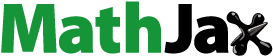
ABSTRACT
Communication of International Large-Scale Assessment (ILSA) results is dominated by reporting average country achievement scores that conceal individual differences between pupils, schools, and items. Educational research primarily focuses on examining differences between pupils and schools, while differences between items are overlooked. Using a variance components model on the Programme for International Student Assessment (PISA) 2018 cognitive domains of reading, mathematics, and science literacy, we estimated how much of the response variation can be attributed to differences between pupils, schools, and items. The results show that uniformly across domains and countries, it mattered more for the correctness of an item response which items were responded to by a pupil (27–35%) than which pupil responded to these items (10–12%) or which school the pupil attended (5–7%). Given the findings, we argue that differences between items in ILSAs constitute a source of substantial untapped potential for secondary research.
International Large Scale Assessments (ILSAs), such as the Programme for International Student Assessment (PISA) by the Organisation for Economic Co-operation and Development (OECD) and the Trends in International Mathematics and Science Study (TIMSS) by the International Association for the Evaluation of Educational Achievement (IEA), have been increasingly used for comparing educational outcomes around the globe (Mullis et al., Citation2020; OECD, Citation2019). Despite the ample research opportunities the sheer magnitude of the data collected in these assessments offers, ILSA results are commonly communicated as simplified rankings of countries’ average scores on various cognitive domains. The simple average scores conceal potentially informative differences and consequently can distort our understanding of the inherent complexities of the educational processes and contexts. Secondary ILSA research is well attuned to the importance of recognising and investigating these differences between pupils, and their respective schools, as they offer valuable insight into the social, economic, and cultural contexts within which the ILSA results can be meaningfully interpreted. Rather than relying on a single average score, researchers focus on establishing the magnitude of the inter-individual differences by quantifying the variance around that average and attempting to explain said variance by considering a range of covariates (for a review, see, e.g., Hopfenbeck et al., Citation2018).
The countries’ average scores, however, are not only averaged across pupils and schools but also across items. Similarly to the inter-individual differences in performance between pupils (and schools), the country average obscures differences that may exist between items within a certain domain. When viewing the performance through the lens of this average, we operate under an unrealistic assumption that, for instance, in low-performing countries, the pupils score low on all of the items covering a domain. In reality, however, both higher- and lower-performing countries can have their weaknesses and relative strengths such that some items are more or less difficult for pupils.
The magnitude of the difficulty differences between items translates into systematic response variation across items (henceforth, ‘item variance’), a topic not often discussed in the current ILSA research. Driven by the prestige of examining pupil performance on blanket constructs, such as reading, mathematics, and science, we tend to take ILSA’s labelling and meaning of these constructs at their face value, without a second thought as to the items that measure them (i.e., the naming fallacy, see, e.g., Kline, Citation2016). The issue is further exacerbated in the secondary analysis, where the availability of the plausible values as measures of pupil achievement allows us to avoid immediate item responses altogether.
Given the breadth of the ILSAs cognitive constructs, we hypothesise that the item variance may be substantial. The reasoning behind this hypothesis is fairly intuitive. That is, the narrower a construct is, the less we would anticipate variance in the items that measure said construct. Conversely, holding test population and testing conditions equal, but moving from a narrow construct to broader constructs such as reading, mathematics, and science, a larger item variance can be expected. Such large item variance would imply substantial differences between items’ difficulties within a construct and call into question whether an average score across all items is a sufficient summary for the entire cognitive domain.
The information to be gained from quantifying the item variance and examining the factors affecting its magnitude could provide more targeted country performance profiles and align with the needs of the educators, curriculum designers, and test developers alike. The items targeting specific content, which prove to be harder or easier for most pupils, could help the educators anticipate the weaker and stronger areas in the curriculum (El Masri et al., Citation2017). Furthermore, the knowledge of the factors affecting item variance could help the test developers and item writers produce questions of higher validity and effectiveness in measuring the constructs (Ahmed & Pollitt, Citation2007; Eijkelhof et al., Citation2013; Le Hebel et al., Citation2017).
Given that the research on the item variance in ILSA is sparse, the study warrants an exploratory approach (Tukey, Citation1980). We do not yet intend to explain the item variation or link it to internal or external factors and item characteristics. Instead, we begin by laying the foundation by quantifying the magnitude of the item variance and identifying potential points of interest and curious patterns that will ultimately generate hypotheses for future inquiry. Using one of the most recent ILSAs, PISA 2018, as a working example, we estimate the so-called variance components or the magnitude of response variation attributable to three key sources of variation – schools, pupils, and items –, for each of the three cognitive domains – reading, mathematical, and science literacy –, in each of the participating countries. We address the following research objectives (RO).
Describe the across-country patterns in variance component profiles.
The objective is two-fold and comprises (i) the assessment of the relative importance of response variation sources and (ii) their consistency across countries and cognitive domains. The latter helps generalise as well as identify distinct country profiles that could invite further research.
Quantify the relative magnitude of response variation due to differences between items (i.e., differences in item difficulties) compared to that due to differences between schools and pupils.
The resulting magnitudes directly address the main concern raised in the current article. That is, the relative magnitudes of the item to person variances will put to the test our hypothesis of substantial item variance in the PISA cognitive domains and either support or oppose our call for more research into the blind side of ILSAs, the items.
Method
The present study viewed the total variance in a pupil’s response on a PISA 2018 cognitive domain item as a composition of two main variance sources, the person and the item. illustrates this notion where responses, the lower-level data units, belong to a pair resulting from crossing two higher-level data units: a tandem of pupils nested in their respective schools on the person side and items on the item side. Hence, a single response was considered a combination of both sides, with each pupil nested within one school responding to several items and each item being responded to by several pupils, reflecting the cross-classified data structure (Van den Noortgate et al., Citation2003).
Following a random-person random-item Item Response Theory (IRT) approach (De Boeck, Citation2008; Rijmen et al., Citation2003), a cross-classified mixed effects model was formulated. The total response variance was partitioned into components attributable to different sources of variation in the data structure (Briggs & Wilson, Citation2007). We allow the probability of a correct response to vary across pupils, schools, and items, and define the core model as
where is the probability that pupil
from school
will answer item
correctly;
is the overall intercept (fixed effect) corresponding to the estimated logit for the probability of a correct response of an average pupil from an average school on an average item;
,
,
are the varying intercepts (random effects) for pupil, school, and item, respectively. The varying intercepts were assumed to follow an independent normal distribution with means fixed to zero and variances
,
, and
, respectively. Hence, the model effectively counted four freely estimated parameters. The three varying intercepts reflected three main effects in a variance partitioning model (i.e., one per source of variance in the data structure), indicating how responses from a specific pupil, school, or item deviated, on average, from the overall response given by an average pupil from an average school on an average item.
The model in EquationEquation 1(1)
(1) implies that the total observed response variation (
) can be partitioned into four parts
where ,
,
correspond to the variances of the pupil, school, and item varying intercepts, and
is the distribution-specific residual variance from the standard logistic distribution due to the applied link function accounting for the binary nature of a response. Applying this model to the PISA 2018 item response data allowed us to derive, across countries and the PISA 2018 cognitive domains, two sets of outcome measures to address our two core research objectives: source-specific variance components and item to person variance components ratios.
Sample
The PISA 2018 item response data for the reading, mathematical and science literacy domains were used in the study. A total of over 45.5 million responses given by approximately 600,000 pupils from over 21,000 schools and 77 countries on nearly 800 items were considered.
PISA 2018 pupils and schools
Of 79 countries and economies that initially participated in the PISA 2018, the current study considered 77. Excluded were Cyprus, due to lack of available data, and Vietnam due to discrepancies in the data comparability addressed in detail in the PISA 2018 technical report (OECD, Citation2020). The total sample size included approximately 600,000 pupils from over 21,000 schools. The PISA 2018 sampling design prescribed to sample, population size permitting, 5250 to 6300 15-year-old pupils from a minimum of 150 schools per participating country (OECD, Citation2020). Country-wise sample sizes varied from 3296 pupils in Iceland to 35,943 in Spain and from 44 schools sampled in Luxembourg to 1089 schools in Spain. , , in Appendix A give pupil and school sample sizes for each of the cognitive domains and considered countries.
PISA 2018 items
PISA 2018 was primarily delivered as a computer-based (CBA) assessment. Sixty-nine countries took the CBA, whereas eight countries participated in the paper-based (PBA) version. The total number of items delivered in each country varied as a function of the administration mode and achievement domain. gives the total numbers of items for the CBA and PBA versions, and the totals are further decomposed into the common items administered in all countries within a mode and the unique items administered only to specific subsets of countries (Appendix B). The major domain of the PISA 2018, reading literacy, included 318 CBA items and 103 PBA items. The minor domains of mathematics and science comprised 115 and 82 CBA items, respectively, and 83 PBA items each. , , in Appendix A give country-specific numbers of items across the domains. In order to reduce test length and minimise pupil fatigue, PISA implemented a rotated booklet design in which each pupil only responded to a subset of items, and each item was responded to by a subset of pupils. Subsequently, each pupil responded on average to roughly 50 items in the reading domain and 24 items in one or two of the minor domains – mathematics, science or global competence. Each reading, mathematics, and science item, on the other hand, was responded to by over 650, 420, and 550 pupils, respectively.
Outcome measures
To address the first research objective, variance components for pupils, schools, and items were computed as the ratios of the specific variance source (i.e., pupil, school or item) to the total variation defined in EquationEquation 2(2)
(2) , reflecting their relative contributions to the overall response variance composition:
To address the second research objective and showcase the magnitude of the item side variance as compared to the person side, ratios of the item to the person variance components (i.e., item to the combined pupil and school variance components) were computed:
where the common denominators of the variance components (as seen in EquationEquation 3)(3)
(3) cancel out simplifying the expression to the ratio of the respective variances.
Statistical Analysis
The cross-classified mixed effects model represented in EquationEquation 1(1)
(1) was fitted to each country’s item response data for each of the three cognitive domains separately (i.e., a total of
model applications) using a marginal maximum likelihood estimation approach. The analysis was conducted using the lme4 package (Bates et al., Citation2015) in version 4.0.3 of the R software environment (R Core Team, Citation2020). Prior to model application, for 59 items across domains that allowed partial credit, partial credit was recoded into no credit, such that all the responses were dichotomously scored (i.e., correct or incorrect), facilitating comparability across items.
Variance components and variance components ratios were computed based on the models’ estimated parameters, summarised across countries, and their consistency across domains was examined. As a final step, two sensitivity analyses were performed. The first analysis addressed potential comparability issues due to country-specific item pools. All models and outcomes of interest were re-estimated using only the common-for-all-countries items. The second analysis tested the robustness of the results when taking other approaches to partial credit response handling. The alternative analyses (1) considered partial credit responses as correct, or (2) omitted partial credit items. The variance components were re-computed and compared to the original variance components (i.e., where partial credit responses were coded as incorrect). The results of the sensitivity analyses are presented in Appendix C.
Results
report the estimated parameters of the cross-classified mixed effects model applied to the response data of the PISA 2018 assessments of reading, mathematical, and science literacy (Appendix A). Those parameters are the fixed effect intercepts () and the variances of the pupil (
), school (
), and item (
) random effects. For ease of interpretation, the initially logit-scaled intercepts from were converted into probabilities of a correct response, and those are visualised by domain in (Appendix D). The fixed intercepts’ ranks corresponded fairly closely to the PISA 2018 rankings of countries’ average scores, with Spearman’s rank correlations of 0.98, 0.97, and 0.98 for the domains of reading, mathematics, and science, respectively (OECD, Citation2019, pp. 57–62).
In each domain and country, the fixed effect intercepts varied over pupils and schools on the person side, and most substantially over items on the item side ( in Appendix A). The pupil, school, item, and residual variance components (see, EquationEquation 3)(3)
(3) computed based on said variance estimates are presented in alongside the ratios of the item to person variance components (see, EquationEquation 4)
(4)
(4) . The residual variance uniformly constituted roughly half of the total response variance across the considered domains and countries. Its magnitude was anticipated given that PISA’s primary goal lies in assessing country-level performance rather than that of individual pupils or individual items.
Figure 2. PISA 2018 reading literacy variance components (VC) for pupils, schools and items plotted against the item to person variance components ratios (VCR).
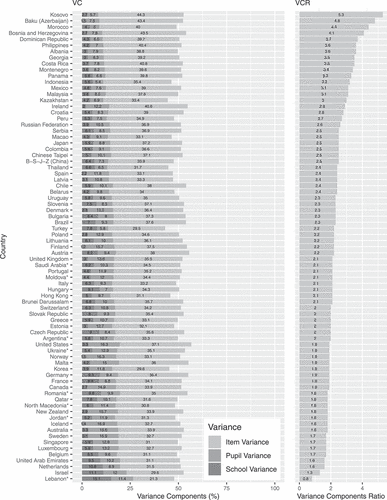
Figure 3. PISA 2018 mathematical literacy variance components (VC) for pupils, schools and items plotted against the item to person variance components ratios (VCR).
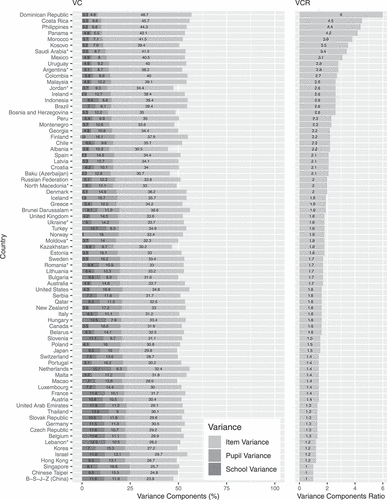
Figure 4. PISA 2018 science literacy variance components (VC) for pupils, schools and items plotted against the item to person variance components ratios (VCR).
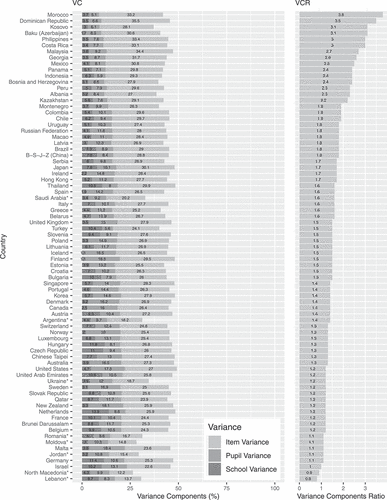
Person variance component
The person variance component combines the pupil and school variance components (see, EquationEquation 2)(2)
(2) to reflect the response variation due to the person side. The pupil and school variance components, in turn, each communicate the amount of the total response variation attributed to differences between pupils and between schools, respectively. The greater the variance component, the greater differences can be expected in performance between pupils within one school and between schools. For instance, in countries with a relatively larger school variance component, the school attended by a pupil may be advantageous or disadvantageous to their level of achievement.
The results show that, on average, roughly 16%, 17%, and 18% of the total response variance in the PISA 2018 reading, science, and mathematics domains, respectively, were attributed to differences between persons. Pupils accounted for about twice the amount of response variation than schools. In the mathematics domain, the average variance in pupil performance accounted for 11.7% () of the total response variance, whereas 6.5% (
) was due to differences between schools. The pupil and school variance components averaged 10.2% (
) and 5.4% (
) in the reading domain, and 11.1% (
) and 5.7% (
), respectively, in science.
Pupil variance component
The most considerable differences in pupil performance within each considered domain were systematically observed, among others, in the Nordic countries and the so-called core Anglosphere. Approximately of the total response variance was due to pupils across three domains in Australia, Canada, Iceland, New Zealand, Norway, Sweden, the United States, and the United Kingdom. On the other hand, differences in pupil performance amounted to only
of the total response variance in Morocco, the Dominican Republic, Indonesia, Panama, and Turkey across all the domains. Moderate to high positive correlations were found between the pupil variance components and the conditional average probabilities of a correct response (i.e., on an average item for an average pupil in an average school per country) (range
across domains), suggesting that, for instance, for lower-performing countries, less differences in pupil ability were observed.
School variance component
The proportion of the school variance was approximately one-tenth of the pupil variance across domains in Denmark, Finland, Iceland and Norway (roughly ). Other countries where achievement was also largely unaffected by the attended schools were Bosnia and Herzegovina, Canada, Ireland, Kosovo, and Spain, where
of the total response variation was accounted for by schools. In contrast,
of the total response variance was due to schools in Israel, Lebanon, Turkey and the United Arab Emirates, as well as in a range of Western and Central European countries (e.g., Belgium, Czech Republic, France, Hungary, the Netherlands).
Several system-level features have been shown to exacerbate or reduce differences between schools. Previous research on educational equity and school effectiveness identified a manifold of such factors. For instance, school differences are commonly examined in relation to the availability of early education (e.g., see, Van Huizen & Plantenga, Citation2018), public education expenditures, public and private schools differentiation (e.g., see, Bodovski et al., Citation2017), curriculum and structural school differentiation (e.g., greater school autonomy; Hanushek et al., Citation2017), and presence of a tracking system (Hanushek & Wöeßmann, Citation2006; Strello et al., Citation2021).
Consistency of person variance components across domains
The person variance components were consistent across the three domains (i.e., correlations between the domain-specific pupil and school variance components were between ). The average range length across the domains (i.e., the absolute difference between the highest and the lowest variance components across three domains) was 2.2% for the pupil and 1.8% for the school variance components.
The least consistent pupil variance components were found in Baku (Azerbaijan), Belarus, B-S-J-Z (China), Chinese Taipei, Macao, Portugal, and Singapore, where larger portions of the total variance could be attributed to differences between pupils in mathematics than in the other two domains (). A similar tendency was noted for the school variance components across domains in the aforementioned B-S-J-Z (China), Chinese Taipei, Thailand, and Hong Kong. In these countries, not only did the pupil ability differ to a greater degree in mathematics, but so did the performance between schools. On the other hand, in the case of Lebanon (range length of 6.4%), these discrepancies in the school variance components were larger between reading and science, an inconsistency shared by other paper-based PISA 2018 participants (e.g., Romania, Saudi Arabia).
Figure 5. PISA 2018 pupil variance components by domain plotted against across-countries average pupil variance components.
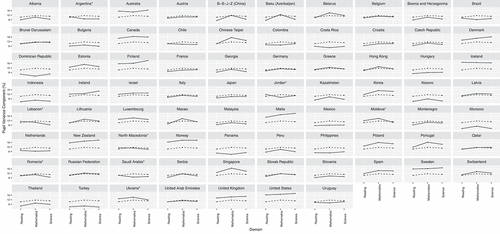
Figure 6. PISA 2018 school variance components by domain plotted against across-countries average school variance components.
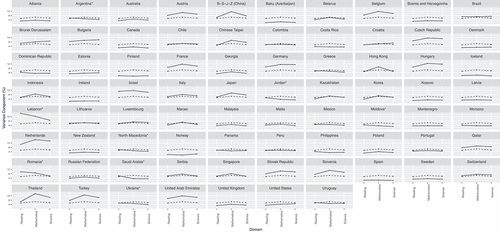
Item variance component
illustrate item to person variance components ratios (VCRs), to the right, for the PISA 2018 reading, mathematics, and science literacy domains. VCR represents the magnitude of the item side variance compared to the person side. VCR above one indicates that more response variation is ascribed to differences between items than to differences between persons. The reverse analogy holds for the VCR below one. On average, across 77 countries, item variance was roughly double the person variance (i.e., VCRs of 2.4, 2.0, and 1.7 for reading, mathematics, and science domains, respectively).
The only countries where the person variance outweighed the item variance were Lebanon in the reading and science domains (VCR = 0.8) and North Macedonia in science (VCR = 0.9). In these countries, item responses depended more on the pupils and the schools they attended than on the items to which they responded. In the B-S-J-Z (China), Chinese Taipei and Singapore in mathematics, and Israel in science, the item and person variances were balanced (i.e., VCR = 1). In the remaining countries, VCRs were consistently greater than one, in some marginally and multiple countries substantially. For example, in the reading domain, the items contributed over four times more variance than persons in Kosovo (VCR = 5.3), Baku (Azerbaijan) (VCR = 4.8) and Morocco (VCR = 4.4). In mathematics, six times more variance was due to items in the Dominican Republic (VCR = 6), and ratios over four were calculated for Costa Rica (VCR = 4.5), Panama (VCR = 4.2), and the Philippines (VCR = 4.4). Finally, over three times larger variance in science was attributed to items in the Dominican Republic (VCR = 3.5) and Morocco (VCR = 3.8).
We now zoom in on the item variance components separately. The item variance component is a portion of the total response variance attributed to differences between items. In countries where this component was smaller, fewer differences between items were observed. Contrariwise, in countries with larger item variance components, the item differences were more pronounced.
The results show that, on average across 77 countries, 35.2% (), 33.7% (
), and 26.4% (
) of the total response variance were due to items in the domains of reading, mathematics, and science, respectively. Items accounted for nearly half of the total mathematics domain variance in the Dominican Republic (48.7%), Costa Rica (45.7%), and the Philippines (44.3%; ). In reading, the largest item variance components were recorded in Kosovo (44.3%) and Bosnia and Herzegovina (43.5%). Roughly
of the total science literacy domain variance was due to items in the Dominican Republic, Malaysia, the Philippines, Morocco, and Costa Rica, countries which also displayed higher than average item variance in the other domains.
Less prominent, yet still sizeable, item variation was observed in Lebanon where 21.3% of the total reading domain variance was due to differences between items, and in B-S-J-Z (China) in mathematics with an item variance component of 23.9% (). Markedly, in the science domain, the lowest item variance components pertained almost exclusively to the countries that participated in the paper-based PISA 2018. The item variance components in these countries were substantially lower than those of the computer-based participants situated at the lower end of the item variance component range. As such, roughly of the total science domain variance was due to items in Jordan, Lebanon, Moldova, and North Macedonia, whereas
were attributed to items in Israel, Malta, and Qatar. One could factor in the differences in the number of science items between the two modes of administration (i.e., 115 items in CBA, 85 in PBA) as affecting the resulting variance; however, even larger item pool differences in the reading domain (i.e., 318 items in CBA, 103 in PBA, see, , Appendix B) would not support this argument.
Lastly, generally large negative correlations () were found between the item variance component and the countries’ conditional average probabilities of a correct response (i.e., on an average item for an average pupil in an average school) for the domains of mathematics, science, and reading, respectively. This finding implies that more differences in item difficulty existed for low-performing participants when compared to high-performing countries.
Consistency of item variance components across domains
Compared to the pupil and school variance components, country-wise item variance components were far less consistent across domains. The amount of the item side variance appeared, to an extent, domain-specific, and its magnitude in one domain did not necessarily coincide with similar magnitudes in the remaining domains. The average range length across the domains (i.e., the absolute difference between the highest and the lowest item variance components across three domains) was approximately 10%. The most consistent item variance components were recorded in Thailand (1.8%) and Korea (2.4%). The largest discrepancies were observed almost exclusively in the PBA countries, where the differences between the item variance components across domains were around 20% due to considerably lower item variances in the science domain.
Aside from the previously mentioned PBA countries, some patterns emerged when examining the least consistent item variance components (). First, compared to the reading and science domains, noticeably larger item variance components in mathematics (range length of around ) were observed in several South and Central American countries such as Costa Rica, the Dominican Republic, Panama, Uruguay, and to a lesser degree, Brazil and Colombia (range length of
differences). Second, an inverse pattern where the mathematics domain had the least item variance compared to reading and science (range length of
) was noted in B-S-J-Z (China) and Chinese Taipei. Finally, in the Balkans (i.e., Bosnia and Herzegovina, Croatia, Kosovo, and Montenegro), reading items exhibited more variation in their difficulty than items of the mathematics or science domains (range length of roughly 13%).
Figure 7. PISA 2018 item variance components by domain plotted against across-countries average item variance components.
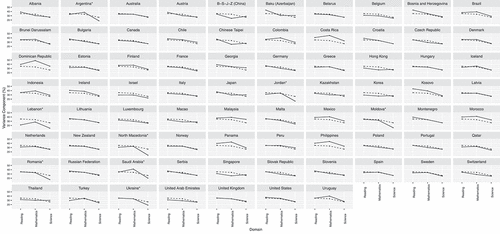
Discussion
Communication of the ILSA results is dominated by reporting countries’ average scores masking variation between pupils, their respective schools, and between items. While a great deal of secondary research focuses on examining this variation between pupils and schools, potentially informative differences between items are largely overlooked, and our knowledge of the item variance magnitude in ILSAs and the drivers behind this variance is scarce.
The present exploratory study took the initial steps towards exploring the item variance in ILSAs. Using a variance components IRT model and the PISA 2018 as a working example, we quantified the item variance in the response data for three cognitive domains of reading, mathematical, and science literacy. We estimated the total item response variance structure for each of the domains across 77 countries and divided that variance into three variance components corresponding to the portions of the total variance attributable to differences between pupils, schools, and items. The variance components computed in this study effectively demonstrated that uniformly across the three PISA 2018 cognitive domains and most of the considered countries, it mattered more which items were responded to by a pupil () than which pupil responded to these items (
) or which school the pupil attended (
).
Given our primary focus to approach the assessment from the item side and the immense volume of existing research on the pupil and school variances, we did not anticipate our analysis to yield any novel insight into the between-pupil and -school differences. This notion held for some, yet not all, of our pupil and school findings which painted a familiar picture to those in educational research. The largest differences in the pupil performance levels were found predominantly in the economically developed educational systems, such as the Nordics and the Anglosphere. On the other hand, in economically developing educational systems, the pupil variance was far less substantial. Previous research, however, cautions against treating the low pupil variances at face value as they may indicate, among other things, the existence of a floor effect for low-performing participants (see, e.g., Rutkowski et al., Citation2019). Minor differences between schools were found in the Nordics, reflective of the Nordic model of education where much of the recent reforms were aimed at the provision of educational equality (see, e.g., Lundahl, Citation2016; Yang Hansen et al., Citation2014). In contrast, we observed larger school differences in some of the Western and Central European countries. These differences could stem from, for instance, socio-economic status differences, school-specific enrolment policies, greater school autonomy, and the presence of a tracking educational system in which pupils are divided based on their achievement (Strello et al., Citation2021).
The mentioned findings are well in line with previous research. The systematic analysis of the variance components consistency across cognitive domains, however, generated several curious results. The pupil and school variance components appeared to be relatively consistent across domains for most countries that administered the computer-based PISA 2018. For countries that took the paper-based version, however, more differences between schools were found in the reading domain compared to the other two domains. Furthermore, several countries showed higher pupil and school variances in mathematics than reading or science (e.g., B-S-J-Z (China), Chinese Taipei, Portugal, Singapore, the Netherlands). Investigating potential drivers behind these domain-specific differences at a country level could present a promising avenue for future inquiry.
One of the key outcomes of PISA is the performance profiles of each participating country. Aside from providing basic indicators of pupils’ knowledge and skills in the cognitive domains, these profiles relate the differences in the pupil and school performance (i.e., the differences we quantified on the person side) to important demographic (e.g., gender), socio-economic and educational indicators. What is lacking from said profiles is the information about countries’ relative strengths and weaknesses regarding different items or topics. Furthermore, secondary analyses using the PISA data mostly extend the knowledge on the relations between person contextual variables and pupils’ outcomes, whereas very few focus on the differences between items.
Even though we hypothesised the item variance to be substantial, we did not anticipate that it would be, with very few exceptions, at least twice the magnitude of the pupil and school variance components combined. Such magnitudes suggest that the current PISA country profiles, focusing exclusively on the person variation, explore only one side of the response data, while the potential of the other side, the item side, remains untapped. Consequently, as opposed to the pupil and school variances discussion, the systematic empirically grounded research to fall back on for potential explanations for the item variance magnitude is lacking. Therefore, the present exploratory study can be positioned as the starting point for mapping out the field and generating research questions for future inquiry. The following summarises our main findings and highlights the potential questions.
The lower item variance components were found in the domain of science (), while in mathematics and reading, the lowest variance components ranged
. Markedly, lower item variance components were observed in countries that participated in the paper-based PISA 2018 science assessment (
) than in their computer-based counterparts. More research is required to examine whether the latter stems, for example, from the existence of a mode effect, although such was not evident in the remaining domains.
The highest item variances were captured in the domains of reading and mathematics. Interestingly, some of the higher item variances clustered in certain regions. For example, nearly half of the total response variance in the reading domain could be ascribed to the items in the Balkans, and in mathematics domain in South and Central America. Furthermore, the higher item variances were observed in the lower-performing countries where more item-level differences existed than in the higher-performing countries. In the Dominican Republic, the total response variance in mathematics could be almost evenly distributed between the residual variance (44%) and the item variance (49%), whereas the pupils and schools, the areas of research that receive most of the attention, contributed only 7%. Large item variances imply that the countries’ averages are not representative of the entire cognitive domains. Rather, there are strengths and weaknesses within each domain, which, if identified and examined, could pave the ways to target and address weaker areas and consolidate the areas of strength within a country or region. These intriguing findings could also serve as motivation for researchers whose areas of interest lie in understanding regional trends in education, for example, in the context of reflecting on differences in curriculum, learning goals, and teacher training across the considered domains. PISA covering three cognitive domains allowed us to generalise our findings across the domains. Nevertheless, future research would benefit from confirming our findings across other ILSAs and multiple cycles of one or more ILSAs to study how the results generalise when a wider net is cast.
Even though we describe the item variances as lower or higher in reference to their ranges in this study, the magnitude of all the computed item variances was substantial. Suppose we were to consider the corresponding pupil and school variances as thresholds for how much variance can be treated as a wake-up call to render the country average obsolete and warrant further investigation. Then, the item variance becomes impossible to blindly ignore. That being said, our goal was by no means to undermine the research on pupil and school differences, as they are the ultimate stakeholders in education. Neither do we wish to undermine the comprehensive item-level analyses performed by PISA as means for item quality control (for an overview of the classical test theory and IRT analyses performed by PISA, see, e.g., OECD, Citation2020); or further between-countries comparisons drawn within the framework of Differential Item Functioning (DIF) aimed at comparing how the items perform in some countries relative to others (e.g., Zwitser et al., Citation2017). Instead, we argue for utilising the collected data to its fullest. Referring to the item variance in the title of this paper as the blind side, we aim to convey that there is still a great deal of untapped response variation that we don’t process on the within-country level despite its great potential to aid in producing more finely-grained country performance profiles (see, e.g., Daus et al., Citation2019). Lastly, we are confident that this paper presents a compelling argument for launching a series of inquiries into the item variance, be it a replication effort in other ILSAs, country-driven exploration, or further explanatory research into the covariates and moderators driving the item variance magnitude. By considering several potential predictors of the item variance on a country level (e.g., item format, item content, length of text), future research may be able to identify and highlight the challenging areas of content and item design features.
Disclosure statement
No potential conflict of interest was reported by the author(s).
Additional information
Notes on contributors
Kseniia Marcq
Kseniia Marcq received her master’s degree in Measurement, Assessment and Evaluation from the University of Oslo, Norway. She is currently a doctoral research fellow at the Centre for Educational Measurement at the University of Oslo, Norway. Her research uses exploratory and meta-analytical approaches to uncover untapped potential in the data of international large-scale assessments.
Johan Braeken
Johan Braeken is a professor of psychometrics at the Centre for Educational Measurement at the University of Oslo, Norway. His research interests are in latent variable modelling, modern test design including adaptive testing, and the information value and data quality in large-scale assessments.
References
- Ahmed, A., & Pollitt, A. (2007). Improving the quality of contextualized questions: An experimental investigation of focus. Assessment in Education: Principles, Policy & Practice, 14(2), 201–232. https://doi.org/10.1080/09695940701478909
- Bates, D., Mächler, M., Bolker, B., & Walker, S. (2015). Fitting linear mixed-effects models using lme4. Journal of Statistical Software, 67(1), 1–48. https://doi.org/10.18637/jss.v067.i01
- Bodovski, K., Byun, S., Chykina, V., & Chung, H. (2017). Searching for the golden model of education: Cross-national analysis of math achievement. Economics of Education Review, 47(5), 722–741. https://doi.org/10.1080/03057925.2016.1274881
- Briggs, D. C., & Wilson, M. (2007). Generalizability in Item Response modeling. Journal of Educational Measurement, 44(2), 131–155. https://doi.org/10.1111/j.1745-3984.2007.00031.x
- Daus, S., Nilsen, T., & Braeken, J. (2019). Exploring content knowledge: Country profile of science strengths and weaknesses in TIMSS. Possible implications for educational professionals and science research. Scandinavian Journal of Educational Research, 63(7), 1102–1120. https://doi.org/10.1080/00313831.2018.1478882
- De Boeck, P. (2008). Random item IRT models. Psychometrika, 73(4), 533–559. https://doi.org/10.1007/s11336-008-9092-x
- Eijkelhof, H. M. C., Kordes, J. H., & Savelsbergh, E. R. (2013). Implications of PISA outcomes for science curriculum reform in the Netherlands. In M. Prenzel, M. Kobarg, K. Schöps, & S. Rönnebeck (Eds.), Research on PISA (pp. 7–21). Springer Netherlands. https://doi.org/10.1007/978-94-007-4458-5_1
- El Masri, Y. H., Ferrara, S., Foltz, P. W., & Baird, J.-A. (2017). Predicting item difficulty of science national curriculum tests: The case of key stage 2 assessments. The Curriculum Journal, 28(1), 59–82. https://doi.org/10.1080/09585176.2016.1232201
- Hanushek, E. A., & Wöeßmann, L. (2006). Does early tracking affect educational inequality and performance? Differences-in-differences evidence across countries. Economic Journal, 116(115), C63–C76. https://doi.org/10.1111/j.1468-0297.2006.01076.x
- Hanushek, E. A., Link, S., & Wöeßmann, L. (2017). Does school autonomy make sense everywhere? Panel estimates from PISA. Journal of Development Economics, 104, 212–232. https://doi.org/10.1016/j.jdeveco.2012.08.002
- Hopfenbeck, T. N., Lenkeit, J., El Masri, Y., Cantrell, K., Ryan, J., & Baird, J.-A. (2018). Lessons learned from PISA: A systematic review of peer-reviewed articles on the Programme for International Student Assessment. Scandinavian Journal of Educational Research, 62(3), 333–353. https://doi.org/10.1080/00313831.2016.1258726
- Kline, R. B. (2016). Principles and practice of structural equation modeling (4th ed.). Guilford Press.
- Le Hebel, F., Montpied, P., Tiberghien, A., & Fontanieu, V. (2017). Sources of difficulty in assessment: Example of PISA science items. International Journal of Science Education, 39(4), 468–487. https://doi.org/10.1080/09500693.2017.1294784
- Lundahl, L. (2016). Equality, inclusion and marketization of Nordic education: Introductory notes. Research in Comparative and International Education, 11(1), 3–12. https://doi.org/10.1177/1745499916631059
- Mullis, I. V. S., Martin, M., Foy, P., Kelly, D., & Fishbein, B. (2020). TIMSS 2019 international results in mathematics and science. TIMSS & PIRLS International Study Center.
- OECD. (2019). PISA 2018 results (Volume 1): What students know and can do. https://doi.org/10.1787/5f07c754-en
- OECD. (2020) . PISA 2018 technical report, PISA.
- R Core Team. (2020). R: A language and environment for statistical computing. R Foundation for Statistical Computing. https://www.R-project.org/
- Rijmen, F., Tuerlinckx, F., De Boeck, P., & Kuppens, P. (2003). A nonlinear mixed model framework for item response theory. Psychological Methods, 8(2), 185–205. https://doi.org/10.1037/1082-989X.8.2.185
- Rutkowski, L., Rutkowski, D., & Liaw, Y.-L. (2019). The existence and impact of floor effects for low-performing PISA participants. Assessment in Education: Principles, Policy & Practice, 26(6), 643–664. https://doi.org/10.1080/0969594X.2019.1577219
- Strello, A., Strietholt, R., Steinmann, I., & Siepmann, C. (2021). Early tracking and different types of inequalities in achievement: Difference-in-differences evidence from 20 years of large-scale assessments. Educational Assessment, Evaluation and Accountability, 33(1), 139–167. https://doi.org/10.1007/s11092-020-09346-4
- Tukey, J. W. (1980). We need both exploratory and confirmatory. The American Statistician, 34(1), 23–25. https://doi.org/10.2307/2682991
- Van den Noortgate, W., De Boeck, P., & Meulders, M. (2003). Cross-classification multilevel logistic models in psychometrics. Journal of Educational and Behavioral Statistics, 28(4), 369–386. https://doi.org/10.3102/10769986028004369
- Van Huizen, T., & Plantenga, J. (2018). Do children benefit from universal early childhood education and care? A meta-analysis of evidence from natural experiments. Economics of Education Review, 66(2), 206–222. https://doi.org/10.1016/j.econedurev.2018.08.001
- Yang Hansen, K., Gustafsson, J.-E., & Rosén, M. (2014). School performance differences and policy variations in Finland, Norway and Sweden. In K. Yang Hansen (Ed.), Northern lights on TIMSS and PIRLS 2011: Differences and similarities in the Nordic countries (pp. 25–47). Nordic Council of Ministers.
- Zwitser, R. J., Glaser, S. S. F., & Maris, G. (2017). Monitoring countries in a changing world: A new look at DIF in international surveys. Psychometrika, 82(1), 210–232. https://doi.org/10.1007/s11336-016-9543-8
Appendix A
Parameter Estimates of the Cross-Classified Mixed Effects Model
Table A1. Country-wise parameter estimates of the cross-classified mixed effects model for the PISA 2018 reading literacy domain.
Table A2. Country-wise parameter estimates of the cross-classified mixed effects model for the PISA 2018 mathematical literacy domain.
Table A3. Country-wise parameter estimates of the cross-classified mixed effects model for the PISA 2018 science literacy domain.
Appendix B
Number of PISA Items by Domain
Table B1. Number of PISA items by domain for computer-based (CBA) and paper-based (PBA) Assessments.
Appendix C
Sensitivity Analysis
The first sensitivity analysis was performed to address potential comparability issues stemming from the non-uniform item pools across countries. We followed the same steps as those of the original analysis, but adjusting the item pools considered. The cross-classified model was fitted exclusively to the responses on the items which were common for all countries within each mode. The analysis considered response data on 70 PBA and 287 CBA items in the reading domain, 58 PBA and 57 CBA items in the mathematical literacy, 83 PBA and 108 CBA items in the science domain (see, ). The variance components and variance components ratios were computed using the obtained variance estimates, and the observed outcome patterns in the new set of results were compared with those of the original results.
The resulting variance components and variance components ratios re-computed based on the parameter estimates of the cross-classified mixed effects model applied to the common items response data across countries and domains are presented in . Given minimal differences in the item pools in mathematics, no substantial deviations from the original results were detected in this domain. Although the re-computed variance components for the remaining domains of reading and science () differed in their magnitude from the original results (), which was anticipated considering the sensitivity analysis was performed on fewer items, the variance components did not differ in their relative proportions of the total variance structure. Moreover, if ordered by a specific variance component magnitude (i.e., pupil, school, item) or the magnitude of the variance components ratios, the countries appeared in nearly identical to the original analysis order. Finally, the re-computed correlation matrix of the outcome measures supported the associations found in the original analyses. Overall, results are fairly robust to the changes in item pools.
The second sensitivity analysis tested the robustness of the results when taking other approaches to partial credit response handling. The impact of different partial credit handling methods (i.e., partial credit responses coded as incorrect, partial credit responses coded as correct, and omitting items that allowed partial credit from analysis) appeared to be minimal. The absolute average differences in item variance components computed using the original and the alternative partial credit handling methods were 1.2–5.2%.
Table C1. Sensitivity analysis resulting country-wise variance components (VC) and variance components ratios (VCR).