ABSTRACT
This article aims to shed light on the infodemic of disinformation, misinformation, and malinformation in the digital era of a new normal where deepfake and fake news are omnipresent. To do so, this article establishes a general theory of information and engages in big data analytics involving a scientometrics (bibliometrics) analysis with topic modeling of the major trends in the field. In doing so, this article reveals three noteworthy findings. First, infodemic is multifaceted in terms of its content, platform, manifestation, and solution. Second, infodemic research delivers the richest insights on misinformation, followed by fake news, disinformation, infodemic solutions, and malinformation. Third, interest in the dissemination of false information is greater than its creation, with the task of ascertaining the intrinsic elements (e.g. maliciousness in disinformation and malinformation) being more challenging than that of extrinsic elements (e.g. truth of misinformation). The article concludes with implications for theory and practice as well as directions for future research.
Introduction
With technology adoption and digital transformation accelerated rapidly and scaled substantially by the COVID-19 pandemic (Lim, Citation2021), the world has inarguably experienced a transformative transition to the digital era (Lim, Citation2022), resulting in a new normal where data is widely regarded as the new oil (Ciasullo & Lim, Citation2022). Just like oil, which is worth much more when it is transformed into something more constructive (e.g. gasoline), data has a much higher value when it is processed into information (i.e. insight), which can help entities (e.g. agencies, companies, consumers) gain a better understanding (e.g. market conditions) and make more informed decisions (e.g. strategy). Unlike oil, which is a scarce commodity, data, and by extension, information, is infinite and fast growing. Yet, data and information are a complex resource that do always produce rosy outcomes.
Of particular interest in this article is information, which can be defined as data that has been processed in a way that conveys meaning and thus holds the power to inform.Footnote1 The recent developments on deepfakes (see Lim et al., Citation2023; Seow et al., Citation2022) and fake news (see Jain et al., Citation2023; Lim et al., Citation2022; Nguyen et al., Citation2022; Raponi et al., Citation2022) in the digital era have ignited significant interest in the nature of information albeit in a concerning way, particularly with regards to the noteworthy rise of an infodemic (i.e. a pandemic of information disorders) involving disinformation (i.e. false information shared by the sender despite knowing its truth—e.g. fabricated or manipulated content), misinformation (i.e. false information shared by the sender thought to be true—e.g. misleading content), and malinformation (i.e. real information shared by the sender with malicious intention—e.g. harassing, hateful, and leaked content). Deepfakes can be described as visual (e.g. image, video) manipulation using artificial intelligence and deep learning technologies to create fake events (e.g. face swap), whereas fake news can be expressed as information that is not true but disguised as news that is supposedly true (e.g. parody news, satire websites). While deepfake is deliberately created and thus obviously intentional, when disseminated, deepfake can turn into fake news, which, in turn, may be shared intentionally (i.e. disinformation; sharing while knowing the truth about falsehood) or unintentionally (i.e. misinformation; sharing while not knowing the truth about falsehood), though it is also important to note that both deepfake and fake news may be produced and shared with (e.g. defamation) and without (e.g. parody) malicious intent. Nevertheless, some supposed deepfakes and fake news could, in fact, turn out to be real and may amount to malinformation when they are shared with malicious intent (e.g. revenge porn). This complexity in the world of information can be synthesized and summarized into a general theory of information, which is originally illustrated in .
Figure 1. A general theory of information.Author’s original illustration. + = positive impact. – = negative impact = infodemic = a pandemic of information disorders = disinformation, misinformation, and malinformation. i = intentional. u = unintentional. m = malicious. nm = non-malicious.
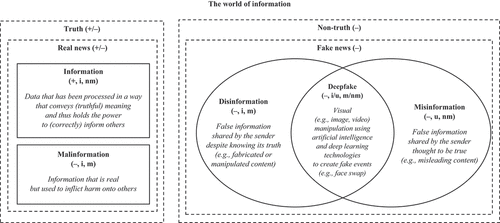
The body of knowledge on the infodemic of disinformation, misinformation, and malinformation in the digital era is undoubtedly very rich. While several scholars have attempted to provide a review of this field, the design, and by extension, outcomes, of their studies remain limited in several notable ways, which, when taken collectively, substantially motivates the need for fresh review of the field. First, past scholars have considered only a limited number of studies (e.g. Di Domenico et al., Citation2021—117 studies) despite the field, in reality, being very much larger (e.g. few thousand studies), which, in turn, limits the representation of insights. Second, prior scholars have offered only a fragmented view of the field (e.g. Kapantai et al., Citation2021—disinformation; Patra et al., Citation2023—fake news) rather than the field in its entirety, which, in turn, limits the breadth or scope of insights. Third, several scholars have tried to provide a holistic view of the field, but they do not adequately discern between the different concepts in the field (e.g. Bran et al., Citation2021—no distinction in findings between different information disorders), which, in turn, limits the depth or rigor of insights. Fourth, some scholars have explored the field only within a restricted context (e.g. Akram et al., Citation2022—social media), which, in turn, limits the generalizability of insights. Fifth and finally, various scholars have reviewed the field, but few have looked into the range of potential solutions available to address the issues (e.g. Ansar & Goswami, Citation2021—detection of fake news using machine learning methods), which, in turn, limits the impact of insights.
To this end, this article aims to shed light on the infodemic of disinformation, misinformation, and malinformation. To do so in a comprehensive and rigorous manner, this article engages in big data analytics involving a scientometrics analysis with topic modeling of the major trends emerging out of research in the field.Footnote2 This approach leverages the power of machine learning to uncover and map the nomological network of scientific knowledge (Donthu et al., Citation2021; Mukherjee et al., Citation2022). Unlike traditional content analysis or thematic analysis, which is often subjective, topic modeling using scientometrics (or bibliometrics) tends to be objective due to its reliance on algorithms and statistics to arrive at the thematic clusters of the most prominent topics in the field (Kraus et al., Citation2022; Lim et al., Citation2022; Paul et al., Citation2021). The topic modeling herein is performed using a co-occurrence analysis of keywords, wherein the keywords that authors specify to represent the essence of their research form a cluster when they frequently appear or occur together (i.e. topic model) (Donthu et al., Citation2021). To deliver greater representation, breadth (scope), depth (rigor), generalizability, and impact in insights, a multi-study approach is undertaken, wherein a co-occurrence analysis of keywords is performed on the field as a collective and across specific areas (e.g. different information disorders; issues versus solutions), which is in line with recent guides (Kraus et al., Citation2022; Lim et al., Citation2022) and practices (Lim et al., Citation2023) for finer-grained reviews of the field. Sensemaking, which involves the process of scanning, sensing, and substantiating through which meaningful interpretations and justifications are attributed to observations following meticulous assessment (Lim & Kumar, Citation2023), is also applied to ensure that the topic models are interpreted in a logical manner (see Kumar et al., Citation2022; Lim, Rasul et al., Citation2022). Scopus is leveraged to acquire the bibliographic records of extant research in the field as it is one of the largest scientific databases (Paul et al., Citation2021), and as compared to Web of Science, which limits the export of bibliographic records, the latest version of Scopus enables the complete download of bibliographical records herein and thus represents the most pragmatic choice of scientific database at the time of study. VOSviewer, which is free to download and user friendly in features, is used to perform topic modeling by means of a co-occurrence analysis of keywords (Van Eck & Waltman, Citation2010).Footnote3 The exact details of the goals, methods, and findings are disclosed in the respective studies and summarized in . In doing so, this article will contribute nuanced insights into the infodemic state of disinformation, misinformation, and malinformation, which, in turn, will serve as an objective basis for curating future directions that endeavor to meaningfully extend the body of knowledge (theoretical contribution) and inform practice (managerial contribution) (Kraus et al., Citation2022; Mukherjee et al., Citation2022). Importantly, in the marketing context, this study offers invaluable information for marketers seeking to optimize their communication strategies in an infodemic-ridden digital landscape. Specifically, the study provides a comprehensive understanding of the various forms of information disorders, equipping marketers with the knowledge needed to maintain the integrity and credibility of their messages amidst prevalent misinformation, disinformation, and malinformation. Moreover, the study’s findings on effective solutions against infodemics can inform and reshape digital marketing strategies, contributing to a more authentic, transparent, and reliable digital marketing environment. This is particularly relevant as marketing communication increasingly relies on digital platforms where these information disorders are rampant. Therefore, the implications of this study are far-reaching, potentially revolutionizing marketing theory and practice in the digital age.
Table 1. Multi-study overview of goals and methods to explore infodemic research.
Table 2. Multi-study overview of major contributors and themes for infodemic research.
Study 1
Goal
The study aims to offer a broad overview of research-informed insights on infodemic (focus). To do so, this study adopts an undifferentiated approach where the scope of infodemic is taken in its entirety (i.e. related concepts are taken collectively rather than independently). In doing so, this study contributes to a state-of-the-art overview of major trends on infodemic.
Method
The study engages in big data analytics of major trends emerging out of research on infodemic.
To curate the dataset (corpus curation), a search for ‘final’ (publication stage)Footnote4 ‘article’ (document type)Footnote5 published in ‘English’ (language)Footnote6 and ‘journal’ (source type)Footnote7 on ‘“infodemic” OR “disinformation” OR “dis-information” OR “misinformation” OR “mis-information” OR “malinformation” OR “mal-information” OR “deepfake” OR “fake news”’ (search keywords)Footnote8 in the ‘article title, abstract, or keywords’ (search field)Footnote9 up to ‘2022’ (search period)Footnote10 was conducted on Scopus (database)Footnote11 on 1 January 2023 (search date), returning a total of 10,406 articles (search results). These considerations and their equivalent rationales in the footnotes are in line with Kraus et al. (Citation2022) and Paul et al. (Citation2021).
To analyze the dataset (corpus analysis), a performance analysis is conducted using the analytics function in the Scopus database to reveal insights on the publication performance of infodemic research. In addition, a topic modeling using a co-occurrence analysis of keywords is performed in the VOSviewer software to uncover scientific insights into the major trends espoused through infodemic research. These two analytical techniques are part and parcel of scientometrics analysis in line with Donthu et al. (Citation2021) and Mukherjee et al. (Citation2022). The interpretation of results is guided by sensemaking, involving scanning, sensing, and substantiating, turning information into insight (Lim & Kumar, Citation2023). Each keyword represents a ‘topic’ while each (colored) cluster of keywords/topics represents a ‘theme’ (Donthu et al., Citation2021; Mukherjee et al., Citation2022).
Findings
Major contributors
Infodemic research has many contributors. The prolific authors include Elizabeth F. Loftus (42 articles), Ullrich K. H. Ecker (36 articles), and Stephan Lewandowsky (34 articles); their most impactful contributions revolve around the infodemic of misinformation – namely, the creation of new memories from misinformation (Loftus & Hoffman, Citation1989) (≥300 citations) and the continued influence and successful debiasing of misinformation (Lewandowsky et al., Citation2012) (≥1,300 citations). The prolific institutions (Harvard University—169 articles; The University of Sydney—93 articles; and University of Oxford—92 articles) and countries (United States—4,050 articles; United Kingdom—1,071 articles; and Australia—607 articles) are fairly consistent, wherein the former are located in the latter. Prolific funders include the National Science Foundation (211 articles), National Institutes of Health (199 articles), and National Natural Science Foundation of China (164 articles), whereas prolific publishers include PLOS ONE (168 articles), International Journal of Environmental Research and Public Health (146 articles), and Journal of Medical Internet Research (129 articles); they represent where infodemic research can be funded and published.
Major themes
The topic model of infodemic research emerging from a co-occurrence analysis of 493 keywords/topics with a minimum of 10 occurrences revealed 11 major clusters/themes ().
Figure 2. Major clusters/themes in infodemic research.Normalization method: Association strength. Visualization scale: 1.00. Weights: Occurrences. Label size: 0.50. Line size: 0.50. Cluster/Theme 1 (Red): Infodemic in public health discourse (145 keywords/topics). Cluster/Theme 2 (Green): Infodemic in political discourse (124 keywords/topics). Cluster/Theme 3 (Blue): Combating infodemic with information literacy (59 keywords/topics). Cluster/Theme 4 (Yellow): Psychology of infodemic (58 keywords/topics). Cluster/Theme 5 (Purple): Combating infodemic with data analytics (50 keywords/topics). Cluster/Theme 6 (Light blue): Infodemic in health information (27 keywords/topics). Cluster/Theme 7 (Orange): Infodemic modeling (20 keywords/topics). Cluster/Theme 8 (Brown): Conspiracies in infodemic (5 keywords/topics). Cluster/Theme 9 (Pink): Infodemic in social practice discourse (2 keywords/topics). Cluster/Theme 10 (Light pink): Information-shaping behavior in infodemic (2 keywords/topics). Cluster/Theme 11 (Light green): Combating infodemic through third-person effect (1 keyword/topic).
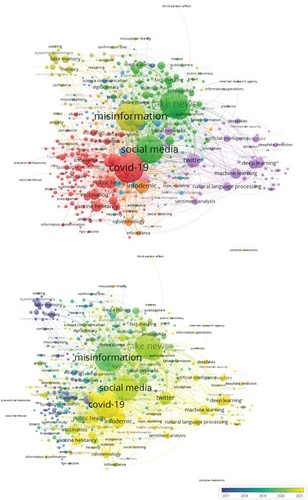
Cluster/Theme 1 captures 145 keywords/topics. The most occurring keywords/topics are ‘covid-19’ (1,404 occurrences), ‘pandemic’ (223 occurrences), ‘public health’ (221 occurrences), ‘vaccine hesitancy’ (193 occurrences), and ‘vaccination’ (174 occurrences) while the most impactful keywords/topics are ‘vaccine confidence’ (55.9 average citations), ‘outbreak’ (48.7 average citations), ‘epidemiology’ (43.9 average citations), ‘treatment’ (41.2 average citations), and ‘vaccines’ (39.6 average citations), which, when taken collectively, accentuate the manifestation of an infodemic in public health discourse.
Cluster/Theme 2 comprises 124 keywords/topics. The most occurring keywords/topics are ‘fake news’ (1,274 occurrences), ‘social media’ (1,250 occurrences), ‘disinformation’ (663 occurrences), ‘Facebook’ (121 occurrences), and ‘fact-checking’ (114 occurrences) while the most impactful keywords/topics are ‘news media’ (70 average citations), ‘political ideology’ (59.1 average citations), ‘civic engagement’ (37.1 average citations), ‘Facebook’ (36 average citations), and ‘political communication’ (30.2 average citations), which, when taken collectively, connote the manifestation of an infodemic in political discourse.
Cluster/Theme 3 consists of 59 keywords/topics. The most occurring keywords/topics are ‘media’ (129 occurrences), ‘communication’ (124 occurrences), ‘trust’ (115 occurrences), ‘credibility’ (76 occurrences), and ‘risk communication’ (53 occurrences) while the most impactful keywords/topics are ‘ignorance’ (142.6 average citations), ‘myths’ (83.9 average citations), ‘learning’ (72.7 average citations), and ‘science literacy’ (42.1 average citations), which, when taken collectively, denote the manifestation of combating infodemic through literacy.
Cluster/Theme 4 contains 58 keywords/topics. The most occurring keywords/topics are ‘misinformation’ (1,418 occurrences), ‘false memory’ (93 occurrences), ‘memory’ (87 occurrences), ‘misinformation effect’ (87 occurrences), and ‘eyewitness memory’ (55 occurrences) while the most impactful keywords/topics are ‘misperceptions’ (130.9 average citations), ‘reasoning’ (112.2 average citations), ‘stress’ (99.1 average citations), ‘motivated reasoning’ (89.9 average citations), and ‘decision making’ (87.8 average citations), which, when taken collectively, espouse the manifestation of the psychology of infodemic.
Cluster/Theme 5 covers 50 keywords/topics. The most occurring keywords/topics are ‘Twitter’ (313 occurrences), ‘machine learning’ (189 occurrences), ‘deep learning’ (178 occurrences), ‘fake news detection’ (150 occurrences), and ‘natural language processing’ (131 occurrences) while the most impactful keywords/topics are ‘news verification’ (116.4 average citations), ‘deception detection’ (85.8 average citations), ‘ai’ (i.e. artificial intelligence—53.9 average citations), ‘svm’ (i.e. support vector machine—49.3 average citations), and ‘information credibility’ (37.9 average citations), which, when taken collectively, highlight the manifestation of combating infodemic with data analytics.
Cluster/Theme 6 encapsulates 27 keywords/topics. The most occurring keywords/topics are ‘content analysis’ (71 occurrences), ‘health information’ (65 occurrences), ‘covid-19 vaccine’ (36 occurrences), ‘health misinformation’ (33 occurrences), and ‘WhatsApp’ (31 occurrences) while the most impactful keywords/topics are ‘inoculation’ (19.6 average citations), ‘covid-19 vaccine’ (18.9 average citations), ‘echo chamber’ (17.6 average citations), ‘middle east’ (15.2 average citations), and ‘content analysis’ (15.1 average citations), which, when taken collectively, illustrate the manifestation of infodemic in health information.
Cluster/Theme 7 entails 20 keywords/topics. The most occurring keywords/topics are ‘infodemic’ (318 occurrences), ‘infodemiology’ (112 occurrences), ‘infoveillance’ (41 occurrences), ‘perception’ (30 occurrences), and ‘big data’ (28 occurrences) while the most impactful keywords/topics are ‘computational social science’ (44.1 average citations), ‘infoveillance’ (34.5 average citations), ‘data science’ (34.4 average citations), ‘collaboration’ (26.5 average citations), and ‘behavior’ (25.8 average citations), which, when taken collectively, imply the manifestation of infodemic modeling.
Cluster/Theme 8 includes of five keywords/topics, namely ‘network analysis’ (23 occurrences with 37 average citations), ‘conspiracy’ (17 occurrences with 29.4 average citations), ‘TikTok’ (13 occurrences with 15.5 average citations), ‘anti-vaccination’ (10 occurrences with 133.7 average citations), and ‘vaccinations’ (10 occurrences with 8.9 average citations), which, when taken collectively, indicate the manifestation of conspiracies in infodemic.
Cluster/Theme 9 is made up of two keywords/topics, namely ‘coronavirus’ (182 occurrences with 31 average citations) and ‘social distancing’ (10 occurrences with 22.7 average citations), which showcase the manifestation of infodemic in social practice discourse.
Cluster/Theme 10 involves two keywords/topics, namely ‘information sources’ (17 occurrences with 11.7 average citations) and ‘vaccine refusal’ (10 occurrences with 23.5 average citations), which signal the manifestation of information-shaping behavior in infodemic.
Cluster/Theme 11 is the smallest cluster with only one keyword/topic, namely ‘third-person effect’ (10 occurrences with 37.2 average citations), which suggest the manifestation of combating infodemic through third-person effect.
Last but not least, the temporal trajectory of infodemic research is promising. Specifically, research in the field has transitioned from understanding the psychology (e.g. confirmation bias, emotion, memory, reasoning) and know-how (e.g. education, knowledge) to exploring current issues (e.g. covid-19, deepfakes) and equivalent solutions (e.g. deepfake detection, machine learning) to combat infodemic.
Study 2
Goal
The study aims to provide finer-grained research-informed insights on the different aspects of infodemic (focus). To do so, this study adopts a differentiated approach where the scope of infodemic is taken separately (i.e. related concepts are taken independently rather than collectively). In doing so, this study contributes to a state-of-the-art overview of major trends on the different aspects of infodemic.
Method
The methods for corpus curation and analysis for the second study are similar to the first study, with the only differences situated in search keywords and results. In particular, five searches were conducted, with one search each for disinformation (or dis-information), misinformation (or mis-information), malinformation (or mal-information), deepfake, and fake news, returning 1,888 articles (disinformation), 7,059 articles (misinformation), 16 articles (malinformation), 233 articles (deepfake), and 2,647 articles (fake news), respectively.
Findings
Major contributors
Disinformation, misinformation, malinformation, deepfake, and fake news research have been contributed by numerous authors, institutions, countries, funders, and publishers. Disinformation, misinformation, and fake news research are mainly contributed by Western researchers (e.g. United States, United Kingdom, Europe), whereas deepfake research is primarily contributed by Eastern researchers (e.g. China, India), which seem to be coherent with the contributions by funders in the region. Most studies have been conducted on misinformation (7,059 articles), followed by fake news (2,647 articles), disinformation (1,888 articles), deepfake (233 articles), and malinformation (16 articles). The major clusters/themes of each information disorder and their manifestation in infodemic are presented in the next sections.
Disinformation
The topic model of disinformation research emerging from a co-occurrence analysis of 76 keywords/topics with a minimum of 10 occurrences revealed six major clusters/themes ().
Figure 3. Major clusters/themes in disinformation research.Normalization method: Association strength. Visualization scale: 1.00. Weights: Occurrences. Label size: 0.50. Line size: 0.50. Cluster/Theme 1 (Red): Disinformation in public health discourse (18 keywords/topics). Cluster/Theme 2 (Green): Disinformation in political discourse (15 keywords/topics). Cluster/Theme 3 (Blue): Combating disinformation with information literacy (13 keywords/topics). Cluster/Theme 4 (Yellow): Combating disinformation with data analytics (5 keywords/topics). Cluster/Theme 5 (Purple): Disinformation as a means for propaganda (13 keywords/topics). Cluster/Theme 6 (Light blue): Disinformation as an expression of free speech (12 keywords/topics).
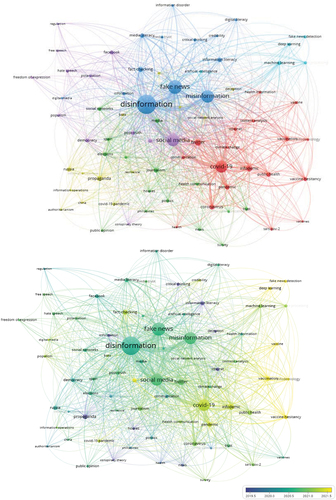
The first cluster/theme concentrates on disinformation in public health discourse involving 18 keywords/topics such as ‘covid-19’ (268 occurrences with 12.8 average citations), ‘public health’ (41 occurrences with 20.4 average citations), and ‘vaccination’ (30 occurrences with 12.7 average citations).
The second cluster/theme encapsulates disinformation in political discourse involving 15 keywords/topics such as ‘journalism’ (41 occurrences with 7.6 citations), ‘elections’ (30 occurrences with 4.8 citations), and ‘political communication’ (17 occurrences with 34.1 citations).
The third cluster/theme entails combating disinformation through literacy involving 13 keywords/topics such as ‘disinformation’ (663 occurrences with 11.2 average citations), ‘fake news’ (351 occurrences with 16 average citations), ‘fact-checking’ (49 occurrences with 12.5 average citations), ‘media literacy’ (37 occurrences with 16.8 average citations), ‘information literacy’ (35 occurrences with 13.4 average citations), and ‘digital literacy’ (15 occurrences with 17.6 average citations). This solution also appears to extend to ‘misinformation’ (351 occurrences with 16 average citations).
The fourth cluster/theme is about disinformation as a means for propaganda involving 13 keywords/topics such as ‘propaganda’ (51 occurrences with 11.9 average citations), ‘bots’ (12 occurrences with 7.2 average citations), and ‘information warfare’ (11 occurrences with 5.6 average citations) among countries such as ‘Russia’ (37 occurrences with nine average citations) and ‘China’ (11 occurrences with 5.5 average citations).
The fifth cluster/theme pertains to disinformation as an expression of free speech involving 12 keywords/topics such as ‘democracy’ (33 occurrences with 4.8 average citations), ‘freedom of expression’ (13 occurrences with 2.8 average citations), and ‘conspiracy theory’ (11 occurrences with 17.9 average citations), which tends to occur over ‘social media’ (279 occurrences with 14.5 average citations).
The sixth cluster/theme relates to combating disinformation with data analytics involving five keywords/topics, namely ‘fake news detection’ (21 occurrences with 12.8 average citations) via ‘artificial intelligence’ (16 occurrences with 11.9 average citations), ‘deep learning’ (21 occurrences with 18.7 average citations), ‘machine learning’ (32 occurrences with 12 average citations), and ‘natural language processing’ (19 occurrences with 7.6 average citations).
Last but not least, the temporal trajectory of disinformation research is fairly recent, ranging from the manifestation of disinformation (e.g. conspiracy theory, propaganda) to current issues (e.g. ‘vaccination’, ‘vaccine hesitancy’) and solutions to detect disinformation (e.g. ‘deep learning’, ‘fake news detection’).
Misinformation
The topic model of misinformation research emerging from a co-occurrence analysis of 194 keywords/topics with a minimum of 10 occurrences revealed 10 major clusters/themes ().
Figure 4. Major clusters/themes in misinformation research.Normalization method: Association strength. Visualization scale: 1.00. Weights: Occurrences. Label size: 0.50. Line size: 0.50. Cluster/Theme 1 (Red): Misinformation in public health discourse (100 keywords/topics). Cluster/Theme 2 (Green): Combating misinformation through literacy (62 keywords/topics). Cluster/Theme 3 (Blue): Psychology of misinformation (34 keywords/topics). Cluster/Theme 4 (Yellow): Combating misinformation with data analytics (30 keywords/topics). Cluster/Theme 5 (Purple): Misinformation in health information (29 keywords/topics). Cluster/Theme 6 (Light blue): Combating misinformation over social media (21 keywords/topics). Cluster/Theme 7 (Orange): Misinformation discourse on social media (9 keywords/topics). Cluster/Theme 8 (Brown): Misinformation diffusion over social networks (7 keywords/topics). Cluster/Theme 9 (Pink): Emotions as a driver of misinformation (1 keyword/topic). Cluster/Theme 10 (Light pink): Information technology as a driver of misinformation (1 keyword/topic).
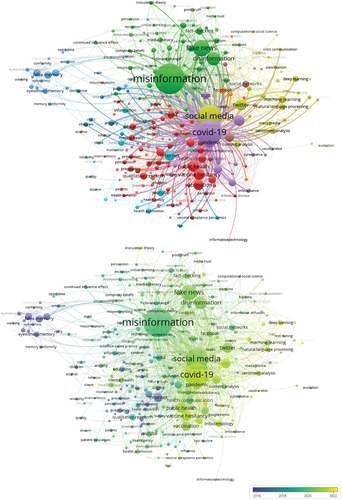
The first cluster/theme concentrates on misinformation in public health discourse involving 100 keywords/topics such as ‘health communication’ (85 occurrences with 29.7 average citations) around ‘public health’ (168 occurrences with 30 average citations) and ‘vaccination hesitancy’ (169 occurrences with 22.2 average citations).
The second cluster/theme focuses on combating misinformation through literacy involving 62 keywords/topics such as ‘digital literacy’ (25 occurrences with 19.2 average citations), ‘information literacy’ (46 occurrences with 14.5 average citations), ‘media literacy’ (48 occurrences with 15.8 average citations), and ‘news literacy’ (11 occurrences with 28 average citations) for ‘fact-checking’ (71 occurrences with 31.9 average citations) in combating ‘misinformation’ (1,418 occurrences with 22.4 average citations) circulated as ‘fake news’ (415 occurrences with 26.2 average citations). This solution also appears to extend to ‘disinformation’ (262 occurrences with 15.3 average citations).
The third cluster/theme encapsulates the psychology of misinformation involving 34 keywords/topics such as ‘confirmation bias’ (13 occurrences with 96.1 average citations), ‘false memory’ (91 occurrences with 20 average citations), and ‘eyewitness memory’ (55 occurrences with 18.9 average citations) in understanding ‘misinformation effect’ (87 occurrences with 14.3 average citations).
The fourth cluster/theme entails combating misinformation with data analytics involving 30 keywords/topics such as ‘artificial intelligence’ (26 occurrences with 12.8 average citations), ‘content analysis’ (45 occurrences with 12.4 average citations), ‘deep learning’ (48 occurrences with 8.8 average citations), ‘machine learning’ (72 occurrences with 14.5 average citations), ‘natural language processing’ (52 occurrences with 9.7 average citations), and ‘sentiment analysis’ (52 occurrences with 14.6 average citations) for ‘fake news detection’ (30 occurrences with 21.2 average citations) and ‘misinformation detection’ (16 occurrences with 10 average citations) over ‘social media’ (799 occurrences with 19.2 average citations) such as ‘Twitter’ (188 occurrences with 17.7 average citations).
The fifth cluster/theme includes misinformation in health information involving 29 keywords/topics such as ‘covid-19’ (963 occurrences with 18.1 average citations), ‘infodemic’ (160 occurrences with 13.6 average citations), ‘pandemic’ (148 occurrences with 18.4 average citations), ‘information sharing’ (15 occurrences with 5.1 average citations), ‘information overload’ (13 occurrences with 24.5 average citations), ‘online misinformation’ (13 occurrences with 11.3 average citations), ‘echo chambers’ (13 occurrences with 11 average citations), ‘ehealth literacy’ (11 occurrences with 17.2 average citations), and ‘covid-19 misinformation’ (10 occurrences with 4.1 average citations).
The sixth cluster/theme involves combating misinformation over social media revolving around 21 keywords/topics such as ‘health education’ (14 occurrences with 7.4 average citations), ‘health information’ (52 occurrences with 8.3 average citations), ‘health literacy’ (45 occurrences with 11.8 average citations), ‘health promotion’ (37 occurrences with 8.7 average citations), and ‘patient education’ (25 occurrences with 10.8 average citations) over the ‘internet’ (136 occurrences with 29.4 average citations) and ‘social network’ (25 occurrences with 21.3 average citations”) such as ‘Instagram’ (23 occurrences with 13.3 average citations) and ‘YouTube’ (64 occurrences with 16.1 average citations”).
The seventh cluster/theme pertains to misinformation discourse on social media involving nine keywords/topics such as ‘hate speech’ (15 occurrences with 4.7 average citations) and ‘rumors’ (14 occurrences with 13.6 average citations) over social media such as ‘Facebook’ (67 occurrences with 42 average citations) and ‘WhatsApp’ (24 occurrences with 11 average citations).
The eight cluster/theme relates to misinformation diffusion through social networks involving seven keywords/topics such as understanding ‘information diffusion’ (15 occurrences with 9.9 average citations) on issues such as ‘coronavirus’ (115 occurrences with 24.5 average citations) over ‘social networks’ (65 occurrences with 21.5 average citations) using techniques such as ‘social network analysis’ (23 occurrences with 22.2 average citations).
The ninth and tenth cluster/theme revolves around ‘emotions’ (10 occurrences with 15.9 average citations) and ‘information technology’ (11 occurrences with 32.2 average citations) as drivers of misinformation.
Last but not last, the temporal trajectory of misinformation research threads along the same lines as infodemic research, wherein the focus has shifted from understanding the psychology of misinformation (e.g. false memory, eyewitness memory, memory conformity) to current issues (e.g. ‘covid-19’, ‘vaccine hesitancy’) and solutions to detect misinformation (e.g. ‘deep learning’, ‘fake news detection’).
Malinformation
The topic model of malinformation research emerging from a co-occurrence analysis of eight keywords/topics with a minimum of two occurrences revealed three major clusters/themes ().Footnote12
Figure 5. Major clusters/themes in malinformation research.Normalization method: Association strength. Visualization scale: 1.00. Weights: Occurrences. Label size: 0.50. Line size: 0.50. Cluster/Theme 1 (Red): Malinformation as an information disorder (4 keywords/topics). Cluster/Theme 2 (Green): Malinformation as an infodemic (3 keywords/topics). Cluster/Theme 3 (Blue): Malinformation on social media (1 keyword/topic).
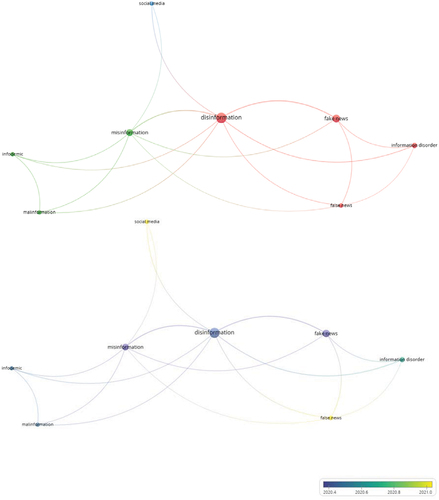
The first cluster/theme encapsulates malinformation as an information disorder involving four keywords/topics, wherein malinformation is an ‘information disorder’ (three occurrences with 1.7 average citations) just like ‘disinformation’ (11 occurrences with 6.5 average citations), ‘fake news’ (six occurrences with 11.7 average citations), and ‘false news’ (two occurrences with eight average citations).
The second cluster/theme entails malinformation as an infodemic involving three keywords/topics, wherein ‘malinformation’ (two occurrences with 3.5 average citations) is an ‘infodemic’ (three occurrences with 3.5 average citations) just like ‘misinformation’ (five occurrences with 11.6 average citations).
The third cluster/theme is dedicated to malinformation on social media involving one keyword/topic, that is, ‘social media’ (two occurrences with 2.5 average citations).
Last but not least, the temporal trajectory of malinformation research suggests that research in the field is fairly new, conceptualizing the differences between malinformation and other forms of information disorders in infodemic and moving into the realm of social media.
Deepfake
The topic model of deepfake research emerging from a co-occurrence analysis of 22 keywords/topics with a minimum of five occurrences revealed six major clusters/themes ().Footnote13
Figure 6. Major clusters/themes in deepfake research.Normalization method: Association strength. Visualization scale: 1.00. Weights: Occurrences. Label size: 0.50. Line size: 0.50. Cluster/Theme 1 (Red): Deepfake detection through generative adversarial networks (6 keywords/topics). Cluster/Theme 2 (Green): Face manipulation in deepfake (5 keywords/topics). Cluster/Theme 3 (Blue): Deepfake as an infodemic (4 keywords/topics). Cluster/Theme 4 (Yellow): Deepfake detection through computer vision (4 keywords/topics). Cluster/Theme 5 (Purple): Combating deepfake detection through data analytics (2 keywords/topics). Cluster/Theme 6 (Light blue): Cybersecurity threat of deepfake (1 keyword/topic).
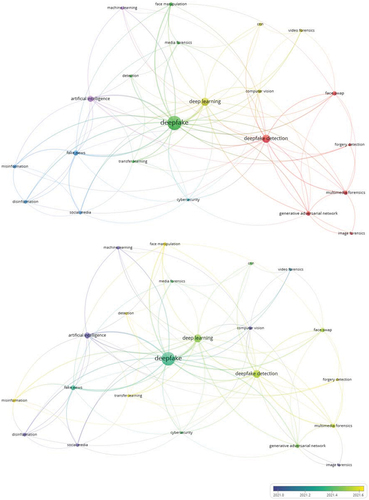
The first cluster/theme concentrates on deepfake detection through generative adversarial networks involving six keywords/topics, namely ‘deepfake detection’ (39 occurrences with 4.7 average citations) of ‘face swap’ (12 occurrences with 2.9 average citations) using ‘generative adversarial network’ (11 occurrences with 8.6 average citations) as part of ‘multimedia forensics’ (11 occurrences with 7.5 average citations), ‘image forensics’ (five occurrences with nine average citations), and ‘forgery detection’ (five occurrences with 3.2 average citations).
The second cluster/theme focuses on face manipulation in deepfake involving five keywords/topics, namely ‘deepfake’ (116 occurrences with 8.7 average citations) ‘detection’ (five occurrences with 1.8 citations) and ‘transfer learning’ (five occurrences with 3.6 average citations) for ‘face manipulation’ (nine occurrences with 23.8 average citations) and ‘media forensics’ (five occurrences with 42 average citations).
The third cluster/theme encapsulates deepfake as an infodemic involving four keywords/topics, which include deepfake manifestation as ‘disinformation’ (eight occurrences with 9.4 average citations) and ‘misinformation’ (eight occurrences with 5.1 average citations) of ‘fake news’ (16 occurrences with 17.2 average citations) on ‘social media’ (seven occurrences with 13 average citations).
The fourth cluster/theme entails deepfake detection through computer vision involving four keywords/topics, namely ‘cnn’ (i.e. convolutional neural network-seven occurrences with 8.1 average citation) and ‘deep learning’ (38 occurrences with 5.3 average citations) in ‘computer vision’ (eight occurrences with 1.8 average citations) for ‘video forensics’ (six occurrences with 7.7 average citations.
The fifth cluster/theme pertains to deepfake detection through data analytics involving two keywords/topics, namely ‘artificial intelligence’ (22 occurrences with 12.1 average citations) and ‘machine learning’ (seven occurrences with 12.9 average citations), whereas the sixth cluster/theme relates to cybersecurity (five occurrences with 2.4 average citations) threat of deepfake (one keyword/topic).
Last but not least, the temporal analysis of deepfake research reveals that its research is fairly recent with an average publication year of 2021.
Fake news
The topic model of fake news research emerging from a co-occurrence analysis of 111 keywords/topics with a minimum of 10 occurrences revealed six major clusters/themes ().
Figure 7. Major clusters/themes in fake news research.Normalization method: Association strength. Visualization scale: 1.00. Weights: Occurrences. Label size: 0.50. Line size: 0.50. Cluster/Theme 1 (Red): Fake news in political discourse (25 keywords/topics). Cluster/Theme 2 (Green): Combating fake news with data analytics (21 keywords/topics). Cluster/Theme 3 (Blue): Fake news discourse on social media (19 keywords/topics). Cluster/Theme 4 (Yellow): Fake news as an infodemic (19 keywords/topics). Cluster/Theme 5 (Purple): Fake news in public health discourse (17 keywords/topics). Cluster/Theme 6 (Light blue): Combating fake news through literacy (10 keywords/topics).
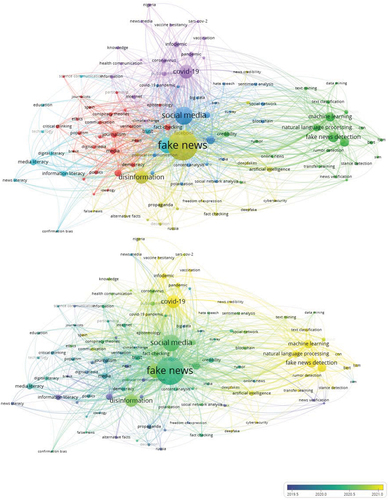
The first cluster/theme concentrates on fake news in political discourse involving 25 keywords/topics such as ‘post-truth’ (68 occurrences with 15 average citations), ‘populism’ (25 occurrences with 23.5 average citations), ‘politics’ (25 occurrences with 16.8 average citations), and ‘conspiracy theories’ (23 occurrences with 8.1 average citations) across ‘digital media’ (20 occurrences with 11 average citations) and ‘journalism’ (60 occurrences with 21.6 average citations) on topics such as ‘Brexit’ (11 occurrences with 21.3 average citations) and ‘Trump’ (15 occurrences with 13.3 average citations).
The second cluster/theme focuses on combating fake news with data analytics involving 21 keywords/topics such as ‘fake news detection’ (150 occurrences with 19.2 average citations) and ‘rumor detection’ (13 occurrences with 22.8 average citations) through ‘deep learning’ (121 occurrences and 13.7 average citations), ‘machine learning’ (120 occurrences with 11.4 average citations), and ‘natural language processing’ (78 occurrences with 13.7 average citations) techniques such as ‘bert’ (i.e. bidirectional encoder representations from transformers—19 occurrences with 11.8 average citations), ‘cnn’ (i.e. convolutional neural network—12 occurrences with 11.3 average citations), ‘lstm’ (i.e. long short-term memory—15 occurrences with 6.5 average citations), and ‘svm’ (i.e. support vector machine—10 occurrences with 49.3 average citations).
The third cluster/theme encapsulates fake news discourse on social media involving 19 keywords/topics such as ‘covid-19 pandemic’ (14 occurrences with 6.3 average citations), ‘elections’ (23 occurrences with 13.8 average citations), ‘polarization’ (18 occurrences with 21.1 average citations), and ‘hoaxes’ (13 occurrences with 19.5 average citations) on ‘social media’ (465 occurrences with 24.7 average citations) and ‘social networks’ (55 occurrences with 10.7 average citations).
The fourth cluster/theme entails fake news as an infodemic involving 19 keywords/topics such as ‘fake news’ (1,274 occurrences with 19 average citations) and ‘false information’ (21 occurrences with 21.6 average citations) manifesting as ‘deception’ (13 occurrences with nine average citations), ‘deepfakes’ (12 occurrences with 22 average citations), ‘disinformation’ (252 occurrences with 15 average citations), and ‘propaganda’ (35 occurrences with 15.5 average citations).
The fifth cluster/theme pertains to fake news in public health discourse involving 17 keywords/topics such as ‘infodemic’ (51 occurrences with 24.6 average citations) on topics around ‘covid-19’ (273 occurrences with 19 average citations), ‘public health’ (27 occurrences with 45.9 average citations), and ‘vaccination’ (19 occurrences with 12.2 average citations).
The sixth cluster/theme relates to combating fake news through literacy involving 10 keywords/topics such as ‘digital literacy’ (23 occurrences with 13.3 average citations), ‘information literacy’ (50 occurrences with 14.9 average citations), ‘media literacy’ (65 occurrences with 18.6 average citations), and ‘news literacy’ (17 occurrences with 17.6 average citations) to fight ‘misinformation’ (333 occurrences with 28 average citations) arising from fake news.
Last but not least, the temporal analysis of fake news research reveals that its research is fairly recent with an average publication year of between 2019 and 2021.
Study 3
Goal
Building on the two previous studies, this study aims to explore the solutions to infodemic (focus). To do so, this study adopts an undifferentiated approach where the scope of infodemic is taken in its entirety (i.e. related concepts are taken collectively rather than independently). In doing so, this study contributes to a state-of-the-art overview of major trends in infodemic solutions.
Method
The methods for corpus curation and analysis for the third study are similar to the first study, with the only differences situated in search keywords and results. More specifically, the same keywords in the first study are employed in this study alongside two additional keywords – i.e. ‘detection’ OR ‘solution’ – returning a total of 1,347 articles.
Findings
Major contributors
Infodemic solutions research has many contributors. The prolific authors include Victoria L. Rubin (eight articles), Jeremy Straub (seven articles), and Matthew Spradling (six articles); their most impactful contributions revolve around the detection of fake news, including automatic deception detection involving linguistic cues and network analysis approaches (Conroy et al., Citation2015) (≥400 citations) and descriptive factual labeling for online content to protect consumers against fake news (Spradling et al., Citation2021) (≥10 citations). The prolific institutions (Chinese Academy of Sciences and Wuhan University—15 articles each; Beijing University of Posts and Telecommunications and Delhi Technological University—12 articles each; and Consiglio Nazionale delle Ricerche, Massachusetts Institute of Technology, Ministry of Education China, and University of Oxford—11 articles each) and countries (United States—329 articles; India—183 articles; and China—172 articles) are fairly consistent, wherein most of the former are located in the latter. Prolific funders include the National Science Foundation of China (82 articles), National Science Foundation (40 articles), and National Research Foundation of Korea (24 articles), whereas prolific publishers include IEEE Access (52 articles), International Journal of Advanced Computer Science and Applications (24 articles), and Expert Systems with Applications (22 articles); they represent where infodemic solutions research can be funded and published.
Major themes
The topic model of infodemic solutions research emerging from a co-occurrence analysis of 47 keywords/topics with a minimum of 10 occurrences revealed four major clusters/themes ().
Figure 8. Major clusters/themes in infodemic solutions research.Normalization method: Association strength. Visualization scale: 1.00. Weights: Occurrences. Label size: 0.50. Line size: 0.50. Cluster/Theme 1 (Red): Textual analytics as an infodemic solution (16 keywords/topics). Cluster/Theme 2 (Green): Data analytics as an infodemic solution (12 keywords/topics). Cluster/Theme 3 (Blue): Social media analytics as an infodemic solution (10 keywords/topics). Cluster/Theme 4 (Yellow): Infodemic issues driving infodemic solutions (9 keywords/topics).
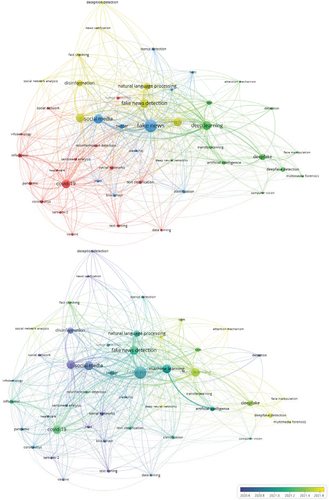
The first cluster/theme concentrates on textual analytics as an infodemic solution involving 16 keywords/topics such as ‘data mining’ (13 occurrences with 23.2 average citations), ‘text classification’ (22 occurrences with 14.4 average citations), ‘text mining’ (18 occurrences with 26.2 average citations), and ‘sentiment analysis’ (16 occurrences with 6.2 average citations) for detecting ‘infodemic’ (98 occurrences with 12.9 average citations) such as ‘misinformation detection’ (16 occurrences with 10 average citations) and ‘rumor detection’ (18 occurrences with 17.7 average citations) on topics such as ‘covid-19’ (137 occurrences with 15.5 average citations) and ‘vaccine’ (12 occurrences with 12 average citations).
The second cluster/theme focuses on data analytics as an infodemic solution involving 12 keywords/topics such as ‘artificial intelligence’ (31 occurrences with 11.2 average citations), ‘computer vision’ (11 occurrences with 13 average citations), ‘cnn’ (i.e. convolutional neural network—39 occurrences with 9.2 average citations), ‘deep learning’ (153 occurrences with 11.4 average citations), and ‘transfer learning’ (16 occurrences with 4.6 average citations) for understanding ‘attention mechanism’ (11 occurrences with 6.3 average citations), ‘deepfake detection’ (39 occurrences with 4.7 average citations), and ‘multimedia forensics’ (12 occurrences with seven average citations).Footnote14
The third cluster/theme encapsulates social media analytics as an infodemic solution involving 10 keywords/topics such as ‘bert’ (i.e. bidirectional encoder representations from transformers—19 occurrences with 11.1 average citations) and ‘lstm’ (i.e. long short-term memory—16 occurrences with 6.8 average citations) for ‘credibility’ (10 occurrences with 3.7 average citations) and ‘stance detection’ (15 occurrences with 11.7 average citations) to filter out ‘fake news’ (292 occurrences with 19.1 average citations) on ‘social media’ (156 occurrences with 25.2 average citations) such as ‘Twitter’ (49 occurrences with 18.1 average citations).
The fourth cluster/theme entails infodemic issues driving infodemic solutions involving nine keywords/topics relating to the need for ‘deception detection’ (14 occurrences with 85.8 average citations), ‘fake news detection’ (150 occurrences with 19.2 average citations), ‘fact checking’ (23 occurrences with 19.5 average citations), and ‘news verification’ (10 occurrences with 135.4 average citations) to address issues such as ‘disinformation’ (77 occurrences with 18 average citations) and ‘misinformation’ (143 occurrences with 20.7 average citations) using techniques such as ‘machine learning’ (127 occurrences with 12.8 average citations), ‘natural language processing’ (81 occurrences with 14.1 average citations), and ‘social network analysis’ (11 occurrences with 3.4 average citations).Footnote15
Last but not least, the temporal analysis of infodemic solutions research reveals that its research is fairly recent with an average publication year of between 2020 and 2021.
Conclusion
The world has transitioned into a state that is popularly known as the new normal following the COVID-19 pandemic and endemic, entailing a digital era where creation and dissemination of information occur at an exponential pace with a wide reach due to large-scale digital transformation and mass technology adoption, enabling the world to remain highly connected and progress steadily regardless of great crises or mega-disruptions (e.g. lockdowns or movement restrictions to curb pandemics or maintain social order) and new-age social practices (e.g. social or physical distancing). Notwithstanding the bright side of information (knowledge) sharing and management (e.g. promises and returns such as improved performance) (Donthu et al., Citation2023; Hosen et al., Citation2023), it is important to recognize and follow up on its dark side as well. In this regard, the present article has addressed and responded to the latter by exploring the major trends (themes) relating to the infodemic of disinformation, misinformation, and malinformation in the digital era of a new normal where deepfake and fake news are omnipresent. Using a multi-study approach with big data analytics via scientometrics analysis with topic modeling, this article has delivered nuanced insights into the state of the art of infodemic research – both its issues and solutions. The key takeaways, and by extension, the theoretical and managerial implications as well as future research directions, from this multi-study article are manifold, with the three major ones presented as follows.
First, the article discovered 10,406 journal articles on infodemic research written in English that were published up to 2022, encompassing aspects of misinformation (7,059 articles), fake news (2,647 articles), disinformation (1,888 articles), infodemic solutions (1,347 articles), deepfake (233 articles), and malinformation (16 articles) (first takeaway). Noteworthily, deepfake is a subset of fake news, which, in turn, could (i) be a representation of disinformation (untrue) or misinformation (untrue) but not malinformation (true) due to the nature of truth, and (ii) explain why studies on the former (deepfake) is smaller than the latter (fake news) (first theoretical implication). When this understanding is extrapolated to practice, it can be deduced that (i) not all information disorders are the same and (ii) infodemic solutions should be tailored to the information disorder that is intended to be addressed (first managerial implication). Hence, future research is encouraged to engage in causal explorations that establish the effectiveness of infodemic solutions against the different variants of information disorders (first future research direction).
Second, this article found that infodemic research on misinformation (7,059 articles) more than triples the magnitude of that on disinformation (1,888 articles) while interest in malinformation (16 articles) is lowest among the three forms of information disorder (second takeaway). This implies that (i) interest in the dissemination (e.g. misinformation) appears to be greater than the creation (e.g. disinformation) of false information and (ii) intrinsic elements of information disorder (e.g. maliciousness in disinformation and malinformation) seem to be more challenging to ascertain than its extrinsic elements (e.g. truth of misinformation) (second theoretical implication). By extension, this signals that consumers of information may be more susceptible to disinformation and malinformation as opposed to misinformation due to (i) a lower understanding of as well as (ii) the difficulty in ascertaining these forms of information disorder than misinformation (second managerial implication). Therefore, future research, both from theoretical (i.e. what and why) and practical (i.e. how) lenses, should explore what motivates the manifestation of the intrinsic elements of information disorder and why, and how they could be detected and mitigated in practice (second future research direction).
Third, this article mapped and synthesized the research-informed insights on infodemic, revealing four major programs/themes relating to (i) the content (e.g. infodemic discourse relating to conspiracies, free speech, health information, public health, politics, propaganda, and social practices), (ii) the platform (e.g. social media and networks as avenues allowing infodemic to manifest), and (iii) the manifestation (e.g. drivers and issues involving emotions, psychology, and information technology that induce and promote the manifestation of the different types of information disorders as well as the modeling of such manifestations) of infodemic, as well as (iv) the solution (e.g. computational or data science methods involving artificial learning, machine learning, and deep learning with bidirectional encoder representations from transformers, computer vision, convolutional neural network, generative adversarial network, long short-term memory, natural language processing, support vector machine, and transfer learning, as well as digital, information, media, and news literacy involving social media and third-person effect) to detect (e.g. forensics, forgery) and mitigate (e.g. fact checking, labeling) infodemic (e.g. pandemic of information disorders such as disinformation and misinformation involving deepfake and fake news as well as malinformation) and its threats (e.g. cybersecurity, deception, hoax) – as discussed across the three studies and summarized in (third takeaway). The nomological network derived through the mapping activity and its resulting synthesis offer theoretical clarity on the major programs/themes in the body of knowledge on infodemic (third theoretical implication) while the solutions uncovered converge into a useful toolbox for combating infodemic in practice (third managerial implication). Yet, future research remains necessary, especially with regards to infodemic mitigating mechanisms, which mainly requires proactive actions (e.g. fact checking), thereby leaving ample room for the development of alternative solutions (e.g. automated alerts, labeling options) that shift the onus of proactiveness from people to machines in preventing consumers of information from falling victim in the infodemic of disinformation, misinformation, and malinformation where deepfake and fake news are rampant (third future research direction).
Table 3. Mapping of major themes in infodemic research.
From a marketing lens, the findings of this study bear significant implications for marketing scholars, practitioners, and policymakers.
For marketing scholars, the study offers a clearer roadmap of the current landscape of infodemic research, as it points out fertile grounds for exploration, such as the understanding and motivations behind the dissemination of various forms of information disorders. This paves the way for causal explorations that lead to more targeted and effective marketing communication strategies, even in an environment fraught with disinformation.
For marketing practitioners, understanding the detailed taxonomy and characteristics of different information disorders is paramount. This knowledge facilitates the development of nuanced and tailored marketing strategies that effectively cut through the infodemic noise. In an era where information disorders are prevalent, maintaining the integrity and credibility of marketing messages is crucial. This knowledge enables brands to strategically navigate and communicate in the digital and social landscape while preserving, or even enhancing, brand trust.
For policymakers with marketing communication and social marketing responsibilities, the study sheds light on potential avenues for proactive measures to combat infodemics. Highlighting the efficacy of solutions such as computational or data science methods informs policy decisions, underscoring the need for regulations that foster transparent and authentic marketing communication. Moreover, the call for ongoing research into automated detection and mitigation strategies illuminates potential policy directions for a more regulated digital marketing environment, one where the spread of false information is kept in check.
Notwithstanding the contributions of this article and its multiple studies (i.e. key takeaways, theoretical and managerial implications, and future research directions), there are several limitations that need to be acknowledged, which, in turn, pave the way forward for alternative reviews in the future. First, this article offers only a high-level overview of infodemic research, which implies that promising opportunities are available for future reviews to provide more specific details about infodemic research, for example, a list of key publications in the field as a whole and across programs/themes using appropriate methods such as bibliographic coupling and co-citation analysis. Second, this article is focused on mapping the nomological network of themes and topics in infodemic research, which signals that much room is available for future reviews to explore the productivity and impact drivers as well as trends over time for infodemic research using relevant methods such as negative binomial regression and temporal analysis. Taken collectively, this article should be useful for academics, consumers, professionals, and policymakers to gain a state-of-the-art understanding of current and future insights on infodemic and its equivalent solutions from both theoretical and managerial standpoints, as well as the potentially fruitful avenues to fertilize and navigate the field in the future.
Special issue
Fact or Fake: Information, Misinformation and Disinformation.
Disclosure statement
No potential conflict of interest was reported by the author(s).
Data availability statement
Data request can be made to the author.
Notes
1. The parsimonious definition of information developed herein aligns with established definitions. For instance, Losee (Citation1997, p. 254) defined information as ‘the characteristics of the output of a process, these being informative about the process and the input’. Similarly, Madden (Citation2000, p. 348) defined information as ‘a stimulus originating in one system that affects the interpretation by another system of either the second system’s relationship to the first or of the relationship the two systems share with a given environment’. The definitions of the remaining concepts in this study have also been reimagined for enhanced clarity, drawing upon the author’s 3Es of exposure, experience, and expertise (Kraus et al., Citation2022).
2. In essence, a scientometrics (bibliometrics) analysis involves performance analysis (i.e. assessment of performance) and science mapping (i.e. unpacking and mapping the topics and themes) of the field (see Donthu et al., Citation2021), wherein the interpretation of results is guided by sensemaking (i.e. scanning, sensing, and substantiating) (see Lim & Kumar, Citation2023). For a detailed explanation on scientometrics (or bibliometrics) analysis as a manifestation of big data analytics, see Kumar et al. (Citation2023).
3. This study opts for topic modeling to decipher the current knowledge in the field because its units of analysis and reporting are based on keywords, making it more parsimonious than alternatives such as bibliographic coupling, which relies on articles (Donthu et al., Citation2021). The choice to conduct topic modeling using a co-occurrence analysis of keywords is guided by pragmatic considerations. For example, author keywords, which reflect the essence of articles (i.e. topics—e.g. concepts, contexts, methods, theories), are readily available (which is not always the case; Donthu et al., Citation2022). This approach objectively captures semantic and contextual relations based on pairwise relationships, and is easily understandable and interpretable, even for readers who may not be familiar with more advanced topic modeling techniques such as natural language processing (Donthu et al., Citation2022) and structured topic modeling (Kraus et al., Citation2023). The analysis is performed using the VOSviewer software (Van Eck & Waltman, Citation2010) rather than its alternatives, such as the bibliometrix package in the R software (Aria & Cuccurullo, Citation2017), due to its user-friendly nature – evidenced by its flexibility, simplicity, and responsiveness to user demands – as well as its superior graphic quality crucial for topic modeling (Arruda et al., Citation2022).
4. Limiting the search to include only documents where the publication stage is ‘final’ (as opposed to ‘in press’) enables (impedes) the replicability, and by extension, the trustworthiness, of the study.
5. Limiting the document type to ‘article’ (as opposed to including others such as ‘note’ and ‘review’), which typically undergoes full peer-review scrutiny, mitigates potential issues involving quality assurance (e.g. absence of peer review in ‘erratum’ and ‘note’) and replication (as in the case of ‘review’).
6. Limiting the language type to English is consistent with the language proficiency of the author.
7. Limiting the source type to ‘journal’ promotes inclusion of high-quality insights (e.g. exploratory rather than explanatory; multiple rounds of peer review), which may not be present in other source types such as ‘book’ and ‘conference proceeding’.
8. The search keywords were curated based on the general theory of information explained in the introduction and illustrated in .
9. Concepts, as espoused by keywords, are assumed to take center stage in research when they are mentioned in the article title, abstract, or keywords for that research.
10. The search period was limited to up to 2022 as this was the latest full year at the time of study.
11. Scopus was chosen for the pragmatic reason explained in the introduction (i.e. complete download of bibliographical records as opposed to limited download in alternatives such as Web of Science).
12. The keyword occurrence is set to the minimum of two occurrences due to the small number of articles in the corpus related to malinformation research.
13. The keyword occurrence is set to the minimum of five occurrences due to the small number of articles in the corpus related to deepfake research. ‘deepfake’ and ‘deepfakes’ were combined to the former. ‘face swap’ and ‘face swapping’ were combined to the former. ‘generative adversarial network’ and ‘generative adversarial networks’ were combined to the former.
14. ‘cnn’, ‘convolutional neural network’, and ‘convolutional neural networks’ were combined to the former. ‘deepfake’ and ‘deepfakes’ were combined to the former.
15. ‘fact checking’ and ‘fact-checking’ were combined to the former.
References
- Akram, M., Nasar, A., & Arshad Ayaz, A. (2022). A bibliometric analysis of disinformation through social media. Online Journal of Communication and Media Technologies, 12(4), e202242. https://doi.org/10.30935/ojcmt/12545
- Ansar, W., & Goswami, S. (2021). Combating the menace: A survey on characterization and detection of fake news from a data science perspective. International Journal of Information Management Data Insights, 1(2), 100052. https://doi.org/10.1016/j.jjimei.2021.100052
- Aria, M., & Cuccurullo, C. (2017). Bibliometrix: An R-tool for comprehensive science mapping analysis. Journal of Informetrics, 11(4), 959–975. https://doi.org/10.1016/j.joi.2017.08.007
- Arruda, H., Silva, E. R., Lessa, M., Proença, Jr D, and Bartholo, R. (2022). VOSviewer and bibliometrix. Journal of the Medical Library Association: Journal of the Medical Library Association, 110(3), 392–395. https://doi.org/10.5195/jmla.2022.1434
- Bran, R., Tiru, L., Grosseck, G., Holotescu, C., & Malita, L. (2021). Learning from each other—a bibliometric review of research on information disorders. Sustainability, 13(18), 10094. https://doi.org/10.3390/su131810094
- Ciasullo, M. V., & Lim, W. M. (2022). Digital transformation and business model innovation: Advances, challenges and opportunities. International Journal of Quality and Innovation, 6(1), 1–5.
- Conroy, N. K., Rubin, V. L., & Chen, Y. (2015). Automatic deception detection: Methods for finding fake news. Proceedings of the Association for Information Science and Technology, 52(1), 1–4. https://doi.org/10.1002/pra2.2015.145052010082
- Di Domenico, G., Sit, J., Ishizaka, A., & Nunan, D. (2021). Fake news, social media and marketing: A systematic review. Journal of Business Research, 124, 329–341. https://doi.org/10.1016/j.jbusres.2020.11.037
- Donthu, N., Kumar, S., Mukherjee, D., Pandey, N., & Lim, W. M. (2021). How to conduct a bibliometric analysis: An overview and guidelines. Journal of Business Research, 133, 285–296. https://doi.org/10.1016/j.jbusres.2021.04.070
- Donthu, N., Kumar, S., Sureka, R., Lim, W. M., & Pereira, V. Foundations of knowledge management: Intellectual structure and citation drivers of the Journal of Knowledge Management. (2023). Journal of Knowledge Management, 27(4), 953–974. Ahead of print. https://doi.org/10.1108/JKM-02-2022-0094
- Donthu, N., Lim, W. M., Kumar, S., & Pattnaik, D. (2022). The Journal of Advertising’s production and dissemination of advertising knowledge: A 50th anniversary commemorative review. Journal of Advertising, 51(2), 153–187. https://doi.org/10.1080/00913367.2021.2006100
- Hosen, M., Ogbeibu, S., Lim, W. M., Ferraris, A., Munim, Z. H., & Chong, Y. L. Knowledge sharing behavior among academics: Insights from theory of planned behavior, perceived trust and organizational climate. (2023). Journal of Knowledge Management, 27(6), 1740–1764. Ahead of print. https://doi.org/10.1108/JKM-02-2022-0140
- Jain, K., Sharma, I., & Behl, A. (2023). Voice of the stars – exploring the outcomes of online celebrity activism. Journal of Strategic Marketing. https://doi.org/10.1080/0965254X.2021.2006275
- Kapantai, E., Christopoulou, A., Berberidis, C., & Peristeras, V. (2021). A systematic literature review on disinformation: Toward a unified taxonomical framework. New Media & Society, 23(5), 1301–1326. https://doi.org/10.1177/1461444820959296
- Kraus, S., Breier, M., Lim, W. M., Dabić, M., Kumar, S., Kanbach, D., Mukherjee, D., Corvello, V., Piñeiro-Chousa, J., Liguori, E., Fernandes, C., Ferreira, J. J., Marqués, D. P., Schiavone, F., & Ferraris, A. (2022). Literature reviews as independent studies: Guidelines for academic practice. Review of Managerial Science, 16(8), 2577–2595. https://doi.org/10.1007/s11846-022-00588-8
- Kraus, S., Kumar, S., Lim, W. M., Kaur, J., Sharma, A., & Schiavone, F. (2023). From moon landing to metaverse: Tracing the evolution of Technological Forecasting and social change. Technological Forecasting and Social Change, 189, 122381. https://doi.org/10.1016/j.techfore.2023.122381
- Kumar, S., Sahoo, S., Lim, W. M., & Dana, L. P. (2022). Religion as a social shaping force in entrepreneurship and business: Insights from a technology-empowered systematic literature review. Technological Forecasting and Social Change, 175, 121393. https://doi.org/10.1016/j.techfore.2021.121393
- Kumar, S., Sharma, D., Rao, S., Lim, W. M., & Mangla, S. K. (2023). Past, present, and future of sustainable finance: Insights from big data analytics through machine learning of scholarly research. Annals of Operations Research. Ahead of print. https://doi.org/10.1007/s10479-021-04410-8
- Lewandowsky, S., Ecker, U. K., Seifert, C. M., Schwarz, N., & Cook, J. (2012). Misinformation and its correction: Continued influence and successful debiasing. Psychological Science in the Public Interest, 13(3), 106–131. https://doi.org/10.1177/1529100612451018
- Lim, W. M. (2021). History, lessons, and ways forward from the COVID-19 pandemic. International Journal of Quality and Innovation, 5(2), 101–108.
- Lim, W. M. (2022). Ushering a new era of Global Business and Organizational Excellence: Taking a leaf out of recent trends in the new normal. Global Business and Organizational Excellence, 41(5), 5–13. https://doi.org/10.1002/joe.22163
- Lim, W. M., Chin, M. W. C., Ee, Y. S., Fung, C. Y., Giang, C. S., Heng, K. S., Kong, M. L. F., Lim, A. S. S., Lim, B. C. Y., Lim, R. T. H., Lim, T. Y., Ling, C. C., Mandrinos, S., Nwobodo, S., Phang, C. S. C., She, L., Sim, C. H., Su, S. I., Wee, G. W. E., & Weissmann, M. A. (2022). What is at stake in a war? A prospective evaluation of the Ukraine and Russia conflict for business and society. Global Business and Organizational Excellence, 41(6), 23–36. https://doi.org/10.1002/joe.22162
- Lim, W. M., Ciasullo, M. V., Douglas, A., & Kumar, S. (2023). Environmental Social Governance (ESG) and Total Quality Management (TQM): A multi-study meta-systematic review. Total Quality Management & Business Excellence, 1–23. Ahead of print. https://doi.org/10.1080/14783363.2022.2048952
- Lim, W. M., Gunasekara, A., Pallant, J. L., Pallant, J. I., & Pechenkina, E. (2023). Generative AI and the future of education: Ragnarök or reformation? A paradoxical perspective from management educators. The International Journal of Management Education, 21(2), 100790. https://doi.org/10.1016/j.ijme.2023.100790
- Lim, W. M., & Kumar, S. (2023). Guidelines for interpreting the results of bibliometrics analysis: A sensemaking approach. Global Business and Organizational Excellence. https://doi.org/10.1002/joe.22229
- Lim, W. M., Kumar, S., & Ali, F. (2022). Advancing knowledge through literature reviews: ‘What’, ‘why’, and ‘how to contribute’. The Service Industries Journal, 42(7–8), 481–513. https://doi.org/10.1080/02642069.2022.2047941
- Lim, W. M., Rasul, T., Kumar, S., & Ala, M. (2022). Past, present, and future of customer engagement. Journal of Business Research, 140, 439–458. https://doi.org/10.1016/j.jbusres.2021.11.014
- Loftus, E. F., & Hoffman, H. G. (1989). Misinformation and memory: The creation of new memories. Journal of Experimental Psychology: General, 118(1), 100–104. https://doi.org/10.1037/0096-3445.118.1.100
- Losee, R. M. (1997). A discipline independent definition of information. Journal of the American Society for Information Science, 48(3), 254–269. https://doi.org/10.1002/(SICI)1097-4571(199703)48:3<254:AID-ASI6>3.0.CO;2-W
- Madden, A. D. (2000). A definition of information. ASLIB Proceedings, 52(9), 343–349. https://doi.org/10.1108/EUM0000000007027
- Mukherjee, D., Lim, W. M., Kumar, S., & Donthu, N. (2022). Guidelines for advancing theory and practice through bibliometric research. Journal of Business Research, 148, 101–115. https://doi.org/10.1016/j.jbusres.2022.04.042
- Nguyen, B., Jaber, F., & Simkin, L. (2022). A systematic review of the dark side of CRM: The need for a new research agenda. Journal of Strategic Marketing, 30(1), 93–111. https://doi.org/10.1080/0965254X.2019.1642939
- Patra, R. K., Pandey, N., & Sudarsan, D. Bibliometric analysis of fake news indexed in Web of Science and Scopus (2001-2020). (2023). Global Knowledge, Memory & Communication, 72(6/7), 628–647. Ahead of print. https://doi.org/10.1108/GKMC-11-2021-0177
- Paul, J., Lim, W. M., O’Cass, A., Hao, A. W., & Bresciani, S. (2021). Scientific procedures and rationales for systematic literature reviews (SPAR‐4‐SLR). International Journal of Consumer Studies, 45(4), O1–O16. https://doi.org/10.1111/ijcs.12695
- Raponi, S., Khalifa, Z., Oligeri, G., & DiPietro, R. (2022). Fake news propagation: A review of epidemic models, datasets, and insights. ACM Transactions on the Web, 16(3), 1–34. https://doi.org/10.1145/3522756
- Seow, J. W., Lim, M. K., Phan, R. C. W., & Liu, J. K. (2022). A comprehensive overview of deepfake: Generation, detection, datasets, and opportunities. Neurocomputing, 513, 351–371. https://doi.org/10.1016/j.neucom.2022.09.135
- Spradling, M., Straub, J., & Strong, J. (2021). Protection from ‘fake news’: The need for descriptive factual labeling for online content. Future Internet, 13(6), 142. https://doi.org/10.3390/fi13060142
- Van Eck, N., & Waltman, L. (2010). Software survey: VOSviewer, a computer program for bibliometric mapping. Scientometrics, 84(2), 523–538. https://doi.org/10.1007/s11192-009-0146-3