Abstract
Plasmodium falciparum parasites export more than 400 proteins to remodel the host cell environment and increase its chances of surviving and reproducing. The endoplasmic reticulum (ER) plays a central role in protein export by facilitating protein sorting and folding. The ER resident member of the Hsp90 family, glucose-regulated protein 94 (Grp94), is a molecular chaperone that facilitates the proper folding of client proteins in the ER lumen. In P. falciparum, Grp94 (PfGrp94) is essential for parasite survival, rendering it a promising anti-malarial drug target. Despite this, its druggability has not been fully explored. Consequently, this study sought to identify small molecule inhibitors targeting the PfGrp94. Potential small molecule inhibitors of PfGrp94 were designed and screened using in silico studies. Molecular docking studies indicate that two novel compounds, Compound S and Compound Z selectively bind to PfGrp94 over its human homologues. Comparatively, Compound Z had a higher affinity for PfGrp94 than Compound S. Further interrogation of the inhibitor binding using molecular dynamics (MD) analysis confirmed that Compound Z formed stable binding poses within the ATP-binding pocket of the PfGrp94 N-terminal domain (NTD) during the 250 ns simulation run. PfGrp94 interacted with Compound Z through hydrogen bonding and hydrophobic interactions with residues Asp 148, Asn 106, Gly 152, Ile 151 and Lys 113. Based on the findings of this study, Compound Z could serve as a competitive and selective inhibitor of PfGrp94 and may be useful as a starting point for the development of a potential drug for malaria.
Communicated by Ramaswamy H. Sarma
1. Introduction
Parasites of the main malaria-causing agent, Plasmodium falciparum, leads a complicated life cycle in two distinct host environments. The transition of the parasite between the homeothermic human and poikilothermic mosquito hosts present physiological stress to the parasite proteome. In order to survive the temperature changes, the parasites depend on a highly efficient protein quality control system to avoid heat-induced proteopathy. As a further complication to parasite survival, the parasite infects terminally differentiated red blood cells that lack protein production machinery to support parasite survival. As such, the parasite exports 8% of its proteome to remodel the host cell to create a conducive environment for its development (Chaubeyet al., Citation2014). The Endoplasmic reticulum (ER) plays a central role in protein export through modulating the folding, maturation, sorting and trafficking of proteins to different cellular locations (Easton et al., Citation2000; McCaffrey & Braakman, Citation2016). Furthermore, the ER protein folding system comprises several molecular chaperones that assist in the folding of protein substrates (Duerfeldt et al., Citation2012). During ER stress, molecular chaperones are overexpressed via the unfolded protein response pathway, which is known to decrease stress and maintain ER homeostasis (Pugh et al., Citation2022).
The Hsp90 ER resident homologues, Grp94 are molecular chaperones involved in protein folding and stabilisation in the rather oxidising ER lumen environment which perturbs protein structure (Duan et al., Citation2021). Grp94 is a homo-dimeric protein consisting of a pre-N domain that regulates ATP hydrolysis, an N-terminal domain (NTD) that facilitates protein dimerization, and client protein maturation (Pugh et al., Citation2022). The NTD exhibit ATPase activity, and is connected to a charged linker region that assist in NTD conformational changes respectively (Eletto et al., Citation2010). The middle domain interacts with client proteins, and the C-terminal domain is known to be involved with its dimerization and co-chaperone recruitment (Hessling et al., Citation2009). Grp94 promotes both the folding of stress-aggregated proteins in the ER and the folding of newly synthesized proteins to achieve a thermodynamical and conformational functional structure (Pugh et al., Citation2022; Taipale et al., Citation2012). Furthermore, Grp94 is involved in the binding of unfolded clients in the ER lumen towards their recruitment to the ER-associated degradation (ERAD) pathway, which targets terminally misfolded proteins for degradation (Pugh et al., Citation2022). In the ER lumen, Grp94 is a major calcium-binding protein making it an essential gene involved in calcium levels regulation inside cells. Intracellular calcium levels are important in maintaining cell signalling and homeostasis (Baviskar & Shields, Citation2010). In Leishmania, Grp94 is required for post translational glycosylation of proteins implicated in virulence, which are important for its survival in the host (Descoteaux et al. Citation2002). The P. falciparum Grp94 (PfGrp94) chaperone is an enigmatic essential protein for parasite survival (Daniyan et al., Citation2019). It is plausible that the PfGrp94 may play a role in protein binding to facilitate proper protein folding, calcium binding to regulate cell signalling and facilitate the post translational glycosylation of parasite virulence proteins to assist in the invasion of new host cells (Jonsdottir et al., Citation2021). Moreover, PfGrp94 is thought to be involved in ensuring the correct folding of parasite proteins in the ER lumen (Stofberg et al., Citation2021). This makes PfGrp94 an attractive anti-malarial drug target. Like its cytosolic homologues, ATP binding and hydrolysis modulate PfGrp94 functional cycle (Stofberg et al., Citation2021).
The ATP mimicking small molecule inhibitors targeting the human Hsp90 NTD have been extensively studied and some have reached the phase II clinical stages as potential cancer treatment options (Li et al., Citation2020). Most of the ATP-mimicking compounds competitively bind into the ATP-binding pocket and abrogate ATP hydrolysis, thereby inhibiting the ATP dependent chaperone function of Hsp90 (Roe et al., Citation1999). Accumulation of unfolded and misfolded parasite proteins lead to proteopathy (Hetz et al., Citation2020). Thus, binding of ATP-mimicking compounds to the NTD of PfGrp94 would inhibit ATP hydrolysis, which provides the energy for the chaperone-assisted protein folding in the ER lumen. This subsequently results in the accumulation of unfolded proteins in the ER lumen ultimately leading to parasite death from global abnormal protein toxicity (Suresh and Haldar, Citation2018; Jonsdottir et al., Citation2021). The ER resident chaperone proteins have been observed to facilitate the unfolded protein response (UPR) in parasites exposed to artemisinin, which is a frontline antimalarial drug (Rocamora et al., Citation2018; Tilley et al., Citation2016; Zhang et al., Citation2017). The activation of UPR has been implicated as a mechanism involved in artemisinin drug resistance by the malaria parasites (Bhattacharjee et al. Citation2018; Mbengue et al., Citation2015; Mok et al., Citation2015). This further highlights the importance of ER-based chaperones as potential drug targets in the arsenal of efforts to fight antimalarial drug resistance. Therefore, in this study, we sought to provide the basis for further antimalarial drug screening targeting the essential PfGrp94 chaperone. In this study, we identified a promising compound, 4‐{5‐[(Z)‐[(isopropoxymethyl)imino]methyl]‐1H,3H,6H‐pyrrolo[3,4‐c]pyridine‐2‐carbonyl}‐6‐isopropylbenzene‐1,3‐diol and its two derivatives, as potential inhibitors of PfGrp94 using in silico screening. Both derivates have favourable binding interactions with PfGrp94 NTD through the key residues Asp 148, Asn 106, Gly 152, Ile 151 and Lys 113, which provides a scaffolding platform for further development of inhibitors as potential antimalarial drugs combating drug resistance in P. falciparum.
2. Methods and materials
2.1. Multiple sequence alignment of PfGrp94 and human Hsp90 homologues
To determine the level of conservation between the parasite and the different human Hsp90 homologues, multiple sequence alignment analysis (MSA) was conducted to identify unique motifs between the parasite and host proteins for selective targeting. The MSA on the respective N-terminal domains (NTD) of Hsp90s was conducted as previously described (Stofberg et al., Citation2021). The Hsp90 amino acid sequences were retrieved from the PlasmoDB for P. falciparum and NCBI for homo sapiens homologues with the respective accession numbers in parenthesis, PfGrp94 (PF3D7_1222300), HsHsp90α (NP_001017963.2), HsHsp90β (NP_031381.2), HsGrp94 (NP_003290.1) and TRAP-1 (NP_057376.2) in FASTA format. The respective amino acid sequences were aligned using ClustalW Multiple alignment on BioEdit v7.2.5 as previously described (Stofberg et al., Citation2021).
2.2. Structure validation of PfGrp94 and the human Hsp90 homologues
Due to the recent improvements in the refinements of three-dimensional X-ray crystallography structures, we used the re-refined and validated Hsp90 NTD crystal structures, which were retrieved from the PDB-REDO web server for PfGrp94 (3PEH), HsHsp90α (1BYQ), HsHsp90β (3NMQ), HsGrp94 (4NH9), and HsTRAP-1 (7C04). The quality of the refined 3D structures was validated using the PROCHECK program on the European Bioinformatics Institute’s PDBsum web server, and Ramachandran plot analysis of the statistical distribution of the Psi and Phi dihedral angles of the amino acids.
2.3. Ligand generation
The structures of the compounds (ligands) binding the NTD of Hsp90s were retrieved from the online publicly available ZINC online database (Irwin et al., Citation2020). The BioSolveIT SeeSAR software was used to predict and screen for compounds with high-binding affinity to Hsp90 NTD as previously described (Zaib et al., Citation2023). From an initial 1 million compounds, a compound library of 900 compounds were initially predicted to exhibit high-binding affinities in the nanomolar range against PfGrp94. The top 20 compounds from the compound library with the highest affinity for PfGrp94 were then re-docked against the four human Hsp90 paralogs: HsHsp90α, HsHsp90β, HsGrp94 and HsTRAP1 using the FlexX docking functionality of SeeSAR (Rarey et al., Citation1996). Compounds that had a predicted high-binding affinity based on docking scores for PfGrp94 and low-binding affinity for all four human Hsp90 paralogs were selected for further investigation. The compound with the most favourable binding properties to PfGrp94 was further modified using the BioSolveIT SeeSAR Inspirator plugin ReCore. The resultant small molecule compounds showing the best selective binding of the parasite Grp94 compared to the human Hsp90 homologs were screened to identify the best hit compounds.
2.4. Protein preparation
The Hsp90 NTD 3D structural models were each prepared for molecular docking using the Protein Preparation Wizard module of the Schrödinger Maestro software 2022-1. The protein preparation generated 3D structures that were energy minimized and structurally corrected by adding missing hydrogen atoms and assigning partial charges as previously described (Pooe et al., Citation2021). Bond orders were assigned to all atoms and hydrogens, and disulphide bonds. Using Epik, the het states were set to generate hydrogen atoms as they would be present at a pH of 7.0 ± 2.0. The hydrogen bonds assignment was set to ‘sample water orientations’ and then optimized by using the PROPKA program at a pH of 7.0. Water molecules that were beyond 3.0 Ǻ were removed and the heavy atoms were converged to a root mean-square deviation (RMSD) of 0.3 Ǻ. The force field was set to Optimized Potentials for Liquid Simulations 2005 (OPLS_2005).
2.5. Ligand preparation
The small molecule hit compounds were prepared for molecular docking using LigPrep of the Schrödinger Maestro software 2022-1 as previously described (Pooe et al., Citation2021). The small molecule compounds (ligand) 2D structures were initially drawn using Chem sketch 2022.2.2. These were prepared to generate energy minimised 3D structures. Furthermore, proper bond orders were assigned to correct the ionization states and protonation of the compounds. The maximum ligand size was set to 500 atoms and the force field at OPLS_2005. The Epik programme was used to generate possible ionization states and desalted tautomer’s at a physiological target pH of 7.0 ± 0.2. Up to 5 possible stereoisomers were generated for each ligand input structure, and the computation was set to retain specified chiralities. Calculations were carried out using the OPLS 2005 force field, and the final Ligprep output was in Maestro format.
2.6. Molecular docking
Molecular docking was performed to understand the selective binding mode of the Hsp90 NTD small molecule inhibitors using the Schrödinger Maestro docking program as previously described (Pooe et al., Citation2021). The standard protocol was used to generate up to 20 poses for the receptor and the box was set to the centre of specific residues of select regions with potential ligand-binding sites. Furthermore, the box size was set to dock ligands with a length of 46 Å or less. The constraints settings were left as default and for the ligand settings, the sampling ring conformations were set to an energy window of 2.5 kcal/mol. The default Glide docking receptor scaling parameters were set as follows: Receptor van der Waals (0.50), Ligand van der Waals (0.50), maximum number of poses (20). The default prime refinement setting was set to refine residues within 5.0 Å of ligand poses and to optimize side chains. For glide redocking, the settings were set to redock into structures within 10.0 kcal/mol of the best structure and within the top 20 structures. The precision was set to standard protocol with a glide and prime CPUs of 2.
2.7. Molecular dynamics simulations
The initial step of the molecular dynamics simulations was to use the system builder function on the Desmond module of Schrodinger suite 2022-1 to solvate the energy minimized docked complex as previously described (Pooe et al., Citation2021). The TIP3P solvent parameter was used, and boundary conditions were described as a cubic box with a volume minimized to 10 Å. The force field was set to OPLS_2005, and the system was neutralized by adding Cl or Na ions based on the total charges and the salt concentration was 0.15 M. The MD simulations of the 3D structure of the PfGrp94 NTD and PfGrp94 NTD–ligand complexes were performed using the Desmond simulation package of the Schrödinger Maestro on a computer with Linux server containing the 2019 GPU enabled Schrodinger Desmond version. In performing molecular dynamics simulation, a GPU enabled molecular dynamics simulation engine implemented in Maestro v12.2 was used on a Linux (Ubuntu) desktop server for Desmond molecular dynamics simulation studies. The ensemble class was set to NPT with bar pressure of 1 bar and a temperature of 300 K in all runs. The length of the simulation was set to 250 ns, and the model system was set to relax before simulation using OPLS_2005 force field. The simulations were repeated as three independent experiments, and the post-dynamic analysis of the behaviour and the type of bonds for the interaction between the protein and ligand was analysed using the Simulation Interaction Diagram tool available in the Desmond MD package. The MD simulation stability was monitored by the protein–ligand Root mean square deviation (RMSD), protein–ligand Root mean square fluctuation (RMSF), Radius gyration, protein–ligand properties, and trajectories of ligand torsion profile.
2.8. Prediction of the pharmacological and ADME/T properties
The absorption distribution metabolism excretion and toxicity (ADME/T) properties of the compounds were predicted using the Schrodinger QikProp module as previously described (Ahamad et al., Citation2023). Briefly, the intestinal absorption, and skin permeability levels were predicted based on model cells. The distribution properties of drugs in the blood − brain barrier (log BB) and central nervous system (CNS) permeability profiles were also computed. The metabolism of the compounds was predicted using parameters for cytochrome models. Subsequent excretion and toxicity propensity of the compounds were also predicted based on hERG inhibition, hepatotoxicity, and skin sensitization. The Lipinski rule descriptors such as the molecular weight, dipole moment, SASA, hydrogen bond donor/acceptor traits were predicted. These parameters provided the predictive pharmacokinetic and pharmacodynamic properties of the best compounds.
3. Results and discussion
3.1. Protein structure validation
The accuracy and quality of the refined Hsp90 NTD 3D structures were validated using the Ramachandran plot analysis (). The 3D structures of the Hsp90 NTDs were observed to be generally composed of 7 beta sheets and 5 alpha helixes across the different proteins (). The sum of the percentage residues in the most favourable region, allowable region the Hsp90 NTDs (Table S1).
Figure 1. Three-dimensional structure validation of P. falciparum and human Hsp90 NTD. The refined 3D NTD structures of the (A) PfGrp94, (B) HsHsp90α, (C) Hsp90 β, (D)HsGrp94, (E) HsTRAP-1 and their respective Ramachandran plot analysis of the Psi and Phi angles. The 3D structures were visualised using ChimeraX v1.5.
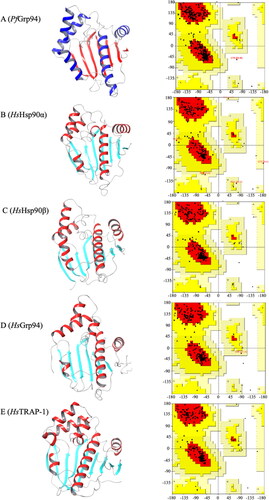
3.2. Small molecule compound screening
The initial screen of small molecule compounds in the ZINC database (a repository of over 1.6 billion compounds) identified 900 compounds that bound to NTD-Hsp90 proteins were retrieved (). The retained compounds were subsequently docked onto NTD-PfGrp94 using on BioSolveIT SeeSAR to identify the top 20 compounds with the highest affinity for PfGrp94 and lowest affinity against each of the four human Hsp90 paralog proteins (HsHsp90α, HsHsp90β, HsGrp94 and HsTRAP1). The top hit (from compounds with a high affinity for PfGrp94) from compounds showing high selectivity was Compound 653 (4‐{5‐[(Z)‐[(isopropoxymethyl)imino]methyl]‐1H,3H,6H‐pyrrolo[3,4‐c]pyridine‐2‐carbonyl}‐6‐isopropylbenzene‐1,3‐diol). Compound 653 was further refined by the incremental construction algorithm on SeeSAR Inspiron to generate 2 compounds, Compound Z (4‐{5‐[(Z)‐{[(cyclopent‐2‐en‐1‐yl)methyl]imino}methyl]‐1H,2H,3H,5H,6H‐ pyrrolo[3,4‐c]pyridine‐2‐carbonyl}‐6‐(propan‐2‐yl)benzene‐1,3‐diol) and Compound S ((9S)‐12‐{2‐[2,4‐dihydroxy‐5‐(propan‐2‐yl)benzoyl]‐1H,2H,3H,5H,6H‐pyrrolo[3,4‐c]pyridin‐5‐yl}‐9‐(propan‐2‐yl)‐7lambda5,11lambda5‐diazatricyclo[9.4.0.02,7]pentadeca‐1(15),2,4,6,11,13‐hexaene‐7,11‐bis(ylium)).
Figure 2. Schematic representation of the screening workflow of ATP-mimicking compounds targeting PfGrp94. The ZINC data base was probed for Hsp90 NTD-binding compounds and screened for selectivity towards PfGrp94 binding using BioSolveIT software. The red box for Compound S and Compound Z indicates the structural changes (compared to Compound 653) that was made to increase selectivity to PfGrp94.
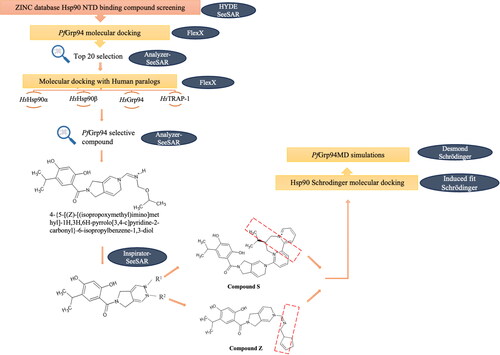
3.3. Molecular docking
The binding energy that stabilized the interactions of ATP, Compound S and Compound Z towards PfGrp94 and its human Hsp90 homologs, were predicted by molecular docking studies. Compound Z showed interaction with amino acid residues Asp 148 and Asn106 on the NTD-PfGrp94 through hydrogen bonded interactions (, ). Several other amino acid residues within 4 Å interacted with Compound Z through hydrophobic interaction which stabilized the docked complex. Based on the docking scores, the PfGrp94-Compound Z complex had the lowest docking value of −7.07 kcal/mol compared to the human homologs (). This indicates that Compound Z is predicted to bind with high affinity to the parasite Grp94 and low affinity to the human homologs. Furthermore, the binding affinities for Compound Z and ATP are within the same order of magnitude with a difference of −0.8 kcal/mol. This suggests that Compound Z is a potential inhibitor competitive towards ATP-binding, and which is selective to PfGrp94. The lower docking scores of Compound Z against human Hsp90 homologs are suggestive of minimal cross reactivity.
Figure 3. Schematic diagrams of Hsp90 NTDs docked with Compound S and Z. (A) represents PfGrp94 in complex with Compound Z and (B) represents PfGrp94 in complex with Compound S. The surface display of the binding cavity showing the orientation of the compounds when bound to the PfGrp94, a zoomed in 3D representation of the compounds interacting with PfGrp94 and a 2D representation of the bond types and interacting residues. The cut-off was set at 4 Å for the best docked stabilizing pose of the complex. The predicted docking poses were generated using Schrödinger Maestro 2022.1.
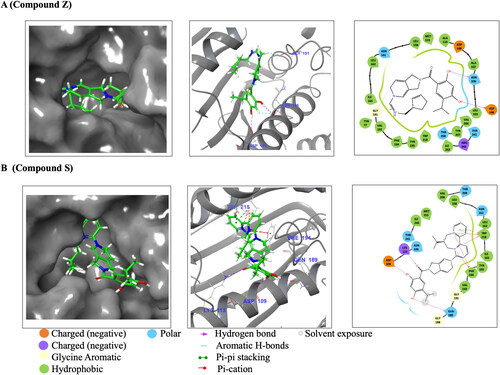
Table 1. Molecular docking analysis of PfGrp94 interacting residues with compounds within 4 Å.
Table 2. Docking scores for Hsp90 NTD homologues in complex with ATP, Compound S and Compound Z.
Similarly, the docking scores also predict that Compound S will selectively bind with more affinity to the PfGrp94 over the human homologues (). Docking results showed that Compound S interacted with residues Lys 113, Asp 109 and Gln 189 through hydrogen bonds, while Trp 218 was involved in a π–π stacking interaction (). The PfGrp94 residues Phe 128 and Trp 218 were involved in Pi-cations interaction with Compound S (). A few amino acid residues interacted with Compound S through hydrophobic interactions (). The comparison between the binding affinities show that PfGrp94 has a higher binding affinity for ATP than Compound S. Despite coming from the same scaffold structure as Compound Z, Compound S does not show big differences in its determined docking scores for binding to the parasite and human Hsp90 NTD binding. Compound S is a potential candidate based on its selective properties but not as competitive as Compound Z.
3.4. ADME/T analysis
The comparative ADMET analysis of Compound S and Compound Z were conducted using QikProp module of the Schrodinger software. Although, both compound Z and compound S molecular weight were withing the allowable reference range (300–700 Da), Compound S violated the Lipniski Rule of 5 as its molecular weight was larger than 500 Da (). The human oral absorption for both compounds were high and all the other parameters were within the acceptable ranges. However, the predicted IC50 value for blockage of HERG K+ channels were a concern for both compounds and Compound S had reduced aqueous solubility at −8.4 mol/dm3. Most of the predicted ADME/T properties of Compound Z were within the reference ranges and more favourable than for Compound S.
Table 3. Comparative ADME/T properties of the compounds S and Compound Z.
3.5. Molecular dynamics simulations
While molecular docking provides a static fixed view of the binding poses of the ligand in the protein active site, molecular dynamics (MD) simulation integrates the Newton’s equation of motion to compute the movement of atoms with respect to time (Adcock and McCammon, Citation2006). In MD, the binding stability of the ligand–protein complex and the dynamic behaviour is further examined through simulating the molecular system over a stipulated time. Therefore, the PfGrp94-Apo was initially simulated followed by the PfGrp94-compound complexes to assess the stability of PfGrp94 upon ligand binding. Using the simulation quality analysis algorithm in Desmond, it was observed that there were no changes in temperature, energy, pressure, and volume during the simulation period ( and Figure S4). This indicates that all the simulated systems were equilibrated correctly, as a validation step for the results.
Table 4. Simulation quality analysis of the three systems simulated over a simulation period of 250 ns.
The dynamic behaviour of protein and protein-ligand complexes were studied by comparing the values of Rg, RMSD, RMSF and the type of protein-ligand intermolecular interactions during the 250 ns simulation period (). Rg indicates the folding rate (Lobanov et al., Citation2008) and measures the level of compactness of the polypeptide during simulation (Munjal et al., Citation2019). Consequently, a higher Rg value means that the protein structure is less compact, while a low Rg value means that the protein structure is compact (Munjal et al., Citation2019). The mean Rg (±SD) of Cα of PfGrp94- Apo was 17.44 ± 0.10 Å, PfGrp94-Compound Z was 17.40 ± 0.09 Å and PfGrp94-Compound S was 17.23 ± 0.18 Å over a simulation period of 250 ns. The Rg profile shows that the PfGrp94-Apo and PfGrp94-Compound Z complex are comparable because there was no significant deviation from the mean Rg, whereas the PfGrp94-Apo and PfGrp94-Compound S showed a significant deviation (). Since the profile of PfGrp94-Apo and PfGrp94-Compound Z are similar, this suggest that when Compound Z is in complex with PfGrp94, the degree of conformational change is minimal resulting in a less compact structure during the simulation period. Compound S causes significant conformational changes to PfGrp94 structure resulting in a more compact structure. According to Tran et al. (Citation2022), if a protein becomes more compact, it suggests that the drug molecule has not caused a significant interference with the protein’s folding mechanism (Tran et al., Citation2022). Therefore, the results suggest that the binding of Compound S does not significantly affect the structure of PfGrp94, in contrast to Compound Z, which does.
Figure 4. RMSD, RMSF and Radius of Gyration plot during MD simulation. (A)The Rg of Cα atoms (B) The RMSD plot of PfGrp94 Cα atoms and over a simulation period of 250 ns (C) NTD-PfGrp94 RMSF of apo and in complex with Compound S or Compound Z (D) The Ligand RMSD graph showing the stability of the compounds with respect to the PfGrp94-binding site (E) Represents the evolution of the compounds during the simulation in a superimposed image. The initial frames are coloured deep shade of blue and the final frame are coloured deep shade of red. Furthermore, a schematic of detailed ligand atom interactions with the protein residues that occur more than 30% of the simulation period within the active site. The plots were sketched using GraphPad prism v10.0.2 and images were generated from Maestro v13.1.
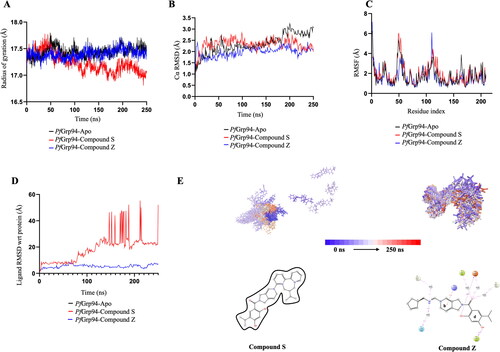
The RMSD of the protein gives comprehension of the protein conformational dynamics of the simulation run. The Cα atom RMSD was monitored for the three systems simulated over 250 ns (). The mean RMSD (±SD) of Cα of PfGrp94-Apo was 2.73 ± 0.35 Å, PfGrp94-Compound Z was 2.34 ± 0,36 Å and the PfGrp94-Compound S was 2.47 ± 0.30 Å. None of the systems flatulated above 3 Å, which can further confirm the results from the simulation quality analysis. Therefore, the generated data are fit for downstream evaluation. When a ligand binds to a protein, the mobility of the structure is expected to decrease due the ligand’s interactions with the binding cavity. This would suggest that the apo form of the protein would have the highest conformational dynamics. The two complexes formed between PfGrp94-Compound Z and PfGrp94-Compound S showed RMSD values less than when each of them is compared to PfGrp94-Apo individually. Since the RMSD of Compound Z complex is lower than that of Compound S, Compound Z complex has less conformational change compared to the Compound S complex. Subsequently the dynamics of the PfGrp94 is restricted, and this may impact the hydrolytic activity of the PfGrp94.
The RMSF analysis of the side chains of PfGrp94 NTD upon ligand binding during the MD simulation reveal information on protein residue flexibility (). A higher RMSF value indicate higher degree of movement whilst a low RMSF indicates a stable structure with minimal movement.(Tran et al., Citation2022) The RMSF profiles of both the apo-protein and the complexes, calculated based on the residues index Cα, overlap with that of the apo-protein (). PfGrp94 fluctuates strongly mainly in two regions: residues 44–55, which belongs to the flexible looped region between the second and third helix and residues 110–120, which belongs to the fourth helix of PfGrp94 NTD (Figure S5). Compound Z does not bind to these fluctuating regions while Compound S binds to the fourth helix PfGrp94 which strongly fluctuates. Therefore, the results indicate that the binding of Compound S does not significantly affect the structure of PfGrp94 while Compound Z does.
Ligand RMSD indicates how stable the ligand is with respect to the protein-binding pocket. Moreover, when a molecule fluctuates away from the protein site, it can result in the loss of binding and a decrease in the stability of the complex (Tran et al., Citation2022). It was observed that the degree of motion for Compound Z was more stable when compared to Compound S (). Compound S was stable only for the first 80 ns and diffused away from the binding site while Compound Z was stable throughout the 250 ns simulation period (). The ligand snapshots throughout the 250 ns simulation further show that Compound S was unstable while Compound Z snapshots indicate that the compound more or less remained in the same position, indicating stability (Supplementary Videos and Figure S8).
The types of protein–ligand intermolecular interactions were analysed throughout the MD simulation for PfGrp94 and the compounds (). The bonds present in the target ligand-protein interaction include hydrogen interactions, hydrophobic contacts, ionic interactions, and water bridges. These different types of bonds contribute to the overall stability and specificity of the ligand–protein complex. The highest interaction fraction of Compound Z was 1.55, indicating a higher number of interactions with residues. On the other hand, Compound S had an interaction fraction of 0.693, suggesting a lower number of interactions with residues. Furthermore, compared to Compound S, Compound Z had dominant hydrogen bond interaction which is the strongest bond and plays a significant role in forming strong and stabilized protein-ligand complexes. Water bridged interactions between Compound Z were also dominant as compared to Compound S. This depicts that during simulation time, Compound Z had more and stronger interactions with residues from PfGrp94 when compared to Compound S (Figure S6).
Figure 5. Analysis of the type and number of contacts between the compounds and PfGrp94. (A) compound S and (B) compound Z as monitored throughout the simulation. The images were generated from the simulation interaction diagram algorithm in Maestro v13.1 (C) Comparison of the human (HsHsp90α, HsHsp90β, HsGrp94, and HsTRAP-1) and plasmodial Hsp90 (PfGrp94) NTD sequence alignment. Conserved residues are shaded in black and similar residues are shaded grey and non-conserved regions are in white. The NTD region of PfGrp94 is highlighted with the red box. The picture was generated by BioEdit v7.2.5.
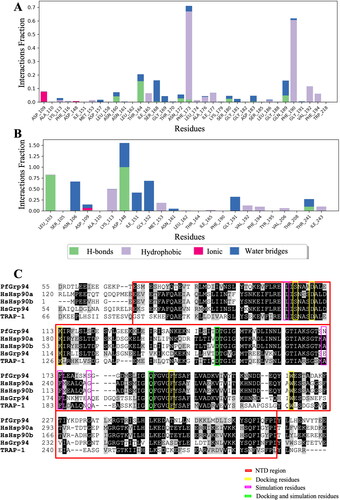
The analysis of the residues that interacted with ligands within 4 Å for more than 30% of the simulation time between compound and protein complex, show the stability of the formed structure. Monitoring the PfGrp94-Compound S complex, there were no stabilizing H bond interactions between the Compound S and PfGrp94 for more than 30% of the simulation time (). This shows that Compound S is incapable of forming the appropriate stable interactions with PfGrp94. On the other hand, on the PfGrp94-Compound Z complex, the hydroxyl groups of Asp148 and Leu 103 formed hydrogen bond interaction with the pyridine ring (ring d) of Compound Z for 99% and 82% of the simulation period respectively (). Residues Asn 106, Gly 191, Gly 152, Asp148 and Ile 151 formed water bridges for 64%–32% of the simulation period. The Compound Z ring b formed π–cation interaction with Lys 113 for 49% of the simulation period. The interaction observed between PfGrp94 and Compound Z was stronger than the PfGrp94-Compound S complex. This further validates binding affinities observed in docking studies suggesting that Compound Z exhibits a higher binding affinity for the PfGrp94-binding pocket than Compound S. Moreover, the changes that occurred in the ligand atom positions during the simulation of the carbon atoms of Compound Z connecting ring a and ring b, registered increased fluctuation with ring a having the highest fluctuation (Figure S7). The RMSF values of the carbon atoms of Compound Z were observed within the range of 0.67 and 4.05 Å while Compound S has RMSF between 11 Å and 15 Å. This shows that Compound S had a negative entropic role in the binding event. Furthermore, it suggests that when designing potential ligands, the changes that occur after carbon 19 affect the fluctuation of the ligand which subsequently affects the binding stability of the ligand to PfGrp94.
3.6. Analysis of the conservation level of compound binding residues on PfGrp94
After the docking and MD simulations, we wanted to determine the conservation level of the compound-binding region among the Hsp90 NTD homologs. A multiple sequence alignment was conducted for PfGrp94 and its human homologs sequences (). Generally, it was observed that the NTD of PfGrp94 and the human homologs exhibits a high sequence conservation. Comparison of the residues that interacted with Compound S/Z in docking studies showed that all the regions from the docking studies were 100% conserved across the Hsp90s. However, the residues that interacted with compounds in MD simulation were 67% conserved when compared to the regions from those conserved in docking studies. This leaves an additional 33% of the non-conserved regions in docking studies which the compounds bound to only during MD simulations. Since MD simulations give a clearer idea of the fate of biological molecules with respect to time, it explains the difference in the interaction results obtained. This suggest that the neighbouring amino acids may influence the binding of ligand to the protein based on how the protein is folded; this effect become more apparent in MD simulation than in docking studies. Furthermore, despite the sequence alignment results showing high conservation on the docking residues, it fails to explain the different docking scores between PfGrp94 and its human counterparts.
4. Conclusion
As an essential protein, the PfGrp94 chaperone ensures the correct folding of parasite-proteins destined for export in the ER, thereby facilitating malarial parasite survival. It is plausible that inhibition of PfGrp94 ATPase activity would have downstream effects. Abrogating the chaperone function could result in client protein misfolding and failure to achieve a functional native conformation. Most previous drug screening efforts have been focused on the cytosolic members of the Hsp90 family, while this study provides evidence for selective targeting of ER resident Hsp90 member in the parasite. Furthermore, the paralog-selective inhibitors identified in this study opens new avenues for drug development using the two hit molecules (Compound S and Compound Z) for scaffolding drug modifications to improve the drug like indices. The findings from this study contributes in efforts to identify novel drug targets in the fight against the drug resistance development of the malaria parasites.
Limitations of study
While in silico studies provide predictive data to assist in screening and optimisation of drugs, they cannot predict real life biological activity of the compounds. Hence, caution should be excised in interpreting the in silico findings and translating them in a biological system.
Authors contributions
MLS: conducted some of the experiments, interpreted the data. FLM: conducted some of the experiments, interpreted the data, wrote the manuscript, IK: analysed and interpreted the data. ES: analysed and interpreted the data. TZ: conceptualised the study, interpreted data, wrote the manuscript, supervised, and implemented the study.
Supplemental Material
Download PDF (5.9 MB)Acknowledgements
We thank the South African Council for Scientific and Industrial Research (CSIR) centre for high performance computing (CHPC) for Schrodinger suite and the BioSolveIT Software company in Sankt Augustin, Germany for SeeSAR software access.
Disclosure statement
No potential conflict of interest was reported by the author(s).
Data availability statement
All data presented in the current study are contained within the manuscript/Supplementary Materials and accessible from the corresponding author including simulation videos upon request.
Additional information
Funding
References
- Adcock, S. A., & McCammon, J. A. (2006). Molecular dynamics: Survey of methods for simulating the activity of proteins. Chemical Reviews, 106(5), 1589–1615. https://doi.org/10.1021/CR040426M
- Ahamad, S., Hema, K., & Gupta, D. (2023). Identification of novel tau-tubulin kinase 2 inhibitors using computational approaches. ACS Omega, 8(14), 13026–13037. https://doi.org/10.1021/acsomega.3c00225
- Baviskar, S. N., & Shields, M. S. (2010). RNAi silenced Dd-grp94 (Dictyostelium discoideum glucose-regulated protein 94 kDa) cell lines in Dictyostelium exhibit marked reduction in growth rate and delay in development. Gene Expression, 15(2), 75–87. https://doi.org/10.3727/105221611X12973615737587
- Bhattacharjee, S., Coppens, I., Mbengue, A., Suresh, N., Ghorbal, M., Slouka, Z., Safeukui, I., Tang, H.-Y., Speicher, D. W., Stahelin, R. V., Mohandas, N., & Haldar, K. (2018). Remodeling of the malaria parasite and host human red cell by vesicle amplification that induces artemisinin resistance. Blood, 131(11), 1234–1247. https://doi.org/10.1182/blood-2017-11-814665
- Chaubey, S., Grover, M., & Tatu, U. (2014). Endoplasmic reticulum stress triggers gametocytogenesis in the malaria parasite. The Journal of Biological Chemistry, 289(24), 16662–16674. https://doi.org/10.1074/JBC.M114.551549
- Daniyan, M. O., Przyborski, J. M., & Shonhai, A. (2019). Partners in mischief: Functional networks of heat shock proteins of Plasmodium falciparum and their influence on parasite virulence. Biomolecules, 9(7), 295. https://doi.org/10.3390/BIOM9070295
- Descoteaux, A., Avila, H. A., Zhang, K., Turco, S. J., & Beverley, S. M. (2002). Leishmania LPG3 encodes a GRP94 homolog required for phosphoglycan synthesis implicated in parasite virulence but not viability. The EMBO Journal, 21(17), 4458–4469. https://doi.org/10.1093/emboj/cdf447
- Duan, X., Iwanowycz, S., Ngoi, S., Hill, M., Zhao, Q., & Liu, B. (2021). Molecular chaperone GRP94/GP96 in cancers: Oncogenesis and therapeutic target. Frontiers in Oncology, 11, 629846. https://doi.org/10.3389/FONC.2021.629846/BIBTEX
- Duerfeldt, A. S., Peterson, L. B., Maynard, J. C., Ng, C. L., Eletto, D., Ostrovsky, O., Shinogle, H. E., Moore, D. S., Argon, Y., Nicchitta, C. V., & Blagg, B. S. J. (2012). Development of a Grp94 inhibitor. Journal of the American Chemical Society, 134(23), 9796–9804. https://doi.org/10.1021/JA303477G/SUPPL_FILE/JA303477G_SI_001.PDF
- Easton, D. P., Kaneko, Y., & Subjeck, J. R. (2000). The Hsp110 and Grp170 stress proteins: Newly recognized relatives of the Hsp70s. Cell Stress & Chaperones, 5(4), 276. https://doi.org/10.1379/1466-1268(2000)005<0276:Thagsp>2.0.co;2
- Eletto, D., Dersh, D., & Argon, Y. (2010). GRP94 in ER quality control and stress responses. Seminars in Cell & Developmental Biology, 21(5), 479–485. https://doi.org/10.1016/J.SEMCDB.2010.03.004
- Hessling, M., Richter, K., & Buchner, J. (2009). Dissection of the ATP-induced conformational cycle of the molecular chaperone Hsp90. Nature Structural & Molecular Biology, 16(3), 287–293. https://doi.org/10.1038/nsmb.1565
- Hetz, C., Zhang, K., & Kaufman, R. J. (2020). Mechanism, regulation and functions of the unfolded protein response. Nature Reviews Molecular Cell Biology, 21(8), 421–438. https://doi.org/10.1038/s41580-020-0250-z
- Irwin, J. J., Tang, K. G., Young, J., Dandarchuluun, C., Wong, B. R., Khurelbaatar, M., Moroz, Y. S., Mayfield, J., & Sayle, R. A. (2020). ZINC20-A free ultralarge-scale chemical database for ligand discovery. Journal of Chemical Information and Modeling, 60(12), 6065–6073. https://doi.org/10.1021/ACS.JCIM.0C00675
- Jonsdottir, T. K., Gabriela, M., Crabb, B. S., de Koning-Ward, T. F., & Gilson, P. R. (2021). Defining the essential exportome of the malaria parasite. Trends in Parasitology, 37(7), 664–675. https://doi.org/10.1016/j.pt.2021.04.009
- Li, L., Wang, L., You, Q.-D., & Xu, X.-L. (2020). Heat shock protein 90 inhibitors: An update on achievements, challenges, and future directions. Journal of Medicinal Chemistry, 63(5), 1798–1822. https://doi.org/10.1021/ACS.JMEDCHEM.9B00940
- Lobanov, M. Y., Bogatyreva, N. S., & Galzitskaya, O. V. (2008). Radius of gyration as an indicator of protein structure compactness. Molecular Biology, 42(4), 623–628. https://doi.org/10.1134/S0026893308040195/METRICS
- Mbengue, A., Bhattacharjee, S., Pandharkar, T., Liu, H., Estiu, G., Stahelin, R. V., Rizk, S. S., Njimoh, D. L., Ryan, Y., Chotivanich, K., Nguon, C., Ghorbal, M., Lopez-Rubio, J.-J., Pfrender, M., Emrich, S., Mohandas, N., Dondorp, A. M., Wiest, O., & Haldar, K. (2015). A molecular mechanism of artemisinin resistance in Plasmodium falciparum malaria. Nature, 520(7549), 683–687. https://doi.org/10.1038/nature14412
- McCaffrey, K., & Braakman, I. (2016). Protein quality control at the endoplasmic reticulum. Essays in Biochemistry, 60(2), 227–235. https://doi.org/10.1042/EBC20160003
- Mok, S., Ashley, E. A., Ferreira, P. E., Zhu, L., Lin, Z., Yeo, T., Chotivanich, K., Imwong, M., Pukrittayakamee, S., Dhorda, M., Nguon, C., Lim, P., Amaratunga, C., Suon, S., Hien, T. T., Htut, Y., Faiz, M. A., Onyamboko, M. A., Mayxay, M., … Bozdech, Z. (2015). Drug resistance. Population transcriptomics of human malaria parasites reveals the mechanism of artemisinin resistance. Science (New York, N.Y.), 347(6220), 431–435. https://doi.org/10.1126/SCIENCE.1260403
- Munjal, N. S., Shukla, R., & Singh, T. R. (2019). Chemometric approach to estimate kinetic properties of paclitaxel prodrugs and their substructures for solubility prediction through molecular modelling and simulation studies. Journal of Chemometrics, 33(11), 3181. https://doi.org/10.1002/cem.3181
- Pooe, K., Worth, R., Iwuchukwu, E. A., Dirr, H. W., & Achilonu, I. (2021). An empirical and theoretical description of Schistosoma japonicum glutathione transferase inhibition by bromosulfophthalein and indanyloxyacetic acid 94. Journal of Molecular Structure, 1223, 128892. https://doi.org/10.1016/j.molstruc.2020.128892
- Pugh, K. W., Alnaed, M., Brackett, C. M., & Blagg, B. S. J. (2022). The biology and inhibition of glucose-regulated protein 94/gp96. Medicinal Research Reviews, 42(6), 2007–2024. https://doi.org/10.1002/MED.21915
- Rarey, M., Kramer, B., Lengauer, T., & Klebe, G. (1996). A fast flexible docking method using an incremental construction algorithm. Journal of Molecular Biology, 261(3), 470–489. https://doi.org/10.1006/JMBI.1996.0477
- Rocamora, F., Zhu, L., Liong, K. Y., Dondorp, A., Miotto, O., Mok, S., & Bozdech, Z. (2018). Oxidative stress and protein damage responses mediate artemisinin resistance in malaria parasites. PLoS Pathogens, 14(3), e1006930. https://doi.org/10.1371/JOURNAL.PPAT.1006930
- Roe, S. M., Prodromou, C., O'Brien, R., Ladbury, J. E., Piper, P. W., & Pearl, L. H. (1999). Structural basis for inhibition of the Hsp90 molecular chaperone by the antitumor antibiotics radicicol and geldanamycin. Journal of Medicinal Chemistry, 42(2), 260–266. https://doi.org/10.1021/JM980403Y
- Stofberg, M. L., Caillet, C., de Villiers, M., & Zininga, T. (2021). Inhibitors of the plasmodium falciparum hsp90 towards selective antimalarial drug design: The past, present and future. Cells, 10(11), 2849. https://doi.org/10.3390/CELLS10112849/S1
- Suresh, N., & Haldar, K. (2018). Mechanisms of artemisinin resistance in Plasmodium falciparum malaria. Current Opinion in Pharmacology, 42, 46–54. https://doi.org/10.1016/j.coph.2018.06.003
- Taipale, M., Krykbaeva, I., Koeva, M., Kayatekin, C., Westover, K. D., Karras, G. I., & Lindquist, S. (2012). Quantitative analysis of HSP90-client interactions reveals principles of substrate recognition. Cell, 150(5), 987–1001. https://doi.org/10.1016/j.cell.2012.06.047
- Tilley, L., Straimer, J., Gnädig, N. F., Ralph, S. A., & Fidock, D. A. (2016). Artemisinin action and resistance in Plasmodium falciparum. Trends in Parasitology, 32(9), 682–696. https://doi.org/10.1016/j.pt.2016.05.010
- Tran, Q. H., et al. (2022). Identification of small molecules as potential inhibitors of interleukin 6: A multi-computational investigation. Molecular Diversity, 1, 1–16. https://doi.org/10.1007/S11030-022-10558-7/FIGURES/9
- Zaib, S., Rana, N., Hussain, N., Ogaly, H. A., Dera, A. A., & Khan, I. (2023). Identification of potential inhibitors for the treatment of Alkaptonuria using an integrated in silico computational strategy. Molecules (Basel, Switzerland), 28(6), 2623. https://doi.org/10.3390/MOLECULES28062623/S1
- Zhang, M., Gallego-Delgado, J., Fernandez-Arias, C., Waters, N. C., Rodriguez, A., Tsuji, M., Wek, R. C., Nussenzweig, V., & Sullivan, W. J. (2017). Inhibiting the Plasmodium eIF2α kinase PK4 prevents artemisinin-induced latency. Cell Host & Microbe, 22(6), 766–776.e4. https://doi.org/10.1016/J.CHOM.2017.11.005