Abstract
Delivery of RNA into cells using lipid nanoparticles (LNPs) has been a significant breakthrough in RNA-based medicine, with clinical applicability expanded through the use of ionizable lipids (ILs). These unique lipids can alter their charge state in response to pH changes, which is crucial for pH-triggered endosomal escape and effective lipid-mediated RNA delivery. In this study, we conducted a comprehensive set of molecular dynamics (MD) simulations to investigate interactions between IL-containing lipid nanodroplets (LNDs) and cell membrane models. Using an atomistic resolution model, we investigated the merging process of LNDs with cell membrane models under neutral conditions relevant to an intercellular environment and acidic pH conditions found in late endosomes. Our observations revealed that at neutral pH, LNDs merged with lipid membranes while preserving the bilayer structure. Under acidic conditions, the LNDs remained attached to the bilayer without fusing into the membranes. Importantly, the presence of ILs did not disrupt the original biomembrane structure during the simulation period. The MD simulations provided valuable atomistic insights into the mechanism of interaction between IL-containing nanodroplets and biomembranes, which could aid the rational design of ILs to develop more efficient LNPs for RNA therapies.
Communicated by Ramaswamy H. Sarma
Introduction
RNA-based therapy is an emerging medical technology that has already proved its potential in vaccinations, as well as treatments for cancer and rare diseases (Hou et al., Citation2021; Jayaraman et al., Citation2012; Wang et al., Citation2020). Safe delivery of therapeutic RNA to its cellular target initially posed a significant barrier which was recently overcome by the encapsulation of RNA into lipid nanoparticles (LNPs) (Patel et al., Citation2019; Shin et al., Citation2018). This capability facilitated the distribution of over a billion doses of mRNA vaccines during the COVID-19 pandemic (COVID-19 Vaccine Doses Administered by Manufacturer, Citationn.d.; Mathieu et al., Citation2021). However, despite significant progress in this field, many important questions remain unanswered. For example, fine details of the mechanism of cargo delivery and the nature of interactions of LNP lipids with cellular compartments, including biomembranes, have not yet been explored.
Use of ionizable lipids (ILs) represents a crucial breakthrough (Jayaraman et al., Citation2012; Semple et al., Citation2010) that has increased pharmaceutical applications of RNA-carrying LNPs. Initially, cationic lipids were considered due to their RNA encapsulation capability, but their practical use was hindered by their cytotoxicity (Kumari et al., Citation2020). Subsequently, they were replaced by ILs because the pH-dependent charge of the IL molecules decreases the LNP cytotoxicity (Cheng et al., Citation2022) whilst preserving the RNA encapsulation capacity. IL-based LNPs are assembled under acidic conditions, which favour electrostatic interactions between the positively charged (protonated) ILs and negatively charged RNA (Eygeris et al., Citation2022). When present in an intercellular medium, the system is neutralized and LNP ILs become uncharged (deprotonated). When uncharged LNPs encounter a cell membrane, they are encapsulated into endosomes (Patel et al., Citation2019). As endosome maturation progresses, the pH decreases and the ILs become reprotonated (Eygeris et al., Citation2022). The apparent pKa of a LNP mixture is a tuneable property, with the peak RNA delivery efficiency usually obtained at pKa 6-7 (Carrasco et al., Citation2021), resulting in an efficient response of LNPs to the pH change during endosome maturation and release of RNA into the cytosol (Eygeris et al., Citation2022).
Gaining atomistic insights into LNP structure and behaviour inside the human body is an important albeit very challenging task. Whereas experimental techniques have provided valuable information about the RNA delivery efficiency, assessment of the inner LNP structure remains an experimentally unexplored field and structural details of the interaction of LNPs with cellular membranes have yet to be investigated. So far, experimental techniques have provided a reliable description of the LNP size, which ranges from 50 to 300 nm in diameter, depending on the methods used for LNP preparation and size measurement (Malburet et al., Citation2022; Shepherd et al., Citation2023). Further, structural insights into LNP organization have been obtained from in vitro cryo-TEM experiments of either onion- (Kulkarni et al., Citation2018) or blebbed-phase (Brader et al., Citation2021) LNPs. Complementary to experiments, molecular dynamics (MD) simulations can provide unique insights into structural and dynamic features of very complex systems on a femtosecond timescale and with atomic resolution. MD simulations have been used to evaluate the lipid phases in COVID-19 vaccine mixtures (Paloncýová et al., Citation2021), structural organization of LNPs (Bruininks et al., Citation2020; Leung et al., Citation2012) and pH-dependent behaviour of LNPs (Paloncýová et al., Citation2023; Trollmann & Böckmann, Citation2022). Recently, simulations of lipid bilayers composed of increasing fractions of deprotonated ILs revealed formation of a separated IL phase at high IL concentration (Dehghani-Ghahnaviyeh et al., Citation2023). Whereas large models of LNPs with radii of dozens of nm focus on the overall arrangement, small systems of single nanometer scales can reveal the behaviour and function of individual molecules.
Small lipid bilayers can serve as good models for MD simulations of biomembranes and lipid-based systems, offering insights into the structure, mechanism and behaviour of these essential natural components. Precise representation of vital properties has been reported, such as interactions with small molecules (Paloncýová et al., Citation2014), peptides and proteins (Sandoval-Perez et al., Citation2017) and nanomaterials (Chen et al., Citation2016; Lazaratos et al., Citation2020). Moreover, previous studies of non-lamellar lipid phases (Marrink & Mark, Citation2004; Marrink & Tieleman, Citation2002; Ramezanpour et al., Citation2020) have provided representative lipid models with adequate and justifiable information even on limited size systems. Hence, lipid bilayer models can be used to unravel molecular mechanisms underlying critical biological processes that cannot be determined by traditional experimental techniques. In the present study, we used a lipid bilayer model to evaluate interactions of cell membrane and LNPs mimicking lipid mixtures in COVID-19 vaccines.
We carried out an extensive set of MD simulations to investigate interactions between LNP-mimicking lipid mixtures and mammalian cell membranes. As cell membranes are complex environments containing a variety of lipid species (Doktorova et al., Citation2020; Harayama & Riezman, Citation2018; Lorent et al., Citation2020), we explored a series of models of various phospholipid bilayers differing in lipid headgroup. To evaluate their interactions with ILs currently used in LNPs, we simulated the lipid bilayer merging with an uncharged or charged lipid nanodroplet (LND) mimicking the LNP lipid composition. We focused on describing the merging process and the effect of ILs and helper lipids (DSPC, CHL) in the LNP composition on the phospholipid bilayer structure and stability. We observed that neutral nanodroplets merged completely with membrane lipids and did not destabilize the membrane structure, whereas with the acidic form of ILs, the LND stayed connected to the lipid membrane but was not fully incorporated into it. These findings provide unique insights into the fate of ILs in an organism, which could aid further structural and functional optimalization of LNPs.
Methods
Composition of the systems
POPC (1-palmitoyl-2-oleoyl-sn-glycero-3-phosphocholine), POPE (1-palmitoyl-2-oleoyl-sn-glycero-3-phosphoethanolamine), POPG (1-palmitoyl-2-oleoyl-sn-glycero-3-phosphoglycerol) and POPS (1-palmitoyl-2-oleoyl-sn-glycero-3-phospho-L-serine) were used to build the membranes. The IL ALC-0315, [(4-hydroxybutyl)azanediyl]di(hexane-6,1-diyl) bis(2-hexyldecanoate), denoted A3D in the deprotonated form and A3P in the protonated form (Figure S1), and the helper lipids cholesterol (CHL) and 1,2-distearoyl-sn-glycero-3-phosphocholine (DSPC) were used to build the LNDs. Membranes of regular size were modelled as bilayers with 100 lipids in each leaflet, and LNDs comprised 100 lipids with a 50:40:10 molar ratio of IL:CHL:DSPC. To evaluate the effect of the size of the system, POPC lipid bilayers were also prepared with 200 or 300 POPC molecules per leaflet. The systems were neutralised and adjusted to the physiological concentration of 0.15 M by adding K+ and Cl− ions.
Simulation protocol
The lipid membranes were assembled, minimised and subjected to a 200 ns equilibration run. The LNDs were assembled, minimised and subjected to a 100 ns equilibration run. Afterwards, both systems were combined and hydrated (see run details below, ), then an unbiased “free” 500 ns simulation of the LND-membrane system was performed. As the unbiased simulation did not lead to a spontaneous merging, the same inputs were then subjected to a 100 ns pulling simulation, during which the centre of mass (CoM) of the LND was pulled towards the CoM of the membrane along the z-axis. The systems were then subjected to a 1000 ns (or 1500 ns in the case of a POPE membrane with an uncharged LND) unbiased production run (see for a schematic of the process). Each of the pulling + production simulations were performed in three replicas.
Software and force fields
All simulations were performed in the GROMACS 2018.1 software (Abraham et al., Citation2015; Berendsen et al., Citation1995; Hess et al., Citation2008; Lindahl et al., Citation2001; Páll et al., Citation2015; Pronk et al., Citation2013; Van Der Spoel et al., Citation2005). The forcefields used were Amber Lipid17 for the standard lipids (Gould et al., Citation2018), OPC (Izadi et al., Citation2014) for the water model and Joung − Cheatham (Joung & Cheatham, Citation2008) for the ions. The ILs used were parametrised in our previous work (Paloncýová et al., Citation2021) by combining parameters of Lipid17 (Gould et al., Citation2018) for the tails and GAFF (J. Wang et al., Citation2004) for the ionizable headgroup.
The pure membrane systems were assembled using the CHARMM-GUI (Jo et al., Citation2008; Lee et al., Citation2016) webpage (Jo et al., Citation2007, Citation2009; Lee et al., Citation2019, Citation2020; Wu et al., Citation2014), whereas the IL containing systems were assembled using an in-house bilayer builder, originally created by Gabin Fabre for (Paloncýová et al., Citation2014). The software used for analyses were as follows: GROMACS 2018.1 (for order parameter calculations), MDAnalysis 2.3.0 (Gowers et al., Citation2016; Michaud-Agrawal et al., Citation2011) (and its dependencies SciPy (Virtanen et al., Citation2020), NumPy (Harris et al., Citation2020) and Biopython (Chapman & Chang, Citation2000; Cock et al., Citation2009; Hamelryck & Manderick, Citation2003)) for density based and system-property based analyses, LiPyphilic 0.10.0 (Smith & Lorenz, Citation2021) (with freud (Ramasubramani et al., Citation2020)) for leaflet-based analyses and gromacs_LS v2016.3 (Torres-Sánchez et al., Citation2015, Citation2016; Vanegas et al., Citation2014; Vanegas & Arroyo, Citation2014) (with LAPACK (Anderson et al., Citation1999)) for lateral pressure calculations. Graphs were prepared using Matplotlib (Hunter, Citation2007). The Python-based analysis tools were run in Python 3.9.12 as standalone scripts or within the Jupyter Notebook (Beg et al., Citation2021). The visualisation software used were PyMol 2.5.5 (Schrödinger & LLC, Citation2015) and VMD 1.9.3 (Humphrey et al., Citation1996).
Simulation details
The pure membrane systems were subjected to steepest descent minimization and multi-step of ∼3 ns subsequent equilibration runs set at 310 K, in accordance with the procedure recommended by CHARMM-GUI. The systems were then subjected to a 200 ns production run.
The lipids used to build the LNDs were selected to mimic the composition of the Pfizer&BioNTech mRNA Comirnaty vaccine. Therefore, a bilayer of 50 ILs (ALC-0315), 40 CHLs and 10 DSPC molecules was created using our in-house script. We avoided using the PEGylated lipid ALC-0159 because its low content required including only two molecules per system, which would likely have generated simulation artefacts. The resulting bilayer was then subjected to the CHARMM-GUI equilibration process. Next, the LND was inserted into a larger box with at least 2 nm of solvent on all sides (corresponding to approximately 12 × 12 × 7 nm box size for the uncharged, and 10 × 10 × 8 nm for the charged system), re-equilibrated and simulated for 100 ns under isotropic conditions.
Each membrane and LND pair (after their respective 200 ns and 100 ns of simulation, ) were manually combined so that the LND lay above the membrane. The system was rehydrated and subjected to the same minimization and then a 50 ps simulation to settle the box. The systems were simulated for 500 ns without any bias. As the LNDs and membranes remained separate in these free simulations, the starting structures were subjected to 100 ns of pulling and 1000 ns of unrestrained simulation (350 ns for large POPC models, see the supplementary spreadsheet). The pulling was performed by applying a harmonic biasing force to decrease the distance along the z-axis by 0.05 nm per ns with a force constant of 10,000 kJ∙mol−1∙nm−2. As the lipid dynamics of the POPE systems with deprotonated IL was still ongoing 1000 ns post-pulling, this one system was prolonged to 1500 ns of free run. The simulations were performed with three replicas for each system for the regular size (100 lipids per leaflet) and two replicas for the larger POPC membrane models.
The simulation step was 2 fs and periodic boundary conditions were applied in all directions. The thermostat was set to 310 K according to Berendsen (Berendsen et al., Citation1984) for the equilibration and Nosé-Hoover (Hoover, Citation1985; Nosé & Klein, Citation1983) for the production runs with a temperature coupling constant of 1.0 ps. The pressure coupling was semi-isotropic (Nosé & Klein, Citation1983; Parrinello & Rahman, Citation1981) with a pressure coupling constant of 5.0 ps, pressure of 1 bar and compressibility of 4.5 × 10−5 bar−1, unless stated otherwise (see the supplementary Systems and Simulation Details spreadsheet). LINCS (Hess, Citation2008) restraints were applied to all H-bonds, and long-range electrostatics were calculated via the particle-mesh Ewald method (Essmann et al., Citation1995). All the simulation .mdp files are available in the supplementary dataset, and the full list of simulated systems, their abbreviations, amount of water/ion molecules and other simulation details can be found in the supplementary spreadsheet. Furthermore, all simulation protocols, starting/final structures, topologies, trajectories and scripts are available in the online Zenodo repository (doi: 10.5281/zenodo.10679359).
In this paper, the membrane side from which the LND was pulled (and to which the spike remained connected) will be called upper and the opposite one lower. The main parts of the systems are depicted in . Division of the system into four sections (above and below the membrane, lower and upper leaflet) was defined based on most populated position of the atoms of the membrane headgroups, averaged in 50 ns blocks (). The headgroups and tail groups used were defined by the Amber Lipid17 forcefield. Peaks were located using the find_peak function of the SciPy (version 1.9.3) Python package with height parameter set to 0.19 (g∙cm−3) and distance parameter set to 55 (histogram bins). Because the centre of membrane moved in the z-axis during the simulation, the centre of the membrane was defined as the average of the position of the two peaks, dynamically in 50 ns blocks. The lipids were assigned a position in the respective section based on the z-axis position of the CoM of each residue. As the ILs remained in the upper curve of the spike until its dissolution, the fraction of ILs above the membrane was used to describe the mixing process.
Figure 2. Outer and inner views of (A) deprotonated and (B) protonated LNDs (ILs in pink (deprotonated) and cyan (protonated), CHL in yellow, helper DSPC lipid headgroup in orange, tails in grey; (C) Structure of IL ALC-0315 (A3D) represented in uncharged (deprotonated) form; (D) Density profile of a starting structure of a deprotonated IL LND and POPC membrane, with mass densities of the headgroup shown in orange, tail in grey, CHL in yellow, IL in pink and water in light blue. The system was divided into four sections (above and below membrane, upper and lower leaflet) based on the position of the headgroup density peak; (E) Representation of parts of the system: LND (containing charged ILs) interacting with upper leaflet of a membrane forming a lipid spike connected to the membrane via the interface.
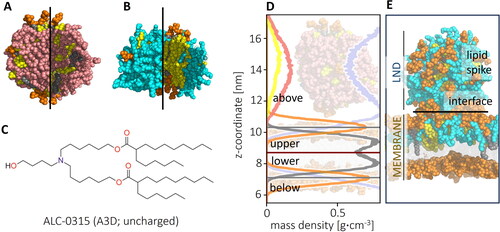
Lateral pressure profile calculations were performed on selected snapshots of the simulation by running a separate 1 ns simulation with 1 ps sampling and 2 nm cut-off treatment of electrostatics. 10,000 frames of this run were selected for analysis in 100 frame blocks, as recommended by the gromacs_LS manual. The pressure profiles were processed with a Gaussian filter with standard deviation of 0.2 nm and visualized in the z-direction.
For calculation of free energy profiles of the flip-flop process, POPC membranes were prepared with 36 lipids per leaflet. The membrane was equilibrated for 100 ns, then a single molecule of A3P, A3D or CHL was added at the top of the simulation box and another 100 ns free simulation was performed. From the position of the added molecule closest to the membrane centre, it was pulled at a rate of 0.01 nm∙ns−1 until it reached the centre of the membrane. Both unbiased and biased trajectories were used to extract starting frames for umbrella simulations separated by 0.1 nm, with an umbrella potential of 2000 kJ∙mol−1∙nm−1 between the CoM of the membrane and a lipid head group (nitrogen atom in A3P and A3D and oxygen in CHL). Free energy profiles were extracted by the weighted histogram analysis method (Hub et al., Citation2010; Kumar et al., Citation1992) from 100 ns umbrella simulations, taking the first 50 ns as an equilibration period.
Results
pH determined the shape of LNDs
To describe the behaviour of the IL-containing mixture in both the neutral intercellular medium and acidic endosomal environment, LND models were built for uncharged and charged forms of the IL (denoted A3D and A3P, respectively). The pKa of the IL was calculated as 10.0 (Eygeris et al., Citation2022), but the deprotonated form of the LND was considered as a model of the lipid mixture behaviour under neutral conditions (pH ∼7) as the apparent pKa of the whole LNP for an experimentally relevant scale was lower, that is, 6.1 (P. Patel et al., Citation2021). MD simulations (100 ns) of the lipid droplets revealed that they remained stable with diameters of ∼6 nm and ∼7.5 nm for deprotonated and protonated IL forms, respectively. The shape of the LNDs depended on the IL protonation state (). In the neutral form of the IL, the LND formed a sphere with IL distributed across the whole volume. In the charged form of the IL, relevant to the acidic endosomal environment, the LND was shaped like a nanodisc, with a CHL-DSPC-rich bilayer-like centre and the IL clustered close to the nanodisc edges, in accord with our previous observations (Paloncýová et al., Citation2021). In both cases, the DSPC molecules preferentially associated with CHL () and CHL molecules diffused between locally stable clusters via the disordered IL-rich phase.
Merging of LNDs with lipid bilayers required an external force
In unbiased 500-ns-long MD simulations, the LNDs did not spontaneously merge with the lipid bilayers (Figure S5) due to a kinetic barrier. Such a barrier preventing spontaneous lipid membrane merging has been described theoretically and observed in simulation (Bruininks et al., Citation2020; Leikin et al., Citation1987; Schneck et al., Citation2012), even though in large-scale experiments, merging would likely occur. However, the kinetic barrier can be overcome, for example, by dehydration of a contact region (Bruininks et al., Citation2020). In the present study, the CoM of the LND was pulled towards the CoM of the membrane for 100 ns. The pulling induced membrane deformation, which spontaneously relaxed within the first 50 ns of the following unbiased simulation (). After release of the biasing force, the LND slid partially out of the membrane due to a high lateral pressure (), forming a spike-like structure. In all cases, extensive lipid exchange between the spike and membrane occurred, as described later.
Figure 3. Time evolution of lateral pressure profiles of an uncharged (top) and charged (bottom) system with POPC lipid and respective simulation snapshots. Negative values in the pressure profiles correspond to attractive forces, whereas positive peaks correspond to repulsive regions. The time values indicate the time from the beginning of the pulling simulation. Therefore, 100 ns corresponds to the end of the pulling simulation, after which an unbiased simulation was run.
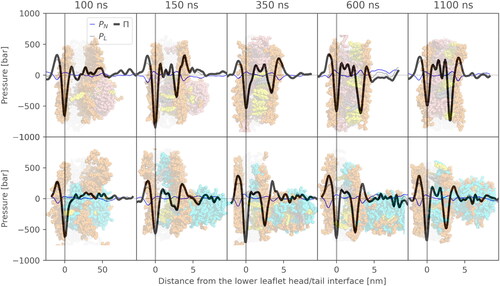
LNDs with neutral ILs dissolved in the lipid membrane
In the case of the uncharged form of the IL, the LND fully merged with the membrane’s bilayer lipids and the spike dissolved into the membrane. However, the dynamics of the process differed between the POPE bilayer and other systems. In the case of bilayers composed of POPC, POPG and POPS lipids, the spike merged with the membrane rapidly (). In the three simulations with deprotonated IL, the LND had dissolved in the bilayers by 350, 400 and 300 ns (median values for the three replicas) after pulling for the POPC, POPG and POPS membranes, respectively. The LND dissolution was accompanied by rapid flip-flop of the IL molecules to the lower membrane leaflet ( and ). Similar results were observed for the larger POPC membranes containing 200 or 300 POPC molecules per leaflet (Figure S7). In POPE, the IL separated into a disordered phase residing in the middle of the membrane, resulting in inverse curvature of the upper leaflet, as documented before for PE lipids (Kaasgaard & Drummond, Citation2006) (Figure S6d). This was due to the smaller volume of the POPE lipid headgroup making a conical molecular shape that favours an inversely curved surface (Ding et al., Citation2015; Kaasgaard & Drummond, Citation2006). After 1300 ns of post-pulling (median value, Table S2), the IL content above the POPE membrane had decreased to under 10%, together with rapid accumulation of the IL in the lower leaflet, analogously to other simulations.
Figure 4. Fraction of IL content above the membrane and in the lower leaflet during simulations averaged over three replicas. The solid lines correspond to systems with deprotonated IL, whereas the dotted lines correspond to the protonated IL. The grey vertical line indicates the end of pulling.
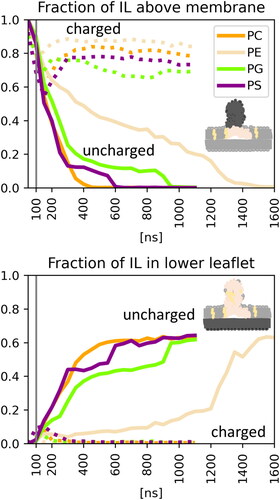
Figure 5. Ratio of different lipids above the membrane (upper panel) and within the two membrane leaflets (middle and lower panel) averaged over three replicas. ML (grey) denotes the membrane lipid content, CHL (yellow), A3D (pink) the deprotonated and A3P (cyan) the protonated form of the IL.
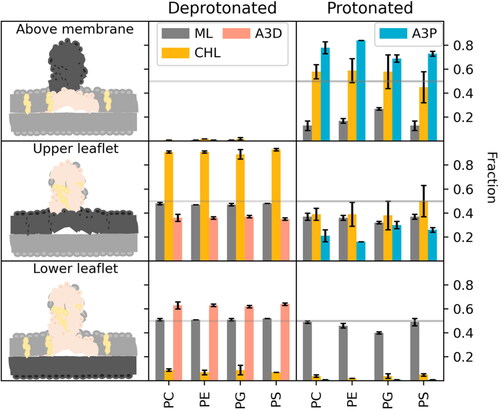
While the uncharged IL possess a very small, low polar head group allowing its facile flip-flop between leaflets, no helper lipids from the LND flipped into the opposite membrane leaflet (). A rare CHL flip-flop was observed with deprotonated ILs in at least one replica per system (Table S1). The flip-flop was facilitated by a highly disordered IL-rich phase created by IL accumulation within the membrane. To evaluate this phenomenon thermodynamically, we created a series of simulations with POPC membrane and a single lipid molecule (CHL, A3D, A3P) pulled inside the membrane. The extracted snapshots were used to calculate a free energy barrier for the flip-flop of ILs and CHL in a pure POPC membrane. While CHL had a 5.8 kcal/mol free energy barrier, A3D had a barrier of only 4.4 kcal/mol (Figure S8) and a further barrier decrease was expected in a disordered A3D-rich phase. Our simulations showed that the flip-flop of uncharged IL was more favourable than that of CHL and the system used it to alleviate the crowded upper membrane ().
The flip-flop mechanism of the uncharged IL makes advantage of the high flexibility of the molecule. In the first step (), the IL head stretched towards the bulk water, while the deprotonated nitrogen was located under the main lipid phosphate (1.1 nm from the middle of the membrane, Figure S3). Next, the whole molecule moved to the area between the leaflets (), where it remained for up to tens of nanoseconds. Afterwards, the tails oriented to the other leaflet (), with the head group still in the membrane core. The membrane core was enriched by polar oxygens located in the middle of IL tails, as also seen in the small symmetric membrane prepared separately (Figure S2). Finally, the headgroup flopped so that it reached the bulk solution on the opposing side of the membrane, with the deprotonated nitrogen remaining under the main lipid phosphates ().
Figure 6. Mechanism of diffusion of (A) uncharged IL (A3D) from the upper to lower leaflet, (B) charged IL (A3P) from an LND-induced spike to the membrane, (C) POPC from the membrane to the spike. The lipid of interest is shown by sticks, other molecules as balls. POPC headgroups are shown in orange, POPC tails in grey, charged IL in cyan/green, uncharged IL in light/bright pink, nitrogens of the lipid of interest in blue and oxygens of the lipid of interest in red. Numbers in the figures of the left column correspond to the position of ionizable nitrogen/POPC phosphorus atoms of the steps on the right.
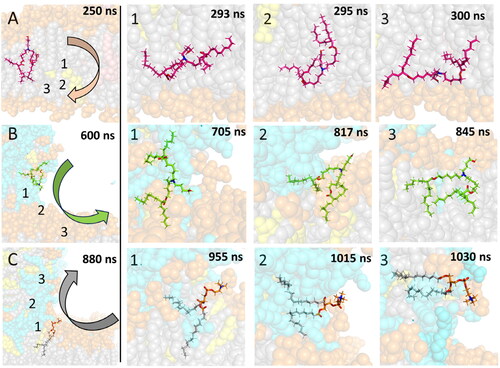
Due to the different abilities of the IL and CHL to flip-flop, the effect of the LND lipids on the membrane itself was leaflet-dependent. In the deprotonated systems, where the entire LND dissolved in the membrane, the upper leaflet took up (almost) all the CHL molecules (). This increased the deuterium order parameters of the membrane palmitoyl tails (Table S2) to the level of a corresponding POPX:CHL (65:35%) membrane (Table S3). Correspondingly, the area per lipid of the upper leaflet decreased by 15–20% (Tables S2 and S3). In contrast, the order parameters of the lower leaflet corresponded to a pure POPX membrane. The area per lipid of the lower leaflet was not significantly different from that of the pure membrane in the POPC system and was slightly higher in the other systems. Other leaflet properties, such as membrane thickness were not significantly affected by the presence of ILs (Table S2).
Mixing of deprotonated ILs with cell membrane phospholipids resulted in a bilayer that was consistent with current knowledge of IL behaviour. Previously, IL phase separation between membrane leaflets was observed for two-chained ILs DLin-KC2-DMA (Ramezanpour et al., Citation2019) and DLin-MC3-DMA (Ermilova & Swenson, Citation2020)). However, our systems contained branched ILs with only ∼16 mol% of IL in the final mixture, more akin to the very recent findings of Dehghani-Ghahnaviyeh et al. (Dehghani-Ghahnaviyeh et al., Citation2023). Under low concentrations (<20% of the IL), Lipid-5 (with one tail less than ALC-0315) was found to be localised both on the membrane surface and deeper in the tail and formed a separated phase only at high IL concentrations.
LND with charged ILs formed a spike connected to the lipid membrane
In the systems with the charged IL, relevant to the acidic environment in late endosomes, the lipid spike remained dynamically stable in all systems, with some differences in the inner spike conformations between systems with different headgroups. In the PC and PS systems, the spike retained a globular shape on the membrane, whereas in the PE and PG systems with smaller area per lipid, the spike connected across the periodic boundary conditions, forming a tube connected to the membrane via a highly disordered IL-rich phase (Figure S6). The conformation of the system allowed the dynamic exchange of lipids between the membrane and the spike. At least 10% of the IL and 20% of CHL diffused to the upper layer of the membrane, and oppositely at least 10% of the upper-layer membrane lipid diffused into the spike (). However, no flip-flop of the charged IL was observed in any of the systems, in contrast to the behaviour under neutral conditions. The flip-flop free energy barrier was estimated to be as high as 15.2 kcal/mol. Due to the absence of the IL flip-flop, the asymmetric lateral pressure could not be equilibrated between the lipid leaflets and equilibrium was reached via the presence of a lipid spike (). Even though the spike induced local deformation of the membrane surface, the overall structure remained intact.
The differences in the lipid mixing process in the neutral system stemmed from the increased hydrophilicity of charged nitrogen ILs. Concerning the mechanism of lipid mixing, two simultaneous processes were observed in the interfacial region: spontaneous translocation of ILs from the lipid spike to the bilayer and diffusion of membrane lipids in the opposite direction. Lipid translocation occurred after diffusion of the ionizable and membrane lipids towards the interfacial region (). The lipids adopted an extended conformation where their tails spread between the lipid bilayer and lipid spike at their interface ( and ), connecting both parts of the system. Subsequently, the remaining lipid tails from both lipids followed the first one ( and ), completing the translocation process. As a result, the protonated nitrogen of the IL resided on the level of the membrane lipid phosphates within the bilayer, whereas membrane lipid accumulated alongside CHL, forming a partially ordered conformation in the lipid spike.
The MD simulations provided an insight into the dynamics of interactions between IL-containing mixtures and lipid biomembrane models, along with an explanation of the mixing process. Furthermore, they revealed that flip-flop of IL molecules may underlie the mixing during their mutual interaction. Our models of LNDs with neutral ILs demonstrated that the LND lipids rapidly integrated with cell membranes, ultimately leading to complete dissolution of the LND into the lipid membrane. In contrast, the presence of charged ILs in the LND led to weaker interaction with the biomembrane facilitated only by the lipid spike on the LND-membrane interface.
Conclusions
We conducted MD simulations to investigate the mechanism of interaction between IL-containing lipid nanodroplets (LNDs) and mammalian membrane lipids. To explore individual stages of lipid-mediated delivery systems, we studied ILs in both their protonation states. In the deprotonated (uncharged) state, which is relevant under neutral pH conditions in the bloodstream, LNDs merged with and dissolved seamlessly into the membrane. The uncharged ILs readily flip-flopped across the membrane, indicating smooth integration with mammalian cell lipids. These findings indicate the high biocompatibility of neutral ILs, causing minimal disruption to the membrane structure. Conversely, in the protonated state, charged IL-containing nanodroplets did not merge into the membrane but instead induced membrane deformation in the form of a lipid spike. This phenomenon may be attributed to the inability of protonated ILs to flip-flop, affecting predominantly only one membrane leaflet. These atomistic insights into IL-membrane interactions offer great potential for advancing the research and rational design of ILs in the rapidly progressing field of RNA medicine. Furthermore, the in-depth understanding presented here opens new avenues for future exploration in IL-biomembrane simulations at larger scales, which could ultimately yield a comprehensive understanding of endosomal escape mechanisms.
Supplemental Material
Download Zip (7.3 MB)Acknowledgements
We acknowledge IT4Innovation, Czech Republic for granting this project access to the LUMI supercomputer owned by the EuroHPC Joint Undertaking, hosted by CSC (Finland) and the LUMI consortium with the support of the Ministry of Education, Youth and Sports of the Czech Republic through e-INFRA CZ (grant 90254). COST Action CA21101 is also acknowledged.
Disclosure statement
MO has a share in a biosimulations company, InSiliBio, which focuses mostly on membrane permeation.
Data availability statement
The starting structures and topologies as well as trajectories, simulation details and scripts are available in the online Zenodo repository (https://doi.org/10.5281/zenodo.10679359).
Additional information
Funding
References
- Abraham, M. J., Murtola, T., Schulz, R., Páll, S., Smith, J. C., Hess, B., & Lindahl, E. (2015). GROMACS: High performance molecular simulations through multi-level parallelism from laptops to supercomputers. SoftwareX, 1-2, 19–25. https://doi.org/10.1016/j.softx.2015.06.001
- Anderson, E., Bai, Z., Bischof, C., Blackford, S., Demmel, J., Dongarra, J., Du Croz, J., Greenbaum, A., Hammarling, S., McKenney, A., & Sorensen, D. (1999). LAPACK Users’ Guide. (3rd ed.). Society for Industrial and Applied Mathematics.
- Beg, M., Taka, J., Kluyver, T., Konovalov, A., Ragan-Kelley, M., Thiery, N. M., & Fangohr, H. (2021). Using Jupyter for reproducible scientific workflows. Computing in Science & Engineering, 23(2), 36–46. https://doi.org/10.1109/MCSE.2021.3052101
- Berendsen, H. J. C., Postma, J. P. M., Van Gunsteren, W. F., DiNola, A., & Haak, J. R. (1984). Molecular dynamics with coupling to an external bath. The Journal of Chemical Physics, 81(8), 3684–3690. https://doi.org/10.1063/1.448118
- Berendsen, H. J. C., Van Der Spoel, D., & Van Drunen, R. (1995). GROMACS: A message-passing parallel molecular dynamics implementation. Computer Physics Communications, 91(1-3), 43–56. https://doi.org/10.1016/0010-4655(95)00042-E
- Brader, M. L., Williams, S. J., Banks, J. M., Hui, W. H., Zhou, Z. H., & Jin, L. (2021). Encapsulation state of messenger RNA inside lipid nanoparticles. Biophysical Journal, 120S0006349521002411. (14), 2766–2770. https://doi.org/10.1016/j.bpj.2021.03.012
- Bruininks, B. M., Souza, P. C., Ingolfsson, H., & Marrink, S. J. (2020). A molecular view on the escape of lipoplexed DNA from the endosome. eLife, 9, e52012. https://doi.org/10.7554/eLife.52012
- Carrasco, M. J., Alishetty, S., Alameh, M.-G., Said, H., Wright, L., Paige, M., Soliman, O., Weissman, D., Cleveland, T. E., Grishaev, A., & Buschmann, M. D. (2021). Ionization and structural properties of mRNA lipid nanoparticles influence expression in intramuscular and intravascular administration. Communications Biology, 4(1), 956. https://doi.org/10.1038/s42003-021-02441-2
- Chapman, B., & Chang, J. (2000). Biopython: Python tools for computational biology. ACM SIGBIO Newsletter, 20(2), 15–19. https://doi.org/10.1145/360262.360268
- Chen, J., Zhou, G., Chen, L., Wang, Y., Wang, X., & Zeng, S. (2016). Interaction of graphene and its oxide with lipid membrane: A molecular dynamics simulation study. The Journal of Physical Chemistry C, 120(11), 6225–6231. https://doi.org/10.1021/acs.jpcc.5b10635
- Cheng, M. H. Y., Brimacombe, C. A., Verbeke, R., & Cullis, P. R. (2022). Exciting times for lipid nanoparticles: How Canadian discoveries are enabling gene therapies. Molecular Pharmaceutics, 19(6), 1663–1668. https://doi.org/10.1021/acs.molpharmaceut.2c00365
- Cock, P. J. A., Antao, T., Chang, J. T., Chapman, B. A., Cox, C. J., Dalke, A., Friedberg, I., Hamelryck, T., Kauff, F., Wilczynski, B., & De Hoon, M. J. L. (2009). Biopython: Freely available Python tools for computational molecular biology and bioinformatics. Bioinformatics (Oxford, England), 25(11), 1422–1423. https://doi.org/10.1093/bioinformatics/btp163
- COVID-19 vaccine doses administered by manufacturer. (n.d.). Retrieved October 5, 2023 https://ourworldindata.org/grapher/covid-vaccine-doses-by-manufacturer?country=European+Union∼USA
- Dehghani-Ghahnaviyeh, S., Smith, M., Xia, Y., Dousis, A., Grossfield, A., & Sur, S. (2023). Ionizable amino lipids distribution and effects on DSPC/cholesterol membranes: Implications for lipid nanoparticle structure. The Journal of Physical Chemistry. B, 127(31), 6928–6939. https://doi.org/10.1021/acs.jpcb.3c01296
- Ding, W., Palaiokostas, M., Wang, W., & Orsi, M. (2015). Effects of lipid composition on bilayer membranes quantified by all-atom molecular dynamics. The Journal of Physical Chemistry. B, 119(49), 15263–15274. https://doi.org/10.1021/acs.jpcb.5b06604
- Doktorova, M., Symons, J. L., & Levental, I. (2020). Structural and functional consequences of reversible lipid asymmetry in living membranes. Nature Chemical Biology, 16(12), 1321–1330. https://doi.org/10.1038/s41589-020-00688-0
- Ermilova, I., & Swenson, J. (2020). DOPC versus DOPE as a helper lipid for gene-therapies: Molecular dynamics simulations with DLin-MC3-DMA. Physical Chemistry Chemical Physics: PCCP, 22(48), 28256–28268. https://doi.org/10.1039/D0CP05111J
- Essmann, U., Perera, L., Berkowitz, M. L., Darden, T., Lee, H., & Pedersen, L. G. (1995). A smooth particle mesh Ewald method. The Journal of Chemical Physics, 103(19), 8577–8593. https://doi.org/10.1063/1.470117
- Eygeris, Y., Gupta, M., Kim, J., & Sahay, G. (2022). Chemistry of lipid nanoparticles for RNA delivery. Accounts of Chemical Research, 55(1), 2–12. https://doi.org/10.1021/acs.accounts.1c00544
- Gould, I. R., Skjevik, Å. A., Dickson, C. J., Madej, B. D., & Walker, R. C. (2018). Lipid17: A comprehensive AMBER force field for the simulation of zwitterionic and anionic lipids.
- Gowers, R., Linke, M., Barnoud, J., Reddy, T., Melo, M., Seyler, S., Domański, J., Dotson, D., Buchoux, S., Kenney, I., & Beckstein, O. (2016). MDAnalysis: A Python package for the rapid analysis of molecular dynamics simulations. 98–105. https://doi.org/10.25080/Majora-629e541a-00e
- Hamelryck, T., & Manderick, B. (2003). PDB file parser and structure class implemented in Python. Bioinformatics (Oxford, England), 19(17), 2308–2310. https://doi.org/10.1093/bioinformatics/btg299
- Harayama, T., & Riezman, H. (2018). Understanding the diversity of membrane lipid composition. Nature Reviews. Molecular Cell Biology, 19(5), 281–296. https://doi.org/10.1038/nrm.2017.138
- Harris, C. R., Millman, K. J., van der Walt, S. J., Gommers, R., Virtanen, P., Cournapeau, D., Wieser, E., Taylor, J., Berg, S., Smith, N. J., Kern, R., Picus, M., Hoyer, S., van Kerkwijk, M. H., Brett, M., Haldane, A., Del Río, J. F., Wiebe, M., Peterson, P., … Oliphant, T. E. (2020). Array programming with NumPy. Nature, 585(7825), 357–362. https://doi.org/10.1038/s41586-020-2649-2
- Hess, B. (2008). P-LINCS: A parallel linear constraint solver for molecular simulation. Journal of Chemical Theory and Computation, 4(1), 116–122. https://doi.org/10.1021/ct700200b
- Hess, B., Kutzner, C., Van Der Spoel, D., & Lindahl, E. (2008). GROMACS 4: Algorithms for highly efficient, load-balanced, and scalable molecular simulation. Journal of Chemical Theory and Computation, 4(3), 435–447. https://doi.org/10.1021/ct700301q
- Hoover, W. G. (1985). Canonical dynamics: Equilibrium phase-space distributions. Physical Review. A, General Physics, 31(3), 1695–1697. https://doi.org/10.1103/PhysRevA.31.1695
- Hou, X., Zaks, T., Langer, R., & Dong, Y. (2021). Lipid nanoparticles for mRNA delivery. Nature Reviews. Materials, 6(12), 1078–1094. https://doi.org/10.1038/s41578-021-00358-0
- Hub, J. S., De Groot, B. L., & Van Der Spoel, D. (2010). g_wham—A free weighted histogram analysis implementation including robust error and autocorrelation estimates. Journal of Chemical Theory and Computation, 6(12), 3713–3720. https://doi.org/10.1021/ct100494z
- Humphrey, W., Dalke, A., & Schulten, K. (1996). VMD: Visual molecular dynamics. Journal of Molecular Graphics, 14(1), 33–38. https://doi.org/10.1016/0263-7855(96)00018-5
- Hunter, J. D. (2007). Matplotlib: A 2D graphics environment. Computing in Science & Engineering, 9(3), 90–95. https://doi.org/10.1109/MCSE.2007.55
- Izadi, S., Anandakrishnan, R., & Onufriev, A. V. (2014). Building water models: A different approach. The Journal of Physical Chemistry Letters, 5(21), 3863–3871. https://doi.org/10.1021/jz501780a
- Jayaraman, M., Ansell, S. M., Mui, B. L., Tam, Y. K., Chen, J., Du, X., Butler, D., Eltepu, L., Matsuda, S., Narayanannair, J. K., Rajeev, K. G., Hafez, I. M., Akinc, A., Maier, M. A., Tracy, M. A., Cullis, P. R., Madden, T. D., Manoharan, M., & Hope, M. J. (2012). Maximizing the potency of siRNA lipid nanoparticles for hepatic gene silencing in vivo. Angewandte Chemie (International ed. in English), 51(34), 8529–8533. https://doi.org/10.1002/anie.201203263
- Jo, S., Kim, T., & Im, W. (2007). Automated builder and database of protein/membrane complexes for molecular dynamics simulations. PLoS One, 2(9), e880. https://doi.org/10.1371/journal.pone.0000880
- Jo, S., Kim, T., Iyer, V. G., & Im, W. (2008). CHARMM-GUI: A web-based graphical user interface for CHARMM. Journal of Computational Chemistry, 29(11), 1859–1865. https://doi.org/10.1002/jcc.20945
- Jo, S., Lim, J. B., Klauda, J. B., & Im, W. (2009). CHARMM-GUI membrane builder for mixed bilayers and its application to yeast membranes. Biophysical Journal, 97(1), 50–58. https://doi.org/10.1016/j.bpj.2009.04.013
- Joung, I. S., & Cheatham, T. E. (2008). Determination of alkali and halide monovalent ion parameters for use in explicitly solvated biomolecular simulations. The Journal of Physical Chemistry. B, 112(30), 9020–9041. https://doi.org/10.1021/jp8001614
- Kaasgaard, T., & Drummond, C. J. (2006). Ordered 2-D and 3-D nanostructured amphiphile self-assembly materials stable in excess solvent. Physical Chemistry Chemical Physics: PCCP, 8(43), 4957–4975. https://doi.org/10.1039/b609510k
- Kulkarni, J. A., Darjuan, M. M., Mercer, J. E., Chen, S., van der Meel, R., Thewalt, J. L., Tam, Y. Y. C., & Cullis, P. R. (2018). On the formation and morphology of lipid nanoparticles containing ionizable cationic lipids and siRNA. ACS Nano, 12(5), 4787–4795. https://doi.org/10.1021/acsnano.8b01516
- Kumar, S., Rosenberg, J. M., Bouzida, D., Swendsen, R. H., & Kollman, P. A. (1992). The weighted histogram analysis method for free-energy calculations on biomolecules. I. The method. Journal of Computational Chemistry, 13(8), 1011–1021. https://doi.org/10.1002/jcc.540130812
- Kumari, P., Pillai, V. V. S., & Benedetto, A. (2020). Mechanisms of action of ionic liquids on living cells: The state of the art. Biophysical Reviews, 12(5), 1187–1215. https://doi.org/10.1007/s12551-020-00754-w
- Lazaratos, M., Karathanou, K., Mainas, E., Chatzigoulas, A., Pippa, N., Demetzos, C., & Cournia, Z. (2020). Coating of magnetic nanoparticles affects their interactions with model cell membranes. Biochimica et Biophysica Acta. General Subjects, 1864(11), 129671. https://doi.org/10.1016/j.bbagen.2020.129671
- Lee, J., Cheng, X., Swails, J. M., Yeom, M. S., Eastman, P. K., Lemkul, J. A., Wei, S., Buckner, J., Jeong, J. C., Qi, Y., Jo, S., Pande, V. S., Case, D. A., Brooks, C. L., MacKerell, A. D., Klauda, J. B., & Im, W. (2016). CHARMM-GUI input generator for NAMD, GROMACS, AMBER, OpenMM, and CHARMM/OpenMM simulations using the CHARMM36 additive force field. Journal of Chemical Theory and Computation, 12(1), 405–413. https://doi.org/10.1021/acs.jctc.5b00935
- Lee, J., Hitzenberger, M., Rieger, M., Kern, N. R., Zacharias, M., & Im, W. (2020). CHARMM-GUI supports the Amber force fields. The Journal of Chemical Physics, 153(3), 035103. https://doi.org/10.1063/5.0012280
- Lee, J., Patel, D. S., Ståhle, J., Park, S.-J., Kern, N. R., Kim, S., Lee, J., Cheng, X., Valvano, M. A., Holst, O., Knirel, Y. A., Qi, Y., Jo, S., Klauda, J. B., Widmalm, G., & Im, W. (2019). CHARMM-GUI membrane builder for complex biological membrane simulations with glycolipids and lipoglycans. Journal of Chemical Theory and Computation, 15(1), 775–786. https://doi.org/10.1021/acs.jctc.8b01066
- Leikin, S. L., Kozlov, M. M., Chernomordik, L. V., Markin, V. S., & Chizmadzhev, Y. A. (1987). Membrane fusion: Overcoming of the hydration barrier and local restructuring. Journal of Theoretical Biology, 129(4), 411–425. https://doi.org/10.1016/S0022-5193(87)80021-8
- Leung, A. K. K., Hafez, I. M., Baoukina, S., Belliveau, N. M., Zhigaltsev, I. V., Afshinmanesh, E., Tieleman, D. P., Hansen, C. L., Hope, M. J., & Cullis, P. R. (2012). Lipid nanoparticles containing siRNA synthesized by microfluidic mixing exhibit an electron-dense nanostructured core. The Journal of Physical Chemistry. C, Nanomaterials and Interfaces, 116(34), 18440–18450. https://doi.org/10.1021/jp303267y
- Lindahl, E., Hess, B., & Van Der Spoel, D. (2001). GROMACS 3.0: A package for molecular simulation and trajectory analysis. Journal of Molecular Modeling, 7(8), 306–317. https://doi.org/10.1007/s008940100045
- Lorent, J. H., Levental, K. R., Ganesan, L., Rivera-Longsworth, G., Sezgin, E., Doktorova, M., Lyman, E., & Levental, I. (2020). Plasma membranes are asymmetric in lipid unsaturation, packing and protein shape. Nature Chemical Biology, 16(6), 644–652. https://doi.org/10.1038/s41589-020-0529-6
- Malburet, C., Leclercq, L., Cotte, J.-F., Thiebaud, J., Bazin, E., Garinot, M., & Cottet, H. (2022). Size and charge characterization of lipid nanoparticles for mRNA vaccines. Analytical Chemistry, 94(11), 4677–4685. https://doi.org/10.1021/acs.analchem.1c04778
- Marrink, S.-J., & Mark, A. E. (2004). Molecular view of hexagonal phase formation in phospholipid membranes. Biophysical Journal, 87(6), 3894–3900. https://doi.org/10.1529/biophysj.104.048710
- Marrink, S.-J., & Tieleman, D. P. (2002). Molecular dynamics simulation of spontaneous membrane fusion during a cubic-hexagonal phase transition. Biophysical Journal, 83(5), 2386–2392. https://doi.org/10.1016/S0006-3495(02)75252-1
- Mathieu, E., Ritchie, H., Ortiz-Ospina, E., Roser, M., Hasell, J., Appel, C., Giattino, C., & Rodés-Guirao, L. (2021). A global database of COVID-19 vaccinations. Nature Human Behaviour, 5(7), 947–953. https://doi.org/10.1038/s41562-021-01122-8
- Michaud-Agrawal, N., Denning, E. J., Woolf, T. B., & Beckstein, O. (2011). MDAnalysis: A toolkit for the analysis of molecular dynamics simulations. Journal of Computational Chemistry, 32(10), 2319–2327. https://doi.org/10.1002/jcc.21787
- Nosé, S., & Klein, M. L. (1983). Constant pressure molecular dynamics for molecular systems. Molecular Physics, 50(5), 1055–1076. https://doi.org/10.1080/00268978300102851
- Páll, S., Abraham, M. J., Kutzner, C., Hess, B., & Lindahl, E. (2015). Tackling exascale software challenges in molecular dynamics simulations with GROMACS. In S. Markidis & E. Laure (Eds.), Solving software challenges for exascale (Vol. 8759, pp. 3–27). Springer International Publishing. https://doi.org/10.1007/978-3-319-15976-8_1
- Paloncýová, M., Čechová, P., Šrejber, M., Kührová, P., & Otyepka, M. (2021). Role of ionizable lipids in SARS-CoV-2 vaccines as revealed by molecular dynamics simulations: From membrane structure to interaction with mRNA fragments. The Journal of Physical Chemistry Letters, 12(45), 11199–11205. https://doi.org/10.1021/acs.jpclett.1c03109
- Paloncýová, M., Fabre, G., DeVane, R. H., Trouillas, P., Berka, K., & Otyepka, M. (2014). Benchmarking of force fields for molecule–membrane interactions. Journal of Chemical Theory and Computation, 10(9), 4143–4151. https://doi.org/10.1021/ct500419b
- Paloncýová, M., Šrejber, M., Čechová, P., Kührová, P., Zaoral, F., & Otyepka, M. (2023). Atomistic insights into organization of RNA-loaded lipid nanoparticles. The Journal of Physical Chemistry. B, 127(5), 1158–1166. https://doi.org/10.1021/acs.jpcb.2c07671
- Parrinello, M., & Rahman, A. (1981). Polymorphic transitions in single crystals: A new molecular dynamics method. Journal of Applied Physics, 52(12), 7182–7190. https://doi.org/10.1063/1.328693
- Patel, P., Ibrahim, N. M., & Cheng, K. (2021). The importance of apparent pKa in the development of nanoparticles encapsulating siRNA and mRNA. Trends in Pharmacological Sciences, 42(6), 448–460. https://doi.org/10.1016/j.tips.2021.03.002
- Patel, S., Kim, J., Herrera, M., Mukherjee, A., Kabanov, A. V., & Sahay, G. (2019). Brief update on endocytosis of nanomedicines. Advanced Drug Delivery Reviews, 144, 90–111. https://doi.org/10.1016/j.addr.2019.08.004
- Pronk, S., Páll, S., Schulz, R., Larsson, P., Bjelkmar, P., Apostolov, R., Shirts, M. R., Smith, J. C., Kasson, P. M., van der Spoel, D., Hess, B., & Lindahl, E. (2013). GROMACS 4.5: A high-throughput and highly parallel open source molecular simulation toolkit. Bioinformatics (Oxford, England), 29(7), 845–854. https://doi.org/10.1093/bioinformatics/btt055
- Ramasubramani, V., Dice, B. D., Harper, E. S., Spellings, M. P., Anderson, J. A., & Glotzer, S. C. (2020). freud: A software suite for high throughput analysis of particle simulation data. Computer Physics Communications, 254, 107275. https://doi.org/10.1016/j.cpc.2020.107275
- Ramezanpour, M., Schmidt, M. L., Bashe, B. Y. M., Pruim, J. R., Link, M. L., Cullis, P. R., Harper, P. E., Thewalt, J. L., & Tieleman, D. P. (2020). Structural properties of inverted hexagonal phase: A hybrid computational and experimental approach. Langmuir: The ACS Journal of Surfaces and Colloids, 36(24), 6668–6680. https://doi.org/10.1021/acs.langmuir.0c00600
- Ramezanpour, M., Schmidt, M. L., Bodnariuc, I., Kulkarni, J. A., Leung, S. S. W., Cullis, P. R., Thewalt, J. L., & Tieleman, D. P. (2019). Ionizable amino lipid interactions with POPC: Implications for lipid nanoparticle function. Nanoscale, 11(30), 14141–14146. https://doi.org/10.1039/C9NR02297J
- Sandoval-Perez, A., Pluhackova, K., & Böckmann, R. A. (2017). Critical comparison of biomembrane force fields: Protein–lipid interactions at the membrane interface. Journal of Chemical Theory and Computation, 13(5), 2310–2321. https://doi.org/10.1021/acs.jctc.7b00001
- Schneck, E., Sedlmeier, F., & Netz, R. R. (2012). Hydration repulsion between biomembranes results from an interplay of dehydration and depolarization. Proceedings of the National Academy of Sciences of the United States of America, 109(36), 14405–14409. https://doi.org/10.1073/pnas.1205811109
- Schrödinger, LLC. (2015). The PyMOL Molecular Graphics System, Version 2.5.
- Semple, S. C., Akinc, A., Chen, J., Sandhu, A. P., Mui, B. L., Cho, C. K., Sah, D. W. Y., Stebbing, D., Crosley, E. J., Yaworski, E., Hafez, I. M., Dorkin, J. R., Qin, J., Lam, K., Rajeev, K. G., Wong, K. F., Jeffs, L. B., Nechev, L., Eisenhardt, M. L., … Hope, M. J. (2010). Rational design of cationic lipids for siRNA delivery. Nature Biotechnology, 28(2), 172–176. https://doi.org/10.1038/nbt.1602
- Shepherd, S. J., Han, X., Mukalel, A. J., El-Mayta, R., Thatte, A. S., Wu, J., Padilla, M. S., Alameh, M.-G., Srikumar, N., Lee, D., Weissman, D., Issadore, D., & Mitchell, M. J. (2023). Throughput-scalable manufacturing of SARS-CoV-2 mRNA lipid nanoparticle vaccines. Proceedings of the National Academy of Sciences of the United States of America, 120(33), e2303567120. https://doi.org/10.1073/pnas.2303567120
- Shin, H., Park, S.-J., Yim, Y., Kim, J., Choi, C., Won, C., & Min, D.-H. (2018). Recent advances in RNA therapeutics and RNA delivery systems based on nanoparticles. Advanced Therapeutics, 1(7), 1800065. https://doi.org/10.1002/adtp.201800065
- Smith, P., & Lorenz, C. D. (2021). LiPyphilic: A Python toolkit for the analysis of lipid membrane simulations. Journal of Chemical Theory and Computation, 17(9), 5907–5919. https://doi.org/10.1021/acs.jctc.1c00447
- Torres-Sánchez, A., Vanegas, J. M., & Arroyo, M. (2015). Examining the mechanical equilibrium of microscopic stresses in molecular simulations. Physical Review Letters, 114(25), 258102. https://doi.org/10.1103/PhysRevLett.114.258102
- Torres-Sánchez, A., Vanegas, J. M., & Arroyo, M. (2016). Geometric derivation of the microscopic stress: A covariant central force decomposition. Journal of the Mechanics and Physics of Solids, 93, 224–239. https://doi.org/10.1016/j.jmps.2016.03.006
- Trollmann, M. F. W., & Böckmann, R. A. (2022). mRNA lipid nanoparticle phase transition. Biophysical Journal, 121(20), 3927–3939. https://doi.org/10.1016/j.bpj.2022.08.037
- Van Der Spoel, D., Lindahl, E., Hess, B., Groenhof, G., Mark, A. E., & Berendsen, H. J. C. (2005). GROMACS: Fast, flexible, and free. Journal of Computational Chemistry, 26(16), 1701–1718. https://doi.org/10.1002/jcc.20291
- Vanegas, J. M., & Arroyo, M. (2014). Force transduction and lipid binding in MscL: A continuum-molecular approach. PloS One, 9(12), e113947. https://doi.org/10.1371/journal.pone.0113947
- Vanegas, J. M., Torres-Sánchez, A., & Arroyo, M. (2014). Importance of force decomposition for local stress calculations in biomembrane molecular simulations. Journal of Chemical Theory and Computation, 10(2), 691–702. https://doi.org/10.1021/ct4008926
- Virtanen, P., Gommers, R., Oliphant, T. E., Haberland, M., Reddy, T., Cournapeau, D., Burovski, E., Peterson, P., Weckesser, W., Bright, J., van der Walt, S. J., Brett, M., Wilson, J., Millman, K. J., Mayorov, N., Nelson, A. R. J., Jones, E., Kern, R., Larson, E., … van Mulbregt, P. (2020). SciPy 1.0: Fundamental algorithms for scientific computing in Python. Nature Methods, 17(3), 261–272. https://doi.org/10.1038/s41592-019-0686-2
- Wang, F., Zuroske, T., & Watts, J. K. (2020). RNA therapeutics on the rise. Nature Reviews. Drug Discovery, 19(7), 441–442. https://doi.org/10.1038/d41573-020-00078-0
- Wang, J., Wolf, R. M., Caldwell, J. W., Kollman, P. A., & Case, D. A. (2004). Development and testing of a general amber force field. Journal of Computational Chemistry, 25(9), 1157–1174. https://doi.org/10.1002/jcc.20035
- Wu, E. L., Cheng, X., Jo, S., Rui, H., Song, K. C., Dávila-Contreras, E. M., Qi, Y., Lee, J., Monje-Galvan, V., Venable, R. M., Klauda, J. B., & Im, W. (2014). CHARMM-GUI Membrane Builder toward realistic biological membrane simulations. Journal of Computational Chemistry, 35(27), 1997–2004. https://doi.org/10.1002/jcc.23702