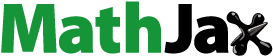
ABSTRACT
Over half of all residential buildings in the Netherlands are owner-occupied. In this study, the influence of behavioural factors on individual decisions toward energy efficiency renovations (EERs) was investigated. This study focused on contextual (e.g. building characteristics), personal (e.g. awareness of energy consumption), and motivational factors (e.g. improving comfort). Logistic regression analyses were selected as the preferred method of analysis. The Netherlands’s housing survey energy modules, which was conducted in 2018, was the basis of these analyses. 2878 homeowners were surveyed. Behavioural factors that influence the homeowners’ decisions were investigated for four types of EERs: (1) double glazing, (2) insulation, (3) photovoltaic (PV) panel, and (4) sustainable heating. It was found that homeowners’ preferences for double glazing were mainly influenced by the characteristics of the building and household and motivation to adopt EERs. Similarly, insulation and PV panels were to be mainly influenced by building characteristics. For sustainable heating, a combination of building and household characteristics and personal factors (e.g. deliberate gas reduction) influenced the decisions regarding this EER. None of the personal factors had a significant impact on the decisions regarding installation of double glazing; in contrast, the installation of PV panels was found to be highly influenced by these factors.
Introduction
In the Paris Climate Agreement (2015), nearly 200 countries agreed to reduce global warming to within 2°C of pre-industrial levels. Buildings contribute about 25% of direct and indirect global greenhouse gas (GHG) emissions. Building sector can substantially mitigate GHG emissions through large-scale energy-efficient renovations and using renewable energy sources (IEA, Citation2017; Sandberg et al., Citation2021). The Netherlands is set to reduce GHG emissions by 49% by 2030 and by 95% by 2050 relative to the 1990 baseline (Government of the Netherlands, Citation2019a). Furthermore, other targets such as producing 67% of total electricity from renewable sources by 2030, and fully climate-neutral electricity by 2050 are mentioned in this agreement. Recent studies have cast doubt on whether these targets will be achieved based on the current trends of GHG emissions (Brouwer, Citation2019; Netherlands Environmental Agency (PBL), Citation2019). To achieve these targets, the amount of GHG emissions that needs to be eliminated in the next 10 years is twice as much as has been eliminated in the last 30 years. These uncertainties are obvious for targets that specifically focus on the residential sector, such as making existing houses gas-free by 2050. The plan is to reach this target by making 30–50 thousand houses gas-free per year at the beginning, and to gradually increase this number to 200 thousand per year. However, such a fundamental change demands significant modifications at the infrastructural level, which are quite difficult to realize. Adapting energy efficiency renovations (EERs), such as highly insulated buildings, appears to be a more realistic measure for moving towards these targets (Government of the Netherlands, Citation2019b). The Dutch government is attempting to reach the target by providing subsidies and loans for different types of renovations while actively informing households about ways to save energy, relevant implementation approaches, and the availability of financial incentives (The Netherlands Enterprise Agency, Citation2019a, Citation2019b).
In the Netherlands, quite a limited number of new dwellings have been built since 2012. The total housing stock consists of 1% of houses newly added within the year (Netherlands Statistics, Citation2020). Accordingly, the renovation of existing dwellings seems to be the most viable solution for realizing the energy efficiency targets (Ritzen et al., Citation2016). Recent studies on this topic suggest that cost savings and increasing comfort are the main reasons for starting EERs, while households tend to pay less attention to the energy efficiency aspects of renovation. Therefore, EERs should be promoted together with other maintenance/renovations (Organ et al., Citation2013; Wilson et al., Citation2015, Citation2018). In addition to motivations, the rates for different EERs are determined by different contextual factors that influence, such as building and household characteristics, and personal factors, such as attitudes and perceptions about energy consumption. More importantly, recent research has identified cognitive biases of the category of personal factors as an important barrier to EERs (De Vries et al., Citation2019; Klöckner & Nayum, Citation2017; Streimikiene et al., Citation2020; Wilson et al., Citation2018). However, empirical research into the exact nature of the cognitive biases and the effectiveness of interventions to de-bias are lacking.
The determined behaviour-influencing factors were different for different sub-sectors (De Vries et al., Citation2019; Ebrahimigharehbaghi et al., Citation2020; Jansma et al., Citation2020). For instance, homeowners are most likely to perceive difficulty with finalizing decisions, purchasing energy efficiency measures, and finding subsidies. On the other hand, tenants are most likely to perceive difficulty with the installation process (De Vries et al., Citation2019). Despite the recent attention, our understanding of the impact of behaviour-influencing factors on EERs is limited. Oversimplifying occupant behaviour and neglecting behaviour-influencing factors in designing energy policies may lead to inadequate results (Hu et al., Citation2020). Yet, this information is vital for policymakers in designing and implementing policies that are effective in reducing the energy consumption of housing stock. As most houses in the Netherlands belong to the owner-occupied sector, further studies are essential to identify the behaviour-influencing factors and their impacts on EERs in this sub-sector. In the building sector in the Netherlands, energy transition policies are designed to allow converting entirely to renewable energy resources. These policies include: (1) near-zero energy indicators for new buildings; (2) large-scale energy renovations for a lower Energy Performance Certificate (EPC)-Levels (D and F); (3) subsidies for heat from renewable sources, more use of solar photovoltaic (PV); (4) raising awareness about renewable energy resources, and (5) switching to electricity for energy (van Leeuwen et al., Citation2017).
This study aims to evaluate the main behavioural factors that influence different types of EERs, and to examine whether current EER policies can be improved by considering the impacts of these behaviour-influencing factors. More specifically, the personal, contextual, and motivational factors that influence the decision-making process of EERs are studied in the Dutch owner-occupied residential sector. To date, most existing studies have limited their focus on a single technology, based on the literature review done in (Camarasa et al., Citation2019). In this study, four types of EERs are investigated, namely (1) double-glazed windows; (2) insulated roofs, walls, and floors; (3) solar PV panels; and (4) sustainable heating systems. These types of EERs comprise the highest percentages of total EERs that used the Netherlands housing survey energy module 2018. In addition to this, based on the literature review, the impacts of reducing energy consumption and improving comfort, and the environmental benefits were the most compared with other EERs (Al-Homoud, Citation2005; Salata et al., Citation2017; Tsagarakis et al., Citation2012; Vieira et al., Citation2017). shows the distribution of energy-efficient measures conducted by Dutch homeowners in 2018. Among these measures, double glazing has the highest percentages (20.2%), and sustainable heating is the second, with 15.2% of houses. Appendix A explains the advantages of installing energy efficiency measures for residents and dwellings.
Figure 1. The percentages of renovators that have used different energy-saving measures (37% of households use no-energy-saving measure).
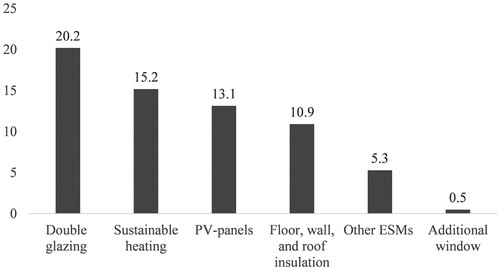
The developed theoretical framework is validated using logistic regression analyses and empirical data from the Netherlands housing survey energy module 2018 for renovators and potential renovators. The energy module 2018 was conducted on a representative sample of Dutch housing stock. This dataset contains valuable information on household and building characteristics. In addition to these variables, the energy and investment behaviour of households, such as the perception of energy consumption compared with other households, are also provided. Moreover, the information regarding whether the household performed any EERs in the past five years and whether the household is planning to implement an EER in the next two years is stored in this dataset. The main contributions of this research are as follow: (a) Empirical investigation of the cognitive biases and the impacts of the other personal factors, such as the perception of households on energy consumption compared with others, using logistic regression. (b) Identifying which behaviour-influencing factors influence the homeowners’ renovation decisions for double glazing, insulation, PV panels, and sustainable heating using logistic regression analyses.
Literature review on policy interventions and behaviour-influencing factors
Policy interventions for the owner-occupied sector
Steg and Vlek (Citation2009) categorized policy interventions that influence human behaviour into structural and informational interventions. Structural interventions modify the conditions in which households make decisions, such as financial incentives (e.g. subsidies, tax) and provide access to energy-efficient technologies. Informational interventions influence people’s motivations, such as providing information with respect to energy efficiency technologies and social norms on energy savings, as well as feedback on these topics (Abrahamse & Schuitema, Citation2020; Sanguinetti et al., Citation2018). Information provision is most commonly used to motivate households to reduce their energy consumption. This category of interventions can be classified into antecedent and consequent interventions. The latter, e.g. labelling, mainly influences the determinants of behaviour, e.g. knowledge and motivation. The former aims to provide the information after the behaviour has been carried out, e.g. feedback provision (Hu et al., Citation2020).
There are many examples of structural interventions in the approach taken by the Dutch government. Currently, 95% of houses use natural gas for heating, hot water, and cooking in the Netherlands. Despite this considerable share, the Dutch government has assigned specific budgets to eliminate natural gas as a source of energy by 2050.
Consequently, €435 million has been allocated to natural gas-free neighbourhoodsFootnote1 between 2018 and 2028 (Government of the Netherlands, Citation2019b). shows the Dutch policy interventions in motivating homeowners to make EERs (Joint Research Centre, Citation2019; Duurzaam Bouwloket, Citation2019; Netherlands Enterprise Agency (RVO), Citation2019; The Netherlands Enterprise Agency, Citation2019a, Citation2019b; Milieu Centraal Information Organization, Citation2019; Independent Association of Homeowner Associations, Citation2019). Among these measures, insulation and PV panels are the focus of attention by local authorities. For ISDE subsidies, the amount requested by the entities (homeowners and companies) were approximately twice as much as the planned budget in 2019.
Table 1. Main policy interventions in the Netherlands.
Subsidies, loans, and taxes are examples of supply-side policies in the market. Other countries provide similar support, such as low interest rates, third-party financing, payment on energy bills, energy efficiency mortgages, and crowdfunding (Bergman & Foxon, Citation2020; Bertoldi et al., Citation2020; Kerr & Winskel, Citation2020; Wilson et al., Citation2015). Flanders, France, Italy, the United Kingdom, and Poland offer energy efficiency obligation programs, in which energy suppliers must provide evidence of contributing energy savings by promoting energy efficiency activities or financial support for residents (Bertoldi et al., Citation2020; Kerr & Winskel, Citation2020). The Green Deal in the United Kingdom was an example of financing by a third party and paying back on energy bills. However, this program was not successful in upscaling EERs (with the goal of one million houses). The reasons were that there was no guarantee of energy savings, the process was complex and bureaucratic, the interest rates were above the mortgage rates, and financial savings were the only objective, rather than households’ comfort and well-being (Bergman & Foxon, Citation2020).
Complementary to these policies, the national environment centre (In Dutch: Milieu Centraal) influences householders’ motivations through informational interventions. This centre provides information on all the possibilities for an energy-efficient and sustainable house, the availability of subsidies and loans, the steps to becoming natural gas-free, and finding a professional/company, etc. Accessing information does not solely result in a change in behaviour because people often make choices based on mental shortcuts and habits (Lehner et al., Citation2016).
To enhance the effectiveness of information provision, the role of social norms has been investigated by several researchers. For instance, from five groups of households having different information interventions, namely (1) save money by conserving energy; (2) protecting the environment by conserving energy; (3) conserving energy for future generations; (4) joining neighbours in conserving energy; and (5) saving energy by using fans instead of air conditioning, group (4) achieved the highest electricity savings. The reason for that is the inclusion of social norms in information provision (Taranu & Verbeeck, Citation2016).
Informational feedback on energy consumption is considered a low-cost strategy for saving energy. This type of intervention has gained increasing attention due to the advancement of information technologies and energy infrastructures. The effectiveness of this behavioural change has been examined in many countries within and outside Europe (Aydin et al., Citation2018; Faruqui et al., Citation2010; Gans et al., Citation2013; Lee et al., Citation2020; Lynham et al., Citation2016; Matsukawa, Citation2004; Shen et al., Citation2020). According to previous studies, the provision of information through in-home displays (IHDs), WeChat, and smart meters diminished electricity consumption by approximately 20%, 16%, and 11–17% compared with houses without this information in the Netherlands, China, and Northern Ireland, respectively (Aydin et al., Citation2018; Gans et al., Citation2013; Shen et al., Citation2020).
Behavioural factors that influence homeowner renovation decisions
Behaviour depends on individuals and their environment. The factors that influence human behaviour can have multiple origins and can be categorized as: motivations (e.g. thermal comfort); barriers (e.g. information); contextual factors; personal factors. Wilson et al. (Citation2015) reviewed behavioural studies on energy efficiency to identify the factors that influenced on homeowner renovation decisions. An example of personal factors is the way individuals evaluate information. Individuals usually evaluate information based on their own perceptions to make decisions (Gigerenzer & Selten, Citation2002). For instance, the energy-efficient measures with the greatest perceived advantages were selected (Nair et al., Citation2010a, Citation2010b). Factors such as awareness of energy consumption and the perception of households of electricity/gas consumption can influence energy efficiency decisions in this way. The combined outcomes of personal and contextual factors create the behaviour (Stren, Citation2000). The main categories of personal, contextual, and motivational factors are presented in .
Steemers and Yun (Citation2009) investigated the direct and indirect effects of different factors on household energy consumption for the residential sector of the United States. The main conclusions were: (1) physical characteristics, e.g. climate and heating system type, are important factors for heating; (2) income has an indirect effect on energy usage for space heating and cooling; (3) the number of heated/cooled rooms, and the frequency of air-conditioning use are the main variables that influence energy consumption for space heating and cooling. Brounen et al. (Citation2013) examined the impact of household and socio-demographic characteristics, awareness, and literacy. The authors measured the energy awareness by defining the question of ‘How much do you pay for their monthly gas/electricity consumption?’. Their conclusions included: (1) age is identified as the main demographic factor that influences energy consumption. Older households with higher income choose higher comfort levels by changing the thermostat settings; (2) No significant relation is identified between consumer behaviour and energy literacy/awareness. The current study used a similar question to measure energy awareness. In a study focused on the Chinese residential sector, the probability of retrofitting of dwellings was higher for households who were older (Yang et al., Citation2017). In another study, Schley and DeKay (Citation2015) investigated the cognitive accessibility of 730 households in 4 similar case studies in the United States. All four studies provided evidence of cognitive accessibility for people’s inaccurate estimates of energy consumption. Regarding EERs, few studies have been conducted on evaluating the impacts of contextual and personal factors. The impact on energy efficiency measures of building and household characteristics, and satisfaction with the existing building envelope were investigated by Nair et al. (Citation2010b).
Huebner et al. (Citation2015) examined the impact of different factors, including building characteristics, socio-demographics, attitudes and, self-reported behaviours on energy consumption in the residential sector in the United Kingdom. They concluded that building characteristics explained the major share of variance in energy consumption. Socio-demographics and attitudes had a lower impact on energy consumption. Vassileva et al. (Citation2012) concluded that household characteristics, type and usage of electrical appliances, and attitudes towards electricity consumption had significant impacts on electricity consumption. In another study by Huebner et al. (Citation2016), appliance types and sizes, and household size were the most significant variables in electricity consumption. The impact of building and household characteristics was evaluated for the residential sector in the Netherlands and Denmark (Van den Brom et al., Citation2019). Household and building characteristics each explained approximately 50% of the variance in heating consumption. In the category of property characteristics, the benefits of using different EERs (e.g. insulation, double glazing, PV panels) and the challenges in implementing these technologies were also identified as important factors. For example, double glazing, insulation, and smart heating systems can improve the indoor climate and building comfort (Jensen et al., Citation2018). Risholt and Berker (Citation2013) studied the owner-occupied sector in Norway. They found a higher probability of EER among energy-conscious households and/or ones with relevant professions and knowledge. In Germany, collaboration and the transfer of knowledge by households were found to be an effective approach for motivating them in conducting EERs (Stieß & Dunkelberg, Citation2013). Pothitou et al. (Citation2016) found significant and positive relations between environmental values and knowledge on energy-saving behaviours, attitudes, and habits in a survey of 249 households in England.
Regarding policy incentives in the category of contextual factors, marketing campaigns and subsidies were found to be influential for Nordic countries. Also, the trustworthiness of one-stop-shops was identified as the main limitation in some cases (Mahapatra & Gustavsson, Citation2009; Mahapatra et al., Citation2013). The impact of feedback, i.e. display (an energy monitoring device), on energy consumption was studied for the Swedish private rental sector by (Nilsson et al., Citation2014). They found no significant impact of displays on energy consumption, the reasons being for this being the difficulty in understanding how to work with displays and the resistance to changing behaviour. The motives in using displays were also identified, such as curiosity and interest, cost savings, and environmental concerns.
Understanding cognitive biases involved in home renovation decisions and designing interventions to overcome them (i.e. de-biasing tools) can help increase the home renovation rate. De Vries et al. (Citation2019) introduced perceived hassle factors as an important barrier in conducting EERs. People generally postpone taking these energy efficiency measures to avoid the stress anticipated due to the accumulation of hassles during the awareness, consideration, and decision stages. Wilson et al. (Citation2018) investigated the importance of attitudes and social norms to renovation and EERs. These influencing factors were identified as significant for different stages of thinking about renovating; planning renovations; and finalizing renovations. Klöckner and Nayum (Citation2017) examined the determinants of EERs for private owners in Norway by exploring the relationship between attitudes and energy efficiency investment decisions. The authors determined the importance of feelings of moral obligation to act, attitudes, and self-efficacy as determinants of the intention to consider EERs. It is important to understand the households’ perceptions in energy consumption relative to other households. This can indicate whether they take action to improve the energy performance of their dwelling (Benoît et al., Citation2009; Larrick et al., Citation2007). Our study includes these types of factors in the regression analyses to evaluate the effects on renovation decisions.
Motivational factors shape the intention to behave, and finally, the actual behaviour (Ajzen, Citation1991; Hardeman et al., Citation2002; Hargreaves, Citation2011). Households’ motivations need to be identified to upscale EERs in the owner-occupied sector (Nair et al., Citation2010a; Organ et al., Citation2013; Wilson et al., Citation2015). Cost savings on energy bills, increased comfort, and carbon footprints are examples of the identified motivational factors for EERs. Various categorizations of motivational factors are provided in the literature: economic, social, and environmental motivations were studied by Organ et al. (Citation2013); commonly identified motivations (e.g. thermal comfort) and occasionally identified motivations (e.g. property value) were examined by Wilson et al. (Citation2015); economical motivations (e.g. paybacks) and non-economical motivations (e.g. increasing thermal comfort) were studied by Friege and Chappin (Citation2014). In a European research project, the motivational factors related to EERs in the building sector were investigated for Cyprus, Denmark, and Sweden. In terms of economic motivation, Danish households were motivated mainly by paybacks, whereas Swedish and Cypriots households were motivated by cost savings. Baumhof et al. (Citation2018) examined the factors that influenced German owner-occupiers of single and multifamily houses. In a case study from Tanzania, the initial decisions for adopting solar PV energy were influenced by the motivational factors of technology, cost, warranty, and service for low-income and young households (Simpson et al., Citation2021). Additional motivational factors were the appearance of houses, lower dependency on fossil fuels, and the improved usability of existing space.
In addition to the motivational factors, personal factors such as the attitudes, values, and beliefs of households were identified as influencing household motivations, e.g. as environmentally friendly or not. Social norms and social influence could be considered as both internal, i.e. the household perception of social norms, and external factors, i.e. acceptability by society, influence on household motivations. Haque et al. (Citation2021) investigated the role of socio-cultural attitudes and practices in the acceptance of energy technologies by low-income households in Mumbai and Cape Town. For instance, households’ attitude for accepting solar energy was to make apparent their energy lifestyles to their communities. In a case study of Canada, the household characteristics and motivational factors of renovators were investigated. The energy cost savings, financial incentives, and costs of EERs were identified as significant factors using econometric analysis and given several building and household characteristics (Gamtessa, Citation2013). presents the main influencing factors that will be evaluated in this study.
Table 2. Personal, contextual, and motivational factors that are going to be tested in this study.
Methodology
The Netherlands housing survey energy module 2018
In this study, the Netherlands housing survey energy module 2018,Footnote2 which is the most recent one, is used. This database comprises 4506 dwellings of which 63% (2878) belong to the owner-occupied sector. Data about the personal and motivational factors are mainly from the survey in 2018. The housing and building characteristics were collected from sources other than the survey. In addition to this, the main purpose of the national survey was to provide a representative sample of Dutch society. From this dataset, the following data are used: (1) renovators and potential renovators per type of EER, i.e. double glazing, insulation, solar PV panel, and, sustainable heating; (2) contextual factors, such as household and building characteristics (part of the extracted data, not the survey); (3) personal factors, such as the perceptions of the household of their own behaviours are assessed in different ways, for instance, whether they deliberately changed their behaviour, or how they perceive themselves compared with other households in terms of energy consumption; and (4) motivations for EERs. In the following, the descriptive analyses of the main variables in the logistic regression analyses are presented.
Building characteristics and household profiles
The percentages of single and multifamily households are around 83% and 17%, respectively. In addition, row houses have the highest percentages, and detached houses have slightly lower percentages than row houses. In 2018, 28% and 23% of houses were row houses and detached houses, respectively. In terms of the age of the buildings, the category for the oldest buildings (including buildings constructed before 1945) contained the highest number of houses (around 22%) in the dataset. Other age categories contained buildings which were built within a period of 10 years ((a,b)).
Figure 3. Building characteristics of ‘The Netherlands housing survey energy module 2018’. Seventeen per cent of values are missing for the building types.
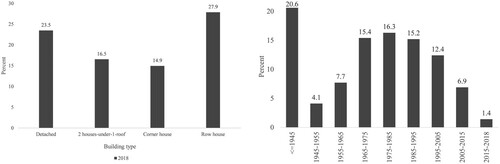
The energy labelsFootnote3 of 2018 are presented in . (1) Labels B and C accounted for the largest percentages of buildings with energy labels; (2) The proportion of buildings having energy labels (G, F, E, and D) was lower than the proportion of houses having energy labels (C, B, A, and A+).
Figure 4. Distribution of buildings with different energy labels in ‘The Netherlands housing survey energy module dataset of 2018’.
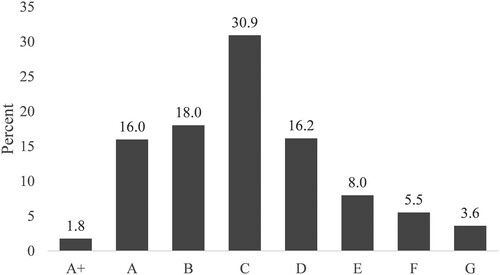
A lower percentage of renovators performed the work by themselves in 2018, namely, 16% compared with 22% in 2012. presents the household profiles, for example, more than 50% of homeowners were 54 years old or older, and more than 50% of households had higher education.
Table 3. Households’ profile (the Netherlands housing survey energy module 2018).
presents the personal factors that were provided in the energy module 2018. For example, the majority of households, approximately 59% and 79%, indicated that they deliberately reduced their gas and electricity consumption, respectively. Furthermore, most respondents (45%) indicated that they were well aware of their energy consumption. Thirty-eight per cent of respondents perceived that they were consuming less energy compared with other households.
Table 4. Personal factors (the Netherlands housing survey energy module 2018).
In the energy module 2018, questions were asked regarding the motivations towards EER for renovators and potential renovators (with yes/no answers). ‘Due to maintenance, to save energy costs, and to improve comfort’ showed the highest percentages among the motivation factors ().
Table 5. Motivation factors (the Netherlands housing survey energy module 2018).
Method of analysis
The impacts of behaviour-influencing factors were investigated for different energy efficiency measures. The dependent variable was whether households had implemented/planned to implement the specific energy-saving measures in the last/next two years. The independent variables were contextual, personal, and motivational factors (). The dependent variables were binary (whether they had installed or will install the energy efficiency measures), therefore, logistic regressions were conducted. This study focused on four types of EERs: double glazing, insulation, solar PV panels, and sustainable heating. These energy-saving measures were investigated for both renovators and potential renovators, and in total, eight regressions were estimated. shows a list of independent variables having different scales.
Table 6. Explanatory variables with different scales.
In the computation, a backward elimination method was used. In this method, a complex model including all the potential variables is developed using a theoretical framework. At each step, the non-significant variables are removed from the regression. The elimination is based on the likelihood ratios, i.e. removal testing is done based on the likelihood-ratio statistic using the maximum partial likelihood estimates. A typical logistic regression output contains the following outputs in addition to the beta coefficients of independent variables (β), and degrees of freedom (df) in Statistical Package for the Social Sciences (SPSS). (1) The odds ratios (column exp(B)), describes the degree of association between the dependent and independent variables, and this measure is used to compare the relative probabilities of the occurrence (chance criterion) of the renovation. For the categorical variables, generally, the chance criterion is compared with the reference category. Binary variables are considered categorical variables with only two categories. The probability of respondents selecting category j can be calculated using the chance criterion . (2) A Wald test demonstrates the significance of each coefficient in the regression. The null hypothesis is that the coefficient of the independent variable is equal to zero. The hypothesis is rejected when the p-value (specified in the column called ‘Sig.’) is lower than the critical p-value of 0.05 (or 0.01, 0.1, etc.). (3) S.E. is the standard error around the coefficient for each variable.
There are some assumptions made in conducting logistic regressions: (a) dependent variable is the log of the binary variables; (b) The independent variables should not indicate multicollinearity; (c) the data should contain a large sample size. The validity of the multicollinearity assumption is verified by calculating the Variance Inflation Factors (VIF). A VIF = 2.5 is the initial point of concern, and a VIF > 10 shows multicollinearity (Midi et al., Citation2010). The VIFs for eight regressions are presented in . There is no serious multicollinearity between the independent variables in the sample.
Table 7. Multicollinearity tests in regressions.
Binary logistic regression model is used to describe the relation between the dependent and independent variables:
(1)
(1) where P is the probability of events, and X represents independent variables. After estimation, the omnibus tests of the model coefficients and the Hosmer and Lemeshow test were applied to validate the models (). The omnibus test checks whether the model estimates the outcome with the explanatory variables better than without (Brant, Citation1990). The omnibus tests were statistically significant, and the models were better with explanatory variables than without. The Hosmer and Lemeshow test illustrated the goodness of fit, which is an insignificant factor for a good model.
Table 8. Assessing the regressions regarding the goodness of fit.
presents the pseudo R-squared values and the likelihood-ratio tests. The pseudo R-squared values are comparable to the R-squared values in terms of scale, i.e. ranging from 0 to 1, and interpretation (i.e. higher values indicate better model fit). The likelihood-ratio test examines whether the differences between two models (for the backward elimination method) are statistically significant (Lewis et al., Citation2011). A p-value <0.05 indicates that the final model fits significantly better than the last estimated model.
Table 9. Pseudo R-squared and Likelihood-ratio test regarding the goodness of fit.
For the visualization of the results, the R programming language and the visreg package were used because the R package contained more options (Breheny & Burchett, Citation2017). Using this package, the surface plots were depicted for two independent variables of the logistic regressions, and the probability of investment in specific types of energy efficiency measures (Leeper, Citation2017).
Results
Renovators
Double glazing
shows the logistic regression for influencing factors on the implementation of double glazing, insulation, PV panels, and sustainable heating by households.Footnote4 Two categories of contextual factors significantly influence the installation of double glazing: building and household characteristics. The identified influencing building characteristics are year of construction and types of non-energy-efficient renovations. Houses that were constructed in the 1980s have the highest probability of installing double glazing. The relative probabilities of installing double glazing together with different non-EERs are investigated. These could be used to focus on the promotion of double glazing with the appropriate non-EERs. Overall, 70% of respondents mentioned that they conducted double glazing with ‘repaired/replaced the window frames’. The main identified household characteristics are income and household compositions. With respect to households’ characteristics, households with children are twice as likely to install double glazing compared with one-person households. The probability of installing double glazing is higher in lower-income groups and families with children when compared with other categories of incomes and household compositions (). No personal factor is significantly identified regarding the decision to install double glazing. Households mainly install the double glazing to improve comfort, to reduce noise, to sell the house at a higher price, and to maintain the house. These reasons were described as primary motivations by 74%, 67%, 61%, and 60% of households.
Table 10. Logistic regression analyses for renovators.
Insulation of floors, roofs and walls
Among contextual factors, the building characteristic, more specifically the construction year, is identified as significant. The houses built between 1945 and 1959 and those built between 1960 and 1969 are significantly identified, and they have a higher probability of insulation installation compared with houses built before 1945. Among the personal factors, the main identified one is ‘deliberately changing behaviour to use less electricity’. Households that changed their electricity consumption are more likely to insulate their houses. About 62% of these households insulated their houses. Another significant personal factor is awareness of energy consumption. The specified question is whether the households know how much gas/electricity they use per year. In this case, the data shows a reverse relationship between the installation of insulation and the claimed awareness of the households with respect to energy consumption. Contrary to what one would expect, the well-aware group has the lowest probability of installing insulation, and the not-fully-aware group has the highest probability. The more reasonable outcome would have been a direct relationship between awareness and performing insulation ((a)). Human bias may play a major role here and requires more in-depth investigation. The last category of behaviour-influencing factors is motivational factors. The main identified motivations are ‘to improve comfort’ and ‘to improve ventilation/moisture problem’. The percentages of households that specified these motivations and insulated their houses are 77.4% and 60%, respectively. (b) shows the highest probability of decision to insulate due to comfort and for buildings constructed before the 1980s.
Solar PV panels
The identified contextual factors belong to the energy labels, household characteristics, agent performing the EERs, and types of maintenance associated with EERs. The implementation of this energy efficiency measure depends strongly on the energy labels. A clear trend can be seen between the installation of PV panels and the energy label of the houses. The houses with higher energy labels are more likely to install PV panels compared to the ones with the worse energy labels. As an example, the households with energy label ‘A’ are 5.6 times more likely to install the PV panels compared to those with energy label ‘B’. The detached houses are more likely to install PV panels compared to 2-under-1-roof and maisonette dwellings. The chances are 2.4 and 10 times, respectively. As expected, 70% of households asked an expert to install the PV panels instead of installing the panels themselves. The installation of PV panels can take place whenever the households ‘replaced/repaired the roof’ or ‘replaced/repaired the windows frames’. Among household characteristics, the coefficients of age and household composition are statistically significant. A clear trend can be identified between the installation of PV panels and the Head of Household’s (HOH) age. Older HOHs are more likely to install PV panels. For instance, HOHs between the ages of 55 and 64 are almost 5 times more likely to install PV panels compared with those between the ages of 17 and 35 years of age. Similar to double glazing, households with children are more likely to install PV panels compared with one-person households or households without children. The chances are 6.2 and 2.2 times, respectively. Furthermore, a dwelling with the energy label ‘A’ has a higher chance of solar PV panel installation. (a) demonstrates the probability of installing solar panels per household composition and the HOH’s age group. There is a greater number of significant personal factors when comparing solar PV panel installation with double glazing and insulation. The first group of personal factors is behavioural changes by households: (1) deliberately replacing the non-energy efficiency devices with efficient ones, and (2) deliberately reducing gas consumption. Overall, the 34% and 38% of households that adopted these behavioural changes installed PV panels for their houses. Second, the household perception of electricity consumption compared to the others is significantly identified, as well. The households that perceived themselves as using more energy than others were 2.6 times more likely to install PV panels compared with households with a perception of similar energy consumption. The last category of behaviour-influencing factors is motivational factors. The most significant motivations are ‘saving energy costs’ and ‘for the environment’. Of those who installed PV panels, 87% and 74% of respondents mentioned these as primary motivations. The other significant motivations are ‘due to maintenance’ and ‘to improve comfort’, mentioned by 26% and 15% of respondents. (b) shows the importance of cost saving on energy bills for different groups of households.
Sustainable heating
Significantly identified contextual factors among building characteristics include the construction year, energy label, and building type. The construction year plays an important role in houses constructed between 1990 and 1999 and 2000 and 2009. Regarding boiler replacement, houses constructed between 1990 and 1999 are 4 times more likely to replace the boiler, and houses constructed between 2000 and 2009 are 4.4 times more likely to replace the boiler than all houses constructed in years outside of these years. The second significant building characteristic is the energy label. In contrast to the PV panel, the houses with lower energy labels have a higher probability of installing or replacing the boilers. The highest significant energy label is for energy label ‘F’, followed by energy labels ‘G’, ‘E’, and ‘D’. The building type is also a significant variable. Among different types of houses, all building types have a significant probability of installing boilers, though with a lower probability than apartments and semi-detached houses. Among the personal factors, deliberately changing gas consumption behaviour is a significant variable. Of the households that changed their behaviour, 69% have installed or replaced sustainable heating. The second significant personal factor is the household’s perception of their energy consumption compared to the other households. The households that perceive higher energy consumption as compared with other households are 2.1 times more likely to replace their boiler compared the ones who perceive lower energy usage. The main identified and highly significant motivating factor is ‘due to maintenance’. Of households that stated this motivation as an important one, 91% have conducted EERs.
Potential renovators
Double glazing
Among the contextual factors, two categories of building and household characteristics are identified significantly (). First, the energy labels significantly influence the decision to double-glaze for potential renovators. The highly significant energy label is ‘F’. The households living in this category of dwellings will be 7.2 times more likely to plan for double glazing installation compared with energy labels A and A+. After ‘F’, the energy labels ‘G’ and ‘D’ have the highest probability of installing double glazing in the future. Second, the households that earn more than twice the most frequent income in the sample are more likely to plan for double glazing. Among eight household composition types, the one-person households with a head of household older than 64 are 3.6 times more likely to double-glaze compared with reference category.Footnote5 (a) indicates that the higher-income groups and the buildings with lower energy labels are planning for double glazing to be completed as well. Personal factors such as deliberately using less gas and electricity as well as awareness of energy consumption are significantly identified. Of the households that have deliberately changed their behaviours by reducing gas consumption, 60% are planning to implement double glazing. (b) indicates the evidence for these personal factors and all levels of income. In contrast, only 35% of households with deliberate changes in electricity consumption are planning to conduct double glazing. The households less aware of energy consumption are planning more for double glazing compared to those partly aware and well-aware households on energy consumption. The main motivating factors are ‘to improve comfort’, ‘to reduce noise’, ‘due to maintenance’, and ‘to improve ventilation or moisture problems’. Overall, 80%, 70%, 65%, and 59% of households that mentioned the importance of these motivations are planning to install double glazing in the next two years, respectively. A lower percentage of households (35%) is significantly identified as conducting double glazing to reduce environmental impact. (c) shows the importance of comfort per energy label of dwellings.
Figure 8. Impacts of the influencing factors regarding the future decision on installing/replacing the double glazing (potential renovators).
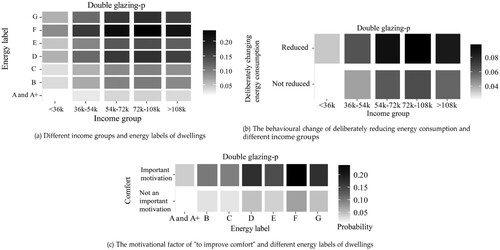
Table 11. Logistic regression analyses for the renovators for potential renovators.
Roof, wall, and floor insulation
Among the contextual factors, the construction year, building type, and ages of the occupants are significantly identified. Construction year is a highly significant factor, especially for the buildings that are constructed between 1945 and 1959. Approximately 70% of the building owners are likely to insulate their houses in the next two years. In addition to this, there is a trend for owners of newer dwellings to be less likely to plan for insulation compared to owners of older dwellings [Exp(B) is decreasing: 2.6, 1.16, 0.51, 0.23, 0.03]. Owners of row houses are 1.9 times more likely to plan for insulating their houses compared to those in apartment houses. (a) shows the impact of construction year and building type on the likelihood of insulation installation. No personal factor is significantly identified. Of those wanting to improve the comfort of the dwelling, 82% are more likely to plan to insulate their houses compared to the others. (b) confirms the importance of comfort per construction period. The older the building, the more likely households were to mention this highly significant motivational factor. Of households that mentioned the importance of maintaining the house, 33% are likely to plan for insulation.
Solar PV panels
Among contextual factors, construction years, household compositions, and level of education significantly influence the planning for installation of PV panels. A clear trend can be observed for construction year. There is a higher probability of planning for PV panel installation for newer buildings. In this respect, buildings built between 2010 and 2018 are 5.14 times more likely to have PV panels than buildings built before 1945. Non-family households, which comprise a group of people, are 6.8 times more likely to install PV panels compared with one-person households. Among personal factors, 40% of households that mentioned deliberately reducing gas consumption plan to install PV panels in the future. Overall, the main identified motivational factors are saving energy costs, increasing the house value, and caring for the environment. Of the households that are planning to install PV panels, 76%, 65%, and 63% described these motivations as the important ones, respectively. shows the importance of energy bills per construction period and may indicate that households with newer buildings and the motivation of cost savings are more likely to install PV panels.
Sustainable heating
The main identified contextual factors are the construction period, age, and income. The majority of construction periods have highly significant coefficients. Similar to the year of construction for renovators, the probability of planning for boiler replacement is highest for the construction period of 2000–2009 due to a boiler’s expected lifespan of 10–13 years. Younger and lower-income household groups are more likely to be planning to replace boilers than older and higher-income groups.
Among personal factors, the awareness of energy consumption is significantly identified. The well-aware households are 1.8 times more likely to be planning to replace a sustainable heating system compared to not-fully-aware households. (a) shows the effects of age and awareness of energy consumption. Among motivating factors, the most significant one is ‘due to maintenance’. Overall, 93% of households that mentioned this motivation are planning to install or replace a boiler in the next two years. The second important motivation is ‘for the environment’. Of households mentioning this motivation, 58% are planning to install a more energy-efficient boiler. Other significant motivational factors are ‘to improve comfort’ and ‘to improve ventilation and moisture problem’. (b) indicates the importance of the motivational factor ‘due to maintenance’ per income group of households. The lowest income groups mentioning installing or replacing a heating system for the reason of maintaining the dwellings are more likely to be planning this type of energy efficiency measure compared with the other two groups.
Discussion
Evidence on behaviour-influencing factors in practice
The influencing factors and the associated empirical results are illustrated using the Netherlands housing survey energy module 2018. presents the main important contextual, personal, and motivational factors per type of EER. In the current study, contextual factors such as household and building characteristics are investigated. For renovators, the installation of double glazing mostly depends on the building and household characteristics. For older dwellings with more occupants, e.g. families with children, the owners are more likely to install double glazing. Houses that were constructed prior to 1980 have the highest probability for double glazing installation. The use of double glazing increased extensively in the 1980s. Therefore, it is probable that houses built after this time period had double glazing installed at the time of construction, while houses built before that require this renovation. For houses that are built before 1945, owners are approximately 3.5 times more likely to install insulation than houses built between 2000 and 2009. The first regulation for the Energy Performance Coefficient (EPC) for buildings was introduced around the 1990s. Dwellings constructed in the years after the introduction of EPC were forced to comply with the regulations of installing insulation; therefore, these houses did not install insulation in recent years. Because there was no such regulation for houses built between 1945 and 1969, these houses installed more insulation in recent years.
Table 12. Contextual, personal, and motivational factors per type of EERs.
Houses with higher energy labels, especially energy label ‘A’, have installed more solar PV panels. Older HOHs and families without children were more likely to install solar PV panels compared with other groups. Buildings constructed from 1990–1999 and 2000–2009 have the highest probability of installing a new boiler. A plausible explanation is that the average lifetime of a boiler is between 10 and 13 years. Therefore, houses constructed between 2000 and 2008 should have changed their boilers in the time period specified in the questionnaire, 2013–2018. Buildings with the lowest energy labels and building types of row middle houses are more likely to install or replace a boiler. For potential renovators, the households with a lower energy label, especially energy label ‘F’, are more likely to be planning to install double glazing. Households with higher incomes are also more likely to plan to install double glazing compared with other income groups. This result indicates the high investment costs of double glazing, which makes people with lower incomes less likely to invest in this type of EER. It is more probable that non-family households and those with new houses plan for solar PV panel installations compared with others.
In terms of personal factors, household awareness of energy consumption is significantly identified for the installation of insulation and sustainable heating. Homeowners who aim ‘to deliberately change their behaviours towards energy consumption’ are more likely to insulate and replace the boilers compared with others. In the context of EERs, motivational factors influence the individuals’ behaviours as mentioned by Organ et al. (Citation2013), Wilson et al. (Citation2015), Friege and Chappin (Citation2014), and Baumhof et al. (Citation2018). In our study, both renovators and potential renovators mentioned ‘improving comfort and maintaining good physical and structural conditions of the houses’ as the main motivations for all types of EERs except for PV panels. Installation of PV panels is also motivated by ‘saving costs on energy bills’, ‘the environment’ (also for sustainable heating), and ‘increasing the house value’. Double glazing has an additional motivational factor of ‘reducing noises’.
Policy recommendations
Promoting EERs should be tailor-made for different cultures and target groups of households (e.g. socio-demographic traits). Dutch municipalities can set clusters of dwellings using household and building characteristics. These two categories of influencing factors are significantly identified for all types of EERs, as presented in . Similar to a study in the United Kingdom (Trotta, Citation2018), the building characteristics have more explanatory power in terms of EER decisions than household characteristics. In studies focused on residential sectors in China, the household characteristics of education level and age groups, as well as the building characteristics of construction year and floor area, are identified as important factors affecting willingness to pay (Huang et al., Citation2021; Lai et al., Citation2021).
Different types of interventions, i.e. structural and informational interventions, can be implemented for different clusters. For instance, if the municipality aims to promote the insulation of the houses, they should probably target the category of the old buildings by supporting grants or subsidies or providing information on the advantages and stages of the renovation process. They could also promote PV panels for newly built dwellings. In Belgium, the ‘ecopack’ program provides higher subsidies for lower-income groups who plan to implement at least two energy-saving measures (Bartiaux et al., Citation2014). In a study of the United Kingdom homeowners, the importance of financial incentives for old dwellings, which require major renovations, and low-income neighbourhoods are emphasized (Wilson et al., Citation2015). Based on a study in China, people adopt energy-efficient technologies with full subsidies rather than separate renovations supported by a partial subsidy (Lai et al., Citation2021). Due to the high potential energy cost savings in old dwellings, the importance of financial incentives is discerned for low-income Canadian homeowners (Gamtessa, Citation2013).
Based on the results of the current study, homeowners who change their behaviour deliberately by using less gas/electricity consumption, for example, are more willing to conduct EERs. The local authorities can target this group of households first. This group can share their knowledge and experiences with other groups. In this way, distributing information regarding the EERs would be more straightforward. In a study in the United Kingdom, the information from the social network increases the probability of adopting EERs by households (McMichael & Shipworth, Citation2013). In the Netherlands, energy commissioners and energy ambassadors, who live in the same neighbourhoods as the residents, actively contribute to making their neighbourhoods more sustainable by initiating programs or helping their neighbours renovate the buildings more efficiently in terms of energy. These actors require the support of public authorities in facilitating the renovation process by, for instance, loans, subsidies, etc.
In terms of motivational factors, ‘improving the comfort and maintaining a good physical and structural conditions of house’ are identified as strongly important influencing factors for almost all types of EERs except solar PV panels. For the latter, cost savings on energy bills and reducing environmental impacts are significantly identified. Earlier studies identified the importance of these motivational factors in European countries (Baumhof et al., Citation2018; Christensen et al., Citation2014; Meijer et al., Citation2009; Michelsen & Madlener, Citation2013; Thuvander et al., Citation2012; Wilson et al., Citation2015). Therefore, ‘improving comfort’ performs better as a promotional message to the homeowners compared with other messages related to energy efficiency. Furthermore, to achieve the highest energy savings, the responsible organizations must reach homeowners conducting home maintenance and renovations and integrate EERs with these activities. Persuading a homeowner to add some energy-efficient renovations when conducting general maintenance can be a behavioural intervention.
Summary and conclusions
The current study aimed to identify behaviour-influencing factors on the energy-efficient renovation (EER) decisions of homeowners in the Netherlands. Applied behavioural studies are reviewed to determine the main influencing factors. The scope of this study is restricted to the specific classes of behaviour-influencing factors, i.e. contextual, personal, and motivational factors. Logistic regression analyses are conducted to examine the impact of these factors on EERs. Four types of EERs are investigated: double glazing, insulation, PV panels, and sustainable heating. These measures are the most popular ones among renovators and potential renovators. A recent dataset, the Netherlands housing survey energy module 2018 (Statistics Netherlands, Citation2019) released by the Ministry of Interior and Kingdom in collaboration with Statistic Netherlands (CBS), is used as the source of data.
Our empirical study provides pieces of evidence to support the importance of a number of contextual factors, e.g. household and building characteristics, especially construction periods, and energy labels. This is in accord with previous studies across different countries that have shown the importance of building and household characteristics on EER decisions. The results also showed that EERs are interrelated with other types of renovation. These results indicate that the preferred type of EER depends on the building and household characteristics. Therefore, responsible organizations can use different clusters of houses in promoting specific types of EERs.
The personal factors of awareness of energy consumption, perceived energy consumption compared to other households, perceived degree of efficiency in consuming heating energy, etc. are mentioned in the behavioural literature. In the regression analysis, the variables of ‘deliberately changing the behaviour to energy consumption’, ‘deliberately replacing the non-efficient devices with efficient ones’, and ‘perception of households regarding energy consumption compared to others’ are included to examine the importance of this group of behaviour-influencing factors in EERs. For all types of EERs, these specific influencing factors are identified as statistically significant, especially for PV panels. In regression analysis, awareness of energy consumption and the importance of energy efficiency behaviours are the indicators of moral obligations. As explained in the literature review, this conclusion is also valid for countries with similar institutional structures, such as Norway. The importance of these factors can be examined in different countries for future studies. For all types of EERs, at least one of these personal factors is significantly identified for renovators or potential renovators. Responsible organizations can first target groups with a higher probability of EERs to promote energy-efficient dwellings. The spread of knowledge and experiences of different types of EER implementation would then be facilitated.
Different types of motivational factors are identified as highly significant per type of EER. For instance, in the case of double glazing, the social motivation for renovators to improve comfort is significantly identified, and for PV panels, the economic motivation to save on energy bills is identified. These findings, specifically for energy-efficient technologies related to solar PV panels, are also in accordance with previous studies internationally. The main motivational factors need to be considered in promoting different types of EERs by responsible authorities.
The theoretical framework of this study is validated by the large sample size of the energy module 2018. Based on this method, the importance and the scale of the effects of behaviour-influencing factors on EERs are identified in the current study. Considering the validation of the theoretical framework with a large sample size, the efficacy of the method in this study is approved. The theoretical framework can be applied for future studies. The outcomes of this research can facilitate the design by public authorities in the Netherlands of more effective policy interventions for different household groups and categories of dwellings per type of EER.
Limitations of the current study and future research. The results of the current study are restricted to the available data from the energy module 2018. This survey is a representative sample of the residential sector in the Netherlands. The list of contextual and personal factors in the literature is more extensive, and few influencing factors are investigated in this study. For instance, the hassle factors were not covered in the energy module 2018. For future studies, these variables can be collected by conducting surveys. This study focuses on the Netherlands, with specific building and household characteristics. The theoretical framework can be examined with similar datasets from other countries. In this way, the robustness of the results can be examined. For future studies, the next step would be exploring other types of cognitive biases influencing EER decisions. The cognitive biases can cause inaction, delay, and unstable decisions. The behaviour of households towards EERs could be better predicted by considering the cognitive biases (Good, Citation2019; Häckel et al., Citation2017). For instance, risk aversion and loss aversion are important cognitive biases for any type of investment. Analysing the impacts of these cognitive biases on energy efficiency investment necessitates a more complicated model.
Disclosure statement
No potential conflict of interest was reported by the author(s).
Additional information
Funding
Notes
1 In 2018, the Dutch government selected 27 neighbourhoods, at least one per province, to support in removing gas as a source of energy. In this program, the dwellings are renovated by a combination of good insulation, economical installations for heating and hot water, and the use of renewable energy sources (Government of the Netherlands, Citation2019b).
2 The Dutch Ministry of the Interior and Kingdom Relations conducts a survey every 5–6 years on the energy consumption, energy behaviour of households, as well as the investment behaviour of households with regard to energy-saving measures in the rental and private building stocks. The Netherlands housing survey energy modules also contains other variables that are collected through the dwelling inspections, reports on energy consumption, other datasets, such as the Netherlands Enterprise Agency (in Dutch: Rijksdienst Voor Ondernemend Nederland (RVO)) dataset, containing building characteristics, such as energy labels (Woononderzoek, Citation2020).
3 An obligatory energy labelling of existing dwellings is dictated for European countries by Energy Performance of Building Directive (EPBD). In the Netherlands, the energy labelling system is implemented since 2008. The energy label is calculated using the building characteristics, heating, ventilation, and cooling systems, and standard usage characteristics (Majcen et al., Citation2013).
4 shows the main outputs of the logistic regressions. Appendix B presents all the outputs including β, df, S.E., Wald test, Exp(b).
5 The categories of household composition differ for the double glazing and solar PV panel. Therefore, this variable is not included in . Please see Appendix B.
References
- Abrahamse, W., & Schuitema, G. (2020). Psychology and energy conservation: Contributions from theory and practice. In Energy and behaviour (pp. 19–44). Elsevier. doi:https://doi.org/10.1016/B978-0-12-818567-4.00001-6
- Ajzen, I., (1991). The theory of planned behavior. Organizational Behavior and Human Decision Processes, 50(2), 179–211. doi:https://doi.org/10.1016/0749-5978(91)90020-T
- Al-Homoud, M. S. (2005). Performance characteristics and practical applications of common building thermal insulation materials. Building and Environment, 40(3), 353–366. doi:https://doi.org/10.1016/j.buildenv.2004.05.013
- Ardente, F., Beccali, M., Cellura, M., & Mistretta, M. (2011). Energy and environmental benefits in public buildings as a result of retrofit actions. Renewable and Sustainable Energy Reviews, 15(1), 460–470. doi:https://doi.org/10.1016/j.rser.2010.09.022
- Aydin, E., Brounen, D., & Kok, N. (2018). Information provision and energy consumption: Evidence from a field experiment. Energy Economics, 71, 403–410. doi:https://doi.org/10.1016/j.eneco.2018.03.008
- Bartiaux, F., Gram-Hanssen, K., Fonseca, P., Ozoliņa, L., & Christensen, T. H. (2014). A practice–theory approach to homeowners’ energy retrofits in four European areas. Building Research & Information, 42(4), 525–538. doi:https://doi.org/10.1080/09613218.2014.900253
- Baumhof, R., Decker, T., Röder, H., & Menrad, K. (2018). Which factors determine the extent of house owners’ energy-related refurbishment projects? A motivation-opportunity-ability approach. Sustainable Cities and Society, 36, 33–41. doi:https://doi.org/10.1016/j.scs.2017.09.025
- Benoît, J.-P., Dubra, J., & Moore, D. A. (2009, February 4). Does the better-than-average effect show that people are overconfident?: An experiment.
- Bergman, N., & Foxon, T. J. (2020). Reframing policy for the energy efficiency challenge: Insights from housing retrofits in the United Kingdom. Energy Research & Social Science, 63, 101386. doi:https://doi.org/10.1016/j.erss.2019.101386
- Bertoldi, P., Economidou, M., Palermo, V., Boza-Kiss, B., & Todeschi, V. (2020). How to finance energy renovation of residential buildings: Review of current and emerging financing instruments in the EU. Wiley Interdisciplinary Reviews: Energy and Environment, 10(1), e384.
- Brant, R. (1990). Assessing proportionality in the proportional odds model for ordinal logistic regression. Biometrics 46(4), 1171–1178. doi:https://doi.org/10.2307/2532457
- Breheny, P., & Burchett, W. (2017). Visualization of regression models using visreg. The R Journal, 9(2), 56. doi:https://doi.org/10.32614/RJ-2017-046
- Brounen, D., Kok, N., & Quigley, J. M. (2013). Energy literacy, awareness, and conservation behavior of residential households. Energy Economics, 38, 42–50. doi:https://doi.org/10.1016/j.eneco.2013.02.008
- Brouwer, E.-J. (2019). Climate and energy exploration 2019: Ambitious goals give energy transition momentum, implementation appears unmanageable. Retrieved from https://www.pbl.nl/nieuws/2019/klimaat-en-energieverkenning-2019-ambitieuze-doelen-geven-energietransitie-elan-uitvoering-blijkt-weerbarstig
- Camarasa, C., Nägeli, C., Ostermeyer, Y., Klippel, M., & Botzler, S. (2019). Diffusion of energy efficiency technologies in European residential buildings: A bibliometric analysis. Energy and Buildings, 202, 109339. doi:https://doi.org/10.1016/j.enbuild.2019.109339
- Christensen, T. H., Gram-Hanssen, K., de Best-Waldhober, M., & Adjei, A. (2014). Energy retrofits of Danish homes: Is the energy performance certificate useful? Building Research & Information, 42(4), 489–500. doi:https://doi.org/10.1080/09613218.2014.908265
- De Vries, G., Rietkerk, M., & Kooger, R. (2019). The hassle factor as a psychological barrier to a green home. Journal of Consumer Policy, 1–8.
- Duurzaam Bouwloket (2019). National subsidies and financial schemes [Dutch: Landelijke subsidies- en financieringsregelingen]. Retrieved from https://www.duurzaambouwloket.nl/subsidie-financieringsregeling/9
- Ebrahimigharehbaghi, S., Qian, Q. K., Meijer, F. M., & Visscher, H. J. (2020). Transaction costs as a barrier in the renovation decision-making process: A study of homeowners in the Netherlands. Energy and Buildings, 109849. doi:https://doi.org/10.1016/j.enbuild.2020.109849
- Faruqui, A., Sergici, S., & Sharif, A. (2010). The impact of informational feedback on energy consumption—A survey of the experimental evidence. Energy, 35(4), 1598–1608. doi:https://doi.org/10.1016/j.energy.2009.07.042
- Friege, J., & Chappin, E. (2014). Modelling decisions on energy-efficient renovations: A review. Renewable and Sustainable Energy Reviews, 39, 196–208. doi:https://doi.org/10.1016/j.rser.2014.07.091
- Gamtessa, S. F. (2013). An explanation of residential energy-efficiency retrofit behavior in Canada. Energy and Buildings, 57, 155–164. doi:https://doi.org/10.1016/j.enbuild.2012.11.006
- Gans, W., Alberini, A., & Longo, A. (2013). Smart meter devices and the effect of feedback on residential electricity consumption: Evidence from a natural experiment in Northern Ireland. Energy Economics, 36, 729–743. doi:https://doi.org/10.1016/j.eneco.2012.11.022
- Gasparella, A., Pernigotto, G., Cappelletti, F., Romagnoni, P., & Baggio, P. (2011). Analysis and modelling of window and glazing systems energy performance for a well insulated residential building. Energy and Buildings, 43(4), 1030–1037. doi:https://doi.org/10.1016/j.enbuild.2010.12.032
- Gigerenzer, G., & Selten, R. (2002). Bounded rationality: The adaptive toolbox. MIT Press.
- Good, N. (2019). Using behavioural economic theory in modelling of demand response. Applied Energy, 239, 107–116. doi:https://doi.org/10.1016/j.apenergy.2019.01.158
- Government of the Netherlands. (2019a). Climate policy. Retrieved from https://www.government.nl/topics/climate-change/climate-policy
- Government of the Netherlands. (2019b). Make existing homes gas-free. Retrieved from https://www.rijksoverheid.nl/onderwerpen/aardgasvrije-wijken/bestaande-gebouwen-aardgasvrij-maken
- Haque, A. N., Lemanski, C., & De Groot, J. (2021). Why do low-income urban dwellers reject energy technologies? Exploring the socio-cultural acceptance of solar adoption in Mumbai and Cape Town. Energy Research & Social Science, 74, 101954. doi:https://doi.org/10.1016/j.erss.2021.101954
- Hardeman, W., Johnston, M., Johnston, D., Bonetti, D., Wareham, N., & Kinmonth, A. L. (2002). Application of the theory of planned behaviour in behaviour change interventions: A systematic review. Psychology and Health, 17(2), 123–158. doi:https://doi.org/10.1080/08870440290013644a
- Hargreaves, T. (2011). Practice-ing behaviour change: Applying social practice theory to pro-environmental behaviour change. Journal of Consumer Culture, 11(1), 79–99. doi:https://doi.org/10.1177/1469540510390500
- Häckel, B., Pfosser, S., & Tränkler, T. (2017). Explaining the energy efficiency gap-expected utility theory versus cumulative prospect theory. Energy Policy, 111, 414–426. doi:https://doi.org/10.1016/j.enpol.2017.09.026
- Hu, S., Yan, D., Azar, E., & Guo, F. (2020). A systematic review of occupant behavior in building energy policy. Building and Environment, 175, 106807.
- Huang, C., Ma, J., & Song, K. (2021). Homeowners’ willingness to make investment in energy efficiency retrofit of residential buildings in China and its influencing factors. Energies, 14(5), 1260. doi:https://doi.org/10.3390/en14051260
- Huebner, G. M., Hamilton, I., Chalabi, Z., Shipworth, D., & Oreszczyn, T. (2015). Explaining domestic energy consumption – The comparative contribution of building factors, socio-demographics, behaviours and attitudes. Applied Energy, 159, 589–600. doi:https://doi.org/10.1016/j.apenergy.2015.09.028
- Huebner, G., Shipworth, D., Hamilton, I., Chalabi, Z., & Oreszczyn, T. (2016). Understanding electricity consumption: A comparative contribution of building factors, socio-demographics, appliances, behaviours and attitudes. Applied Energy, 177, 692–702. doi:https://doi.org/10.1016/j.apenergy.2016.04.075
- IEA. (2017). Energy technology perspectives 2017. In Catalysing energy technology transformations.
- Independent Association of Homeowner Associations. (2019). Energy subsidies. Retrieved from https://www.eigenhuis.nl/energie/Subsidies#/
- Jansma, S. R., Gosselt, J. F., & de Jong, M. D. (2020). Kissing natural gas goodbye? Homeowner versus tenant perceptions of the transition towards sustainable heat in the Netherlands. Energy Research & Social Science, 69, 101694. doi:https://doi.org/10.1016/j.erss.2020.101694
- Jensen, P. A., Maslesa, E., Berg, J. B., & Thuesen, C. (2018). 10 questions concerning sustainable building renovation. Building and Environment, 143, 130–137. doi:https://doi.org/10.1016/j.buildenv.2018.06.051
- Joint Research Centre. (2019). Accelerating energy renovation investments in buildings: Financial and fiscal instruments across the EU (Tech. rep.). European Commissions.
- Kerr, N., & Winskel, M. (2020). Household investment in home energy retrofit: A review of the evidence on effective public policy design for privately owned homes. Renewable and Sustainable Energy Reviews, 123, 109778. doi:https://doi.org/10.1016/j.rser.2020.109778
- Klöckner, C. A., & Nayum, A. (2017). Psychological and structural facilitators and barriers to energy upgrades of the privately owned building stock. Energy, 140, 1005–1017. doi:https://doi.org/10.1016/j.energy.2017.09.016
- Lai, Y., Li, Y., Feng, X., & Ma, T. (2021). Green retrofit of existing residential buildings in China: An investigation on residents’ perceptions. Energy & Environment. doi:https://doi.org/10.1177/0958305X21998043
- Larrick, R. P., Burson, K. A., & Soll, J. B. (2007). Social comparison and confidence: When thinking you’re better than average predicts overconfidence (and when it does not). Organizational Behavior and Human Decision Processes, 102(1), 76–94. doi:https://doi.org/10.1016/j.obhdp.2006.10.002
- Lee, E., Kang, M., Song, J., & Kang, M. (2020). From intention to action: Habits, feedback and optimizing energy consumption in South Korea. Energy Research & Social Science, 64, 101430. doi:https://doi.org/10.1016/j.erss.2020.101430
- Leeper, T. J. (2017). Interpreting regression results using average marginal effects with r’s margins (Reference manual 32).
- Lehner, M., Mont, O., & Heiskanen, E. (2016). Nudging – A promising tool for sustainable consumption behaviour? Journal of Cleaner Production, 134, 166–177. doi:https://doi.org/10.1016/j.jclepro.2015.11.086
- Lewis, F., Butler, A., & Gilbert, L. (2011). A unified approach to model selection using the likelihood ratio test. Methods in Ecology and Evolution, 2(2), 155–162. doi:https://doi.org/10.1111/j.2041-210X.2010.00063.x
- Lynham, J., Nitta, K., Saijo, T., & Tarui, N. (2016). Why does real-time information reduce energy consumption? Energy Economics, 54, 173–181. doi:https://doi.org/10.1016/j.eneco.2015.11.007
- Mahapatra, K., & Gustavsson, L. (2009). Influencing Swedish homeowners to adopt district heating system. Applied Energy, 86(2), 144–154. doi:https://doi.org/10.1016/j.apenergy.2008.03.011
- Mahapatra, K., Gustavsson, L., Haavik, T., Aabrekk, S., Svendsen, S., Vanhoutteghem, L., Paiho, S., & Ala-Juusela, M. (2013). Business models for full service energy renovation of single-family houses in Nordic countries. Applied Energy, 112, 1558–1565. doi:https://doi.org/10.1016/j.apenergy.2013.01.010
- Majcen, D., Itard, L., & Visscher, H. (2013). Energy labels in Dutch dwellings – Their actual energy consumption and implications for reduction targets. ECEEE 2013 Summer Study – Rethink, Renew, Restart.
- Matsukawa, I. (2004). The effects of information on residential demand for electricity. The Energy Journal, 25(1). doi:https://doi.org/10.5547/ISSN0195-6574-EJ-VOL25-NO1-2.NADEL
- McMichael, M., & Shipworth, D. (2013). The value of social networks in the diffusion of energy-efficiency innovations in UK households. Energy Policy, 53, 159–168. doi:https://doi.org/10.1016/j.enpol.2012.10.039
- Meijer, F., Itard, L., & Sunikka-Blank, M. (2009). Comparing European residential building stocks: Performance, renovation and policy opportunities. Building Research & Information, 37(5-6), 533–551. doi:https://doi.org/10.1080/09613210903189376
- Michelsen, C. C., & Madlener, R. (2013). Motivational factors influencing the homeowners’ decisions between residential heating systems: An empirical analysis for Germany. Energy Policy, 57, 221–233. doi:https://doi.org/10.1016/j.enpol.2013.01.045
- Midi, H., Sarkar, S. K., & Rana, S. (2010). Collinearity diagnostics of binary logistic regression model. Journal of Interdisciplinary Mathematics, 13(3), 253–267. doi:https://doi.org/10.1080/09720502.2010.10700699
- Milieu Centraal Information Organization. (2019). Energy subsidy/loan guide [Dutch: Energiesubsidiewijzer]. Retrieved from https://www.verbeterjehuis.nl/energiesubsidiewijzer/
- Nair, G., Gustavsson, L., & Mahapatra, K. (2010a). Factors influencing energy efficiency investments in existing Swedish residential buildings. Energy Policy, 38(6), 2956–2963. doi:https://doi.org/10.1016/j.enpol.2010.01.033
- Nair, G., Gustavsson, L., & Mahapatra, K. (2010b). Owners perception on the adoption of building envelope energy efficiency measures in Swedish detached houses. Applied Energy, 87(7), 2411–2419. doi:https://doi.org/10.1016/j.apenergy.2010.02.004
- The Netherlands Enterprise Agency. (2019a). ISDE: Sustainable energy subsidy for private individuals [Dutch: ISDE: Subsidie duurzame energie voor particulieren]. Retrieved from https://www.rvo.nl/subsidie-en-financieringswijzer/isde/particulieren
- The Netherlands Enterprise Agency. (2019b). Subsidy energy saving own home (SEEH) for owner and resident [Dutch: Subsidie energiebe- sparing eigen huis (SEEH) voor eigenaar én bewoner]. Retrieved from https://www.rvo.nl/subsidie-en-financieringswijzer/seeh/eigenaar-n-bewoner
- Netherlands Enterprise Agency (RVO). (2019). Subsidy energy saving homeowners and tenants. https://www.rijksoverheid.nl/onderwerpen/aardgasvrije-wijken/bestaande-gebouwen-aardgasvrij-maken
- Netherlands Environmental Agency (PBL). (2019). The climate agreement: Effects and points of attention (Tech. rep.). Netherlands Environmental Agency.
- Netherlands Statistics. (2020). Tock homes and non-homes; mutations, use function, region. Retrieved from https://opendata.cbs.nl/statline/#/CBS/nl/dataset/81955ned/table?dl=31571
- Nilsson, A., Bergstad, C. J., Thuvander, L., Andersson, D., Andersson, K., & Meiling, P. (2014). Effects of continuous feedback on households’ electricity consumption: Potentials and barriers. Applied Energy, 122, 17–23. doi:https://doi.org/10.1016/j.apenergy.2014.01.060
- Organ, S., Proverbs, D., & Squires, G. (2013). Motivations for energy efficiency refurbishment in owner-occupied housing. Structural Survey, 31(2), 101–120.
- Pothitou, M., Hanna, R. F., & Chalvatzis, K. J. (2016). Environmental knowledge, pro-environmental behaviour and energy savings in households: An empirical study. Applied Energy, 184, 1217–1229. doi:https://doi.org/10.1016/j.apenergy.2016.06.017
- Risholt, B., & Berker, T. (2013). Success for energy efficient renovation of dwellings—Learning from private homeowners. Energy Policy, 61, 1022–1030. doi:https://doi.org/10.1016/j.enpol.2013.06.011
- Ritzen, M., Haagen, T., Rovers, R., Vroon, Z., & Geurts, C. (2016). Environmental impact evaluation of energy saving and energy generation: Case study for two Dutch dwelling types. Building and Environment, 108, 73–84. doi:https://doi.org/10.1016/j.buildenv.2016.07.020
- Salata, F., Golasi, I., Domestico, U., Banditelli, M., Basso, G. L., Nastasi, B., & de Lieto Vollaro, A. (2017). Heading towards the nZEB through CHP + HP systems. A comparison between retrofit solutions able to increase the energy performance for the heating and domestic hot water production in residential buildings. Energy Conversion and Management, 138, 61–76. doi:https://doi.org/10.1016/j.enconman.2017.01.062
- Sandberg, N. H., Næss, J. S., Brattebø, H., Andresen, I., & Gustavsen, A. (2021). Large potentials for energy saving and greenhouse gas emission reductions from large-scale deployment of zero emission building technologies in a national building stock. Energy Policy, 152, 112114.
- Sanguinetti, A., Dombrovski, K., & Sikand, S. (2018). Information, timing, and display: A design-behavior framework for improving the effectiveness of eco-feedback. Energy Research & Social Science, 39, 55–68. doi:https://doi.org/10.1016/j.erss.2017.10.001
- Schley, D. R., & DeKay, M. L. (2015). Cognitive accessibility in judgments of household energy consumption. Journal of Environmental Psychology, 43, 30–41. doi:https://doi.org/10.1016/j.jenvp.2015.05.004
- Shen, M., Lu, Y., Wei, K. H., & Cui, Q. (2020). Prediction of household electricity consumption and effectiveness of concerted intervention strategies based on occupant behaviour and personality traits. Renewable and Sustainable Energy Reviews, 127, 109839. doi:https://doi.org/10.1016/j.rser.2020.109839
- Simpson, N. P., Rabenold, C. J., Sowman, M., & Shearing, C. D. (2021). Adoption rationales and effects of off-grid renewable energy access for African youth: A case study from Tanzania. Renewable and Sustainable Energy Reviews, 141, 110793. doi:https://doi.org/10.1016/j.rser.2021.110793
- Statistics Netherlands. (2019). WoON 2018 Energiemodule. Retrieved from https://disco.datawonen.nl/jaargang?bronid=WOON&jaargangid=2018_EM
- Steemers, K., & Yun, G. Y. (2009). Household energy consumption: A study of the role of occupants. Building Research & Information, 37(5-6), 625–637. doi:https://doi.org/10.1080/09613210903186661
- Steg, L., & Vlek, C. (2009). Encouraging pro-environmental behaviour: An integrative review and research agenda. Journal of Environmental Psychology, 29(3), 309–317. doi:https://doi.org/10.1016/j.jenvp.2008.10.004
- Stieß, I., & Dunkelberg, E. (2013). Objectives, barriers and occasions for energy efficient refurbishment by private homeowners. Journal of Cleaner Production, 48, 250–259. doi:https://doi.org/10.1016/j.jclepro.2012.09.041
- Streimikiene, D., Balezentis, T., & Alebaite, I. (2020). Climate change mitigation in households between market failures and psychological barriers. Energies, 13(11), 2797. doi:https://doi.org/10.3390/en13112797
- Stren, P. (2000). Toward a coherent theory of environmentally significant behaviour. Journal of Social Issues, 56(3), 407–424. doi:https://doi.org/10.1111/0022-4537.00175
- Taranu, V., & Verbeeck, G. (2016). Overview of dual process behavioural models and their implications on decision-making of private dwellers regarding deep energy renovation. In WBC16 Proceedings: Volume II Environmental Opportunities and Challenges Constructing Commitment and Acknowledging Human Experiences (pp. 591–603). TUT–Tampere University of Technology.
- Thuvander, L., Femenías, P., Mjörnell, K., & Meiling, P. (2012). Unveiling the process of sustainable renovation. Sustainability, 4(6), 1188–1213. doi:https://doi.org/10.3390/su4061188
- Trotta, G. (2018). The determinants of energy efficient retrofit investments in the English residential sector. Energy Policy, 120, 175–182. doi:https://doi.org/10.1016/j.enpol.2018.05.024
- Tsagarakis, K. P., Karyotakis, K., & Zografakis, N. (2012). Implementation conditions for energy saving technologies and practices in office buildings: Part 2. Double glazing windows, heating and air-conditioning. Renewable and Sustainable Energy Reviews, 16(6), 3986–3998. doi:https://doi.org/10.1016/j.rser.2012.03.007
- Van den Brom, P., Hansen, A. R., Gram-Hanssen, K., Meijer, A., & Visscher, H. (2019). Variances in residential heating consumption–importance of building characteristics and occupants analysed by movers and stayers. Applied Energy, 250, 713–728. doi:https://doi.org/10.1016/j.apenergy.2019.05.078
- van Leeuwen, R. P., de Wit, J. B., & Smit, G. J. (2017). Review of urban energy transition in the Netherlands and the role of smart energy management. Energy Conversion and Management, 150, 941–948. doi:https://doi.org/10.1016/j.enconman.2017.05.081
- Vassileva, I., Wallin, F., & Dahlquist, E. (2012). Analytical comparison between electricity consumption and behavioral characteristics of Swedish households in rented apartments. Applied Energy, 90(1), 182–188. doi:https://doi.org/10.1016/j.apenergy.2011.05.031
- Vieira, F. M., Moura, P. S., & de Almeida, A. T. (2017). Energy storage system for self-consumption of photovoltaic energy in residential zero energy buildings. Renewable Energy, 103, 308–320. doi:https://doi.org/10.1016/j.renene.2016.11.048
- Wilson, C., Crane, L., & Chryssochoidis, G. (2015). Why do homeowners renovate energy efficiently? Contrasting perspectives and implications for policy. Energy Research & Social Science, 7, 12–22. doi:https://doi.org/10.1016/j.erss.2015.03.002
- Wilson, C., Pettifor, H., & Chryssochoidis, G. (2018). Quantitative modelling of why and how homeowners decide to renovate energy efficiently. Applied Energy, 212, 1333–1344. doi:https://doi.org/10.1016/j.apenergy.2017.11.099
- Woononderzoek. (2020). Insights from the WoON 2018 energy module. Retrieved from https://www.woononderzoek.nl/nieuws/Inzichten-uit-de-Energiemodule-WoON-2018/93
- Yang, H., Liu, L., Li, X., Liu, C., & Jones, P. (2017). Tailored domestic retrofit decision making towards integrated performance targets in Tianjin, China. Energy and Buildings, 140, 480–500. doi:https://doi.org/10.1016/j.enbuild.2016.12.040