ABSTRACT
Working memory is frequently impaired in children with complex congenital heart disease (CHD), but little is known about the functional neuronal correlates. Sleep slow wave activity (SWA; 1–4.5 Hz EEG power) has previously been shown to reliably map neurofunctional networks of cognitive abilities in children with and without neurodevelopmental impairments. This study investigated whether functional networks of working memory abilities are altered in children with complex CHD using EEG recordings during sleep. Twenty-one children with complex CHD (aged 10.9 [SD: 0.3] years) and 17 typically-developing peers (10.5 [0.7] years) completed different working memory tasks and an overnight high-density sleep EEG recording (128 electrodes). The combined working memory score tended to be lower in children with complex CHD (CHD group: −0.44 [1.12], typically-developing group: 0.55 [1.24], d = 0.59, p = .06). The working memory score and sleep SWA of the first hour of deep sleep were correlated over similar brain regions in both groups: Strong positive associations were found over prefrontal and fronto-parietal brain regions – known to be part of the working memory network – and strong negative associations were found over central brain regions. Within these working memory networks, the associations between working memory abilities and sleep SWA (r between −.36 and .58, all p < .03) were not different between the two groups (no interactions, all p > .05). The current findings suggest that sleep SWA reliably maps working memory networks in children with complex CHD and that these functional networks are generally preserved in these patients.
Introduction
Children with complex congenital heart disease (CHD) are at risk for neurodevelopmental impairments, including a wide range of cognitive, motor, social, and behavioral domains (Abda et al., Citation2019; Feldmann et al., Citation2021; Latal, Citation2016). Structural and functional alterations of the brain, potentially resulting from delayed brain maturation starting in-utero, and perioperative brain injuries, have been suggested to contribute to these neurodevelopmental problems (Claessens et al., Citation2017; Mebius et al., Citation2017; Peyvandi et al., Citation2019).
Working memory is the ability to temporarily store and manipulate information necessary for various cognitive activities. The most widely accepted model describes working memory as a multicomponent system to temporarily store and update verbal and visuospatial information, alongside a central executive to control and regulate both of these components (Baddeley, Citation2012). The capacity of working memory is limited (Cowan, Citation2010) and individual differences in working memory capacity have been associated with differences in important everyday life functions, including academic outcomes (Friso-van den Bos et al., Citation2013; Hall et al., Citation2015; Pressler et al., Citation2013). In children with complex CHD, working memory is among the most affected functions. In fact, both verbal and visuospatial working memory deficits have been identified as early as preschool age and can persist throughout childhood, adolescence, and into adulthood (Asschenfeldt et al., Citation2020; Bellinger et al., Citation2015; Calderon et al., Citation2010; Cassidy et al., Citation2015; Gaudet et al., Citation2021; Sanz et al., Citation2017; Schaefer et al., Citation2013; Siciliano et al., Citation2021; Spillmann et al., Citation2021), likely impacting academic and everyday life functions of these patients. A number of studies have investigated potential structural neuronal correlates of working memory problems in patients with complex CHD: Reduced total (von Rhein et al., Citation2014) and regional brain volume, particularly of the hippocampus (Fontes et al., Citation2019; Latal et al., Citation2016) and the cerebellum (Verrall et al., Citation2021; von Rhein et al., Citation2014), and altered frontal sulcal patterns (Morton et al., Citation2020) have been found to be associated with poor working memory in patients with complex CHD but not in typically-developing peers. Further, the frontal white matter microstructure was found to be altered in adolescents with complex CHD compared to typically-developing adolescents, with a similar association between better white matter integrity and better working memory in both groups (Ehrler et al., Citation2020). To date, only two studies have investigated functional neuronal correlates of working memory deficits in children with complex CHD. Using functional MRI, they reported that children and adolescents with complex CHD activate similar fronto-parietal brain regions during a working memory task as do typically developing peers, but the level of task-induced activation and deactivation differs between the groups (King et al., Citation2017; Klaver et al., Citation2016). This suggests that alongside structural brain alterations associated with working memory deficits, the functional networks of working memory abilities may also be altered in individuals with complex CHD.
When investigating neurofunctional networks of cognitive abilities, factors related to wakefulness, including the level of attention, motivation, and differences in individual cognitive capacity, may affect data acquisition (Lustenberger & Huber, Citation2012). Assessing brain activity during sleep – when individuals are virtually disconnected from the environment (Tononi & Cirelli, Citation2006) – circumvents some of these challenges. Likely, in children at risk for neurodevelopmental impairments, this is particularly beneficial. In addition, while some children with complex CHD may not undergo MR imaging due to safety concerns associated with implants, sleep EEG can be recorded. Importantly, a high-density EEG provides the required spatial resolution to assess specific neurofunctional networks of cognitive abilities (Lustenberger & Huber, Citation2012; Mouthon & Huber, Citation2015).
Sleep slow wave activity (SWA) – the EEG spectral power between 1 and 4.5 Hz during non-rapid eye movement (NREM) sleep – reflects the synchronous neural activity and synaptic strength within cortical networks (Tononi & Cirelli, Citation2006, Citation2014; Vyazovskiy & Harris, Citation2013). Interestingly, sleep SWA has been shown to reliably map neurofunctional networks of motor and cognitive abilities in both typically-developing children and in children with neurodevelopmental deficits (Furrer et al., Citation2020; Kurth et al., Citation2012; Lustenberger et al., Citation2017; Pugin et al., Citation2015; Wehrle et al., Citation2017; Wilhelm et al., Citation2014). For example, in children born very preterm, more sleep SWA was associated with better executive function abilities over similar frontal brain regions as in typically-developing peers (Wehrle et al., Citation2017). In the case of working memory, a study in typically-developing children and adolescents showed an increase in sleep SWA over fronto-parietal brain regions known to be part of the working memory network after 3 weeks of intense working memory training. This SWA increase was positively associated with the increase in working memory performance (Pugin et al., Citation2015). Similarly, in healthy adults, more SWA over fronto-parietal brain regions was associated with better working memory performance (Ferrarelli et al., Citation2019). This suggests that sleep SWA could be a useful tool to investigate potential alterations of the functional networks of working memory abilities in children with complex CHD.
Thus, this study assesses sleep SWA in children with complex CHD and in typically-developing peers to investigate potential alterations in the neurofunctional network of working memory abilities in complex CHD. A positive association between sleep SWA and working memory abilities is expected over fronto-parietal brain regions, with the strength of the association potentially being different between children with complex CHD and typically-developing peers.
Material and methods
Participants
Patients with complex CHD were recruited from a prospective cohort study: In short, 300 children who had undergone cardiopulmonary bypass (CPB) surgery before 6 years of age at the University Children’s Hospital Zurich, Switzerland, between 2004 and 2009 were enrolled in the Research and Child Health Outcome (REACHOUT) study. Neurodevelopmental status was assessed prior to surgery and at 1, 4, and 6 years of age in these children. For the 10-year follow-up, children with a suspected or confirmed genetic syndrome or diagnosis (n = 75) were excluded and only children without (n = 135) were assessed (Spillmann et al., Citation2021). After completing the 10-year follow-up assessment (n = 135), families were informed about the current study, either by the examiner or through a letter, and were asked to participate. Twenty-six children with complex CHD agreed to participate in the current study.
Additionally, 17 typically-developing children were recruited from peers and siblings of the participating children with complex CHD and through flyers, and included into a typically-developing control group. Exclusion criteria were neurodevelopmental disorders (e.g., attention-deficit hyperactivity disorder, specific learning disorders or autism) and CHD.
Three children with complex CHD agreed to take part in the neuropsychological but not the sleep EEG assessment and two children with complex CHD refused to sleep with the EEG net on the day of testing. This led to a sample of 21 children with complex CHD and 17 typically-developing children with available sleep EEG, whose data were used for further analyses (see below for details on the EEG assessment).
Procedure
The study protocol included a neurodevelopmental assessment, an overnight sleep EEG recording, and a cerebral MR imaging. Assessments took place at the Child Development Center, the Sleep Laboratory, and the Center for MR Research, all at the University Children’s Hospital Zurich, Switzerland. Data were collected between July 2016 and July 2019 on weekdays, weekends and during holidays depending on the participants’ and families’ preferences.
The study was approved by the ethics committee of the Canton of Zurich, Switzerland. Written informed consent was obtained from a parent and/or legal guardian and participants provided oral consent. Children received a gift certificate for their participation and travel expenses were reimbursed.
Neurodevelopmental assessment
Neurodevelopmental outcome was assessed by a pediatric neuropsychologist or a developmental pediatrician. The assessment protocol included tests of executive functions, fine motor abilities, and general intellectual abilities. For further analyses, working memory scores assessed with two subtests of the Wechsler Intelligence Scale for Children – fourth edition (WISC-IV; Petermann & Petermann, Citation2008), the Corsi block-tapping test (Corsi, Citation1972) and a subtest of the Test Battery for Attentional Performance (TAP; Zimmermann & Fimm, Citation2012) were selected. In children with complex CHD, the WISC-IV had been assessed as part of the 10-year follow-up assessment of the REACHOUT study, either in the morning or in the afternoon. Typically-developing children completed various selected subtests of the WISC-IV as part of the current study protocol, either in the morning or in the afternoon. The Corsi block-tapping test and the TAP were completed in the afternoon, prior to the sleep EEG, in all children.
Verbal working memory was assessed with the working memory index of the WISC-IV (Petermann & Petermann, Citation2008): During the “Letter-Number Sequencing” test, participants are required to sort a sequence of numbers and letters of increasing length. In the “Digit Span” test, participants have to recall an increasing sequence of numbers that is read out by the examiner, both in the same (forward condition) and in reverse (backward condition) order. The two tasks are combined in a working memory index (WISC working memory index). The age standardized scores were calculated according to the manual (Petermann & Petermann, Citation2008).
To assess visual-spatial working memory, the Corsi block-tapping test was performed (Corsi, Citation1972). Participants observe an examiner tapping a sequence of blocks of increasing length and are required to repeat the sequence either in the same (forward condition) or in reversed (backward condition) order. The total forward and total backward performance was combined to estimate visual-spatial working memory.
The working memory subtest of the TAP (Zimmermann & Fimm, Citation2012) is a computerized 2-back task. A consecutive sequence of digits is presented to the participants, and they are required to indicate whether a digit is the same as the one presented two digits before. Omission and commission errors were added and used as outcome variable. For better comparison with the other working memory tasks, values were inversed, so that higher values indicate better performance.
Typically-developing children completed a short version of the WISC-IV (Waldmann, Citation2008), including one subtest per index, thus, retaining the conceptual structure of the WISC-IV to preserve construct validity. This short-form IQ-estimate was adjusted to avoid overestimation of high-performance and underestimation of low-performance (Ehrler et al., Citation2020). Due to the increased risk of lower general intellectual abilities in children with complex CHD (e.g., Feldmann et al., Citation2021), and, thus, the risk of biased estimates due to underestimation of low scores (Ehrler et al., Citation2020), the general intellectual ability estimate assessed with the full version of the WISC-IV was used for children with complex CHD.
Family socio-economic status (SES) was assessed based on a scoring system of maternal and paternal education (range 2–12), with higher scores indicating higher SES. Parents completed a questionnaire on their child’s health status, including questions related to any neurodevelopmental disorders (e.g., cerebral palsy and ADHD) and the use of glasses and/or hearing aids. Medical information of patients with complex CHD was collected from chart review.
Recording and preprocessing of sleep EEG data
During the seven days prior to the assessment, participants were instructed to adhere to a regular sleep-wake rhythm following their habitual sleep time. Compliance was verified by wrist accelerometry (GeneActiv accelerometer, ActivInsight Ltd.) and by self-reported sleep diaries. On the day of testing, bed- and wake-up times were scheduled according to the usual bedtimes and routines of the next day (e.g., school). In two children with complex CHD and two typically-developing children, recordings were terminated before the usual wake-up time due to discomfort in the EEG net.
A high-density sleep EEG system with 128 channels (Electrical Geodesics Sensor Net for long-term monitoring, Electrical Geodesics Inc., EGI, Eugene, OR, USA) was used to record all-night sleep. In the evening, electrodes were filled with an electrolyte gel. In addition, two gold electrodes (Grass Technologies, West Warwick, RI, USA) were used for chin electromyography (EMG), required for the scoring of sleep stages (Iber, Citation2007), and two as earlobe-references. Impedances were kept below 50 kΩ. EEG was recorded with a sampling frequency of 500 Hz, referenced to the vertex and filtered between 0.01 and 200 Hz. Subsequently, the data were bandpass filtered between 0.5 and 40 Hz and down sampled to 128 Hz. Visual sleep scoring was performed according to the rules of the AASM Manual for scoring sleep (Iber, Citation2007); however, 20-s rather than 30-s epochs were used for a better alignment with spectral analysis, which was performed in 4 s intervals resulting in a standard resolution of 0.25 Hz without any loss of data. Artifacts were identified on a 20 s basis by means of visual inspection during scoring and using a semiautomatic method, which is based on power thresholds in the 0.75–4.5 Hz and 20–30 Hz bands (Lustenberger et al., Citation2015). If more than 96% NREM sleep epochs with artifacts were identified in one EEG channel, the whole channel was excluded from further analysis. Otherwise, epochs with artifacts in any channel were excluded for all channels to ensure the temporal alignment of the signal and to avoid biasing local SWA. After having excluded EEG channels with overall poor signal and electrodes below the ears (including the EMG), data were re-referenced to the average value across the remaining electrodes.
For topographical SWA analyses, the spectral power was calculated for 20 s epochs by means of a fast Fourier transform routine (Hanning window, averages of five 4 s epochs, no overlap, frequency resolution of 0.25 Hz). Subsequently, power values were averaged over the first hour of artifact-free NREM sleep (stages N2 and N3) and 1–4.5 Hz. This time window was chosen because in four participants, recordings did not cover the whole night of sleep (due to EEG-net removal), to include the same number of NREM epochs for each participant (i.e., 180 N2 and N3 epochs) and because this constitutes the most consolidated part of sleep. A similar number of epochs with artifacts, wakefulness or N1/REM sleep was omitted in both groups (13.99 ± 5.34% and 12.64 ± 3.83% in the CHD and the typically-developing group, respectively, p > .05). SWA was then normalized by dividing the power at each electrode by the mean power across all electrodes. Normalization eliminates global EEG power differences, allowing us to investigate local aspects, as it was done previously (Ringli et al., Citation2013; Wehrle et al., Citation2017; Wilhelm et al., Citation2014). Electrode channels with poor signal were interpolated using spherical linear interpolation leading to 109 included channels for each participant.
Statistical analyses
Age, family SES, and sleep architecture were compared between groups with independent t-tests. Sex was compared using Pearson’s Chi-squared test.
All working memory outcomes and IQ were positively correlated with SES (r between 0.36 and 0.60, all p < 0.05, groups pooled); thus, SES was taken into account for group comparisons, applying Analysis of Covariance (ANCOVA). Group differences while controlling for SES are expressed as Cohen’s d (negligible effect d < 0.2, small effect >0.2, moderate effect >0.5, large effect >0.8 (Cohen, Citation1988), F-statistics converted to d (Del Re, Citation2013)). None of the working memory outcome were associated with age at assessment (r between −0.27 and 0.03, all p > 0.05, groups pooled); thus, age was not included when comparing groups. To contrast SWA topography between groups, an independent t-test was performed for each electrode.
To reduce the dimensionality of the working memory outcome for further correlates with SWA, a principal component analysis (PCA) was performed on the Corsi block-tapping test and the WISC working memory index. Because TAP data of five typically-developing children were missing due to technical difficulties, this measure was excluded from the PCA. In PCA, the maximum possible amount of variance of the initial variables is explained by the first principal component (PC1). PC1 covered 79% of variance in performance in the Corsi block-tapping test and the WISC working memory index. PC1 was used as a composite measure of working memory performance for further analyses.
To investigate the association between working memory and SWA, for each electrode, Pearson partial correlation (controlling for SES) was performed between SWA and the working memory composite score, and between SWA and the WISC working memory index, the Corsi block-tapping test and the TAP working memory task, separately. All correlates were computed separately for the CHD and typically-developing groups, and for the pooled cohort. R-values are interpreted according to Cohen as negligible (r < 0.1), weak (r > 0.1), moderate (r > 0.3), and strong (r > 0.5) correlates (Cohen, Citation1988).
For each participant, SWA was then averaged over the significant clusters that resulted from electrode-wise partial correlates of SWA with the working memory composite in the pooled cohort. Linear regression models were computed with working memory as outcome, and local SWA in these clusters, group and the interaction between SWA and group as predictor variables while taking into account the family SES. Because age was neither associated with SWA in these clusters (r between −0.13 and 0.23, all p > 0.05), nor with working memory (see above), it was not taken into account in the final models. The interaction between SWA and group was non-significant for all clusters (left-parietal: t = −0.33, df = 31, p = 0.74; mid-frontocentral: t = 0.09, df = 31, p = 0.93; right-prefrontal: t = −0.26, df = 31, p = 0.79), and, thus, dropped from the final models.
The alpha level of all statistical tests was set to 0.05. Normality of residuals was checked by visual inspection of QQ-plots. All analyses were performed in MATLAB (MathWorks, R2017b) and the R computing environment (version 3.4.2; R Core Team, Citation2020).
Results
Participant characteristics
provides data on socio-demographic and health variables and on general intellectual abilities at 10 years of age in children with complex CHD and typically-developing children, and neonatal and perioperative information in children with complex CHD. Children with complex CHD were seen twice, once for the 10-year follow-up assessment when the WISC-IV was assessed (age range: 9.7 to 11.2 years) and once for the current study (age range: 10.1 and 11.6 years). Typically-developing children were between 9.4 and 11.9 years old at the time of the current study (i.e., all assessments took place at the same time-point). Children with complex CHD were older than typically-developing children at the time of the current study. While the groups were comparable in terms of family SES, IQ scores were lower in children with complex CHD compared to typically-developing children.
Table 1. Sample characteristics.
Group differences in working memory
presents data on the performance in the different working memory tasks in the two groups. The scores in all individual working memory tasks were lower in children with complex CHD compared to typically-developing children, with negligible to moderate effects (d = 0.00 to 0.71). The group differences in the WISC working memory index were statistically significant (t = −2.29, df = 34, p = 0.03, others t = −1.07, df = 32 and t = 0.01, df = 27, all p > .29). The working memory composite was lower in children with complex CHD, with the group difference being moderate (d = 0.59; t = −1.95, df = 33, p = 0.06).
Table 2. Group differences in working memory between children with complex congenital heart disease (CHD) and typically-developing children.
Group comparison of sleep architecture and SWA topography
Sleep efficiency was high in children with complex CHD and typically-developing children and did not differ between the groups (84 [7.3]% and 89 [9.4]%, t = −1.69, p = .10). Across the whole night, children with complex CHD spent more time in stage N1 (t = 3.44, p < .01) and less time in rapid eye movement (REM) sleep (t = −2.58, p = 0.01) compared to typically-developing children. No further parameters of the sleep architecture differed between the CHD and the typically-developing group (Supplementary Table S1).
Neither the length of the time window during which the first hour of NREM sleep occurred – the time interval used for all further analyses – nor the composition of the sleep stages within this window were different between the groups (all p > .12).
The expected topographical distribution of sleep SWA (Kurth et al., Citation2010) with a fronto-central predominance was apparent in the children with complex CHD and in typically-developing children. Electrode-wise comparisons of SWA showed similar SWA topography in both groups (all p > .05, Supplementary Figure S1).
Mapping working memory networks
To map working memory networks, SWA at each electrode was correlated separately with the working memory composite () and the WISC working memory index, the Corsi block-tapping test, and the TAP working memory task, respectively (). Across all participants, the working memory composite correlated strongly positively with SWA in a cluster of seven electrodes over a left-parietal and with a cluster of four electrodes over a right-prefrontal brain region. A strong negative correlation was apparent in a cluster of six electrodes over a mid-frontocentral brain region. The patterns were similar in both groups, and SWA within these clusters of interest was not different between groups (t between −0.11 and −0.81, all p > 0.05; CHD group: 0.7 [0.1], 1.2 [0.2], and 1.0 [0.2]; typically-developing group: 0.7 [0.1], 1.2 [0.2], and 1.1 [0.3], respectively, within the left-parietal, mid-frontocentral, and right prefrontal cluster; mean [SD]). The analyses for the individual working memory tasks resulted in a similar distribution of correlation coefficients across the scalp, with associations being most pronounced in the Corsi block-tapping test.
Figure 1. Correlation of the working memory composite score (a) and the individual working memory tasks (b) with slow wave activity (SWA) topography. Correlation coefficients result from Pearson partial correlates between working memory and normalized SWA (first hour, 1–4.5 Hz), corrected for family socio-economic status. R-values are color-coded and plotted on the scalp-model and significant electrodes are represented as white dots (p <0.05). The working memory composite stems from a principal component analysis on the Corsi block-tapping test and the WISC working memory index.
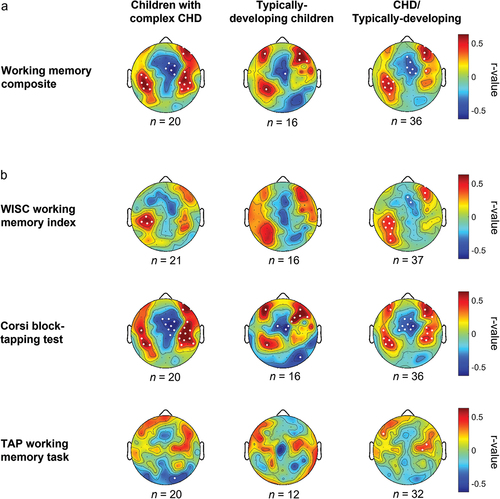
Relationship between working memory and SWA in three regions of interest
To investigate potential alterations within the identified working memory networks in children with complex CHD, the relationship between working memory and SWA in three regions of interest was further investigated. For this, sleep SWA was averaged within each of the three significant clusters that resulted from the correlation of SWA topography and the working memory composite in the pooled cohort (see : all; “Regions of Interest”). SWA in these regions of interest was correlated with the WISC working memory index and the Corsi block-tapping test performance – the tasks contributing to the composite score. Potential interactions with group were investigated but were non-significant (p > .05) and, thus, dropped from the final models. illustrates the relationship between SWA in the three regions of interest, and the WISC working memory index and the Corsi block-tapping test performance. Moderate to strong positive correlates were found for the left-parietal (r = 0.48 and r = 0.44, respectively, both p < 0.01) and the right-prefrontal (r = 0.43 and r = 0.58, respectively, both p < 0.05) regions of interest and moderate to strong negative correlates were found for the mid-frontocentral region of interest (r = −0.36 and r = −0.54, respectively, both p < 0.05). The correlates between SWA in the three regions of interest and the TAP working memory task were negligible to weak (r = −0.01, −0.11, and 0.11, respectively, all p > .55).
Figure 2. Association between slow wave activity (SWA) in three regions of interest and working memory performance. SWA was averaged over each of the three clusters with significant correlates of SWA with the working memory composite (i.e., three regions of interest). R- and p-values result from the partial correlation (controlling for family socio-economic status) between SWA in the regions of interest and working memory performance. Linear regression models revealed no significant interactions between SWA and group and the interaction term was, thus, dropped from the final model.
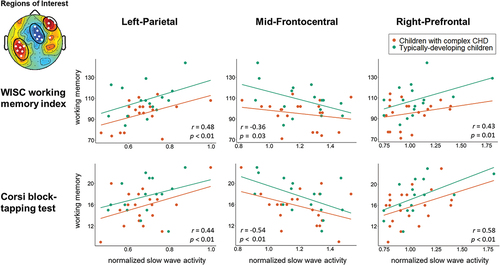
Discussion
This study investigated the functional networks of working memory abilities in children with complex CHD and in typically-developing children using high-density sleep EEG. The results indicate that sleep SWA reliably maps working memory networks in children with and without complex CHD and that the association between sleep SWA and working memory performance is comparable between these groups.
Sleep EEG activity has been suggested to reflect individual traits of functional neuroanatomy, both in children and in adults, due to the high stability of its topographical distribution within and its considerable variability between individuals (De Gennaro et al., Citation2005; Finelli et al., Citation2001; Geiger et al., Citation2012; Lustenberger et al., Citation2017). Sleep SWA – a prominent marker of deep sleep – has been used in numerous studies to successfully map the functional neuroanatomy of motor and cognitive networks. This includes children born very preterm (Wehrle et al., Citation2017) – another clinical population of survivors of neonatal critical illness at risk for neurodevelopmental impairments – and children with attention-deficit/hyperactivity disorder (ADHD; Furrer et al., Citation2020) – a neurodevelopmental impairment quite common in children with complex CHD (Tsao et al., Citation2017).
Interestingly, a previous study in typically-developing children and adolescents had shown that an intensive three-week working memory training resulted in an increase of sleep SWA over a left fronto-parietal brain region and to a lesser degree over a similar region in the right hemisphere (Pugin et al., Citation2015). In the current study, a positive association between sleep SWA and a working memory composite score was identified in overlapping fronto-parietal regions, with a similar predominance in the left hemisphere. Additionally, in the current study, more sleep SWA over a right prefrontal brain region was also associated with better working memory performance. The prefrontal cortex has previously been identified as part of the working memory network in typically-developing children and adolescents using structural and functional imaging modalities (e.g., Gomez et al., Citation2018; Rosenberg et al., Citation2020; Tamnes et al., Citation2013). This implies that sleep SWA assessed with high-density EEG, indeed, may be used to map the key parts of the functional network supporting working memory abilities in children.
The association between less sleep SWA and better working memory abilities over central brain regions that was apparent in the current study has not previously been reported in either children (Pugin et al., Citation2015) or adults (Ferrarelli et al., Citation2019). However, for an executive function composite score, a similar negative association with sleep SWA over central brain regions has been found in children born very preterm and in typically-developing peers – alongside a positive association over frontal regions known to be part of the executive function network (Wehrle et al., Citation2017). It was speculated that this negative association reflects the increased suppression of the default-mode network during task-execution as seen in fMRI studies (Anticevic et al., Citation2012). While this explanation remains speculative, it is interesting that the current study also identified such a negative association when mapping the functional networks of cognitive abilities with sleep SWA. Meanwhile, a functional MRI study in adolescents with complex CHD and typically-developing peers also reported regions of positive associations between activation during a working memory task and performance, predominantly in frontal brain regions, and regions of negative correlates in more central regions (King et al., Citation2017). Further, a study in young adults with CHD reported increased functional connectivity during rest in the default-mode network (Enguix et al., Citation2022), and in children with CHD, reduced regional blood flow in the default mode/salience network was found to mediate poorer cognitive performance (Schmithorst et al., Citation2021). Future studies should continue to investigate this.
In the current study, the functional regions that were mapped to working memory networks were similar in children with complex CHD and in typically-developing children, and the association between sleep SWA and working memory abilities within these regions was not different between the groups. This provides evidence that the functional networks of working memory abilities, generally, are shared between these groups. This is in line with two previous functional MRI studies, which identified overlapping regions of activity during a working memory task in a small sample of seven children with d-transposition of the great arteries and healthy peers (Klaver et al., Citation2016) and in a sample of adolescents and young adults with various types of complex CHD and healthy peers (King et al., Citation2017). Interestingly, in the current study, despite this general overlap, the regions with associations between SWA and working memory appeared to be more widespread in children with complex CHD than in typically-developing children. This may in part be a result of the somewhat larger sample of children with complex CHD. However, along the same lines, larger regions of positive associations between white matter integrity and working memory abilities have previously been reported in adolescents with complex CHD compared to typically-developing peers in a study using diffusion tensor imaging (Ehrler et al., Citation2020). The same study further reported similar associations between better white matter integrity and working memory performance in adolescents with complex CHD and typically-developing peers (Ehrler et al., Citation2020). This corroborates the current findings of similar associations between sleep SWA and working memory abilities in both groups. Other studies have reported differential associations between brain volume and sulcal patterns, and working memory abilities, with patients with CHD showing stronger associations than typically-developing peers (Latal et al., Citation2016; Morton et al., Citation2020; von Rhein et al., Citation2014). Possibly, this reflects different compensational mechanisms of the brain, and, thus, illustrates the persistent impact of early altered brain development on the structural and functional neuroanatomy of patients with complex CHD.
Limitations
A number of limitations require consideration. The children with complex CHD assessed in the current study tended to perform poorer than typically-developing children in all working memory tasks – consistent with what has previously been reported in the literature (e.g., Feldmann et al., Citation2021). However, the group differences were generally non-significant. This should be interpreted cautiously as the sample size of this study was small and, thus, the statistical power was limited. Also, the WISC-IV was assessed either in the afternoon or in the morning in both groups. Possibly, a time-of-day effect may have contributed to the variability and should be addressed in future studies.
The results of the current study were not corrected for multiple comparisons to avoid overly strict interpretation of statistical significance in light of the small sample size. Effect sizes should be used to guide the interpretation of the findings instead. Nonetheless, it is important to note that two of the three clusters identified as part of the functional network of working memory, namely, the left-parietal and the mid-frontocentral cluster, exceeded the level of chance findings due to type-1 error: They include more electrodes than expected by chance, i.e., six and seven electrodes, respectively, compared to 5.5 electrodes expected by chance (5% of 109 electrodes; note that this is a conservative threshold due to the additional requirement that electrodes need to be adjacent to be considered a cluster). While the right-prefrontal cluster included only four electrodes, this brain region is known to be part of the working memory network; thus, this cluster is plausible nonetheless. Several children with complex CHD felt uncomfortable to spend the night at the sleep laboratory at the University Children’s Hospital Zurich. The frequent hospital visits associated with their heart defect may have reduced their willingness to participate in a study involving an overnight assessment. In young children, high-density EEG has successfully been used in a home-setting (LeBourgeois et al., Citation2019). Using this approach in children with complex medical conditions, likely, would increase the participation rate and, thus, result in a larger sample that is representative of the population of children with complex CHD. In fact, it has previously been reported that the prevalence of ADHD is increased in children with complex CHD to approximately 5 to more than 40%, with rates varying substantially between studies and across CHD sub-types (e.g., Davidson et al., Citation2015; DeMaso et al., Citation2014, Citation2017; Hansen et al., Citation2012; Holland et al., Citation2017; Tsao et al., Citation2017). However, in the current study, none of the children with complex CHD was diagnosed with ADHD. This may be due to the small sample size or because children with complex CHD and an ADHD comorbidity were less likely to participate due to the comprehensive study protocol, including an overnight sleep EEG. Assessments in a home-setting could aid the recruitment of these children and improve the generalizability of findings to this sub-group of the CHD population.
The current study focused on sleep SWA (i.e., 1–4.5 Hz). Previously, other frequency bands, most prominently in the sigma range, have also been shown to be associated with cognitive abilities in both children and adults (e.g., Geiger et al., Citation2012; Hahn et al., Citation2019; Schabus et al., Citation2006). Moreover, characteristics of the sleep EEG other than slow waves, such as sleep spindles, have been reported as feasible markers of cognitive functioning (e.g., Reynolds et al., Citation2018). In the current study, this approach was chosen because SWA is a well-established feature of the sleep EEG to map the functional neuroanatomy of cognitive and motor networks in typically-developing children (Kurth et al., Citation2012; Lustenberger et al., Citation2017; Wilhelm et al., Citation2014). Further, a working memory training study has established a causal relationship between increased sleep SWA over specific brain regions and improved abilities, thus, allowing for a hypothesis-driven approach to investigate the neurofunctional network of working memory (Pugin et al., Citation2015). Nonetheless, future studies should take a broader approach and investigate additional frequency bands and characteristics of the sleep EEG, including sleep spindles. Even more, functional connectivity analyses, such as phase lag index or network-based analyses of low frequencies, may be helpful to identify alterations in brain networks of patients with complex CHD as has previously been shown for typically-developing individuals and individuals with neurodevelopmental disorders (Buckley et al., Citation2015; Ujma et al., Citation2019).
Conclusion
Sleep SWA is a reliable tool to map neurofunctional networks of cognitive abilities in both typically-developing children and in children at risk for neurodevelopmental impairments. The current study showed that the functional networks of working memory abilities are generally preserved in children with complex CHD.
Supplemental Material
Download MS Word (511.5 KB)Acknowledgments
We thank everyone involved in recruitment, data collection, and pre-processing, especially Lea Korevaar, Selina Venzin, Susanne Polentarutti, and Caroline Droux. Also, we thank our colleagues at the Department of Pediatric Cardiology, University Children’s Hospital Zurich, Switzerland, for their support of the study. We thank all participating families for their time and loyalty to our studies.
Disclosure statement
No potential conflict of interest was reported by the author(s).
Data availability statement
The approval granted by the ethical committee does not allow the publication of the raw data online. If readers would like to re-analyze the data set (for different purposes), additional ethical approval (on an individual user and purpose basis) will be required. The authors are happy to support additional ethical approval applications from researchers for access to this data set.
Supplemental data
Supplemental data for this article can be accessed at https://doi.org/10.1080/09297049.2022.2140796
Additional information
Funding
References
- Abda, A., Bolduc, M. E., Tsimicalis, A., Rennick, J., Vatcher, D., & Brossard-Racine, M. (2019). Psychosocial outcomes of children and adolescents with severe congenital heart defect: A systematic review and meta-analysis. Journal of Pediatric Psychology, 44(4), 463–477. https://doi.org/10.1093/jpepsy/jsy085
- Anticevic, A., Cole, M. W., Murray, J. D., Corlett, P. R., Wang, X. J., & Krystal, J. H. (2012). The role of default network deactivation in cognition and disease. Trends in Cognitive Sciences, 16(12), 584–592. https://doi.org/10.1016/j.tics.2012.10.008
- Asschenfeldt, B., Evald, L., Heiberg, J., Salvig, C., Ostergaard, L., Dalby, R. B., Eskildsen, S. F., & Hjortdal, V. E. (2020). Neuropsychological status and structural brain imaging in adults with simple congenital heart defects closed in childhood. Journal of the American Heart Association, 9(11). https://doi.org/10.1161/JAHA.120.015843
- Baddeley, A. (2012). Working memory: Theories, models, and controversies. Annual Review of Psychology, 63(63), 1–29. https://doi.org/10.1146/annurev-psych-120710-100422
- Bellinger, D. C., Watson, C. G., Rivkin, M. J., Robertson, R. L., Roberts, A. E., Stopp, C., Dunbar‐Masterson, C., Bernson, D., DeMaso, D.R., Wypij, D. & Newburger, J. W. (2015). Neuropsychological status and structural brain imaging in adolescents with single ventricle who underwent the Fontan procedure. Journal of the American Heart Association, 4(12). https://doi.org/10.1161/JAHA.115.002302
- Buckley, A. W., Scott, R., Tyler, A., Mahoney, J. M., Thurm, A., Farmer, C., Swedo, S., Burroughs, S.A., & Holmes, G. L. (2015). State-dependent differences in functional connectivity in young children with Autism spectrum disorder. EBioMedicine, 2(12), 1905–1915. https://doi.org/10.1016/j.ebiom.2015.11.004
- Calderon, J., Bonnet, D., Courtin, C., Concordet, S., Plumet, M. H., & Angeard, N. (2010). Executive function and theory of mind in school-aged children after neonatal corrective cardiac surgery for transposition of the great arteries. Developmental Medicine and Child Neurology, 52(12), 1139–1144. https://doi.org/10.1111/j.1469-8749.2010.03735.x
- Cassidy, A. R., White, M. T., DeMaso, D. R., Newburger, J. W., & Bellinger, D. C. (2015). Executive function in children and adolescents with critical cyanotic congenital heart disease. Journal of the International Neuropsychological Society, 21(1), 34–49. https://doi.org/10.1017/S1355617714001027
- Claessens, N. H. P., Kelly, C. J., Counsell, S. J., & Benders, M. J. N. L. (2017). Neuroimaging, cardiovascular physiology, and functional outcomes in infants with congenital heart disease. Developmental Medicine and Child Neurology, 59(9), 894–902. https://doi.org/10.1111/dmcn.13461
- Cohen, J. (1988). Statistical power analysis for the behavioral sciences. Lawrence Erlbaum Associates.
- Corsi, P. M. (1972). Human memory and the medial temporal region of the brain. Dissertation Abstracts International, 1972;819B.
- Cowan, N. (2010). The magical mystery four: How is working memory capacity limited, and why? Current Directions in Psychological Science, 19(1), 51–57. https://doi.org/10.1177/0963721409359277
- Davidson, P., Gringras, P., Fairhurst, C., & Simpson, J. (2015). Physical and neurodevelopmental outcomes in children with single-ventricle circulation. Archives of Disease in Childhood, 100(5), 449–453. https://doi.org/10.1136/archdischild-2014-306449
- De Gennaro, L., Ferrara, M., Vecchio, F., Curcio, G., & Bertini, M. (2005). An electroencephalographic fingerprint of human sleep. Neuroimage, 26(1), 114–122. https://doi.org/10.1016/j.neuroimage.2005.01.020
- Del Re, A. C. (2013). Compute.es: Compute effect sizes. R Package
- DeMaso, D. R., Calderon, J., Taylor, G. A., Holland, J. E., Stopp, C., White, M. T., Bellinger, D. C., Rivkin, M. J., Wypij, D., & Newburger, J. W. (2017). Psychiatric disorders in adolescents with single ventricle congenital heart disease. Pediatrics, 139(3), e20162241. https://doi.org/10.1542/peds.2016-2241
- DeMaso, D. R., Labella, M., Taylor, G. A., Forbes, P. W., Stopp, C., Bellinger, D. C., Rivkin, M. J., Wypij, D., & Newburger, J. W. (2014). Psychiatric disorders and function in adolescents with d-transposition of the great arteries. The Journal of Pediatrics, 165(4), 760–766. https://doi.org/10.1016/j.jpeds.2014.06.029
- Ehrler, M., Latal, B., Kretschmar, O., von Rhein, M., & Tuura, R. O. (2020). Altered frontal white matter microstructure is associated with working memory impairments in adolescents with congenital heart disease: A diffusion tensor imaging study. NeuroImage: Clinical, 25, 102123. https://doi.org/10.1016/j.nicl.2019.102123
- Ehrler, M., Latal, B., Polentarutti, S., von Rhein, M., Held, L., & Wehrle, F. M. (2020). Pitfalls of using IQ short forms in neurodevelopmental disorders: A study in patients with congenital heart disease. Pediatric Research, 87(5), 917–923. https://doi.org/10.1038/s41390-019-0667-2
- Enguix, V., Easson, K., Gilbert, G., Saint-Martin, C., Rohlicek, C., Luck, D., Lodygensky, G. A., & Brossard-Racine, M. (2022). Altered resting state functional connectivity in youth with congenital heart disease operated during infancy. PLoS One, 17(4), e0264781. https://doi.org/10.1371/journal.pone.0264781
- Feldmann, M., Bataillard, C., Ehrler, M., Ullrich, C., Knirsch, W., Gosteli-Peter, M. A., Held, U., & Latal, B. (2021). Cognitive and executive function in congenital heart disease: A meta-analysis. Pediatrics, 148(4). https://doi.org/10.1542/peds.2021-050875
- Ferrarelli, F., Kaskie, R., Laxminarayan, S., Ramakrishnan, S., Reifman, J., & Germain, A. (2019). An increase in sleep slow waves predicts better working memory performance in healthy individuals. Neuroimage, 191, 1–9. https://doi.org/10.1016/j.neuroimage.2019.02.020
- Finelli, L. A., Achermann, P., & Borbely, A. A. (2001). Individual “fingerprints” in human sleep EEG topography. Neuropsychopharmacology, 25(5), S57–62. https://doi.org/10.1016/S0893-133x(01)00320-7
- Fontes, K., Rohlicek, C. V., Saint-Martin, C., Gilbert, G., Easson, K., Majnemer, A., Marelli, A., Chakravarty, M. M., & Brossard-Racine, M. (2019). Hippocampal alterations and functional correlates in adolescents and young adults with congenital heart disease. Human Brain Mapping, 40(12), 3548–3560. https://doi.org/10.1002/hbm.24615
- Friso van den Bos, I., van der Ven, S. H. G., Kroesbergen, E. H., & van Luit, J. E. H. (2013). Working memory and mathematics in primary school children: A meta-analysis. Educational Research Review, 10, 29–44. https://doi.org/10.1016/j.edurev.2013.05.003
- Furrer, M., Ringli, M., Kurth, S., Brandeis, D., Jenni, O. G., & Huber, R. (2020). The experience-dependent increase in deep sleep activity is reduced in children with attention-deficit/hyperactivity disorder. Sleep Medicine, 75, 50–53. https://doi.org/10.1016/j.sleep.2019.09.018
- Gaudet, I., Paquette, N., Bernard, C., Doussau, A., Harvey, J., Beaulieu-Genest, L., Pinchefsky, E., Trudeau, N., Poirier, N, Simard, M-N., & Gallagher, A. (2021). Neurodevelopmental outcome of children with congenital heart disease: A Cohort study from infancy to preschool age. The Journal of Pediatrics, 239, 126±. https://doi.org/10.1016/j.jpeds.2021.08.042
- Geiger, A., Huber, R., Kurth, S., Ringli, M., Achermann, P., & Jenni, O. G. (2012). Sleep electroencephalography topography and children’s intellectual ability. Neuroreport, 23(2), 93–97. https://doi.org/10.1097/WNR.0b013e32834e7e8f
- Gomez, C. M., Barriga-Paulino, C. I., Rodriguez-Martinez, E. I., Rojas Benjumea, M. A., Arjona, A., & Gomez-Gonzalez, J. (2018). The neurophysiology of working memory development: From childhood to adolescence and young adulthood. Reviews in the Neurosciences, 29(3), 261–282. https://doi.org/10.1515/revneuro-2017-0073
- Hahn, M., Joechner, A. K., Roell, J., Schabus, M., Heib, D. P. J., Gruber, G., Peigneux, P., & Hoedlmoser, K. (2019). Developmental changes of sleep spindles and their impact on sleep-dependent memory consolidation and general cognitive abilities: A longitudinal approach. Developmental Science, 22(1), e12706. https://doi.org/10.1111/desc.12706
- Hall, D., Jarrold, C., Towse, J. N., & Zarandi, A. L. (2015). The developmental influence of primary memory capacity on working memory and academic achievement. Developmental Psychology, 51(8), 1131–1147. https://doi.org/10.1037/a0039464
- Hansen, E., Poole, T. A., Nguyen, V., Lerner, M., Wigal, T., Shannon, K., Wigal, S. B., & Batra, A. S. (2012). Prevalence of ADHD symptoms in patients with congenital heart disease. Pediatrics International, 54(6), 838–843. https://doi.org/10.1111/j.1442-200X.2012.03711.x
- Holland, J. E., Cassidy, A. R., Stopp, C., White, M. T., Bellinger, D. C., Rivkin, M. J., Newbuger, J. W., & DeMaso, D. R. (2017). Psychiatric disorders and function in adolescents with tetralogy of fallot. The Journal of Pediatrics, 187, 165–173. https://doi.org/10.1016/j.jpeds.2017.04.048
- Iber, C. (2007). The AASM manual for the scoring of sleep and associated events: Rules. Terminology and Technical Specification.
- King, T. Z., Smith, K. M., Burns, T. G., Sun, B., Shin, J., Jones, R. A., Drossner, D., & Mahle, W. T. (2017). fMRI investigation of working memory in adolescents with surgically treated congenital heart disease. Applied Neuropsychology Child, 6(1), 7–21. https://doi.org/10.1080/21622965.2015.1065185
- Klaver, P., Knirsch, W., Wurmitzer, K., & von Allmen, D. Y. (2016). Children and adolescents show altered visual working memory related brain activity more than one decade after arterial switch operation for d-transposition of the great arteries. Developmental Neuropsychology, 41(4), 261–267. https://doi.org/10.1080/87565641.2016.1243115
- Kurth, S., Ringli, M., Geiger, A., LeBourgeois, M., Jenni, O. G., & Huber, R. (2010). Mapping of cortical activity in the first two decades of life: A high-density sleep electroencephalogram study. Journal of Neuroscience, 30(40), 13211–13219. https://doi.org/10.1523/Jneurosci.2532-10.2010
- Kurth, S., Ringli, M., LeBourgeois, M. K., Geiger, A., Buchmann, A., Jenni, O. G., & Huber, R. (2012). Mapping the electrophysiological marker of sleep depth reveals skill maturation in children and adolescents. Neuroimage, 63(2), 959–965. https://doi.org/10.1016/j.neuroimage.2012.03.053
- Latal, B. (2016). Neurodevelopmental outcomes of the child with congenital heart disease. Clinics in Perinatology, 43(1), 173–185. https://doi.org/10.1016/j.clp.2015.11.012
- Latal, B., Patel, P., Liamlahi, R., Knirsch, W., O’Gorman Tuura, R., & von Rhein, M. (2016). Hippocampal volume reduction is associated with intellectual functions in adolescents with congenital heart disease. Pediatric Research, 80(4), 531–537. https://doi.org/10.1038/pr.2016.122
- LeBourgeois, M. K., Dean, D. C., Deoni, S. C. L., Kohler, M., & Kurth, S. (2019). A simple sleep EEG marker in childhood predicts brain myelin 3.5 years later. Neuroimage, 199, 342–350. https://doi.org/10.1016/j.neuroimage.2019.05.072
- Lustenberger, C., & Huber, R. (2012). High density electroencephalography in sleep research: Potential, problems, future perspective. Frontiers in Neurology, 3, 77. https://doi.org/10.3389/fneur.2012.00077
- Lustenberger, C., Mouthon, A.-L., Tesler, N., Kurth, S., Ringli, M., Buchmann, A., Jenni, O. G., & Huber, R. (2017). Developmental trajectories of EEG sleep slow wave activity as a marker for motor skill development during adolescence: A pilot study. Developmental Psychobiology, 59(1), 5–14. https://doi.org/10.1002/dev.21446
- Lustenberger, C., Wehrle, F., Tushaus, L., Achermann, P., & Huber, R. (2015). The multidimensional aspects of sleep spindles and their relationship to word-pair memory consolidation. Sleep, 38(7), 1093–1103. https://doi.org/10.5665/sleep.4820
- Mebius, M. J., Kooi, E. M. W., Hard, C. M., & Bos, A. F. (2017). Brain injury and neurodevelopmental outcome in congenital heart disease: A systematic review. Pediatrics, 140(1). https://doi.org/10.1542/peds.2016-4055
- Morton, S. U., Maleyeff, L., Wypij, D., Yun, H. J., Newburger, J. W., Bellinger, D. C., Roberts, A. E., Rivkin, M. J., Seidman, J. G., Seidman, C. E., Grant, P. E., & Im, K. (2020). Abnormal left-hemispheric sulcal patterns correlate with neurodevelopmental outcomes in subjects with single ventricular congenital heart disease. Cerebral Cortex, 30(2), 476–487. https://doi.org/10.1093/cercor/bhz101
- Mouthon, A. L., & Huber, R. (2015). Methods in pediatric sleep research and sleep medicine. Neuropediatrics, 46(3), 159–170. https://doi.org/10.1055/s-0035-1550232
- Petermann, F., & Petermann, U. (2008). Hawik-iv. Kindheit Und Entwicklung, 17(2), 71–75. https://doi.org/10.1026/0942-5403.17.2.71
- Peyvandi, S., Latal, B., Miller, S. P., & McQuillen, P. S. (2019). The neonatal brain in critical congenital heart disease: Insights and future directions. Neuroimage, 185, 776–782. https://doi.org/10.1016/j.neuroimage.2018.05.045
- Pressler, A. L., Krajewski, K., & Hasselhorn, M. (2013). Working memory capacity in preschool children contributes to the acquisition of school relevant precursor skills. Learning and Individual Differences, 23, 138–144. https://doi.org/10.1016/j.lindif.2012.10.005
- Pugin, F., Metz, A. J., Wolf, M., Achermann, P., Jenni, O. G., & Huber, R. (2015). Local increase of sleep slow wave activity after three weeks of working memory training in children and adolescents. Sleep, 38(4), 607–614. https://doi.org/10.5665/sleep.4580
- R Core Team. (2020). R: a language and environment for statistical computing. R Foundation for Statistical Computing.
- Reynolds, C. M., Short, M. A., & Gradisar, M. (2018). Sleep spindles and cognitive performance across adolescence: A meta-analytic review. Journal of Adolescence, 66(1), 55–70. https://doi.org/10.1016/j.adolescence.2018.04.003
- Ringli, M., Souissi, S., Kurth, S., Brandeis, D., Jenni, O. G., & Huber, R. (2013). Topography of sleep slow wave activity in children with attention-deficit/hyperactivity disorder. Cortex, 49(1), 340–347. https://doi.org/10.1016/j.cortex.2012.07.007
- Rosenberg, M. D., Martinez, S. A., Rapuano, K. M., Conley, M. I., Cohen, A. O., Cornejo, M. D., … Hagler, D. J. Jr, Meredith, W. J., Anderson, K. M., Wager, T. D., Feczko, E., Earl, E., Fair, D. A., Barch, D. M., Watts, R., & Casey, B.J. (2020). Behavioral and neural signatures of working memory in childhood. The Journal of Neuroscience, 40(26), 5090–5104. https://doi.org/10.1523/Jneurosci.2841-19.2020
- Sanz, J. H., Berl, M. M., Armour, A. C., Wang, J. C., Cheng, Y. I., & Donofrio, M. T. (2017). Prevalence and pattern of executive dysfunction in school age children with congenital heart disease. Congenital Heart Disease, 12(2), 202–209. https://doi.org/10.1111/chd.12427
- Schabus, M., Hodlmoser, K., Gruber, G., Sauter, C., Anderer, P., Klosch, G., Parapatics, S., Saletu, B., Klimesch, W., & Zeitlhofer, J. (2006). Sleep spindle-related activity in the human EEG and its relation to general cognitive and learning abilities. The European Journal of Neuroscience, 23(7), 1738–1746. https://doi.org/10.1111/j.1460-9568.2006.04694.x
- Schaefer, C., von Rhein, M., Knirsch, W., Huber, R., Natalucci, G., Caflisch, J., Landolt, M. A., & Latal, B. (2013). Neurodevelopmental outcome, psychological adjustment, and quality of life in adolescents with congenital heart disease. Developmental Medicine and Child Neurology, 55(12), 1143–1149. https://doi.org/10.1111/dmcn.12242
- Schmithorst, V. J., Badaly, D., Beers, S. R., Lee, V. K., Weinberg, J., Lo, C. W., & Panigrahy, A. (2021). Relationships between regional cerebral blood flow and neurocognitive outcomes in children and adolescents with congenital heart disease. Seminars in Thoracic and Cardiovascular Surgery, 25(4), 335–347.
- Siciliano, R. E., Murphy, L. K., Prussien, K. V., Henry, L. M., Watson, K. H., Patel, N. J., Lee, C. A., McNally, C. M., Markham, L. W., Compas, B. E., & Jordan, L. C. (2021). Cognitive and attentional function in children with hypoplastic left heart syndrome: A pilot study. Journal of Clinical Psychology in Medical Settings, 28(3), 619–626. https://doi.org/10.1007/s10880-020-09753-1
- Spillmann, R., Polentarutti, S., Ehrler, M., Kretschmar, O., Wehrle, F. M., & Latal, B. (2021). Congenital heart disease in school-aged children: Cognition, education and participation in leisure activities. Pediatric Research, 1–7.
- Tamnes, C. K., Walhovd, K. B., Grydeland, H., Holland, D., Ostby, Y., Dale, A. M., & Fjell, A. M. (2013). Longitudinal working memory development is related to structural maturation of frontal and parietal cortices. Journal of Cognitive Neuroscience, 25(10), 1611–1623. https://doi.org/10.1162/jocn_a_00434
- Tononi, G., & Cirelli, C. (2006). Sleep function and synaptic homeostasis. Sleep Medicine Reviews, 10(1), 49–62. https://doi.org/10.1016/j.smrv.2005.05.002
- Tononi, G., & Cirelli, C. (2014). Sleep and the price of plasticity: From synaptic and cellular homeostasis to memory consolidation and integration. Neuron, 81(1), 12–34. https://doi.org/10.1016/j.neuron.2013.12.025
- Tsao, P. C., Lee, Y. S., Jeng, M. J., Hsu, J. W., Huang, K. L., Tsai, S. J., Chen, M.H., Soong, W.J., & Kou, Y. R. (2017). Additive effect of congenital heart disease and early developmental disorders on attention-deficit/hyperactivity disorder and autism spectrum disorder: A nationwide population-based longitudinal study. European Child & Adolescent Psychiatry, 26(11), 1351–1359. https://doi.org/10.1007/s00787-017-0989-8
- Ujma, P. P., Konrad, B. N., Simor, P., Gombos, F., Körmendi, J., Steiger, A., Dresler, M., & Bódizs, R. (2019). Sleep EEG functional connectivity varies with age and sex, but not general intelligence. Neurobiology of Aging, 78, 87–97. https://doi.org/10.1016/j.neurobiolaging.2019.02.007
- Verrall, C. E., Yang, J. Y. M., Chen, J., Schembri, A., D’Udekem, Y., Zannino, D., … Cordina, R. (2021). Neurocognitive dysfunction and smaller brain volumes in adolescents and adults with a Fontan circulation. Circulation, 143(9), 878–891. https://doi.org/10.1161/CIRCULATIONAHA.120.048202
- von Rhein, M., Buchmann, A., Hagmann, C., Huber, R., Klaver, P., Knirsch, W., & Latal, B. (2014). Brain volumes predict neurodevelopment in adolescents after surgery for congenital heart disease. Brain, 137(Pt 1), 268–276. https://doi.org/10.1093/brain/awt322
- Vyazovskiy, V. V., & Harris, K. D. (2013). Sleep and the single neuron: The role of global slow oscillations in individual cell rest. Nature Reviews Neuroscience, 14(6), 443–451. https://doi.org/10.1038/nrn3494
- Waldmann, H.-C. (2008). Kurzformen des HAWIK-IV: Statistische Bewertung in verschiedenen Anwendungsszenarien. Diagnostica, 54(4), 202–210. https://doi.org/10.1026/0012-1924.54.4.202
- Wehrle, F. M., Latal, B., O’Gorman, R. L., Hagmann, C. F., & Huber, R. (2017). Sleep EEG maps the functional neuroanatomy of executive processes in adolescents born very preterm. Cortex, 86, 11–21. https://doi.org/10.1016/j.cortex.2016.10.011
- Wilhelm, I., Kurth, S., Ringli, M., Mouthon, A. L., Buchmann, A., Geiger, A., Jenni, O. G., & Huber, R. (2014). Sleep slow-wave activity reveals developmental changes in experience-dependent plasticity. Journal of Neuroscience, 34(37), 12568–12575. https://doi.org/10.1523/Jneurosci.0962-14.2014
- Zimmermann, P., & Fimm, B. (2012). Testbatterie zur Erfassung von Aufmerksamkeitsstörungen (TAP 2.3.): Psytest.