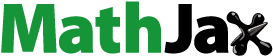
Abstract
In this article, we derive expressions for conditional expectations in terms of regular expectations without conditioning but involving some weights. For this purpose, we apply two approaches: the conditional density method and the Malliavin method. We use these expressions for the numerical estimation of the price of American options and their deltas in a Lévy and jump-diffusion setting. Several examples of applications to financial and energy markets are given including numerical examples.
1. Introduction
Popular models for the fluctuation of the underlying process in finance and energy markets are determined by Brownian motions and are therefore continuous in the sense that they do not generate jumps in the sample paths. Their popularity follows from the fact that they are easy to handle. However, as it turns out from observed data, the absence of jumps is not realistic. Therefore, the use and study of discontinuous models have seen an important boost in the last decades.
In this article, we consider the problem of computing conditional expectations of functionals of Lévy processes and jump diffusions which are involved in pricing and hedging procedures as, e.g. of American options, and which usually require numerical evaluation techniques. In general, it is not possible to obtain analytical expressions for conditional expectations and thus numerical methods are called for. Fournié et al. [Citation1] derive expressions for the conditional expectations in terms of regular expectations for models which have a density and for diffusion models using, respectively, the density method and the Malliavin calculus. In this article, we generalize these two approaches to obtain representations for conditional expectations and their derivatives (with respect to the underlying) in a jump-diffusion setting. The representations we derive are expressed in terms of regular expectations without conditioning but involving a Heaviside step function and some weights. We apply the developed theory to the numerical estimation of American option prices and their deltas.
There is a wide literature on the use of Malliavin calculus in finance, see, e.g. Montero and Kohatsu-Higa [Citation2]. For an overview, we refer to Nualart [Citation3] for continuous processes and Di Nunnio et al. [Citation4] for jump processes. As for the numerical computation of American options, several approaches appeared in this field. von Sydow et al. [Citation5] compared some approaches grouped as Monte Carlo methods, Fourier methods, finite difference methods, and radial basis function methods but only for continuous underlying processes when pricing American options. Broadie and Glasserman [Citation6] built up a tree in order to obtain a discretisation of the underlying diffusion on a grid (see also Barranquand and Martineau [Citation7]). Longstaff and Schwartz [Citation8] use a regression method on a truncated basis of L2 and then choose a basis of polynomials for the numerical estimation of conditional expectations. Shu et al. [Citation9] and Kovalov et al. [Citation10] use a partial differential equations approach. Zhang and Oosterlee [Citation11] use a Fourier method based on Fourier cosine-series expansions. We follow an approach similar to the one by Mrad et al. [Citation12] and Bally and Pagès [Citation13] based on a Monte Carlo method.
Considering a random variable F, a scalar random variable G, and a function f on , Fournié et al. [Citation1] provide the following representation for the conditional expectation
where π is a random variable called weight and H is the Heaviside step function increased with some constant,
. The authors use two approaches: the density method and the Malliavin method (MM). The density method requires that the couple (F, G) has a density p(x, y),
such that its log is C1 in the second argument. In the Malliavin approach, they use a Malliavin derivative of the Wiener process and provide expressions for conditional expectations, where F and G are modeled by continuous diffusions. One of the goals in the present paper is to relax the conditions imposed on the random variables F and G and in particular to allow for random variables which do not necessarily have a known density and which might originate from processes with jumps.
We recall that the density method introduced in [Citation1] requires the knowledge of the density of (F, G). However when F and G are random variables generated from jump processes, the density of the couple (F, G) is in general not known or very hard to compute. To overcome this shortcoming, we use the conditional density method introduced by Benth et al. [Citation14]. For example, in the case of a geometric Lévy process, we only need the knowledge of the joint density of the continuous parts, which we do know. Thus, to apply the conditional density method a separability assumption on the random variables F and G will be required. F and G should consist of a part with known density. The density of the other part is not necessarily known.
For the Malliavin method, we work with the Malliavin derivative for jump processes developed by Petrou [Citation15]. The idea is to use the Malliavin derivative in the direction of the Wiener term in the jump-diffusion process. Using this approach, there is no separability assumption imposed, since the Malliavin calculus as presented in [Citation15] does not require any, as opposed to the Malliavin calculus used in Davis and Johansson [Citation16] or in Benth et al. [Citation17]. We use this approach to compute conditional expectations in our setting as was developed in [Citation1] in a continuous set up.
Furthermore, we provide expressions for the derivative of conditional expectations using both approaches and we illustrate our results with several examples of models which are commonly used in financial and energy markets. Notice that we present our study in the one-dimensional case for the ease of notation, although all results can be extended to a setting in higher dimensions.
The representations that we develop are interesting from a probabilistic point of view. Indeed we derive expressions for the conditional expectations of functionals of random variables involving only unconditional expectations of these functionals. Moreover, these representations are interesting from a numerical point of view. In this article, we apply them to the numerical estimation of American option prices and their deltas, the delta being the sensitivity of the option price with respect to the state of the underlying asset.
To perform the numerical experiments, American options are approximated, through a time discretisation, by Bermudan options as in Bally and Pagès [Citation13] or Abbas-Turki and Lapeyre [Citation18]. We make use of a localization technique and a control variate to minimize the variance, see, e.g. Glasserman [Citation19]. To reduce the memory capacity of the algorithm for the estimation of the American option price and the delta, we suggest to simulate the underlying stock price process backwards in time. This backward simulation technique turns out to be a specific application of Lévy bridges, see Baldeaux [Citation20].
To check the accuracy of the proposed algorithms, we first compute European option prices and their deltas at time t > 0 where we assume a Merton model for the price process. We compare the values obtained by our algorithm to the analytical solutions proposed by Merton [Citation21]. Then considering the same model we estimate the prices and the deltas of American options, which we in turn compare to estimates found in the literature. In addition we compute for the prices a confidence interval according to Bouchard and Warin [Citation22].
In their article [Citation22], Bouchard and Warin performed a numerical comparison and discussed the efficiency and the level of complexity of the regression based Longstaff and Schwartz [Citation8] algorithm and the Malliavin approach algorithm in the continuous setting. In our numerical examples, we investigate how the CDM and the MM perform in the discontinuous setting. The fundamental difference between the Longstaff–Schwartz approach and the (conditional) density or Malliavin approach is the way the conditional expectations are approximated. However, the Longstaff–Schwartz method may show rank deficiency for a certain choice of the parameters of the model (see Mostovyi [Citation23]) and is unable to provide an ad hoc method for the computation of the delta. It has to be combined with other methods such as the likelihood ratio method or pathwise sensitivities based approaches in order to obtain an approximation of the delta. The approaches presented in this article allow the computation of the price of the American option for any parameters choice and lead to representation formulas for the derivative of conditional expectations and consequently provide an estimation of the delta using its own specific method.
The article is organized as follows. In Sections 2 and 3, we develop a representation for conditional expectations via the conditional density method and the MM, respectively. In Section 4, we present variance reduction techniques to obtain acceptable convergence results in numerical applications. In Section 5, we present numerical examples to illustrate our results. Section 6 concludes the article.
2. Conditional expectation via the conditional density method
Let be a complete probability space equipped with a filtration
for time horizon T > 0, satisfying the usual conditions (see Protter [Citation24]). We introduce the generic notation
, for a Lévy process on the given probability space. We set
by convention and work with the right-continuous with left limits version of the Lévy process. Let
indicate the jump of the Lévy process L at time t. Denote the Lévy measure of L by
. Recall that
is a σ-finite Borel measure on
.
In this article, we express the realization of a conditional expectation in terms of regular expectations. Here f is a Borel measurable function (think for instance of the payoff function of a call option), S is an
-adapted price process which may have jumps, and α is a real number. We also rewrite its differential w.r.t. α, i.e. the delta, by only using unconditional expectations.
2.1. Representation results
First, we state a general result for the conditional expectation , where F and G are two random variables satisfying the following separability assumptions.
Assumptions 2.1.
(Separability). Let F and G be two random variables such that
(2.1)
(2.1)
The couple (X, U) is independent of (Y, V). Moreover
(X, U) has a density
with respect to the Lebesgue measure,
is differentiable, for all
, and
, i.e.
.
The functions g1 and g2 are Borel measurable and there exist a Borel measurable function and a strictly increasing differentiable function h such that
(2.2)
(2.2)
for all
.
We apply the conditional density method (CDM) as it is developed in Benth et al. [Citation14]. The CDM method is based on the density method (DM) introduced in Glasserman [Citation19] and used in Fournié et al. [Citation1], but allows for more general dynamics than the latter. Indeed, given F and G as in (2.1), the CDM does not require the knowledge of the density of the couple (F, G) as it is the case in the DM but only the knowledge of the density (X, U). The density of (X, U) plays the most important role in this method. The results follow from straightforward computations based on properties of (conditional) expectations.
We denote the Heaviside step function increased by an arbitrary number by
(2.3)
(2.3)
and its distributional derivative, the Dirac delta function by δ0.
In the upcoming theorem, we present the first representation result of this paper. In order to make the proof comprehensible, we follow a formal approach. The formal proof follows similar derivations as in [Citation1, Section 4.1] by Fournié et al. The main difference is that we apply the CDM instead of the DM by conditioning on . A rigorous proof is included in Appendix A.
Theorem 2.2.
Let F and G be as described in Assumptions 2.1 and let f be a Borel measurable function such that . Then it holds for any
that
(2.4)
(2.4)
where
Proof.
Formally, for the conditional expectation, we know that
(2.5)
(2.5)
Moreover we have that
where
is the σ-algebra generated by Y and V. From Assumptions 2.1(1) we derive
For a function with a single root, the composition rule for the Dirac delta function (see Raju [Citation25]) states that
where u1 is such that
and
. Because of relation (2.2), we apply this composition rule to the function
, with root
, and obtain that
The Dirac delta function is the distributional derivative of the Heaviside step function. Hence by integration by parts, we find that
Finally we conclude that
Applying the latter result with for the denominator of (2.5) we prove the statement. □
Note that the weights in the representation (2.4) are not unique and one can derive infinitely many of them. This was discussed in the result (4.25)–(4.26) in [Citation1] for random variables admitting a density. In Proposition 2.1 of this latter article, the authors discussed also the optimality of the weights in a minimal variance setting. The extension of these results to our jump-diffusion setting using the CDM is straightforward and follows similar derivations as in the proof of Theorem 2.2. For more details we refer to Daveloose [Citation26].
Figure 2. Estimates for the Bermudan put option price obtained through the CDM and MM representations with control variate and with localization technique, against the number of simulated paths. In the right graph the vertical axis is restricted to .
![Figure 2. Estimates for the Bermudan put option price obtained through the CDM and MM representations with control variate and with localization technique, against the number of simulated paths. In the right graph the vertical axis is restricted to [9.5,12].](/cms/asset/d949cb18-b6c5-4623-be3a-a06ab9419b00/lsaa_a_1561306_f0002_c.jpg)
In the next theorem, we deduce a representation for the sensitivity of (2.4) with respect to α.
Theorem 2.3.
Let F and G be as described in Assumptions 2.1, where possesses a second order derivative in its second argument and let the Borel measurable function f guarantee
. Then it holds for any
that
where
Proof.
From Theorem 2.2, it follows immediately that
For the derivatives in the right hand side, it holds by the square integrability assumption for f(F) that
Along the lines of the formal proof of Theorem 2.2, we derive
which concludes the proof.
□
2.2. Examples: Jump-diffusion models
In many applications in mathematical finance, one can make grateful use of Theorem 2.2. In fact, we are able to express a realization of the conditional expectation of the form in terms of regular expectations. Here, f is a function e.g. a payoff function,
represents a Markovian stock price process,
, and α is a real number. We will use Monte Carlo simulations to evaluate these conditional expectations using their representations as regular expectations. In the sequel, we consider different jump-diffusion models for the stock price process to illustrate our results with specific examples.
Remark 2.4.
(Processes with independent and identically distributed increments). In case it holds that the stock price process S is modeled in terms of a process with increments which are independent and identically distributed we remark the following about a conditional expectation of the form . Consider for instance
where L denotes a Lévy process. Then, given that
, it holds, due to the independence and the identical distribution of the increments, that for any
Therefore
and the conditional expectation equals a regular expectations which can easily be estimated via a Monte Carlo method. Although it is possible to use Monte Carlo simulations exploiting the independence and stationarity property of the increments, the application of the obtained representations avoids nested simulations in the pricing of American options, as explained further on in Remark 5.1. Hence, this allows to obtain estimations based on a smaller number of simulated paths.
2.2.1. Jump-diffusion model
The next proposition considers a representation of conditional expectations in terms of unconditional expectations, when the price process S is an exponential jump-diffusion process. This type of stock price model is common in finance and conditional expectations appear when determining (American) option prices (which we will illustrate in Section 5 with numerical experiments).
Proposition 2.5.
Consider a price process S defined by , where L is a Lévy jump diffusion process with decomposition
. Here W is a standard Brownian motion,
is a compound Poisson process independent of W, and μ and β are constant parameters. Then, for any Borel measurable function f fulfilling
, any strictly positive number α, and
, it holds that
where
Proof.
Following the notation of Theorem 2.2, we set F = St and G = Ss. The random variables and
and the functions
, and
, with
, satisfy Assumptions 2.1. The random variables X and U (resulting from a scaled Brownian motion) have a joint normal distribution with density function
To determine the weight in (2.4) we calculate
such that we obtain
□
Example:
Merton model: In the light of the numerical experiments that we perform in Section 5, we derive the representation (2.4) for the following Merton model given by
(2.6)
(2.6)
where r > 0 is the risk-free interest rate, β is a positive constant, and W is a Wiener process. The jump part is determined by a Poisson process N with jump intensity μ and the random variables Yi are i.i.d. with distribution
.
Since the Merton model is a special case of the exponential Lévy jump-diffusion model, the representations obtained via the conditional density method, i.e. through Proposition 2.5 and Theorem 2.3, are as follows, for and
,
(2.7)
(2.7)
(2.8)
(2.8)
where
2.2.2. Additive model
Now we observe a model which is often used to price energy products (see for example Benth et al. [Citation27]). The price process is given by an additive model
(2.9)
(2.9)
The process Y is adapted to the filtration and does not have to be specified here. The process X is a so called
-Ornstein-Uhlenbeck process, see Section 17 in Sato [Citation28]. Namely, it is a process following the dynamics
(2.10)
(2.10)
where
and L is a subordinator, admitting a stationary distribution for the process X which is here
. Hence, this means that Xt has a
-distribution for all t > 0. The solution of the stochastic differential equation (2.10) equals
An interesting property of this type of non-Gaussian OU-processes is the fact that the autocorrelation is independent of the stationary distribution, see, e.g. Barndorff–Nielsen and Shephard [Citation29] or Sato [Citation28], it equals
(2.11)
(2.11)
Proposition 2.6.
Let us observe the additive model described by (2.9) and (2.10). Then it holds for any Borel measurable function f satisfying , and
, that
where
Herein, is the modified Bessel function of the first kind with index a,
Proof.
As in Theorem 2.2, we put , and
to satisfy Assumptions 2.1. To obtain the weight we need the density function of the vector (Xt, Xs). Since X is a
-OU process we know that Xt and Xs are both
distributed and by (2.11) we know that
. According to Brewer et al. [Citation30], the density function of this bivariate gamma distribution with non-zero correlation equals
where is the modified Bessel function of the first kind with index a. We compute
For the function , it holds that
and
. Using the recurrence formulas for modified Bessel functions (see Bowman [Citation31]), we get
According to (2.4) we conclude the statement. □
3. Conditional expectation via MM
Fournié et al. [Citation1] used Malliavin calculus defined for functions on the Wiener space to obtain representations for the conditional expectations. Therefore, their approach is applied to continuous diffusions. In this section, we extend their method to allow for the computation of conditional expectations in a Lévy and a jump-diffusion framework. For this purpose we use a Malliavin derivative of the combination of Gaussian and pure jump Lévy noises, see, e.g. Di Nunno et al. [Citation4] and Solé et al. [Citation32]. In our setting, we use the Malliavin derivative developed by Petrou [Citation15].
Notice that the MM allows for more general dynamics than the conditional density method (CDM). Indeed, no density is required to be known when using the MM. However, the CDM is easier to apply and follows using basic knowledge in probability theory.
Let be a complete probability space in which Lévy processes are well-defined. The Malliavin derivative in the Brownian direction is defined by Petrou [Citation15] in a subspace of
and is essentially a derivative with respect to the Brownian part of a Lévy process L. We denote it by
. Its dual, the Skorohod integral is also defined in [Citation15] and denoted by
. In this section we make use of some notations, definitions, computational rules and properties which are summarized in Appendix B. Recall the function H defined in (2.3).
3.1. Representation results
The ideas and concepts of the proofs of the results presented in this section are based on the proofs of Theorem 4.1 and Corollary 4.1 in Fournié et al. [Citation1] and follow similar lines of derivations. The difference in our approach is that we use a Malliavin derivative defined for Lévy processes. This allows us to generalize the results in [Citation1] to processes with jumps.
In the following theorem we derive a first representation result for the conditional expectation , where the function f possesses a bounded and continuous derivative.
Theorem 3.1.
Denote by the space of continuously differentiable functions with bounded derivative. Moreover, Let
be a Borel measurable function, F and G be in
, u be in
such that
is in
, and
(3.1)
(3.1)
Then it holds for any that
(3.2)
(3.2)
Proof.
We note that
As a first step we show that for any ,
(3.3)
(3.3)
where
Hereto we approximate the function by a sequence of bounded continuous functions
. Specifically,
has support
and equals
on
. Moreover the graph of
connects the points
and
and the points
and
via straight lines. Besides, we define
. Then we have by the duality formula, the chain rule, and relation (3.1) that
Because of the facts that and
, the latter expression converges to
by the definition of the sequence
. Besides
, and the sequence
approximates
. Hence this proves (3.3).
Next, by the integration by parts formula we find
Then applying the latter result for too, shows that
Since it holds that , and
for ε tending to zero, this concludes the proof.□
The latter theorem provides us with a representation formula for the conditional expectation for f being a continuously differentiable function. However in many applications in finance, we often have to consider non-smooth functions. In order to deal with the potential non-smoothness of f, we include an additional assumption on the process u introduced in Theorem 3.1 leading to the following theorem.
Theorem 3.2.
Let F and G be in and f be a Borel measurable function such that
. Consider a process u in
, guaranteeing
is in
, satisfying (3.1) and, in addition,
(3.4)
(3.4)
Then the following representation holds for
(3.5)
(3.5)
Proof.
i) First, let us consider a Borel measurable function , such that we can apply Theorem 3.1. Because of the properties of conditional expectations and relation (3.4), we have in representation (3.2) that
Thus we obtain representation (3.5).ii) Now we observe a Borel measurable function f for which . Let
and
be the space of infinitely differentiable functions with compact support respectively defined in
and Ω. Since
is dense in
there exists a sequence of functions fn in
, such that
converges to f(F) in
. In part i) we concluded that for any function fn in this sequence representation (3.5) holds. By convergence arguments, we conclude that expression (3.5) also holds for the limit function f as follows. For any fn we denote
Besides we define
Via the Cauchy–Schwarz inequality, we derive that
For any we have
By the density argument converges to f(F) in L2-sense, hence we obtain that
Thus, convergences to
. Moreover it holds that
converges to
by the conditional dominated convergence theorem. Therefore, we conclude that
equals the latter conditional expectation. □
Via the MM we also deduce a representation for the delta in terms of unconditional expectations.
Theorem 3.3.
Consider the same setting as in Theorem 3.2 and assume that
(3.6)
(3.6)
Then the delta is given by
(3.7)
(3.7)
where
Proof.
The structure of formula (3.7) follows clearly from the derivation of representation (3.5). Now we focus on the derivative
i) First, we consider a Borel measurable function
. It can be shown, as before in Theorem 3.1, that
and therefore
By the chain rule and the integration by parts formula, we obtain
By expression (3.6), the latter expectation equals
Hence, we conclude that
(3.8)
(3.8)
ii) Via a density argument which follows the same lines as the proof of Theorem 3.2, we conclude that Equationequation (3
(3.1)
(3.1) .8) also holds for a Borel measurable function f such that
.
□
Remark 3.4.
Remark that condition (3.6) is fulfilled by a combination of condition (3.4) and being
-measurable, since in this case
Next, some practical examples motivated from financial applications are given.
3.2. Examples: Jump-diffusion models
We start by considering a stock price process which is modeled by a general stochastic differential equation (SDE). For this model we derive representations based on the results developed in Subsection 3.1. Further on, we illustrate these by looking into some specific types of SDEs such as exponential Lévy and stochastic volatility models. Let S satisfy the following stochastic differential equation
(3.9)
(3.9)
where W is a Wiener process and
is a compensated Poisson random measure with Lévy measure
. We assume that
for all
. The coefficient functions
are Lipschitz continuous in the second argument, for all
. The coefficients also satisfy the following linear growth condition
for all
, where C is a positive constant. The existence and uniqueness of the solution S is ensured by Theorem 9.1. Chap IV collected from Ikeda and Watanabe [Citation33].
The first variation process V related to S equals and satisfies
We assume that the coefficients are such that V is strictly positive. The stock price St is in for all
, and its Malliavin derivative can be expressed in terms of the first variation process (see Theorem 3 and Proposition 7 in Petrou [Citation15])
(3.10)
(3.10)
The aim is to find a representation formula for the conditional expectation and
, containing only regular expectations. First we mention the following lemma. We do not present the proof since it is an adaptation of the proof of [Citation1, Lemma 4.1] to our setting.
Lemma 3.5.
It holds that
where
. In other words ζ is the solution of the SDE
Now we have all the ingredients to obtain a useful expression for the conditional expectation , for
. First we make use of Theorem 3.1 and later on we apply Theorem 3.2.
Proposition 3.6.
Let , and
. In the setting described by the stochastic differential equation (3.9) we assume that
(3.11)
(3.11)
Then the following representation holds for the conditional expectation
(3.12)
(3.12)
where the Malliavin weights equal
(3.13)
(3.13)
Proof.
We apply Theorem 3.1 and to fulfill condition (3.1) we define
Note that the process is predictable. By the first condition in (3.11) it turns out that this process is in
. Moreover by Lemma 3.5 and the chain rule it holds that
is in
. The second part of condition (3.11) allows us to conclude that
is in
.
The first weight that we calculate is the Skorohod integral of . Thereto we perform integration by parts,
Because of the chain rule we rewrite this as
Now we make use of Lemma 3.5 and obtain that the latter equals
which is the mentioned expression for π1.
The second weight in (3.2) is
□
Theorem 3.2 can also be applied in this setting, which is interesting in case of non-differentiable functions f.
Proposition 3.7.
Consider again the setting defined by the stochastic differential equation (3.9). For any Borel measurable function f for which , and
it holds, under conditions (3.11), that
where the Malliavin weight π differs from π1 in (3.13) as follows
(3.14)
(3.14)
Proof.
For the application of Theorem 3.2, we need the process
(3.15)
(3.15)
By comparing this with the intermediate process used in the proof of Proposition 3.6, we conclude that is in
. Moreover by the integration by parts formula and the fact that
is predictable, we obtain
where π1 is defined in (3.13). The last term equals zero, since by Lemma 3.5 the Malliavin derivative
introduces a factor
. This concludes the proof. □
In the sequel, we present some models to illustrate our results from Propositions 3.6 and 3.7. The first two are defined by a linear SDE and the third one concerns stochastic volatility models.
3.2.1. Exponential Lévy model
We consider a stock price process S modeled by a stochastic exponential of a Lévy process, therefore let S satisfy the following linear SDE
where μ and
are constants. We assume that
. All assumptions imposed on model (3.9) are satisfied. In this particular example, the first variation process V equals
and
and conditions (3.11) are fulfilled. From Proposition 3.6, we find that the expression (3.12) holds with
(3.16)
(3.16)
and
Substitution of the expressions for π1 and π2 into (3.12) leads to
where
, and
.
On the other hand, we apply Proposition 3.7 for the linear SDE we are observing now. The weight π differs from the weight π1 in Proposition 3.6, when the intermediate process is of the form (3.15), only by the second term in (3.14). In the present setting, this term equals
Hence combining this with (3.16) gives
(3.17)
(3.17)
For any Borel measurable function f guaranteeing that , and
the conditional expectation can be rewritten as
3.2.2. Merton model
In the light of the numerical experiments, we consider again the Merton model (2.6) which is a special case of the exponential Lévy model. The representations obtained through the MM, thus via Proposition 3.7 and Theorem 3.3, are as follows, for and
,
(3.18)
(3.18)
(3.19)
(3.19)
with
where the weight
is given by (3.17) and
is obtained through similar computations as above in this section.
3.2.3. Stochastic volatility models
Let us consider the following model
(3.20)
(3.20)
with
and
. Herein,
is the jump measure of a compound Poisson process with Lévy measure
, and
and
are two correlated standard Brownian motions with
(3.21)
(3.21)
Moreover , the functions a and b on
are Lipschitz continuous and differentiable in the second argument for all t, v is a positive function which is Lipschitz continuous and differentiable on
, and ψ is a function on
such that
. The process S may then perform the role of the stock price process, while v(Y) is interpreted as the stochastic volatility process. In many stochastic volatility models, the volatility v(Y) equals
and some conditions should be included to guarantee the non-negativity of the process Y. Some interesting examples are the Bates model (see [Citation34]) and the Ornstein–Uhlenbeck stochastic volatility model (see [Citation29, Citation35]).
From (3.21) we know there exists a Brownian motion , independent of
, such that we express
in terms of
and
by
Using the notations of Propositions 3.6 and 3.7, where we consider the Malliavin derivative in the direction of the Brownian motion , we have
(3.22)
(3.22)
Applying Proposition 3.6, we find for the weights in representation (3.12)
and
. When we prefer not to use the derivative of the function f, we apply Proposition 3.7. The weight is then given by
Considering the model (3.20), we derived a representation for . In the sequel we observe the conditional expectation
(3.23)
(3.23)
for a certain Borel measurable function
. Our motivation to consider the latter expression comes from a paper by Martynov and Rozanova [Citation36], where the authors are interested in the computation of conditional moments of Y. Thus, they consider (3.23) for w(x) = x and
. Moreover, in [Citation37] van der Stoep et al. consider (3.23) for
, which is interesting for the study of stochastic local volatility.
We consider model (3.20) and a function w. It is clear that since Y only depends on
, which is independent of
. Thus condition (3.4) is satisfied for any process u in
. Thus when condition (3.1) is fulfilled, the conditional expectation can be written in the form (3.5). From expression (3.10) and previous derivations (3.22) we deduce that
Therefore the process satisfying condition (3.1) is given by
The Skorohod integral of this process is computed similarly as in the proof of Proposition 3.6 and it equals
By the chain rule, the second term in the last equation equals
Finally we conclude that
4. Variance reduction
In the representations considered in the previous sections the random variables whose expectation should be estimated can have a large variance. To obtain a smaller variance and satisfactory convergence results in the context of Monte Carlo simulations, one might include variance reduction techniques. In Moreni [Citation38], a variance reduction technique based on importance sampling is proposed for the Monte Carlo pricing of American options via the Longstaff–Schwartz algorithm. For our conditional expectation representations, we study in subsection 4.1 the localization technique. This technique was used in Bally et al. [Citation39] but we adapt it here to our setting. Moreover we include control variates to reduce the variance. We handle this approach in subsection 4.2.
4.1. Localisation
We adapt the localization technique of Bally et al. [Citation39] for both methods; the conditional density method and the MM. For the proofs of the two following propositions, we refer the reader to Appendix C.
Proposition 4.1.
Assume the setting of Theorem 2.2. Then for any function satisfying
and for all
, we have
where
is given by
where
.
Proposition 4.2.
Assume the setting of Theorem 3.2, then for any continuous function with bounded derivative satisfying
and for all
, we have
where
is given by
where
.
Once we have introduced the localized versions of the representation formulas for the conditional expectation, one natural question arises, namely what is the optimal choice of the localizing function ψ. To find this optimal function, we assume that the additional constant c in the function H is zero, i.e. . Let Z represent either the factor
in case of the conditional density method or the factor 1 when the MM is considered. Then, practically speaking, an expectation of the form
is estimated via Monte Carlo simulation. More precisely if we denote by N the number of simulated values of F and G, we have the following estimation
In order to reduce the variance, the idea is to minimize the integrated mean squared error with respect to the localizing function ψ. Thus, we have to solve the following optimization problem (this criterion has been introduced by Kohatsu-Higa and Petterson [Citation40])
(4.1)
(4.1)
where
and I equals the integrated variance up to a constant (in terms of ψ)
(4.2)
(4.2)
The choice of the optimal localizing function ψ is given in the following proposition. It is obvious that the optimal localization function will be different for the numerator and denominator since the optimization problem is different. (The proof in Bally et al. [Citation39] can easily be extended to the current setting.)
Proposition 4.3.
The infimum of the optimization problem (4.1) with given by (4.2) and
, is reached at
, where
is the probability density of the Laplace distribution with parameter
, i.e. for all
, where
(4.3)
(4.3)
The localizing function defined in the previous proposition is optimal in the sense of minimal variance, however it is not optimal in numerical experiments when it comes to the computational effort. Therefore, Bouchard and Warin [Citation22] considered the exponential localizing function
(4.4)
(4.4)
where
is given by (4.3). In paragraph 5.2.4 we show how the use of this function reduces the computational effort. We perform numerical experiments for both localizing functions in Section 5.
The representations for the derivatives in Theorems 2.3 and 3.3 have a localized version too. We state the localized versions as well as the choice of the optimal localizing function ψ in the following propositions. We do not present the proofs since they follow along similar lines as Propositions 4.1, 4.2, and 4.3.
Proposition 4.4.
Assume the setting of Theorem 2.3, then for any function satisfying
and for all
, we have
where
,
Proposition 4.5.
Assume the setting of Theorem 3.3, then for any continuous function with bounded derivative satisfying
and for all
, we have
where
,
The optimal localizing functions minimize the integrated variance
(4.5)
(4.5)
Proposition 4.6.
The infimum of the optimization problem , with
given by (4.5), where
, is reached at
, where
is the probability density of the Laplace distribution with parameter
, i.e. for all
, where
4.2. Control variate
Another approach to obtain variance reduction (besides localization) is to include a control variate, see, e.g., [Citation19, Section 4.1]. The advantage of adding a control variate is to use the observed error in estimating a known quantity to adjust an estimator for an unknown quantity. In case of American option pricing, the control variate can be the corresponding European option price. The price of the American and respectively the European option with maturity T and payoff function , on an asset with value α at time t is denoted by
, respectively
. Let us define the function
, for a real number γ close to 1. Then it holds that
where
denotes the set of all stopping times in
. The price of the American option at time 0 is given by
and its delta equals
. We rewrite this formula for the American option price as
From this expression, the advantage of adding a control variate is clear. Indeed, the error between and an estimation of
for each
is used to adjust the estimation of the American option price
.
Example 4.7.
(Merton model). The European option price in the Merton model is derived in Merton [Citation21]. In the setting described in paragraph 2.2.1, where the price process is as described in (2.6), the European put option price is given by the series
(4.6)
(4.6)
Herein μ is the jump intensity of the Poisson process N introduced in (2.6) and is the Black-Scholes price of the European put option with the same maturity, strike, and interest rate r, and where the underlying stock price process has variance
. The first 20 terms in the series are sufficient for a good approximation for the put option price.
5. Numerical experiments
In this section, we apply our results to estimate the price and delta of American options at time zero. We illustrate our methods with numerical results in a specified jump-diffusion model.
5.1. Algorithm to estimate prices and deltas of American options
American options can be executed at any time prior to maturity. Since it is practically impossible to observe the possibility to execute the option at infinitely many times, an American option is often approximated by a Bermudan option with the same maturity and payoff function. To obtain this approximation, the time interval is discretised into n time periods with step size
. The Bermudan option can then be executed at the n discrete times
. When the number of time periods increases, the Bermudan option converges to the American option (see Bally and Pagès [Citation13]). Bermudan options can be priced through a Bellman dynamic programing principle, see Bellman [Citation41] and Bally et al. [Citation39]. Let
denote the payoff function and S the underlying stock price process with initial value s0. Then the price of the Bermudan option
follows from the recursive computations
(5.1)
(5.1)
The sensitivity of the option price with respect to the initial value of the underlying asset, i.e. the delta of the option , can be derived as follows
Hence to obtain a numerical estimation of the price and the delta at time zero, we proceed by estimating the prices and the deltas recursively and backwards in time. For estimations based on simulated values for the underlying stock price, one can simulate the number of required paths at the discrete time points and store them all before performing the recursive computations. On the other hand, since the pricing program and computations of the deltas go backwards in time, it is more convenient to simulate the stock price process simultaneously. Simulating the stock price process backwards in time too leads to more efficiency concerning memory capacity.
Remark 5.1.
(Nested simulations). To estimate the values for for
, the estimation of the conditional expectation requires the simulation of N values of
starting from the value of
. In total this results into Nk used simulated values for
for any k. The Bellman dynamic programing principle requires nested simulations to estimate a function of conditional expectations similarly to the compound option case in [Citation19, Example 1.1.3] and illustrated in Figure 1.3 of that book. However, by the use of the obtained representations, the number of simulated values can be considerably reduced. The resulting algorithm needs one single set of simulated paths. For any
only N simulated values for
will be required.
5.2. Implementations for American put options in a Merton model
We consider American put options on a stock price process S defined by the Merton model (2.6). The put payoff function equals . Since we want to compare our results to the analysis in Amin [Citation42], we use the parameter setting as in his paper. That explains our choice of this specific connection between the jump mean and jump variance. This simplifies the Merton formula (4.6).
5.2.1. Representations
The conditional expectations and their derivatives in (5.1) and (5.2) can be estimated based on the representations we developed in the previous sections. In particular, in the present Merton setting, the representations are presented in paragraphs 2.2.1 and 3.2.2. Throughout this section we consider .
The regular expectations appearing in the representations (2.7), (2.8), (3.18), and (3.19) can easily be estimated by a Monte Carlo simulation. For example, consider the estimation of the numerator of representation (2.7). We require N simulated values of St, Ss, and , belonging to the same path. If we denote the j-th simulated values by
, and
, then we approximate
(5.3)
(5.3)
5.2.2. Variance reduction techniques
As discussed in paragraph 4.1, we include the localizing technique. The estimation (5.3) is then replaced by
where Zs equals Ss in case of the CDM and 1 in case of the MM. The functions ψ and
are defined by Proposition 4.3.
On the other hand, we include a control variate, see subsection 4.2. For the estimation of the American option price and delta
, we include the European option as a control variate. In the current setting, the European option price and delta can be obtained through Merton’s approach, see Example 4.7. Consider the algorithm for the price of Bermudan options (5.1). To introduce the control variate, we proceed in two steps. First we replace the put payoff function at each time
, by
where γ is a real number close to one and
denotes the European option price, obtained through Merton’s approach, at time
. Secondly, in the last step (k = 0) we add
(respectively
) to obtain the American option price
(respectively the American option delta
).
5.2.3. Backward simulation
As remarked at the end of subsection 5.1 the algorithm for the pricing of a Bermudan option goes backwards in time and we can simulate the different stochastic variables backwards in time too. For the Brownian motion we base the backward simulation on a Brownian bridge (see Bally et al. [Citation39]). To simulate the compound Poisson process backwards in time, we base our method on results of Karatzas and Shreve [Citation43] and Baldeaux [Citation20]. We split the simulation of a compound Poisson process in the simulation of a Poisson process and in the simulation of the sum of the jump sizes. First we mention the following proposition implying a backward simulation algorithm for a Poisson process. This is covered by [Citation20, Lemma 3.1].
Proposition 5.2.
Let N be a Poisson process with intensity μ. For any time t > 0 it holds that Nt has a distribution. Moreover for any
it holds that Ns, conditioned on Nt = z, follows a
distribution.
Secondly we present the following proposition considering the (conditional) distribution of sums of independent and identically normal distributed variables. This result is a consequence of Brownian bridges, see Karatzas and Shreve [Citation43].
Proposition 5.3.
Consider the following sum
where Yi are i.i.d.
. For any k > 0 it holds that
has a
distribution. Moreover for any
it holds that
, given that
, has a
distribution.
The backward simulation technique is interesting in numerical applications and following Baldeaux [Citation20], this technique can also be derived for the Kou model, see Kou [Citation44].
5.2.4. Reduction of computational effort
Bouchard and Warin [Citation22] observed that the computational effort to estimate the American option prices by a MM is reduced by sorting the estimated stock prices. Consider the Bermudan dynamic programing algorithm (5.1). For a fixed k in we estimate the conditional expectations for
by our representations, including localization, as follows
where
If we consider the exponential localizing function (4.4), then it holds that
Now let us sort the simulated paths such that the values increase for q going from 1 to N and let us indicate this by the superscript s, say
. Then we write for each q
Thus for q going from N to 1, the sums in the numerator and denominator get only one additional term. Hence to estimate for each q, we make use of the previously performed computations for q + 1.
5.3. Numerical results for the Merton model
In this subsection, we present the numerical results obtained via our representations in the context of Bermudan options. We compare our results for the prices to those reported by Amin [Citation42] and to the regression based method introduced by Longstaff and Schwartz [Citation8]. For the deltas, we compare our results to those of Hilliard and Schwartz [Citation45]. To evaluate the accuracy of our representations, we consider European options since there are analytic formulas at hand in the Merton model, whereas there are non for Bermudan and American options. To overcome this problem, we compute confidence intervals for the prices as discussed in Section 3.1 in Bouchard and Warin [Citation22].
The following parameter set for a put option on the underlying stock price process S is used,
(5.4)
(5.4)
5.3.1. Accuracy of the method
European options may only be executed at time of maturity T. However, they can be traded at any moment between time 0 and T. Consider the risk-free interest rate r and the underlying stock price process S, then the price at time t > 0 of a European option with payoff function equals
(5.5)
(5.5)
The delta at time t equals
(5.6)
(5.6)
The conditional expectations and their derivatives appearing in (5.5) and (5.6) are estimated following the techniques described in subsection 5.2 (except the control variate). As an example, we estimate the prices and deltas
of a European put option with maturity T = 1 and strike K = 45 on the underlying S described in (5.4), at times
and for
. We do not consider European option prices or deltas at time zero since they do not involve conditional expectations. The estimation of the prices or deltas based on the CDM or MM approach includes the localizing technique. Each estimate results from the same set of
simulated paths. In , we present the CDM and the MM estimates for the option prices for
. We also report the relative errors in percentages to the Merton option prices, see Example 4.7. Similar results were obtained for the other values of
. shows the corresponding results for the deltas. It turns out that the relative errors when comparing our approach to the one of Merton [Citation21] are very small. Hence the algorithm we developed based on our representations is accurate for European options.
Table 1. Estimates of European put option prices (5.5) via the CDM and MM approach, with relative errors to the Merton prices in percentages.
Table 2. Estimates of European put option deltas (5.6) via the CDM and MM approach, with relative errors to the Merton deltas in percentages.
5.3.2. Results for Bermudan option prices and deltas
We consider a Bermudan put option on the stock price process S with parameters given in (5.4), the strike is fixed at K = 45. Amin [Citation42] and Hilliard and Schwartz [Citation45] developed a tree method to estimate Bermudan and American option prices. In the current setting their estimate for the option price equals 9.954. The Merton European option price at time zero equals 9.422. We choose n = 10. The dynamic programing algorithm presented in subsection 5.1 and our representations are used to estimate and
.
and illustrate the influence of the variance reduction techniques on the estimates for the price. The graphs on the right hand side are obtained by zooming in on the left graphs. Notice the difference in scale between the left and the right graph on the vertical axis. For , we simulated N paths of the underlying at the discrete time points jT/n,
, and we estimated the option price at time zero through the CDM and the MM, with and without control variate and optimal localization technique. In case the European option is included as a control variate, we put
.
Figure 1. Estimates for the Bermudan put option price obtained through the CDM and MM representations without control variate and without localization technique, against the number of simulated paths. In the right graph the vertical axis is restricted to .
![Figure 1. Estimates for the Bermudan put option price obtained through the CDM and MM representations without control variate and without localization technique, against the number of simulated paths. In the right graph the vertical axis is restricted to [0,100].](/cms/asset/1266c6b0-d996-4dba-bcbd-92da19d3452c/lsaa_a_1561306_f0001_c.jpg)
The variance reduction techniques have a remarkable improvement on the results obtained via the CDM and MM approaches. It appears that the CDM results show some more variation than the MM results.
presents the estimated prices of the Bermudan put option with strikes 30, 35, 40, 45, and 50, obtained through the sorted CDM and MM approach including the control variate and the exponential localization function. For these estimates, a time discretisation is performed for n = 10 and paths were simulated. We include the estimates for the prices obtained respectively by Amin [Citation42] and by using a regression based method with control variate as in Longstaff and Schwartz [Citation8].
Table 3. Estimates of Bermudan put option prices and confidence intervals for parameter set (5.4), obtained through the sorted CDM and MM approach with control variate and exponential localization and by the Longstaff–Schwartz method [Citation8] with a control variate (LSM). n = 10 and .
To evaluate the accuracy of our method, we include confidence intervals for the prices. The computation of these confidence intervals is discussed in [Citation22, Section 3.1]. In our case, we include two confidence intervals which we compute using respectively the CDM and the MM. It turns out that the prices we find lay within the confidence intervals and thus they are in an acceptable range.
As described in paragraph 5.2.4, the computational effort is reduced when we perform a sorted algorithm. presents the CPU time in seconds for the LSM, CDM, and MM, respectively. We do not compare the time to Amin [Citation42] and Hilliard and Schwartz [Citation45] methods since there is no clear indication about how long their algorithms take. It turns out that when considering 10 time steps, our algorithms are comparably fast to the regression based algorithm. As analyzed in Bouchard and Warin [Citation22], the complexity of our algorithms for both the MM and the CDM, when considering the sorted localizing method is of order , where d is the dimension of the underlying factor. The complexity of the LSM method depends on the choice of the basis of polynomials as well as the number and power of these polynomials. However, this is not the aim of the study of this paper and we refer to many articles in the literature that studied this issue for LSM. In, e.g. the article by Zhou [Citation46], the author investigated whether there exists an optimal regression complexity in the LSM framework for options pricing. See also the analysis in Bouchard and Warin [Citation22] and in the thesis of Plavsic [Citation47]. Notice that in this latter thesis, the author pointed out that the computational time for LSM is proportional to the average number of in-the-money paths (i.e. the option’s moneyness). That explains why for increasing values of the strike K, the CPU time for LSM increases in .
Table 4. CPU time in seconds for the different methods to compute the estimates of Bermudan put option prices for parameter set (5.4), obtained through the sorted CDM and MM approach with control variate and exponential localization and by the Longstaff–Schwartz method [Citation8] with control variate (LSM). n = 10 and .
presents the estimated deltas of the Bermudan put option with strikes 30, 35, 40, 45, and 50, obtained through the sorted CDM and MM approach including the control variate and the exponential localization function. For these estimates a time discretisation is performed for n = 10 and paths were simulated.
Table 5. Estimates of Bermudan put option deltas for parameter set (5.4), obtained through the sorted CDM and MM approach with control variate and exponential localization. n = 10 and .
We conclude that the extension that we provided to the geometric Brownian motion model observed in Bally et al. [Citation39] by adding normally distributed jumps to the driving process leads to numerical results which are in line with those found in the literature. As is the case in Bouchard and warin [Citation22], the Monte Carlo method showed to be very promising in the context of jump-diffusions and could be further improved.
5.4. Numerical results for the additive model
In this subsection, we consider the additive model (2.9) with X as described in (2.10) and Y given by
(5.7)
(5.7)
where
is a compound Poisson process with intensity μ and exponentially distributed jumps with parameter ν.
The aim is to compute the price of Bermudan options as time-discrete approximations to American options written on such models using the conditional density representation. For the numerical example to be relevant for the energy markets, we note that Benth et al. [Citation48] showed empirically that the spot model fits the Phelix Base electricity price index at the European Power Exchange (EEx) very well. In their article, they estimated the parameters in the suggested model and found the following values
(5.8)
(5.8)
We compare our conditional density method to the regression based method as in Longstaff and Schwartz [Citation8]. represents the estimated prices of the American put option with strikes 5.5, 5.25, 5, 4.5 obtained through the sorted conditional density method including the exponential localization function. For these estimates, the number of exercise dates n = 10 and paths were simulated. For the same parameters, we include the estimates for the prices obtained by the regression method which we denote in the table by LSM and also the cost in seconds for each method.
Table 6. Estimates of American put option prices for parameter set (5.8), obtained through the sorted CDM approach with exponential localization. n = 10 and .
In the case, the strike K = 5 and n = 10 the LSM indicates rank deficiency. That could explain the difference in the prices given respectively by the LSM and the CDM in the third row of . This is due to the fact that the LSM algorithm for pricing American options is unstable when the time parameter is small. See for example the discussion in Mostovyi [Citation23].
The CDM with sorting as shown in is comparably fast to the LSM. It can always be applied to compute the price of the American option in contrary to the LSM.
6. Conclusion
Conditional expectations play an important role in the pricing and hedging of financial derivatives. In the literature, there exist several methods for the numerical computations of conditional expectations. One of the methods is to use Monte Carlo by rewriting conditional expectations in terms of expectations without conditioning but involving weights. This was first discussed in Fournié et al. [Citation1] in a continuous framework. In this paper we extended this latter approach to include Lévy and jump-diffusion processes. For this purpose we used two approaches: the conditional density method and the MM. We applied the developed theory to the estimation of Bermudan and American option prices and their deltas. We used a localization technique and a control variate to improve the estimation of the involved expectations. Moreover, we illustrated our results with different examples and found accurate numerical results.
As far as further investigations are concerned, one may study other choices of the weights in the representation of the conditional expectations. Notice that there are infinitely many possibilities for the weights and thus infinitely many representations of the conditional expectations. Moreover, one can study parallelization for the Monte Carlo method with Malliavin calculus to improve the numerical results as discussed in Abbas-Turki and Lapeyre [Citation18].
Finally, one can also study the MM for the computation of conditional expectations where conditioning is considered w.r.t. two random variables. One might think for example of the hedging of Asian options or spread options. In this context, the Longstaff–Schwartz method is not suited since the regression with two-dimensional explanatory data becomes numerically involved. The MM can be promising in this case.
Disclosure statement
No potential conflict of interest was reported by the authors.
Additional information
Funding
References
- Fournié, E., Lasry, J.-M., Lebuchoux, J., Lions, P.-L. (2001). Applications of Malliavin calculus to Monte Carlo methods in finance II. Finance Stochast. 5(2):201–236. DOI: 10.1007/PL00013529.
- Montero, M., Kohatsu-Higa, A. (2003). Malliavin calculus applied to finance. Physica A Statis. Mech. Appl. 320:548–570. DOI: 10.1016/S0378-4371(02)01531-5.
- Nualart, D. (2006). The Malliavin Calculus and Related Topics. Berlin, Germany: Springer.
- Di Nunno, G., Meyer-Brandis, T., Øksendal, B., Proske, F. (2005). Malliavin calculus and anticipative itô formulae for lévy processes. Infin. Dimens. Anal. Quantum Probab. Relat. Top. 8(2):235–258. DOI: 10.1142/S0219025705001950.
- von Sydow, L., Josef Höök, L., Larsson, E., Lindström, E., Milovanović, S., Persson, J., Shcherbakov, V., Shpolyanskiy, Y., Sirén, S., Toivanen, J., et al., Zhao, Y. (2015). BENCHOP-the BENCH marking project in option pricing. Int. J. Comput. Math. 92(12):2361–2379. DOI: 10.1080/00207160.2015.1072172.
- Broadie, M., Glasserman, P. (1997). Pricing American-style securities using simulation. J. Econ. Dynam. Control 21(8-9):1323–1352. DOI: 10.1016/S0165-1889(97)00029-8.
- Barraquand, J., Martineau, D. (1995). Numerical valuation of high dimensional multivariate American securities. J. Finance Quantitative Anal. 30(3):383–405. DOI: 10.2307/2331347.
- Longstaff, F. A., Schwartz, E. S. (2001). Valuing American options by simulations: a simple least squares approach. Rev. Finan. Stud. 14(1):113–148. DOI: 10.1093/rfs/14.1.113.
- Shu, J., Gu, Y., Zheng, W. (2002). A novel numerical approach of computing American option. Int. J. Found. Comput. Sci. 13(05):685–693. DOI: 10.1142/S0129054102001394.
- Kovalov, P., Linetsky, V., Marcozzi, M. (2007). Pricing multi-asset American options: a finite element method-of-lines with smooth penalty. J. Sci. Comput. 33(3):209–237. DOI: 10.1007/s10915-007-9150-z.
- Zhang, B., Oosterlee, C. W. (2012). Fourier cosine expansions and put–call relations for Bermudan options. In: Carmona, A. R., Del Moral, P., Hu, P., and Oudjane, N., (eds.). Numerical Methods in Finance: Bordeaux. Berlin: Springer, pp. 323–350.
- Mrad, M., Touzi, N., Zeghal, A. (2006). Monte Carlo estimation of a joint density using Malliavin calculus, and application to American options. Comput. Econ. 27(4):497–531. DOI: 10.1007/s10614-005-9005-3.
- Bally, V., Pagès, G. (2003). Error analysis of the quantization algorithm for obstacle problems. Stochastic Process. Appl. 106(1):1–40. DOI: 10.1016/S0304-4149(03)00026-7.
- Benth, F. E., Di Nunno, G., Khedher, A. (2010). Lévy models robustness and sensitivity. In QP-PQ: Quantum Probability and White Noise Analysis, Proceedings of the 29th Conference in Hammamet, Tunisia, 1318 October 2008. H. Ouerdiane and A Barhoumi (eds.). 25:153–184.
- Petrou, E. (2008). Malliavin calculus in lévy spaces and applications to finance. Electron. J. Probab. 13(0):852–879. DOI: 10.1214/EJP.v13-502.
- Davis, M. H. A., Johansson, M. P. (2006). Malliavin Monte Carlo Greeks for jump-diffusions. Stochastic Process. Appl. 116(1):101–129. DOI: 10.1016/j.spa.2005.08.002.
- Benth, F. E., Di Nunno, G., Khedher, A. (2011). Robustness of option prices and their deltas in markets modelled by jump-diffusions. Commun. Stochastic Anal. 5(2):285–307.
- Abbas-Turki, L. A., Lapeyre, B. (2012). American options by Malliavin calculus and nonparametric variance and bias reduction methods. SIAM J. Finan. Math. 3(1):479–510. DOI: 10.1137/11083890X.
- Glasserman, P. (2004). Monte Carlo Methods in Financial Engineering. Berlin: Springer.
- Baldeaux, J. (2008). Quasi-Monte Carlo methods for the Kou model. Monte Carlo Methods Appl. 14(4):281–302.
- Merton, R. (1976). Option pricing when underlying stock returns are discontinuous. J. Finan. Econ. 3(1-2):125–144. DOI: 10.1016/0304-405X(76)90022-2.
- Bouchard, B., Warin, X. (2012). Monte-Carlo valuation of American options: Facts and new algorithms to improve existing methods. Num. Meth. Finan. Springer Proceedings in Mathematics 12:215–255.
- Mostovyi, O. (2013). On the stability the least squares Monte Carlo. Optim. Lett. 7(2):259–265. DOI: 10.1007/s11590-011-0414-z.
- Protter, P. (2005). Stochastic Integration and Differential Equations. 2nd edn, Version 2.1. No. 21 in Stochastic Modelling and Applied Probability. Berlin: Springer.
- Raju, C. K. (1982). Products and compositions with the Dirac Delta function. J. Phys. A Math. Gen. 15(2):381–396. DOI: 10.1088/0305-4470/15/2/011.
- Daveloose, C. (2016). Essays on Pricing and Hedging in Markets with Imperfections. Ph.D. thesis, Ghent University.
- Benth, F. E., Kallsen, J., Meyer-Brandis, T. (2007). A non-Gaussian Ornstein-Uhlenbeck process for electricity spot price modeling and derivatives pricing. Appl. Math. Finance 14(2):153–169. DOI: 10.1080/13504860600725031.
- Sato, K.-I. (1999). Lévy Processes and Infinitely Divisible Distributions. No. 68 in Cambridge Studies in Advanced Mathematics. Cambridge: Cambridge University Press.
- Barndorff-Nielsen, O. E., Shephard, N. (2001). Modelling by Lévy processes for financial econometrics. Barndorff-Nielsen, O., Mikosch, T., and Resnick, S. (eds.), Lévy Processes: Theory and Applications. Basel, Switzerland: Birkhäuser, pp. 283–318.
- Brewer, D. W., Tubbs, J. D., Smith, O. E. (1987). A differential equations approach to the modal location for a family of bivariate gamma distributions. J. Multivariate Anal. 21(1):53–66. DOI: 10.1016/0047-259X(87)90098-4.
- Bowman, F. (1958). Introduction to Bessel Functions. New York: Dover Publications Inc.
- Solé, J. L., Utzet, F., Vives, J. (2007). Canonical Lévy process and Malliavin calculus. Stochastic Process. Appl. 117(2):165–187. DOI: 10.1016/j.spa.2006.06.006.
- Ikeda, N., Watanabe, S. (1981). Stochastic Differential Equations and Diffusion Processes. New York: Elsevier Science.
- Bates, D. (1996). Jumps and stochastic volatility: exchange rate processes implicit in Deutsche Mark options. Rev. Finan. Stud. 9(1):69–107. DOI: 10.1093/rfs/9.1.69.
- Barndorff-Nielsen, O. E., Shephard, N. (2002). Econometric analysis of realized volatility and its use in estimating stochastic volatility models. J. Royal Stat. Soc. B 64(2):253–280. DOI: 10.1111/1467-9868.00336.
- Martynov, M., Rozanova, O. (2013). On dependence of volatility on return for stochastic volatility models. Stochastics 85(5):917–927. DOI: 10.1080/17442508.2012.673616.
- van der Stoep, A. W., Grzelak, L. A., Oosterlee, C. W. (2014). The Heston stochastic-local volatility model: efficient Monte Carlo simulation. Int. J. Theor. Appl. Finan. 17(7):14050045.
- Moreni, N. (2004). A variance reduction technique for American option pricing. Physica A Statis. Mech. Appl. 338(1-2):292–295. DOI: 10.1016/j.physa.2004.02.055.
- Bally, V., Caramellino, L., Zanette, A. (2005). Pricing American options by Monte Carlo methods using a Malliavin calculus approach. Monte Carlo Methods Appl. 11:97–133.
- Kohatsu-Higa, A., Pettersson, R. (2002). Variance reduction methods for simulation of densities on wiener space. SIAM J. Numer. Anal. 40(2):431–450. DOI: 10.1137/S0036142901385507.
- Bellman, R. (1957). Dynamic Programming. Princeton, NJ: Princeton University Press.
- Amin, K. I. (1993). Jump diffusion option valuation in discrete time. J. Finan. 48(5):1833–1863. DOI: 10.2307/2329069.
- Karatzas, I., Shreve, S. (1991). Brownian Motion and Stochastic Calculus. Berlin: Springer.
- Kou, S. (2002). A jump-diffusion model for option pricing. Manag. Sci. 48(8):1086–1101. DOI: 10.1287/mnsc.48.8.1086.166.
- Hilliard, J., Schwartz, A. (2005). Pricing European and American derivatives under a jump-diffusion process: a bivariate tree approach. J. Finan. Quant. Anal. 40(03):671–691.
- Zhou, Y. (2004). On the existence of an optimal regression complexity in the Least-Squares Monte Carlo (LSM) framework for options pricing. 39th Actuarial Research Conference, Iowa Memorial Union, The University of Iowa, Iowa City, April.
- Plavsic, M. (2011). Pricing American options - aspects of computation. Ph.D. thesis, Imperial College London.
- Benth, F. E., Kallsen, J., Meyer‐Brandis, T. (2007). A critical empirical study of two electricity spot price modeling and derivatives pricing. Appl. Math. Finance 14(2):153–169. DOI: 10.1080/13504860600725031.
Appendix A. Proof in Section 2
Proof of Theorem 2.2.
We consider the following approximation
and know by the conditional density method and Assumptions 2.1(1) that
We introduce two functions
By relation (2.2) and integration by parts we have
Combining the previous steps leads to the observation that
(A.1)
(A.1)
Because of the facts that is bounded in ε, that
, and that
, the limit can be brought inside the integrals in the numerator and denominator. Thus we determine
The numerator of (A.1) therefore equals
Simiatolarly the denominator of (A.1) equals
This concludes the proof of Theorem 2.2. □
Appendix B. Malliavin calculus
In this article, we make use of the Malliavin calculus as defined in Petrou [Citation15]. The following properties and definitions concerning the Malliavin derivative in the direction of the Wiener process are applied.
The Malliavin derivative in the direction of the Brownian motion is denoted by
. The space
contains all the random variables in
that are differentiable in the Wiener direction.
Chain rule
Let
and
. Then it holds that
and
Skorohod integral
Let
be the adjoint operator of the directional derivative
. The operator
maps
to
. The set of processes
such that
for all
, is the domain of
, denoted by
. For every
we define
as the Skorohod integral in the Wiener direction of u by
for any
. The equation above is called the duality formula.
Integration by parts
Let
, where
, and
. Then
and
if and only if the right hand side of the latter equation is in
.
Predictable processes
Let u be a predictable process such that
. Then
and the Skorohod integral coincides with the Ito-integral
Appendix C. Proofs localization
Proof of Proposition 4.1.
Adding and subtracting the same expression, keeping in mind that , we get for the numerator of representation (2.4), the following equality
The last term equals
Using the integration by parts formula, we get
and the result follows.
□
Proof of Proposition 4.2.
Adding and subtracting the same expression, keeping in mind that , we get for the numerator of representation (3.5) that
Applying the same arguments as in Theorem 3.2, we show that the last term equals
and the result follows.
□