Abstract
Current writing support tools tend to focus on assessing final or intermediate products, rather than the writing process. However, sensing technologies, such as keystroke logging, can enable provision of automated feedback during, and on aspects of, the writing process. Despite this potential, little is known about the critical indicators that can be used to effectively provide this feedback. This article proposes a participatory approach to identify the indicators of students’ writing processes that are meaningful for educational stakeholders and that can be considered in the design of future systems to provide automated, personalized feedback. This approach is illustrated through a qualitative research design that included five participatory sessions with five distinct groups of stakeholders: bachelor and postgraduate students, teachers, writing specialists, and professional development staff. Results illustrate the value of the proposed approach, showing that students were especially interested in lower-level behavioral indicators, while the other stakeholders focused on higher-order cognitive and pedagogical constructs. These findings lay the groundwork for future work in extracting these higher-level indicators from in-depth analysis of writing processes. In addition, differences in terminology and the levels at which the indicators were discussed, highlight the need for human-centered, participatory approaches to design and develop writing analytics tools.
Introduction
Academic writing plays a critical role in higher education, but it is a difficult skill for students to develop irrespective of their language background – i.e. first language (L1) or second language (L2) writers (Ferris, Citation2011; Staples, Egbert, Biber, & Gray, Citation2016). Several meta-analyses have shown that strategy instruction is one of the most effective interventions in improving writing (Graham, McKeown, Kiuhara, & Harris, Citation2012; Graham & Perin, Citation2007). Strategy instruction is defined as ‘explicitly and systematically teaching students strategies for planning, revising, and/or editing text’ (Graham & Perin, Citation2007, p. 449). For strategy instruction, and especially for targeting higher education students who already adopted some (un)successful writing strategies, it is important to gain insight into students’ writing processes; including the cognitive and behavioral actions involved in writing. This can allow teachers to assess students’ writing to evoke students to reflect on the current strategies, and to teach new and more effective writing strategies.
However, it is often difficult or even impossible for teachers to gain an understanding of students’ writing processes, especially in large classrooms or online settings. This may be one of the main reasons why most teachers provide feedback on the characteristics of students’ final writing products rather than the process. Similarly, the number of writing studies that focus on the relationship between the characteritsics of the final text and quality indicators outnumbers by far the studies focused on the writing process (cf. Crossley, Citation2020). This is partly because it is often challenging to gain awareness of the writing processes, as some processes and writing strategies might be implicit and hard to ‘see’. Some insight into these processes can be gained via direct observations, video analysis, or think-aloud protocols (e.g. Solé, Miras, Castells, Espino, & Minguela, Citation2013; Tillema, van den Bergh, Rijlaarsdam, & Sanders, Citation2011). Yet, these approaches are time-consuming, not scalable, and hard to put into practice in realistic educational situations and for the purposes of providing feedback to students.
Automatic data collection has been increasingly proposed as a potential way to shed light on the process writers follow to create their final writing products. One example is keystroke analysis, which has been used to examine the timing of every key pressed during the writing process (Lindgren & Sullivan, Citation2019). Keystroke analysis has been proposed as a scalable solution to capture evidence that could be used to help teachers gain insight into students’ writing processes (Ranalli, Feng, & Chukharev-Hudilainen, Citation2018). However, current indicators extracted from keystroke data are still relatively low-level behavioral features (Galbraith & Baaijen, Citation2019). These include, for example, keystroke frequencies or timings between keystroke events. These indicators require additional sources of contextual information to be meaningful or to point at critical cognitive processes.
As a result, more work is needed to gain a deeper understanding about which indicators need to be extracted from keystroke or alternative sources of data to gain meaningful insights into students’ writing processes. This can inform the design of computer-based language tools that assist writing strategy instruction. We argue that it is important to first determine what indicators of the writing process are desired by different stakeholders (e.g. students and teachers) according to their learning and/or pedagogical intentions. These indicators in turn can be assessed to identify whether they are useful and technically feasible to be obtained. To the best of our knowledge, no prior studies have systematically examined which indicators of the writing process can be useful to support teaching and learning, according to authentic stakeholders’ needs. Therefore, this article proposes a participatory approach to identify what evidence would be useful to extract from the writing process and its potential instructional uses in higher education. These indicators can ultimately be used to develop a computer-based system designed to support writing: a writing analytics tool (or writing tool in short).
Literature review
Writing process models
Writing processes have been deeply studied over the past decades (Bereiter & Scardamalia, Citation1987; Flower & Hayes, Citation1981; Hayes, Citation1996, Citation2012; Kellogg, Citation1996; Leijten, Van Waes, Schriver, & Hayes, Citation2014). Comprehensive overviews of classic writing process models have been reported by Alamargot and Chanquoy (Citation2001), Becker (Citation2006) and Galbraith (Citation2009). In this article, we adopt Flower and Hayes’ (1981) model, as it is the pragmatic model that fits our design purpose. Although the model was originally employed to explain the writing processes in L1 language learning, it has been further adopted in studies aimed at understanding writing across L1 and L2 contexts (e.g. Chenoweth & Hayes, Citation2001, Citation2003). The model defines three cognitive processes involved in writing composition, namely planning, translating, and reviewing. Planning consists in the generation of ideas, organization, and goal setting; translating describes the process of translating these ideas into (written or typed) language; and reviewing consists in evaluating and revising the text produced so far.
These cognitive processes are highly dependent on the writers’ environment, and hence need to be explored within the context of this environment (Van Lier, Citation2000). In addition, these processes are not randomly distributed over the time of the writing process, and hence need to be explored in relation to time, or when they occur during the writing process (Rijlaarsdam & Van den Bergh, Citation1996). Specifically, time needs to be considered because it might give more information about the order and purpose of the processes (Rijlaarsdam & Van den Bergh, Citation1996). As a result, later in this article we will consider the writing processes in relation to time and the particular aspects of the writers’ task environment. These aspects are: (1) the collaborators and critics of the student’s writing (e.g. comments from teachers and peers); (2) transcribing technology used (e.g. using a keyboard versus handwriting); (3) task materials and written plans (e.g. readings and task descriptions); and (4) the text written so far (e.g. the tone of the current version of a manuscript; Hayes, Citation2012).
Data about writing processes
Keystroke analysis has been proposed as a useful tool to gain insights into the writing process (Leijten & Van Waes, Citation2013; Lindgren & Sullivan, Citation2019). However, the use of keystroke data has been criticized because it is hard to associate low-level behavioral actions with higher-level cognitive processes (Galbraith & Baaijen, Citation2019). Yet, various elements, such as pauses, revisions, and production bursts have been successfully related to theory and models on writing processes.
For example, the identification of pauses while writing and, in particular, pauses between words and between sentences (rather than within words) have been related to Flower and Hayes’ (1980) planning and reviewing processes (Baaijen, Galbraith, & de Glopper, Citation2012; Medimorec & Risko, Citation2017). Longer pauses are suggested to indicate a higher cognitive effort (Van Waes, van Weijen, & Leijten, Citation2014; Wallot & Grabowski, Citation2013; Wengelin, Citation2006). Revisions have been related to the reviewing process (Van Waes et al., Citation2014) and non-linearity in the writing process (Baaijen & Galbraith, Citation2018). Lastly, production bursts (i.e. sequences of text production without a long pause; Kaufer, Hayes, & Flower, Citation1986) are described as part of Flower and Hayes’ (1980) translation process. These instances can be identified from keystroke data as fluently produced sequences of keystrokes, without a long pause. Writing processes that are characterized by longer and more frequent production bursts, have been related to higher writing proficiency (Deane, Citation2014).
In sum, keystroke data can, at least to some extent, be used to automatically gain insight into writing processes. However, the current variables extracted from keystroke data, such as keystroke frequency or low-level pauses between keystrokes, may not be directly useful to provide writing feedback. Therefore, in this study we aim to identify what elements of students’ writing processes are desirable for providing feedback on the writing process.
Writing tools: process data and their design
Providing personalized and timely feedback on writing is a time-intensive task for teachers. To address this problem, a wide variety computer-based systems have been developed to support writing instruction and assessment (for an overview, see Allen, Jacovina, & McNamara, Citation2015; Limpo, Nunes, & Coelho, Citation2020). Three main categories of writing tools have been identified based on their functionality: automated essay scoring (AES), automated writing evaluation (AWE), and intelligent tutoring systems (ITS; Allen et al., Citation2015).
AESs are grading systems that can be used for summative assessment, to replace or assist teachers in assessing writing quality (Dikli, Citation2006), for example e-rater (Attali & Burstein, Citation2006). By contrast, AWEs are intended as formative assessment tools, providing more detailed feedback and correction suggestions (Cotos, Citation2015), for example Criterion (Link, Dursun, Karakaya, & Hegelheimer, Citation2014) and AWA/AcaWriter (Knight, Shibani, Abel, Gibson, & Ryan, Citation2020, Knight, Martinez-Maldonado, Gibson, & Buckingham Shum, Citation2017). ITSs are the most complex systems. They can provide automated feedback and instructional interventions, enable interactivity, and can provoke students’ reflection through probing questions (Ma, Adesope, Nesbit, & Liu, Citation2014). ITSs are widely available in domains such as mathematics and business, but less in more ill-defined domains such as reading and writing (Steenbergen-Hu & Cooper, Citation2014). Two examples of ITSs targeted at supporting writing are eWritingPal (Roscoe, Allen, Weston, Crossley, & McNamara, Citation2014) and ThesisWriter (Rapp & Kauf, Citation2018).
All three types of systems have been extensively studied in the writing context and have been shown to enhance student motivation, autonomy, and improve writing quality to different extents (Cotos, Citation2015). However, the majority of these systems use a product-oriented approach, in which feedback is provided on students’ written products (Cotos, Citation2015; Wang, Shang, & Briody, Citation2013). Only some tools provide additional resources to aid the writing process. For example, Criterion provides a portfolio history of drafts, to gain insight into one’s writing progress over time (Link et al., Citation2014); eWritingPal includes lecture videos with animated agents to teach strategies for pre-writing, drafting, and revising (Roscoe et al., Citation2014); ThesisWriter suggests some strategies for improving research report writing (Rapp & Kauf, Citation2018), and the Inputlog Process Report consists of an automatically generated text file addressing different perspectives of the writing process: pausing, revision, source use, and fluency (Vandermeulen, Leijten, & Van Waes, Citation2020). Apart from the latter, none of these tools yet collect evidence from the writing process nor provide feedback on specific writing processes.
Indeed, automated, personalized writing tools are generally hard to develop for writing, for at least two reasons. First, as writing is an ill-defined domain (Allen et al., Citation2015; Steenbergen-Hu & Cooper, Citation2014), writing specialists need to be involved in the development of such tools (Cotos, Citation2015). Second, these systems are used less and less effective if they are not integrated into instructors’ learning design (i.e. the teaching materials, the sequencing of learning tasks; Link et al., Citation2014; Lockyer, Heathcote, & Dawson, Citation2013). Most of current studies reporting on writing tools have included specialists, teachers, and other stakeholders only after the development of such tools (El Ebyary & Windeatt, Citation2010; Rapp & Kauf, Citation2018; Roscoe et al., Citation2014).
The issue of not invoking the voices of educational stakeholders in the design of data-intensive, learning analytics tools is also prevalent in other areas of application (Prieto-Alvarez, Martinez-Maldonado, & Anderson, Citation2018). In response, there has been a growing interest in collaborating with educational stakeholders early on in the design of writing analytics tools, and learning analytics tools in general (e.g. Buckingham Shum, Ferguson, & Martinez-Maldonado, Citation2019; Dollinger, Liu, Arthars, & Lodge, Citation2019; Martinez-Maldonado et al., Citation2016; Wise & Jung, Citation2019) By including information from writing specialists to identify why and how particular affordances are needed, rather than simply including all features that are technically feasible, the design could be improved (Cotos, Citation2015). This way, the design can also be better tuned to the educational context (Conde & Hernández-García, Citation2015). When writing tools are tuned to the educational context, they can be perceived more positively by students, resulting in a higher adoption (Shibani, Knight, & Shum, Citation2019).
This article presents a study which illustrates our participatory approach by conducting participatory sessions with educational stakeholders before the design of writing analytics tools, to determine what indicators of students’ writing processes are desirable to provide automated, personalized writing feedback in higher education and how these can be connected with teachers’ learning designs.
Approach
A qualitative research design was implemented to identify what evidence would be useful to extract from the writing process and its potential instructional uses in higher education. The importance of qualitative research in computer assisted language learning has been recently emphasized, as it can inform the design, development, and evaluation of language tools through a deeper understanding of the stakeholders involved (Levy & Moore, Citation2018). As a result, participatory sessions were conducted, using the focus group technique (Kidd & Parshall, Citation2000). These sessions aimed at capturing multiple stakeholders’ perspectives on the indicators that can provide meaningful insights into students’ writing processes and that can be used in the design of writing analytics tools.
Within a participatory approach, it is important to include different groups of stakeholders, as the ideas might differ across stakeholders (Woolner, Hall, Wall, & Dennison, Citation2007). Different stakeholders in writing instruction have shown to feature quite different perceptions on academic writing (Itua, Coffey, Merryweather, Norton, & Foxcroft, Citation2014; Lea & Street, Citation1998; Wolsey, Lapp, & Fisher, Citation2012). For example, students have indicated content and knowledge as the two most important criteria items for assessing essay writing (Norton, Citation1990), while teachers consider argument and structure to be the key items they use in their assessments (Lea & Street, Citation1998; Norton, Citation1990). Therefore, we included five groups of stakeholders in our study: bachelor students, PhD students, teachers, professional development staff, and writing researchers. Bachelor and PhD students were chosen to represent groups of students with relatively low and relatively high experience in academic writing, respectively. More expert writers tend to be more strategic in their writing processes, compared to novice writers (Kaufer et al., Citation1986), and hence might desire insight into different aspects of their writing process. Teachers and professional development staff were included, to identify desired indicators from the teacher and teacher trainers’ perspective. Lastly, writing researchers were included to identify desired indicators based on writing research and theory, and to better connect writing analytics to educational practice (cf. Buckingham Shum et al., Citation2016).
Outcomes from the participatory sessions can be used by researchers, designers, and educational technology vendors to inform the design and development of computer-assisted writing tools. In addition to design recommendations, we specify what information needs to be collected to provide (automated) feedback and identify to what extent this could be done using keystroke analysis. Accordingly, this study illustrates how a human-centered approach can be adopted into the particular context of writing analytics, which can also be useful for the broader area of learning analytics.
Specifically, we aim to address the following research questions:
RQ1: What indicators of students’ writing processes are considered desirable by multiple stakeholders for providing feedback on the writing process?
RQ1(a): What are the desired indicators per stakeholder group?
RQ1(b): What are the similarities and differences in the desired indicators across stakeholder groups?
RQ2: How can indicators of students’ writing processes be integrated into (computer-based) learning and teaching practices?
Method
Participants
Five participatory sessions were conducted with the five representative groups of stakeholders. In total 25 stakeholders participated: four university teachers, five bachelor students, six PhD students, six professional development staff (teacher trainers), and four writing experts (writing researchers). The bachelor students were recruited via the university’s participant pool. The teachers and professional development staff were recruited by email via the university’s language center. The PhD students and writing researchers were recruited via the authors’ personal network. Both bachelor and PhD students were selected based on whether they completed an academic writing course. Teachers were selected based on their years of experience (>10 years) in teaching academic writing, professional development staff were selected based on their years of experience in teacher training (>5 years), and writing experts were selected based on their years of experience in writing research (>2 years). Students came from the fields of Sociology, Communication, Cognitive Science, and Artificial Intelligence, and were a mix of L1 and L2 writers (i.e. three students were L1 English writers; five were L1 Dutch and L2 English writers; and three were L2 English writers). Teachers and professional development staff worked across a wide variety of fields, including Arts, Social Sciences, Business, Law, Science, and Engineering, teaching both L1 and L2 writers.
Materials and procedure
After the participants provided informed consent, participants were asked to fill out a short demographics’ questionnaire. Thereafter, the goals, procedure, and rules for the focus group were explained. The focus group consisted of two parts, focused on the respective research questions. For these two parts, a semi-structured, open-ended schedule was developed.
The first part focused on capturing participants’ perspectives on the writing process and how evidence about the writing process could be used to support teaching and learning. In the sessions with teachers, writing researchers, and professional development staff, two questions were asked in the following order:
What do you think an instructor would like to learn about students’ writing processes?
What do think would be useful to show a student about their writing process?
For both student focus group sessions there were three questions:
What would you, as a student, like to learn about your writing process?
What do you think an instructor would like to learn about students’ writing processes?
What do you think an instructor should not see about students’ writing processes?
To avoid social pressure, participants were first asked to write down their ideas on sticky notes (one idea per note). Participants got two minutes per question. Thereafter, they were asked to read their ideas out loud and discuss them (10 minutes). Participants were encouraged to write down new ideas if needed and they were asked to cluster the sticky notes with similar ideas, and to name these clusters. Lastly, participants were asked to vote for what they consider were the three ‘best ideas’.
In the second part, participants were asked to write a use case of an intervention using one or more of the ideas generated earlier. An exemplar was first shown for them to understand the expected format of their use case (e.g. see ). Then, participants had 10 minutes to write their own use cases, emphasizing the context (learning design of the learning situation), state and form of the intervention (tool set, strategies/actions needed and by whom?), and expected outcomes. Afterwards, participants were given 10 minutes to discuss and expand their cases.
Table 1. Example of the use case for an intervention provided to the participants.
By the end of the session, participants had the possibility to add any further ideas or ask questions in a debrief. All sessions lasted 60–75 minutes and were moderated by the first author of this article. To minimize the influence of the moderators’ viewpoint on the discussion, participants were encouraged to moderate the discussions by themselves. When necessary, the moderator only asked open format follow-up questions, such as: Could you provide some more details? or Why do you feel this is important?
Analysis
NVivo was used to transcribe the audio recordings of the sessions and for the qualitative analysis of the transcripts, sticky notes, and use cases (NVivo, Citation2015). The names of the clusters of sticky notes produced by participants were used as topics of desired indicators following an inductive thematic analysis approach (Braun & Clarke, Citation2006), in which codes emerged from participants’ discussed topics. The dialogue of participants while generating the notes was then coded using these topics, allowing us to interpret the (clusters of) sticky notes in the context of the dialogue. Using the coded transcripts and sticky note clusters, the first author analyzed which indicators were most important, and which were highly connected to other concepts. The importance of a topic was determined by the number of sticky notes and votes on that topic. The connectedness of the topics was determined based on the co-occurrence of topics in the conversation.
The prevalence of topics was then compared across the five groups of stakeholders. To compare the topics generated in each session, they were mapped to the theoretical model of writing, developed by Flower and Hayes (Citation1981), which distinguishes the three cognitive processes in writing defined above (planning, translating, and reviewing). We also included the ‘monitoring’ process, which describes the strategic or self-regulation processes controlling these three cognitive processes or the writing process in general which monitors the writer across the cognitive processes. All topics were mapped into one of the three writing processes or into the monitoring process. Additionally, we indicated whether a topic was discussed in the context of an aspects of the writers’ task environment, as defined by Hayes (Citation2012): (1) collaborators and critics; (2) transcribing technology; (3) task materials and written plans; and (4) text written so far. The mapping of the topics into the theoretical models of writing was conducted by four of the authors, via thorough discussion and developing consensus.
Previous work on stakeholders’ perceptions of academic writing distinguished lower-level indicators, related to behavior and higher-level indicators, related to cognitive processes (Lea & Street, Citation1998; Norton, Citation1990). Therefore, the same four authors categorized the topics in terms of the level at which the indicators of the writing processes were discussed, via thorough discussion and developing consensus. Topics were coded as behavioral when behaviors or actions were described, while topics were coded as cognitive, when the topics were related to cognitive processes, e.g. when the dialogue around this topic mostly consisted of words such as develop, ideas, thoughts, understand, and experience (e.g. how do ideas develop?). During the discussion, a clear distinction emerged between lower-level and higher-level behavioral indicators, and hence these were coded as separate categories. Specifically, the topics were coded as low-level behavioral indicators when the dialogue around this topic solely consisted of words related to frequency (the number of), total time spent, or occurrence of behavior (e.g. do they plan?). The topics were coded as higher-level behavioral indicators when they went beyond the lower-level metrics by describing the indicators in the larger context of writing, for example by describing a sequence of behaviors (e.g. how do students plan?), behavior in relation to the writing product (e.g. which sections required a lot of effort?), or behavior in relation to time or the writing process (e.g. how do revisions change over time?).
Lastly, the use cases were analyzed. We compared the main focus of the use cases in each focus group, in regard to how stakeholders’ ideas can be integrated into the learning design. We especially contrasted the desired properties of the tool, the strategies and actions needed, and the actors involved in the intervention.
Results
This section first presents the topics of desired indicators of students’ writing processes per stakeholder group. Next, the indicators are compared and contrasted across the stakeholder groups. Lastly, results of the analysis of the use cases are presented, which indicate stakeholders’ opinions on how these indicators can be integrated into learning and teaching practices.
Identifying topics of desired indicators per stakeholder group
Bachelor students
The bachelor students wrote a total of 40 ideas on sticky notes. These were categorized into nine topics (one idea was left uncategorized because the students argued it was not related to the other ideas). An overview of the topics, ordered by the number of sticky notes, followed by the number of votes is shown in . Although only discussed once, typing patterns received the most votes and sticky notes of all topics. This topic was mostly related to keyboarding skills and was the only topic that was considered desirable for both students and teachers. Planning was rated as second most important topic. The students would like teachers to know how they prepared and planned for the task and what their initial ideas were, especially to be able to receive feedback on these ideas. In addition, students would like information on the number of words and characters typed, categorized as general structure, to be able to determine whether they met the assignment requirements.
Table 2. Topics identified in the bachelor students focus group (N = 5).
In general, students stated that teachers could use the information on students’ writing process to improve instruction. For example, a student stated this as follows: ‘… in terms of sentence framing, grammar usage, APA style, fonts and stuff, the teachers would want know what students’ exposure is on these kinds of terms. And I think based on that, you could build a lecture or a class around it’. The students differed in opinion whether certain aspects of the writing process should remain invisible to teachers. Some stated there was nothing they wanted to hide, but others noted that they would not like the teacher to know about the grammatical errors they already fixed. Students were especially worried that insufficient time spent, or a messy draft could negatively influence the teachers’ perception on their writing.
PhD students
The PhD students wrote 36 ideas and categorized those into eight topics (two ideas were uncategorized; ). Time or productivity were the central themes addressed at several points throughout the discussion. Students were interested in how they could reduce ‘staring at an empty screen time’ and whether it would be possible to predict the best time of the day to write. The main goal of this was to be more productive or postpone less. The PhD students considered that information on time and productivity should not only be available to themselves, for time self-regulation, but also to their supervisors. This was stated by one PhD student as follows: ‘I would really like my supervisors to be able to help me to produce something earlier’. However, some students disagreed with that viewpoint. They rather not let their supervisors know how much time they spent on writing, or whether they wrote in the middle of the night, because they did not want to get criticized on this ‘unhealthy work habit’.
Table 3. Topics identified in the PhD students focus group (N = 6).
In addition, the PhD students were interested in their revision behavior, by detailing where and when they revised. In particular, they were interested in how feedback and comments from supervisors or reviewers affected their writing, both positively and negatively. Some PhD students argued they did not want to disclose this information to their teachers. A student stated ‘I do not want [my supervisors] to know that I don't agree with what I'm writing […] sometimes you just want to please your supervisor. —I specially have this with reviewers as well’. Again, some PhD students disagreed and said they had nothing to hide.
Teachers
The teachers wrote 37 ideas and categorized those into 10 topics (see ). They provided detailed headers and, accordingly, most topics were discussed only once. The teachers were mostly interested to provide students with information on their language, especially regarding style or ‘language that is not necessarily incorrect’. For example, feedback could be provided on how to improve the text by making the language more formal or using a wider variety of sentence structures. They stressed that this feedback should not be directive, but rather should focus on what could be improved. In this way, students still need to think about how to improve the language and style.
Table 4. Topics identified in the teachers focus group (N = 4).
Teachers were interested in improving their own instruction about the linearity of writing. For example, some teachers would like to know in what order the different sections were written by the students. Additionally, they wanted to gain an understanding about how feedback, and specifically peer feedback, can play a role during revision. One teacher suggested that it would be useful to reflect on evidence to answer the following: ‘How do students use feedback to revise their work? Do they go through comments one by one, or do they focus on one type of error comment?’. In addition, the depth of students’ revisions was highlighted, such as the significance of the changes and the difference between language versus content revisions.
Professional development staff
The professional development staff wrote 46 ideas spread over 11 topics (). A main theme in the first two topics was source-based writing, or how students use information in their writing (using evidence). These topics were also highly related to reading. For example, a staff member rose the following question that would ideally be desirable to be addressed with evidence: ‘What kind of information do students extract from literature and how do they extract this?’. The professional development staff would like to provide this information to students, to show them how to map their evidence, and how to use resources judiciously; but also, to teachers, to determine whether students needed additional instruction. For example, a staff member suggested this could be achieved by providing them workshops on reading into writing to ‘scaffold the reading, evaluating, and synthesizing processes’.
Table 5. Topics identified in the professional development staff focus group (N = 6).
The concepts of reading into writing and using evidence were also related to plagiarism. A staff member mentioned: ‘We assume that everyone is going to draw on published readings for assessments in some way, or readings provided by the lecturer, but I want to know, what else are they using?’. In addition, the professional development staff highlighted the critical role of metacognition for students to understand the processes involved when writing. They would like students to know whether they are on the ‘right track’ or provide information on what steps they need to go through when writing an assignment.
Writing researchers/specialists
Lastly, the writing researchers generated 22 ideas, which were grouped into seven topics (one idea was uncategorized; ). First, they would like teachers to know where students struggle during the writing assignment. This idea was rather clear for all researchers and only discussed briefly. Second, time was a recurring theme during the discussions. Time was discussed in terms of duration, or the time spent on the assignment, but also in terms of the order of the different activities during writing, such as when students think and reflect on their writing. In addition, the periodicity of writing was discussed. ‘Did they write everything at once, or in regular or irregular chunks spread over a period of time?’.
Table 6. Topics identified in the writing researchers focus group (N = 4).
Third, researchers were interested to gain information on students’ revisions; whether these are good enough to improve writing quality. The main goal was to encourage students to engage in critical thinking, to ‘help students write more critically rather than descriptively’ or to simply think or revise more, or to revise at deeper levels. This was also related again to the time spent on writing, as shown in the following statement from one of the researchers: ‘Give [the students] a little bit of information on how much time they spent and how much time the other students are spending. And then suggest them to reflect on what they have written so far’.
Comparing topics of desired indicators across stakeholders
To compare and contrast the topics across stakeholder groups, the topics were mapped into the planning, translating, reviewing, and monitoring processes. In addition, they were ordered in terms of the level at which the indicators were described: low-level behavioral; behavioral in relation to time (ordering, scheduling) or the writing process; or higher-level cognitive. depicts the mapping of the topics and shows several similarities and differences between the topics discussed. All stakeholders discussed the three writing processes: planning, translating, and reviewing as well as the monitoring process. In addition, all stakeholders emphasized time. Lastly, all focus groups discussed topics related to the task environment and discussed both behavioral and cognitive indicators of the writing process.
Figure 1. Overview of the topics of desired indicators discussed by all stakeholders.
Notes. Topics are mapped to the process they address in Flower and Hayes’ (1981) model of writing processes (x-axis) and level of the indicators (behavioral versus cognitive; y-axis). Topics related to concepts in the writing environment (Hayes, Citation2012) are grouped in the rectangular boxes. Letters in the circles (T = teacher, S = student, NT = not teacher) indicate the stakeholders who should have access to these data.
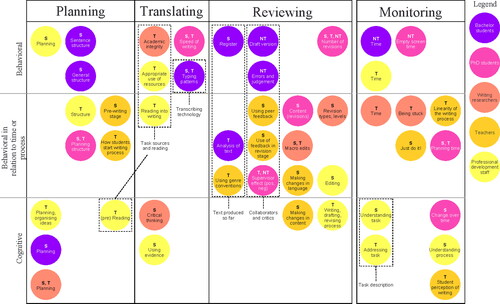
Several differences were found across the stakeholder groups:
First, some stakeholder groups focused more on behavioral indicators (first row in ), while others focused more on cognitive indicators. Bachelor students discussed mostly low-level behavioral indicators (e.g. number of keystrokes). PhD students also discussed behavioral indicators, but usually in relation to scheduling time or the writing process (e.g. what is the best time of the day to write). Teachers, writing researchers, and, especially, professional development staff discussed higher-level cognitive indicators, such as the understanding of the writing process or critical thinking.
Second, the different aspects of the task environment (e.g. task sources or collaborators/critics; rectangular boxes) were not discussed by all groups. For example, the task description was only discussed by the professional development staff, while the text produced so far and collaborators/critics were only discussed by bachelor students, PhD students, and teachers.
Lastly, some stakeholder groups argued that a certain topic would only be of interest for either students or teachers (indicated by an S or T in , respectively), but not for both, while other groups considered that the topics would be of interest for both. For example, the professional development staff thought it would be useful for students to know whether they understood the task, while it would be of interest for teachers to know whether the students addressed the task.
A closer look into the discussions revealed one additional key difference between stakeholders in terms of the terminology each group used. These differences were especially apparent in discussions around time, planning, and revision. For example, all stakeholders discussed time in terms of duration, or how long it took to write. However, while most stakeholders reported duration was something the teachers should see, bachelor students specifically stated that teachers should not see this. All stakeholders except from teachers discussed time in terms of the time until the deadline or when the student started to write. All stakeholders, except from bachelor students, discussed time at a deeper level. On the one hand, teachers and writing researchers mentioned the ordering of the writing process, such as at what points in time students stop to reflect and revise, and the ordering of the writing product, such as which paragraph was written first. On the other hand, PhD students focused on scheduling writing during the day and over multiple sessions.
Likewise, different conceptualizations and properties of planning and revision were discussed. Planning was discussed in terms of planning structure, content, or language use, where planning structure was most often discussed. Planning content was only discussed by PhD students, teachers, and professional development staff, while planning language was only mentioned by professional development staff and bachelor students. Revision was discussed in terms of the different characteristics of revision. Depth of revision, such as surface-level versus structure or document (deep-level) changes was heavily discussed by all stakeholder groups, but with different terminologies. Other properties of revision discussed were included: the temporal location of revision, when revisions were made (PhD students, professional development staff, writing researchers); the spatial location of revision, such as which parts have been revised (PhD students, writing researchers); the quality of revision (professional development staff, writing researchers); and the order of revisions (teachers).
Integration into the learning design
After identifying the desired indicators for the stakeholders, we examined how these indicators could be integrated into learning and teaching practices, by designing use cases. Interestingly, most stakeholders within the same focus group choose the same or a similar idea to integrate into the learning design. All use cases consisted of tools that would not ‘fix’ a given problem, but rather would advise or suggest strategies to address the problem.
Specifically, professional development staff would like to have a tool to help students during reading, for integrating resources in their writing, and for synthesizing evidence. This tool would need to automatically pop-up during reading and writing, and help students by scaffolding reading into writing, with models, examples, guidelines, and strategies. It would need to be tailored to the disciplinary context, and students might actively choose what they want help with, and what kind of text (discipline) they are reading. The writing researchers proposed a similar tool, to help students critically reflect on their text. A message would pop-up when few or only low-level revisions are made or after a long time of inactivity. The tool would address what could be improved by using examples (from their own writing) and encourage students to critically reflect on what they wrote so far.
The tools envisioned by the bachelor students and teachers were focused on lower-level aspects of writing. Bachelor students envisioned a tool similar to a spell-checker, built in into their word processing software. The tool would flag incorrect referencing format, suggest words if the student is struggling finishing a sentence, and suggest synonyms if a word is not from the academic register. Teachers came up with a comparable tool, to flag informal words and suggest more formal words. In this way, students would spend less time on these lower-level aspects of the text and would have more time left for structuring their argument. PhD students would like to have a dashboard, which keeps track of their productivity and number of revisions per section, for each writing session. This dashboard would be used before a new writing session, to identify the most productive time of the day, which section requires more attention, or the best time when to take a break.
Regarding the tools to aid teachers, professional development staff would like to show videos of an expert’s writing process to first-year students, to show how ideas develop over time. This would be used for workshops and instructions (face-to-face or blended) on strategies for approaching and scaffolding reading and writing. Another tool mentioned would measure the amount of critical reflection. This would be used to inform instruction, by using models and examples to explain how to critically reflect on reading and writing within the specific discipline.
Discussion
In this study, we aimed to determine the indicators of higher education students’ writing processes that are desirable to provide automated, personalized writing feedback for, and how this could be implemented into the learning design. Ultimately, this can be used to inform the design and development of (process-oriented) writing tools. The indicators were elicited and use cases for these indicators were developed through participatory sessions with bachelor students, PhD students, teachers, professional development staff, and writing researchers. All groups noted a variety of indicators which were grouped into self-generated categories. We mapped these categories into the planning, translating, reviewing, and monitoring processes as described by Flower and Hayes (Citation1981). In addition, we coded the level of the indicators, ranging from low-level behavioral to higher-level cognitive indicators. This classification proved to be useful to compare and contrast the ideas between the different stakeholders and resulted in four main findings. These main findings and their implications for writing tool design as well as writing process research are discussed below.
First, we identified which indicators are desired by different stakeholders for providing automated feedback on the writing process. Currently, many writing tools solely provide summative and formative feedback on the writing product, rather than the writing process (Allen et al., Citation2015). Our findings provide insight into desirable features and functionality to be considered in the development of a new writing tool or to extend existing writing tools. All stakeholder groups identified features in each of the major writing processes: planning, translating, reviewing, and monitoring, as described by Flower and Hayes (Citation1981). Desired indicators for each of these processes, respectively, were, for example, information on students’ planning strategies, how students used evidence in their writing, the depth of revisions, and students’ understanding of the task. These desired indicators show which indicators may be prioritized to be automatically extracted from sensing technologies such as keystroke logging, to be included into writing tools. For example, the depth of the revision was discussed by all stakeholders. Accordingly, a writing tool could be developed to provide insight into the depth of the revision, which could be used by students to support their reflection and by teachers to provide more effective feedback.
Second, we showed that the level at which the indicators were discussed varied between the five stakeholder groups. These findings corroborate previous literature, which also indicated that students and teachers differ in their perceptions of academic writing (Itua et al., Citation2014; Lea & Street, Citation1998; Wolsey et al., Citation2012). Students focus more on lower-level indicators such as content and knowledge (Norton, Citation1990), while teachers focus more on higher-level indicators, such as argument and structure (Lea & Street, Citation1998; Norton, Citation1990). However, these previous studies mostly determined differences in perceptions of writing in relation to the writing product. In the current study, we showed that these differences also hold for perceptions of the writing process. Bachelor students focused on rather low-level behavioral indicators of the writing process, such as the number of keystrokes. By contrast, teachers, writing researchers, and especially the professional development staff focused on higher-level cognitive indicators, including critical thinking and the understanding of the writing process.
Third, extending on previous work which identified two levels at which indicators were discussed (Lea & Street, Citation1998; Norton, Citation1990), we distinguished a third (intermediate) category, in which behavioral indicators were discussed in relation to time or the writing process. Researchers have argued that time needs to be considered when studying writing processes, as it might provide information regarding the purpose of writing processes or how sequences of cognitive processes differ across writers (Rijlaarsdam & Van den Bergh, Citation1996). For example, both novice and expert writers might show the same frequency of cognitive activities, but expert writers might know when they need to engage in which activity. Indeed, we found that PhD students more often discussed behavioral indicators in relation to time, e.g. what is the best time of the day to write, compared to bachelor students. This indicates that bachelor students, to become more expert writers, might need more active instruction to consider their writing actions in relation to time and the writing process.
Lastly, next to the different levels at which the indicators where discussed, we also found that the terminology on similar aspects of the writing process differed across the stakeholder groups. This indicates that students might need additional explanations to understand the higher-level aspects of the writing process. These explanations can come from the teachers (e.g. face-to-face or blended, in combination with the writing tool) or might be automatically triggered. Previous work already showed that feedback related to specific parts in the student text (specific feedback) is more effective and requires less mental effort compared to general feedback (Ranalli, Citation2018). Hence, to provide better explanations of the writing process, it might be good to tie the feedback to specific examples in the writing product.
Overall, these findings validate the usefulness of engaging multiple stakeholders in the identification of the key metrics that could be included into the design of writing analytics tools.
Implications for designing writing tools
Our findings have implications for the design of the writing tools. The differences in terminology and levels at which the indicators were discussed further highlight the need for a human-centered approach (Giacomin, Citation2014) and, hence, the need for stakeholder involvement in the development of writing tools. In addition that, this indicates that either a common language need to be created to talk about writing processes, or different interfaces need to be developed for different stakeholders (Gabriska & Ölveckỳ, Citation2018; Teasley, Citation2017).
Finally, the use cases provide some practical implications for the (further) development of writing tools and the integration into the learning design. First, the tool should be tailored to the disciplinary context. The tool should (preferably automatically) detect a problem, but should not fix the problem, rather, it should provide instruction to address it. Professional development staff still preferred this instruction to be face-to-face or, if necessary, blended, indicating the importance of instructors’ willingness to adopt the technology into the classroom (cf. Link et al., Citation2014). In addition, this indicates that the resulting intervention should include both the detection of the problem (with the tool), as well as instruction (with or without the tool). This further stresses the claim made by Wise and Jung (Citation2019) who also indicated the importance of studying how tools are used in real educational contexts.
Implications for writing process research
The indicators identified in this study have important implications for writing process research, as writing process research should determine whether and how these indicators could be automatically extracted. This can advance writing process research into a direction that might be more suitable for writing instruction. Several of the indicators identified by the stakeholders have already been extracted by keystroke analysis. This specifically holds for the lower-level behavioral features, such as the number of keystrokes (e.g. Allen et al., Citation2016), total time spent writing (e.g. Bixler & D’Mello, Citation2013), and the number of characters that stayed in the final product (e.g. Van Waes et al., Citation2014). However, our study showed that for providing automated and personalized feedback, it is critical to extract these behavioral indicators in relation to time or when they happen in the writing process. For example, this includes indicators such as the order of error revisions and the change in writing fluency over time. To date, little work has examined the temporal aspects of the keystroke data, with some exceptions (Likens, Allen, & McNamara, Citation2017; Xu, Citation2018; Zhang, Hao, Li, & Deane, Citation2016). Therefore, we suggest future work should focus on sequence mining and temporal analysis of the keystroke data, rather than only extracting summarized frequency metrics.
We also showed that higher-level cognitive features are considered desirable for providing feedback, such as how students synthesize evidence sources into their writing or how their ideas and concepts develop over time. Some of these indicators might not be accessible via keystroke data and thinking-aloud or structured reflection and planning tasks might be more suitable methods. Alternatively, these aspects can be explicitly addressed in writing instruction, as part of a pedagogical approach that focuses on writing processes. To further fill the gap between keystroke data and cognitive processes, and especially to provide feedback, future work should investigate these data in combination with other sources of contextual information (Galbraith & Baaijen, Citation2019). For example, natural language processing on the text composed during the writing process in combination with temporal analysis could be used to extract different features related to revision, which could indicate the depth, timing, and location of the revision (see e.g. Zhang & Litman, Citation2015). In this way, the indicators within a writing tool will be better aligned with the desires or pedagogical intentions of the educational stakeholders.
Limitations
The findings in this study are limited in two ways. First, we only analyzed five stakeholder groups. Within these groups, all students came from similar disciplines, while the teachers had different backgrounds. Disciplinary background has shown to have an impact on teachers’ opinions on most important elements of students’ writing (Lea & Street, Citation1998) and on students’ conceptions of essay writing (Hounsell, Citation1984, Citation1997). Therefore, additional focus groups with different disciplines could have resulted in more and other indicators. However, we did not aim to provide a full overview of all indicators desirable for providing feedback. We showed how a participatory approach could provide insight into what types of indicators are considered useful and how this could be integrated into the learning design. A possible future step in the design process would be to feed these insights back to the stakeholders, to comment on each other’s insights and close the feedback loop.
Second, we focused on indicators that would be considered desirable to provide automatic and personalized feedback. However, desirable indicators are not necessarily technically feasible or useful indicators. This study described the first step into the development of a process-oriented writing tool and hence additional iterations of evaluation and prototyping are necessary to further specify the indicators, the design of the tool, and the integration of the tool into learning and teaching practices. For the indicators specifically, future work needs to determine which indicators can be automatically extracted (see also Implications for writing process research). In addition, the indicators do not necessarily improve writing proficiency and might not even have an impact on writing quality of a specific writing product. Although several studies have shown that indicators of the writing process have a relation with writing quality (e.g. Allen et al., Citation2016; Xu, Citation2018) and several writing tools have shown to improve motivation or writing quality (Cotos, Citation2015), the evidence is still limited and usually generalized over a whole tool, rather than for specific indicators. Therefore, future (empirical) studies are necessary to evaluate whether these indicators can positively impact writing and how these should be integrated into the learning design to positively impact writing.
Conclusion
This article, in contrast to user-centric evaluations of specific writing tools conducted after the development, presented a participatory approach that happened before the development. Through an illustrative study, we showed which indicators are considered desirable by students, teachers, writing researchers, and professional development staff to provide automated, personalized writing feedback in higher education. Bachelor students focused mostly on lower-level behavioral indicators and PhD students mostly on behavioral indicators in relation to time, while teachers, writing researchers and professional development staff focused more on higher-level cognitive indicators. These lower-level behavioral indicators can be extracted automatically using keystroke logging. However, it is important that writing process research goes beyond these lower-level features, focusing on temporal analysis of keystroke data and natural language processing of the text written so far, to gain a better understanding of the relationship between keystroke data and cognitive writing processes. This can inform the design and information necessary for the development of writing tools. Future work should further analyze how information on the writing process may be incorporated into writing tools and the learning design. We showed how stakeholder involvement in the form of a participatory approach can be valuable to further this goal.
Disclosure statement
No potential conflict of interest was reported by the authors.
Additional information
Funding
Notes on contributors
Rianne Conijn
Rianne Conijn is an assistant professor in the Human-Technology Interaction group at Eindhoven University of Technology, the Netherlands. Her research interests include the analysis and interpretation of (sequences of) online and offline learning behavior to improve learning and teaching.
Roberto Martinez-Maldonado
Roberto Martinez-Maldonado is a senior lecturer at Monash University, in Melbourne, Australia. He has a background in Computing Engineering. His areas of research include Human-Computer Interaction, Learning Analytics, Artificial Intelligence in Education, and Collaborative Learning (CSCL).
Simon Knight
Simon Knight is a lecturer at the University of Technology Sydney, Faculty of Transdisciplinary Innovation. He holds degrees in Philosophy and Psychology (BSc), Philosophy of Education (MA), Educational Research Methods (MPhil), and Learning Analytics (PhD). His research investigates the use of data and learning analytics by learners and educators, in particular the use of writing analytics tools.
Simon Buckingham Shum
Simon Buckingham Shum is Professor of Learning Informatics at the University of Technology Sydney, Australia, where he serves as Director of the Connected Intelligence Centre. He holds degrees in Psychology, Ergonomics and Human-Computer Interaction, and a career-long fascination with making thinking visible using software. He has focused in the last decade on helping to define Learning Analytics as a field.
Luuk Van Waes
Luuk Van Waes is a Professor in Professional Communication at the University of Antwerp, Belgium. He has been involved in different types of writing research, with a focus on digital media and (professional) writing processes.
Menno van Zaanen
Menno van Zaanen is a Professor in Digital Humanities at the North West University, South Africa. He has a background in computational linguistics. His areas of research include multi-modal structuring of data, multi-modal information retrieval, and applying digital techniques in the humanities.
References
- Alamargot, D., & Chanquoy, L. (2001). Through the models of writing (Vol. 9). Dordrecht: Springer. doi:10.1007/978-94-010-0804-4
- Allen, L. K., Jacovina, M. E., Dascalu, M., Roscoe, R. D., Kent, K., Likens, A. D., & McNamara, D. S. (2016). {ENTER}ing the Time Series {SPACE}: Uncovering the writing process through keystroke analyses. Paper presented at the 9th International Conference on Educational Data Mining (EDM) (pp. 22–29), Raleigh, NC. https://eric.ed.gov/?id=ED592674.
- Allen, L. K., Jacovina, M. E., & McNamara, D. S. (2015). Computer-based writing instruction. In C. A. MacArthur, S. Graham, & J. Fitzgerald (Eds.), Handbook of Writing Research (pp. 316–329). New York, NY: The Guilford Press Publisher. Retrieved from https://eric.ed.gov/?id=ED586512.
- Attali, Y., & Burstein, J. (2006). Automated essay scoring with e-rater® V. 2. The Journal of Technology, Learning and Assessment, 4(3), 1–21. doi:10.1002/j.2333-8504.2004.tb01972.x
- Baaijen, V. M., & Galbraith, D. (2018). Discovery through writing: Relationships with writing processes and text quality. Cognition and Instruction, 36(3), 199–125. doi:10.1080/07370008.2018.1456431.
- Baaijen, V. M., Galbraith, D., & de Glopper, K. (2012). Keystroke analysis: Reflections on procedures and measures. Written Communication, 29(3), 246–277. doi:10.1177/0741088312451108.
- Becker, A. (2006). A review of writing model research based on cognitive processes. In A. Horning & A. Becker (Eds.), Revision: History, theory, and practice (pp. 25–49). West Lafayette, IN: Parlor Press. Retrieved from https://wac.colostate.edu/books/horning_revision/chapter3.pdf
- Bereiter, C., & Scardamalia, M. (1987). The psychology of written composition. Hillsdale, NJ: Lawrence Erlbaum Associates, Inc.
- Bixler, R., & D’Mello, S. (2013). Detecting boredom and engagement during writing with keystroke analysis, task appraisals, and stable traits. Paper presented at the 2013 International Conference on Intelligent User Interfaces (pp. 225–234), Santa Monica, CA. doi:10.1145/2449396.2449426
- Braun, V., & Clarke, V. (2006). Using thematic analysis in psychology. Qualitative Research in Psychology, 3(2), 77–101. doi:10.1191/1478088706qp063oa.
- Buckingham Shum, S., Ferguson, R., & Martinez-Maldonado, R. (2019). Human-centred learning analytics. Journal of Learning Analytics, 6(2), 1–9. doi:10.18608/jla.2019.62.1.
- Buckingham Shum, S., Knight, S., McNamara, D., Allen, L., Bektik, D., & Crossley, S. (2016). Critical perspectives on writing analytics. Paper presented at the Sixth International Conference on Learning Analytics & Knowledge (pp. 481–483), New York. doi:10.1145/2883851.2883854
- Chenoweth, N. A., & Hayes, J. R. (2001). Fluency in writing: Generating text in L1 and L2. Written Communication, 18(1), 80–98. doi:10.1177/0741088301018001004.
- Chenoweth, N. A., & Hayes, J. R. (2003). The inner voice in writing. Written Communication, 20(1), 99–118. doi:10.1177/0741088303253572.
- Conde, M. Á., & Hernández-García, Á. (2015). Learning analytics for educational decision making. Computers in Human Behavior, 47, 1–3. doi:10.1016/j.chb.2014.12.034.
- Cotos, E. (2015). Automated Writing Analysis for writing pedagogy: From healthy tension to tangible prospects. Writing & Pedagogy, 7(2–3), 197–231. doi:10.1558/wap.v7i2-3.26381.
- Crossley, S. (2020). Linguistic features in writing quality and development: An overview. Journal of Writing Research, 11(3), 415–443. doi:10.17239/jowr-2020.11.03.01.
- Deane, P. (2014). Using writing process and product features to assess writing quality and explore how those features relate to other literacy tasks. ETS Research Report Series, 2014(1), 1–23. doi:10.1002/ets2.12002.
- Dikli, S. (2006). An overview of automated scoring of essays. The Journal of Technology, Learning and Assessment, 5(1). Retrieved from http://ejournals.bc.edu/ojs/index.php/jtla/article/view/1640
- Dollinger, M., Liu, D., Arthars, N., & Lodge, J. (2019). Working together in learning analytics towards the co-creation of value. Journal of Learning Analytics, 6(2), 10–26. doi:10.18608/jla.2019.62.2.
- El Ebyary, K., & Windeatt, S. (2010). The impact of computer-based feedback on students’ written work. International Journal of English Studies, 10(2), 121–142. doi:10.6018/ijes/2010/2/119231.
- Ferris, D. (2011). Responding to student errors: Issues and strategies. In D. Ferris (Ed.), Treatment of error in second language student writing (pp. 49–76). Ann Arbor, MI: University of Michigan Press.
- Flower, L., & Hayes, J. R. (1980). The cognition of discovery: Defining a rhetorical problem. College Composition and Communication, 31(1), 21–32. doi:10.2307/356630.
- Flower, L., & Hayes, J. R. (1981). A cognitive process theory of writing. College Composition and Communication, 32(4), 365–387. http://www.jstor.org/stable/356600.
- Gabriska, D., & Ölveckỳ, M. (2018). Issues of adaptive interfaces and their use in educational systems. Paper presented at the 16th International Conference on Emerging eLearning Technologies and Applications (ICETA) (pp. 173–178), Stary Smokovec, Slovakia. doi:10.1109/ICETA.2018.8572096
- Galbraith, D. (2009). Writing about what we know: Generating ideas in writing. In R. Beard, D. Myhill, J. Riley, & M. Nystrand (Eds.), The SAGE handbook of writing development (pp. 48–64). London, UK: Sage. doi:10.4135/9780857021069.n4
- Galbraith, D., & Baaijen, V. M. (2019). Aligning keystrokes with cognitive processes in writing. In Observing writing (pp. 306–325). Leiden, Netherlands: Brill.
- Giacomin, J. (2014). What is human centred design? The Design Journal, 17(4), 606–623. doi:10.2752/175630614X14056185480186
- Graham, S., McKeown, D., Kiuhara, S., & Harris, K. R. (2012). A meta-analysis of writing instruction for students in the elementary grades. Journal of Educational Psychology, 104(4), 879–896. doi:10.1037/a0029185
- Graham, S., & Perin, D. (2007). A meta-analysis of writing instruction for adolescent students. Journal of Educational Psychology, 99(3), 445–476. doi:10.1037/0022-0663.99.3.445
- Hayes, J. R. (1996). A new framework for understanding cognition and affect in writing. In C. M. Levy & S. Ransdell (Eds.), The science of writing: Theories, methods, individual differences, and applications (pp. 1–27). NJ: Erlbaum.
- Hayes, J. R. (2012). Modeling and remodeling writing. Written Communication, 29(3), 369–388. doi:10.1177/0741088312451260
- Hounsell, D. (1984). Essay planning and essay writing. Higher Education Research and Development, 3(1), 13–31. doi:10.1080/0729436840030102
- Hounsell, D. (1997). Contrasting conceptions of essay-writing. The Experience of Learning, 2, 106–125.
- Itua, I., Coffey, M., Merryweather, D., Norton, L., & Foxcroft, A. (2014). Exploring barriers and solutions to academic writing: Perspectives from students, higher education and further education tutors. Journal of Further and Higher Education, 38(3), 305–326. doi:10.1080/0309877X.2012.726966
- Kaufer, D. S., Hayes, J. R., & Flower, L. (1986). Composing written sentences. Research in the Teaching of English, 20(2), 121–140. Retrieved from http://www.jstor.org/stable/40171073
- Kellogg, R. T. (1996). A model of working memory in writing. In C. M. Levy & S. Ransdell (Eds.), The science of writing: Theories, methods, individual differences, and applications (pp. 57–71). NJ: Erlbaum.
- Kidd, P. S., & Parshall, M. B. (2000). Getting the focus and the group: Enhancing analytical rigor in focus group research. Qualitative Health Research, 10(3), 293–308. doi:10.1177/104973200129118453
- Knight, S., Martinez-Maldonado, R., Gibson, A., & Buckingham Shum, S. (2017). Towards mining sequences and dispersion of rhetorical moves in student written texts. Paper presented at the Seventh International Learning Analytics & Knowledge Conference (pp. 228–232), Vancouver, Canada. doi:10.1145/3027385.3027433
- Knight, S., Shibani, A., Abel, S., Gibson, A., & Ryan, P. (2020). AcaWriter: A learning analytics tool for formative feedback on academic writing. Journal of Writing Research, 12(1), 141–186. doi:10.17239/jowr-2020.12.01.06.
- Lea, M. R., & Street, B. V. (1998). Student writing in higher education: An academic literacies approach. Studies in Higher Education, 23(2), 157–172. doi:10.1080/03075079812331380364.
- Leijten, M., & Van Waes, L. (2013). Keystroke logging in writing research: Using Inputlog to analyze and visualize writing processes. Written Communication, 30(3), 358–392. doi:10.1177/0741088313491692.
- Leijten, M., Van Waes, L., Schriver, K., & Hayes, J. R. (2014). Writing in the workplace: Constructing documents using multiple digital sources. Journal of Writing Research, 5(3), 285–337. doi:10.17239/jowr-2014.05.03.3.
- Levy, M., & Moore, P. J. (2018). Qualitative research in CALL. Language Learning & Technology, 22(2), 1–7. https://www.lltjournal.org/item/10125-44638/.
- Likens, A. D., Allen, L. K., & McNamara, D. S. (2017). Keystroke dynamics predict essay quality. Paper presented at the 39th Annual Meeting of the Cognitive Science Society (CogSci 2017) (pp. 2573–2578), London.
- Limpo, T., Nunes, A., & Coelho, A. (2020). Introduction to the special issue on technology-based writing instruction: A collection of effective tools. Journal of Writing Research, 12(1), 1–7. doi:10.17239/jowr-2020.12.01.01.
- Lindgren, E., & Sullivan, K. P. (2019). Observing writing: Insights from keystroke logging and handwriting. Leiden, Netherlands: Brill. doi:10.1163/9789004392526
- Link, S., Dursun, A., Karakaya, K., & Hegelheimer, V. (2014). Towards best ESL practices for implementing automated writing evaluation. Calico Journal, 31(3), 323–344. https://www.jstor.org/stable/calicojournal.31.3.323.
- Lockyer, L., Heathcote, E., & Dawson, S. (2013). Informing pedagogical action: Aligning learning analytics with learning design. American Behavioral Scientist, 57(10), 1439–1459. doi:10.1177/0002764213479367.
- Ma, W., Adesope, O. O., Nesbit, J. C., & Liu, Q. (2014). Intelligent tutoring systems and learning outcomes: A meta-analysis. Journal of Educational Psychology, 106(4), 901–918. doi:10.1037/a0037123.
- Martinez-Maldonado, R., Pardo, A., Mirriahi, N., Yacef, K., Kay, J., & Clayphan, A. (2016). LATUX: An iterative workflow for designing, validating and deploying learning analytics visualisations. Journal of Learning Analytics, 2(3), 9–39. doi:10.18608/jla.2015.23.3.
- Medimorec, S., & Risko, E. F. (2017). Pauses in written composition: On the importance of where writers pause. Reading and Writing, 30(6), 1267–1285. doi:10.1007/s11145-017-9723-7.
- Norton, L. S. (1990). Essay-writing: What really counts? Higher Education, 20(4), 411–442. doi:10.1007/BF00136221.
- NVivo. (2015). NVivo: NVivo qualitative data analysis software (Version 11). [Computer software]. Doncaster, Australia: QSR International Pty Ltd.
- Prieto-Alvarez, C. G., Martinez-Maldonado, R., & Anderson, T. (2018). Co-designing learning analytics tools with learners. In J. M. Lodge, J. C. Horvath, & L. Corrin (Eds.), Learning analytics in the classroom: Translating learning analytics research for teachers (pp. 93–110). London: Routledge.
- Ranalli, J. (2018). Automated written corrective feedback: How well can students make use of it? Computer Assisted Language Learning, 31(7), 653–674. doi:10.1080/09588221.2018.1428994.
- Ranalli, J., Feng, H.-H., & Chukharev-Hudilainen, E. (2018). The affordances of process-tracing technologies for supporting L2 writing instruction. Language Learning & Technology, 23(2), 1–11. https://scholarspace.manoa.hawaii.edu/items/236c5a21-a813-4594-943c-b09cb0272292.
- Rapp, C., & Kauf, P. (2018). Scaling academic writing instruction: Evaluation of a scaffolding tool (Thesis Writer). International Journal of Artificial Intelligence in Education, 28(4), 590–615. doi:10.1007/s40593-017-0162-z.
- Rijlaarsdam, G., & Van den Bergh, H. (1996). The dynamics of composing – An agenda for research into an interactive compensatory model of writing: Many questions, some answers. In C. M. Levy & S. Ransdell (Eds.), The science of writing: Theories, methods, individual differences, and applications (pp. 107–125). NJ: Lawrence Erlbaum Associates, Inc.
- Roscoe, R. D., Allen, L. K., Weston, J. L., Crossley, S. A., & McNamara, D. S. (2014). The Writing Pal intelligent tutoring system: Usability testing and development. Computers and Composition, 34, 39–59. doi:10.1016/j.compcom.2014.09.002.
- Shibani, A., Knight, S., & Shum, S. B. (2019). Contextualizable learning analytics design: A generic model and writing analytics evaluations. Paper presented at the 9th International Conference on Learning Analytics & Knowledge (pp. 210–219), New York, NY. doi:10.1145/3303772.3303785.
- Solé, I., Miras, M., Castells, N., Espino, S., & Minguela, M. (2013). Integrating information: An analysis of the processes involved and the products generated in a written synthesis task. Written Communication, 30(1), 63–90. doi:10.1177/0741088312466532.
- Staples, S., Egbert, J., Biber, D., & Gray, B. (2016). Academic writing development at the university level: Phrasal and clausal complexity across level of study, discipline, and genre. Written Communication, 33(2), 149–183. doi:10.1177/0741088316631527.
- Steenbergen-Hu, S., & Cooper, H. (2014). A meta-analysis of the effectiveness of intelligent tutoring systems on college students’ academic learning. Journal of Educational Psychology, 106(2), 331–347. doi:10.1037/a0034752.
- Teasley, S. D. (2017). Student facing dashboards: One size fits all? Technology, Knowledge and Learning, 22(3), 377–384. doi:10.1007/s10758-017-9314-3.
- Tillema, M., van den Bergh, H., Rijlaarsdam, G., & Sanders, T. (2011). Relating self reports of writing behaviour and online task execution using a temporal model. Metacognition and Learning, 6(3), 229–253. doi:10.1007/s11409-011-9072-x.
- Van Lier, L. (2000). From input to affordance: Social-interactive learning from an ecological perspective. In J. Lantolf (Ed.), Sociocultural theory and second language learning (Vol. 78, pp. 245–259). Oxford: Oxford University Press.
- Van Waes, L., van Weijen, D., & Leijten, M. (2014). Learning to write in an online writing center: The effect of learning styles on the writing process. Computers & Education, 73, 60–71. doi:10.1016/j.compedu.2013.12.009.
- Vandermeulen, N., Leijten, M., & Van Waes, L. (2020). Reporting writing process feedback in the classroom: Using keystroke logging data to reflect on writing processes. Journal of Writing Research, 12(1), 109–140. doi:10.17239/jowr-2020.12.01.05.
- Wallot, S., & Grabowski, J. (2013). Typewriting dynamics: What distinguishes simple from complex writing tasks? Ecological Psychology, 25(3), 267–280. doi:10.1080/10407413.2013.810512.
- Wang, Y.-J., Shang, H.-F., & Briody, P. (2013). Exploring the impact of using automated writing evaluation in English as a foreign language university students’ writing. Computer Assisted Language Learning, 26(3), 234–257. doi:10.1080/09588221.2012.655300.
- Wengelin, Å. (2006). Examining pauses in writing: Theory, methods and empirical data. In K. P. H. Sullivan & E. Lindgren (Ed.), Computer key-stroke logging and writing: Methods and applications (Studies in Writing) (Vol. 18, pp. 107–130). Oxford: Elsevier.
- Wise, A. F., & Jung, Y. (2019). Teaching with analytics: Towards a situated model of instructional decision-making. Journal of Learning Analytics, 6(2), 53–69. doi:10.18608/jla.2019.62.4.
- Wolsey, T. D., Lapp, D., & Fisher, D. (2012). Students’ and teachers’ perceptions: An inquiry into academic writing. Journal of Adolescent & Adult Literacy, 55(8), 714–724. doi:10.1002/JAAL.00086.
- Woolner, P., Hall, E., Wall, K., & Dennison, D. (2007). Getting together to improve the school environment: User consultation, participatory design and student voice. Improving Schools, 10(3), 233–248. doi:10.1177/1365480207077846.
- Xu, C. (2018). Understanding online revisions in L2 writing: A computer keystroke-log perspective. System, 78, 104–114. doi:10.1016/j.system.2018.08.007.
- Zhang, F., & Litman, D. (2015). Annotation and classification of argumentative writing revisions. Paper presented at the Tenth Workshop on Innovative Use of NLP for Building Educational Applications (pp. 133–143), Denver, COL. doi:10.3115/v1/W15-0616
- Zhang, M., Hao, J., Li, C., & Deane, P. (2016). Classification of writing patterns using keystroke logs. In L. A. van der Ark, D. M. Bolt, W.-C. Wang, J. A. Douglas, & M. Wiberg (Eds.), Quantitative psychology research: The 80th annual meeting of the Psychometric Society, Beijing, 2015 (pp. 299–314). Beijing, China: Springer. doi:10.1007/978-3-319-38759-8_23.