Abstract
Ecosystem models have emerged as vital tools for evaluating management strategies and are increasingly used by policymakers. We reviewed the history of modeling and identified research gaps based on policy needs for ecosystem-based management of Narragansett Bay (RI, USA)—a highly-impacted system with a history of research and monitoring dating back to the 1950s. There is a need to disentangle the impacts of nutrient reductions versus climate change on species interactions as well as understand the ecological and socio-economic tradeoffs of management actions. Within this context, we consider the following to be important for future research and creation of ecosystem models for Narragansett Bay: (i) account for multi-scale processes and patterns through two-way model coupling; (ii) incorporate human behavior as part of model predictions; and (iii) lessen the impact of model uncertainty by identifying robust management strategies that will sustain resources under a range of potential future scenarios. Ecosystem modeling that builds on prior models and is informed by knowledge gaps will be a powerful tool to operationalize ecosystem-based management in Narragansett Bay and globally, providing sustainable pathways for nature and people.
1. Introduction
The need to balance ecological and human well-being in the face of rapid environmental change has compelled scientists to work across the natural, physical, and social sciences in pursuit of ecosystem-based management (EBM; Arkema, Abramson, and Dewsbury Citation2006; Berkes Citation2012). EBM recognizes the need to: (1) address the multiple and cumulative ways in which humans use and impact the environment (Link and Browman Citation2014), (2) account for the interdependence among the physical, biological, and socioeconomic components within and among systems (McLeod et al. Citation2005), and (3) better understand how society can support the resilience and robustness of ecosystem goods and services (Levin and Lubchenco Citation2008). To accomplish these goals, EBM requires a diversity of disciplinary input from environmental science, ecology, economics, mathematics, as well as the physical, earth, social, and political sciences. One way to synthesize these inputs while also exploring tradeoffs across objectives is using ecosystem models. Ecosystem models are a powerful tool for EBM; they have been used to study nutrient loading (Álvarez-Romero et al. Citation2014), land use (Mango et al. Citation2011), and fisheries management (Latour, Brush, and Bonzek Citation2003; Christensen and Walters Citation2005). However, ecosystem models are challenging to build and parameterize. This is often because of the large data needs, complexity in interactions among components, and the uncertainty in data inputs particularly for human behavioral processes (Link et al. Citation2012).
Ecosystem modeling can be used for all types of aquatic environments. However, modeling estuaries and coastal ecosystems differ from that of other systems (Ji Citation2017). Estuaries are located where oceanic, riverine, and terrestrial ecosystems meet, thus introducing a great deal of variability in physical, biological, and social processes. As a result, estuaries are characterized by strong spatial and temporal gradients, which can introduce additional difficulty to modeling (Elliott and Whitfield Citation2011). For example, variables like salinity (Berounsky and Nixon Citation1993; Telesh and Khlebovich Citation2010) and plankton abundance (Soetaert and Van Rijswijk Citation1993; Park and Marshall Citation2000) can differ greatly from the top of an estuary to the outlet into the ocean. Humans have long used estuaries as hubs of development and there is heterogeneity in uses that range from transportation of goods, fishing, and tourism (Lotze et al. Citation2006). These multifaceted characteristics of estuaries and their interactions across space and time present unique challenges to scientists attempting to model processes, as well as to managers forming policies for sustainable use.
The goal of this study is to review the history of modeling for Narragansett Bay (Rhode Island, USA) in order to provide a lens for future research that better serves ecosystem-based management (EBM). In this review, we consider Narragansett Bay as a study site and provide some historical context on the setting (Sec. 1.1). Then we classify previous modeling approaches and their characteristics, restricting our examination to only those performed with Narragansett Bay as its focal area (Sec. 2). We summarize the main findings and synthesize across studies (Sec. 3.1), and with input from managers, identify research needs to be able to take EBM from concept to reality (Sec. 3.2). Finally, within the context of past modeling efforts and current management needs, we provide suggestions for how these knowledge gaps could be filled using modeling methods (Sec. 3.3).
1.1. Study site and context
Narragansett Bay waters cover an area of 328 km2 (4766 km2 watershed and 685 km coastline), making it the largest estuary along the New England (USA) coast. It has a long history of settlement dating back to the early 16th century (Chinman and Nixon Citation1985; Boothroyd and August Citation2008) and shares its name with the Indigenous Tribe that occupied most of present-day Rhode Island, the Narragansett people. Archival sources and oral tradition indicate that before European contact, the Narragansett People, whose descendants still exist today, were primarily a hunting and fishing culture relying on the bay’s natural resources for their subsistence (Herndon and Sekatau Citation1997).
With the settlement of European colonialists, Narragansett Bay continued to play an important role in the development of the state of Rhode Island, both for its fisheries resources and as a passage for maritime trade. Although the bay experienced rapid population growth during colonial times, industries were low impact into the late 1700s (Desbonnet and Lee Citation1991). Major increases in nutrients (nitrogen and phosphorous) began around the late 1800s with the development of sewer systems during the Industrial Revolution (Desbonnet and Lee Citation1991; Nixon Citation1995). By the late 1900s, nutrient inputs into the bay had increased at least 500% as a result of human activities (Nixon Citation1997). During this period and over just a 50-year time span, average spring-summer water temperatures in Narragansett Bay increased 1.5 °C (Collie, Wood, and Jeffries Citation2008). As human population increased in the areas surrounding the bay, pollution and nutrient loading from sewage outfalls and land development also increased (Hamburg et al. Citation2008; Vadeboncoeur, Hamburg, and Pryor Citation2010), coinciding with changes to species composition and decreases in fisheries yields (Oviatt et al. Citation2003; Collie, Wood, and Jeffries Citation2008). In the last several decades, concerted efforts have been made to reduce these inputs (Oviatt et al. Citation2017), in part due to interest in restoring fisheries, promoting tourism, developing aquaculture, and a general desire to improve the water quality of the bay. These efforts, which included upgrading wastewater treatment systems, have decreased nitrogen input by over 50% in the last decade (Oczkowski et al. Citation2018). While there have been improvements, the bay still faces challenges related to nitrogen and phosphorus runoff (Schmidt et al. Citation2020).
There is a long history of data collection and scientific study in Narragansett Bay, which has paved the way for the creation of models. Ongoing data collection and monitoring activities gather data for a variety of purposes to inform multiple stakeholders (). Temperature, salinity, nutrient concentrations (e.g., nitrogen, phosphorus), phytoplankton, invertebrates, and fish abundance have been measured weekly in the bay for nearly 60 years. Other biophysical processes (e.g., zooplankton biomass) have been monitored weekly for shorter time periods, while other measurements (e.g., benthic metabolism, water circulation) have been more opportunistic (project-based) and began within the last 25 years. To our knowledge, there are no regularly collected socioeconomic datasets for Narragansett Bay, except for fisheries catch. While past modeling efforts have made use of these datasets (), the majority of the models only represent one component of the larger ecosystem. There is still a need to create linked and multi-scale models, or ecosystem models, to understand how social-ecological processes interact.
Table 1. Current data availability for Narragansett Bay (RI, USA) including model parameter, sampling frequency, data duration, and variables measured.
The Rhode Island Department of Environmental Management (RIDEM) is charged with managing the Narragansett Bay ecosystem, including its water quality and marine resources. Ecosystem modeling focused on water quality and lower trophic levels has been sought after by state managers to understand the bottom-up drivers of coastal hypoxia, a detriment to many marine species. Various models have been evaluated by state managers to inform water quality conditions for Narragansett Bay and how nutrient loading should be regulated. Hydrodynamic modeling using the Regional Ocean Modeling System (ROMS) and Environmental Fluid Dynamics Code (EFDC) coupled with the EcoGEM and Water Quality Analysis Simulation Program (WASP), respectively, have been used to understand how estuarine circulation allow for nutrients to be available for phytoplankton assimilation (Kremer et al. Citation2010; Vaudrey Citation2016; Rashleigh et al. Citation2015; Ullman et al. Citation2019). Such modeling has provided insight into the circulation of the bay, its influence on nutrient standing stocks, and the connectivity to eutrophication and subsequently hypoxia.
Management of the bay’s marine resources, such as recreational and commercial fish and invertebrates, uses scientific monitoring and analytics to support informed decision making on regulatory measures. The resources are managed through various fisheries management plans that utilize a spectrum of management tools (e.g., size limits, possession limits, temporal restrictions, limited access, gear restrictions, spatial closures) to ensure sustainable fisheries. The State’s fisheries management is collaborative with federal and state agencies and regional management entities (such as the New England Fisheries Management Council, Mid-Atlantic Fisheries Management Council, Atlantic States Marine Fisheries Commission), given the migratory, transboundary nature of many species. Instances of ecosystem-based management for Narragansett Bay fish and invertebrates have primarily been tied to regional fisheries management efforts. For example, Atlantic menhaden total allowable catch is informed by ecological reference points for the species, which consider both the single species population dynamics of menhaden and the foraging needs of Atlantic striped bass (Chagaris et al. Citation2020; Anstead et al. Citation2021). These reference points are informed by food web modeling using Ecopath w/Ecosim of the northwest Atlantic continental shelf (Anstead et al. Citation2021). However, specific ecosystem minded management efforts in Narragansett Bay do exist. To ensure forage fish are available for both harvest and ecosystem function, the commercial menhaden fishery is managed in the bay using various harvest caps and triggers (informed predicted biomass estimates from a depletion model and spotter pilot-based abundance estimates) to open and close the fishery. The caps and triggers are designed to ensure that menhaden can also support higher tropic levels of the bay ecosystem, such as predatory piscivores.
2. Methods
When reviewing models, our inclusion criteria were peer-reviewed studies with simulation models (not statistical or correlative models) performed for all, or greater than 50% total area, of Narragansett Bay. One exception to our inclusion criteria was ecosystem services models. While not used for predictive purposes, they are still important tools for stakeholder engagement, decision-making, and ultimately EBM, and therefore included here. We built on the model classification scheme of Piroddi and colleagues (2015) to group peer-reviewed published studies of Narragansett Bay into six model classes that account for various aspects of the physical, biological, or human components of the ecosystem. The six model classes included: (1) physical; (2) hydro-biogeochemical; (3) food-web; (4) agent-based; (5) economic input-output; and (6) ecosystem services ().
Figure 1. Box model of different model classes identified for Narragansett Bay (RI, USA), as well as their connections to one another.
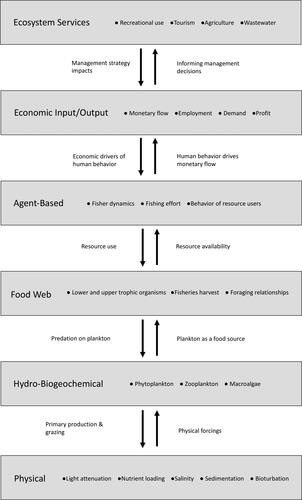
To identify future research needs, we (including coauthors JM and CM from the Rhode Island Department of Environmental Management) worked to isolate the largest knowledge gaps impeding EBM. These knowledge gaps were also mentioned by recent studies () as potential next steps for improving predictive modeling of Narragansett Bay (see Sec. 3.3 for further detail).
Table 2. Narragansett Bay (RI, USA) modeling studies and their details (class, inputs, and outputs), listed in chronological order of each model’s first iteration.
3. Results and discussion
3.1. Reviewing modeling approaches for Narragansett Bay
Many types of models have been developed for Narragansett Bay, but the majority have focused on physical processes without attempts to link them to other parts of the ecosystem (). Over a period of four decades, there has also been an emphasis on hydro-biogeochemical models of plankton dynamics, focusing entirely on the lower trophic levels of the food web. Much less effort has been devoted to other ecosystem components, including the higher trophic levels, and even less attention has been given to the integration of human dimensions, specifically behavior and decision-making of resource users.
Physical models, the most prevalent model type created for Narragansett Bay (), are concerned with predicting the distribution (e.g., transport, diffusion) of one or more of an ecosystem’s physical components. Topics of the existing physical models in Narragansett Bay range from the mobility of trace metals (Santschi et al. Citation1983, Citation1987), freshwater and salinity inputs (Pilson Citation1985), and nutrient loading into the bay (Vadeboncoeur, Hamburg, and Pryor 2010). These models have important management implications, informing how managers should address pollutants in aquatic systems as well as characterizing the residence times of water and nutrients that are important for considering the “self-cleaning” properties of Narragansett Bay.
Additional physical models of Narragansett Bay have important implications for other components of the ecosystem. These models have focused on topics such as advection and dispersal (Kremer et al. Citation2010), residence and exposure time (Abdelrhman Citation2007), light attenuation (Abdelrhman Citation2016, Citation2017), sedimentation rates (Doering and Oviatt Citation1986), and larval transport (Mcmanus et al. Citation2020). In some cases, physical characteristics can even be predictive of biological processes. For example, Shull (Citation2001) used a physical model to describe how benthic deposit feeders and sedimentation were important drivers of bioturbation in Narragansett Bay, and Abdelrhman and Cicchetti (Citation2012) quantified how an intermediate nitrogen load maximizes the bioturbation activities of benthic invertebrates. Implications of these physical studies are especially important for managing water quality and the health of algae and seagrass beds. These studies demonstrate that physical processes can be used as proxies for making biological predictions.
Hydro-biogeochemical models take physical models one step further by focusing on predicting the response dynamics of lower-level trophic groups such as phytoplankton and zooplankton. Narragansett Bay, like many estuaries, has a long history of studying plankton dynamics (Kremer and Nixon Citation1978). Several of these models use mechanistic approaches incorporating physical forcing, nutrient cycling, food-web structure (limited to lower-trophic levels), as well as biological processes such as growth, predation, respiration, and decay (Kremer and Nixon Citation1978; Kremer and Kremer Citation1982; Nixon, Granger, and Nowicki Citation1995) in order to predict the population dynamics of plankton groups. Another approach that has proven effective in simplifying these models is the use of empirically-based relationships to represent certain key processes (Brush et al. Citation2002; Brawley et al. Citation2003; Brush and Brawley Citation2009; Brush and Nixon Citation2017). This method integrates multiple processes into simplified bulk functions, resulting in a less complex model that is still robust in its prediction ability (Brush and Nixon Citation2010, Citation2017; Borkman and Smayda Citation2016). Hydro-biogeochemical models have been important for simulating hypoxic events, climate change, and nutrient inputs in Narragansett Bay (Vaudrey Citation2016; Brush and Nixon Citation2017).
Food web models are another important model class and tend to include both lower and higher trophic level organisms. One example is the Ecopath model (Christensen and Pauly Citation1992; Christensen and Walters Citation2004) which has now been used multiple times in Narragansett Bay, first to predict the carrying capacity of shellfish aquaculture production (Byron et al. Citation2011) and more recently to create a fisheries-focused time-dynamic food web model capable of forecasting organism biomass (Innes-Gold et al. Citation2020). These models operate using principles in energy transfer that is mediated by predator-prey interactions and human removal. The Narragansett Bay Ecopath models included mid and higher trophic groups like invertebrates and fishes, as well as lower trophic level groups like benthic algae and plankton but had no representation of hydrodynamics or physical forcings. One potential way around this drawback is to link food web models to physical models, which also makes them more relevant to managers. For example, the Byron et al. (Citation2011) model was updated and used by the EPA to explore how changing nitrogen availability would impact the upper trophic level functional groups (Rashleigh et al. Citation2015), using data from a model of nitrogen loading to Narragansett Bay (Vadeboncouer, Hamburg, and Pryor 2010). Another example of a food web model including lower trophic level data is the Atlantis model for the northeastern US (Link, Fulton, and Gamble Citation2010; Olsen et al. Citation2016), which incorporated information from physical forcing components (e.g., upwelling time series, temperature, and salinity) as well as socioeconomics (e.g., catch and effort of various fishing fleets). However, the spatial scale of the model (i.e., the entire northeastern US) is too broad for the purposes of informing managers of Narragansett Bay.
Food web models for Narragansett Bay have been linked to economic input-output models (Byron, Jin, and Dalton Citation2015). Economic models are used to assess the implications (e.g., employment, income, tax revenues) of a particular action or policy across industries or socioeconomic groups (e.g., different consumer income levels). Byron and colleagues (2014) were able to assess the consequences of changes in certain fisheries stocks across multiple marine-based industries.
One approach that has embraced both the complexity and diversity of human behavior is agent-based modeling whereby individual behaviors or decisions, as well as their interactions, have been modeled with the goal of considering their collective consequence on the system (Dreyfus-León Citation1999; Soulié and Thébaud Citation2006). For example, Holland and Sutinen (Citation1999) accounted for the heterogeneity of human fishing behavior in Narragansett Bay by incorporating information from ethnographic interviews of skippers of large trawlers to predict fishing locations. This was based on variables like average revenue, total effort in the past, trip length, and surface area. Such an approach has been useful for managers to consider how individual fishers may respond to management controls on total catch or effort levels in Narragansett Bay (Holland and Sutinen Citation1999). There is potential to link agent-based models to biologically focused models. For example, Innes-Gold et al. (Citation2021) created an agent-based model of the Narragansett Bay recreational fishery for piscivorous fish and linked it to the existing Ecopath model (Innes-Gold et al. Citation2020) to explore how dynamic human behavior influenced the food web and vice versa.
Finally, ecosystem service models combine the physical, biological, and socioeconomic components of a system under one conceptual framework. The focus of these models is on the value of natural resources to human and societal well-being as well as the flow, provision, and use of goods and services produced by nature (Daily et al. Citation2009; Villa et al. Citation2011; Boumans et al. Citation2015). One such model that was developed for Narragansett Bay has been actively used in dialogues between federal and local government agencies, as well as non-governmental stakeholders. It allows users to explore possible management actions including the treatment of wastewater, sewage reductions, and infrastructure development and visualize the results by projecting economic, social, and environmental indicators (Fiksel et al. Citation2014).
3.2. Identifying management-relevant knowledge gaps for Narragansett Bay
From our review (Sec. 3.1) and RIDEM’s current management efforts (Sec. 1.1), we have identified the following topics as knowledge gaps impeding ecosystem-based management (EBM) for Narragansett Bay.
3.2.1. Isolating the effects of nutrient reduction vs. climate change on ecosystem functioning
Two major sources of environmental uncertainty—the effects of nutrient reduction (a local-scale issue) and climate change (a global-scale issue)—are now complicating managers’ and scientists’ understanding of future trajectories in Narragansett Bay. In 2003, a fish kill occurred due to hypoxic conditions and resulted in more than one million dead fish washed ashore (Rhode Island Department of Environmental Management Citation2003). This event galvanized various stakeholders and added new urgency to addressing water quality issues in the bay, adding to many efforts that had been taking place for decades (Rhode Island Department of Environmental Management Citation2003). Subsequently, concerted efforts to reduce nutrient input into Narragansett Bay have resulted in fewer hypoxia events and improved water quality (Oviatt et al. Citation2017).
One concern, however, is the potential tradeoff that exists between nutrient reduction and overall productivity. A reduction in total suspended solids and nitrogen inputs have resulted in decreased phytoplankton productivity, particularly in the most southern area of Narragansett Bay (Borkman and Smayda Citation2016). Furthermore, Narragansett Bay has been experiencing a concurrent decrease in the magnitude and frequency of the winter-spring diatom bloom. This bloom is the signature ecological event in many northern U.S. estuaries, providing a major source of nutrient flux and benthic metabolism (Nixon et al. Citation2008; Fulweiler and Nixon Citation2009; Nixon et al. Citation2009). In recent decades, however, warmer winters associated with climate change have been tied to years when the bloom failed to occur (Oviatt et al. Citation2017), potentially due to increased zooplankton grazing (Oviatt, Keller, and Reed Citation2002).
Decreased primary productivity in Narragansett Bay, due in part to lowered nutrient inputs, may now be exacerbated by the effects of climate change. In Narragansett Bay, the temperature has risen 1.5 °C since 1950 (Fulweiler et al. Citation2015). As a result, there have been clear shifts in species assemblages with warm-water species abundance and residence times continually increasing (Oviatt et al. Citation2003; Collie, Wood, and Jeffries Citation2008). Climate impacts on organisms, ecosystems, and the services they support have increasingly been explored through climate-driven models (Brander Citation2015). Studies point to the need to investigate the interacting roles of climate change and nutrients in affecting the bay’s food web and overall human well-being (Fiksel et al. Citation2014). Various stakeholders, including commercial fishers, have voiced similar interests in understanding and mitigating the root causes of perceived losses in the bay’s fisheries, with nutrient reductions being commonly blamed (Cox, Haas, and Markey Citation2018; Schumann Citation2018).
3.2.2. Identifying ecological vs. socio-economic tradeoffs
Another major knowledge gap for managers is the need to understand the tradeoffs between harvesting a species (i.e., socio-economic benefits) and its role within the food-web and wider ecosystem (i.e., ecological benefits). For example, Atlantic menhaden (Brevoortia tyrannus), valued for its use as fish meal, fish oil, and bait for other fisheries and aquaculture farms, currently supports the largest directed fishery on the U.S. East Coast (Buchheister, Miller, and Houde Citation2017). Menhaden, however, also serve as prey for other commercially and recreationally important species including striped bass (Morone saxatilis), bluefish (Pomatomus saltatrix), and weakfish (Cynoscion regalis; Buchheister, Miller, and Houde Citation2017; McNamee Citation2018). Managing menhaden requires an understanding of its value as a harvested species against its food web role as prey for other species that have socio-economic implications.
Similarly, for wild and farmed shellfish, managers must consider the value of harvest against the value of ecosystem services such as water filtration, benthic-pelagic coupling, erosion and flood risk mitigation, and serving as habitat for other species. A major hindrance to understanding these tradeoffs is the lack of data including stakeholder perceptions and other drivers related to human behavior and interactions with ecosystem services not valued in the traditional economic marketplace (Byron, Jin, and Dalton Citation2015). While some stakeholders have expressed enthusiasm for growing Rhode Island’s aquaculture industry, others would prefer that this growth be restricted (Lord Citation2008). This underscores the need for managers and scientists to work together for continued stakeholder engagement.
An additional example of ecological and socio-economic tradeoffs relates to water quality and tourism. As discussed above, nutrient levels and temperature interact to have both ecological impacts as well as social consequences. Beach tourism and visitation goes down when water quality is poor and advisories are issued (Busch Citation2009; Pendleton et al. Citation2011). In Rhode Island, recreational users have been found to prioritize esthetic value coastal waters and when algal blooms and macroalgae such as Ulva sp. wash up on beaches, local economies that depend on tourism suffer (Dalton and Thompson Citation2013). Recently, it was found that users’ perceptions of water quality in Narragansett Bay are influenced by not only observations but also personal beliefs (Hamel, Lacasse, and Dalton Citation2021). However, there is a need to more explicitly link water quality to beach usage in order to fully understand the ways coastal resource users are impacted by and respond to changes in the bay’s water quality.
3.3. A Roadmap for future research in Narragansett Bay
In order to explore the future research priorities discussed below, it will be essential to employ a multi-model approach and link models that represent different ecosystem subcomponents. This requires an interdisciplinary team to coordinate efforts, as the different subcomponents span a variety of fields. While this is a significant undertaking, it has been successful in other locations. For example, the Alaska Climate Integrated Modeling project used a multi-model approach to characterize and forecast climate-driven changes to the eastern Bering Sea, spanning from hydrodynamics all the way up to fisheries (Hollowed et al. Citation2020). The multi-model approach taken in this project, and others (e.g., Kaplan et al. Citation2019; Lotze et al. Citation2019) provides a framework for how to evaluate ecosystem-level questions in other marine systems, such as the two research areas listed below.
3.3.1. Employ two-way model coupling to disentangle the effects of nutrient reduction vs. climate change on ecosystem functioning
Nutrient inputs and climate change are multi-scale issues. For example, nutrient input in Narragansett Bay is not limited to point-sources of wastewater outfall. Transport of waters from deep slope and shelf waters must also be considered (Kincaid, Bergondo, and Rosenberger Citation2008; Ullman et al. Citation2014), as well as nutrient transport from B. tyrannus migration into Narragansett Bay (Durbin and Durbin Citation1998). Similarly, climate change will act on multiple scales, with regional scale changes in sea-level altering local shorelines and precipitation patterns, ultimately modifying the interaction of marine and estuarine waters as well as local input of freshwater into estuaries, respectively. As we have seen in our review of past models (), one way to explicitly model multi-scale processes is to link, or couple multiple models together (Link, Fulton, and Gamble Citation2010; Byron, Jin, and Dalton Citation2015; Innes-Gold et al. Citation2021).
Ecologists have long argued for a philosophical shift away from a top-down and bottom-up dichotomy, and toward a more holistic view that both processes are significant and occurring simultaneously (Hunt and McKinnell Citation2006; Frank et al. Citation2007). Although two-way coupling is more computationally intensive, the alternative, assuming only a one-way linkage may be risky for forecasting. For example, when coupling biogeochemical lower trophic level models with higher trophic level food-web models, one approach would be to use the biogeochemistry model to simulate plankton dynamics forward in time, which is then used as input to a food-web model to simulate wider effects on higher trophic levels (Travers et al. Citation2009). This bottom-up approach (i.e., forcing), however, leaves out the potential of predation mortality to in turn affect prey (i.e., density dependence [Travers et al. Citation2009; Rose et al. Citation2010]) and other top-down effects. The major assumption, and risk, of only including one-way linkage is that the dominance and directionality of this linkage remains constant, even for unobserved, future scenarios driven by climate change.
3.3.2. Integrate human behavior into simulation models to understand ecological vs. socio-economic tradeoffs
Identifying and navigating socio-economic and ecological tradeoffs requires that sufficient data are available to explore not only how the environment and food web will respond to management scenarios, but also how people will respond. Human behavior, despite being identified as a key source of uncertainty in management (Arlinghaus, Cooke, and Potts Citation2013; Fulton et al. Citation2011), is most frequently overlooked in modeling. Therefore, better integration of human dimensions into ecosystem modeling and prediction represents one of the most relevant advances that scientists can work toward in support of EBM.
The development of agent-based modeling (ABM) has proven to be a promising approach for integrating multiple drivers of decision-making, while also embracing the unique attributes of individuals and their interactions as a means of characterizing the complexity of human behavior (An Citation2012; Milner-Gulland Citation2012). There are numerous examples from the agent-based modeling literature wherein economic considerations are used to drive human behaviors in a fisheries context (e.g., Gao and Hailu Citation2013; Tilman, Levin, and Watson Citation2018; Bailey et al. Citation2019). However, human behavior is heavily influenced by multiple non-economic factors, including existing social ties and cultural norms, as well as individual perceptions (Symes and Phillipson Citation2009). Non-economic considerations may be particularly important for Narragansett Bay and other estuaries, where recreational boating accounts for close to two thirds of all human activities (Dalton, Thompson, and Jin Citation2010).
The agent-based model of the Narragansett Bay recreational fishery focused solely on non-economic drivers of behavior and linked it to a food web model (Innes-Gold et al. Citation2021). While this model demonstrated effective methods of how to link human behavior and biological models, it was largely theoretical due to a lack of data on fisher behavior. In addition, agent-based modeling could also be used to explore the social-ecological tradeoffs relating to water quality and beach-goers. This kind of model could utilize existing data on how perceptions and beliefs influence water use (Hamel, Lacasse, and Dalton Citation2021) to quantify the socio-economic impacts of changing water quality.
3.3.3. Lessen the impact of model uncertainty by identifying robust management strategies
While all the models identified in were predictive, uncertainty in these predictions was rarely acknowledged or quantified. If uncertainty was addressed, little attention was given to communicating what this uncertainty means for managers. This uncertainty represents an ongoing communication challenge between scientists and managers.
An important strategic use for ecosystem models is in the comparison of multiple scenarios or model types (e.g., management strategy evaluation or model ensemble; Fulton Citation2010; Steele Citation2012). Managers and scientists should compare predictions across multiple models and identify management actions that are robust to uncertainty about the future (e.g., Kaplan et al. Citation2019). Thanks to increases in computing power (Lempert and Schlesinger Citation2000), quantitative decision-support methods allow for predictions to evaluate different management actions, ultimately allowing for a large ensemble of plausible scenarios to be presented to policy makers. This type of decision-support analysis has been increasingly deployed for climate change policy analysis (Lempert and Schlesinger Citation2000), including estuarine flood risk management (Wilby and Keenan Citation2012). By using models to explore the realm of possible outcomes, this type of analysis can begin to highlight management actions that would be robust against multiple potential futures.
The caveat with looking for robust strategies is that they are designed to address a range of possibilities and would likely be sub-optimal for any one particular outcome. Therefore, the implementation of robust strategies will be most effective when management frameworks are built to be flexible and adaptable (Haasnoot et al. Citation2013; Arlinghaus et al. Citation2017). Numerous examples exist that illustrate that excessively rigid management systems (Schindler and Hilborn Citation2015; Arlinghaus et al. Citation2017; Cline, Schindler, and Hilborn Citation2017) can ultimately result in lost revenue and opportunities. Ecosystems will continue to change in unpredictable ways, meaning that policymaking and implementation must balance the need for stable regulations with the need to adjust course as necessary within EBM frameworks.
4. Conclusions
The complexity of estuaries and the multiple ecosystem services they provide present considerable challenges from both a modeling and management perspective. The scope of ecosystem models has grown to include the physical, biological, and human components of the ecosystem, but to meet EBM needs, these components must not be treated as independent factors. Increasingly, experts from traditionally separated disciplines are now spanning these boundaries and giving rise to a new type of scientist who bridges and connects disciplines (Palmer Citation2018). This type of interdisciplinary collaboration will be key to address the knowledge gaps surrounding ecosystem-level change and to conduct research in a fashion that will be most useful to managers.
From our review, it is apparent that different models have been created to address different questions in Narragansett Bay. The amount of data available and previously created models opens exciting future possibilities to use model coupling to ask large-scale ecosystem questions. However, it remains important to keep in mind that the integration of ecosystem components through modeling is a means to answer a question, rather than a goal. Overall, our review of past models and current management needs has allowed us to provide general recommendations for future estuarine ecosystem modeling efforts, including a focus on interactive environmental stressors and social-ecological tradeoffs. Despite centuries of species extraction, habitat degradation, and eutrophication altering their ecosystem functioning, estuaries retain the capacity to recover and ecosystem modeling will be a powerful tool for moving toward this goal.
Acknowledgements
All authors acknowledge support from NSF EPSCoR-funded Rhode Island Consortium for Coastal Ecology Assessment, Innovation, and Modeling (RI C-AIM; NSF grant No. OIA-1655221). The funding sources did not have a role in the preparation of this manuscript. Thanks to the many colleagues who discussed these topics with us as well as to the anonymous reviewers who helped to review and improve this manuscript.
References
- Abdelrhman, M. A. 2005. Simplified modeling of flushing and residence times in 42 embayments in New England, USA, with special attention to Greenwich Bay, Rhode Island. Estuarine, Coastal and Shelf Science 62 (1–2):339–51. doi: https://doi.org/10.1016/j.ecss.2004.09.021.
- Abdelrhman, M. A. 2007. Embayment characteristic time and biology via tidal prism model. Estuarine, Coastal and Shelf Science 74 (4):742–55. doi: https://doi.org/10.1016/j.ecss.2007.05.008.
- Abdelrhman, M. A. 2016. Modeling water clarity and light quality in oceans. Journal of Marine Science and Engineering 4 (4):80. doi: https://doi.org/10.3390/jmse4040080.
- Abdelrhman, M. A. 2017. Quantifying contributions to light attenuation in estuaries and coastal embayments: Application to Narragansett Bay, Rhode Island. Estuaries and Coasts 40 (4):994–1012. doi: https://doi.org/10.1007/s12237-016-0206-x.
- Abdelrhman, M. A., and G. Cicchetti. 2012. Relationships between nutrient enrichment and benthic function: Local effects and spatial patterns. Estuaries and Coasts 35 (1):47–59. doi: https://doi.org/10.1007/s12237-011-9418-2.
- Álvarez-Romero, J. G., S. N. Wilkinson, R. L. Pressey, N. C. Ban, J. Kool, and J. Brodie. 2014. Modeling catchment nutrients and sediment loads to inform regional management of water quality in coastal-marine ecosystems: A comparison of two approaches. Journal of Environmental Management 146:164–78. doi: https://doi.org/10.1016/j.jenvman.2014.07.007.
- An, L. 2012. Modeling human decisions in coupled human and natural systems: Review of agent-based models. Ecological Modelling 229:25–36. doi: https://doi.org/10.1016/j.ecolmodel.2011.07.010.
- Anstead, K. A., K. Drew, D. Chagaris, A. M. Schueller, J. E. McNamee, A. Buchheister, G. Nesslage, J. H. Uphoff Jr., M. J. Wilberg, A. Sharov, et al. 2021. The path to an ecosystem approach for forage fish management: A case study of Atlantic menhaden. Frontiers in Marine Science 8:491. doi: https://doi.org/10.3389/fmars.2021.607657.
- Arkema, K. K., S. C. Abramson, and B. M. Dewsbury. 2006. Marine ecosystem based management: From characterization to implementation. Frontiers in Ecology and the Environment 4 (10):525–32. doi: https://doi.org/10.1890/1540-9295(2006)4[525:MEMFCT.2.0.CO;2]
- Arlinghaus, R., J. Alós, B. Beardmore, K. Daedlow, M. Dorow, M. Fujitani, D. Hühn, W. Haider, L. M. Hunt, B. M. Johnson, et al. 2017. Understanding and managing freshwater recreational fisheries as complex adaptive social-ecological systems. Reviews in Fisheries Science & Aquaculture 25 (1):1–41. doi: https://doi.org/10.1080/23308249.2016.1209160.
- Arlinghaus, R., S. J. Cooke, and W. Potts. 2013. Towards resilient recreational fisheries on a global scale through improved understanding of fish and fisher behaviour. Fisheries Management and Ecology 20 (2–3):91–8. doi: https://doi.org/10.1111/fme.12027.
- Bailey, R. M., E. Carrella, R. Axtell, M. G. Burgess, R. B. Cabral, M. Drexler, C. Dorsett, J. K. Madsen, A. Merkl, S. Saul, et al. 2019. A computational approach to managing coupled human–environmental systems: The POSEIDON model of ocean fisheries. Sustainability Science 14 (2):259–75. doi: https://doi.org/10.1007/s11625-018-0579-9.
- Berkes, F. 2012. Implementing ecosystem-based management: Evolution or revolution? Fish and Fisheries 13 (4):465–76. doi: https://doi.org/10.1111/j.1467-2979.2011.00452.x.
- Berounsky, V. M., and S. W. Nixon. 1993. Rates of nitrification along an estuarine gradient in Narragansett Bay. Estuaries 16 (4):718–30. doi: https://doi.org/10.2307/1352430.
- Boothroyd, J. C., and P. V. August. 2008. Geologic and contemporary landscapes of the Narragansett Bay ecosystem. In Science for ecosystem-based management: Narragansett Bay in the 21st century, edited by A. Desbonnet and B. Costa-Pierce, 1–34. New York: Springer Science.
- Borkman, D. G., and T. J. Smayda. 2016. Coincident patterns of waste water suspended solids reduction, water transparency increase and chlorophyll decline in Narragansett Bay. Marine Pollution Bulletin 107 (1):161–9. doi: https://doi.org/10.1016/j.marpolbul.2016.04.004.
- Boumans, R., J. Roman, I. Altman, and L. Kaufman. 2015. The multiscale integrated model of ecosystem services (MIMES): Simulating the interactions of coupled human and natural systems. Ecosystem Services 12:30–41. doi: https://doi.org/10.1016/j.ecoser.2015.01.004.
- Brander, K. 2015. Improving the reliability of fishery predictions under climate change. Current Climate Change Reports 1 (1):40–8. doi: https://doi.org/10.1007/s40641-015-0005-7.
- Brawley, J. W., M. J. Brush, J. N. Kremer, and S. W. Nixon. 2003. Potential applications of an empirical phytoplankton production model to shallow water ecosystems. Ecological Modelling 160 (1–2):55–61. doi: https://doi.org/10.1016/S0304-3800(02)00310-1.
- Brush, M. J., and J. W. Brawley. 2009. Adapting the light · biomass (BZI) models of phytoplankton primary production to shallow marine ecosystems. Journal of Marine Systems 75 (1–2):227–35. doi: https://doi.org/10.1016/j.jmarsys.2008.10.003.
- Brush, M. J., and S. W. Nixon. 2010. Modeling the role of macroalgae in a shallow sub-estuary of Narragansett Bay, RI (USA). Ecological Modelling 221 (7):1065–79. doi: https://doi.org/10.1016/j.ecolmodel.2009.11.002.
- Brush, M. J., and S. W. Nixon. 2017. A reduced complexity, hybrid empirical-mechanistic model of eutrophication and hypoxia in shallow marine ecosystems. In Modeling coastal hypoxia, edited by D. Justic, K. Rose, R. Hetland, and K. Fennel, 61–93. Cham: Springer.
- Brush, M. J., J. W. Brawley, S. W. Nixon, and J. N. Kremer. 2002. Modeling phytoplankton production: Problems with the Eppley curve and an empirical alternative. Marine Ecology Progress Series 238:31–45. doi: https://doi.org/10.3354/meps238031.
- Buchheister, A., T. J. Miller, and E. D. Houde. 2017. Evaluating ecosystem-based reference points for Atlantic Menhaden. Marine and Coastal Fisheries 9 (1):457–78. doi: https://doi.org/10.1080/19425120.2017.1360420.
- Busch, J. 2009. Surfer and beachgoer responsiveness to coastal water quality warnings. Coastal Management 37 (6):529–49. doi: https://doi.org/10.1080/08920750903044865.
- Byron, C. J., D. Jin, and T. M. Dalton. 2015. An integrated ecological-economic modeling framework for the sustainable management of oyster farming. Aquaculture 447:15–22. doi: https://doi.org/10.1016/j.aquaculture.2014.08.030.
- Byron, C. J., J. Link, B. Costa-Pierce, and D. Bengtson. 2011. Calculating ecological carrying capacity of shellfish aquaculture using mass-balance modeling: Narragansett Bay, Rhode Island. Ecological Modelling 222 (10):1743–55. doi: https://doi.org/10.1016/j.ecolmodel.2011.03.010.
- Chagaris, D., K. Drew, A. Schueller, M. Cieri, J. Brito, and A. Buchheister. 2020. Ecological reference points for Atlantic menhaden established using an ecosystem model of intermediate complexity. Frontiers in Marine Science 7:1043. doi: https://doi.org/10.3389/fmars.2020.606417.
- Chinman, R. A., and S. W. Nixon. 1985. Depth-area-volume relationships in Narragansett Bay. NOAA/Sea Grant Marine Technical Report 87. Narragansett, RI.
- Christensen, V., and C. J. Walters. 2004. Ecopath with Ecosim: Methods, capabilities and limitations. Ecological Modelling 172 (2–4):109–39. doi: https://doi.org/10.1016/j.ecolmodel.2003.09.003.
- Christensen, V., and C. J. Walters. 2005. Using ecosystem modeling for fisheries management: Where are we. ICES CM 1000 (19): 1–16.
- Christensen, V., and D. Pauly. 1992. ECOPATH II—A software for balancing steady-state ecosystem models and calculating network characteristics. Ecological Modelling 61 (3–4):169–85. doi: https://doi.org/10.1016/0304-3800(92)90016-8.
- Cline, T. J., D. E. Schindler, and R. Hilborn. 2017. Fisheries portfolio diversification and turnover buffer Alaskan fishing communities from abrupt resource and market changes. Nature Communications 8:14042–7. doi: https://doi.org/10.1038/ncomms14042.
- Collie, J. S., A. D. Wood, and H. P. Jeffries. 2008. Long-term shifts in the species composition of a coastal fish community. Canadian Journal of Fisheries and Aquatic Sciences 65 (7):1352–65. doi: https://doi.org/10.1139/F08-048.
- Cox, M. A., M. Haas, and H. Markey. 2018. Changes in Narragansett Bay. In Proceedings of the 2017 Ronald C. Baird Sea Grant Science Symposium, edited by M. A. Cox, 17. Narragansett, RI: University of Rhode Island.
- Daily, G. C., S. Polasky, J. Goldstein, P. M. Kareiva, H. A. Mooney, L. Pejchar, T. H. Ricketts, J. Salzman, and R. Shallenberger. 2009. Ecosystem services in decision making: Time to deliver. Frontiers in Ecology and the Environment 7 (1):21–8. doi: https://doi.org/10.1890/080025.
- Dalton, T., and R. Thompson. 2013. Recreational boaters’ perceptions of scenic value in Rhode Island coastal waters. Ocean & Coastal Management 71:99–107. doi: https://doi.org/10.1016/j.ocecoaman.2012.10.002.
- Dalton, T., R. Thompson, and D. Jin. 2010. Mapping human dimensions in marine spatial planning and management: An example from Narragansett Bay, Rhode Island. Marine Policy 34 (2):309–19. doi: https://doi.org/10.1016/j.marpol.2009.08.001.
- Desbonnet, A., and V. Lee. 1991. Historical trends: Water quality and fisheries, Narragansett Bay. The University of Rhode Island Coastal Resources Center Contribution No. 100 and National Sea Grant Publication #RIU-T-90-001. Narragansett, RI.
- Doering, P. H., and C. A. Oviatt. 1986. Application of filtration rate models to field populations of bivalves: An assessment using experimental mesocosms. Marine Ecology Progress Series 31:265–75. doi: https://doi.org/10.3354/meps031265.
- Dreyfus-León, M. J. 1999. Individual-based modelling of fishermen search behaviour with neural networks and reinforcement learning. Ecological Modelling 120:287–97.
- Durbin, A. G., and E. G. Durbin. 1998. Effects of menhaden predation on plankton populations in Narragansett Bay, Rhode Island. Estuaries 21 (3):449–65. doi: https://doi.org/10.2307/1352843.
- Elliott, M., and A. K. Whitfield. 2011. Challenging paradigms in estuarine ecology and management. Estuarine, Coastal and Shelf Science, 94 (4):306–314.
- Fiksel, J., R. Bruins, A. Gatchett, A. Gilliland, and M. T. Brink. 2014. The triple value model: A systems approach to sustainable solutions. Clean Technologies and Environmental Policy 16:691–702.
- Frank, K. T., B. Petrie, L. Nancy, and N. L. Shackell. 2007. The ups and downs of trophic control in continental shelf ecosystems. Trends in Ecology & Evolution 22 (5):236–42. doi: https://doi.org/10.1016/j.tree.2007.03.002.
- Fulton, E. A. 2010. Approaches to end-to-end ecosystem models. Journal of Marine Systems 81 (1–2):171–83. doi: https://doi.org/10.1016/j.jmarsys.2009.12.012.
- Fulton, E. A., A. D. M. Smith, D. C. Smith, and I. E. Van Putten. 2011. Human behaviour: The key source of uncertainty in fisheries management. Fish and Fisheries 12 (1):2–17. doi: https://doi.org/10.1111/j.1467-2979.2010.00371.x.
- Fulweiler, R. W., A. J. Oczkowski, K. M. Miller, C. A. Oviatt, and M. E. Q. Pilson. 2015. Whole truths vs. half truths - And a search for clarity in long-term water temperature records. Estuarine, Coastal and Shelf Science 157:A1–A6. doi: https://doi.org/10.1016/j.ecss.2015.01.021.
- Fulweiler, R. W., and S. W. Nixon. 2009. Responses of benthic-pelagic coupling to climate change in a temperate estuary. In Eutrophication in coastal ecosystems, edited by J. H. Anderson and D. J. Conley, 147–56. Dordrecht: Springer.
- Gao, H., and A. Hailu. 2013. Identifying preferred management options: An integrated agent-based recreational fishing simulation model with an AHP-TOPSIS evaluation method. Ecological Modelling 249:75–83. doi: https://doi.org/10.1016/j.ecolmodel.2012.07.002.
- Haasnoot, M., J. H. Kwakkel, W. E. Walker, and J. ter Maat. 2013. Dynamic adaptive policy pathways: A method for crafting robust decisions for a deeply uncertain world. Global Environmental Change 23 (2):485–98. doi: https://doi.org/10.1016/j.gloenvcha.2012.12.006.
- Hamburg, S. P., Pryor, D., and M. A. Vadeboncoeur. 2008. Nitrogen inputs to Narragansett Bay: An historical perspective. In Science for Ecosystem-based Management (pp. 177–210). Springer, New York, NY.
- Hamel, K., K. Lacasse, and T. Dalton. 2021. Recreational users’ perceptions of coastal water quality in Rhode Island (USA): Implications for policy development and management. Marine Pollution Bulletin 172:112810. doi: https://doi.org/10.1016/j.marpolbul.2021.112810.
- Herndon, R. W., and E. W. Sekatau. 1997. The right to a name: The Narragansett people and Rhode Island officials in the revolutionary era. Ethnohistory 433–462.
- Holland, D. S., and J. G. Sutinen. 1999. An empirical model of fleet dynamics in New England trawl fisheries. Canadian Journal of Fisheries and Aquatic Sciences 56 (2):253–64. doi: https://doi.org/10.1139/f98-169.
- Hollowed, A. B., K. K. Holsman, A. C. Haynie, A. J. Hermann, A. E. Punt, K. Aydin, et al. 2020. Integrated modeling to evaluate climate change impacts on coupled social-ecological systems in Alaska. Frontiers in Marine Science 14 (6):775.
- Hunt, G. L., and S. McKinnell. 2006. Interplay between top-down, bottom-up, and wasp-waist control in marine ecosystems. Progress in Oceanography 68 (2–4):115–24. doi: https://doi.org/10.1016/j.pocean.2006.02.008.
- Innes-Gold, A., M. Heinichen, K. Gorospe, C. Truesdale, J. Collie, and A. Humphries. 2020. Modeling 25 years of food web changes in Narragansett Bay as a tool for ecosystem-based management. Marine Ecology Progress Series 654:17–33. doi: https://doi.org/10.3354/meps13505.
- Innes-Gold, A., T. Pavlowich, M. Heinichen, M. C. McManus, J. McNamee, J. Collie, and A. Humphries. 2021. Exploring social-ecological trade-offs in fisheries using a coupled food web and human behavior model. Ecology and Society 26 (2):40. doi: https://doi.org/10.5751/ES-12451-260240.
- Ji, Z. G. 2017. Hydrodynamics and water quality: Modeling rivers, lakes, and estuaries. Hoboken: Wiley.
- Kaplan, I. C., T. B. Francis, A. E. Punt, L. E. Koehn, E. Curchitser, F. Hurtado-Ferro, K. F. Johnson, S. E. Lluch-Cota, W. J. Sydeman, T. E. Essington, et al. 2019. A multi-model approach to understanding the role of Pacific sardine in the California Current food web. Marine Ecology Progress Series 617–618:307–21. doi: https://doi.org/10.3354/meps12504.
- Kincaid, C., D. L. Bergondo, and K. Rosenberger. 2008. The dynamics of water exchange between Narragansett Bay and Rhode Island sound. In Science for ecosystem-based management: Narragansett Bay in the 21st century, edited by A. Desbonnet and B. A. Costa-Pierce. New York: Springer Science; 301–24.
- Kremer, J. N., and P. Kremer. 1982. A three-trophic level estuarine model: Synergism of two mechanistic simulations. Ecological Modelling 15 (2):145–57. doi: https://doi.org/10.1016/0304-3800(82)90058-8.
- Kremer, J. N., and S. W. Nixon. 1978. A coastal marine ecosystem: Simulation and analysis. Berlin: Springer-Verlag.
- Kremer, J. N., J. M. P. Vaudrey, D. S. Ullman, D. L. Bergondo, N. LaSota, C. Kincaid, D. L. Codiga, and M. J. Brush. 2010. Simulating property exchange in estuarine ecosystem models at ecologically appropriate scales. Ecological Modelling 221 (7):1080–8. doi: https://doi.org/10.1016/j.ecolmodel.2009.12.014.
- Latour, R. J., M. J. Brush, and C. F. Bonzek. 2003. Toward ecosystem-based fisheries management: Strategies for multispecies modeling and associated data requirements. Fisheries 28 (9):10–22. doi: https://doi.org/10.1577/1548-8446(2003)28[10:TEFM.2.0.CO;2]
- Lempert, R. J., and M. E. Schlesinger. 2000. Robust strategies for abating climate change. Climatic Change 45 (3/4):387–401. doi: https://doi.org/10.1023/A:1005698407365.
- Levin, S. A., and J. Lubchenco. 2008. Resilience, robustness, and marine ecosystem-based management. BioScience 58 (1):27–32. doi: https://doi.org/10.1641/B580107.
- Link, J. S., and H. I. Browman. 2014. Integrating what? Levels of marine ecosystem-based assessment and management. ICES Journal of Marine Science 71 (5):1170–3. doi: https://doi.org/10.1093/icesjms/fsu026.
- Link, J. S., E. A. Fulton, and R. J. Gamble. 2010. The northeast US application of ATLANTIS: A full system model exploring marine ecosystem dynamics in a living marine resource management context. Progress in Oceanography 87 (1–4):214–34. doi: https://doi.org/10.1016/j.pocean.2010.09.020.
- Link, J. S., T. F. Ihde, C. J. Harvey, S. K. Gaichas, J. C. Field, J. K. T. Brodziak, H. M. Townsend, and R. M. Peterman. 2012. Dealing with uncertainty in ecosystem models: The paradox of use for living marine resource management. Progress in Oceanography 102:102–14. doi: https://doi.org/10.1016/j.pocean.2012.03.008.
- Lord, P. 2008. Limit aquaculture to 5 percent R.I. waters, experts say. Providence Journal.
- Lotze, H. K., H. S. Lenihan, B. J. Bourque, R. H. Bradbury, R. G. Cooke, M. C. Kay, S. M. Kidwell, M. X. Kirby, C. H. Peterson, and J. B. C. Jackson. 2006. Depletion, degradation, and recovery potential of estuaries and coastal seas. Science (New York, N.Y.) 312 (5781):1806–9. doi: https://doi.org/10.1126/science.1128035.
- Lotze, H. K., D. P. Tittensor, A. Bryndum-Buchholz, T. D. Eddy, W. W. L. Cheung, E. D. Galbraith, M. Barange, N. Barrier, D. Bianchi, J. L. Blanchard, et al. 2019. Global ensemble projections reveal trophic amplification of ocean biomass declines with climate change. Proceedings of the National Academy of Sciences of the United States of America 116 (26):12907–12. doi: https://doi.org/10.1073/pnas.1900194116.
- Rhode Island Department of Environmental Management. 2003. The Greenwich Bay fish kill – August 2003: Causes, impacts and responses. Providence, RI DEM. http://www.dem.ri.gov/programs/benviron/water/bart/fishkill-greenwich-bay-2003.pdf.
- Mango, L. M., A. M. Melesse, M. E. McClain, D. Gann, and S. G. Setegn. 2011. Land use and climate change impacts on the hydrology of the upper Mara River Basin, Kenya: Results of a modeling study to support better resource management. Hydrology and Earth System Sciences 15 (7):2245–58. doi: https://doi.org/10.5194/hess-15-2245-2011.
- McLeod, K., J. Lubchenco, S. Palumbi, and A. A. Rosenberg. 2005. Scientific consensus statement on marine ecosystem-based management.
- Mcmanus, M. C., D. S. Ullman, S. D. Rutherford, and C. Kincaid. 2020. Northern quahog (Mercenaria mercenaria) larval transport and settlement modeled for a temperate estuary. Limnology and Oceanography 65 (2):289–15. doi: https://doi.org/10.1002/lno.11297.
- McNamee, J. E. 2018. A multispecies statistical catch-at-age (MSSCAA) model for a mid-Atlantic species complex. Open Access Dissertations, Paper 758: 288.
- Milner-Gulland, E. J. 2012. Interactions between human behaviour and ecological systems. Philosophical Transactions of the Royal Society of London, Series B: Biological Sciences 367 (1586):270–8. doi: https://doi.org/10.1098/rstb.2011.0175.
- Monaco, M. E., and R. E. Ulanowicz. 1997. Comparative ecosystem trophic structure of three US mid-Atlantic estuaries. Marine Ecology Progress Series 161:239–254.
- Nixon, S. W. 1995. Coastal marine eutrophication: A definition, social causes, and future concerns. Ophelia 41 (1):199–219. doi: https://doi.org/10.1080/00785236.1995.10422044.
- Nixon, S. W. 1997. Prehistoric nutrient inputs and productivity in Narragansett Bay. Estuaries 20 (2):253–61. doi: https://doi.org/10.2307/1352341.
- Nixon, S. W., B. A. Buckley, S. L. Granger, L. A. Harris, A. J. Oczkowski, R. W. Fulweiler, et al. 2008. Nitrogen and phosphorus inputs to Narragansett Bay: Past, present, and future. In Science for ecosystem-based management: Narragansett Bay in the 21st century, edited by A. Desbonnet and B. A. Costa-Pierce, 101–75. New York: Springer Science.
- Nixon, S. W., R. W. Fulweiler, B. A. Buckley, S. L. Granger, B. L. Nowicki, and K. M. Henry. 2009. The impact of changing climate on phenology, productivity, and benthic-pelagic coupling in Narragansett Bay. Estuarine, Coastal and Shelf Science 82 (1):1–18. doi: https://doi.org/10.1016/j.ecss.2008.12.016.
- Nixon, S. W., S. L. Granger, and B. L. Nowicki. 1995. An assessment of the annual mass balance of carbon, nitrogen, and phosphorus in Narragansett Bay. Biogeochemistry 31 (1):15–61. doi: https://doi.org/10.1007/BF00000805.
- Oczkowski, A., C. Schmidt, E. Santos, K. Miller, A. Hanson, D. Cobb, J. Krumholz, A. Pimenta, L. Heffner, S. Robinson, et al. 2018. How the distribution of anthropogenic nitrogen has changed in Narragansett Bay (RI, USA) following major reductions in nutrient loads. Estuaries and Coasts 41 (8):2260–76. doi: https://doi.org/10.1007/s12237-018-0435-2.
- Olsen, E., G. Fay, S. Gaichas, R. Gamble, S. Lucey, and J. S. Link. 2016. Ecosystem model skill assessment. Yes we can!. PLoS One 11 (1):e0146467. doi: https://doi.org/10.1371/journal.pone.0146467.
- Oviatt, C. A., A. Keller, and L. Reed. 2002. Annual primary production in Narragansett Bay with no bay-wide winter-spring phytoplankton bloom. Estuarine, Coastal and Shelf Science 54 (6):1013–26. doi: https://doi.org/10.1006/ecss.2001.0872.
- Oviatt, C. A., S. Olsen, M. Andrews, J. Collie, T. Lynch, and K. Raposa. 2003. A century of fishing and fish fluctuations in Narragansett Bay. Reviews in Fisheries Science 11 (3):221–42. doi: https://doi.org/10.1080/10641260390244413.
- Oviatt, C., L. Smith, J. Krumholz, C. Coupland, H. Stoffel, A. Keller, M. C. McManus, and L. Reed. 2017. Managed nutrient reduction impacts on nutrient concentrations, water clarity, primary production, and hypoxia in a north temperate estuary. Estuarine, Coastal and Shelf Science 199:25–34. doi: https://doi.org/10.1016/j.ecss.2017.09.026.
- Palmer, L. 2018. Meeting the leadership challenges for interdisciplinary environmental research. Nature Sustainability 1 (7):330–3. doi: https://doi.org/10.1038/s41893-018-0103-3.
- Park, G. S., and H. G. Marshall. 2000. Estuarine relationships between zooplankton community structure and trophic gradients. Journal of Plankton Research 22 (1):121–36. doi: https://doi.org/10.1093/plankt/22.1.121.
- Pendleton, L., P. King, C. Mohn, D. G. Webster, R. Vaughn, and P. N. Adams. 2011. Estimating the potential economic impacts of climate change on Southern California beaches. Climatic Change 109 (1):277–298.
- Pilson, M. E. Q. 1985. On the residence time of water in Narragansett Bay. Estuaries 8 (1):2. doi: https://doi.org/10.2307/1352116.
- Rashleigh, B., H. Walker, T. Gleason, M. Abdelrhman, L. Charlestra, E. Dettman, et al. 2015. Quantitative models describing past and current nutrient fluxes and associated ecosystem level responses in the Narragansett Bay Ecosystem. U.S. Environmental Protection Agency, Washington, DC, EPA/600/R-15/174, 1–50.
- Rose, K. A., J. I. Allen, Y. Artioli, M. Barange, J. Blackford, F. Carlotti, R. Cropp, U. Daewel, K. Edwards, K. Flynn, et al. 2010. End-to-end models for the analysis of marine ecosystems: Challenges, issues, and next steps. Marine and Coastal Fisheries 2 (1):115–30. doi: https://doi.org/10.1577/C09-059.1.
- Santschi, P. H., M. Amdurer, D. Adler, P. O’Hara, Y.-H. Li, and P. Doering. 1987. Relative mobility of radioactive trace-elements across the sediment-water interface in the MERL model-ecosystems of Narragansett Bay. Journal of Marine Research 45 (4):1007–1048. doi: https://doi.org/10.1357/002224087788327172.
- Santschi, P. H., Y. H. Li, D. M. Adler, M. Amdurer, J. Bell, and U. P. Nyffeler. 1983. The relative mobility of natural (Th, Pb and Po) and fallout (Pu, Am, Cs) radionuclides in the coastal marine environment: Results from model ecosystems (MERL) and Narragansett Bay. Geochimica et Cosmochimica Acta 47 (2):201. doi: https://doi.org/10.1016/0016-7037(83)90133-3.
- Schindler, D. E., and R. Hilborn. 2015. Sustainability. Prediction, precaution, and policy under global change. Science (New York, N.Y.) 347 (6225):953–954. doi: https://doi.org/10.1126/science.1261824.
- Schmidt, C. E., E. Monroy, M. Cantwell, J. King, and C. Roman. 2020. A synthesis of case studies from Narragansett Bay (RI/MA, USA) emphasizing implementation of the US Clean Water Act using adaptive management. NBEP Technical Report 2020. NBEP-20-228. http://nbep.org/publications/synthesis-report.pdf.
- Schumann, S. 2018. Commercial fisheries resilience planning: A tool for industry empowerment. http://resilientfisheriesri.org/planning-guide/.
- Shull, D. H. 2001. Transition-matrix model of bioturbation and radionuclide diagenesis. Limnology and Oceanography 46 (4):905–916. doi: https://doi.org/10.4319/lo.2001.46.4.0905.
- Soetaert, K. E. R., and P. Van Rijswijk. 1993. Spatial and temporal patterns of the zooplankton in the Westerschelde estuary. Marine Ecology Progress Series 97:47–59. doi: https://doi.org/10.3354/meps097047.
- Soulié, J. C., and O. Thébaud. 2006. Modeling fleet response in regulated fisheries: An agent-based approach. Mathematical and Computer Modelling 44 (5–6):553–564. doi: https://doi.org/10.1016/j.mcm.2005.02.011.
- Steele, J. H. 2012. Prediction, scenarios and insight: The uses of an end-to-end model. Progress in Oceanography 102:67–73. doi: https://doi.org/10.1016/j.pocean.2012.03.005.
- Symes, D., and J. Phillipson. 2009. Whatever became of social objectives in fisheries policy? Fisheries Research 95 (1):1–5. doi: https://doi.org/10.1016/j.fishres.2008.08.001.
- Telesh, I. V., and V. V. Khlebovich. 2010. Principal processes within the estuarine salinity gradient: A review. Marine Pollution Bulletin 61 (4–6):149–155. doi: https://doi.org/10.1016/j.marpolbul.2010.02.008.
- Tilman, A., S. Levin, and J. Watson. 2018. Revenue-sharing clubs provide economic insurance and incentives for sustainability in common-pool resource systems. Journal of Theoretical Biology 454:205–214. doi: https://doi.org/10.1016/j.jtbi.2018.06.003.
- Travers, M., Y.-J. Shin, S. Jennings, E. Machu, J. A. Huggett, J. G. Field, and P. M. Cury. 2009. Two-way coupling versus one-way forcing of plankton and fish models to predict ecosystem changes in the Benguela. Ecological Modelling 220 (21):3089–3099. doi: https://doi.org/10.1016/j.ecolmodel.2009.08.016.
- Ullman, D. S., D. L. Codiga, A. Pfeiffer-Herbert, and C. R. Kincaid. 2014. An anomalous near-bottom cross-shelf intrusion of slope water on the southern New England continental shelf. Journal of Geophysical Research: Oceans 119 (3):1739–1753. doi: https://doi.org/10.1002/2013JC009259.
- Ullman, D. S., C. Kincaid, C. Balt, and D. L. Codiga. 2019. Hydrodynamic modeling of Narragansett Bay in support of the EcoGEM ecological model. University of Rhode Island GSO technical report 2019 Jan. Report no. 2019–01.
- Vadeboncoeur, M. A., S. P. Hamburg, and D. Pryor. 2010. Modeled nitrogen loading to Narragansett Bay: 1850 to 2015. Estuaries and Coasts 33 (5):1113–1127. doi: https://doi.org/10.1007/s12237-010-9320-3.
- Vaudrey, J. M. P. EcoGEM: Modeling response of hypoxia to changes in nutrient inputs to Narragansett Bay, RI, USA. University of Connecticut Department of Marine Sciences technical report. 2016. Prepared for NOAA-CHRP.
- Villa, F., K. Bagstad, G. Johnson, and B. Voigt. 2011. Scientific instruments for climate change adaptation: estimating and optimizing the efficiency of ecosystem service provision.
- Wilby, R. L., and R. Keenan. 2012. Adapting to flood risk under climate change. Progress in Physical Geography: Earth and Environment 36 (3):348–378. doi: https://doi.org/10.1177/0309133312438908.