ABSTRACT
In successful geoinformatics education, students’ active role in the learning process, e.g. through applying self-assessment, show an increasing interest but the evidence of benefits and challenges of self-assessment are sporadic. In this article, we examine the usefulness of an online self-assessment tool developed for geoinformatics education. We gathered data in two Finnish universities on five courses (n = 11–73 students/course) between 2019 and 2021. We examined 1) how the students’ self-assessed knowledge and understanding in geoinformatics subject topics changed during a course, 2) how the competencies at the end of a course changed between the years in different courses, and 3) what was the perceived usefulness of the self-assessment approach among the students. The results indicate support for the implementation of self-assessment, both as a formative and summative assessment. However, it is crucial to ensure that the students understand the contents of the self-assessment subject topics. To increase students’ motivation to take a self-assessment, it is crucial that the teacher actively highlights how it supports their studying and learning. As the teachers of the examined courses, we discuss the benefits and challenges of the self-assessment approach and the applied tool for the future development of geoinformatics education.
Introduction
A common approach to teaching in higher education is constructive alignment, which is based on the theory of constructivism, and the idea that assessment in teaching should be aligned with the intended learning outcomes (Biggs & Tang, Citation2011). A learner actively constructs their knowledge in the learning process, and interprets the concepts being discussed through schemata, or mental models that they hold. This, in turn, means that in order for learning to happen, the learners must be central, active participants in the learning process.
Self-assessment is a core approach that supports constructive learning (Andrade, Citation2019). Self-assessment refers to a range of acts where the students describe, evaluate, and sometimes monitor their competence, the learning process, and its outcome (Brown & Harris, Citation2013; Epstein et al., Citation2008; Panadero et al., Citation2016). Thus, the student evaluates their abilities to understand specific concepts and perform specific tasks, their own learning methods, motivation and efficiency, and the products (such as exercise outputs) they have created during a learning activity (Andrade, Citation2019). Partly, the self-assessment reflects the student’s self-efficacy; in social learning theory, self-efficacy is a key concept in cognitive theory and refers to the learner’s beliefs in their abilities, e.g. abilities to understand specific concepts and perform tasks (Bandura, Citation1977). Self-efficacy further influences the learner’s choices and their actual performance (Bandura, Citation1977). Motivation is an individual’s state-of-mind that is based on motives and leads to a goal-oriented behaviour (e.g. Heckhausen & Heckhausen, Citation2018).
Previous studies suggest that the general purpose of self-assessment is to provide feedback, both for the student and the teacher, on the learning and performance in order to promote them through adjustments in the process (Andrade, Citation2009, Citation2019). A large number of studies have been conducted on self-assessments as a method to increase self-efficacy, enhance motivation, target learning activities, and to assess learning outcomes (Colthart et al., Citation2008; Knight, Citation2020; Nikou & Economides, Citation2016; Panadero et al., Citation2017; Yan et al., Citation2020). Self-assessment results are also known to be good indicators for the teacher of the learners’ motivation and their satisfaction with a learning activity (Sitzmann et al., Citation2010). However, Colthart et al. (Citation2008) note that a notable number of studies have not reached a level of sufficient methodological rigor to be considered as solid evidence regarding the benefits of self-assessments. Most studies have compared the self-assessed learning outcomes to the results based on external standards, mainly as a part of the assessment at the end of a learning event (Colthart et al., Citation2008). Also, the practice and implementation of self-assessment in didactic geoinformatics education is sporadic (Colthart et al., Citation2008).
Geoinformatics, or geospatial and GIScience skills, are taught at the higher education institutions within several science curricula, such as Geography and different fields of technologies and engineering (Mathews & Wikle, Citation2019). Geoinformatics addresses student’s abilities to collect, manage, analyse, and disseminate geospatial data, along with their ability to use location technologies and geographical information systems (GIS) in various sectors of the society, where location intelligence in decision-making is critical. Typical application fields are surveying, positioning, remote sensing, geospatial analytics and modelling, web-mapping, and cartography (Goodchild, Citation2009; Mathews & Wikle, Citation2019).
Successful geoinformatics education combines theoretical understanding and practical skills in such a way that students feel that their proficiency about the substance increases. This enables students to handle more demanding challenges in geospatial data management, analysis, and problem solving; each time, attaining a higher level in professional competence by actively constructing their knowledge and understanding. Students’ constructivist learning and competency-based curriculum development have become cornerstones in geoinformatics education (e.g. Prager & Plewe, Citation2009). Developing competence is linked to self-efficacy, which is a central emotional driver of learning (Bandura, Citation1977). It consists of the learner’s case-specific beliefs and assessment of their capabilities as a learner (Bandura, Citation1986). Due to the rapid development of the geoinformatics field and diversification within the geospatial application fields in society, it has become necessary to diversify the pathways through which students become more active in recognizing relevant skills and taking a self-directing role in building their personal growth as experts (Gong et al., Citation2017; Mathews & Wikle, Citation2019). Therefore, adding elements of self-assessment into the syllabus of the courses has potential to promote students’ own perception of and reflection on their geoinformatics skills development, and for developing teaching in the field.
The role of self-assessment in teaching and learning geoinformatics, or any other subject, depends on whether it is implemented as a part of formative or summative assessment (e.g. Andrade, Citation2019). For the student, self-assessment at the beginning or in the middle of a learning activity, i.e. as a form of the formative assessment, helps them focus on learning activities and increases their motivation (Andrade, Citation2019; Colthart et al., Citation2008; Khoiriyah et al., Citation2015). Formative self-assessment of the learning process is an integral component of self-regulated learning (Zimmerman & Schunk, Citation2011). Self-regulated learning is a key concept in educational psychology, and refers to learning that is driven by the learner’s metacognition (thinking about one’s thinking), their own strategic actions, and motivation to learn (Zimmerman, Citation1989). This positive impact of formative self-assessment on learning has also been demonstrated in higher-education in geography (Thompson et al., Citation2005). Moreover, previous studies suggest that when a formative self-assessment is introduced to students as a tool for improving their own learning, its results are somewhat consistent with the assessments of teachers (e.g. Bol et al., Citation2012; Chang et al., Citation2013; Lopez & Kossack, Citation2007).
Ideally, self-assessment at the end of a learning event, i.e. as a form of a summative assessment, gives insights into the individual learner’s learning process and the learning outcomes directly related to the learning objectives (Bourke, Citation2016). It also aims at prompting learners to self-reflect and increase their self-efficacy (Panadero et al., Citation2017). Previous studies indicate that summative self-assessments have only a moderate relationship with cognitive learning, i.e. self-assessed and teacher-assessed learning outcomes do not have a strong correlation (e.g. meta-analysis by Sitzmann et al., Citation2010). Indeed, Andrade (Citation2019) argues that self-assessments should rather be formative, since its main purpose is to support the learning process.
The increase in remote learning (i.e. e-learning), boosted by the COVID-19 pandemic, sets new demands for both students and teachers. Our experience suggests that major changes in the teaching arrangements occured done in geoinformatics courses. These have had dramatic impacts on communication, along with the role and form of teacher and peer support with hands-on exercises. However, the impact of these changes on students’ learning has not yet been studied, and the results of objective summative assessments at the end of a course provide evidence of only one aspect of the learning process, the outcome, or products. Hence, it is important to study the impact of the pandemic-enforced changes in teaching geoinformatics. Previous studies also suggest that the role of self-assessment for supporting learning increases in remote learning (Chilton & McCracken, Citation2017; Cook & Dupras, Citation2004; Gong et al., Citation2017; Khogali et al., Citation2011). This implies that not only is self-assessment a means to examine the pandemic’s impact on geoinformatics learning, but it may also be necessary for the students when they need to take a more active role in motivating, timing and monitoring their work.
In this article, our overall aim is to examine how a simple, easily accessible online self-assessment tool supports students’ learning in and developing geoinformatics teaching in in higher education. Our case stems from the use of a Self-Assessment Tool, developed within Geoportti, the national hub for geospatial research and academic education resources in Finland. Within five different geoinformatics courses that ran two times (except one course that ran one time) between 2019 and 2021, we asked students to perform a self-assessment of knowledge and understanding of different subject topics in geoinformatics. This data allows demonstration of the type of data gathered with the Self-Assessment Tool and the possibilities to analyse it. We also gathered students’ experiences on the impact of the self-assessment on their studying and learning processes. Based on this data, our objectives in this article are:
to examine changes in self-assessed knowledge and understanding in geoinformatics subject topics during a course (between the initial and final assessment);
to examine how the students’ self-assessed knowledge and understanding in geoinformatics subject topics at the end of the course changed between years in different courses (based on the final self-assessment); and
to examine the perceived impacts of the use of the Self-Assessment Tool on students’ studying and the learning processes.
The second research objective also deals with the potential effects of COVID-19 on one of the courses. Based on our empirical data, we discuss our experiences as teachers on the benefits and challenges of self-assessment for developing teaching in geoinformatics.
Methods
Geoportti self-assessment tool
The Self-Assessment Tool was developed in 2019 when Geoportti, the Hub for Finnish Geospatial Research and Education Resources (https://geoportti.utu.fi/), was co-designed and created by the country’s leading universities and research institutions engaged in geospatial research and education. Geoportti is an open research infrastructure consisting of several online accessible research and learning services for Finnish geospatial scientists. The Self-Assessment Tool is one of Geoportti’s Skills Development and Innovation Services, and was designed to support students in their self-assessment of their knowledge of specific geoinformatics subject topics and teachers in teaching geoinformatics. The design of the Tool was done at the University of Turku (UTU), Department of Geography and Geology, and coding was implemented by the UTU IT Services using WordPress.
The original idea behind the Geoportti Self-Assessment Tool was to develop nationally applicable surveys that cover different aspects of geoinformatics in higher education and accumulate longitudinal information. The Self-Assessment Tool can be easily adjusted in terms of topic contents (e.g. drone image acquisition, neighbourhood analysis, cartographic visualisation) and to support learners at multiple performance levels (basic, advanced, expert). Teachers can use existing self-assessments available in Geoportti, or they can create new ones that cover specific learning objectives and course contents. One of the initially planned practical applications of the Tool was to guide students to self-assess their knowledge and understanding related to diverse subject topics and content prior to the course, and then repeat the self-assessment at the end of the course. Hence, it was aimed at giving feedback and supporting the learning process both as a formative and summative assessment (Andrade, Citation2019). Taking a self-assessment was expected to encourage students to reflect upon their learning and to promote their learning self-efficacy (Panadero et al., Citation2017). The anonymous results can also be utilised by the teacher to assess and improve the learning experience (Andrade, Citation2019).
The Self-Assessment Tool gathers all data anonymously. At the beginning of a self-assessment, the student decides whether to consent to storing their answers into a separate, secure database hosted at UTU () or not. While users can take the assessment without allowing their data to be stored, only stored data, including answers to background questions (age, gender, profession, discipline, sector, level of education, and an optional course identifier; ), can be accessed and downloaded by the teacher.
Figure 1. Flowchart of the Geoportti self-assessment Tool from the users’ perspective. 1Nine questions for the UEF geoinformatics advanced course (“Advanced”).
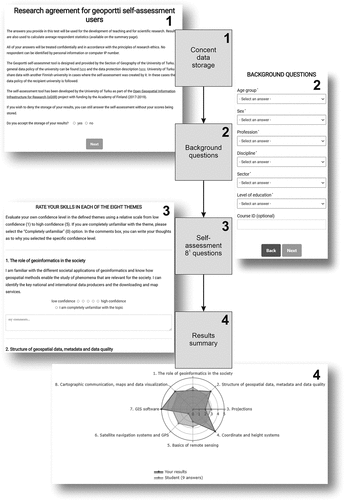
By default, each self-assessment has a teacher-defined number of subject topics (default eight), that cover the main contents of a specific course. Each subject topic includes a short written description to help the respondent assess their knowledge and understanding (). Students are asked to evaluate their own knowledge and understanding in each subject topic using an ordinal scale from low (1) to high knowledge and understanding (5) (https://geoportti.utu.fi/; ). An additional option, Completely unfamiliar (0), is included in case the respondent feels that the topic is completely new. The subject topics are formulated in a way that a student primarily assesses their knowledge and understanding of specific concepts or tasks (c.f. Andrade, Citation2019), not the learning process or its products (). In addition, each self-assessment subject topic includes a text box where the student has an option to express their thoughts on why they chose a specific score (). The optional comments may reveal some aspects of the student’s learning process or its products (c.f. Andrade, Citation2019). After submitting their self-assessment, the student receives a summarised page of their results, including a spider graph (), that compares the student’s scores to the average scores of other respondents. The summarised page can be exported as a PDF document. Teachers can download the data of a group of students (e.g. based on a course identifier) as a spreadsheet document. Results can be compared at the group level between self-assessments made at the beginning and at the end of a course, or between years and courses ().
Figure 2. The spider graph results from the Geoportti self-assessment Tool for three exemplary students in the “geoinformatics advanced course” (UEF 2019) show the change in their self-assessed knowledge and understanding in nine subject topics during the course. Acronyms T1–9 refer to the nine subject topics of the self-assessment (). For example, the scores of student 1 had generally increased, except for subject topic T9 that had decreased by one unit. Note that, unlike a default self-assessment with eight subject topics, this assessment had nine topics. Also, note that the scales of the graphs vary based on the maximum score (4 or 5) of an individual evaluation, and display the actual output from the Tool.
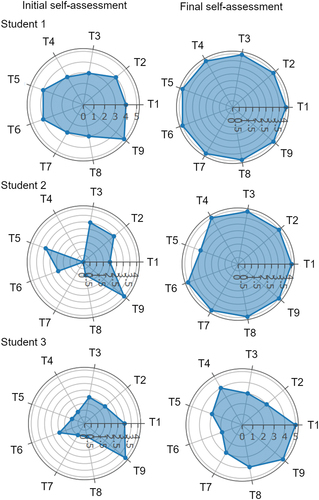
Learning objectives and self-assessments of examined geoinformatics courses
We gathered data between 2019 and 2021 in two Finnish universities, at the University of Turku (UTU) and at the University of Eastern Finland (UEF) within three Bachelor’s and one Master’s level courses in geography (). Each course was held by the same lecturer during the timeframe of this research. We examined self-assessments from a basic-level geoinformatics course that aims at introducing the role of geoinformatics in research and society, basic concepts, different sources of geospatial data, GIS methods and cartographic visualisation (referred to as the “Basic” course later in the text; implemented at UTU in 2020 and UEF in 2020 and 2021). We also examined data from two intermediate-level courses that build upon the basic-level course. The first intermediate-level course is dedicated to the introduction to remote sensing, including its physical basis, Earth observation data, image processing methods, visualisation of multi-spectral data and Earth observation’s role in research (referred to as the “Remote sensing” course, implemented at UTU in 2019 and 2020). The second intermediate-level course deepens the students’ understanding of geoinformatics, i.e. data structures, data production and advanced geospatial and GIS methods (referred to as the “Advanced” course, implemented at UEF in 2019 and 2020). In addition, we examined one Master’s level specialisation course on geoinformatics in the context of participatory spatial planning, introducing related fields and approaches, specialised tools and methods, and ethics and data protection (referred to as the “Participatory mapping” course, implemented at UTU in 2019 and 2021).
Table 1. Details of the self-assessment datasets examined in this study.
The learning objectives of the examined geoinformatics courses were developed separately in each university’s public curriculum (UTU: 2018–2020 and 2020–2022; UEF: 2018–2021), which are generally in effect for 2–3 years. The learning objectives were developed either by the lecturer of the course, or by the group of the local geoinformatics teaching staff. Generally, the curricula for these five geoinformatics courses (four of which were Bachelor’s level courses) emphasised methodological learning objectives (factual, conceptual and procedural knowledge) and lower-order cognitive skills (remembering, understanding and applying; Anderson & Krathwohl, Citation2001). Appendix 1 gives a full description of the learning objectives and course contents of each course.
The lecturer adopted these curriculum-based learning objectives for designing the course structure and learning activities each time the course was held, and introduced them to the students at the beginning of the course. Generally, the courses were divided into subject topics, each covering one part of the methodological content described in the learning objectives. Each subject topic usually corresponded to one of the methodological learning objectives, including the skills to remember concepts related to the method and understand and apply it independently. The learning activities for each subject topic typically included a theoretical lecture, introduction to a practical assignment, and self-paced learning with the practical assignment. Students synthesised their outcomes from the assignment to a report, which was evaluated by the teacher.
Each of the examined courses utilised a self-assessment created for the purposes of the course (, Appendix 1). The intention was that the self-assessments are general enough to be applied in the courses at different universities and by individuals outside the courses. Thus, the subject topics were not directly copied from the curricula of individual universities.
Table 2. The subject topics of the examined self-assessments in brief. See Appendix 1 for detailed descriptions.
Since the authors of this paper were involved in the development of the Geoportti infrastructure, the self-assessments that were utilised in this study were mainly designed by the responsible teacher of the course, and the same self-assessment was used each time the course was held. The self-assessments for the “Basic” course were developed earlier by a former responsible teacher at UTU and used in the subsequent courses at UTU and UEF.
The self-assessments, subject topics reflected the course content, and therefore, mainly reflected the intended learning objectives with lower-order cognitive skills and factual, conceptual, and procedural knowledge (Anderson & Krathwohl, Citation2001). The self-assessment subject topics were formulated as intended learning outcomes, using the learner’s voice. They followed a style that was previously designed for Geoportti self-assessments, often beginning with “I am familiar with … ”, “I know … ”, or “I understand … ”. For all except the Participatory mapping course, the subject topics presented in Appendix 1 are English translations from the original Finnish self-assessments.
Data collection
In most courses, the students took the self-assessment at the beginning and at the end of the course. At UEF, we were able to match the initial and final self-assessment results for the purposes of this study by requesting students to upload their results to the course learning management system as a part of the course’s mandatory tasks. We assigned pseudonymisation codes for the data. For the UEF courses, the responses were analysed only for those students who took both the initial and the final self-assessments. For all courses, corrupted and late returns were excluded from the analyses.
In addition, after finishing the self-assessment at the end of the course, perceived impacts of the use of the Self-Assessment Tool on studying and learning among students were assessed for all courses except the UTU Bachelor-level courses. The students (n = 205) were asked to fill in an online Webropol survey, which included two questions that students were advised to respond briefly in their own words. These questions were: “Reflect on how the use of the Self-Assessment Tool affected your studying during the course.” and “Reflect on how the use of the Self-Assessment Tool affected your learning during the course.” With these two questions, we aimed to understand how the self-assessment was linked to studying-related aspects, such as self-efficacy, motivation, or targeting learning activities (Colthart et al., Citation2008; Knight, Citation2020; Nikou & Economides, Citation2016; Panadero et al., Citation2017; Yan et al., Citation2020), and on the other hand, how it contributed to learning and performance (Andrade, Citation2009, Citation2019). In addition, the same background questions were asked as in the Geoportti self-assessment.
Analysis
First, in order to analyse how the student’s overall self-assessed knowledge and understanding in geoinformatics subject topics changed during the course, the UEF results of the initial and final self-assessments were compared at the individual level. We compared the numeric change in the initial and final self-assessment scores for each course across the years to analyse the positive, negative or no changes in the self-assessed knowledge and understanding. Based on the frequencies and means of the self-assessment scores, we identified the subject topics with highest and lowest scores in the initial and final self-assessments, and which of the subject topics had the largest or smallest increase during the course. Additionally, we read the written descriptions given by the students in relation to the different subject topics. Only some students described the reasons for a specific score and the written responses were few. In particular, the students with a positive trend in their response scores between the initial and final self-assessments rarely left comments, thus making it impossible to obtain a consistent analysis.
Secondly, for all courses, we used the final self-assessment results to analyse the students’ self-assessed knowledge and understanding in geoinformatics subject topics at the end of the course, and how it had changed between two years for different groups of students. We calculated each student’s median score, and examined the distribution of the median scores over the course group to compare courses and years. Moreover, the remote sensing course at UTU offered a chance to examine the influence of changes to teaching methods, made in response to the COVID-19 pandemic, to students’ self-assessed knowledge and understanding. We examined the impact of the transformation of a supervision and peer support-intensive course to an entirely online learning-based course (20 students in 2019 and 34 students in 2020). The changes included a shift from classroom lectures to remote and pre-recorded lectures, from classroom guidance with course assignments to reduced and online guidance, and a distinct reduction in group work and peer support. The role of continuous assessment in the course evaluation also increased slightly, thus requiring the students to put continuous effort toward the course. In addition, we compared the final self-assessed knowledge and understanding in individual course subject topics for all courses, aiming at identifying topics where students were the most or least confident or where their confidence varied the most or least. This was done by examining the distribution of scores for each subject topic. The data allowed us to examine changes in each subject topic from one year to another.
Finally, to study the students’ perceptions on the impacts of utilising the Self-Assessment Tool, the responses written by the students regarding the two questions addressing impacts on studying and learning processes were treated with an inductive content analysis. The responses were repeatedly read, and the most typical impacts that emerged from the responses were identified and coded in a data table in Excel. This was done for each question separately. The coding was refined iteratively. This meant that first, 40 responses were coded and thereafter discussed by three different researchers prior to coding the entire data, which was performed by one researcher. Descriptive statistics were calculated for the diverse impacts, and statistical tests were performed with the Pearson Chi square test to identify associations between the impacts of utilising the Self-Assessment Tool (no impact, negative impact, or positive impact) and the respondents’ background variables of gender, main study field (geography or non-geography), and level of education (Bachelor’s or Master’s).
Results
Changes in self-assessed knowledge and understanding in geoinformatics subject topics during a course
As expected, the majority of the UEF geoinformatics students (74.2–85.1%) showed a positive change in their self-assessed knowledge and understanding between the initial and final assessments in relation to each subject topic across the years (). No change in knowledge and understanding was observed for 13.7–21.5% of students. Both of these change trends were more common in the Basic courses than the Advanced courses. The assessment scores also indicate that some students (1.2–4.9%), particularly in the Advanced course, showed a negative trend in knowledge and understanding.
Table 3. Change (the proportion of students with positive changes, no changes, or negative changes) in their self-assessed knowledge and understanding in geoinformatics subject topics between the initial and final self-assessments in the basic and advanced courses at UEF. See for the frequencies of the self-assessment scores related to each subject topic in each course.
Overall, there were no gender differences in the number of positive or negative change trends (). Regarding the students with no change in knowledge and understanding during a course, the proportion of females was clearly smaller than that of males (~2–7% less depending on the year), except in the Advanced course in 2019. Their self-assessed knowledge and understanding during a course varied more between students who had geography as their main field of study (or not). In the case of a negative change, the majority of students were non-geographers in the Basic 2021 courses and the Advanced 2019 courses, but they were geographers in the Basic and Advanced courses in 2020 (). Among the students indicating no change in knowledge and understanding, geographers were the majority in Basic courses both years, but non-geographers were the majority in Advanced courses both years ().
The subject topic with the highest increase in self-assessed knowledge and understanding between the initial and final self-assessments differed each year, while the topics with the lowest increase were consistent (). The subject topics with the largest increases in self-assessed knowledge and understanding included geospatial data structure, metadata, and data quality (T2) and GIS software (T7) in the Basic course; GIS workflow and its sketching (T4) and network and cost-efficiency analyses, uncertainties, and GWR (T8) in the Advanced course. The subject topic with the smallest increase was the role of geoinformatics in the society (T1) in the Basic course, and data visualisation and thematic maps (T9) in the Advanced course (), as the students who evaluated their knowledge and understanding in that subject topic rated their knowledge of this area as very high in the initial self-assessment, so there was little room for further improvement of the rating.
Table 4. The subject topic where the students had the highest and lowest self-assessed knowledge and understanding in the initial and final assessments in the Basic and Advanced courses at UEF. The acronyms of the subject topic are explained in (i.e. Tn). Each self-assessment subject topic is mentioned briefly when first mentioned.
In the Basic course, the written descriptions indicated that the self-assessments with a negative trend were associated with certain subject topics, such as familiarity with GIS software (T7; three students in 2021), projections (T3; four students in 2020), and cartographic communication, maps and data visualisation (T8; four students in 2020). Descriptions also highlighted the role of the student’s primary field of study. For example, two minor subject students raised the fact that despite having become familiar with the GIS software and analyses, they did not yet feel that they were experts in applying the software. In the Advanced course, six subject topics had individual (1–3 students) reports of decreased self-assessed knowledge and understanding. The written descriptions revealed that in the case of some students, the negative trend was due to not understanding the subject topic descriptions in detail. In some cases, the written descriptions were also contradictory to the scores given.
Self-assessed knowledge and understanding in geoinformatics subject topics at the end of the course and changes between years in different courses
Across all courses and subject topics, the median self-assessed knowledge and understanding at the end of the course was 4 (). The shape of the score distribution was similar for all Basic courses, with a notable peak at score 4, a low frequency of scores 2 and 5 and an intermediate frequency of score 3 (). At UEF in 2021, the frequency of the highest scores (5) was notably lower, and the frequency of score 3 higher than the previous year ().
Figure 3. Students’ self-assessed median knowledge and understanding (1 = low, 5 = high) at the end of a course across all course subject topics.
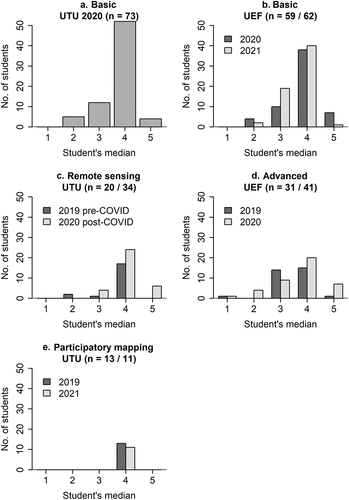
For the UTU Remote sensing course, the distribution showed a tail of low-to-intermediate scores 2 and 3 in 2019, but the median scores were higher in 2020 (). Transforming the Remote sensing course to an online course in response to the COVID-19 pandemic was accompanied with higher self-assessment ratings than the previous year ().
Students in the UEF Advanced course rated their overall self-assessed knowledge and understanding along a wide range from 1 to 5 in both years (). It was the only course where the median rating by some students was at the lowest end of the range, creating the largest variation between individual students. However, the frequency of scores 4 and 5 was higher in 2020 than the previous year. In the Participatory mapping course, the median score of all students during both years was 4 ().
In the Basic courses at both universities, the students were generally the most confident in cartographic communication, maps and data visualisation (T8, ). Regarding the role of geoinformatics in society (T1), the students were most unanimous, with almost no variation around score 4 (). At UEF, students had the least self-assessed knowledge and understanding in the basics of remote sensing (T5), and there was also the most variation between students in that subject topic (). At UTU, knowledge and understanding was more evenly distributed between subject topics, but projections, coordinate systems, and remote sensing (T3–5) were considered more difficult than others ().
Figure 4. Distribution of self-assessments scores at the end of a course across the course subject topics. See for the description of course subject topics.
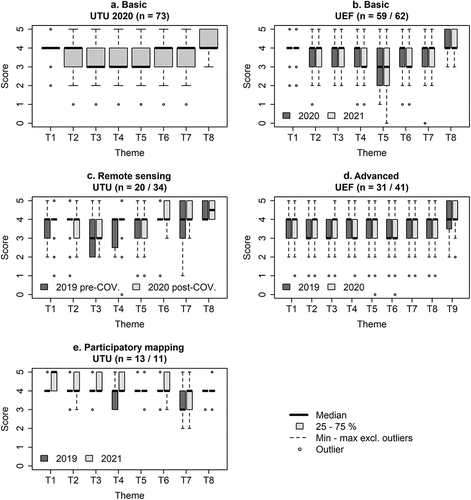
In the Remote sensing course, self-assessed knowledge and understanding in remote sensing applications was the highest in both years (T8) and photogrammetry was the lowest (T3) (). Generally, subject topics 1–5 scored lower than the topics 6–8 (). The first five topics covered the physical basis of remote sensing, remote sensing systems, different types of corrections, and image processing. Other than that, the two years had notably different distributions in scores between subject topics. Subject topic 4, geometric and atmospheric corrections, had the most uneven scores in 2020, ranging from 0 to 5 ().
In the Advanced courses at UEF, the students had the highest self-assessed knowledge and understanding in visualising data and designing thematic maps (T9) in both years (). All other subject topics had scores 3–4 (). Subject topics 2, 3, 4, and 9, related to data and workflow and its sketching, showed notable differences between the years ().
The Participatory mapping course showed a large variation in scores across different subject topics (). The subject topics with the lowest scores, particularly in 2019, were 4 and 7 (), corresponding to ethical issues and data management and protection (). While the students were generally unanimous in their self-assessed knowledge and understanding, their scores in subject topic 7 (data management and protection) varied the most ().
Perceived impacts of the use of the self-assessment tool on students’ studying and learning processes
More than half of the students (54.0%, n = 206) indicated that the use of the self-assessment did not affect their studying in any way or it created unnecessary work (). The most frequently identified positive impacts on studying, identified by 46.0% of the students, were introduction to course topics and help for assessing knowledge and understanding at the beginning of the course. These were more prominently identified by women than men (χ2(2) = 14.253, p < 0.001***, ). Approximately half of the students (48.5%) believed that the use of self-assessment did not impact their learning (). However, 51.5% of the students indicated positive impacts, mainly that the Tool helped to identify that the students had developed their knowledge and understanding and concretely identified topics that they learned during the course.
Figure 5. Individual categories denoting the impacts of utilising the Geoportti self-assessment Tool on studying and learning processes as perceived by the students. A response from one student (n = 206) could link to several impacts. In total, the various impacts were mentioned 285 times for studying, and 262 times for learning (100%).
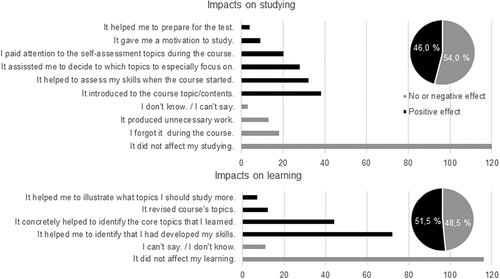
Discussion
In this article, we empirically demonstrated the usefulness of an online self-assessment tool developed specifically for learning geoinformatics and developing teaching in geoinformatics in higher education. In light of the results, as the teachers of the examined courses, we discussed below the benefits and challenges of the self-assessment approach and the Geoportti Self-Assessment Tool.
At the beginning of a course, self-assessment results provide teachers with valuable information regarding the variation in students’ knowledge and understanding in different subject topics, and potentially reflect their starting level and motivation. In line with previous research (Andrade, Citation2019), we believe this information helps teachers pay attention to subject topics and student groups that may need more attention during the course. Creating the self-assessment survey content prior to a course also supports the constructive alignment (Biggs & Tang, Citation2011) of teaching geoinformatics by targeting intended learning objectives and activities of each course. We also find it useful that the quantitative comparison of the initial and final self-assessment results allows teachers to examine the learning process at the group level, and identify subject topics with particularly low or high increases in self-assessed knowledge and understanding during a course. Furthermore, teachers can identify if there are students who do not experience an increase in knowledge and understanding, or experience increased confusion in some of the course subject topics.
A closer look at UEF students’ development during a course revealed, as expected, that a clear majority of the students experienced an increase in knowledge and understanding. However, some students experienced either a negative or no change in knowledge and understanding in specific subject topics. Generally, based on our data, gender does not have an impact on the (positive, no or negative) change in self-assessed knowledge and understanding during a geoinformatics course. Then again, non-geography students in some courses may be more likely to experience a negative change in their knowledge and understanding than geography students, but the results are generally independent of the student’s discipline. This is likely the result of a common practice in geoinformatics teaching where the focus is mostly on the technology, methods, and applications instead of teaching that requires a theoretical background knowledge of geography (Kedron et al. Citation2016).
The changes in the self-assessed knowledge and understanding during a course showed that the self-assessment highlighted various subject topics where the changes were the most prominent, although at the beginning of the courses, the scores were more consistent. This indicates heterogeneity in students’ efforts during the course, and potentially subjective targeting in specific learning objectives based on the initial self-assessment (Colthart et al., Citation2008). This is, hence, in line with the initial aims of the Geoportti Self-Assessment Tool.
While numerical self-assessment questions were quicker to respond and more straightforward to analyse, written descriptions revealed important aspects of the students’ experienced knowledge and understanding. These responses indicated various reasons, particularly for negative changes in self-assessed knowledge and understanding. In our data, these include differences in the students’ background and confusion about the subject topic descriptions. These responses highlight that it is important to use language that students understand easily when describing the specific subject topics. As an example, both the lectures and exercises in the Advanced course at UEF included overlay and neighbourhood analyses; thus, the result that the student felt least competent in these topics is surprising. We assume this could be explained by insufficient terminological knowledge, i.e. students had not recognized from the descriptions the skills they actually had learned in the course.
The final self-assessment results provide information on the experienced knowledge and understanding at the end of the course, which we consider to be relevant information for the teacher since the summative assessment is performed by the teacher. For example, in our case study, the teachers of all examined courses found that the learning objectives were generally well-fulfilled since individual students rated their self-confidence at the end of the course relatively high (median 4). As the results of self-assessments have been noted to indicate learners’ motivation and satisfaction (Sitzmann et al., Citation2010), we were able to conclude that it was rather high on the examined courses.
Self-assessment results, and particularly their comparison between the years, gave insights on the impact of changes in teaching arrangements on the students’ learning experience. For example, our results suggest that the student’s self-assessed knowledge and understanding at the end of the course did not suffer from the impacts of COVID-19 and subsequent changes in the teaching and learning methods, which is in line with a previous study on the transition to remote learning (Chang et al., Citation2014). We acknowledge the fact that not all changes were related to the pandemic situation and online transformation due to the considerable changes in the structure of the course between the two years. While the summative results improved, the number of course dropouts increased. We hypothesise that the increase in self-regulated and independent learning increased the self-efficacy of most students, which was clearly seen in the self-assessment data. At the same time, it created an insurmountable obstacle for certain students, whose input is missing from our final self-assessment data. It has been pointed out that self-assessment and self-assessment skills are vital since they guide learners to target their learning activities in self-regulated learning (Khoiriyah et al., Citation2015; Koston et al., Citation2012), e.g. in online courses that offer greater freedom or more generally in life-long learning. Hence, based on our experience, we follow this recommendation to use self-assessment both at the beginning and at the end of a course in relation to geoinformatics teaching in the remote mode.
Based on students’ perceptions of the perceived effects of utilising the Self-Assessment Tool on studying and learning processes, half of the students had positive opinions. In line with previous research (Bourke, Citation2016; Colthart et al., Citation2008; Khoiriyah et al., Citation2015), self-assessment helped the learners to focus on course subject topics and concretely identify topics that they learned during the course. We expect this to increase their self-efficacy in geoinformatics-related tasks (Bandura, Citation1986). However, self-reflection and motivation are not strongly evident in our results. In fact, in terms of studying, 20% of the negative comments highlighted that the self-assessment task produced unnecessary work or that the students forgot it during the course. For most students, the use of the Self-Assessment Tool was most likely driven by an extrinsic motivation, such as a task to complete during a course, and only some students connected it to an intrinsic motivation being meaningful and rewarding, and thus, a goal by itself (Deci & Ryan, Citation2012). However, the usefulness was studied based on open responses that were short in some cases as the students might have had low level of interest in responding to the survey at the end of the course. Nevertheless, we consider that an important factor to increase intrinsic motivation is that the teacher actively informs the students about the relevance of the self-assessment for their studying and learning to increase their awareness of learners’ active participation in the learning process.
While our results clearly demonstrate the usefulness of self-assessment in geoinformatics education, the data must be examined with its limitations under consideration. To start with, all self-assessment results should be interpreted, acknowledging that the students will rate their knowledge and understanding subjectively. Hence, both the students’ individual characteristics (e.g. concentration capacity, personal traits and motivation) and their interpretation of the situation (e.g. interpretation of the assessment’s influence on the course grade or the student-teacher relationship) have an impact on the scores given. Also, the representativeness of the responses should be considered. Our experience demonstrates that, naturally, the voice of the course dropouts is not heard through the final self-assessments even if the assessment is obligatory. This means that the experience of those students who struggle the most should be examined with different kinds of methods.
The results from a self-assessment can guide the development of the course syllabus, and from a wider perspective, the curriculum. Although, a critical examination of the used subject topics and how well they describe the intended learning outcomes is needed. We recognize that the subject topics of the self-assessments presented in this article mainly focused on the intended learning objectives from the perspective of lower-order cognitive skills. The descriptions were partly in line with the terminology of Bloom’s revised taxonomy, but in retrospect, the self-assessment subject topics should have been formulated more uniformly with unambiguous verbs from the Bloom’s (revised) taxonomy (Anderson & Krathwohl, Citation2001), beginning with “I remember/understand/can apply … ”. In addition, in some courses described in this paper, students might have underestimated their knowledge and understanding of a specific subject topic due to combining several learning objectives for a specific topic in the question (e.g. T8 on Basic course of UTU and UEF). Therefore, the results might be different if only discrete learning objectives had been distinguished in the assessment instead of the subject topics. On the other hand, in that case, it might be that the students could also assess more specific subject topics in a more critical way. However, as a down side, including all learning outcomes and their sub-categories in a very detailed way would make the self-assessment longer and compromise it as a quick approach to be used at the beginning and the end of a course. Furthermore, applying specific learning outcomes in the assessment would have changed the whole intention of the self-assessment approach. The intention was to keep the Geoportti Self-Assessments Tool general enough to be applied in the courses at different universities and by individuals outside the courses. Thus, the questions were not directly copied from the curricula of individual universities, and the subject topics do not directly map onto learning outcomes. If the students participated in lectures, completed the related exercises and report writing, they would know all the aspects of each subject topic under question in the self-assessment tool at the end of the courses. Therefore, we consider the approach reliable. Further, in the initial self-assessment at the beginning of the course, the teachers wanted to include all these different aspects in the subject topics, so that this self-assessment would simultaneously introduce the subject topics taught in the courses more in detail to the students than if it was only based on learning outcomes. Thus, taking the self-assessment also acts as an introduction to the course and shows the most important subject topics to be taught during the course.
Internationally, a collective effort by academic, business and public sector actors has been made to guide curriculum development, and identify standards for the knowledge and competencies of geoinformatics and Earth observation professionals. These include, for example, the GIS and Technology (GIS&T) Book of Knowledge (BoK) (DiBiase et al., Citation2006), its European update in the GI-N2K project (Vandenbroucke & Vancauwenberghe, Citation2016), and the EO4GEO BoK, which adds the concepts and skills that are specific to Earth observation (Hofer et al., Citation2020). The use of such resources to guide the competency-oriented curriculum design in higher education has been successful previously (e.g. Prager & Plewe, Citation2009). These resources could be used to further develop the Geoportti Self-Assessment Tool, and create self-assessments that go further beyond the syllabus of a single course and focus on intended learning outcomes and the knowledge and understanding of geoinformatics professionals in general. While the international standards provide a good structure for teaching and curriculum planning, they are still only guidelines, and the actual design of courses will always be context-dependent (Prager, Citation2011). Therefore, when implemented well, the self-assessment can identify context-specific shortcomings in teaching and motivate the teacher to focus on the development of specific parts in the course.
Conclusions
We conclude that self-assessment implemented at the beginning and the end of a course, both as a formative and summative assessment, is useful for higher education in geoinformatics. The Geoportti Self-Assessment Tool concretely supports students’ learning process by visually illustrating their self-assessed level of knowledge and understanding across various course subject topics, and by helping to identify subject topics and learning objectives that require specific attention. This is beneficial for self-efficacy in geoinformatics-related tasks. Similarly, self-assessment supports constructive alignment in teaching geoinformatics and the identification of group level competencies, as well as specific subject topics or groups of students that would benefit from attention by teachers. We also consider that self-assessment at the end of the course provides teachers with relevant information regarding the fulfilment of the learning objectives, supporting summative assessment (such as exams). Furthermore, we found particular support toward the application of self-assessments for remote teaching. However, it is crucial to ensure that the students understand the contents of the self-assessment subject topics. In order to increase students’ motivation towards self-assessment as an approach in geoinformatics, or any other subject, it is crucial that the teacher actively highlights how it supports their studying and learning. In the future, the Geoportti Self-Assessment Tool may enable additional research on the relationship between the final self-assessed knowledge and understanding and the results of the summative assessment. It would also be important to systematically gather teachers’ perceptions on the usefulness of the Self-Assessment Tool. Finally, the Tool is generic, and could be applied in other disciplines and for other types of skill sets than geoinformatics.
TableS6_Advanced_UEF_2019_final.csv
Download Comma-Separated Values File (855 B)TableS1_Basic_UTU_2020_final.csv
Download Comma-Separated Values File (1.8 KB)TableS2_Basic_UEF 2020_final.csv
Download Comma-Separated Values File (1.4 KB)TableS4_RemoteSensing_UTU_2019_final.csv
Download Comma-Separated Values File (524 B)TableS8_ParticipatoryMapping_UTU_2019_final.csv
Download Comma-Separated Values File (356 B)TableS7_Advanced_UEF_2020_final.csv
Download Comma-Separated Values File (1.1 KB)TableS3_Basic_UEF_2021_final.csv
Download Comma-Separated Values File (1.5 KB)TableS5_RemoteSensing_UTU_2020_final.csv
Download Comma-Separated Values File (860 B)TableS10_Impacts_on_studying_and_learning.csv
Download Comma-Separated Values File (59.4 KB)TableS9_ParticipatoryMapping_UTU_2021_final.csv
Download Comma-Separated Values File (308 B)Acknowledgements
The contribution of N.K. and V.A. was supported by the Transferring contact, technology- and field-based education to digital: methods and tools for geosciences training (DigiGeo, project no. 2020-1-CZ01-KA226-HE-094425), funded by the European Union within the Erasmus + program. The contribution of R.K. was supported by Open Geospatial Information Infrastructure for Research (oGIIR, grant number 306537), funded by the Academy of Finland.
Disclosure statement
No potential conflict of interest was reported by the author(s).
Data availability statement
The data supporting the analysis presented in this article is available in the Supplementary Material. However, the UEF data regarding initial and final self-assessment results is not published due to the potential identification of individual student responses.
Supplementary data
Supplemental data for this article can be accessed online at https://doi.org/10.1080/03098265.2023.2251021.
Correction Statement
This article was originally published with errors, which have now been corrected in the online version. Please see Correction (http://dx.doi.org/10.1080/03098265.2023.2263227).
Additional information
Funding
References
- Anderson, L. W., & Krathwohl, D. R. (Eds.). (2001). A taxonomy for learning, teaching, and assessing: A revision of Bloom’s taxonomy of educational objectives. Addison Wesley Longman, Inc.
- Andrade, H. L. (2009). Students as the definitive source of formative assessment: Academic self-assessment and the self-regulation of learning. In H. L. Andrade & G. J. Cizek (Eds.), Handbook of formative assessment (pp. 90–105). Routledge. https://doi.org/10.4324/9780203874851
- Andrade, H. L. (2019). A critical Review of research on student self-assessment. Frontiers in Education, 87(4). https://doi.org/10.3389/feduc.2019.00087
- Bandura, A. (1977). Self-efficacy: Toward a unifying theory of behavioral change. Psychological Review, 84(2), 191–215. https://doi.org/10.1037/0033-295x.84.2.191
- Bandura, A. (1986). Social foundations of thought and action: A social cognitive theory. Prentice-Hall.
- Biggs, J. B., & Tang, C. (2011). Teaching for quality learning at university: What the student does. McGraw-hill education (UK).
- Bol, L., Hacker, D. J., Walck, C. C., & Nunnery, J. A. (2012). The effects of individual or group guidelines on the calibration accuracy and achievement of high school biology students. Contemporary Educational Psychology, 37(4), 280–287. https://doi.org/10.1016/j.cedpsych.2012.02.004
- Bourke, R. (2016). Liberating the learner through self-assessment. Cambridge Journal of Education, 46(1), 97–111. https://doi.org/10.1080/0305764X.2015.1015963
- Brown, G. T. L., & Harris, L. R. (2013). Student Self-Assessment. In SAGE handbook of research on classroom assessment (pp. 367–393). SAGE Publications, Inc. https://doi.org/10.4135/9781452218649.n21
- Chang, C.-C., Liang, C., & Chen, Y.-H. (2013). Is learner self-assessment reliable and valid in a web-based portfolio environment for high school students? Computers & Education, 60(1), 325–334. https://doi.org/10.1016/j.compedu.2012.05.012
- Chang, C.-C., Shu, K.-M., Liang, C., Tseng, J.-S., & Hsu, Y.-S. (2014). Is blended e-learning as measured by an achievement test and self-assessment better than traditional classroom learning for vocational high school students?. IRRODL 15. https://doi.org/10.19173/irrodl.v15i2.1708
- Chilton, H., & McCracken, W. (2017). New technology, changing pedagogies? Exploring the concept of remote teaching placement supervision. Higher Education Pedagogies, 2(1), 116–130. https://doi.org/10.1080/23752696.2017.1366276
- Colthart, I., Bagnall, G., Evans, A., Allbutt, H., Haig, A., Illing, J., & McKinstry, B. (2008). The effectiveness of self-assessment on the identification of learner needs, learner activity, and impact on clinical practice: BEME guide no. 10. Medical Teacher, 30(2), 124–145. https://doi.org/10.1080/01421590701881699
- Cook, D. A., & Dupras, D. M. (2004). A practical guide to developing effective web-based learning. Journal of General Internal Medicine, 19(6), 698–707. https://doi.org/10.1111/j.1525-1497.2004.30029.x
- Deci, E. L., & Ryan, R. M. (2012). Motivation, Personality, and Development within Embedded Social Contexts: An Overview of Self-Determination Theory. In R. M. Ryan (Ed.), Oxford Handbook of Human Motivation (pp. 85–107). Oxford, UK: Oxford University Press. https://doi.org/10.1093/oxfordhb/9780195399820.013.0006
- DiBiase, D., DeMers, M., Johnson, A., Kemp, K., & Taylor Luck, A. (2006). Geographic information science and technology body of knowledge(Plewe B. (Eds.) 1st ed. Association of American Geographers.
- Epstein, R. M., Siegel, D. J., & Silberman, J. (2008). Self-monitoring in clinical practice: A challenge for medical educators. Journal of Continuing Education in the Health Professions, 28(1), 5–13. https://doi.org/10.1002/chp.149
- Gong, X., Wang, Y., & Zhang, J. (2017). The self-assessment in e-learning and personalized feedback education. Proceedings of the 2017 9th International Conference on Education Technology and Computers - ICETC 2017, 141–145. https://doi.org/10.1145/3175536.3175571
- Goodchild, M. F. (2009). Geographic information systems and science: Today and tomorrow. Annals of GIS, 15(1), 3–9. https://doi.org/10.1080/19475680903250715
- Heckhausen, J., & Heckhausen, H. (Eds.) (2018). Motivation and action (3rd). Springer. https://doi.org/10.1007/978-3-319-65094-4
- Hofer, B., Casteleyn, S., Aguilar-Moreno, E., Missoni‐Steinbacher, E.-M., Albrecht, F., Lemmens, R., Lang, S., Albrecht, J., Stelmaszczuk‐Górska, M., Vancauwenberghe, G., & Monfort‐Muriach, A. (2020). Complementing the European earth observation and geographic information body of knowledge with a business-oriented perspective. Transactions in GIS, 24(3), 587–601. https://doi.org/10.1111/tgis.12628
- Kendron, P., Frazier, A., Greene, C., & Mitchell, D. (2016). Curriculum design for upper- and Advanced-level GIS classes: Are New skills being taught and Integrated? Journal for Geographic Information Science, 4(1), 324–335. https://doi.org/10.1553/giscience2016_01_s324
- Khogali, S. E. O., Davies, D. A., Donnan, P. T., Gray, A., Harden, R. M., Mcdonald, J., Pippard, M. J., Pringle, S. D., & Yu, N. (2011). Integration of e-learning resources into a medical school curriculum. Medical Teacher, 33(4), 311–318. https://doi.org/10.3109/0142159X.2011.540270
- Khoiriyah, U., Roberts, C., Jorm, C., & Van der Vleuten, C. P. M. (2015). Enhancing students’ learning in problem based learning: Validation of a self-assessment scale for active learning and critical thinking. BMC Medical Education, 15(140). https://doi.org/10.1186/s12909-015-0422-2
- Knight, A. (2020). Using self-assessment to build self-efficacy and intrinsic motivation in athletes: A mixed methods Explanatory design on Female adolescent volleyball players. The Qualitative Report, 25(2), 320–346. https://doi.org/10.46743/2160-3715/2020.3737
- Koston, D., van Gog, T., & Paas, F. (2012). Training self-assessment and task-selection skills: A cognitive approach to improving self-regulated learning. Learning and Instruction, 22(2), 121–132. https://doi.org/10.1016/j.learninstruc.2011.08.004
- Lopez, R., & Kossack, S. (2007). Effects of Recurring use of self-assessment in University courses. The International Journal of Learning: Annual Review, 12(4), 203–216. https://doi.org/10.18848/1447-9494/CGP/v14i04/45277
- Mathews, A. J., & Wikle, T. A. (2019). GiS&T pedagogies and instructional challenges in higher education: A survey of educators. Transactions in GIS, 23(5), 892–907. https://doi.org/10.1111/tgis.12534
- Nikou, S. A., & Economides, A. A. (2016). The impact of paper-based, computer-based and mobile-based self-assessment on students’ science motivation and achievement. Computers in Human Behavior, 55, 1241–1248. https://doi.org/10.1016/j.chb.2015.09.025
- Panadero, E., Brown, G. T. L., & Strijbos, J.-W. (2016). The future of student self-assessment: A Review of known unknowns and potential directions. Educational Psychology Review, 28(4), 803–830. https://doi.org/10.1007/s10648-015-9350-2
- Panadero, E., Jonsson, A., & Botella, J. (2017). Effects of self-assessment on self-regulated learning and self efficacy: Four meta-analyses. Educational Research Review, 22, 74–98. https://doi.org/10.1016/j.edurev.2017.08.004
- Prager, S. D. (2011). Using the GIS&T body of knowledge for curriculum design: Different design for different contexts. In D. J. Unwin, K. E. Foote, N. J. Tate, & D. DiBiase (Eds.), Teaching geographic information science and technology in higher education (p. 498). First Edition.
- Prager, S. D., & Plewe, B. (2009). Assessment and evaluation of GIScience curriculum using the geographic information science & technology body of knowledge. Journal of Geography in Higher Education, 33(sup1), 46–69. https://doi.org/10.1080/03098260903034012
- Sitzmann, T., Ely, K., Brown, K. G., & Bauer, K. N. (2010). Self-assessment of knowledge: A cognitive learning or affective measure? Academy of Management Learning & Education, 9(2), 169–191. https://doi.org/10.5465/amle.9.2.zqr169
- Thompson, G., Pilgrim, A., & Oliver, K. (2005). Self-assessment and reflective learning for first-year University geography students: A simple guide or simply misguided? Journal of Geography in Higher Education, 29(3), 403–420. https://doi.org/10.1080/03098260500290959
- Vandenbroucke, D., & Vancauwenberghe, G. (2016). Towards a new body of knowledge for geographic information science and technology. International Scientific Journal: Micro Macro & Mezzo Geo Information, 6, 7–19. https://mmm-gi.geo-see.org/wp-content/uploads/MMM-GI_6/Vandenbroucke_D-Vancauwenberghe-G.pdf
- Yan, Z., Chiu, M. M., & Ko, P. Y. (2020). Effects of self-assessment diaries on academic achievement, self-regulation, and motivation. Assessment in Education-Principles Policy & Practice, 27(5), 562–583. https://doi.org/10.1080/0969594X.2020.1827221
- Zimmerman, B. J. (1989). A social cognitive view of self-regulated academic learning. Journal of Educational Psychology, 81(3), 329–339. https://doi.org/10.1037/0022-0663.81.3.329
- Zimmerman, B. J., & Schunk, D. H. (Eds.). (2011). Handbook of self-regulation of learning and performance. Routledge. https://doi.org/10.4324/9780203839010
Appendices
Appendix 1. Detailed descriptions of geoinformatics courses studied in this article and the Geoportti self-assessment subject topics.
BASIC
Learning objectives, course content and self-assessment subject topics for the courses “Introduction to Geoinformatics” at UTU and “Introduction to Geoinformatics and Cartography” at UEF1.
Learning objectives (UTU): After completing the course, the student is able to: describe the central application of geoinformatics in society and in geography, explain and use the basic concepts of geoinformatics, search for geospatial data online from different key sources, use the desktop GIS software (QGIS) and web-based map and data services to view spatial data, produce and modify simple spatial data, analyse spatial data using basic methods, and visualise the spatial data as maps using the basic principles of cartographic communication.
Course content (UTU): The course is divided into the following interconnected subject topics: geospatial applications in society, spatial data in Finland, Mapmaking and cartography, projections and coordinate reference systems, field measurements and remote sensing, geographic information systems (GIS) and software, location techniques and applications, spatial analyses and interpretation of spatial data, cartography and geospatial visualisation, web map and data services. During the course, the students will mainly use open-source GIS software, data and online map and data services.
Learning objectives (UEF): In the practical sessions, the students learn how to manage, analyse, and produce thematic maps from different data sets using the ArcGIS software. After the completion of the course, the student is able to independently produce thematic maps based on spatial data sets, and by using good principles of cartographic visualisation and map-making.
Course content (UEF): The goal of the course is to familiarise the student with the basic methods of geospatial data analysis and cartographic presentation through multiple practical examples. The lectures on cartography focus, among other topics, on the history of mapping in Finland, projections, coordinate reference systems, and the cartographic principle of making thematic maps. In the lectures on geoinformatics, the student becomes familiar with different geospatial data types, basic analyses, and with data access and key data sources online. In the practical sessions, the students learn how to modify, manage, and analyse geospatial data and how to produce thematic maps.
Subject topics (T) and their descriptions in the Geoportti self-assessments (English translation by the authors):
1The UTU self-assessment for the course Introduction to Geoinformatics was also taken in the corresponding course at UEF. Due to slight differences in the course content, (i.e., the measurement and usage of self-gathered data (T6) is not covered by this course at UEF), the UEF students were instructed to evaluate their confidence in one subject topic based on a more restricted criteria (namely in subject topic 6, to only evaluate the part “I understand the operating principles of satellite navigation systems and related sources of error” and to ignore the part “I know how to measure the location by using a GPS receiver, and to analyse and modify my own data with GIS software”).
REMOTE SENSING
Learning objectives, course content and self-assessment subject topics for the course “Methods in Remote Sensing” at UTU.
Learning objectives: After completing the course, the students: can identify different ways that remote sensing based approaches can be used in society, know where to find and search for different open remote sensing data, find out how a remote sensing data set has been produced and what kind of different qualities it has, evaluate the suitability of a data set for a specific-use case, process multispectral remote sensing imagery in remote sensing and GIS software (such as SNAP, ERDAS IMAGINE, QGIS, and Cloud Compare), process 3D point cloud data, use basic automatic image processing methods, visually interpret remote sensing imagery using a systematic approach, and participate in the planning and implementation of a simple laser scanning project with the help of written instructions for software and hardware.
Content: The course is divided in to the following subject topics: introduction to remote sensing of the environment, electromagnetic radiation and the physical principles of remote sensing, remote sensing systems, data sets and software, aerial photography and photogrammetry, visual interpretation of remote sensing images, multispectral remote sensing of the environment from space, atmospheric and geometric rectifications, radiometric, spectral and spatial processing methods, classification of multispectral image, principles of laser scanning and point cloud data processing, and application of remote sensing in society.
Subject topics (T) and their descriptions in the Geoportti self-assessments (English translation by the authors):
ADVANCED
Learning objectives, course content and self-assessment subject topics for the “Geoinformatics advanced course” at UEF.
Learning objectives: The student has gained an understanding of advanced geospatial analysis methods and application possibilities of geoinformatics. The student understands in what type of research the analysis methods can be applied, and the type of opportunities that are provided by geospatial data sets and what the limitations are in their usage. The student is able to apply his/her cartographic and other geospatial skills in working-life within the university, and in both the public and the private sector.
Content: During the course, it is continued (continuation to the Introduction to Geoinformatics and Cartography -course) in getting familiar with the basic concepts of geoinformatics and its special characteristics, and with geospatial analysis skills by using different data sets. The lectures focus on the theoretical background and special characteristics of geoinformatics. The practical sessions are done using ArcGIS software, and focus on a variety of analysis methods using different data sources. The teaching materials are the different (population, agriculture, snow cover, etc.) vector and raster data sets.
Subject topics (T) and their descriptions in the Geoportti self-assessments (English translation by the authors):
PARTICIPATORY MAPPING
Learning objectives, course content and self-assessment subject topics for the “Participation, spatial planning and GIS” at UTU.
Learning objectives: After the completion of the course, the student can explain ways in which geospatial technologies and data can strengthen recognition and argumentation of stakeholder values and preferences in participatory spatial planning. The student identifies the core values of public participation, and how participation can support the processes of democratic spatial planning and resource management. The student is able to describe Public Participation GIS (PPGIS), Participatory GIS (PGIS) and citizen science methods and practices, and identify their various applications in research and planning processes. The student can describe how place-based knowledge has been applied in participatory landscape and land-use planning processes and natural resource management. The student can apply GIS and other methods to analyse data created through participatory mapping. The learning methods of the course enhance the student’s skills in coordinating and conducting a project in a group, in problem-solving, in scientific writing, and in oral presentation.
Course content: Lectures have focused on theoretical, conceptual, and methodological aspects of stakeholder participation, place-based approaches (PPGIS/PGIS, citizen science), and landscape and land-use planning. Students will get acquainted with different modes of planning through different case studies, both from Finland and abroad. In group work, students use geospatial data and GIS to manage, analyse, and present PPGIS/PGIS data as maps and through other visual and statistical means. Students apply their knowledge and understanding to formulate a plan for their project, which is then analytically executed and reported in the final course report.
Stakeholder participation in spatial planning is a precondition for achieving sustainable societies and equal opportunities for wellbeing, both for people and the planet. The course touches particularly on Sustainable Development, Goals 11; Sustainable Cities and Communities, and Goal 15; Life on Land.
Subject topics (T) and their descriptions in the Geoportti self-assessments (English translation by the authors):
Appendix 2.
Tables
Table A.1. The frequencies of the self-assessment scores related to each subject topic given in the Basic and Advanced courses at UEF. The largest frequencies are colored. The comparison of scores between the initial and final self-assessments shows the negative, no change (same value in initial and final self-assessment), or positive change in the self-assessed knowledge and understanding between the initial and final self-assessments.
Table A2. The percentages (as a percentage of all students) of gender and main study field categories among students with positive, no, and negative change trends between the initial and final self-assessments. See the n values for respondents from .
Table A3. Differences (percentages) between the student’s background and the perceived impact of utilising the Geoportti self-assessment tool on studying and learning, which is distinguished based on the “no or negative” impacts and positive impacts. Statistically significant differences between groups were tested through the Pearson chi square test (the significant result is bolded).