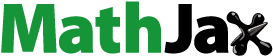
ABSTRACT
This paper estimates the impact of the different proximity dimensions on the subsequent innovation performance of firms which received radical knowledge spillovers. The analysis for the case of biotechnology small- and medium-sized firms (SMEs) in Germany is based on a dataset covering the period from 1998 to 2017. Results indicate the positive impact of social and organizational proximity on the innovation performance of radical knowledge recipients. Geographical proximity has a negative impact, whereas for cognitive proximity a U-shaped relation is observed. The paper contributes to the innovation economics and economic geography literature by underlining the peculiarities of the effectiveness of radical knowledge spillovers. Additionally, the importance of policy support for SMEs across regions and technological fields is highlighted.
1. Introduction
Radical innovation in Schumpeterian sense presents a recombination of already existing knowledge in a novel and complex way (Hervas-Oliver, Sempere-Ripoll, and Moll Citation2022). Such innovations open new technological trajectories which impact the future of economic sectors (Kovacs et al. Citation2019). However, the diffusion of radical knowledge is far from being completely understood, due to their complex character. This is especially true for the case of unintended knowledge spillovers, which often mean unconscious learning and require ‘scientific intuition’ (Howells Citation2002).
Existing literature mostly focuses on the ways to define radical innovation (Verhoeven, Bakker, and Veugelers Citation2016; Kovacs et al. Citation2019; Knuepling, Wessendorf, and Basilico Citation2022) and on the circumstances influencing its emergence (Arant et al. Citation2019; Grashof, Hesse, and Fornahl Citation2019; Xiao, Bao, and Wang Citation2021). The studies on radical knowledge transfer are limited to following the pattern of radical citations (Hesse Citation2020) or evaluating the importance of cooperation with radical innovators (Shkolnykova and Kudic Citation2022). Nevertheless, knowledge exchange does not always happen within contractual relations. Informal communication or close observation of radical innovators might lead to the unintentional transfer of knowledge and may influence the innovation performance of its recipient (Savic, Lawton Smith, and Bournakis Citation2020; Xiao, Bao, and Wang Citation2021). An additional source of an unintended spillover may be the absorption and appropriation of the scientific language from patents or publications (Tödtling and Grillitsch Citation2015).
Possible explanations of the factors influencing the impact of unintended knowledge spillovers may lie in proximity measures—i.e. closeness to radical innovators according to specific criteria. Different proximity dimensions were found to positively impact the success of cooperation or joint innovation (Hinzmann, Cantner, and Graf Citation2019) and foster the process of knowledge transfer, either through network-building (Broekel and Boschma Citation2012) or knowledge flows (Caragliu and Nijkamp Citation2016). As radical innovation contains complex and divergent knowledge (e.g. Kovacs et al. Citation2019), the impact of proximity dimensions for the case of radical knowledge spillovers may deviate from the general case.
The aim of this paper is thus to estimate the impact of the different proximity dimensions (geographical, cognitive, organizational and social) on the subsequent innovation performance of the recipients of radical spillovers. Throughout this paper, recipients of radical knowledge are defined as firms which used knowledge, covered by radical patent, in their subsequent innovation(s).
The analysis is performed for the case of German biotechnology SMEs. Reasons for that lie in the composition of the field. Firstly, biotechnology firms operate with complex science-based knowledge, which requires a high level of analytical skill. This may often lead to innovations of radical nature (Halkier et al. Citation2012). Secondly, SMEs in German biotechnology need to compete with biotech ‘giants’ and need to find a niche to perform activities (Zidorn and Wagner Citation2013). Thus, on the one hand, SMEs’ innovations may break field routines (Dahlin and Behrens Citation2005), and on the other hand, SMEs may profit from learning from radical peers through well-established social networks and dense location (Hao and Feng Citation2016; Arant et al. Citation2019).
In this study, the initial radical patent sample is generated based on novelty in knowledge combinations criteria (Grashof, Hesse, and Fornahl Citation2019; Shkolnykova and Kudic Citation2022). Next, the recipients of radical knowledge and their patents are defined by estimating the similarity of technical descriptions between radical patents and all subsequent biotech patents (Younge and Kuhn Citation2016; Feng Citation2020).
To identify the direction and strength of the effects of proximity dimensions, a longitudinal unbalanced panel dataset for the period 1998–2017 is constructed. An analysis is performed to test the importance of proximity dimensions. The results of this paper underline the special characteristics of unintended radical knowledge spillover and its difference from usual knowledge transfers. The positive impact of organizational and social proximity on radical knowledge diffusion was confirmed. However, the results for geographical and cognitive proximity are contradicting literature-based expectations (Grashof, Hesse, and Fornahl Citation2019; Hesse Citation2020): radical knowledge transfer has the most impact on geographically distant firms and on firms that are cognitively either close or distant.
The relevance of this paper is manifold. It is important for both science and management to understand the processes of diffusion of radical innovations. A recent example of the societal importance of such innovation can be seen in the development of treatments and vaccines to conquer COVID-19 (Possas et al. Citation2021). By learning from radical peers, firms and research institutions can help society by creating additional possibilities, for example, to cure diseases.
Researchers can learn about the specificities of radical diffusion processes and support policymakers in promoting social networks and patenting. The results of this paper also show entrepreneurs how they can profit from outside knowledge and which factors they should consider in the first place.
2. State of the art
2.1. Radical innovation
One widely used dichotomy of innovation activities distinguishes between incremental and radical innovations, as being opposite poles with respect to both content and impact (Knuepling, Wessendorf, and Basilico Citation2022). Radical innovations are often connected to the Schumpeter (Citation1934) definition of a ‘creative destruction’. In contrast, incremental innovations reflect Kirzner's (Citation1973) idea of the usage of existing knowledge in order to innovate (Graf and Menter Citation2022). Whereas incremental innovations present a small inventive step, radical innovations relate to rapid changes in technology and are connected to higher risk (Hervas-Oliver, Sempere-Ripoll, and Moll Citation2022). Radical innovations can result in substantially new products and ways of production, while incremental innovations are focused on the improvement of the existing ones (Freixanet and Rialp Citation2022; Hao and Feng Citation2016).
Especially radical innovations have recently drawn the interest of the scientific community—caused by global challenges, such as the climate crisis or COVID-19 pandemic. The solutions to these challenges often lie in a paradigm change, which is not possible without innovations of a disruptive character (Verhoeven, Bakker, and Veugelers Citation2016).
Despite its relevance, the operationalization of radical innovation is far from being univocal (Knuepling, Wessendorf, and Basilico Citation2022). Some authors follow Schumpeterian definition, which underlines the recombinatory power of these innovations, i.e. their capacity to combine previously unconnected knowledge or reference it (Verhoeven, Bakker, and Veugelers Citation2016). Others focus on the impact radical innovations have on the future of specific economic sectors (Dahlin and Behrens Citation2005; Verhoeven, Bakker, and Veugelers Citation2016). Thus, Kovacs et al. (Citation2019) performed the bibliometric analysis in order to distinguish radical innovation definitions. According to their results, ‘radical’ innovations mostly refer to novel knowledge combinations. Knuepling, Wessendorf, and Basilico (Citation2022) further mention, that the term ‘radical’ may include both a novelty and an impact dimension.
Recent literature investigated the conditions, which influence the emergence of radical innovation. Here, the geographical component was found to foster radical knowledge emergence (Grashof, Hesse, and Fornahl Citation2019). On the other hand, firm internal capabilities (Hervas-Oliver, Sempere-Ripoll, and Moll Citation2022) and diverse cooperation patterns (Arant et al. Citation2019; Graf and Menter Citation2022; Doehne and Rost Citation2021) appear to be unambiguously connected to radical innovation emergence.
Thus, there exists considerable literature on the characteristics and emergence of radical knowledge at different levels. Still, the research in this field has gaps regarding the processes after the introduction of radical innovations, e.g. which actors may grasp radical knowledge and profit from it.
2.2. Process of knowledge diffusion
Considering the cumulative character of knowledge, it becomes especially relevant to follow the changes of knowledge stock over time (Hanusch and Pyka Citation2007). Consequentially, the question of knowledge emergence and diffusion is often seen as a core element of firm development, economic performance, and technological growth (Döring and Schnellenbach Citation2006; Clayton, Lanahan, and Nelson Citation2022). Even though the contractual process of knowledge diffusion is formalized, often knowledge diffuses via a so-called unintended knowledge spillover. Classical examples of knowledge spillovers include informal communications between employees of different firms or knowledge exchange during conferences and workshops (Howells Citation2002). Because of their unpredictability and complexity, knowledge spillover processes attracted the attention of the scientific community (Grashof Citation2021; Feng Citation2020).
The characteristics of the spillover process depend on different factors, including the type of knowledge in question, firm characteristics, and firm environment. These factors often interact and influence the diffusion process simultaneously (Clayton, Lanahan, and Nelson Citation2022).
The type of knowledge is usually categorized as either explicit or tacit (Polanyi Citation1966). Explicit knowledge can be transmitted through formal language, for example, in patents (Döring and Schnellenbach Citation2006). The diffusion of tacit knowledge requires a more intimate form of communication than formal language (Fallah and Ibrahim Citation2004). The two types of knowledge often co-exist in different combinations (Asheim, Grillitsch, and Trippl Citation2017). This combination can be observed for radical innovation, as it is often documented in form of patents, scientific articles or reports (Halkier et al. Citation2012). Albeit, specific qualifications and skills are needed to decode the explicit knowledge stated in complex documentation, which make it partially tacit. This is especially prominent in high-tech industries, which are characterized by inputs of high complexity (Asheim Citation2007).
Differences in the knowledge transfer processes on can also be observed depending on the firm size. Large firms often possess resources and market power, which leads to innovations being based on internal firm capabilities (Ortega-Argilés, Vivarelli, and Voigt Citation2009). SMEs, in turn, often rely on external knowledge to develop new products and processes (Hervas-Oliver et al. Citation2020). It is widely accepted, that management efforts to promote knowledge sharing often lead to SMEs’ success (Egwunatum and Oboreh Citation2022). Motivated by competitive pressure, SMEs may innovate radically in order to stand out and remain in the market (Zidorn and Wagner Citation2013).
2.3. Proximity dimensions
Additional factors, which impact the effectiveness of the unintended spillover, may lie outside the firm boundaries. Thus, the literature considers factors, which describe the proximity between sender and recipient of the knowledge spillover (Caragliu and Nijkamp Citation2016; Hesse Citation2020). One of the most comprehensive classifications was introduced by Boschma (Citation2005), who distinguished between geographical, cognitive, institutional, organizational and social proximity.
Geographical proximity suggests that knowledge, especially tacit knowledge, is more likely to the spillover across small distances. Some scholars argue that scientific conferences and innovations in communication technology help to overcome a lack of geographical proximity (Breschi, Lissoni, and Montobbio Citation2005). However, innovation’s complex nature suggests that firms may benefit from being located close to innovative actors because they can better follow the trajectories of innovations (Hohberger Citation2014). Though often found to be important (Broekel and Boschma Citation2012; Bednarz and Broekel Citation2019), geographical proximity is not the only factor influencing the success and intensity of knowledge diffusion (Hinzmann, Cantner, and Graf Citation2019).
Cognitive (or technological) proximity has been established as another factor influencing knowledge diffusion. The basic idea is that when new knowledge is related to the recipient’s knowledge base, it is easier for the recipient to absorb it (Boschma Citation2005). Nooteboom et al. (Citation2007) claim that the optimal cognitive proximity function has an inverted U-shaped form. This means, when knowledge diffusion occurs in a cognitively too close or too distant environment, the probability of new knowledge creation will be lower (Broekel and Boschma Citation2012).
Organizational proximity can be broadly defined as the level of interdependencies between organizations (Boschma Citation2005). Balland (Citation2012) identified two perspectives related to this proximity measure. The first one is based on the interactions between two firms operating in different economic sectors. The second perspective sees organizational proximity as interactions between firms from the same corporate group. Broekel and Boschma (Citation2012) extend organizational proximity to include interactions between different types of firms and organizations.
Social proximity is seen as one of the most important factors influencing knowledge diffusion, especially for innovative SMEs (Sternberg Citation2007). Often, innovative SMEs cannot rely on formal relationships; instead, the presence of social ties between the actors of SMEs may provide advantages.
Finally, institutional proximity reflects situations and regimes that appear on the macro-level (Boschma Citation2005). Sternberg (Citation2007) suggests that this factor may be more important for old and well-established firms, rather than knowledge-based entrepreneurial ventures that are less dependent on institutional closeness.
2.4. Development of research hypotheses
The last chapter underlined the factors which influence the effect and speed of knowledge. However, based on the special nature of radical knowledge – namely, its complexity and divergence from routines – it can be expected, that the effect of spillover is different in this case (Hesse Citation2020; Shkolnykova and Kudic Citation2022). Additionally, the effect may be more accentuated for high-tech SMEs, as they are the most reliant on complex external knowledge (Egwunatum and Oboreh Citation2022; Hervas-Oliver et al. Citation2020). Thus, this chapter introduces hypotheses, connected to the main research question: What impact do different proximity dimensions have on the subsequent innovation performance of the recipients of unintended radical knowledge spillovers? Because this paper focuses only on German biotechnology SMEs, which experience similar institutional influences, this factor is not considered.
Clusters appear to be one of the factors which influence the emergence of radical innovation (Grashof, Hesse, and Fornahl Citation2019). Firms in close proximity to radical innovation may profit from it first, especially in the case of tacit knowledge diffusion. Although this effect may be diminished by the active interaction of SMEs with international corporations and repeated ties with regional actors (Zuluaga Citation2013), empirical literature indicates that geographical closeness may significantly influence knowledge diffusion in knowledge-intensive industries (Fornahl, Broekel, and Boschma Citation2011; Broekel and Boschma Citation2012). Hinzmann, Cantner, and Graf (Citation2019) further highlight the special importance of geographical proximity in explorative projects connected to radical knowledge generation. Hence, the first hypothesis states:
H1: Greater geographical distance from a radical innovator has a negative influence on the innovation performance of the recipients of radical knowledge spillovers.
H2: Cognitive proximity has an inverted U-shaped influence on the innovation performance of the recipients of radical knowledge spillovers.
H3: Organizational proximity has a positive influence on the innovation performance of the recipients of radical knowledge spillovers.
H4: Social proximity has a positive influence on the innovation performance of the recipients of radical knowledge spillovers.
3. Research design and data-generation strategy
3.1. Research design
In order to test the above-stated hypotheses a four-step research design is applied:
The sample of radical innovations is defined. This paper uses patents as a proxy for innovation and a measure of innovative performance. Although patents are criticized for not covering every innovation, they are empirically proven to be a suitable proxy for industries, where patenting is a common way of innovation protection (Hohberger Citation2014). The literature on the impact of proximity dimensions on innovation performance used patent counts as a main variable of interest (Marrocu, Paci, and Usai Citation2013; Xiao, Bao, and Wang Citation2021).
The recipient patents firms are identified. This is done, based on two criteria: (1) recipient patents should possess the same combinations of technological classes as radical patents; (2) textual elements of recipient patents should have sufficiently high similarity with radical ones.
The four proximity dimensions are operationalized along with the set of control variables.
An unbalanced panel is created and the analysis is performed.
The research design is further visualized in and explained in subsections 3.2–3.5.
3.2. Identification of radical patents
This paper applies the Schumpeterian notion of radical innovation being a novel combination of previously unconnected knowledge. This definition is broadly applied in the empirical literatureFootnote1 (Kovacs et al. Citation2019; Verhoeven, Bakker, and Veugelers Citation2016; Grashof, Hesse, and Fornahl Citation2019). Technically radical innovation is seen as the combination of previously unconnected technological fields. Patent technological fields are reflected in assigned classes according to International Patent Classification (IPC) or Cooperative Patent Classification (CPC) (Jaffe, Trajtenberg, and Henderson Citation1993). One patent may be related to more than one technological field. Thus, recombinant patents should include a dyad of IPC/CPC classes, which has not been applied in the field before.
In order to identify such patents, German biotechnology actors are determined with the help of the data from BIOCOM AG, a provider of firm-level data in biotechnology and life sciences in Germany. The firm population was further filtered to include only dedicated biotechnology SMEs. The verification dataset, which was used for IPC dyads comparison, includes all biotechnology-related firms, pharmaceutical firms and research institutions.
Patent population is then identified for both SMEs and the verification dataset. The procedure follows the radical patents’ identification strategy by Shkolnykova and Kudic (Citation2022). As a result, 4937 applications from dedicated biotechnology SMEs and 17,280 applications from the verification firm dataset were identified.Footnote2 These patents correspond to 31,727 IPC combinations for SMEs and 111,272 combinations for the verification dataset. A further step included the comparison of SMEs’ IPC combinations between datasets on the subclass level. The patent was considered to be radical if it included at least one dyad, which was either not found in the verification dataset or was applied for by a verification firm at most one year earlier than by the SME. The latter condition was introduced because of publication lags. The list of recombinant IPC dyads can be found in the appendix.
This procedure resulted in 396 novel IPC combinations from 286 patents. From these patents, 138 were applied for in German or European patent offices or according to the Patent Cooperation Treaty. As these patents reflect the German patent space, they are used for further analysis.
3.3. Identification of recipient patents and related firms
Next, the dataset of recipient patents is created. Patent citations are the most widespread measure used in the literature to follow unintended knowledge spillovers (Jaffe, Trajtenberg, and Henderson Citation1993) and trajectories (Bednarz and Broekel Citation2019). However, recent literature criticized their usage. Thus, Kuhn, Younge, and Marco (Citation2020) identified that the technological similarity between cited and citing patents is falling over time. The reasons for that are the strategic citing, citations added by patent examiners and cross-citations between large patent families. Thus, the literature proposes the usage of machine learning techniques and text vectors (Younge and Kuhn Citation2016; Feng Citation2020) with similarity measures to identify related patents (Feng Citation2020). As sole reliance on text-based measures may lead to ‘noisy’ results, technology classes can be considered (Feng Citation2020). This approach is also used in this paper. The analysis is performed in PATSTAT 2019a (Spring edition).
The patents which contain the same combination of IPC classes as the radical patents are identified. Additionally, the recipient patent’s earliest application should be filed later than the first publication of the radical patent. To ensure, that only German innovation fields are depicted, the patents must have at least one applicant or inventor with German affiliation. Furthermore, the patent should have German, European or World coverage. Thus, 16,899 patent applications are identified, with the first application between 1998 and 2017.Footnote3 These patent applications are subsequently connected to respective radical patent(s), based on the same IPC combination(s). One referencing patent can thus be assigned to more than one radical patent. As a result, 29,304 radical-recipient patent concordances are created.
The second criterion considers the similarity of texts between radical and recipient patents, namely their titles and abstracts.Footnote4 These parts of the patent documents provide the most relevant information and keywords regarding the invention.Footnote5 Mathematically, the cosine similarity measure is used in order to determine the semantic closeness of the patents:
(1)
(1) with referencing to the word vector of the words in the radical patent’s text and applying to the vector of the words in the recipient patent’s text. Thus, the cosine similarity can take the value between 0 and 1, with 1 meaning completely identical texts and 0 meaning texts without a single common word. The cut-off value for the cosine similarity is then identified as being located 1.5 standard deviations from the mean over all observations, i.e. values higher than 0.0852.Footnote6 As the result of this filter, 2581 entries from 1745 patents were left.
Next, relevant firms assigned to patents are extracted. Only firms that are characterized as SMEs according to the Orbis definitionFootnote7 database are considered. Subsequently, out of 297 German firms, 93 are left in the sample. These firms cite 37 patents from 25 radical firms.Footnote8 Concordance between radical and recipient firms can be found in the appendix. The number of patents per firm per yearFootnote9 is used as an indicator of the innovation performance of these firms and as a dependent variable in the analysis.
3.4. Operationalization of proximity dimensions and control variables
After identifying the sample of recipient SMEs, the variables pertinent to each of the hypotheses are examined. First, geographical proximity is measured as the distance in km between the radical innovator and the recipient firm (Broekel and Boschma Citation2012). The logarithm of distance is calculated in order to account for possible outliers (Broekel and Boschma Citation2012). If one of the recipient or radical firms moved, the distance measure is recalculated.
Second, cognitive proximity is identified. This proximity dimension reflects the similarity of knowledge bases of recipient firms and radical innovators. For the case of patent data, the technological knowledge base is most commonly depicted with the usage of IPC classes (Stiller, van Witteloostuijn, and Cambré Citation2021). In order to calculate the similarity between two vectors of IPC classes, cosine similarity is calculated as (Broekel and Boschma Citation2012):
(2)
(2) where i is the index for technology class, and l and m indicate the number of patents of a radical innovator and the recipient firm, respectively, in class i.
The number of patents is calculated cumulatively for a specific IPC class and specific firm over time. Cognitive proximity might take a value between 0 and 1, with 0 denoting complete technological dissimilarity, and 1 denoting complete technological similarity. Cognitive proximity may increase or decrease over time as firms’ technological paths change. A squared term is calculated for this indicator to check for the inverted U-shaped relationship.
Third, organizational proximity is estimated. It can be present when firms share similar routines and organizational arrangements, e.g. privately owned versus publicly owned firms (Boschma Citation2005; Broekel and Boschma Citation2012). Balland (Citation2012) added the role of shared corporate structure to this definition. Thus, firms are organizationally proximate, if they have the same parent company. As this analysis includes only SMEs, the most prominent distinction based on these theoretical considerations is the corporate group membership. This idea is supported by the evidence of the differences in organizational and decision-making processes between these firm types as well as differences in their cooperation patterns (Müller, Buliga, and Voigt Citation2021). Thus, firms are considered organizationally proximate if they both either belong to a corporate group or operate as a single entity, and organizationally distant if one of the two firms operate as a single entity. For acquired firms, the year of acquisition is taken as the starting point of corporate group affiliation, thus, changing the value of this indicator.
Fourth, social proximity is measured using the definition of Marrocu, Paci, and Usai (Citation2013), which is based on patent co-inventorship. Working on the same invention can be seen as a sign of close cooperation. Because employees may change workplaces or collaborate on a specific project with another firm, both joint co-applicants and co-inventors are included. Additionally, the importance of second-order ties (through a common partner without a direct tie between two firms) was highlighted by Martínez Ardila, Mora Moreno, and Camacho Pico (Citation2020). Hence, learning from second-order partners may positively impact the innovation performance. Thus, such connections are considered here. The earliest filing year of the patent with a common applicant or inventor is considered as the starting point of social proximity. This value of this indicator may change over time if the common patent was introduced after the start of the observation period.Footnote10
shows an overview of the proximity variables and the data sources used. A special case might arise when one firm is receiving the knowledge of more than one radical innovator, which happens for two firms. Here, the minimum value for geographical distance and the maximum value for technological, social and organizational proximity are taken.
Table 1. Proximity measures.
Several control variables that could influence innovation performance are introduced. Here Standard measures established by previous research for firm-level and regional-level variables are implemented (Díez-Vial and Fernández-Olmos Citation2014). The overview of the variables is presented in .
Table 2. Control variables.
3.5. Panel compilation and regression analysis
After the generation of the variables, the unbalanced panel is created. For each firm, the observation period starts with the earliest filing year of the recipient patent and ends with 2017 (the last year, for which complete patent data is available), except in the case of earlier firm dissolution. As the result, 919 firm-year observations are generated.
As the dependent variable is expressed through the number of patents, a count model is suitable for the analysis. Based on existing literature on knowledge diffusion, a negative binomial regression model is chosen (Bednarz and Broekel Citation2019), as it reflects the unbalanced panel structure of the dataset. Moreover, negative binomial regressions allow accounting for overdispersion (Cincera Citation1997), which is present in this data; thus, making the usage of the Poisson model inappropriate. The model used in this paper can be generalized as follows (Cincera Citation1997):
(3)
(3) where X stands for independent and control variables. Based on the results of a Hausman test and a likelihood ratio, a fixed-effects model specification is chosen over a random-effects one.
4 Results
4.1. Descriptive statistics
The correlations between variables are presented in . It shows several high correlation coefficients, especially for geographical and social proximities. Therefore, variance inflation factors (VIF) were calculated to check for multicollinearity. The highest VIF value of 2.27 is estimated for HUMRES variable, with an overall average VIF of 1.38. Thus, no multicollinearity is present in the dataset.
Table 3. Correlation coefficients.
presents descriptive statistics for all variables. GEO, AGE, TECHPARK, FEREG and HUMRES variables are presented as natural logarithms. Therefore, negative values for AGE and TECHPARK can be observed. No recipient firm is located in the vicinity of a radical innovator, with a minimum geographical distance of almost 6 km between TransTissue Technologies GmbH and Merete Holding GmbH (Berlin); the maximum distance is almost 700 km between PLANTON GmbH (Kiel) and Novaprot GmbH (Reischach, Bavaria). The highest distance to a technological park is estimated at 142 km for Tiegel GmbH (Radeberg, Saxony). Furthermore, most of the firms are located in the same NUTS 2 region with the largest biotech or pharma companies, whereas only 40% of the firms are located in a BioRegio- or BioProfile-funded region.
Table 4. Descriptive statistics.
The variation of cognitive distance is quite high. As IPC class combinations were one of the filters for recipient firms, no zero values are present here. However, lowest value of cognitive proximity estimates at 1% (NorthCo Ventures GmbH & Co. KG and Pharmasol), whereas the maximum level of cognitive proximity lies at more than 90% (atlantiChem GmbH and BBT Bergedorfer Biotechnik GmbH). The average value of COG is 35.1%.
Additionally, 90% of the entries have the same organizational structure. With regards to the SOC variable, 43.5% of the entries show the same co-inventor/co-applicant on the first- or second-order ties between the recipient and the radical firm. Interestingly, only 1.6% of entries would have social ties (i.e. SOC = 1), if only first-order ties would be accounted for.
4.2. Results of model estimation
presents the results of the negative binomial regression analysis. Models I–V include only one proximity dimension: Model I – GEO, Model II – COG, Model IV – ORG, and Model V – SOC. To test the hypothesis of an inverted U-shaped influence of cognitive proximity, the squared term COG2 was included in Model III together with COG. Model VI includes all variables besides COG2, and Model VII includes all variables.
Table 5. Negative binomial regression results.Table Footnotea
Contrary to hypothesis 1, greater geographical distance has a positive impact on the innovation performance of recipient firms across all model specifications. It means that complex radical knowledge spills over into space. Additionally, firms may learn more from looking ‘outside the box’ of their own region, as localized networks may seem well-known and outdated (Boschma Citation2005).Footnote11 This idea is supported by negative signs of TECHPARK, BIGCOMP and BIOREGIO variables: not only may recipient firms not profit from a close relationship to biotech clusters or industry giants, but it may even harm their innovation performance, for example, by dragging them into service projects (Kahl Citation2015; Hervas-Oliver, Sempere-Ripoll, and Moll Citation2022).
Hypothesis 2, concerning the inverted U-shaped relationship between cognitive proximity and innovation performance, is not supported by the data. Contrary, a U-shaped relationship is observed. This means that either a high or low overlap between knowledge bases of a radical innovator and a recipient firm leads to an increase in the recipient’s innovation performance. Though this result contradicts the major literature, it may hint at the specificity of radical knowledge as an old trajectories-breaker (Dahlin and Behrens Citation2005; Verhoeven, Bakker, and Veugelers Citation2016). Thus, recipient firms may link radical knowledge to development trajectories, which diverge from standard biotech knowledge, by either using radical knowledge in a completely new way or following the ideas of a radical innovator. This extends the evidence of Hervas-Oliver, Sempere-Ripoll, and Moll (Citation2022), who highlight the special importance of radical innovation emergence. Thus, not only the emergence but also the spillover of radical innovation depends on the technological knowledge base.
Hypotheses 3 and 4 are supported by the data, as both ORG and SOC variables have a positive impact on the innovation performance of the recipient firms. Thus, similar organizational routines foster the absorption of radical knowledge (Balland (Citation2012); Broekel and Boschma (Citation2012)). Additionally, in line with Martínez Ardila, Mora Moreno, and Camacho Pico (Citation2020) first- and second-order ties between radical and recipient firms positively impact the performance of the latter through mechanisms of trust and cooperation. Therefore, a radical knowledge of familiar firms is more likely to be positively implemented and further used. Personal encounters and close work relationships with creative firms show in the higher innovative performance of recipient firms.
5. Discussion and conclusion
The aim of this paper is to estimate the impact of different proximity dimensions – i.e. geographical, cognitive, organizational and social proximity – on the innovation performance of the recipients of unintended radical knowledge spillovers. By addressing the special case of radical innovation, this paper adds a new perspective on factors which influence the performance of firms after receiving knowledge spillovers. Here, the focus is shifted from the innovation performance of radical innovators themselves to the recipient firms.
The results of the analysis accentuate the peculiarities of radical knowledge diffusion. In line with the existing literature (Broekel and Boschma Citation2012; Caragliu and Nijkamp Citation2016; Clayton, Lanahan, and Nelson Citation2022), organizational and social proximity positively impact the subsequent innovation performance of recipient firms. This strengthens the empirical evidence for the importance of social networks for the spillover of complex radical knowledge to have a positive impact on the innovation performance of its recipient, as suggested by Clayton, Lanahan, and Nelson (Citation2022); Doehne and Rost (Citation2021). Moreover, this result underlines the importance of close collaboration and personal contacts in conjunction with similar routines for the unintended spillover of complex radical knowledge (Xiao, Bao, and Wang Citation2021; Savic, Lawton Smith, and Bournakis Citation2020). Thus, firms may especially profit from participation at conferences, workshops, and fairs, where the knowledge can flow between the peers.
However, for geographical and cognitive proximity, the results diverge from the expectations. Geographically distant partners experience a more positive impact of radical knowledge spillovers. Similar results were found in Shkolnykova and Kudic (Citation2022) for the transition of radical knowledge to formal project partners. Based on its highly complex and science-based character, radical knowledge is often written down, which means it can travel across distances (Halkier et al. Citation2012) and be applied by firms outside the region of the emergence. This result for geographical proximity also implies that recipient firms’ performance improves when they look for new knowledge outside the region, being free from regional lock-ins.
Additionally, the special case was found for cognitive proximity: recipient firms profit from either a high or low level of cognitive proximity. This corresponds to the idea of radical knowledge having the potential to open new trajectories and to influence different economic sectors (Dahlin and Behrens Citation2005). Contrary to major literature (e.g. Nooteboom et al. Citation2007; Fitjar, Huber, and Rodríguez-Pose Citation2016) for the case of unintended radical knowledge spillover no optimal proximity level could be observed. Hence, to profit the most from radical knowledge in terms of innovation performance, firms adopt radical knowledge and either follow radical innovators’ trajectory or creatively adapt the radical knowledge in a distinct way. In both cases, internal firm capabilities play a more important role in radical knowledge adoption than the specific location (Hervas-Oliver, Sempere-Ripoll, and Moll Citation2022).
The analysis has several limitations. As some entries for employee numbers or turnovers are missing, EMPL variable received a binary character. In case the longitudinal data for the number of employees and turnover becomes available, this variable could be elaborated. Additionally, Orbis definition of SMEs was applied. The sample could differ slightly if different criteria (e.g. of European Commission) were used. Furthermore, the specification of some variables (especially ORG and SOC variables) was bounded to the dataset, consisting of SMEs, with radical SMEs being mostly independent entities. The quantitative nature of this study also did not allow observing the collaboration patterns between firms not reflected in the patent data. In the case of the dataset and methodology extension in the further research (for example qualitative firm observations), different operationalization of these variables can be considered. Additionally, the PATSTAT dataset had some typos and translation ambiguities, which could mean that not all patents belonging to a specific firm could be determined.
The results of the paper have several far-reaching implications. Thus, several results diverge from the major literature on unintended knowledge spillovers, which leads to important theoretical considerations. Thus, contrary to the general case of knowledge diffusion (e.g. Fornahl, Broekel, and Boschma Citation2011; Hinzmann, Cantner, and Graf Citation2019), radical and complex knowledge, codified in patents, can travel through space and across industries, which can be considered when conducting studies on complex knowledge diffusion, especially for the high-tech industries. The evidence that the firms which can apply the obtained knowledge in a creative way and can efficiently communicate with radical peers will benefit the most from it, may extend the views on the factors impacting firm’s absorptive capacity (e.g. Nooteboom et al. Citation2007). Furthermore, the positive impact of social and organizational proximity confirms theory-based results (e.g. Clayton, Lanahan, and Nelson Citation2022; Xiao, Bao, and Wang Citation2021) and provides an additional empirical evidence of the importance of social networks and ties of different strengths also for unintended radical spillovers. Thus, an interesting avenue for further research may be to apply social network analysis for the relevant fields and identify the development trajectories of the networks around radical innovators. Additionally, the analysis may be extended to large firms.
The paper generates implications for policy-makers by underlining the importance of the SMEs promotion. Support should not be limited to a specific field, as radical innovation has an impact on the innovation performance of both technologically close and distant firms. This study also shows that knowledge diffusion is not regionally bounded, emphasizing the importance of funding across regions. Additionally, the importance of social proximity underlines the necessity for the funding of network projects. Specific funding measures may include the creation of co-working spaces and centres and subsidies for cooperation projects with special promotion of interdisciplinary project teams. Furthermore, programs which support SMEs’ patenting and knowledge codification are important. Further research may use different sources of innovation measurement, such as product, trademark or open-source codes. Additionally, the differences in spillovers of radical and incremental innovations may be identified and evaluated in order to update policy programs accordingly.
Code availability (software application or custom code)
Econometric estimation was performed with the help of Stata 16 and RStudio. Codes are available on request.
Supplemental Material
Download MS Word (24.5 KB)Acknowledgements
The author thanks the anonymous reviewers for their insightful comments and suggestions.
Disclosure statement
No potential conflict of interest was reported by the author(s).
Data availability statement
The datasets generated during and/or analysed during the current study are available from the corresponding author on reasonable request.
Additional information
Funding
Notes
1 There exist other operationalizations of radical innovation based on the structure of backwards citations (Shane Citation2001) or the difference in the technological structure of the cited and focal patents (Dahlin and Behrens Citation2005). The operationalization of Shane (Citation2001) was used by the Organisation for Economic Co-operation and Development (Squicciarini, Dernis, and Criscuolo Citation2013). Additionally, forward citations may be accounted for Shkolnykova and Kudic (Citation2022). In this paper, wider sample is used as a patent impact was based on alternative indicators.
2 In the reference dataset, no year restrictions were set.
3 The endpoint is explained by the data availability, as no complete patent population after 2017 could be obtained.
4 With the help of text2vec package (https://CRAN.R-project.org/package=text2vec).
5 The usage of titles and abstracts is criticized because of the generality (Feng Citation2020). Thus, additional data (e.g. claims) can be used. For this paper, abstracts and titles provide a sufficient amount of data, as specific technological fields are considered.
6 With the mean value of 0.0128 and standard deviation of 0.0483.
7 A firm is considered an SME, if it has: less than 150 employees, operating revenue less than 10 million euro or total assets of less than 20 million euro. Subsidiaries remained in the sample, if they could be separated from the headquarter, e.g. Bayer Chemicals GmbH was excluded because of confusion with the patents of Bayer AG.
8 The identification of the recipient firms is based on the Neo-Schumpeterian assumption of knowledge cumulativeness (Hanusch and Pyka Citation2007). To prove the adequacy of recipient firms’ identification, their subsequent patents were checked. It appeared, that 76% of firms re-used the radical IPC combinations, meaning that radical patents left a visible footprint in the recipient’s inventions.
9 On the simple patent family level.
10 The common co-applicants or co-inventors are identified based on psn_id.
11 As Boschma (Citation2005) implied that geographical proximity may impact other proximity dimensions, the interaction effects between GEO and COG, GEO and ORG, and GEO and SOC were additionally investigated. Results are presented in the appendix and show a positive impact of geographical proximity on the organizational and cognitive dimension with no impact on social proximity. However, this result needs to be treated with caution based on the model’s non-linearity.
References
- Arant, W., D. Fornahl, N. Grashof, K. Hesse, and C. Söllner. 2019. “University-Industry Collaborations – The Key to Radical Innovations?” Review of Regional Research 39: 119–141.
- Asheim, B. 2007. “Differentiated Knowledge Bases and Varieties of Regional Innovation Systems.” Innovation 20 (3): 223–241. doi:10.1080/13511610701722846.
- Asheim, B., M. Grillitsch, and M. Trippl. 2017. “Smart Specialization as an Innovation-Driven Strategy for Economic Diversification: Examples from Scandinavian Regions.” In Advances in the Theory and Practice of Smart Specialization, edited by S. Radosevic, A. Curaj, R. Gheorghiu, L. Andreescu, and I. Wade, 73–97. London: Academic Press.
- Balland, P.-A. 2012. “Proximity and the Evolution of Collaboration Networks: Evidence from Research and Development Projects Within the Global Navigation Satellite (GNSS) Industry.” Regional Studies 46 (6): 741–756. doi:10.1080/00343404.2010.529121
- Bednarz, M., and T. Broekel. 2019. “The Relationship of Policy Induced R&D Networks and Inter-Regional Knowledge Diffusion.” Journal of Evolutionary Economics 29: 1459–1481. doi:10.1007/s00191-019-00621-2
- Boschma, R. 2005. “Proximity and Innovation: A Critical Assessment.” Regional Studies 39 (1): 61–74. doi:10.1080/0034340052000320887
- Breschi, S., F. Lissoni, and F. Montobbio. 2005. “The Geography of Knowledge Spillovers: Conceptual Issues and Measurement Problems.” In Clusters, Networks and Innovation, edited by S. Breschi and F. Malerba, 343–378. Oxford/ New York: Oxford University Press.
- Broekel, T., and R. Boschma. 2012. “Knowledge Networks in the Dutch Aviation Industry: The Proximity Paradox.” Journal of Economic Geography 12: 409–433. doi:10.1093/jeg/lbr010
- Caragliu, A., and P. Nijkamp. 2016. “Space and Knowledge Spillovers in European Regions: The Impact of Different Forms of Proximity on Spatial Knowledge Diffusion.” Journal of Economic Geography 16 (3): 749–774. doi:10.1093/jeg/lbv042
- Cassi, L., and A. Plunket. 2014. “Proximity, Network Formation and Inventive Performance: In Search of the Proximity Paradox.” The Annals of Regional Science 53 (2): 395–422. doi:10.1007/s00168-014-0612-6
- Cincera, M. 1997. “Patents, R&D, and Technological Spillovers at the Firm Level: Some Evidence from Econometric Count Models for Panel Data.” Journal of Applied Economics 12 (3): 265–280. doi:10.1002/(SICI)1099-1255(199705)12:3<265::AID-JAE439>3.0.CO;2-J
- Clayton, P., L. Lanahan, and A. Nelson. 2022. “Dissecting Diffusion: Tracing the Plurality of Factors That Shape Knowledge Diffusion.” Research Policy 51 (1): 104389. doi:10.1016/j.respol.2021.104389
- Dahlin, K., and D. Behrens. 2005. “When is an Invention Really Radical?: Defining and Measuring Technological Radicalness.” Research Policy 34 (5): 717–737. doi:10.1016/j.respol.2005.03.009
- Díez-Vial, I., and M. Fernández-Olmos. 2014. “Knowledge Spillovers in Science and Technology Parks: How Can Firms Benefit Most?” The Journal of Technology Transfer 40: 70–84. doi:10.1007/s10961-013-9329-4
- Doehne, M., and K. Rost. 2021. “Long Waves in the Geography of Innovation: The Rise and Decline of Regional Clusters of Creativity Over Time.” Research Policy 50 (9): 104298. doi:10.1016/j.respol.2021.104298
- Döring, T., and J. Schnellenbach. 2006. “What Do We Know About Geographical Knowledge Spillovers and Regional Growth?: A Survey of the Literature.” Regional Studies 40 (03): 375–395. doi:10.1080/00343400600632739
- Egwunatum, S., and J. Oboreh. 2022. “Factors Limiting Knowledge Management among Construction Small and Medium Enterprises.” Journal of Engineering, Project, and Production Management 12 (1): 1. doi:10.32738/JEPPM-2022-0003.
- Fallah, M., and S. Ibrahim. 2004. Knowledge Spillover and Innovation in Technological Clusters. In Proceedings, IAMOT 2004 Conference, Washington, DC.
- Feng, S. 2020. “The Proximity of Ideas: An Analysis of Patent Text Using Machine Learning.” PLoS One 15 (7): e0234880. doi:10.1371/journal.pone.0234880.
- Fitjar, R., F. Huber, and A. Rodríguez-Pose. 2016. “Not Too Close, Not Too far: Testing the Goldilocks Principle of ‘Optimal’ distance in Innovation Networks.” Industry and Innovation 23 (6): 465–487. doi:10.1080/13662716.2016.1184562
- Fornahl, D., T. Broekel, and R. Boschma. 2011. “What Drives Patent Performance of German Biotech Firms? The Impact of R&D Subsidies, Knowledge Networks and Their Location.” Papers in Regional Science 90 (2): 395–419. doi:10.1111/j.1435-5957.2011.00361.x
- Freixanet, J., and J. Rialp. 2022. “Disentangling the Relationship Between Internationalization, Incremental and Radical Innovation, and Firm Performance.” Global Strategy Journal 12 (1): 57–81. doi:10.1002/gsj.1412
- Graf, H., and M. Menter. 2022. “Public Research and the Quality of Inventions: The Role and Impact of Entrepreneurial Universities and Regional Network Embeddedness.” Small Business Economics 58 (2): 1187–1204. doi:10.1007/s11187-021-00465-w
- Grashof, N. 2021. “Spill Over or Spill out? A Multilevel Analysis of the Cluster and Firm Performance Relationship.” Industry and Innovation 28 (10): 1298–1331. doi:10.1080/13662716.2021.1967728
- Grashof, N., K. Hesse, and D. Fornahl. 2019. “Radical or not? The Role of Clusters in the Emergence of Radical Innovations.” European Planning Studies 27 (10): 1904–1923. doi:10.1080/09654313.2019.1631260
- Halkier, H., L. James, M. Dahlström, and J. Manniche. 2012. “Knowledge Dynamics, Regions and Public Policy.” European Planning Studies 20 (11): 1759–1766. doi:10.1080/09654313.2012.723419
- Hanusch, H., and A. Pyka. 2007. “Principles of Neo-Schumpeterian Economics.” Cambridge Journal of Economics 31 (2): 275–289. doi:10.1093/cje/bel018
- Hao, B., and Y. Feng. 2016. “How Networks Influence Radical Innovation: The Effects of Heterogeneity of Network Ties and Crowding out.” Journal of Business and Industrial Marketing. doi:10.1108/JBIM-09-2012-0165.
- Hervas-Oliver, J., F. Sempere-Ripoll, C. Boronat-Moll, and S. Estelles-Miguel. 2020. “SME Open Innovation for Process Development: Understanding Process-Dedicated External Knowledge Sourcing.” Journal of Small Business Management 58 (2): 409–445. doi:10.1080/00472778.2019.1680072
- Hervas-Oliver, J., F. Sempere-Ripoll, and C. Moll. 2022. “Zooming Into Firms’ Location, Capabilities and Innovation Performance: Does Agglomeration Foster Incremental or Radical Innovation?” European Research on Management and Business Economics 28 (2): 100186. doi:10.1016/j.iedeen.2021.100186
- Hesse, K. 2020. “Related to Whom? The Impact of Organizational and Regional Capabilities on Radical Breakthroughs.” Bremen Papers on Economics & Innovation, 1–29. doi:10.26092/elib/198.
- Hinzmann, S., U. Cantner, and H. Graf. 2019. “The Role of Geographical Proximity for Project Performance: Evidence from the German Leading-Edge Cluster Competition.” The Journal of Technology Transfer 44 (6): 1744–1783. doi:10.1007/s10961-017-9600-1
- Hohberger, J. 2014. “Searching for Emerging Knowledge: The Influence of Collaborative and Geographically Proximate Search.” European Management Review 11 (2): 139–157. doi:10.1111/emre.12031
- Howells, J. 2002. “Tacit Knowledge, Innovation and Economic Geography.” Urban Studies 39 (5-6): 871–884. doi:10.1080/00420980220128354
- Jaffe, A., M. Trajtenberg, and R. Henderson. 1993. “Geographic Localization of Knowledge Spillovers as Evidenced by Patent Citations.” The Quarterly Journal of Economics 108 (3): 577–598. doi:10.2307/2118401
- Kahl, J. 2015. The Determinants of Firm Growth in High Technology Industries. An Empirical Analysis of Spatial, Relational and Institutional Factors in the German Biotechnology Industry. Schriftenreihe innovative betriebswirtschaftliche Forschung und Praxis, Band 443, Hamburg.
- Kirzner, I. 1973. Competition and Entrepreneurship. Chicago: University of Chicago Press.
- Knuepling, L., C. Wessendorf, and S. Basilico. 2022. “Revisiting Innovation Typology: A Systemic Approach.” Jena Economic Research Papers 002.
- Kovacs, A., C. Marullo, D. Verhoeven, and B. Van Looy. 2019. “Radical, Disruptive, Discontinuous and Breakthrough Innovation: More of the Same?” In Academy of Management Proceedings 2019 vols, No. 1, 14866. Briarcliff Manor, NY: Academy of Management.
- Kuhn, J., K. Younge, and A. Marco. 2020. “Patent Citations Reexamined.” The RAND Journal of Economics 51 (1): 109–132. doi:10.1111/1756-2171.12307
- Marrocu, E., R. Paci, and S. Usai. 2013. “Proximity, Networking and Knowledge Production in Europe: What Lessons for Innovation Policy?” Technological Forecasting & Social Change 80: 1484–1498. doi:10.1016/j.techfore.2013.03.004
- Martínez Ardila, H., J. Mora Moreno, and J. Camacho Pico. 2020. “Networks of Collaborative Alliances: The Second Order Interfirm Technological Distance and Innovation Performance.” The Journal of Technology Transfer 45: 1255–1282. doi:10.1007/s10961-018-9704-2
- Müller, J., O. Buliga, and K. Voigt. 2021. “The Role of Absorptive Capacity and Innovation Strategy in the Design of Industry 4.0 Business Models-A Comparison Between SMEs and Large Enterprises.” European Management Journal 39 (3): 333–343. doi:10.1016/j.emj.2020.01.002
- Nooteboom, B., W. Van Haverbeke, G. Duysters, V. Gilsing, and A. van der Oord. 2007. “Optimal Cognitive Distance and Absorptive Capacity.” Research Policy 36 (7): 1016–1034. doi:10.1016/j.respol.2007.04.003
- Ortega-Argilés, R., M. Vivarelli, and P. Voigt. 2009. “R&D in SMEs: A Paradox?” Small Business Economics 33 (1): 3–11. doi:10.1007/s11187-009-9187-5
- Polanyi, M. 1966. “The Logic of Tacit Inference.” Philosophy 41 (155): 1–18. doi:10.1017/S0031819100066110
- Possas, C., A. Antunes, A. Oliveira, M. Ramos, S. Schumacher, and A. Homma. 2021. “Genomic Vaccines for Pandemic Diseases in Times of COVID-19: Global Trends and Patent Landscape.” In Bio# Futures, edited by E. Koukios and A. Sacio-Szymańska, 249–274. Cham: Springer.
- Savic, M., H. Lawton Smith, and I. Bournakis. 2020. “Innovation and External Knowledge Sources in Knowledge Intensive Business Services (KIBS): Evidence from de-Industrialized UK Regions.” Entrepreneurship & Regional Development 32 (9-10): 805–826. doi:10.1080/08985626.2020.1789751
- Schumpeter, J. 1934. The Theory of Economic Development. Cambridge: Harvard University Press.
- Shane, S. 2001. “Technological Opportunities and new Firm Creation.” Management Science 47 (2): 205–220. doi:10.1287/mnsc.47.2.205.9837
- Shkolnykova, M., and M. Kudic. 2022. “Who Benefits from SMEs’ Radical Innovations? – Empirical Evidence from German Biotechnology.” Small Business Economics 58: 1157–1185. doi:10.1007/s11187-021-00464-x.
- Squicciarini, M., H. Dernis, and C. Criscuolo. 2013. Measuring Patent Quality: Indicators of Technological and Economic Value, OECD Science, Technology and Industry Working Papers, No. 2013/03, OECD Publishing, Paris.
- Sternberg, R. 2007. “Entrepreneurship, Proximity and Regional Innovation Systems.” Royal Dutch Geographical Society KNAG 98 (5): 652–666. doi:10.1111/j.1467-9663.2007.00431.x.
- Stiller, I., A. van Witteloostuijn, and B. Cambré. 2021. “Do Current Radical Innovation Measures Actually Measure Radical Drug Innovation?” Scientometrics 126 (2): 1049–1078. doi:10.1007/s11192-020-03778-x
- Tödtling, F., and M. Grillitsch. 2015. “Does Combinatorial Knowledge Lead to a Better Innovation Performance of Firms?” European Planning Studies 23 (9): 1741–1758. doi:10.1080/09654313.2015.1056773
- Verhoeven, D., J. Bakker, and R. Veugelers. 2016. “Measuring Technological Novelty with Patent-Based Indicators.” Research Policy 45 (3): 707–723. doi:10.1016/j.respol.2015.11.010
- Xiao, J., Y. Bao, and J. Wang. 2021. “Which Neighbor is More Conducive to Innovation? The Moderating Effect of Partners’ Innovation.” The Journal of Technology Transfer. doi:10.1007/s10961-021-09905-x.
- Younge, K., and J. Kuhn. 2016. “Patent-to-Patent Similarity: A Vector Space Model.” doi:10.2139/ssrn.2709238.
- Zidorn, W., and M. Wagner. 2013. “The Effect of Alliances on Innovation Patterns: An Analysis of the Biotechnology Industry.” Industrial and Corporate Change 22 (6): 1497–1524. doi:10.1093/icc/dts042
- Zuluaga, J. 2013. “Knowledge Sourcing, Local and International Spillovers and the Novelty of Technological Innovation in Developing Count.” Conferência Internacional LALICS 2013 “Sistemas Nacionais de Inovação e Políticas de CTI para um Desenvolvimento Inclusivo e Sustentável”.