi.org/10.1126/sciadv.1700404.
- Schiffbaenker, H., M. Haas, and F. Holzinger. 2022. “The Gendered Nature of Independence in the Context of Research Funding and Excellence.” SN Social Sciences 2: 275. https://doi.org/10.1007/s43545-022-00563-w.
- Shauman, K. A., and Y. Xie. 1996. “Geographic Mobility of Scientists: Sex Differences and Family Constraints.” Demography 33: 455–68.
- Shibayama, S. 2019. “Sustainable Development of Science and Scientists: Academic Training in Life Science Labs.” Research Policy 48: 676–92. https://doi.org/10.1016/j.respol.2018.10.030.
- Shibayama, S., Y. Baba, and J. P. Walsh. 2015. “Organizational Design of University Laboratories: Task Allocation and Lab Performance in Japanese Bioscience Laboratories.” Research Policy 44: 610–22. https://doi.org/10.1016/j.respol.2014.12.003.
- Stephan, P. E. 1996. “The Economics of Science.” Journal of Economic Literature 34: 1199–1235.
- Stephan, P. 2012. “Research Efficiency: Perverse Incentives.” Nature 484: 29–31. https://doi.org/10.1038/484029a.
- Stephan, P. E., and S. G. Levin. 1992. Striking the Mother Lode in Science. The Importance of Age, Place, and Time. Oxford: Oxford University Press.
- Stephan, P. E., and S. G. Levin. 1997. “The Critical Importance of Careers in Collaborative Scientific Research.” Revue d’économie Industrielle 79: 45–61. https://doi.org/10.3406/rei.1997.1652.
- Stephan, P., and S. Levin. 2002. “The Importance of Implicit Contracts in Collaborative Scientific Research.” In Science Bought and Sold: Essays in the Economics of Science, edited by Philip Miroski and Esther- Mirjam Sent, 412–430. Chicago: The University of Chicago Press.
- The University of Arizona. 2009. “Promotion Tips and Strategies for Assistant Professors.” Accessed August 31, 2022. https://hr.arizona.edu/sites/default/files/AdviceFromOthers.pdf.
- van den Besselaar, P., and U. Sandström. 2019. “Measuring Researcher Independence Using Bibliometric Data: A Proposal for a New Performance Indicator.” PLoS One 14: e0202712. https://doi.org/10.1371/journal.pone.0202712.
- van Dijk, D., O. Manor, and L. B. Carey. 2014. “Publication Metrics and Success on the Academic Job Market.” Current Biology 24: R516–17. https://doi.org/10.1016/j.cub.2014.04.039.
- Yoshioka-Kobayashi, T., and S. Shibayama. 2020. “Early Career Training and Development of Academic Independence: a Case of Life Sciences in Japan.” Studies in Higher Education September: 1–23. https://doi.org/10.1080/03075079.2020.1817889.
- Zuckerman, H., and R. K. Merton. 1972. “Age, Aging, and Age Structure in Science.” Higher Education 4: 1–4.