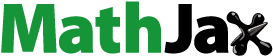
ABSTRACT
Concerns about a crisis of mass irreplicability across scientific fields (“the replication crisis”) have stimulated a movement for open science, encouraging or even requiring researchers to publish their raw data and analysis code. Recently, a rule at the US Environmental Protection Agency (US EPA) would have imposed a strong open data requirement. The rule prompted significant public discussion about whether open science practices are appropriate for fields of environmental public health. The aims of this paper are to assess (1) whether the replication crisis extends to fields of environmental public health; and (2) in general whether open science requirements can address the replication crisis. There is little empirical evidence for or against mass irreplicability in environmental public health specifically. Without such evidence, strong claims about whether the replication crisis extends to environmental public health – or not – seem premature. By distinguishing three concepts – reproducibility, replicability, and robustness – it is clear that open data initiatives can promote reproducibility and robustness but do little to promote replicability. I conclude by reviewing some of the other benefits of open science, and offer some suggestions for funding streams to mitigate the costs of adoption of open science practices in environmental public health.
Introduction
Over the past decade, the scientific community has focused a great deal of attention on an ongoing epistemic crisis in which experimental studies fail to replicate much more often than would be expected. Often called “the replication crisis,” this crisis of mass irreplicability has unfolded primarily in social psychology and preclinical biomedical research (Spellman Citation2015; Harris Citation2017); but researchers in many fields are concerned that the problem might extend to their own areas (Lash, Collin, and Van Dyke Citation2018). One common response to the replication crisis has been to call for open science. For example, the Transparency and Openness Promotion (TOP) Guidelines (Nosek et al. Citation2015) have been adopted by many major academic publishers, including the American Association for the Advancement of Science (AAAS), Springer Nature, and the Public Library of Science (PLoS). (Lash Citation2015 explains why the journal Epidemiology declined to adopt the TOP guidelines.) At the highest level of stringency, the TOP Guidelines require data, code, and study materials to be “posted to a trusted repository” and “reported analyses … reproduced independently before publication” (Nosek et al. Citation2015, 1424). While the term “open science” is understood in a variety of different ways (Levin et al. Citation2016), in this paper I focus on open science in the sense of the publication of a study’s data and code in a more-or-less publicly available venue and format.
In the fields of environmental public health, recent discussions of open science have precipitated around “Strengthening Transparency in Regulatory Science,” a rule first proposed by the US Environmental Protection Agency (US EPA) in 2018 (US EPA Citation2018b; henceforth Strengthening Transparency). This rule would have required US EPA to ensure that the “data and models underlying pivotal regulatory science are publicly available in a manner sufficient for independent validation” (US EPA Citation2018b, 18,773).Footnote1 The rule was highly controversial. Critics of Strengthening Transparency argued that the data from many public health studies cannot be made publicly available without violating the privacy of study participants, and so Strengthening Transparency would undermine the evidence base for numerous regulations (Cornwall Citation2018). These critics included editors of scientific journals as well as high-profile proponents of open science (Berg et al. Citation2018; Ioannidis Citation2018; Nosek Citation2019; Boronow et al. Citation2020).
Other critics of Strengthening Transparency noted that industry and its allies have long used calls for access to “raw data” to attempt to delay regulation (Lerner Citation2017; Dockery and Pope Citation2020).Footnote2 Readers may be familiar with the Six Cities study (Dockery et al. Citation1993), which provided key evidence used by US EPA to justify more stringent regulation of PM emissions. After pressure from industry, a third-party reanalysis of the Six Cities data – checking both reproducibility and robustness, in the terminology I introduce below – confirmed its findings (Kaiser Citation1997; Krewski et al. Citation2005b, Citation2005a). Nonetheless, decades later, critics affiliated with the fossil fuels industry continue to complain that the data are not publicly available (Milloy Citation2019). There has been similar controversy over an epidemiological study of chlorpyrifos (US EPA Citation2018a), which continued even after Columbia University offered to “allow EPA staff to review and/or re-analyze the original individual-level data in a secure data enclave onsite at Columbia” (letter from Dean Linda Fried dated 18 May 2016). In the 2010s, these ad hoc industry efforts coalesced into a legislative strategy. Republican members of the US Congress repeatedly introduced a bill, variously called the Secret Science Act or HONEST Act, that would have imposed a strong open data requirement on US EPA. After these bills repeatedly failed, in January 2018 Representative Lamar Smith worked with Scott Pruitt, then administrator of US EPA, to develop Strengthening Transparency (Waldman and Farah Citation2018).
Both the Notice of Proposed Rulemaking (NPR) for Strengthening Transparency and supportive commentary justified the need for a strong open science requirement at US EPA by appealing to the replication crisis (US EPA Citation2018b, 18,770; Lewis Citation2020; Richardson Citation2021). The NPR uses this phrase explicitly, giving citations to major commentaries on the crisis including Munafò et al. (Citation2017), Ioannidis (Citation2005), McNutt (Citation2014), and Goodman, Fanelli, and Ioannidis (Citation2016). This argument for Strengthening Transparency involves two key claims: first, that there is a widespread crisis of mass irreplicability; and second, that open data requirementsFootnote3 will help solve the crisis. Both claims were taken from the replication crisis discourse, and indeed proponents of Strengthening Transparency frequently cited major proponents of open science (including Nosek and Ioannidis, whose criticisms of Strengthening Transparency were cited above). However, despite talk of a general or widespread crisis in science, the evidence in the replication crisis discourse primarily comes from either social psychology or preclinical biomedical research. For example, Munafò et al. (Citation2017) write that “Data from many fields suggests reproducibility is lower than is desirable” (Munafò et al. Citation2017, 1, emphasis mine), supporting this claim with 8 citations. But 6 of the 8 were published in the Lancet, 7 are specific to preclinical biomedical research, and the last was a survey of researchers’ perceptions of whether there was a replication crisis. Similarly, writing in support of Strengthening Transparency, Lewis (Citation2020) cites an article in the Economist, which reviews findings of replication problems in social psychology and preclinical biomedical research along with a handful of individual cases in physics and economics. It’s hard to see how broad generalizations across scientific fields can be supported by such a narrow base of evidence. Specifically, evidence of mass irreplicability in the specific fields of social psychology and preclinical biomedical research doesn’t provide support for claims of mass irreplicability in environmental public health. Apparently overlooking this evidential gap, proponents of Strengthening Transparency rarely provided evidence that the replication crisis extends to environmental public health.
The adoption of open science – whether as a recommendation or requirement – would not be a trivial undertaking for the environmental public health research community. Fields such as molecular biology and environmental science have led the way in developing technologies to disseminate public data and code, and to create secure enclaves for sharing sensitive data. There are also well-developed standards for open data, such as FAIR (findable, accessible, interoperable, and reusable; Wilkinson et al. Citation2016). But many environmental public health researchers are not familiar with these novel technologies and standards, which have substantial learning curves. Simply posting files on a public repository is not sufficient: both data and code need to be documented, so that others can understand how the data are organized, what the code is supposed to do and how to run it, what additional software needs to be installed first, and how outputs are intended to relate to the content of a paper. Specifically, Wilson et al. (Citation2014) list 24 high-level principles of software engineering that, they argue, should be adopted by researchers using computationally intensive methods. Recognizing that these principles are too advanced for many researchers, Wilson et al. (Citation2017) provide a list of 28 practices comprising “a minimum set of tools and techniques” for computationally intensive research (2). Outside of software engineering, I suspect that very few graduate programs teach their students any of these 52 principles, tools, or techniques. In addition, data and code repositories require regular maintenance, requiring other specific skills and resources (Leonelli Citation2016; Powell Citation2021). Without support for training and maintenance, strong open science requirements would likely significantly slow environmental public health research, with downstream impacts on the ability of regulatory agencies to develop protective, science-based regulation. Given these substantial costs, it’s worthwhile to consider whether Strengthening Transparency or similar open science requirements would actually solve a genuine problem in environmental public health.
In this essay, I critically examine both key claims in the argument for Strengthening Transparency. I first introduce a distinction among three kinds of evidence for mass irreplicability – a priori mathematical models, and indirect vs. direct empirical evidence – and examine the state of available evidence that environmental public health might be afflicted by the mass irreplicability that has appeared in other scientific fields. I find that this evidence has not been systematically collected, and what evidence is available is weak and does not support claims that the replication crisis extends to environmental public health. Next, I distinguish three concepts – reproducibility, replicability, and robustness – and consider generally whether open science requirements can effectively promote replicability. I argue that, while open science can promote reproducibility and robustness, it is unlikely to promote replicability. So, even if the replication crisis does extend to environmental public health, a strong open science requirement does not seem to be an appropriate response.
In the Conclusion, I step back from the debate over Strengthening Transparency. Proponents of open science have identified other benefits beyond (purportedly) addressing the replication crisis, and some critics of industry-sponsored science in environmental policy have argued that an open data requirement might address problems with industry-funded research and the misuse of confidential business information (CBI) designations. These points suggest that adopting open science practices in environmental public health might have substantial benefits. After reviewing these arguments in favor of open science, I offer some recommendations for funding streams to support open science practices within environmental public health that would mitigate the challenges and costs associated with open science.
A replication crisis in environmental public health?
In this section, I consider the first key claim in the argument for Strengthening Transparency: that there is a widespread crisis of mass irreplicability, and specifically that this crisis extends to environmental public health. I distinguish among three kinds of evidence for mass irreplicability, and argue that, for fields of environmental public health, this evidence has not been systematically collected, and what evidence is available is weak and does not support claims that the replication crisis extends to these fields.
It is worth considering at the outset that replication might not be an appropriate standard for many areas of environmental public health. Leonelli (Citation2018) notes that, as an epistemic ideal, replication comes from 19th-century laboratory-based physical sciences, such as chemistry and physics. In these fields, it’s relatively easy to design an experimental setup that includes the single causal process of interest, to isolate that process from external influences, and to expect that process to work in exactly the same way in every other appropriately equipped lab. Given these assumptions, it’s reasonable to expect the same experimental setup to produce similar results time after time. (Below, I’ll give more precise definitions of replicability, reproducibility, and robustness.) However, isolating a single causal processes from all outside influences is more challenging in fields of laboratory-based biology (e.g., toxicology), and still more challenging for observational studies that use “opportunistic” data – such as patient medical records – over which the researchers have little or no control. While randomized controlled trials (RCTs) are often regarded as the “gold standard” of biomedical research, they may be impractical or even epistemically suboptimal for some research questions in environmental public health (Naumova Citation2017; Fernández Pinto and Hicks Citation2019, 3). In addition, the complex interactions between environmental, physiological, and social processes may mean that causal relationships vary across time, space, or social groups. For example, accounting for interactions of mixtures of chemicals is a longstanding challenge in toxicology (Rider et al. Citation2021); and structural racism may mean that communities of color have both greater exposure to pollutants and poorer access to health care, making race or ethnicity a statistical moderator of the relationship between exposures and outcomes (Chay and Greenstone Citation2003; Mohai, Pellow, and Roberts Citation2009, 423; Jorgenson et al. Citation2020).
If these kinds of complexities are common in environmental public health, and researchers have limited understanding of and ability to account for them, then rigorous and accurate studies in environmental public health might appear to “fail to replicate” much more often than expected. That is, complexity could produce the appearance of a replication crisis. But this would be misleading. It’s not that the results of such “irreplicable” studies are false. Rather, the phenomena that they identify have a limited scope or generalizability, and it doesn’t make sense to talk about superficially similar studies conducted outside that scope as “replications” in the first place. In this sense, the concept of replication might be inappropriate. Instead of attempting “replication” after “replication,” a more appropriate aim for follow-up studies would be to delim the boundaries of this limited scope – where and why does this phenomenon appear or not? (Feest Citation2019 suggests this kind of approach for social psychology.)
However, I take it that typically both environmental public health researchers and policymakers who use their results expect research findings to be highly generalizable and replicable. So, for the remainder of this paper, I set aside the arguments of the last two paragraphs, and instead assume that mass irreplicability would be a serious epistemic problem for any field of environmental public health.
The first kind of evidence for mass irreplicability comes from a priori (non-empirical) mathematical models of scientific research. Sterne and Smith (Citation2001), Ioannidis (Citation2005), Lash (Citation2017), and Bartell (Citation2019) use slightly different versions of the same model, calculating the positive predictive value (PPV) of a statistically significant finding given a range of assumptions about study power and the “pre-study odds” or “prevalence” of true hypotheses. These authors conclude from this kind of model that, as Ioannidis titles one section, “Most Research Findings are False for Most Research Designs and for Most Fields” (Ioannidis Citation2005, 0699). Other examples of a priori models draw on mathematical models of biological evolution, including evolutionary game theory, to model various epistemic-social aspects of research communities (Smaldino and McElreath Citation2016; Zollman Citation2007; Bright Citation2017; Holman and Bruner Citation2017; Weatherall, O’Connor, and Bruner Citation2018).
It’s often not clear how well a priori models support claims about real-world scientific practice. These models might be more useful for characterizing potential causes of phenomena (“how-possibly” explanations) and suggesting potential interventions, but less useful for supporting claims about the existence of phenomena (for example, that there is mass irreplicability in a particular scientific field). There are at least three challenges when trying to extrapolate from a priori models of research systems to real-world systems. First, the findings supported by these models depend on their parameter values, and these parameters are often not supported with any empirical evidence (Martini and Pinto Citation2016). For example, one PPV model paper (Bartell Citation2019) cites 4 different works to support 6 different estimates for the “prevalence” of true hypotheses in various areas. Two of these citations are for previous PPV studies that, in turn, provide no empirical justification for the values they use (Ioannidis Citation2005; Lash Citation2017). The third citation justifies its choice of values simply by positing that “by 1985 nearly 300 risk factors for coronary heart disease had been identified, and it is unlikely that more than a small fraction of these actually increase the risk of the disease” (Sterne and Smith Citation2001, 1465–66). The final citation is a brief summary of drug approvals made by the US Food and Drug Administration in 2016, and it is not clear what information in this summary is supposed to support the cited value (Mullard Citation2017). As Bartell puts it, “These are mostly educated guesses” (Bartell Citation2019). A second challenge with a priori models is that it might not clear how some parameters could be operationalized and measured empirically at all. For example, the specific model used in Ioannidis (Citation2005) assumes that 30% of scientific research is produced only due to “bias,” defined as “the combination of various design, data, analysis, and presentation factors that tend to produce research findings when they should not be produced” (Ioannidis Citation2005, 0697; Goodman and Greenland Citation2007). A third difficulty is that, like all models, these models involve various simplifications and idealizations in the way they represent the research process (Potochnik Citation2017; Smaldino Citation2019). For example, models based on statistical hypothesis testing generally assume that every study is designed to test a single hypothesis (often itself simplified, such as a dichotomous effect/no effect hypothesis). Actual observational research may have other, more complex goals, such as estimating the strength of an exposure–outcome relationship or trying to understand heterogeneity in exposure–outcome relationships across space, time, or social groups. Whether a model’s idealizations are acceptable depends on the specific use being made of the model (Parker Citation2020); and researchers can reasonably disagree about the acceptability of particular idealizations. In short, an a priori mathematical model on its own does not provide much evidence that there is an actual crisis of mass irreplicability. Parameter values need to be supported with empirical evidence, and modelers need to address the question of whether their simplifying assumptions can survive extrapolation to complex real-world cases.
The second kind of evidence of mass irreplicability is indirect empirical evidence, based on statistical analyses of findings reported in primary studies and intended to detect “questionable research practices” such as low power or p-hacking. For example, Dumas-Mallet et al. (Citation2017) examined meta-analyses for 10 diseases across three domains (neurological, psychiatric, and somatic diseases) to estimate the average power of primary studies in each domain, finding that median power was generally quite low, in the range of 10–30%. When combined with publication bias, underpowered research tends to produce inflated false-positive rates and biased effects estimates (sometimes called the “winner’s curse”; Fraley and Vazire Citation2014; Romero Citation2016; van Zwet and Cator Citation2020). Another example is the p-curve, a graphical method developed to detect p-hacking by examining a distribution of p-values (Simonsohn, Nelson, and Simmons Citation2014). P-hacking – the practice of trying variant analyses until one passes the conventional threshold for “statistical significance” – is a common explanation for mass irreplicability in behavioral science (Yong Citation2015; Munafò et al. Citation2017). The relevance of p-curve analysis to observational studies is controversial: Bruns and Ioannidis (Citation2016) use a simulation study to show that the combination of p-hacking and omitted variable bias can produce a right-skewed p-curve (which would be interpreted as evidence that there was no p-hacking); Simonsohn, Nelson, and Simmons (Citation2019) reply that in these cases the relationship of interest is certainly confounded (it would be incorrect to draw a causal conclusion) but also entirely replicable (we would expect similar associations to show up in similar studies with the same omitted variable bias). The upshot of this debate seems to be that, even if the p-curve cannot reliably detect p-hacking in observational research, it may still be able to detect problems of irreplicability.
There are also established techniques in meta-analysis – funnel plots, Egger’s test, trim-and-fill, and the p-uniform technique – for detecting and adjusting for publication bias (Egger et al. Citation1997; Peters et al. Citation2007; van Aert, Wicherts, and van Assen Citation2019). While publication bias is frequently identified as a major cause of the replication crisis, it does not necessarily lead to substantially biased estimates. For example, Anderson et al. (Citation2005) use Egger’s test and trim-and-fill to examine the impacts of publication bias across 9 combinations of ambient particulate air pollution measures, short-term adverse health effects, and single-city vs. multicity designs. Egger’s test was statistically significant in 4/9 combinations (without accounting for multiple comparisons), but in 3/4 combinations the trim-and-fill adjustment did not substantially change the estimate; for example, one estimate went from a relative risk of 1.006 (95% CI 1.005–1.007) to 1.005 (1.003–1.006). Out of nine combinations, the only substantial change after using trim-and-fill was from 1.025 (1.011–1.039) to 1.015 (1.001–1.029). More broadly, in a large-scale analysis of meta-analyses in psychology and medicine, van Aert, Wicherts, and van Assen (Citation2019) conclude that “Overestimation was minimal but statistically significant” (van Aert, Wicherts, and van Assen Citation2019, 22); while Mathur and Van der Weele (Citation2020) find that publication bias can be mitigated in a meta-analysis so long as “a large number of studies (at least 40)” are included.
These meta-analysis-based methods have some notable limitations. Lau et al. (Citation2006) argue that study heterogeneity can lead to asymmetric funnel plots, for example, if smaller studies focus on high-risk patients or different studies work with different populations. Simonsohn (Citation2017) argues that funnel plot analysis depends on the assumption that there is no correlation between effect size and sample size, which is likely to be false if researchers tend to use larger samples with smaller, more difficult to detect effects. Using simulation methods, van Aert, Wicherts, and van Assen (Citation2019) find that publication bias tests can be severely underpowered with respect to moderate publication bias. And perhaps most importantly for claims of mass irreplicability, typically a given meta-analysis paper examines at most a small number of associations. This might give us (indirect or direct) empirical evidence for the replicability of these associations; but not whether there is a problem of mass irreplicability across an entire field. A systematic review of meta-analyses – sometimes called a “meta-meta-analysis” – might provide such evidence (Dumas-Mallet et al. Citation2017; Mathur and Van der Weele Citation2020). A PubMed search for the query meta-meta-analysis (https://pubmed.ncbi.nlm.nih.gov/?term=meta-meta-analysis) returned 29 results, only one of which appeared to be specific to environmental public health (Spitzer Citation1991 is a commentary on a critique of a meta-analysis of environmental tobacco smoke and lung cancer); though this lack of results might just be due to lack of adoption of the “meta-meta-analysis” term. Still, broader searches of PubMed and Google Scholar did not find any such systematic reviews or aggregates of meta-analyses that were specific to environmental public health.
The third kind of evidence for mass irreplicability that I will consider here is direct empirical evidence, produced by replication attempts. In social psychology, considerable effort has been put into several large-scale multi-lab replication projects (Open Science Collaboration Citation2015; Klein et al. Citation2014, Citation2018). These projects are generally regarded as having provided strong direct evidence of mass irreplicability in social psychology. A similar project is currently underway with preclinical cancer biology (Errington et al. Citation2014), though as of 15 April 2021, results are available for only 17 out of 50 studies on the project’s website (https://elifesciences.org/collections/9b1e83d1/reproducibility-project-cancer-biology). I was unable to find any similar project for fields of environmental public health.
A meta-analysis can also provide this kind of direct empirical evidence, insofar as the primary studies it aggregates can be understood as replications of each other. For example, Boffetta et al. (Citation2008) give two examples of findings that, upon replication and meta-analytic synthesis, appeared to fail to replicate. (Presumably these two examples were chosen to illustrate this possibility, and so don’t necessarily generalize.) However, as with the provision of indirect evidence, meta-analyses are limited by their focus on a small number of associations. Again, “meta-meta-analysis” would be necessary to support claims about whole fields of research, and I was unable to find any such high-level studies specific to environmental public health.
All together, the available evidence for mass irreplicability in environmental public health appears to be extremely limited. While familiar methods, such as meta-analysis, could be used to aggregate and develop relevant evidence, it appears that this has not yet been done. Unless and until evidence has been collected more systematically – and this evidence indicates that the replication crisis extends to environmental public health – there does not seem to be strong grounds for that thinking that the replication crisis extends to environmental public health policymaking.
This argument might seem to have committed the fallacy of appealing to ignorance – I was unable to find evidence of mass irreplicability in environmental public health, and so there is no problem of mass irreplicability. That is, an absence of evidence isn’t the same thing as evidence of absence. As this paper has traversed the peer review process, several readers have raised this point, including reviewers at a major environmental public health journal who insisted that mass irreplicability was obviously a problem in the field. When I requested examples, reviewers either did not respond or provided citations to papers that were incorporated into the discussion above (including Sterne and Smith Citation2001; Lash Citation2017; Bartell Citation2019). Every credible citation that I have found or been given as evidence of a replication crisis in environmental public health is discussed in the current version of this paper. (Hicks Citationn.d.b. discusses some indirect empirical evidence that, I show, is not credible.) An appeal to ignorance is not a fallacy if there has been significant effort to search for the absent evidence.
In Bayesian terms, I began this project with a somewhat skeptical prior about claims of mass irreplicability in environmental public health. The evidence that I have found or been given is weak in the sense that, after Bayesian updating, my posterior is basically unchanged from my prior. However, some readers may have a more credulous prior, and have come into this section with a strong subjective probability that the replication crisis extends to environmental public health. The weak evidence that I have reviewed in this section probably did not do much to change their priors either. All of this is as it should be: weak evidence should not change anyone’s mind. But I do think it puts the burden of proof on those who believe that there is a problem of mass irreplicability for environmental public health: until they can provide some stronger empirical evidence, there is no reason for someone with my prior to think that the replication crisis does extend to these fields.
Proponents of Strengthening Transparency might respond that, as long as a problem of mass irreplicability is a live possibility, open science requirements can provide us with a kind of epistemic insurance: if there is a replication problem in environmental public health, then open science will help mitigate it. This leads us to the second key claim of their argument.
Definitions: Reproducibility is not the same as replicability
I turn now to the second key claim of the argument of Strengthening Transparency, namely, that an open data requirement will help solve the replication crisis. In the next section, I will argue that open science requirements cannot address such concerns. To make that argument, in this section first we must distinguish three frequently confused ideas in the discourse surrounding the replication crisis: replicability, reproducibility, and robustness.
The National Academies consensus report Reproducibility and Replicability in Science (RRS; National Academies of Sciences, Engineering, and Medicine Citation2019) defines reproducibility as the capacity to “obtain[] consistent results using the same input data, computational steps, methods, and code, and conditions of analysis” (National Academies of Sciences, Engineering, and Medicine Citation2019, 4, my emphasis). RRS notes that reproducibility “is synonymous with ‘computational reproducibility.’” By contrast, RSS defines replicability as the capacity to “obtain[] consistent results across studies aimed at answering the same scientific question, each of which has obtained its own data” (National Academies of Sciences, Engineering, and Medicine Citation2019, 4, my emphasis throughout). The primary differences between reproducibility and replicability are (1) whether the data used are the same or different, and (2) how the outcomes are compared.Footnote4
According to these definitions, a study is reproducible if, and only if, the outputs of the original analysis and the reproducibility check are quantitatively identical. Note that reproducibility means that both the data and the entire analysis pipeline – including data cleaning, handling missing or extreme values, and model specification – are held constant between the original study and reproducibility checks. While conducting a reproducibility check can be quite difficult – analysis outputs may depend on specific software versions or even specific hardware – in principle the conclusions of the check are not controversial: either the outputs are quantitatively identical or they are not.
By contrast, a study is replicable if, and only if, the design and findings of the original study and replication attempts are qualitatively similar. Because this qualitative similarity depends on substantive assumptions about which similarities and differences are important or unimportant; these assumptions apply to every element of the study, including the design, instruments, sampling frame, analytical plan, and so on; and these assumptions are often implicit and difficult to articulate explicitly, replicability is almost always ambiguous and can be highly controversial (Feest Citation2016).
Reproducibility and replicability are logically independent of each other. A study would be reproducible but not replicable if its original data and analysis code produce quantitatively identical results on different computers but new data lead to qualitatively different conclusions. And a study would be replicable but not reproducible if the original statistical analysis was not adequately documented (and so conducting exactly the same calculations in exactly the same order is impossible) but replication attempts that generate and analyze new data support qualitatively similar conclusions. This logical independence means that measures that promote reproducibility do not necessarily promote replicability.
Despite this logical independence, as RSS notes, these terms are frequently used interchangeably, which leads to significant confusion in the discourse surrounding the replication crisis, open science, and Strengthening Transparency specifically. Consider Lutter and Zorn (Citation2018), which has been frequently cited as evidence that “Posting study data has proven to be effective at improving the reliability of research in economics” (18). This report treats “reliability,” reproducibility, and replicability as synonymous, grouping together studies of different phenomena. For example, the first paragraph cites concerns about mass irreplicability (including a citation to Open Science Collaboration Citation2015, a replication study); while the second paragraph cites Dewald, Thursby, and Anderson (Citation1988) and Moffitt and Glandon (Citation2011), both of which – in the RSS terminology – studied reproducibility and state explicitly that they did not attempt to replicate any of the papers examined. Later, Lutter and Zorn (Citation2018) argue that “Access to the data necessary to replicate scientific studies is essential because the results of so many peer-reviewed scientific publications have proven to be impossible to reproduce,” (Lutter and Zorn Citation2018, 15, my emphasis) supporting this with a mix of studies that examine either reproducibility or replicability but in no case both. Note that access to the original data is neither necessary nor obviously helpful when attempting to replicate a study, which by definition involves collecting new data.
The term “replication” is also sometimes used synonymously with “reanalysis,” which “allow[s] assessment of the robustness of the original analysis and conclusions by, for instance, showing the variability that can occur when a previously omitted variable is added to the statistical model, different functional form assumptions are made …, or different assumptions are made when estimating standard errors and drawing statistical inferences … ” (US EPA Citation2020, 15,400, my emphasis). These kinds of checks are also called sensitivity analyses.
Michaels (Citation2008) observes that reanalysis or robustness has repeatedly been used by industry to delay regulation (50–57, 69–78, 86–88). He describes a strategy that might be called reverse p-hacking. In p-hacking, researchers or analysts conduct variant analyses, attempting to find at least one that produces a statistically significant result. In reverse p-hacking, the aim is to find a variant analysis where the result is not statistically significant. Industry can then claim that the original analysis was flawed or not robust and so should be discounted or ignored for regulatory purposes. Michaels (Citation2020) argues that, in the context of environmental public health, there is a crucial asymmetry between p-hacking and reverse p-hacking (242–243). On the one hand, if there is no effect, then only a few variant analyses will yield statistical significance, and so p-hacking amounts to searching for a needle in a haystack. It is possible, but difficult, especially for academic researchers with limited resources. On the other hand, unless the effect is quite large relative to the sample size (that is, the study has extremely high power), for a real effect a fair number of variant analyses will not be statistically significant. This makes reverse p-hacking much easier, especially for analysts with substantial resources provided by industry. In addition, like other scientific fields environmental public health often (though not always; Hicks Citation2018) assumes that false positives (incorrectly accepting the hypothesis that there is an effect) are much worse than false negatives (incorrectly rejecting the hypothesis that there is an effect). For example, a statistical significance threshold of 5% and test power of 80% (when power is considered at all) implies that avoiding type I errors is four times more important than avoiding type II errors (Di Stefano Citation2001; Fernández Pinto and Hicks Citation2019, 2). From this perspective, any reason to question a study’s findings – such as a single non-significant variant analysis – can become a reason to completely dismiss the study as a false positive.
Robustness requires qualitatively similar findings using the same data but different analytical methods. This makes it distinct from both reproducibility (same data; same analytic methods) and replicability (different data; same or similar analytic methods). Specifically, robustness checks are not informative about replicability. Alternative analyses of the same data do not tell us whether and to what extent similar conclusions would be supported if different data were gathered from a (more or less) different sample under (more or less) different conditions. In other words, replicability is a matter of generalizability and extrapolation, from the original study’s sample to another sample. Merely applying different statistical techniques to the original study’s sample will not tell us whether and to what extent we can generalize its findings. This point is related to the distinction between internal and external validity. While robustness checks may give us some indications of the internal validity of a study, they tell us nothing about how broadly its conclusions can be generalized (Cartwright Citation2007).
It is important to recognize that the replication crisis is, using the RSS terminology, a replication crisis. The most high-profile empirical study in the replication crisis discourse, Open Science Collaboration (Citation2015), reported the results of a collection of replication attempts, not reproducibility or robustness checks. Failures of reproducibility are worrying – if the numbers reported in a study don’t match the output of the analysis script, it seems that something has gone significantly wrong – but are likely less important than whether a study’s results can be generalized – that is, replicated. Similarly, failures of robustness raise concerns about p-hacking and internal validity, but again these are likely less important than replicability.
Open science and replicability
Observers of the replication crisis have proposed numerous possible causes. Some have argued that psychology draws on “theory” in a nebulous and imprecise way (Klein Citation2014; Muthukrishna and Henrich Citation2019; McPhetres et al. Citation2021) or tends to work with underspecified conceptualizations of the variables of interest (Wolfgang Stroebe and Strack Citation2014; Redish et al. Citation2018; Feest Citation2019). Across fields, observers have raised concerns about statistical power (Fraley, Vazire, and Ouzounis Citation2014; Dumas-Mallet et al. Citation2017; Romero Citation2016; van Zwet and Cator Citation2020), the representatives of samples (Henrich, Heine, and Norenzaya Citation2010), noisy measurement (Loken and Gelman Citation2017), and data mismanagement (Viglione Citation2020). With respect to statistical analysis, there have been concerns about p-hacking (Simmons, Nelson, and Simonsohn Citation2011; John, Loewenstein, and Prelec Citation2012), the overinterpretation of p-values (Amrhein, Trafimow, and Greenland Citation2018), coding errors and other software bugs (Herndon, Ash, and Pollin Citation2014), and reporting errors (Nuijten et al. Citation2016), along with longstanding debates over frequentist vs. Bayesian statistics (Lash Citation2017; Weinberg Citation2017). Beyond the scope of an individual study, some observers have pointed to publication bias (Gerber and Malhotra Citation2008; Scheel, Schijen, and Lakens Citation2020), publish-or-perish incentive structures (Smaldino and McElreath Citation2016), and fraud and other forms of scientific misconduct (Stroebe, Postmes, and Spears Citation2012). It is highly plausible that many of these factors contribute to the problem of mass irreplicability, where it exists; interact with each other, either synergistically or antagonistically; and vary across scientific disciplines. Given this complexity, it is difficult to make claims about the relative importance of different factors or the relative effectiveness of different interventions.
Instead, my focus is on just one class of interventions, namely, open science requirements.Footnote5 As a preliminary point, publishing data and code is likely to be irrelevant to many of the proposed causes listed above. Open science is simply the wrong kind of intervention to increase sample sizes, improve the interpretation of p-values, or mitigate pernicious incentive structures; these factors require other kinds of interventions, such as using consortia to achieve large sample sizes (Kaufman et al. Citation2012), improving statistical standards in peer review (Smaldino, Turner, and Contreras Kallens Citation2019), and reforming the criteria used for academic hiring, tenure, and promotion. Still, open science might be thought to improve replicability in at least two ways: by enabling peer reviewers (and others) to identify coding errors and by promoting robustness.
Coding errors
In some cases, coding errors in analysis scripts can lead to spurious conclusions that are qualitatively different from the results of otherwise similar studies. That is, coding errors might lead to replicability problems. One high-profile example was a macroeconomic study that, due to coding errors, inappropriately discarded some data and incorrectly concluded that high public debt stifles economic growth. This error was discovered when another team of researchers examined the working spreadsheet (including both data and code) used by the original researchers (Herndon, Ash, and Pollin Citation2014).
While open science is useful for catching these kinds of coding errors, this would make it useful for addressing concerns about mass irreplicability only insofar as these kinds of coding errors are a widespread problem. However, two recent reproducibility studies – one in psychology and the other in cognitive science – suggest that, while coding issues leading to reproducibility failures can be common, these issues do not frequently produce replicability failures.
First, Hardwicke et al. (Citation2018) examined the effect of an open data requirement at the journal Cognition using a pre-post design. In the pre-intervention sample, only 23/417 (6%) of papers had data that was judged to be “accessible, complete, and understandable”; this increased dramatically, to 85/174 (49%), in the post-intervention sample (Hardwicke et al. Citation2018, 6). The authors then attempted a reproducibility check on 1324 values from a sample of 35 papers with adequate data. They developed a coding scheme with four types of reproducibility problems: “insufficient information error” when the study and supplemental materials did not include information necessary to conduct the reproducibility attempt; minor and major numerical errors, when the absolute difference between reported and reproduced values were less than or greater than, respectively, 10% of the reported value; and “decision errors” when the reproduced p-value “fell on the opposite side of the 0.05 boundary” to the reported p-value (Hardwicke et al. Citation2018, 9). The authors found two insufficient information errors, 146 minor numerical errors, 64 major numerical errors, and no decision errors. Furthermore, “There were major errors related to effect sizes in only five cases, and for four of these the magnitude of the error was low” (Hardwicke et al. Citation2018, 11). The authors also incorporated a qualitative assessment of “whether any non-reproducibility of target outcomes affected the corresponding substantive conclusions presented in the original articles” (Hardwicke et al. Citation2018, 10), which can be understood as an indicator for potential replicability problems. They concluded that “in almost all cases … it appeared unlikely that the reproducibility issues we encountered have substantial implications for the corresponding conclusions outlined in the original articles” (Hardwicke et al. Citation2018, 11). That is, despite some irreproducible results, these authors did not find evidence that these results might lead to replication failures.
Next, Obels et al. (Citation2020) conducted reproducibility checks of preregistered studies in psychology. Out of their sample of 62 studies, data and code were obtained for 36 studies (58%) and results were reproducible for 21 studies (21/36 = 58%). They note that these rates are higher than previous reproducibility checks in psychology (Obels et al. Citation2020, 234), plausibly because of the association between preregistration and the open science movement. For the 15 studies for which data and code were available but the results could not be reproduced, Obels et al. (Citation2020) identified 3 main problems: “code to reproduce some values was missing,” “code gave errors (e.g., variables in the data set were missing, or functions did not run as expected),” and in one case the code ran but extremely slowly (Obels et al. Citation2020, 233). While the authors identify specific areas for improvement in making open data and code genuinely usable and reproducible, in no case did they find indications that coding errors might have rendered the studies irreplicable.
I was unable to find any similar large-scale reproducibility study in an environmental public health field. Page et al. (Citation2018) examined a sample of systematic reviews from across the biomedical literature, to determine whether primary study details (such as effect estimates and standard errors) were reported with enough detail that one could, in principle, reproduce meta-analytic effects estimates. Sixty-five percent of the systematic reviews in the sample reported all the necessary data, and 33% included data or code accessibility statements. However, Page et al. (Citation2018) did not attempt to actually reproduce any values.
Robustness
Open data certainly enables robustness checks, and thereby promotes robustness. But, as I noted above, robustness checks are not informative about replicability. A study might be robust in one particular sample, but fail to generalize to other samples, and thus fail to be replicable. And a study might replicate well but only so long as one particular analysis strategy (such as choice of covariates) is used. This latter kind of case might seem to be concerning. But, in particular instances, there may be good reasons – based on well-established theory or background assumptions – for using only that particular analysis strategy. For example, suppose a researcher has good reasons for assuming a certain set of causal relations among an exposure, outcome, and other variables, as expressed by a directed acyclic graph (DAG). Given this DAG, causal inference theory might entail that any other choice of covariates would produce biased estimates (Pearl, Glymour, and Jewell Citation2016; Hernán and Robins Citation2019). This kind of case is probably uncommon, but is also far from impossible, especially as more researchers in observational fields adopt the causal inference framework.
Robustness might be considered valuable on its own, independent of both reproducibility and replicability. I agree that it’s good to understand how statistical estimates might vary across the range of reasonable analytical approaches. However, open science practices are not the only way to ensure robustness, and strict open science requirements might not be preferable in a policy setting. For example, when evaluating study quality for inclusion in a systematic review, along with features such as the study design, US EPA might consider whether and to what extent robustness checks were conducted and reported. Robustness checks and sensitivity analyses are already standard practice in many fields, including environmental public health; researchers frequently conduct and report robustness checks in the primary text of their papers, and sometimes include extensive details in the supplemental materials. Recently, methodologists have developed computational methods such as “multiverse analysis” and specification curve analysis to make robustness checks more rigorous and systematic (Steegen et al. Citation2016; Simonsohn, Simmons, and Nelson Citation2020). Work in this area has already developed software packages that simplify conducting such analyses in popular statistical programming languages, such as R (Masur and Scharkow Citation2020) and Stata (Young and Holsteen Citation2021). So, insofar as robustness is valuable on its own, it seems that an agency, such as US EPA, could evaluate it without introducing a strong open data requirement. Insofar as robustness checks might be less burdensome than an open data requirement on researchers and agency staff, there may be a compelling cost-benefit argument against an open data requirement.
In short, while open data requirements, such as Strengthening Transparency, can enable robustness checks, these checks do not do much to address concerns about replicability, and might go beyond what would be required to encourage researchers to examine and report the robustness of their findings.
An easier critique of strengthening transparency?
It might be argued that the work that I have done in the last few sections is unnecessary.Footnote6 Instead, we might simply argue that the costs of a strong open science requirement like Strengthening Transparency – the delay in new science as researchers adopt open science methods; restricting policymakers from using certain kinds of observational studies; overturning established regulations because the studies used to support them have been disqualified – outweigh the benefits. However, if proponents of Strengthening Transparency are right, and there is a problem of mass irreplicability in environmental public health, then these costs are either negligible or impossible to estimate. If certain kinds of observational studies really are irreplicable “junk science,” then there is no cost to excluding them from consideration in developing regulations. Indeed, excluding bad, unreliable studies might lead to better regulation in terms of overall human welfare. Or, suppose we have no reliable estimates of, say, the health impacts of PM. In this case, it’s impossible for us to reliably estimate the costs and benefits of PM
regulation, and so impossible for us to reliably estimate the costs of overturning such regulation.
I agree with the argument that the costs of Strengthening Transparency would have far outweighed the benefits. But this is because I’m skeptical of the proponents’ claim that the replication crisis extends to environmental public health. So I think the “simple” cost-benefit argument assumes the kind of analysis that I am giving in this paper.
Conclusion: Moving forward with open science in environmental public health
In this paper, I have argued that the primary argument for Strengthening Transparency has two crucial weaknesses: there is no evidence of mass irreplicability in environmental public health; and even if there is a problem of mass irreplicability in these fields, an open data requirement would not address it. But there are a number of other arguments for open data, both generally and in the context of environmental public health specifically. In this final section, I first review these arguments, recall the discussion of the costs of open science from the introduction, and discuss how specific lines of funding might be used to promote the benefits of open science while reducing the costs to practicing researchers.
There are at least four general arguments for open science, and open data and code specifically. First, pedagogically, open science enables students to learn analysis techniques by examining and emulating how “real data” are analyzed in “real research,” well before they have the resources and skill to collect similar data on their own (Toelch and Ostwald Citation2018). Journal articles and statistical models necessarily simplify the details of working with data, especially activities such as data cleaning and exploratory data analysis that are not usually taught in traditional statistics courses (Peng and Dominici Citation2008; Leonelli and Tempini Citation2020). Similarly, many researchers might find it easier to learn a new analytical technique by reproducing the analysis of a paper that uses the technique, rather than trying to implement the technique from scratch on their own based only on an abstract methods paper.
Second, in some cases open data sets produced by two or more sources can be linked, supporting the development of new insights (Leonelli and Tempini Citation2018). For example, common approaches to studying the health impacts of air pollution combine air pollution monitoring data with medical records (either individual or aggregate) and perhaps census demographic data, linked using geographic identifiers (as in Tessum et al. Citation2021). Open science practices can promote these uses both by supporting the publication of data not available elsewhere or previously – for example, redacted medical records – and by making data more readily findable – for example, rather than navigating a confusing government website, readers can download the dataset using a link provided with the paper.
Third, open science can make scientific studies more accessible to community groups working outside of academia and government, such as environmental justice advocates. Consider an environmental justice coalition that does not have any members or partners who are trained epidemiologists; but does have one or two members who have undergraduate training in statistics using the open-source software R. Following the first point, these members might lack the background necessary to understand a scientific paper by reading the text alone; but might be able to understand it much better by reproducing its analysis. Then, following the second point, these environmental justice advocates might be able to conduct analyses relevant to their own concerns by linking published datasets from one or more studies, government data, and so on. In this way, open science might promote environmental justice, a key aim of environmental public health (Bezuidenhout et al. Citation2017; Elliott and Resnik Citation2019; Fernández Pinto and Hicks Citation2019).
Fourth, open data and code do promote reproducibility and robustness checks. These checks can be informative about aspects of the reliability and relevance of a study even if they are less important than replicability.
There is also an argument for open science in environmental public health specifically. Michaels (Citation2008) argues that regulated industries misuse protections for confidential business information (CBI) to protect data on the hazards of their products from public scrutiny (249–251) and that open data requirements should be applied equally to independent, government-sponsored, and industry-sponsored study data (253–255).Footnote7 Brock et al. (Citation2021) develop this argument, arguing that various open science practices (including open data and code, as well as preregistration and open access publishing) “might help alleviate public skepticism and increase public involvement” in environmental policymaking “by making all value judgments (e.g., the definition of specific protection goals), data, and evaluation tools used in decision-making accessible and transparent for the public” (2). (Though Elliott et al. Citation2017 give some evidence suggesting that values disclosures, specifically, might decrease perceived trustworthiness.)
For these reasons, environmental public health researchers and policymakers might find open science practices compelling even if they cannot address concerns about irreplicability. But open science practices are not without cost. As discussed in the introduction, doing open data well requires significant infrastructure, training, and maintenance; without support for these components, a strong open science requirement is likely to be highly burdensome for scientists, especially those who work with sensitive data, such as patient medical records. In this scenario, many researchers would be likely to do open science poorly or simply ignore open science requirements (the findings of Obels et al. (Citation2020) suggest this has happened in psychology). Then, insofar as many observers continue to confuse replicability and reproducibility, mass irreproducibility (due to code and data that are not available or properly prepared for reproducibility checks) might be taken incorrectly as evidence for mass irreplicability. In addition, as discussed above, there are epistemic concerns that “special interest groups [might] spread misleading reanalyses of [open] data” (Elliott and Resnik Citation2019, 075002–3).
Given the expense required to adopt open science practices – and do them well – I would recommend that Congress allocate substantial funds to US EPA, NIEHS, and other relevant research funding bodies to support infrastructure development and training for open science in environmental public health. Specifically, I recommend four major areas of research funding. First, insofar as some researchers and observers are concerned about the possibility of mass irreplicability in environmental public health, one line of funding might be used to support efforts to close the evidence gaps that I identified above. Meta-analysts might be encouraged to use funnel plots or other techniques to detect publication bias and to conduct meta-meta-analyses. This line of funding might also be used to support large-scale reproducibility and replication checks (with the caveat that replication checks might not be feasible for some kinds of study designs).
A second line of funding would be for designing, constructing, and especially maintaining secure data enclaves and other infrastructure, with which environmental public health researchers can make their data available to other researchers in ways that will protect the privacy of participants (Lash and Vandenbroucke Citation2012 propose “a publicly available registry that describes data already collected for observational studies of human subjects”). Third, for training researchers in open science methods and technologies. Perhaps the most obvious target for such training is graduate students, who will not have to struggle with unlearning old practices and can become the leaders of their fields in the future. But I would suggest also providing training for interested members of the general public, such as environmental justice advocates, through workshops, weekend courses, and community-based participatory research (CBPR) projects that incorporate a training component for community partners. Even if these kinds of efforts do not enable members of the general public to conduct their own analyses of open environmental public health data, it is likely to improve their ability to understand and constructively critique such analyses (Elliott and Resnik Citation2019; Garzón-Galvis, Richardson, and Solomon Citation2020).
While second and third lines of funding can do much to support the adoption of open science practices in environmental public health over time, they cannot do so overnight. So, fourth, I recommend funding long-term staff scientist positions, for data managers and other open science specialists who can provide support to established researchers who are not themselves familiar with open science practices. It is unreasonable to expect a researcher who is approaching retirement to learn radically different ways of conducting and disseminating their science. These established researchers should have ready access to open science specialists who can do this work for them. Because staff scientists in these positions will be responsible for maintaining the integrity of open data and code over significant periods of time, it is important that their contracts are for relatively long durations, such as 5–10 years.
Disclosure statement
No potential conflict of interest was reported by the author.
Supplemental data for this article can be accessed on the publisher’s website
Notes
1. The rule was finalized in the closing days of the Trump administration in January 2021, but vacated and remanded in February 2021 for procedural violations (King Citation2021). The Biden administration declined to pursue further work on the rule and formally abandoned it a few months later.
2. Hicks Citationn.d.a. examines the tension between the epistemic value of scrutinizing raw data and the pragmatic value of quickly enacting regulation to protect human health and the environment.
3. “Open science” is a broad label, covering numerous different practices (Munafò et al. Citation2017 Table 1). Strengthening Transparency made no reference to preregistration, the practice of publishing a time-stamped analysis plan before beginning data collection. Preregistration is frequently discussed in the open science/replication crisis discourse, where it is widely regarded as essentially for addressing concerns about p-hacking and publication bias (Wagenmakers et al. Citation2012). However, preregistration is likely to be less appropriate for some key study designs in environmental public health, including long-term cohort studies – where research questions might not be formulated until decades after data collection has begun – and the use of administrative data – where the exact details of the analysis plan might depend on which variables turn out to be available (Blair et al. Citation2009, 1810; Lash and Vandenbroucke Citation2012). Because it was not included in Strengthening Transparency and is often inappropriate in environmental public health, I do not consider preregistration in my analysis of open science here.
4. Other authors give similar definitions, including Bollen et al. (Citation2015), Patil, Peng, and Leek (Citation2019), and Schöch (Citation2021). Note that Schöch flips the definitions of replicability and reproducibility, relative to the RSS definitions. S. N. Goodman, Fanelli, and Ioannidis (Citation2016) initially follow the definitions from Bollen et al. (Citation2015), then complain that these “definitions do not provide clear operational criteria for what constitutes successful replication or reproduction”; they go on to distinguish three senses of replicability (in the RSS terminology), mostly using the term “reproducibility” but sometimes using “replicability” as a synonym. Penders, Holbrook, and de Rijcke (Citation2019) review several alternative definitions of these terms, designed to get at different distinctions. Patil, Peng, and Leek (Citation2019) offers perhaps the most general framework for precise definitions of reproducibility, replicability, and related terms. For my purposes here, it is not important exactly which term is associated with which definition. What is important for my purposes is the distinction between “repeating research” (to use Schöch’s general term) that uses old data vs. that which gathers new data. Open data is relevant to the former but not the latter. Thanks to an anonymous reviewer for suggesting some of these alternative definitions.
5. Again, by “open science” I mean publishing data and code. I explicitly set aside preregistration because it is less appropriate for key study designs in environmental public health and was not included in the Strengthening Transparency requirements.
6. Thanks to an anonymous reviewer for suggesting this objection.
7. Notably, Michaels seems to favor the Health Effects Institute model, rather than publicly accessible data, calling for “a well-funded office with the power to review all of the science used by the regulators, including privately funded science, and to advocate for standards that would truly protect the public” (Michaels Citation2008, 255).
References
- Amrhein, V., D. Trafimow, and S. Greenland. 2018. “Inferential Statistics as Descriptive Statistics: There Is No Replication Crisis if We Don’t Expect Replication.” The American Statistician 73 (sup1): 262–270. doi:10.1080/00031305.2018.1543137.
- Anderson, H. R., R. W. Atkinson, J. L. Peacock, M. J. Sweeting, and L. Marston. 2005. “Ambient Particulate Matter and Health Effects: Publication Bias in Studies of Short-term Associations.” Epidemiology 16 (2): 155–163. doi:10.1097/01.ede.0000152528.22746.0f.
- Bartell, S. M. 2019. “Understanding and Mitigating the Replication Crisis, for Environmental Epidemiologists.” Current Environmental Health Reports 6 (1): 8–15. doi:10.1007/s40572-019-0225-4.
- Berg, J., P. Campbell, V. Kiermer, N. Raikhel, and D. Sweet. 2018. “Joint Statement on EPA Proposed Rule and Public Availability of Data.” Science 360 (6388): eaau0116. doi:10.1126/science.aau0116.
- Bezuidenhout, L. M., S. Leonelli, A. H. Kelly, and B. Rappert. 2017. “Beyond the Digital Divide: Towards a Situated Approach to Open Data.” Science & Public Policy 44 (4): 464–475. doi:10.1093/scipol/scw036.
- Blair, A., R. Saracci, P. Vineis, P. Cocco, F. Forastiere, P. Grandjean, M. Kogevinas, D. Kriebel, A. McMichael, N. Pearce, et al. 2009. “Epidemiology, Public Health, and the Rhetoric of False Positives.” Environmental Health Perspectives 117 (12): 1809–1813. doi:10.1289/ehp.0901194.
- Boffetta, P., J. K. McLaughlin, C. La Vecchia, R. E. Tarone, L. Lipworth, and W. J. Blot. 2008. “False-Positive Results in Cancer Epidemiology: A Plea for Epistemological Modesty.” JNCI: Journal of the National Cancer Institute 100 (14): 988–995. doi:10.1093/jnci/djn191.
- Bollen, K., J. T. Cacioppo, R. M. Kaplan, J. A. Krosnick, and J. L. Olds. 2015. “Social, Behavioral, and Economic Sciences Perspectives on Robust and Reliable Science.” Subcommittee on Replicability in ScienceAdvisory Committee to the National Science Foundation Directorate for Social, Behavioral, and Economic Sciences. https://www.nsf.gov/sbe/AC_Materials/SBE_Robust_and_Reliable_Research_Report.pdf
- Boronow, K. E., L. J. Perovich, L. Sweeney, J. S. Yoo, R. A. Rudel, P. Brown, and J. G. Brody. 2020. “Privacy Risks of Sharing Data from Environmental Health Studies.” Environmental Health Perspectives 128 (1): 017008. doi:10.1289/EHP4817.
- Bright, L. K. 2017. “Decision Theoretic Model of the Productivity Gap.” Erkenntnis 82 (2): 421–442. doi:10.1007/s10670-016-9826-6.
- Brock, T. C. M., K. C. Elliott, A. Gladbach, C. Moermond, J. Romeis, T.-B. Seiler, K. Solomon, and G. P. Dohmen. 2021. “Open Science in Regulatory Environmental Risk Assessment.” Integrated Environmental Assessment and Management. doi:10.1002/ieam.4433.
- Bruns, S. B., and J. P. A. Ioannidis. 2016. “P-Curve and p-Hacking in Observational Research.” PLoS ONE 11 (2): e0149144. doi:10.1371/journal.pone.0149144.
- Cartwright, N. 2007. “Are RCTs the Gold Standard?” BioSocieties 2 (1): 11–20. doi:10.1017/S1745855207005029.
- Chay, K. Y., and M. Greenstone. 2003. “The Impact of Air Pollution on Infant Mortality: Evidence from Geographic Variation in Pollution Shocks Induced by a Recession*.” The Quarterly Journal of Economics 118 (3): 1121–1167. doi:10.1162/00335530360698513.
- Cornwall, W. 2018. “Critics See Hidden Goal in EPA Data Access Rule.” Science 360 (6388): 472–473. doi:10.1126/science.360.6388.472.
- Dewald, W. G., J. G. Thursby, and R. G. Anderson. 1988. “Replication in Empirical Economics: The Journal of Money, Credit and Banking Project.” American Economic Review 78 (5): 1162–1163. https://ideas.repec.org/a/aea/aecrev/v78y1988i5p1162-63.html
- Di Stefano, J. 2001. “Power Analysis and Sustainable Forest Management.” Forest Ecology and Management 154 (1–2): 141–153. doi:10.1016/S0378-1127(00)00627-7.
- Dockery, D. W., and C. A. Pope. 2020. “The Threat to Air Pollution Health Studies behind the Environmental Protection Agency’s Cloak of Science Transparency.” American Journal of Public Health 110 (3): 286–287. doi:10.2105/AJPH.2019.305531.
- Dockery, D. W., C. A. Pope, X. Xu, J. D. Spengler, J. H. Ware, M. E. Fay, B. G. Ferris, and F. E. Speizer. 1993. “An Association between Air Pollution and Mortality in Six U.S. Cities.” New England Journal of Medicine 329 (24): 1753–1759. doi:10.1056/NEJM199312093292401.
- Dumas-Mallet, E., K. S. Button, T. Boraud, F. Gonon, and M. R. Munafò. 2017. “Low Statistical Power in Biomedical Science: A Review of Three Human Research Domains.” Royal Society Open Science 4 (2): 160254. doi:10.1098/rsos.160254.
- Egger, M., G. D. Smith, M. Schneider, and C. Minder. 1997. “Bias in Meta-Analysis Detected by a Simple, Graphical Test.” BMJ 315 (7109): 629–634. doi:10.1136/bmj.315.7109.629.
- Elliott, K. C., A. M. McCright, S. Allen, and T. Dietz. 2017. “Values in Environmental Research: Citizens’ Views of Scientists Who Acknowledge Values.” PLOS ONE 12 (10): e0186049. doi:10.1371/journal.pone.0186049.
- Elliott, K. C., and D. B. Resnik. 2019. “Making Open Science Work for Science and Society.” Environmental Health Perspectives 127 (7): 075002. doi:10.1289/EHP4808.
- Errington, T. M., E. Iorns, W. Gunn, F. E. Tan, J. Lomax, and B. A. Nosek. 2014. “An Open Investigation of the Reproducibility of Cancer Biology Research.” eLife 3 (December): e04333. Edited by Peter Rodgers. doi10.7554/eLife.04333.
- Feest, U. 2016. “The Experimenters’ Regress Reconsidered: Replication, Tacit Knowledge, and the Dynamics of Knowledge Generation.” Studies in History and Philosophy of Science Part A 58 (August): 34–45. doi:10.1016/j.shpsa.2016.04.003.
- Feest, U. 2019. “Why Replication Is Overrated.” Philosophy of Science 86 (5): 895–905. doi:10.1086/705451.
- Fernández Pinto, M., and D. J. Hicks. 2019. “Legitimizing Values in Regulatory Science.” Environmental Health Perspectives 127 (3): 035001. doi:10.1289/EHP3317.
- Fraley, R. C. and S. Vazire. 2014. “The N-Pact Factor: Evaluating the Quality of Empirical Journals with respect to Sample Size and Statistical Power.” PLOS ONE 9 (10): e109019. doi:10.1371/journal.pone.0109019
- Garzón-Galvis, C., M. J. Richardson, and G. M. Solomon. 2020. “Tracking Environmental and Health Disparities to Strengthen Resilience before the Next Crisis.” Environmental Justice, September. doi:10.1089/env.2020.0025.
- Gerber, A., and N. Malhotra. 2008. “Do Statistical Reporting Standards Affect What Is Published? Publication Bias in Two Leading Political Science Journals.” Quarterly Journal of Political Science 3 (3): 313–326. doi:10.1561/100.00008024.
- Goodman, S., and S. Greenland. 2007. “Why Most Published Research Findings are False: Problems in the Analysis.” PLOS Medicine 4 (4): e168. doi:10.1371/journal.pmed.0040168.
- Goodman, S. N., D. Fanelli, and J. P. A. Ioannidis. 2016. “What Does Research Reproducibility Mean?” Science Translational Medicine 8 (341): 341ps12. doi:10.1126/scitranslmed.aaf5027.
- Hardwicke, T. E., M. B. Mathur, K. MacDonald, G. Nilsonne, G. C. Banks, M. C. Kidwell, A. H. Mohr, et al. 2018. “Data Availability, Reusability, and Analytic Reproducibility: Evaluating the Impact of a Mandatory Open Data Policy at the Journal Cognition.” Royal Society Open Science 5 (8): 180448. doi:10.1098/rsos.180448.
- Harris, R. 2017. Rigor Mortis: How Sloppy Science Creates Worthless Cures, Crushes Hope, and Wastes Billions. 1 ed. Basic Books.
- Henrich, J., S. J. Heine, and A. Norenzaya. 2010. “The Weirdest People in the World?” Behavioral and Brain Sciences 33 (2–3): 61–83. doi:10.1017/S0140525X0999152X.
- Hernán, M. A., and J. M. Robins. 2019. Causal Inference. Taylor & Francis.
- Herndon, T., M. Ash, and R. Pollin. 2014. “Does High Public Debt Consistently Stifle Economic Growth? A Critique of Reinhart and Rogoff.” Cambridge Journal of Economics 38 (2): 257–279. doi:10.1093/cje/bet075.
- Hicks, D. J. 2018. “Inductive Risk and Regulatory Toxicology: A Comment on De Melo-Martín and Intemann.” Philosophy of Science 85 (1): 164–174. doi:10.1086/694771.
- Hicks, D. J. n.d.a. “When Virtues are Vices: ‘Anti-science’ Epistemic Values in Environmental Politics.” Unpublished.
- Hicks, D. J. n.d.b. “Young’s p-Value Plot Does Not Provide Evidence against Air Pollution Hazards.” Unpublished.
- Holman, B., and J. Bruner. 2017. “Experimentation by Industrial Selection.” Philosophy of Science 84 (5): 1008–1019. doi:10.1086/694037.
- Ioannidis, J. P. A. 2005. “Why Most Published Research Findings Are False.” PLoS Medicine 2 (8): e124. doi:10.1371/journal.pmed.0020124.
- Ioannidis, J. P. A. 2018. “All Science Should Inform Policy and Regulation.” PLOS Medicine 15 (5): e1002576. doi:10.1371/journal.pmed.1002576.
- John, L. K., G. Loewenstein, and D. Prelec. 2012. “Measuring the Prevalence of Questionable Research Practices with Incentives for Truth Telling.” Psychological Science 23 (5): 524–532. doi:10.1177/0956797611430953.
- Jorgenson, A. K., T. D. Hill, B. Clark, R. P. Thombs, P. Ore, K. S. Balistreri, and J. E. Givens. 2020. “Power, Proximity, and Physiology: Does Income Inequality and Racial Composition Amplify the Impacts of Air Pollution on Life Expectancy in the United States?” Environmental Research Letters 15 (2): 024013. doi:10.1088/1748-9326/ab6789.
- Kaiser, J. 1997. “Showdown Over Clean Air Science.” Science 277 (5325): 466–469. doi:10.1126/science.277.5325.466.
- Kaufman, J. D., S. D. Adar, R. W. Allen, R. G. Barr, M. J. Budoff, G. L. Burke, A. M. Casillas, et al. 2012. “Prospective Study of Particulate Air Pollution Exposures, Subclinical Atherosclerosis, and Clinical Cardiovascular Disease: The Multi-Ethnic Study of Atherosclerosis and Air Pollution (MESA Air).” American Journal of Epidemiology 176 (9): 825–837. doi:10.1093/aje/kws169.
- King, P. 2021. “EPA: Judge Scraps Trump’s ‘Secret Science’ Rule.” Accessed 1 February 2021. https://www.eenews.net/stories/1063724017
- Klein, R. A., K. A. Ratliff, M. Vianello, R. B. Adams Jr., Š. Bahník, M. J. Bernstein, K. Bocian, et al. 2014. “Investigating Variation in Replicability: A ‘Many Labs’ Replication Project.” Social Psychology 45 (3): 142–152. doi:10.1027/1864-9335/a000178.
- Klein, R. A., M. Vianello, F. Hasselman, B. G. Adams, R. B. Adams, S. Alper, M. Aveyard, et al. 2018. “Many Labs 2: Investigating Variation in Replicability across Samples and Settings.” Advances in Methods and Practices in Psychological Science 1 (4): 443–490. doi:10.1177/2515245918810225.
- Klein, S. B. 2014. “What Can Recent Replication Failures Tell Us about the Theoretical Commitments of Psychology?” Theory & Psychology 24 (3): 326–338. doi:10.1177/0959354314529616.
- Krewski, D., R. T. Burnett, M. Goldberg, K. Hoover, J. Siemiatycki, M. Abrahamowicz, P. J. Villeneuve, and W. White. 2005a. “Reanalysis of the Harvard Six Cities Study, Part II: Sensitivity Analysis.” Inhalation Toxicology 17 (7–8): 343–353. doi:10.1080/08958370590929439.
- Krewski, D., R. T. Burnett, M. Goldberg, K. Hoover, J. Siemiatycki, M. Abrahamowicz, and W. White. 2005b. “Reanalysis of the Harvard Six Cities Study, Part I: Validation and Replication.” Inhalation Toxicology 17 (7–8): 335–342. doi:10.1080/08958370590929402.
- Lash, T. L. 2015. “Declining the Transparency and Openness Promotion Guidelines.” Epidemiology 26 (6): 779–780. doi:10.1097/EDE.0000000000000382.
- Lash, T. L. 2017. “The Harm Done to Reproducibility by the Culture of Null Hypothesis Significance Testing.” American Journal of Epidemiology 186 (6): 627–635. doi:10.1093/aje/kwx261.
- Lash, T. L., and J. P. Vandenbroucke. 2012. “Commentary: Should Preregistration of Epidemiologic Study Protocols Become Compulsory?: Reflections and a Counterproposal.” Epidemiology 23 (2): 184–188. doi:10.1097/EDE.0b013e318245c05b.
- Lash, T. L., L. J. Collin, and M. E. Van Dyke. 2018. “The Replication Crisis in Epidemiology: Snowball, Snow Job, or Winter Solstice?” Current Epidemiology Reports 5 (2): 175–183. doi:10.1007/s40471-018-0148-x.
- Lau, J., J. P. A. Ioannidis, N. Terrin, C. H. Schmid, and I. Olkin. 2006. “The Case of the Misleading Funnel Plot.” BMJ 333 (7568): 597–600. doi:10.1136/bmj.333.7568.597.
- Leonelli, S. 2016. “Open Data: Curation Is Under-Resourced.” Nature 538 (7623): 41. doi:10.1038/538041d.
- Leonelli, S. 2018. “Rethinking Reproducibility as a Criterion for Research Quality.” In Research in the History of Economic Thought and Methodology, edited by L. Fiorito, S. Scheall, and C. E. Suprinyak, Vol. 36, 129–146. Emerald Publishing Limited. doi:10.1108/S0743-41542018000036B009.
- Leonelli, S., and N. Tempini. 2018. “Where Health and Environment Meet: The Use of Invariant Parameters in Big Data Analysis.” Synthese, June 1–20. doi:10.1007/s11229-018-1844-2.
- Leonelli, S., and N. Tempini, eds. 2020. Data Journeys in the Sciences. Springer Nature.
- Lerner, S. 2017. “Republicans are Using Big Tobacco’s Secret Science Playbook to Gut Health Rules.” The Intercept. Accessed 5 February 2017. https://theintercept.com/2017/02/05/republicans-want-to-make-the-epa-great-again-by-gutting-health-regulations/
- Levin, N., S. Leonelli, D. Weckowska, D. Castle, and J. Dupré. 2016. “How Do Scientists Define Openness? Exploring the Relationship between Open Science Policies and Research Practice.” Bulletin of Science, Technology & Society 36 (2): 128–141. September. doi:10.1177/0270467616668760.
- Lewis, M., Jr. 2020. “Comments Submitted by Marlo Lewis, Jr., Competitive Enterprise Institute.” https://www.regulations.gov/document?D=EPA-HQ-OA-2018-0259-12692
- Loken, E., and A. Gelman. 2017. “Measurement Error and the Replication Crisis.” Science 355 (6325): 584–585. doi:10.1126/science.aal3618.
- Lutter, R., and D. Zorn. 2018. “On the Benefits and Costs of Public Access to Data Used to Support Federal Policy Making.” SSRN Electronic Journal. doi:10.2139/ssrn.3191414.
- Martini, C., and M.Fernández Pinto. 2016. “Modeling the Social Organization of Science.” European Journal for Philosophy of Science, August. 1–18. doi:10.1007/s13194-016-0153-1.
- Masur, P. K., and M. Scharkow. 2020. Specr: Conducting and Visualizing Specification Curve Analyses (version 0.2.1). https://CRAN.R-project.org/package=specr
- Mathur, M. B., and T. J. Van der Weele. 2020.“Estimating Publication Bias in Meta-analyses of Peer-reviewed Studies: A Meta-meta-analysis across Disciplines and Journal Tiers.” Research Synthesis Methods, October. doi:10.1002/jrsm.1464.
- McNutt, M. 2014. “Reproducibility.” Science 343 (6168): 229. doi:10.1126/science.1250475.
- McPhetres, J., N. Albayrak-Aydemir, A. B. Mendes, E. C. Chow, P. Gonzalez-Marquez, E. Loukras, A. Maus, et al. 2021. “A Decade of Theory as Reflected in Psychological Science (2009–2019).” PLOS ONE 16 (3): e0247986. doi:10.1371/journal.pone.0247986.
- Michaels, D. 2008. Doubt Is Their Product How Industry’s Assault on Science Threatens Your Health. Oxford: Oxford Univ. Press.
- Michaels, D. 2020. The Triumph of Doubt: Dark Money and the Science of Deception. Oxford University Press.
- Milloy, S. 2019. “Milloy Presentation to EPA CASAC Re Claim that PM Kills.” JunkScience.com (blog). Accessed 22 October 2019. https://web.archive.org/web/20200105130936/https://junkscience.com/2019/10/milloy-presentation-to-epa-casac-re-claim-that-pm-kills/
- Moffitt, R. A., and P. J. Glandon. 2011. “Report of the Editor: American Economic Review.” American Economic Review 101 (3): 684–699. doi:10.1257/aer.101.3.684.
- Mohai, P., D. Pellow, and J. T. Roberts. 2009. “Environmental Justice.” Annual Review of Environment and Resources 34 (1): 405–430. doi:10.1146/annurev-environ-082508-094348.
- Mullard, A. 2017. “2016 FDA Drug Approvals.” Nature Reviews Drug Discovery 16 (2): 73–76. doi:10.1038/nrd.2017.14.
- Munafò, M. R., B. A. Nosek, D. V. M. Bishop, K. S. Button, C. D. Chambers, N. P. du Sert, U. Simonsohn, E.-J. Wagenmakers, J. J. Ware, and J. P. A. Ioannidis. 2017. “A Manifesto for Reproducible Science.” Nature Human Behaviour 1 (1): 1–9. doi:10.1038/s41562-016-0021.
- Muthukrishna, M., and J. Henrich. 2019. “A Problem in Theory.” Nature Human Behaviour 3 (3): 221–229. doi:10.1038/s41562-018-0522-1. February 1.
- National Academies of Sciences, Engineering, and Medicine. 2019. Reproducibility and Replicability in Science. Washington, DC: National Academies Press. doi:10.17226/25303.
- Naumova, E. N. 2017. “Beyond RCTs in Public Health Policy Research: ‘Who’s the Fairest of Them All?’.” Journal of Public Health Policy 38 (2): 216–220. doi:10.1057/s41271-016-0062-8.
- Nosek, B. 2019. “Testimony of Brian A. Nosek, Ph.D.” Committee on Science, Space, and Technology, US House of Representatives. https://web.archive.org/web/20200601150315/https://science.house.gov/hearings/strengthening-transparency-or-silencing-science-the-future-of-science-in-epa-rulemaking
- Nosek, B. A., G. Alter, G. C. Banks, D. Borsboom, S. D. Bowman, S. J. Breckler, S. Buck, C. D. Chambers, G. Chin, G. Christensen, et al. 2015. “Promoting an Open Research Culture.” Science 348 (6242): 1422–1425. doi:10.1126/science.aab2374.
- Nuijten, M. B., C. H. J. Hartgerink, M. A. L. M. van Assen, S. Epskamp, and J. M. Wicherts. 2016. “The Prevalence of Statistical Reporting Errors in Psychology (1985–2013).” Behavior Research Methods 48 (4): 1205–1226. doi:10.3758/s13428-015-0664-2.
- Obels, P., D. Lakens, N. A. Coles, J. Gottfried, and S. A. Green. 2020. “Analysis of Open Data and Computational Reproducibility in Registered Reports in Psychology.” Advances in Methods and Practices in Psychological Science 3 (2): 229–237. doi:10.1177/2515245920918872.
- Open Science Collaboration. 2015. “Estimating the Reproducibility of Psychological Science.” Science 349 (6251): aac4716. doi:10.1126/science.aac4716.
- Page, M. J., D. G. Altman, L. Shamseer, J. E. McKenzie, N. Ahmadzai, D. Wolfe, F. Yazdi, F. Catalá-López, A. C. Tricco, and D. Moher. 2018. “Reproducible Research Practices are Underused in Systematic Reviews of Biomedical Interventions.” Journal of Clinical Epidemiology 94 (February): 8–18. doi:10.1016/j.jclinepi.2017.10.017.
- Parker, W. 2020. “Model Evaluation: An Adequacy-for-Purpose View.” Philosophy of Science 87 (3): 457–477. doi:10.1086/708691.
- Patil, P., R. D. Peng, and J. T. Leek. 2019. “A Visual Tool for Defining Reproducibility and Replicability.” Nature Human Behaviour 3 (7): 650. doi:10.1038/s41562-019-0629-z.
- Pearl, J., M. Glymour, and N. P. Jewell. 2016. Causal Inference in Statistics: A Primer. John Wiley & Sons.
- Penders, B., J. B. Holbrook, and S. de Rijcke. 2019. “Rinse and Repeat: Understanding the Value of Replication across Different Ways of Knowing.” Publications 7 (3): 52. doi:10.3390/publications7030052.
- Peng, R. D., and F. Dominici. 2008. Statistical Methods for Environmental Epidemiology with R: A Case Study in Air Pollution and Health. New York: Springer-Verlag. Use R! doi:10.1007/978-0-387-78167-9.
- Peters, J. L., A. J. Sutton, D. R. Jones, K. R. Abrams, and L. Rushton. 2007. “Performance of the Trim and Fill Method in the Presence of Publication Bias and Between-Study Heterogeneity.” Statistics in Medicine 26 (25): 4544–4562. doi:10.1002/sim.2889.
- Potochnik, A. 2017. Idealization and the Aims of Science. University of Chicago Press.
- Powell, K. 2021. “The Broken Promise That Undermines Human Genome Research.” Nature 590 (7845): 198–201. doi:10.1038/d41586-021-00331-5.
- Redish, A. D., E. Kummerfeld, R. L. Morris, and A. C. Love. 2018. “Opinion: Reproducibility Failures are Essential to Scientific Inquiry.” Proceedings of the National Academy of Sciences 115 (20): 5042–5046. doi:10.1073/pnas.1806370115.
- Richardson, V. 2021. “Greens Rip EPA’s New ‘Secret Science’ Rule as Last-Ditch Attempt to Hamstring Biden.” The Washington Times. Accessed 5 January 2021. https://www.washingtontimes.com/news/2021/jan/5/andrew-wheeler-epa-secret-science-rule-ripped-atte/
- Rider, C. V., C. M. McHale, T. F. Webster, L. Lowe, W. H. Goodson, M. A. La Merrill, G. Rice, L. Zeise, L. Zhang, and M. T. Smith. 2021. “Using the Key Characteristics of Carcinogens to Develop Research on Chemical Mixtures and Cancer.” Environmental Health Perspectives 129 (3): 035003. doi:10.1289/EHP8525.
- Romero, F. 2016. “Can the Behavioral Sciences Self-Correct? A Social Epistemic Study.” Studies in History and Philosophy of Science Part A 60 (December): 55–69. doi:10.1016/j.shpsa.2016.10.002.
- Scheel, A. M., M. Schijen, and D. Lakens. 2020. “An Excess of Positive Results: Comparing the Standard Psychology Literature with Registered Reports.” PsyArXiv. doi:10.31234/osf.io/p6e9c.
- Schöch, C., dir. 2021. Seminar 2: Christof Schöch - UBL & Elsevier Seminars on Reproducible Research. https://www.youtube.com/watch?v=ugzgvWl7nAo&list=PL-vTxWFyVBpEllXo6thA8ZQ_OpYkThIfQ&index=8
- Simmons, J. P., L. D. Nelson, and U. Simonsohn. 2011. “False-Positive Psychology.” Psychological Science 22 (11): 1359–1366. doi:10.1177/0956797611417632.
- Simonsohn, U. 2017. “[58] the Funnel Plot Is Invalid because of This Crazy Assumption: R(n,d)=0.” Data Colada (blog). Accessed 21 March 2017. http://datacolada.org/58
- Simonsohn, U., J. P. Simmons, and L. D. Nelson. 2020. “Specification Curve Analysis.” Nature Human Behaviour, July. 1–7. doi:10.1038/s41562-020-0912-z.
- Simonsohn, U., L. D. Nelson, and J. P. Simmons. 2014. “P-Curve: A Key to the File-Drawer.” Journal of Experimental Psychology. General 143 (2): 534–547. doi:10.1037/a0033242.
- Simonsohn, U., L. D. Nelson, and J. P. Simmons. 2019. “P-curve Won’t Do Your Laundry, but It Will Distinguish Replicable from Non-replicable Findings in Observational Research: Comment on Bruns & Ioannidis (2016).” PloS One 14 (3): e0213454. doi:10.1371/journal.pone.0213454.
- Smaldino, P. 2019. “Five Models of Science, Illustrating How Selection Shapes Methods.” Preprint. SocArXiv. doi:10.31235/osf.io/ghb4p.
- Smaldino, P., M. A. Turner, and P. A. Contreras Kallens. 2019. “Open Science and Modified Funding Lotteries Can Impede the Natural Selection of Bad Science.” Royal Society Open Science 6 (7): 190194. doi:10.1098/rsos.190194.
- Smaldino, P. E., and R. McElreath. 2016. “The Natural Selection of Bad Science.” Royal Society Open Science 3 (9): 160384. doi:10.1098/rsos.160384.
- Spellman, B. A. 2015. “A Short (Personal) Future History of Revolution 2.0.” Perspectives on Psychological Science 10 (6): 886–899. doi:10.1177/1745691615609918.
- Spitzer, W. O. 1991. “Meta-meta-analysis: Unanswered Questions about Aggregating Data.” Journal of Clinical Epidemiology 44 (2): 103–107. doi:10.1016/0895-4356(91)90258-b.
- Steegen, S., F. Tuerlinckx, A. Gelman, and W. Vanpaemel. 2016. “Increasing Transparency through a Multiverse Analysis.” Perspectives on Psychological Science 11 (5): 702–712. doi:10.1177/1745691616658637.
- Sterne, J. A. C., and G. D. Smith. 2001. “Sifting the Evidence—What’s Wrong with Significance Tests?” Physical Therapy 81 (8): 1464–1469. doi:10.1093/ptj/81.8.1464.
- Stroebe, W., and F. Strack. 2014. “The Alleged Crisis and the Illusion of Exact Replication.” Perspectives on Psychological Science 9 (1): 59–71. doi:10.1177/1745691613514450.
- Stroebe, W., T. Postmes, and R. Spears. 2012. “Scientific Misconduct and the Myth of Self-Correction in Science.” Perspectives on Psychological Science 7 (6): 670–688. doi:10.1177/1745691612460687.
- Tessum, C. W., D. A. Paolella, S. E. Chambliss, J. S. Apte, J. D. Hill, and J. D. Marshall. 2021. “Pm2.5 Polluters Disproportionately and Systemically Affect People of Color in the United States.” Science Advances 7 (18): eabf4491. doi:10.1126/sciadv.abf4491.
- Toelch, U., and D. Ostwald. 2018. “Digital Open Science—Teaching Digital Tools for Reproducible and Transparent Research.” PLOS Biology 16 (7): e2006022. doi:10.1371/journal.pbio.2006022.
- US EPA. 2018a. “Chlorpyrifos: EPA’s Seven Year Quest for Columbia’s Raw Data.” US EPA. Accessed 26 January 2018. https://web.archive.org/web/20180127080741/https://www.epa.gov/ingredients-used-pesticide-products/chlorpyrifos-epas-seven-year-quest-columbias-raw-data
- US EPA. 2018b. “Strengthening Transparency in Regulatory Science.” Federal Register. 83 (April): 18768–18774. https://www.federalregister.gov/documents/2018/04/30/2018-09078/strengthening-transparency-in-regulatory-science
- US EPA. 2020. “Strengthening Transparency in Regulatory Science.” Federal Register. 85 (March): 15396–15406. https://www.federalregister.gov/documents/2020/03/18/2020-05012/strengthening-transparency-in-regulatory-science
- van Aert, R. C. M., J. M. Wicherts, and M. A. L. M. van Assen. 2019. “Publication Bias Examined in Meta-analyses from Psychology and Medicine: A Meta-meta-analysis.” PloS One 14 (4): e0215052. doi:10.1371/journal.pone.0215052.
- van Zwet, E., and E. Cator. 2020. “The Significance Filter, the Winner’s Curse and the Need to Shrink.” arXiv:2009.09440 [Stat], September. http://arxiv.org/abs/2009.09440
- Viglione, G. 2020. “‘Avalanche’ of Spider-Paper Retractions Shakes Behavioural-Ecology Community.” Nature, February. doi:10.1038/d41586-020-00287-y.
- Wagenmakers, E.-J., R. Wetzels, D. Borsboom, H. L. J. van der Maas, and R. A. Kievit. 2012. “An Agenda for Purely Confirmatory Research.” Perspectives on Psychological Science 7 (6): 632–638. doi:10.1177/1745691612463078.
- Waldman, S., and N. H. Farah. 2018. “EPA: Smith Pitched Pruitt on ‘Secret Science.’ Now It’s Happening.” E&E News, Accessed 20 April 2018. https://www.eenews.net/stories/1060079655
- Weatherall, J. O., C. O’Connor, and J. P. Bruner. 2018.“How to Beat Science and Influence People: Policy-Makers and Propaganda in Epistemic Networks.” The British Journal for the Philosophy of Science, August. doi:10.1093/bjps/axy062.
- Weinberg, C. R. 2017. “Invited Commentary: Can Issues with Reproducibility in Science Be Blamed on Hypothesis Testing?” American Journal of Epidemiology 186 (6): 636–638. doi:10.1093/aje/kwx258.
- Wilkinson, M. D., M. Dumontier, I. J. Aalbersberg, G. Appleton, M. Axton, A. Baak, N. Blomberg, et al. 2016. “The FAIR Guiding Principles for Scientific Data Management and Stewardship.” Scientific Data 3 (1): 1–9. doi:10.1038/sdata.2016.18.
- Wilson, G., D. A. Aruliah, C. T. Brown, N. P. Chue Hong, M. Davis, R. T. Guy, S. H. D. Haddock, et al. 2014. “Best Practices for Scientific Computing.” PLoS Biology 12 (1): e1001745. doi:10.1371/journal.pbio.1001745.
- Wilson, G., J. Bryan, K. Cranston, J. Kitzes, L. Nederbragt, and T. K. Teal. 2017. “Good Enough Practices in Scientific Computing.” PLOS Computational Biology 13 (6): e1005510. doi:10.1371/journal.pcbi.1005510.
- Yong, T., Ed. 2015. “How Reliable Are Psychology Studies?” The Atlantic, Accessed 27 August 2015. http://www.theatlantic.com/health/archive/2015/08/psychology-studies-reliability-reproducability-nosek/402466/
- Young, C., and K. Holsteen. 2021. “MULTIVRS: Stata Module to Conduct Multiverse Analysis”. Boston College Department of Economics. https://ideas.repec.org/c/boc/bocode/s458927.html
- Zollman, K. 2007. “The Communication Structure of Epistemic Communities.” Philosophy of Science. http://www.journals.uchicago.edu/doi/abs/10.1086/525605