ABSTRACT
Previous research demonstrates links between student social identification, perceived learning norms, learning approaches and academic outcomes and indicates the value of bolstering student social identification in higher education settings. The current study aimed to examine whether the models identified in this previous research replicated in a fully online environment. This is critical knowledge in the context of the industry-wide debate on the gains and losses of online university. Self-report survey data (N = 112), e-learning analytics and grades were used to examine student social identification, perceptions, behaviours, and outcomes over a 4-month period of online learning during the COVID-19 pandemic. Results were unexpected: previous models failed to replicate in our data. Exploratory analysis identified three ways forward: examination of student learning activity outside of institutionally provided online contexts, revisiting the use of SPQ as a measure of learning approach, and examining student social interactions and identification in a social media-rich online environment.
While there is some evidence on the ways social identity processes play out in online higher education (Chu & Chen, Citation2016; Mingfang & Qi, Citation2018), this data suffers from the inevitable self-selection bias that, prior to 2020, students had chosen to enrol in a course being delivered online. The COVID-19 pandemic and associated isolation procedures in Australia presented us with an opportunity to examine these processes in all students – including those who would never choose online education – as higher education suddenly moved fully online. While not a formal experiment with random assignment or a control group, the data presented in the current study represent an opportunity for comparison. The circumstances that surround this data could never ethically be produced experimentally in a real-world educational setting. They provide us with a unique opportunity to examine the experience of real students, in a real educational setting, where elements of choice that could never be withheld for the sake of research were limited by global circumstances. The current paper presents a case study of a small Australian medical school, where all teaching and learning was moved online during the second month of the 2020 academic year. The study uses multiple data sources, including student self-report surveys, analytic data from e-learning resource usage, and academic performance data to track the impacts of social identification on engagement with e-learning resources, learning approaches, academic confidence, academic performance and self-reported wellbeing and life satisfaction.
There are two key corollaries of the fully online approach that are of interest. First, the move to fully online teaching impacts the degree and manner of interaction possible in the classroom. This impacts on the social aspects of learning, including attempts to engage students in collaborative work and constructivist learning tasks. As such, student learning approaches will be affected. Second, fully online learning, particularly during the public health lockdown imposed in Australia during mid-2020, impacts students’ ability to interact with one another. This is particularly the case for crucial informal interactions that might lead to interpersonal bonding or the formation of a medical student social identity and the process of identification with that identity.
The social experience of fully online education
Online delivery approaches, modalities and technologies have developed at incredible speeds. While educators have access to an array of tools, research evidence on these tools has failed to keep pace (Njenga & Fourie, Citation2010). As a result, the rapid shift to online delivery necessitated by the COVID-19 pandemic (Amemado, Citation2020) raised a number of questions about how to maximise student learning, engagement and wellbeing. One of the key questions, and the question with which we grapple here, is about how the move to fully online learning, and the associated loss of casual social interactions with peers, might impact student social identification and its established relationship with perceived learning norms, learning approaches and academic outcomes (e.g., Bliuc et al., Citation2011a; Smyth, Mavor, & Platow, Citation2017; Smyth et al., Citation2019).
Evidence suggests that attending class in person provides social and collaborative interaction that is not truly replicable online (Kemp & Grieve, Citation2014). There is some literature on the ways in which education-related social interactions online might play out. A review of the evidence (Mehall, Citation2020) suggests that interpersonal interaction in online environments can bolster perceived learning, student satisfaction and academic achievement. Evidence from the distance learning context suggests that communication interactions with peers and staff are the key driver of a sense of connection to the university and resulting academic success (Ragusa & Crampton, Citation2018). There was also early evidence (not replicated recently in a post-social media online context) that difficulties in online social interactions can exacerbate loneliness and limit the success of study (McInnerney & Roberts, Citation2004); students may struggle with the nature of online social interactions (Irwin & Berge, Citation2006); and that the ways in which students interact online is qualitatively different to social interactions in face-to-face class (Wang, Citation2005). This is in line with findings from the distance education literature that suggests students undertaking distance education take a personal orientation, rather than a social orientation to their studies (Richardson, Citation2000). There is some more recent evidence that online learning is equivalent to in-person learning in terms of engagement (Li et al., Citation2014) and academic performance (Paul & Jefferson, Citation2019). However, there is also evidence to suggest that students may fail to utilise available online peer-to-peer interactions (Muhisn et al., Citation2019). What we yet do not know, however, is how the quality and frequency of these interactions might impact the development of a student’s social identification and the flow-on effects to perceived norms, learning approaches and academic success.
In order to pursue this question, we build on existing work examining the relationships between social identification with the field of study and: academic outcomes (Bliuc et al., Citation2011a), perceived learning norms (Smyth et al., Citation2015; Smyth, Mavor, Platow, & Grace, Citation2017; Smyth et al., Citation2018), and learning approaches (Bliuc et al., Citation2011b; Smyth, Mavor, & Platow, Citation2017; Smyth et al., Citation2019). This work uses Social Identity Theory (Tajfel & Turner, Citation2004) to understand the ways in which the social context might inform cognitive and behavioural choices in the specific context of higher education. The findings from this precedent work indicate a clear need to prioritise the fostering and maintenance of student social identification and perception of deep learning norms in face-to-face learning, as these are demonstrably linked to more effective approaches to learning and better academic performance. As such, our first research question is: to what extent do the relationships between social identification, perceived norms, learning approaches and student outcomes demonstrated in face-to-face tertiary education replicate in a fully online learning environment?
Learning approaches online
Building on this work, however, presents us with a second set of challenges: there is little agreement on how learning approaches might function in a fully online context. There are two main types of learning approach – deep learning, when a student aims to contextualise new content in the context of an existing broader framework, and surface learning, which focusses on efficiency and learning required information for assessment tasks, such as by rote learning (Biggs, Citation1999). These are typically measured using a self-report scale – often a variant of the Study Process Questionnaire (SPQ) (Biggs et al., Citation2001). There is some evidence from blended learning contexts (e.g., Guy et al., Citation2018) that a deeper learning approach is associated with more frequent accessing of a broader range of online resources. The in-person learning approaches literature, however, rarely uses behavioural measures like resource usage and the evidence for the relationships between scores on learning approach scales and actual learning behaviour is fairly weak (Choy et al., Citation2012). As such, our second research question is: to what extent do existing methods for measuring learning approaches translate to a fully online environment and how do these relate to actual learning behaviours?
The pandemic context
The COVID-19 pandemic in which this research was conducted is novel and bears explication. The pandemic necessitated a range of public health measures to limit the spread of disease. In the Australian response, these took the form of ‘lockdowns’ and the isolation of individuals to their homes. Opportunities for in-person interactions with peers and educators were extremely limited, which we would expect to have impact on the development of new social identities (for commencing students) and may also reduce identification with established identities (for continuing students). In this novel pandemic context, we also needed to account for a range of novel factors, including frequency and vectors of socialisation, employment, and living situation.
We seek to apply existing models of student social experience and learning in this unique setting where medical students were required to engage in online learning from the isolation of their own homes due to campus shutdown. While the involuntary nature of the transition is unique and somewhat unpredictable in this setting, pre-existing evidence on volitional online learning suggests the model could be replicable.
The current study
This study examined student experience, behaviour and outcomes, aiming to address two key questions related to applying Social Identity Theory to higher education:
Do the relationships between social identification, perceived norms and learning approaches demonstrated in in-person tertiary education replicate in an online remote learning environment, and what are the flow-on effects to academic performance and confidence?
Do existing methods for measuring learning approaches translate to a fully online remote learning environment and how do these relate to actual learning behaviours?
Our hypotheses were as follows:
(H1) We anticipate the relationships between identification, perceived norms and learning approaches in online remote learning will be analogous to those demonstrated in traditional in-person higher education (Bliuc et al., Citation2011a, Citation2011b; Smyth et al., Citation2015; Smyth, Mavor, & Platow, Citation2017; Smyth et al., Citation2019), such that identification and perceived norms will each have a direct positive effect on deep learning approaches and will interact to predict learning approaches (see (a)).
Figure 1. Hypothesised relationships between identification, norms, learning approach and outcomes, drawn from Smyth et al. (Citation2019).
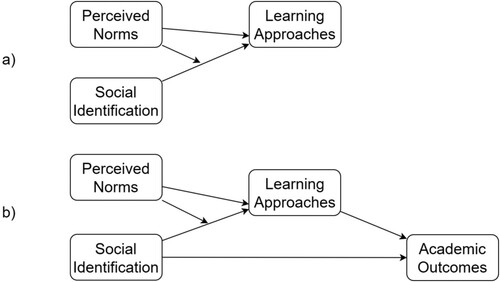
(H2) We anticipate, based on the same literature, that the model in H1 will be extendable in the same manner as Smyth et al. (Citation2019): a moderated-mediation model where greater social identification, deeper learning approaches and stronger deep learning norms will be associated with stronger academic outcomes (graded performance and perceived academic confidence) (see (b)).
(H3) We have no directional expectations for the relationship between learning approaches scores and indicators of actual learning behaviour using e-learning analytics. The evidence for the relationships between SPQ scores and actual learning behaviour is fairly weak (Choy et al., Citation2012), even in the in-person context, so this analysis is largely exploratory. Should self-reported SPQ scores be representative of student actual approaches, we might anticipate a direct, positive relationship. In the case where students have very little insight into their own learning, we might expect no relationship.
Method
Context
Data were collected from students in a four-year graduate-entry medical program at the ANU Medical School. Students (approximately 110 per year cohort) must complete a previous bachelor's degree but there are no specific course prerequisites. In the cohorts under examination here, the majority had a previous science or health-based degree. Prior to the COVID-19 pandemic, the program already included a range of multimedia-rich online learning activities and students were already familiar with the majority of online learning modalities that proceeded to form the core of their studies during 2020. The medical school traditionally relies on in-person small-group teaching in the form of problem-based learning, clinical skills tutorials and medical science practical laboratories. Students are actively encouraged to collaborate with peers and the assessment approach (a ‘fail/course requirement satisfied/higher level pass' model) is explicitly derived to reduce counter-productive competition among students. When COVID-19 containment measures came into effect, students had completed approximately six weeks of the academic year (excluding the orientation week). For our first-year cohort, this meant they were only able to learn and socialise together for a maximum of 30 study days. In contrast, the second-year cohort had undertaken one whole academic year and eight weeks of the new academic year prior to the campus closure. In March 2020, the campus was closed in response to the COVID-19 pandemic and all education activities were rapidly transitioned to fully online remote learning.
Participants
Participants were 112 graduate-entry medical students, enrolled across the two pre-clinical years (NB: 60% of respondents were first-year students) of a medical program. Depending on the participants’ consent patterns, they were tracked across survey responses (N = 112), e-learning analytics (N = 49) and academic grades (N = 49). Mean age was 24.46 (SD = 3.43) years and the gender balance skewed female (M:F:undisclosed = 37:60:15). The majority of respondents were native English speakers (78%), living in a metropolitan area (70%), living with their family or partner (62%) and not working outside of their studies during the lockdown period (58%). Nearly half (48%) were isolating during COVID in the same territory (Australian Capital Territory) as the medical school.
Self-report measures
An online self-report survey comprised the bulk of the data collection. The survey was made available to students for one month at the end of their first online semester after receiving their first-semester assessment grades. The survey measured social identification, perceived social support, learning approach, self-report e-learning approach, learning norms, wellbeing norms, academic confidence, life satisfaction, mental wellbeing and a range of demographic and COVID-situational data. Unless otherwise indicated, items were on a 5-point Strongly Agree/Strongly Disagree Likert scale and scales were constructed by averaging.
Social identification
Student social identification with their peers was measured using 7 items commonly used and adapted from Haslam and colleagues (Citation2004). Items included ‘I have strong ties with other medical students at ANU' and ‘I would RATHER NOT tell other people that I am a medical student at ANU' (reverse-coded). In the current data, this scale was acceptably reliable (α = .81).
Learning approaches
SPQ
Learning approaches were measured using 12 items adapted from the R-SPQ-2F (Biggs et al., Citation2001). This subset of items has been used before with acceptable psychometrics (e.g., Smyth et al., Citation2015). Items included ‘I find I can get by in most assessments by memorising key sections, rather than trying to understand them' (surface) and ‘I find that I am only satisfied when I have done enough work on a topic so that I can form my own conclusions' (deep).
E-learning approaches
As the SPQ items address only a typical in-person learning context and we are examining fully online remote learning, we also generated six items that measured e-learning-specific strategies and practices. These items included: ‘When going through [online] lessons, I tend to skim for things I think will be on the exam'; ‘If a[n online] quiz has a written question, I make a genuine attempt at answering before looking at the model answer'; ‘I only tend to do the compulsory [online] lessons'; and ‘I make sure I keep up with lecture recordings and extra activities on [Learning Management System] as they’re released, rather than leaving them until the last minute'.
Combined learning approaches
In the current research, we are less interested in the effects of the two separate scales (i.e., deep vs surface) than we are in the overall tendency toward one or the other. We were also only interested in deep learning approaches as they applied to online learning. As such, we considered both the SPQ items and the new e-learning items a single scale. Following the approach of Smyth et al. (Citation2018), these 18 items were collated into a single net-tendency measure (surface learning items were reverse-coded and the whole scale was averaged). The new 18-item combined scale was found to be acceptably reliable (α = .75)Footnote1 and was used for further analysis.
Norms
Perceived norms for both learning and wellbeing behaviours were included. Learning norms were measured using the items from Smyth and colleagues (Citation2015). Items included ‘[institution] medical students typically prefer to focus on learning efficiently by memorising key information and minimising study time' (surface) and ‘[institution] medical students typically prefer to look at a variety of different resources when studying up a topic' (deep). In the current data, the surface norms subscale was not reliable (α = .19) and was discarded.Footnote2 The deep norms subscale was acceptably reliable (α = .70) and was retained. This decision is non-problematic, as our learning approach measure is similarly geared toward deep learning.
Academic confidence
As grades can be a poor measure of learning and we are interested in academic outcomes, we also collected data on participants’ self-reported confidence in their mastery of the content. Items were: ‘On the whole I feel I have been able to meet the stated learning objectives in Semester 1 2020'; ‘I feel confident in applying what I learned in Semester 1 2020'; and ‘I feel confident that I understood material covered Semester 1 2020'. This scale was acceptably reliable in our data (α = .84).
Wellbeing
To control for any wellbeing effects that might arise from the novel, stressful pandemic environment, wellbeing was measured with two scales. The first scale was the 5-item Satisfaction With Life Scale (SWLS; Diener et al., Citation1985). This scale was acceptably reliable in the current data (α = .82). The second was a 7-item subset from the Warwick-Edinburgh Mental Wellbeing Scale (WEMWBS; Stewart-Brown & Janmohamed, Citation2008). This subset was acceptably reliable in the current data (α = .86).
E-learning data
Student engagement with e-learning was derived from analytics extracted from the lecture recording platform (Echo 360, Reston, VA). During the campus shutdown, the majority of content delivery across the program occurred via this system. The engagement score (%) downloaded for each consenting student represents the percentage of lecture recording time that each student viewed, across all lecture recordings available during the lockdown period of their first semester (14 weeks). Students were also able to report on external online resources they had used during the isolation period in a free-text item.
Academic outcomes
Academic outcome measures were obtained for first- and second-year students for their first-semester 2020 exam results. Grades were obtained as sub-scores on short-answer question (SAQ) and multiple-choice question (MCQ) grades which cumulatively were weighted to produce an overall grade for the semester (Total). These scores were then standardised (converted to z-scores), using the whole-cohort mean and standard deviation (i.e., from the entire year group, not just those consenting to research), to control for any self-selection bias.
Results
Sample
A final sample of 112 students participated in at least one aspect of the research. Of these, all 112 completed the social identification and learning approaches items. The majority of these (97) also completed the full survey, including norms, demographics, wellbeing, and COVID-19 isolation items. Samples for analytics data and academic performance data were smaller (N = 49 in each group, but these do not perfectly overlap). To ensure consent patterns did not bias our sample, attrition analyses were conducted. Those who provided access to e-learning analytics did not significantly differ from those who didn’t for the majority of key variables – with the exception of higher social identification (t(20.53) = 2.33, p = .02) and greater life satisfaction (t(16.93) = 2.24, p = .03). Those who provided access to academic performance only demonstrated higher social identification (t(28.89) = 2.53, p = .01). These differences necessitate we acknowledge that analyses on the analytics and performance data were only demonstrated in students who were comparatively more socially identified.
Descriptive analysis
Mean scores on key variables were compared across participant attributes, including basic demographics and self-reported circumstances during the period of isolated online learning. Few differences were detected, with one key pattern: first-year students had a substantively different experience to those in later years of the program. First years reported significantly higher mean levels of social identification, deep learning norms, deep learning approaches, greater proportions of lecture recordings accessed and academic confidence (see ). Three other differences emerged across the analyses: those who lived with family or a partner were significantly more academically confident than those who lived with peers or alone (F(2,91) = 3.66, p = .03); those engaging in paid in-person work during the isolation period perceived the learning norms as significantly less deep than those working from home or not at all (F(2,94) = 3.55, p = .03) and those engaging in a greater variety of interaction with others during isolation reported greater life satisfaction (t(80.85) = 2.21, p = .03).
Table 1. Means and comparisons of key variables across participant categories.
Zero-order correlations among key variables were also considered and broadly followed the patterns in the literature (). Of note: social identification was positively related to deep learning norms, deep learning approaches, life satisfaction, wellbeing and confidence. Further, proportion of lecture recordings accessed was not associated with any of the identity or learning approach variables but was significantly related to scores on SAQ items and overall exam score. While the sample for the analysis addressing lecture recordings was smaller than the total sample (n = 49), owing to consent patterns, a post-hoc power analysis in GPower indicates the two-sided correlations conducted using this data are sufficiently powered (1 − β = 0.99).
Table 2. Zero-order correlations among key variables.
Replicating findings from the literature
Our first model replicated the simple moderation pattern in the literature (Smyth et al., Citation2015), predicting learning approach from social identification, perceived norms and their interaction. We only found that perceived norms have a direct effect on learning approach (B = 0.17, p = .03). No effect for identification nor the interaction was detected. Full-model results are in . Again, to ensure our opportunistic sample for these analyses meet the threshold for meaningful analysis, a post-hoc power analysis was conducted using GPower using the full-model effect size (f2 = .12; 1 − β = 0.81).
Table 3. Simple moderation model (PROCESS Model 1, N = 102).
Our second model replicated the moderated-mediation model in Smyth and colleagues (Citation2019), where this simple moderation model is extended to academic performance. In our data, this was run four times, with the three available exam scores (MCQ z-score, SAQ z-score and total z-score), as well as academic confidence. These models are not a good fit for the data, with no demonstrated link between identification, learning approach and academic performance in this sample. Results from the confidence analysis data indicate only a significant positive relationship between deep learning approach and confidence (B = 0.80, p < .001). Full-model results are in and . Owing to the bootstrapping approach to indirect effects taken by the PROCESS macros (Hayes, Citation2017) used in the analysis, further Monte Carlo simulation-based power analysis was not conducted.
Table 4. Moderated-mediation model (PROCESS Model 7, N = 48).
Table 5. Moderated-mediation model (PROCESS Model 7, N = 100).
Exploratory analyses
We also conducted some exploratory analysis to get a fuller picture of the ways students were learning. The primary e-learning analytics available to the project were data on frequency and duration of access to the lecture recordings. During the period under investigation, the degree was primarily being delivered via pre-recorded lectures (live online lectures were added to the curriculum after the study had concluded) and, as such, these data represent a good indicator of student engagement with the taught curriculum. Correlations between the two available indices (frequency and proportion of available minutes) and our variables of interest indicate a relationship between proportion of recordings accessed and both total academic performance (r = .31, p = .04) and the SAQ sub-score (r = .31, p = .04), but not any of the social, learning or wellbeing items. No relationship was detected between access frequency and any variables of interest.
External online resources students reported using were categorised into four types: flashcard or quiz based (1), video (2), databases or textbooks (3) and student-generated resources (4). For each student, a count of each type of resource was calculated. Rank-based correlations indicate two things of interest:
The use of more student-generated (including peer-generated) resources was positively associated with social identification (rho = 0.30, p = .001), life satisfaction (rho = 0.21, p = .04) and academic confidence (rho = 0.31, p = .002).
The use of more video resources was associated with perceptions of deep learning norms (rho = 0.22, p = .03)
Discussion
The present study had two key aims. The first was to examine how well models of the established relationships between social identification, student norms, and learning approaches translate to a fully online environment. The second was to examine the online learning environment more closely, including behavioural measures of e-learning resource usage and how these fit in the models. These aims were pursued with a medical student population during the COVID-19 pandemic.
Hypothesised analyses
The key message from the analysis of the simple moderation model was that it was a poor fit. Our data demonstrate no association between social identification and any of the grade or confidence variables, in disagreement with Hypotheses 1 and 2. Only deep learning approach was found to be related to perceived confidence. These findings, at odds with the precedent literature (e.g., Bliuc et al., Citation2011b; Smyth, Mavor, & Platow, Citation2017; Smyth et al., Citation2019), warrant some consideration. There are three possible explanations for this. First, these models have never been tested in a fully online environment and it is possible that peer-to-peer interactions and social identification with fellow students are less important in a learning environment that doesn’t necessitate in-person contact or at least co-location with those peers. It may be that, in a fully online learning context, one’s fellow students are no longer the relevant social identity and that the student social experience of learning is anchored elsewhere. This is an interesting possibility and one that warrants significant further study in a world where online learning is increasingly the norm. Second, and perhaps most obviously, the novel social and educational environment engendered by the COVID pandemic could be contributing to the lack of effects we encountered (Aristovnik et al., Citation2020). Students may be feeling, behaving, or perceiving others behaving differently during the pandemic which may affect our proposed model. Due to the unprecedented context, it is difficult to guess how students may have been affected, socially. Third, grades are an established poor measure of university student learning (e.g., Schwab et al., Citation2018) and this is particularly the case in the first semester of postgraduate medical school, where much of the content is not new to those with prior qualifications in medical science. Our measure of grades is further complicated by timing: respondents were aware of their grades at the time of survey completion and this may have coloured their perceptions. However, the lack of significant association between academic confidence and grades, or between social identification and grades, would suggest that the effect on perceptions of a student’s own competence (and any related knock-on effects to identification) is limited.
Exploratory analyses
We also analysed student engagement with online material and external resources. We find no association between self-reported learning approaches and the patterns of usage of the online resources. This raised a couple of tantalising possibilities – only one of which we could examine. The first possibility was that the e-learning analytics available to us (on the institutionally provided resources) was only part of the story, in terms of the online resources and learning activities accessed by students taking a deep learning approach. To examine this, we analysed student self-directed external (non-institutional) learning resource usage. Use of a greater number of student-generated resources was associated with increased social identification and academic confidence. In this cross-sectional data, it is not possible to comment on whether more highly identified students engage more with student-generated resources or if engagement with these resources might boost identification, but we would anticipate this effect likely exists as a feedback loop. In either case, this finding is suggestive of a need to examine use of external resources in trying to map highly identified student learning behaviour.
The second possibility is that the self-report learning approach scale was not a good reflection of actual learning behaviours online. This is an issue that plagues learning approach scales used in face-to-face contexts as well (Choy et al., Citation2012) and is currently irrefutable. It may reflect the possibility that responses on self-report learning approach scales are more reflective of identification in the first place (i.e., responses are an impression-management act. The more highly identified student is more likely to report learning behaviours that reflect a greater degree of care and engagement).
Unexpected findings
One unexpected finding warrants further discussion. A pattern was identified in the demographic correlation data where the first-year students reported significantly higher mean levels of social identification, learning norms, learning approaches, proportions of lecture recordings accessed and academic confidence than their later-year counterparts. Where we might expect that the students who had had less time to interact and spent most of their studies isolated might have poorer social connections, less clear norms, and less engagement with their study program, we find the opposite. This expectation, however, is built on an assumption that students act as passive receptors of the environments, materials and social milieus provided to them and do not account for the active role they might take in building community and collaborating on their own learning. As medical schools aim to select highly motivated students (Wouters et al., Citation2017), this population may be further driven to engage with the limited interaction afforded to them from their course and classmates, benefitting students through deeper learning and improved confidence.
These findings, and those from the e-learning analytics, hint at what is possibly the key challenge in mapping the online learning environment: much of the actual interaction occurs outside of the institutionally provided online context. As our research team includes medical students, we are uniquely positioned to be factually aware that our data collection was unable to tap the vast student interaction, resource exchange and socialisation that occurs via study-linked (but privately managed) social media. Future studies may qualitatively approach this issue to explore the ‘behind the scenes' social media interactions as part of the student experience which are otherwise difficult to isolate.
Limitations and future directions
There are some key limitations that must be borne in mind in interpreting our findings. We are principally limited by the unprecedented pandemic context and our cross-sectional methods of measurement. Through qualitative analysis and further longitudinal follow-up, these limitations may be reconciled. The COVID-19 pandemic is both novel and far-reaching and has likely affected students and their learning in a multitude of ways, which we could not have anticipated at the time of data collection. There is an emerging body of research on the student learning experience during the pandemic, hinting at some key factors to consider in future research. For example, the lectures in our study were delivered asynchronously, which may benefit students through convenience but could alternatively be associated with a reduction in students’ academic motivation to work through the content (Nguyen et al., Citation2021). The asynchronous online environment lends itself to students who are more self-directed and resilient (Wallace et al., Citation2021), being able to develop study techniques on their own or organise their own online study groups. Additionally, student- or educator-level technological challenges may also impact students’ perceptions of and confidence in e-learning, impacting how they engage and learn from the material (Wallace et al., Citation2021). These factors, in addition to our proposition that online peer-to-peer interactions are complex and occur outside the institutional online environments (i.e., on social media), mean that collection of interview data for qualitative analysis would be valuable to explore the reasons why our model was unable to predict student learning approaches or outcomes.
The second significant limitation of our study was the cross-sectional nature of our survey and lack of initial baseline measures. One implication of this is we can neither generally study the long-term changes in identification and learning processes, nor can we specifically follow the longer-term impacts of COVID-19 on this cohort. There are limited longitudinal data on variation over time in identification and learning approaches or the link between them in both the traditional and online learning environments. For example, Platow et al. (Citation2013) established the relationship between learning approach and social identification over time and the importance of deep learning approach for academic outcomes and continuing engagement. Additionally, Piumatti and colleagues (Citation2021) showed that medical students may replace an initial deep approach with more surface learning throughout their degree. Future research should aim to fill this gap, investigating social and learning factors while also gaining COVID-specific data surrounding the pandemic and shift to e-learning.
Conclusions
The results of this study have implications for future educators when planning online curricula or transitioning to online learning due to external forces. We were unable to replicate the moderation or moderated-mediation models which were previously demonstrated in traditional face-to-face higher education. This raises questions about the factors which led to this finding – including the roles of pandemic-caused social isolation and student engagement with e-learning. Identifying key drivers of the lack of effects would be a critical addition to the literature. Finally, students appeared to remain resilient and resourceful in the face of the pandemic to build a social network online of their own accord, an unexpected feature which may play an important role in relationships between social identification and learning for future settings.
Data-availability system
Due to the potential risk for re-identification due to the small pool of students (total N = 200) from which we draw our data, data are not publically available, but are available from the corresponding author on reasonable request.
Ethics
Ethical components of the research were approved by the ANU Human Research Ethics Committee (protocol 2020/224).
Disclosure statement
No potential conflict of interest was reported by the author(s).
Notes
1 NB: reliability does not indicate unidimensionality and future research in larger samples would benefit from PCA analysis of this combined measure to determine what, exactly, is being measured.
2 This reliability issue warrants discussion, as this scale has been validated. As the sub-scale is only three items, it is easily destabilised by one rogue item and, in our data, intercorrelations indicated that the item “[institution] medical students typically prefer to cram a lot of information before exams” was the cause of this problem. This item was negatively correlated with the other two items, likely indicating that, regardless of the valence of the norm (toward deep or surface learning), students in this particular context were engaging in “cramming” behaviour. This is not unusual in medical school contexts.
References
- Amemado, D. (2020). COVID-19: An unexpected and unusual driver to online education. International Higher Education, (102), 12–14. https://doi.org/10.36197/IHE.2020.102.06
- Aristovnik, A., Keržič, D., Ravšelj, D., Tomaževič, N., & Umek, L. (2020). Impacts of the COVID-19 pandemic on life of higher education students: A global perspective. Sustainability, 12(20), 8438. https://doi.org/10.3390/su12208438
- Biggs, J. (1999). What the student does: Teaching for enhanced learning. Higher Education Research & Development, 18(1), 57–75. https://doi.org/10.1080/0729436990180105
- Biggs, J., Kember, D., & Leung, D. Y. (2001). The revised two-factor study process questionnaire: R-SPQ-2F. British Journal of Educational Psychology, 71(Pt 1), 133–149. https://doi.org/10.1348/000709901158433
- Bliuc, A. M., Ellis, R. A., Goodyear, P., & Hendres, D. M. (2011a). The role of social identification as university student in learning: Relationships between students’ social identity, approaches to learning, and academic achievement. Educational Psychology, 31(5), 559–574. https://doi.org/10.1080/01443410.2011.585948
- Bliuc, A. M., Ellis, R. A., Goodyear, P., & Hendres, D. M. (2011b). Understanding student learning in context: Relationships between university students’ social identity, approaches to learning, and academic performance. European Journal of Psychology of Education, 26(3), 417–433. https://doi.org/10.1007/s10212-011-0065-6
- Choy, J. L. F., O’Grady, G., & Rotgans, J. I. (2012). Is the study process questionnaire (SPQ) a good predictor of academic achievement? Examining the mediating role of achievement-related classroom behaviours. Instructional Science, 40(1), 159–172. https://doi.org/10.1007/s11251-011-9171-8
- Chu, T.-H., & Chen, Y.-Y. (2016). With good we become good: Understanding e-learning adoption by theory of planned behavior and group influences. Computers & Education, 92–93, 37–52. https://doi.org/10.1016/j.compedu.2015.09.013
- Diener, E., Emmons, R. A., Larsen, R. J., & Griffin, S. (1985). The satisfaction with life scale. Journal of Personality Assessment, 49(1), 71–75. https://doi.org/10.1207/s15327752jpa4901_13
- Guy, R., Byrne, B., & Dobos, M. (2018). Optional anatomy and physiology e-learning resources: Student access, learning approaches, and academic outcomes. Advances in Physiology Education, 42(1), 43–49. https://doi.org/10.1152/advan.00007.2017
- Haslam, S. A. (2004). Psychology in organizations: The social identity approach (2nd ed.). Sage.
- Hayes, A. F. (2017). Introduction to mediation, moderation, and conditional process analysis: A regression-based approach. Guilford Publications.
- Irwin, C., & Berge, Z. (2006). Socialization in the online classroom. E-Journal of Instructional Science and Technology, 9(1), n1. ISSN 1324-0781.
- Kemp, N., & Grieve, R. (2014). Face-to-face or face-to-screen? Undergraduates’ opinions and test performance in classroom vs. Online learning. Frontiers in Psychology, 5, 1278. https://doi.org/10.3389/fpsyg.2014.01278
- Li, F., Qi, J., Wang, G., & Wang, X. (2014). Traditional classroom vs e-learning in higher education: Difference between students’ behavioral engagement. International Journal of Emerging Technologies in Learning (iJET), 9(2), 48–51. https://doi.org/10.3991/ijet.v9i2.3268
- McInnerney, J. M., & Roberts, T. S. (2004). Online learning: Social interaction and the creation of a sense of community. Journal of Educational Technology & Society, 7(3), 73–81. ISSN 11763647.
- Mehall, S. (2020). Purposeful interpersonal interaction in online learning: What is it and how is it measured? Online Learning, 24(1), 182–204. https://doi.org/10.24059/olj.v24i1.2002
- Mingfang, Z., & Qi, W. (2018). Empirical research on relationship between college students’ social identity and online learning performance: A case study of Guangdong province. Higher Education Studies, 8(2), 97–106. https://doi.org/10.5539/hes.v8n2p97
- Muhisn, Z., Ahmad, M., Omar, M., & Muhisn, S. (2019). The impact of socialization on collaborative learning method in e-learning management system (eLMS). International Journal of Emerging Technologies in Learning (iJET), 14(20), 137–148. https://doi.org/10.3991/ijet.v14i20.10992
- Nguyen, T., Netto, C. L. M., Wilkins, J. F., Bröker, P., Vargas, E. E., Sealfon, C. D., Puthipiroj, P., Li, K. S., Bowler, J. E., Hinson, H. R., Pujar, M., & Stein, G. M. (2021). Insights into students’ experiences and perceptions of remote learning methods: From the COVID-19 pandemic to best practice for the future. Frontiers in Education, 6, 91. https://doi.org/10.3389/feduc.2021.647986
- Njenga, J. K., & Fourie, L. C. H. (2010). The myths about e-learning in higher education. British Journal of Educational Technology, 41(2), 199–212. doi:https://doi.org/10.1111/j.1467-8535.2008.00910.x
- Paul, J., & Jefferson, F. (2019). A comparative analysis of student performance in an online vs. face-to-face environmental science course from 2009 to 2016. Frontiers in Computer Science, 1, 7. https://doi.org/10.3389/fcomp.2019.00007
- Piumatti, G., Abbiati, M., Gerbase, M. W., & Baroffio, A. (2021). Patterns of change in approaches to learning and their impact on academic performance among medical students: Longitudinal analysis. Teaching and Learning in Medicine, 33(2), 173–183. https://doi.org/10.1080/10401334.2020.1814295
- Platow, M. J., Mavor, K. I., & Grace, D. M. (2013). On the role of discipline-related self-concept in deep and surface approaches to learning among university students. Instructional Science, 41(2), 271–285. https://doi.org/10.1007/s11251-012-9227-4
- Ragusa, A. T., & Crampton, A. (2018). Sense of connection, identity and academic success in distance education: Sociologically exploring online learning environments. Rural Society, 27(2), 125–142. https://doi.org/10.1080/10371656.2018.1472914
- Richardson, J. T. (2000). Researching student learning: Approaches to studying in campus-based and distance education. Open University Press.
- Schwab, K., Moseley, B., & Dustin, D. (2018). Grading grades as a measure of student learning. SCHOLE: A Journal of Leisure Studies and Recreation Education, 33(2), 87–95. https://doi.org/10.1080/1937156X.2018.1513276
- Smyth, L., Chandra, V., & Mavor, K. I. (2018). Social identification and normative conflict: When student and educator learning norms collide. Journal of Applied Social Psychology, 0(0), https://doi.org/10.1111/jasp.12505
- Smyth, L., Mavor, K. I., & Platow, M. J. (2017). Learning behaviour and learning outcomes: The roles for social influence and field of study. Social Psychology of Education, 20(1), 69–95. https://doi.org/10.1007/s11218-016-9365-7
- Smyth, L., Mavor, K. I., & Platow, M. J. (2019). Social identification and academic performance: Integrating two existing models of tertiary student learning. Educational Psychology: An International Journal of Experimental Educational Psychology, 39(3), 409–425. https://doi.org/10.1080/01443410.2018.1524853
- Smyth, L., Mavor, K. I., Platow, M. J., & Grace, D. M. (2017). Understanding social identity in education: The modifying role for perceived norms. In K. I. Mavor, M. J. Platow, & B. Bizumic (Eds.), Self and social identity in educational contexts (pp. 212–223). Routledge.
- Smyth, L., Mavor, K. I., Platow, M. J., Grace, D. M., & Reynolds, K. J. (2015). Discipline social identification, study norms and learning approach in university students. Educational Psychology, 35(1), 53–72. https://doi.org/10.1080/01443410.2013.822962
- Stewart-Brown, S., & Janmohamed, K. (2008). Warwick-Edinburgh mental well-being scale. User Guide Version, 1.
- Tajfel, H., & Turner, J. C. (2004). The social identity theory of intergroup behavior. In J. T. Jost & J. Sidanius (Eds.), Political psychology (pp. 276–293). Psychology Press. reprinted chapter originally appeared in (Psychology of Intergroup Relations [ed. by S.Worchel; WG Austin], 1986, 7–24.
- Wallace, S., Schuler, M. S., Kaulback, M., Hunt, K., & Baker, M. (2021). Nursing student experiences of remote learning during the COVID-19 pandemic. Paper presented at the Nursing Forum.
- Wang, H. (2005). A qualitative exploration of the social interaction in an online learning community. International Journal of Technology in Teaching and Learning, 1(2), 79–88. ISSN 1551-2576.
- Wouters, A., Croiset, G., Schripsema, N. R., Cohen-Schotanus, J., Spaai, G. W. G., Hulsman, R. L., & Kusurkar, R. A. (2017). A multi-site study on medical school selection, performance, motivation and engagement. Advances in Health Sciences Education, 22(2), 447–462. https://doi.org/10.1007/s10459-016-9745-y