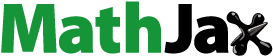
ABSTRACT
Research on geographies of knowledge sourcing examines the organizational structure of innovation activities within the firm, the mechanisms by which knowledge is extracted from various external sources and the geography of these different activities. We augment this literature by exploring knowledge sourcing within multi-plant firms operating in Europe. Analysis makes use of linked patent-firm data recording the location of knowledge production and its ownership. The results add value to existing research in three ways. First, the establishments of multi-plant firms are shown to produce different kinds of knowledge in different locations. Second, the patents generated within a firm's establishments are linked to the knowledge stocks of the regions where they operate, supporting a vision of geographical knowledge sourcing. Third, the complexity of knowledge produced within firms is positively related to the number of plants in which they innovate.
Introduction
Successful competition for individual firms, especially over the long-run, depends heavily on access to new technological know-how. That knowledge can be developed within the firm through learning and dedicated processes of research and development (Arrow Citation1962; Pavitt Citation1991), and it can be sourced from outside the firm through more or less formal market and non-market interactions (Von Hippel Citation1988; Chesbrough Citation2003). While incremental innovations can be generated by economic agents in many locations, more complex and valuable innovation relies on the recombination of knowledge subsets that are not widely available (Mewes Citation2019). Indeed, Balland et al. (Citation2020) report that more complex forms of knowledge are increasingly confined to larger cities, sites where highly specialized economic agents with diverse sets of capabilities are more likely to be found. Coupled with the difficulty of moving complex technological ideas (see Sorenson, Rivkin, and Fleming Citation2006 and Balland and Rigby Citation2017), a relatively small number of innovative regions have become locational targets for firms engaged in knowledge sourcing. Recent research confirms that the production of complex knowledge confers growth advantages on firms located within a limited number of sub-national regions in advanced and emerging economies alike (Mewes and Broekel Citation2022; Pintar and Scherngell Citation2022; Rigby et al. Citation2022; Li and Rigby Citation2022).
A rapidly growing literature on knowledge sourcing explores how firms access and utilize external sources of knowledge (Almeida Citation1996; Chung and Alcácer Citation2002; Tödtling, Lehner, and Trippl Citation2006; Huggins et al. Citation2015). For the majority of firms that are single-plant businesses, external knowledge sourcing means tapping into local networks of workers, firms and other research producing organizations, while also looking to access networks of economic agents that reach into non-local knowledge pools (Amin and Cohendet Citation1999; Bathelt, Malmberg, and Maskell Citation2004). Within economic geography, research on knowledge sourcing (Bathelt, Malmberg, and Maskell Citation2004; Lorentzen Citation2007; Fitjar and Rodríguez-Pose Citation2013) has been directed at long-standing claims of the local bias of knowledge flows (Marshall Citation1890; Jaffe, Trajtenberg, and Henderson Citation1993; Storper and Venables Citation2004), on whether the ‘buzz and pipelines’ model of Bathelt, Malmberg, and Maskell (Citation2004) is sufficient to capture the heterogeneity of knowledge sourcing practices over space (Trippl, Tödtling, and Lengauer Citation2009), on knowledge flows in different regional innovation systems and across knowledge bases (Asheim and Gertler Citation2005; Martin and Moodysson Citation2013; Tödtling, Grillitsch, and Hoeglinger Citation2012), and on the benefits of combining local and non-local forms of knowledge (Bathelt Citation2007).
We seek to add to the literature above through examining the knowledge sourcing practices of multi-locational firms operating within Europe. Unlike most single-plant businesses, multi-plant firms have the capacity to embed themselves within different local knowledge bases, raising the possibilities for combining heterogeneous sets of valuable knowledge. Furthermore, multi-locational firms generate internally many of the different sources of proximity outlined by Boschma (Citation2005) and so face lower costs of knowledge integration than others. Still, we do not have much information about the knowledge sourcing practices of multi-plant firms, especially at the subnational level. We ask three questions regarding the geography of innovation within multi-plant firms. First, do these firms produce different kinds of knowledge across their geographically disparate operational units? Second, is the knowledge they produce at these locations linked to the character of the local knowledge base? That is, do we see evidence of geographical knowledge sourcing by multi-plant firms? Third, are these firms able to integrate the knowledge sourced from different sites and increase the average value of the technologies they generate?
The research questions are addressed by using patent records matched to firm-level ownership and geographical data covering multi-locational firms in Europe. Our focus is placed on the knowledge production activities of these firms across European NUTS2 regions. Results show that close to 70% of firms investigated produce patents in knowledge classes that are significantly different across the locations in which they operate. This share reaches close to 100% for firms with more than 10 establishments, as compared to slightly below 40% for firms with only two establishments. We find that the patents generated within the establishments of multi-unit organizations reflect the broader technological character of the regions in which they are located. This adds to the body of evidence that multi-plant firms strategically locate their operations in order to access place-specific stocks of knowledge. Finally, analysis reveals that the number of establishments across which the firm distributes its innovative activities is positively associated with the production of more complex and valuable patents.
The rest of the paper is divided into four sections. Section two reviews the economic geography literature on knowledge sourcing. In Section three we outline construction of a linked firm-patent data set and provide summary statistics for our sample of multi-unit firms. Section four presents the results of our analysis and Section five summarizes the research findings.
Knowledge sourcing in economic geography
For many firms and regions in the contemporary market economy competitive advantage increasingly rests on the production of non-ubiquitous, tacit knowledge (Maskell and Malmberg Citation1999). Within the firm, knowledge mainly originates from processes of research and development (Pavitt Citation1991) and learning (Arrow Citation1962). For Von Hippel (Citation1988) and Chesbrough (Citation2003), technological knowledge is also acquired outside the firm through market-based interaction, formal and informal exchanges, spillovers and learning from others. Cohen and Levinthal (Citation1990) lay the foundations of firm knowledge development at the intersection of internal and external knowledge sources.
There is growing evidence that different sites of economic activity produce distinct subsets of knowledge whether measured by firm and industry product and process technologies (Rigby and Essletzbichler Citation1997), in terms of broad knowledge bases (Asheim and Gertler Citation2005; Martin and Moodysson Citation2011), or in the mix of patents generated (Kogler, Rigby, and Tucker Citation2013). Much of this knowledge is not easily moved (Jaffe, Trajtenberg, and Henderson Citation1993; Audretsch and Feldman Citation1996), especially that which is tacit (Polanyi Citation1966; Maskell and Malmberg Citation1999; Gertler Citation2003; Nonaka and Takeuchi Citation1995) and complex (Sorenson, Rivkin, and Fleming Citation2006; Balland and Rigby Citation2017). The geographical knowledge landscape then is highly uneven, a low-level surface of widely available codified knowledge interspersed by jagged peaks representing concentrations of complex and tacit knowledge that are broadly aligned with larger cities (Balland et al. Citation2020).
Regardless of how knowledge is developed, spatial clusters of firms, workers and related organizations tend to dominate innovation (Feldman Citation1994). In industries and knowledge fields where innovation largely occurs within the firm, access to venture capital funding (Sorenson and Stuart Citation2001), basic research from universities and labs (Fritsch and Schwirten Citation1999; Bramwell and Wolfe Citation2008; Laursen, Reichstein, and Salter Citation2011), and the availability of teams of specialized knowledge workers (Alcácer and Chung Citation2014) and specialized service providers (Muller and Doloreux Citation2009; Shearmur and Doloreux Citation2019) limit locational possibilities. Where innovation results more from external exchanges with customers and suppliers, the density of partners plays a critical role (Duranton and Puga Citation2001). To varying degrees, these arguments sustain a long literature within economic geography from Marshall’s (Citation1890) agglomerations and Porter’s (Citation1990, Citation1998) clusters, to the industrial districts and milieu of Brusco (Citation1986), Becattini (Citation1991) and Camagni (Citation1991), through to learning regions (Lundvall and Johnson Citation1994; Cooke Citation2002; Morgan Citation2007) and the regional innovation systems they develop (Braczyk and Heidenreich Citation1998; Cooke, Boekholt, and Tödtling Citation2000). The takeaway from much of this literature is that firms and other economic agents who are part of an innovative cluster are advantaged in ways that outsiders are not. ‘Being there’, as Gertler (Citation2003) notes, smoothes the sharing of ideas, whether they are generated by ‘local buzz’ (Storper and Venables Citation2004), delivered in the form of spillovers (Jaffe, Trajtenberg, and Henderson Citation1993; Sonn and Storper Citation2008), through worker mobility (Almeida and Kogut Citation1999) or networks of economic agents (Giuliani Citation2013). For Saxenian (Citation1994) and Storper (Citation1995), following Brown and Duguid (Citation1991), place-specific communities of practice and institutional development foster trust, collective learning (Lawson and Lorenz Citation1999), shared capabilities and various forms of proximities (Boschma Citation2005) that reduce the cost of interaction.
More recent work challenges the local bias of the knowledge exchange literature (Bathelt, Malmberg, and Maskell Citation2004). That access to external knowledge plays a central role in firm innovation is the foundation for a vibrant literature in economic geography on knowledge sourcing. Combining arguments from the regional innovation systems literature (Cooke, Boekholt, and Tödtling Citation2000; Asheim and Gertler Citation2005), from knowledge-base ideas (Asheim and Coenen Citation2005 and Martin and Moodysson Citation2011) and research on modes of learning (Jensen et al. Citation2007), this work explores how firms in different industries acquire external knowledge, focusing on the sources of that knowledge, the mechanisms through which it is obtained and the geographical scales at which those mechanisms operate (Tödtling, Lehner, and Trippl Citation2006; Trippl, Tödtling, and Lengauer Citation2009; Fitjar and Rodríguez-Pose Citation2013; Grillitsch and Trippl Citation2014; Isaksen and Trippl Citation2017). Much of this work pushes beyond the buzz and pipelines binary to examine how different forms of interaction for knowledge exchange are organized, from informal linkages to more durable network collaborations (see also Powell, Koput, and Smith-Doerr Citation1996 and Ter Wal and Boschma Citation2009), involving value-chain partners, universities, research labs and competitors located in a single cluster, or distributed more widely over space.
Although, it is not straightforward to summarize the results of this literature, it is safe to say first, that different types of knowledge, market and technological, product and process, tacit and codified appear to circulate in different ways (Tödtling, Grillitsch, and Hoeglinger Citation2012; Fitjar and Rodríguez-Pose Citation2020). Second, knowledge-based interactions appear to be at least as likely to occur between non-local as local partners, though this seems to rest heavily on industrial sector and the fecundity of local/regional innovation systems (Tödtling, Lengauer, and Höglinger Citation2011 and Trippl Citation2011). Third, the nature of the underlying knowledge base (analytical, synthetic or symbolic) is correlated with the geography of knowledge circulation (Martin and Moodysson Citation2013), though the interaction of the innovation system and the knowledge base complicate simple readings (Tödtling, Grillitsch, and Hoeglinger Citation2012). Grillitsch and Trippl (Citation2014) and Isaksen and Trippl (Citation2017) synthesize the key insights from many of the aforementioned studies in their analysis of knowledge sourcing and patterns of regional innovation.
The knowledge sourcing literature within economic geography is very much focused upon the acquisition of external knowledge by firms that are considered, at least implicitly, as single establishment businesses that operate from a fixed location. This literature says relatively little about how the structure of the firm might evolve to exploit the geography of technological heterogeneity. However, it is clear from the literature in international business and management that increasing numbers of multinational enterprises (MNEs) have divided their operations, at least in part, to embed themselves within different sites of knowledge production (Ghoshal and Bartlett Citation1988; Cantwell Citation1989; Dunning Citation1998; Chung and Alcácer Citation2002; Almeida and Phene Citation2004; Cantwell and Mudambi Citation2011). These locations are often well-connected global city-regions (Cantwell and Iammarino Citation2003; Goerzen, Asmussen, and Nielsen Citation2013; Iammarino and McCann Citation2013; Castellani et al. Citation2022) containing relatively dense clusters of innovative economic agents (Berry Citation2015; Li and Bathelt Citation2018) with high potentials for knowledge acquisition (Alcácer and Chung Citation2014; Jindra, Hassan, and Cantner Citation2016). Of course, access to multiple sources of knowledge is not the only reason for the multi-locational structure of many business organizations. Following Dunning (Citation1977) there is a vast literature on the factors shaping the organization of MNEs and the geography of their activities (see Dunning Citation1998 and Neilsen, Asmusen, and Goerzen Citation2018).
We extend the knowledge sourcing discussion within economic geography by examining the activities of multi-unit firms operating within Europe. Value is added to earlier work by tracing connections between the establishments of multi-locational firms and the technological capabilities found in different regions (Cantwell and Iammarino Citation2001; Lo Turco and Maggioni Citation2016, Citation2019; Neffke et al. Citation2018; Elekes, Boschma, and Lengyel Citation2019 and Crescenzi, Dyèvre, and Neffke Citation2022). While recent work on the geography of knowledge sourcing restricts the technological search of firms in different sectors to broad knowledge types, we show that individual firms access different kinds of technological knowledge in different places and that they benefit from this practice.
Data
Knowledge sourcing and technological diversification in multi-locational firms operating in Europe are explored by combining patent data from the European Patent Office (EPO) with firm-level data from the Bureau Van Dijk (BVD)'s Orbis database. Patent assignees (firms in our sample) are regrouped by corporate ownership. Company groups in the sample include branches, that are fully-owned legal extensions of the firm, and majority-owned subsidiaries. For the latter, we collect merger and acquisition data from Zephyr to track changes in ownership. Patents generated by subsidiaries are linked to a parent firm only if the application year corresponds to the period of actual ownership.
Utility patent records from the EPO and associated locational information are used to distribute knowledge production across the establishments of the firm, while the nature of technologies is assessed through Cooperative Patent Classification (CPC) technology classes. We focus on patents owned by multi-locational firms operating in the EU27 plus Switzerland, Iceland, Norway and the UK. EPO records do not directly link inventors to unique establishments in multi-unit firms. This can be an issue when the location of an inventor does not correspond with any of the locations over which a firm's establishments are distributed. We use establishment-level information from the BVD to identify all European locations in which multi-locational firms are active. A patent is assigned to one or more establishments of an assignee if the location of the patent inventor (or co-inventor) coincides with one of the locations of the assignee organization. In cases where a patent is assigned to firm X and a co-inventor on the patent is located in region A, if region A contains no establishment associated with firm X, then the patent is not allocated to region A. The geographical matching was performed at the NUTS2 level. Note that NUTS2 regions vary in size and in terms of the political units they represent. While some NUTS2 regions may demarcate city-regions, they more commonly capture broader subnational spaces, and in limited cases represent entire countries. The heterogeneity of these spatial units means that ascribing causation to the locational decisions of multi-plant businesses is difficult and thus some caution must be exercised interpreting the results presented.
The final sample covers 633 unique firms, 3045 establishments and 114,717 patents covering the years 2001–15. Note that some patents are produced by co-inventors located in different establishments within the firm. The sample includes firms with a minimum of two establishments that have patented in at least two consecutive 3-year time periods between the years 2001–15. Multi-year activity is required to generate measures of technological variance within establishments, required by MANOVA tests. The multi-locational firms in our sample account for over 25% of all patents granted in Europe in each time period.Footnote1 Summary statistics for firms in the sample are presented in . On average, sample firms control three establishments, distributed across two countries. Those firms produce an average of 52 patents with 62 different inventors.
Table 1. Summary statistics.
Empirical analysis
Do firms produce different technologies across their establishments?
The first part of the empirical analysis investigates whether there are statistically significant differences in the technological portfolios of establishments belonging to a multi-locational firm. That is, do firms produce different kinds of knowledge across the establishments that they control? A standard test would be an analysis of variance to compare the distribution of patents across technology classes within the establishments of a single firm. Because patents are distributed across 652 CPC technology classes, a multivariate analysis of variance (MANOVA) is required. However, a large majority of technology classes contain zero patents in most establishments and thus the assumptions of a standard MANOVA are violated. We therefore turn to a permutation based MANOVA (PERMANOVA), a non-parametric test that operates over a geometric partitioning of the variation in a dataset that may be linked to multiple factors. In our case, analysis focuses upon the variance in patent counts across CPC technology classes that is distributed within and between the establishments that comprise a single firm.
The geometric partitioning of patents across technology classes is defined in the space of a dissimilarity index. The dissimilarity measure employed in this paper is based on the technological relatedness between patent classes (Breschi, Lissoni, and Malerba Citation2003; Kogler, Rigby, and Tucker Citation2013). A relatedness score is generated for each pair of technology classes based on the co-occurrence of those classes across EPO patent records. The co-classification counts for CPC classes i and j are standardized in the form of a cosine index, yielding a measure of the relatedness or technological proximity between classes i and j in a given period:
The standardized proximity matrix
is subsequently used to calculate a dissimilarity score between all pairs of establishments by measuring the (inverse) average relatedness distance between the patents they generate across CPC classes. Class counts are aggregated into five three-year periods (2001–03, 2004–06, … , 2013–15) to smoothe annual fluctuations in patenting behaviour and to yield measures of the variance in technology within individual establishments.
The within-group variance is given by the technological dissimilarity of individual establishments between time periods, while the between-group variance is represented by the distance between the centroids of the establishments of a given multi-locational firm. Statistical inference is obtained by the permutation of patent observations across a firm's plants, from which we obtain a distribution of randomly generated F statistics. The null hypothesis is that the observed differences between the centroids of a firm's establishments are not statistically different than what would be observed if the patents were randomly distributed across establishments (Anderson Citation2017).
Due to the large size of the dissimilarity matrix and the limitations associated with interpreting a single test with a large number of firms, we perform separate PERMANOVA tests for each firm. The raw p-value and an adjusted p-value are reported because of repeated hypothesis testing. The adjusted p-value is calculated from the false discovery rate approach (fdr), which is similar to the Bonferroni correction but generally better suited to large-scale multiple testing. The PERMANOVA tests were performed using the ‘vegan’ package in R (Oksanen et al. Citation2020).
presents the results of the PERMANOVA tests grouped by firm size. Using the adjusted p-value, 68% of firms show differences in technology production across the plants they operate that are significant at the 0.1 level. However, the percentages vary considerably across group sizes. For firms with two patenting establishments, close to 40% produced significant results, and that percentage increased steadily with firm size to reach approximately 97% for firms with 11–20 establishments, and 100% for firms with more than 20 establishments. The results therefore indicate that firms controlling larger numbers of establishments tend to produce more differentiated subsets of knowledge across their plants than do firms controlling fewer establishments.
Table 2. Summary of firm-level PERMANOVA results.
Do the establishments of firms produce technologies that are related to the knowledge stocks of the regions where they are located (evidence of geographical knowledge sourcing)?
Using secondary data, it is difficult to test whether firms locate plants in particular regions to access specific kinds of knowledge. Indeed, it is likely that many factors shape the location decisions of multi-unit firms. However, if we can link the technologies generated within a firm's establishments to the knowledge stocks of regions where the establishments are located then we have at least identified a pattern of location consistent with the practice of geographical knowledge sourcing. We examine this linkage using a logistic regression model. To understand the structure of the model, assume that a firm comprises three establishments each located in a different city. From these data we generate nine observations where each establishment is associated with each of the three cities. For that set of observations, the dependent variable is coded 0 when the establishment is linked with a NUTS2 region that does not match its actual location, and the dependent variable is coded 1 when the establishment is linked with the NUTS2 region in which it is located. Our task is to predict when the dependent variable will assume the value 1. The key independent variable for this task is a measure of the technological similarity between the patents generated within each of the establishments and those generated within the set of NUTS2 regions with which the establishments are associated. The technological similarity variable is measured for each of the three-year time periods between 2001 and 2015 when a firm's establishments are producing patents.
The measure of technological similarity employed is a standardized count of the number of patent classes in which both the establishment and NUTS2 region exhibit revealed technological advantage (RTA). Following standard practice, RTA is recorded for a technology class in an establishment (region) when the share of patents in that class exceeds the share of a reference group. The reference group for establishments (regions) comprises the set of all establishments (regions) in our sample. The count of overlapping RTA values between establishments and regions is standardized as a cosine index (see Eck and Waltman Citation2009). The model of geographical knowledge sourcing, as applied to multi-plant businesses, suggests that firms distribute establishments to locations in order to access specific pools of knowledge. Thus, we anticipate a positive sign for the measure of technological similarity in our logit model. reveals the results of this statistical test.
Table 3. Logit model predicting region-establishment pairings based on technological similarity between establishments and NUTS2 regions.
The logit model is estimated with firm and time fixed effects and with robust standard errors. The results in show that there is a strong, positive relationship between the technological similarity of establishments and the NUTS2 regions in which they are located. This finding is significant at the 0.01 level and provides support for the idea that multi-unit firms distribute their R&D establishments over space to access specific knowledge subsets. While these results alone cannot confirm the practice of geographical knowledge sourcing, it is difficult to imagine an alternative scenario that would generate the results above alongside a pattern of knowledge development consistent with the technological demands of the firms examined.
Does geographical knowledge sourcing raise the complexity value of firm patents?
In general, we know that firms benefit from developing new technologies (Bettis and Hitt Citation1995; Roberts Citation1999). Those technologies may be generated entirely in-house, ‘sourced’ externally through various mechanisms, or developed as a hybrid of internal and external practices. More valuable forms of complex and tacit knowledge are difficult to move across geographical, organizational and institutional spaces (Amin and Cohendet Citation2004; Gertler Citation2003; Boschma Citation2005; Balland and Rigby Citation2017). As a consequence, it is argued that multi-unit firms exploit their organizational structure to embed establishments in different locations in order to access place-specific repositories of knowledge and lower the cost of integrating heterogeneous knowledge subsets (Cantwell Citation1989; Cantwell and Mudambi Citation2005; Meyer, Mudambi, and Narula Citation2011). An important question is whether multi-unit firms benefit from this activity. The empirical literature has so far provided mixed results on this issue. For instance, Singh (Citation2008) reported that the geographical dispersion of R&D in large multinational firms was negatively associated with the value of innovative output, as measured by the average number of forward citations to firm patents. Scalera, Perri, and Hannigan (Citation2018) report a positive relationship between the geographical dispersion of R&D and the technological scope of patents in US firms, both domestic and multinational, though this relationship is bounded by managerial bandwidth at the subnational level.
We contribute to this debate using a similar methodology to Singh (Citation2008). Unlike Singh (Citation2008), who uses forward citations to capture the (tacit knowledge) value of patents, we measure the value of patents using the complexity of the classes into which they are placed. We suspect that tacit knowledge dampens citations precisely because it is so difficult to move across spatial, organizational and institutional distances. The concept of economic complexity has recently been developed by Hidalgo and Hausmann (Citation2009) to value different kinds of economic activity. For them, complexity reflects the difficulty of producing various types of goods. Countries that have developed broad sets of capabilities specialize in the production of many types of goods, while countries with relatively few capabilities tend to specialize in only a few different sectors. Complex, high-value goods require many capabilities for their production and so tend to be produced in a relatively small number of countries. Conversely, less complex, lower valued goods tend to be produced in a much larger number of locations reflecting the broad availability of a smaller range of capabilities across different countries.
Measuring complexity requires patent data for CPC classes that are distributed over regions of interest. These data are used to build a bipartite (sector-by-region) network of innovation. The complexity measure of Hidalgo and Hausmann (Citation2009) represents the eigenvector centrality for such a bipartite network (He et al. Citation2016). Following Hidalgo and Hausmann (Citation2009), we estimate complexity scores for the 652 technology classes of the CPC. Individual patents make knowledge claims across a series of these classes. We calculate the complexity value of individual EU patents as the weighted average complexity of the technology classes in which their knowledge claims are reported. The resulting patent complexity values are standardized to fall over the interval 0–1. We argue that this proxy for the value of knowledge comes close to the idea of tacit knowledge, as it captures dimensions of rarity and non-replicability that benefit firms in their search for competitive advantage (Kogut and Zander Citation1992; Alcácer and Zhao Citation2012). A review of new work on economic complexity is provided by Balland et al. (Citation2022).
If multi-locational firms are able to integrate the knowledge developed across different localized knowledge pools, we expect that greater levels of geographical dispersion in knowledge sourcing will be positively associated with the production of more complex patents. We examine this issue using an OLS regression model where patents are linked to the firms and establishments in which they were created. The observations in the model are individual patents. We do not aggregate the value of patents by firm because we wish to control for a number of individual patent characteristics that are known to influence their value, such as the number of co-inventors (Ln inventor_count), the number of technology classes (Ln class_count) and the number of knowledge claims (Ln claim_count) they make (Breitzman and Thomas Citation2015). We measure the geographic distribution of R&D by counting the number of establishments over which a multi-plant firm distributes its patent production (Ln estab_count).
Sourcing knowledge from foreign locations could potentially broaden the scope of technologies and expertise involved in the innovation process. This is captured by a dichotomous variable taking the value 1 if there is a foreign inventor on a patent. If headquarters (HQ) establishments are the locations at which firms do most intra-firm knowledge integration (Mudambi Citation2002), we anticipate that HQ units might generate more valuable knowledge. Adding a dichotomous variable (HQ) indicating whether a patent is generated within an HQ establishment controls for this effect. Similarly, a binary variable indicates whether the plant where a patent is generated is a subsidiary (Sub) or not. A control for the size of the firm, the total number of patents generated (Ln firm_count), separates the influence of firm size from the spatial dispersion of a firm's R&D activities. Regional patent counts for each NUTS2 region where establishments are located (Ln nuts2_count), control for spatial variations in opportunities for knowledge sourcing, while the standardized measure of technological similarity between establishment and region knowledge stocks captures the influence of the quality of knowledge matching between establishment and region on patent value (Tech_similarity). This measure might also be thought of as a proxy for firm absorptive capacity (Cohen and Levinthal Citation1990). The regression models include fixed effects for the time-period and the primary class of patents to control for variations in patent complexity value over time and across technological sectors. Firm fixed effects help control for unobserved heterogeneity at the firm level. All continuous independent variables in the models are logged to handle issues of skew and standard errors are robust to heteroscedasticity. provides summary statistics for the continuous variables employed in the regression analysis. These statistics are generated for raw variables, before logging.
Table 4. Summary statistics for continuous variables in the regression analysis.
Results from the regression analysis are shown in . Note that these regressions are run across the full set of 114,717 patent observations. In cases where patents were split across co-inventors located in different establishments, the location of the first-listed co-inventor is used. , Model 1 shows that in the absence of covariates there is a positive and significant relationship between the log number of establishments over which a firm conducts its knowledge production and the average complexity value of the firm's patents. Model 2 adds covariates to Model 1 showing that larger numbers of CPC classes and larger numbers of inventors on individual patents are positively associated with complexity, while the number of knowledge claims on patents is negatively related to complexity. Patents that are produced with a foreign co-inventor tend to be significantly more complex. Model 2 also shows that there is no statistically significant relationship between HQ plants and average patent complexity, while subsidiaries tend to produce patents that are less complex than average within the firm. Greater levels of technological similarity between establishments and the regions where they are located increases patent complexity. Firm size, measured by the number of patents produced, is positively associated with more complex patents, while host region size is not significantly related to patent complexity. In Model 2, the relationship between the number of establishments over which a firm's R&D is distributed and the average complexity of patents produced remains positive and significant.
Table 5. Knowledge complexity and the extent of knowledge sourcing.
Following Singh (Citation2008), measures of intra-firm knowledge integration are proxied by dummies indicating whether the establishment that generates a patent cites patents produced by other establishments within the firm over the previous five years (Intra-firm citation), and whether the establishment that generates a patent has inventors that collaborated with inventors from other establishments of the firm in the preceding five years (Intra-firm collaboration). Examining these variables within the regression model should tell us whether integration of knowledge production activities within firms raises the value of knowledge output, and whether one of these measures of integration is more important than the other. The dummy variables for knowledge integration within the firm were also interacted with the firm's establishment count. Model 3 reveals that there is no statistically significant influence of within firm integration on patent complexity, measured by citations or co-inventor collaboration. The interaction effects of establishment count and the integration measures are similarly insignificant. These results suggest that higher levels of patent complexity are generated by firms when they locate establishments in places where the value of patents is higher than average, rather than as the outcome of integrating subsets of knowledge from different establishments. However, it may be the case that our analysis has not captured all forms of knowledge integration within the multi-unit firm. More work is required to investigate the origins of more complex and valuable patents, how and why knowledge complexity varies over space.
We ran a series of robustness checks on these results. A hierarchical regression model with establishments and firms representing two aggregate levels above the patent observations (captured as random effects) produced qualitatively similar findings. Including individual patents across the different locations at which their co-inventors are located increases the number of observations to 124,480. Running the regressions over this set of observations and using sample weights that give individual patents an equal value produced almost identical results to those reported in . Finally, using forward citations to measure patent value generated a negative and significant coefficient on the measure of a firm's R&D dispersion, echoing the results of Singh (Citation2008). Clearly, forward citations and patent complexity are not capturing the same form of knowledge value.
Conclusions
As we seek to understand the competitive advantage of firms and regions within the contemporary capitalist economy, the knowledge sourcing literature has taken on added importance. Within economic geography, that literature has tended to ignore the operations of multi-unit firms. This is surprising because firms likely adopt a multi-unit organizational structure, at least in part, to exploit the heterogeneous knowledge sourcing possibilities found in different places. The first research goal of this paper was to explore how multi-unit firms distribute their knowledge production/knowledge sourcing practices across establishments in different locations. Using a firm-patent database that allowed us to track the ultimate corporate ownership of patents, we found that firms use their branch plants and subsidiaries to develop different kinds of technologies. We have not identified earlier research that shows statistically significant evidence of subnational technological variations in patent production within the same firm. These differences become more pronounced as the number of establishments within the firm increase. How this technological variety is distributed between branches of the firm and its subsidiaries, and how it shapes the geography and history of firm and region knowledge stocks are important questions for future research.
A second research question examined links between the types of technology generated within the establishments of multi-unit firms and the knowledge stocks of the regions in which those establishments were located. Results revealed that the location of establishments within the firm could be predicted by the technological similarity of the patents produced in those establishments and that of the regions in which the firm's plants were located. This suggests to us that multi-unit firms take the geographic distribution of knowledge assets into account as they plan the location of their R&D activities. However, this does not mean that geographical knowledge sourcing is the only factor that might have influenced the locational choices of the multi-unit firms in our sample.
That our interpretation of the geography of patent data in multi-unit firms is credible would be strongly supported if these firms could be shown to benefit from knowledge sourcing. In economic geography, there is scant evidence that geographies of knowledge sourcing benefit firms. Within the international business literature, that evidence is mixed. The most compelling results from Singh (Citation2008) show that the international dispersion of firm R&D activities does not raise the forward citation value of firm patents. A third research question in this paper revisits this issue at the subnational level. We question the usefulness of forward citations as a proxy measure of tacit knowledge and suggest that the complexity of technology is a better measure of patent value. Our results show that the more a firm spreads knowledge production across establishments located in different NUTS2 regions of Europe, the greater the average complexity value of the firm's patents. There are at least two mechanisms that might explain this finding. First, that the integration of knowledge produced across establishments leads to more complex patents on average. Second, that by spreading technology development across different centres of innovation, firms might be able to tap into more complex and valuable subsets of knowledge. The integration story is not supported by citation or collaboration linkages between a firm's establishments. More work is clearly required to understand how complex knowledge is discovered and captured by the firm.
Disclosure statement
No potential conflict of interest was reported by the author(s).
Notes
1 Before applying the restrictions to generate the final sample for the analysis, multi-locational firms account for about half of all patents generated in Europe, as defined, between 2001 and 2015.
References
- Alcácer, J., and W. Chung. 2014. “Location Strategies for Agglomeration Economies.” Strategic Management Journal 35 (12): 1749–1761. doi:10.1002/smj.2186.
- Alcácer, J., and M. Zhao. 2012. “Local R&D Strategies and Multilocation Firms: The Role of Internal Linkages.” Management Science 58 (4): 734–753. doi:10.1287/mnsc.1110.1451.
- Almeida, P. 1996. “Knowledge Sourcing by Foreign Multinationals: Patent Citation Analysis in the U.S. Semiconductor Industry.” Strategic Management Journal 17 (S2): 155–165. doi:10.1002/smj.4250171113.
- Almeida, P., and B. Kogut. 1999. “Localization of Knowledge and the Mobility of Engineers in Regional Networks.” Management Science 45 (7): 905–917. doi:10.1287/mnsc.45.7.905.
- Almeida, P., and A. Phene. 2004. “Subsidiaries and Knowledge Creation: The Influence of the MNC and Host Country on Innovation.” Strategic Management Journal 25 (89): 847–864. doi:10.1002/smj.388.
- Amin, A., and P. Cohendet. 1999. “Learning and Adaptation in Decentralised Business Networks.” Environment and Planning D: Society and Space 17 (1): 87–104. doi:10.1068/d170087.
- Amin, A., and P. Cohendet. 2004. Architectures of Knowledge: Firms, Capabilities, and Communities. . Oxford: Oxford University Press.
- Anderson, M. 2017. “Permutational Multivariate Analysis of Variance (PERMANOVA).” Wiley Statsref: Statistics Reference Online, 1–15. doi:10.1002/9781118445112.stat07841.
- Arrow, K. 1962. “The Economic Implications of Learning by Doing.” Review of Economic Studies 29: 155–173.
- Asheim, B., and L. Coenen. 2005. “Knowledge Bases and Regional Innovation Systems: Comparing Nordic Clusters.” Research Policy 34 (8): 1173–1190. doi:10.1016/j.respol.2005.03.013.
- Asheim, B., and M. Gertler. 2005. “The Geography of Innovation: Regional Innovation Systems.” In The Oxford Handbook of Innovation, edited by J. Fagerberg, D. Mowery, and R. Nelson, 291–317. Oxford: Oxford University Press.
- Audretsch, D., and M. Feldman. 1996. “R&D Spillovers and the Geography of Innovation and Production.” The American Economic Review 86 (3): 630–640.
- Balland, P., T. Broekel, D. Diodato, E. Giuliani, R. Hausmann, N. O’Clery, and D. Rigby. 2022. “The New Paradigm of Economic Complexity.” Research Policy 51 (3): 104450. doi:10.1016/j.respol.2021.104450.
- Balland, P., C. Jara-Figueroa, S. Petralia, M. Steijn, D. Rigby, and C. Hidalgo. 2020. “Complex Economic Activities Concentrate in Large Cities.” Nature Human Behaviour 4 (3): 248–254. doi:10.1038/s41562-019-0803-3.
- Balland, P., and D. Rigby. 2017. “The Geography of Complex Knowledge.” Economic Geography 93 (1): 1–23. doi:10.1080/00130095.2016.1205947.
- Bathelt, H. 2007. “Buzz-and-Pipeline Dynamics: Towards a Knowledge-Based Multiplier Model of Clusters.” Geography Compass 1 (6): 1282–1298. doi:10.1111/j.1749-8198.2007.00070.x.
- Bathelt, H., A. Malmberg, and P. Maskell. 2004. “Clusters and Knowledge: Local Buzz, Global Pipelines and the Process of Knowledge Creation.” Progress in Human Geography 28 (1): 31–56. doi:10.1191/0309132504ph469oa.
- Becattini, G. 1991. “Italian Industrial Districts: Problems and Perspectives.” International Studies of Management & Organization 21 (1): 83–90. doi:10.1080/00208825.1991.11656551.
- Berry, H. 2015. “Knowledge Inheritance in Global Industries: The Impact of Parent Firm Knowledge on the Performance of Foreign Subsidiaries.” Academy of Management Journal 58 (5): 1438–1458. doi:10.5465/amj.2013.0724.
- Bettis, R., and M. Hitt. 1995. “The New Competitive Landscape.” Strategic Management Journal 16 (S1): 7–19. doi:10.1002/smj.4250160915.
- Boschma, R. 2005. “Proximity and Innovation: A Critical Assessment.” Regional Studies 39 (1): 61–74. doi:10.1080/0034340052000320887.
- Braczyk, H., and M. Heidenreich. 1998. “Regional Governance Structures in a Globalized World.” In Regional Innovation Systems, edited by H. Braczyk, and M. Heienreich, 414–440. London: UCL Press.
- Bramwell, A., and D. Wolfe. 2008. “Universities and Regional Economic Development: The Entrepreneurial University of Waterloo.” Research Policy 37 (8): 1175–1187. doi:10.1016/j.respol.2008.04.016.
- Breitzman, A., and P. Thomas. 2015. “Inventor Team Size as a Predictor of the Future Citation Impact of Patents.” Scientometrics 103 (2): 631–647. doi:10.1007/s11192-015-1550-5.
- Breschi, S., F. Lissoni, and F. Malerba. 2003. “Knowledge-Relatedness in Firm Technological Diversification.” Research Policy 32 (1): 69–87.
- Brown, J., and P. Duguid. 1991. “Organizational Learning and Communities-of-Practice: Toward a Unified View of Working, Learning, and Innovation.” Organization Science 2 (1): 40–57. doi:10.1287/orsc.2.1.40.
- Brusco, S. 1986. “Small Firms and Industrial Districts: The Experience of Italy.” In New Firms and Regional Development in Europe, edited by D. Keeble, and E. Wever, 184–202. London: Croom Helm.
- Camagni, R. 1991. “Local’milieu’, Uncertainty and Innovation Networks: Towards a Dynamic Theory of Economic Space.” In Innovation Networks: Spatial Perspectives, edited by R. Camagni, 121–144. London: Belhaven Press.
- Cantwell, J. 1989. Technological Innovation and Multinational Corporations. Oxford: Blackwell.
- Cantwell, J., and S. Iammarino. 2001. “EU Regions and Multinational Corporations: Change, Stability and Strengthening of Technological Comparative Advantages.” Industrial and Corporate Change 10 (4): 1007–1037. doi:10.1093/icc/10.4.1007.
- Cantwell, J., and S. Iammarino. 2003. Multinational Corporations and European Regional Systems of Innovation. London: Routledge.
- Cantwell, J., and R. Mudambi. 2005. “MNE Competence-Creating Subsidiary Mandates.” Strategic Management Journal 26 (12): 1109–1128. doi:10.1002/smj.497.
- Cantwell, J., and R. Mudambi. 2011. “Physical Attraction and the Geography of Knowledge Sourcing in Multinational Enterprises.” Global Strategy Journal 1 (3-4): 206–232. doi:10.1002/gsj.24.
- Castellani, D., K. Lavoratori, A. Perri, and V. Scalera. 2022. “International Connectivity and the Location of Multinational Enterprises’ Knowledge-Intensive Activities: Evidence from US Metropolitan Areas.” Global Strategy Journal 12 (1): 82–107. doi:10.1002/gsj.1404.
- Chesbrough, H. 2003. Open Innovation: The New Imperative for Creating and Profiting from Technology. Cambridge, MA: Harvard Business Press.
- Chung, W., and J. Alcácer. 2002. “Knowledge Seeking and Location Choice of Foreign Direct Investment in the United States.” Management Science 48 (12): 1534–1554. doi:10.1287/mnsc.48.12.1534.440.
- Cohen, W., and D. Levinthal. 1990. “Absorptive Capacity: A New Perspective on Learning and Innovation.” Administrative Science Quarterly 35 (1): 128–152. doi:10.2307/2393553.
- Cooke, P. 2002. “Regional Innovation Systems: General Findings and Some New Evidence from Biotechnology Clusters.” The Journal of Technology Transfer 27 (1): 133–145. doi:10.1023/A:1013160923450.
- Cooke, P., P. Boekholt, and F. Tödtling. 2000. The Governance of Innovation in Europe: Regional Perspectives on Global Competitiveness. London: Pinter.
- Crescenzi, R., A. Dyèvre, and F. Neffke. 2022. “Innovation Catalysts: How Multinationals Reshape the Global Geography of Innovation.” Economic Geography 98: 199–227. doi:10.1080/00130095.2022.2026766.
- Dunning, J. 1977. “Trade, Location of Economic Activity and the MNE: A Search for an Eclectic Approach.” In The International Allocation of Economic Activity, edited by P. Hesselborn, B. Ohlin, and P. Wijkman, 395–418. London: Palgrave Macmillan.
- Dunning, J. 1998. “Location and the Multinational Enterprise: A Neglected Factor?” Journal of International Business Studies 29 (1): 45–66. doi:10.1057/palgrave.jibs.8490024.
- Duranton, G., and D. Puga. 2001. “Nursery Cities: Urban Diversity, Process Innovation, and the Life Cycle of Products.” American Economic Review 91 (5): 1454–1477. doi:10.1257/aer.91.5.1454.
- Eck, N., and L. Waltman. 2009. “How to Normalize Cooccurrence Data? An Analysis of Some Well-Known Similarity Measures.” Journal of the American Society for Information Science and Technology 60 (8): 1635–1651. doi:10.1002/asi.21075.
- Elekes, Z., R. Boschma, and B. Lengyel. 2019. “Foreign-owned Firms as Agents of Structural Change in Regions.” Regional Studies 53 (11): 1603–1613. doi:10.1080/00343404.2019.1596254.
- Feldman, M. 1994. The Geography of Innovation. Boston, MA: Kluwer Academic.
- Fitjar, R., and A. Rodríguez-Pose. 2013. “Firm Collaboration and Modes of Innovation in Norway.” Research Policy 42 (1): 128–138. doi:10.1016/j.respol.2012.05.009.
- Fitjar, R., and A. Rodríguez-Pose. 2020. “Where Cities Fail to Triumph: The Impact of Urban Location and Local Collaboration on Innovation in Norway.” Journal of Regional Science 60 (1): 5–32. doi:10.1111/jors.12461.
- Fritsch, M., and C. Schwirten. 1999. “Enterprise-University Co-Operation and the Role of Public Research Institutions in Regional Innovation Systems.” Industry and Innovation 6 (1): 69–83. doi:10.1080/13662719900000005.
- Gertler, M. 2003. “Tacit Knowledge and the Economic Geography of Context, or the Undefinable Tacitness of Being (There).” Journal of Economic Geography 3 (1): 75–99. doi:10.1093/jeg/3.1.75.
- Ghoshal, S., and C. Bartlett. 1988. “Creation, Adoption and Diffusion of Innovations by Subsidiaries of Multinational Corporations.” Journal of International Business Studies 19 (3): 365–388. doi:10.1057/palgrave.jibs.8490388.
- Giuliani, E. 2013. “Network Dynamics in Regional Clusters: Evidence from Chile.” Research Policy 42 (8): 1406–1419. doi:10.1016/j.respol.2013.04.002.
- Goerzen, A., C. Asmussen, and B. Nielsen. 2013. “Global Cities and Multinational Enterprise Location Strategy.” Journal of International Business Studies 44 (5): 427–450. doi:10.1057/jibs.2013.11.
- Grillitsch, M., and M. Trippl. 2014. “Combining Knowledge from Different Sources, Channels and Geographical Scales.” European Planning Studies 22 (11): 2305–2325. doi:10.1080/09654313.2013.835793.
- He, X., M. Gao, M.-Y. Kan, and D. Wang. 2016. “BiRank: Towards Ranking on Bipartite Graphs.” IEEE Transactions on Knowledge and Data Engineering. Submission 2016: 1–15.
- Hidalgo, C., and R. Hausmann. 2009. “The Building Blocks of Economic Complexity.” Proceedings of the National Academy of Sciences 106 (26): 10570–10575. doi:10.1073/pnas.0900943106.
- Huggins, R., H. Izushi, D. Prokop, and P. Thompson. 2015. “Network Evolution and the Spatiotemporal Dynamics of Knowledge Sourcing.” Entrepreneurship & Regional Development 27 (7-8): 474–499. doi:10.1080/08985626.2015.1070538.
- Iammarino, S., and P. McCann. 2013. Multinationals and Economic Geography: Location, Technology and Innovation. Cheltenham: Edward Elgar.
- Isaksen, A., and M. Trippl. 2017. “Exogenously Led and Policy-Supported New Path Development in Peripheral Regions: Analytical and Synthetic Routes.” Economic Geography 93 (5): 436–457. doi:10.1080/00130095.2016.1154443.
- Jaffe, A., M. Trajtenberg, and R. Henderson. 1993. “Geographic Localization of Knowledge Spillovers as Evidenced by Patent Citations.” The Quarterly Journal of Economics 108 (3): 577–598. doi:10.2307/2118401.
- Jensen, M., B. Johnson, E. Lorenz, B. Lundvall, and B. Lundvall. 2007. “Forms of Knowledge and Modes of Innovation.” Research Policy 36 (5): 680–693. doi:10.1016/j.respol.2007.01.006.
- Jindra, B., S. Hassan, and U. Cantner. 2016. “What Does Location Choice Reveal About Knowledge-Seeking Strategies of Emerging Market Multinationals in the EU?” International Business Review 25 (1): 204–220. doi:10.1016/j.ibusrev.2014.11.008.
- Kogler, D., D. Rigby, and I. Tucker. 2013. “Mapping Knowledge Space and Technological Relatedness in US Cities.” European Planning Studies 21 (9): 1374–1391. doi:10.1080/09654313.2012.755832.
- Kogut, B., and U. Zander. 1992. “Knowledge of the Firm, Combinative Capabilities, and the Replication of Technology.” Organization Science 3 (3): 383–397. doi:10.1287/orsc.3.3.383.
- Laursen, K., T. Reichstein, and A. Salter. 2011. “Exploring the Effect of Geographical Proximity and University Quality on University–Industry Collaboration in the United Kingdom.” Regional Studies 45 (4): 507–523. doi:10.1080/00343400903401618.
- Lawson, C., and E. Lorenz. 1999. “Collective Learning, Tacit Knowledge and Regional Innovative Capacity.” Regional Studies 33 (4): 305–317. doi:10.1080/713693555.
- Li, P., and H. Bathelt. 2018. “Location Strategy in Cluster Networks.” Journal of International Business Studies 49 (8): 967–989. doi:10.1057/s41267-017-0088-6.
- Li, Y., and D. Rigby. 2022. “Relatedness, Complexity, and Economic Growth in Chinese Cities.” International Regional Science Review. doi:10.1177/01600176221082308.
- Lorentzen, A. 2007. “The Geography of Knowledge Sourcing—A Case Study of Polish Manufacturing Enterprises.” European Planning Studies 15 (4): 467–486. doi:10.1080/09654310601133252.
- Lo Turco, A., and D. Maggioni. 2016. “On Firms’ Product Space Evolution: The Role of Firm and Local Product Relatedness.” Journal of Economic Geography 16 (5): 975–1006. doi:10.1093/jeg/lbv024.
- Lo Turco, A., and D. Maggioni. 2019. “Local Discoveries and Technological Relatedness: The Role of MNEs, Imports and Domestic Capabilities.” Journal of Economic Geography 19 (5): 1077–1098. doi:10.1093/jeg/lby060.
- Lundvall, B., and B. Johnson. 1994. “The Learning Economy.” Journal of Industry Studies 1 (2): 23–42. doi:10.1080/13662719400000002.
- Marshall, A. 1890. The Principles of Economics. London: Macmillan.
- Martin, R., and J. Moodysson. 2011. “Innovation in Symbolic Industries: The Geography and Organization of Knowledge Sourcing.” European Planning Studies 19 (7): 1183–1203. doi:10.1080/09654313.2011.573131.
- Martin, R., and J. Moodysson. 2013. “Comparing Knowledge Bases: On the Geography and Organization of Knowledge Sourcing in the Regional Innovation System of Scania, Sweden.” European Urban and Regional Studies 20 (2): 170–187. doi:10.1177/0969776411427326.
- Maskell, P., and A. Malmberg. 1999. “Localised Learning and Industrial Competitiveness.” Cambridge Journal of Economics 23 (2): 167–185. doi:10.1093/cje/23.2.167.
- Mewes, L. 2019. “Scaling of Atypical Knowledge Combinations in American Metropolitan Areas from 1836 to 2010.” Economic Geography 95 (4): 341–361. doi:10.1080/00130095.2019.1567261.
- Mewes, L., and T. Broekel. 2022. “Technological Complexity and Economic Growth of Regions.” Research Policy 51 (8): 104156. doi:10.1016/j.respol.2020.104156.
- Meyer, K., R. Mudambi, and R. Narula. 2011. “Multinational Enterprises and Local Contexts: The Opportunities and Challenges of Multiple Embeddedness.” Journal of Management Studies 48 (2): 235–252. doi:10.1111/j.1467-6486.2010.00968.x.
- Morgan, K. 2007. “The Learning Region: Institutions, Innovation and Regional Renewal.” Regional Studies 41 (S1): S147–S159. doi:10.1080/00343400701232322.
- Mudambi, R. 2002. “Knowledge Management in Multinational Firms.” Journal of International Management 8 (1): 1–9.
- Muller, E., and D. Doloreux. 2009. “What We Should Know About Knowledge-Intensive Business Services.” Technology in Society 31 (1): 64–72. doi:10.1016/j.techsoc.2008.10.001.
- Neffke, F., M. Hartog, R. Boschma, and M. Henning. 2018. “Agents of Structural Change: The Role of Firms and Entrepreneurs in Regional Diversification.” Economic Geography 94 (1): 23–48. doi:10.1080/00130095.2017.1391691.
- Neilsen, B., C. Asmusen, and A. Goerzen. 2018. “Toward a Synthesis of Micro and Macro Factors That Influence Foreign Direct Investment Location Choice.” In The Routledge Companion to the Geography of International Business, edited by G. Cook, J. Johns, F. McDonald, J. Beaverstock, and N. Pandit, 190–212. New York: Routledge.
- Nonaka, I., and H. Takeuchi. 1995. The Knowledge-creating Company: How Japanese Companies Create the Dynamics of Innovation. New York: Oxford University Press.
- Oksanen, J., F. Blanchet, M. Friendly, R. Kindt, P. Legendre, D. McGlinn, P. Minchin, et al. 2020. “vegan: Community Ecology Package.” R Package Version 2: 5–7.
- Pavitt, K. 1991. “Key Characteristics of the Large Innovating Firm.” British Journal of Management 2 (1): 41–50. doi:10.1111/j.1467-8551.1991.tb00014.x.
- Pintar, N., and T. Scherngell. 2022. “The Complex Nature of Regional Knowledge Production: Evidence on European Regions.” Research Policy 51 (8): 104170. doi:10.1016/j.respol.2020.104170.
- Polanyi, M. 1966. The Tacit Dimension. New York: Doubleday.
- Porter, M. 1990. The Competitive Advantage of Nations. New York: Free Press.
- Porter, M. 1998. “Clusters and the New Economics of Competition.” Harvard Business Review 76 (6): 77–90.
- Powell, W., K. Koput, and L. Smith-Doerr. 1996. “Interorganizational Collaboration and the Locus of Innovation: Networks of Learning in Biotechnology.” Administrative Science Quarterly 41: 116–145. doi:10.2307/2393988.
- Rigby, D., and J. Essletzbichler. 1997. “Evolution, Process Variety, and Regional Trajectories of Technological Change in U.S. Manufacturing.” Economic Geography 73 (3): 269–284. doi:10.2307/144484.
- Rigby, D., C. Roesler, D. Kogler, R. Boschma, and P. Balland. 2022. “Do EU Regions Benefit from Smart Specialisation Principles?” Regional Studies, 1–16. doi:10.1080/00343404.2022.2032628.
- Roberts, P. 1999. “Product Innovation, Product-Market Competition and Persistent Profitability in the U.S. Pharmaceutical Industry.” Strategic Management Journal 20 (7): 655–670. doi:10.1002/(SICI)1097-0266(199907)20:7<655::AID-SMJ44>3.0.CO;2-P.
- Saxenian, A. 1994. Regional Advantage: Culture and Competition in Silicon Valley and Route 128. Cambridge, MA: Harvard University Press.
- Scalera, V., A. Perri, and T. Hannigan. 2018. “Knowledge Connectedness within and Across Home Country Borders: Spatial Heterogeneity and the Technological Scope of Firm Innovations.” Journal of International Business Studies 49 (8): 990–1009. doi:10.1057/s41267-017-0109-5.
- Shearmur, R., and D. Doloreux. 2019. “KIBS as Both Innovators and Knowledge Intermediaries in the Innovation Process: Intermediation as a Contingent Role.” Papers in Regional Science 98 (1): 191–209. doi:10.1111/pirs.12354.
- Singh, J. 2008. “Distributed R&D, Cross-Regional Knowledge Integration and Quality of Innovative Output.” Research Policy 37 (1): 77–96. doi:10.1016/j.respol.2007.09.004.
- Sonn, J., and M. Storper. 2008. “The Increasing Importance of Geographical Proximity in Knowledge Production: An Analysis of US Patent Citations, 1975–1997.” Environment and Planning A: Economy and Space 40 (5): 1020–1039. doi:10.1068/a3930.
- Sorenson, O., J. Rivkin, and L. Fleming. 2006. “Complexity, Networks and Knowledge Flow.” Research Policy 35 (7): 994–1017. doi:10.1016/j.respol.2006.05.002.
- Sorenson, O., and T. Stuart. 2001. “Syndication Networks and the Spatial Distribution of Venture Capital Investments.” American Journal of Sociology 106 (6): 1546–1588. doi:10.1086/321301.
- Storper, M. 1995. “The Resurgence of Regional Economies, Ten Years Later: The Region as a Nexus of Untraded Interdependencies.” European Urban and Regional Studies 2 (3): 191–221. doi:10.1177/096977649500200301.
- Storper, M., and A. Venables. 2004. “Buzz: Face-to-Face Contact and the Urban Economy.” Journal of Economic Geography 4 (4): 351–370. doi:10.1093/jnlecg/lbh027.
- Ter Wal, A., and R. Boschma. 2009. “Applying Social Network Analysis in Economic Geography: Framing Some Key Analytic Issues.” The Annals of Regional Science 43 (3): 739–756. doi:10.1007/s00168-008-0258-3.
- Tödtling, F., M. Grillitsch, and C. Hoeglinger. 2012. “Knowledge Sourcing and Innovation in Austrian ICT Companies—How Does Geography Matter?” Industry & Innovation 19 (4): 327–348. doi:10.1080/13662716.2012.694678.
- Tödtling, F., P. Lehner, and M. Trippl. 2006. “Innovation in Knowledge Intensive Industries: The Nature and Geography of Knowledge Links.” European Planning Studies 14 (8): 1035–1058. doi:10.1080/09654310600852365.
- Tödtling, F., L. Lengauer, and C. Höglinger. 2011. “Knowledge Sourcing and Innovation in “Thick” and “Thin” Regional Innovation Systems—Comparing ICT Firms in Two Austrian Regions.” European Planning Studies 19 (7): 1245–1276. doi:10.1080/09654313.2011.573135.
- Trippl, M. 2011. “Regional Innovation Systems and Knowledge-Sourcing Activities in Traditional Industries—Evidence from the Vienna Food Sector.” Environment and Planning A: Economy and Space 43 (7): 1599–1616. doi:10.1068/a4416.
- Trippl, M., F. Tödtling, and L. Lengauer. 2009. “Knowledge Sourcing Beyond Buzz and Pipelines: Evidence from the Vienna Software Sector.” Economic Geography 85 (4): 443–462. doi:10.1111/j.1944-8287.2009.01047.x.
- Von Hippel, E. 1988. Sources of Innovation. Oxford: Oxford University Press.