Abstract
In recent years, many studies have been used in feed-forward neural network (FNN) to develop decision-making systems. The primary objective is to get the least error by finding the best combination of control parameters. It has been observed that FNNs using meta-heuristics techniques always converges very quickly towards the optimal positions but suffers from slow searching speeds at later stages of generation. Due to slow convergence, it is a prevalent phenomenon that traditional optimization does not ensure to find global optima. As a result, it falls under local optima. Recently, another meta-heuristic optimization-based algorithm called sine cosine algorithms (SCA) was introduced to solve the aforementioned issues. The algorithm is fundamentally predicated on two trigonometric functions, one being sine and the other being cosine. However, like other traditional approaches, SCA has a tendency to be stuck in sub-optimal regions due to poor exploration and exploitation capabilities. This paper proposes an improved version of SCA named chaotic oppositional SCA (COSCA) by integrating with chaos theory and oppositional based learning into the SCA optimization process. It is an incipient training method employed to train an FNN. Three benchmark problems are used to examine the precision and performance of FNNs equipped with COSCA, COPSO, OSCA, SCA, PSO, and backpropagation. The experimental results showed that, relative to other meta-heuristic optimization techniques, the COSCA technique is able to improve performance.
Disclosure statement
No potential conflict of interest was reported by the author(s).
Additional information
Notes on contributors
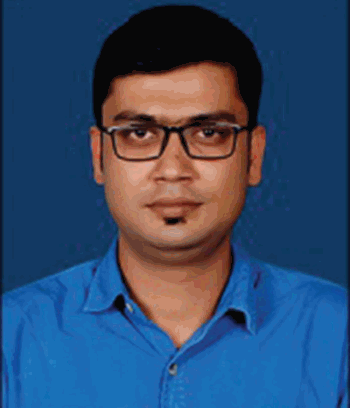
Rana Pratap Mukherjee
Rana Pratap Mukherjee was born in Bankura, West Bengal, India, in 1990. He obtained an MCA degree in computer applications from the Maulana Abul Kalam Azad University of Technology, West Bengal, in 2015. He is now pursuing PhD and working as a software engineer in the operations department at Capillary Technologies, Bangalore, India. His research interest includes machine learning, deep learning, optimization, natural language processing, computer vision, etc. Corresponding author. Email: [email protected]

Provas Kumar Roy
Provas Kumar Roy obtained BTech and PhD degrees in electrical engineering from National Institute of Technology Durgapur. He received his master's degree in electrical machines from Jadavpur University. Presently, he is working as a professor in the Electrical Engineering Department at Kalyani Government Engineering College, West Bengal, India. He was the recipient of the Outstanding Reviewer Award for IJEPES (Elsevier, 2018), EAAI (Elsevier, 2017), Renewable Energy Focus (Elsevier, 2018), ASEJ (Elsevier, 2017). He was also the recipient of the Top Reviewer Award from Publon in 2019. He has published more than 160 research papers in national/international journals and conferences and more than 75 articles published in reputed SCI and Scopus indexed journals, and 14 book chapters, and two books of international standard. Six research scholars have obtained their PhD degree under his guidance, and eight students are pursuing their PhD. His research interests include economic load dispatch, optimal power flow, FACTS, automatic generation control, radial distribution network, power system stabilizer, image processing, machine learning, evolutionary techniques, etc. Email: [email protected]
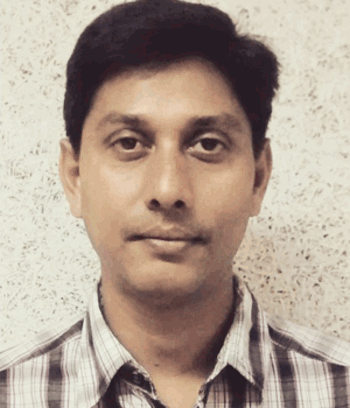
Dinesh Kumar Pradhan
Dinesh Kumar Pradhan is an assistant professor of information technology at Dr B C Roy Engineering College, Durgapur, India. Where he has been a faculty member since 2009. With a PhD in computer science and engineering from NIT Durgapur, he has published extensively and notably in the field of data science and machine intelligence. Outside of professional interests, he travels widely, reads, writes, sails, and believes in happy living. Email: [email protected]