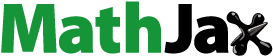
ABSTRACT
Engineering structures consume a significant fraction of resources and contribute to greenhouse gas emissions worldwide. A conducted literature review shows that most existing approaches to improve the environmental performance of structures concern the adoption of decisions during the conceptual design stage (e.g. on the choice of material), often in connection with life cycle assessment. However, approaches for addressing environmental objectives in practice are often hampered by economic interests pursuing short-term profit. Moreover, such approaches are rather descriptive and lack criteria for assessing the acceptability of specific solutions. Sustainable development of our built environment requires hence a shift of paradigm on how engineering structures are designed. In this paper it is claimed that this should be approached at the strategical level of structural design codes, which contain the rules that support everyday engineering decisions in regard to structural safety and functionality. The paper discusses the reasons why these rules as conceived do not foster an optimal use of materials and explores possibilities for savings of resources and greenhouse gas emissions through modifications of these rules. The potential of risk-informed decision approaches in this context is highlighted and illustrated by a case study – the design of steel beams in building structures.
1. Introduction
In the light of the tremendous challenges our society is facing, e.g. in regard to climate change or resource scarcity, the research community is in demand to find solutions that provide the foundation for sustainable societal development. While employed in a wide context, it has been agreed that sustainable development must be addressed by a joint consideration of society, economy and the environment (Webb and Ayyub Citation2017). A key driver for all three of these pillars is the construction sector. Besides its evident importance to the society – it develops the entire built environment, i.e. infrastructure and buildings, that support our societal activities – and its economic significance – about 10 trillion U.S.$ spent globally on construction-related goods and services every year (McKinsey Citation2017) – the sector causes important environmental concerns (CIB Citation1999). Among these, emission of greenhouse gases and hence global warming potential, are specific of importance. The construction sector is estimated to be responsible for more than 20% of the total CO emissions produced by the global economic activities (Huang et al. Citation2018, European Comission Citation2021b). About the half of this share can be associated with building construction (GABC Citation2020). Relatively recent studies indicate a remaining carbon budget for the twenty-first century of about 1000 Gt CO
in order to limit average global warming to 2
C compared to preindustrial levels with high likelihood as demanded by the Paris Agreement on Climate Change, what is equivalent to fewer than 30 years of current global emissions (Kriegler et al. Citation2014) and to about 35% of the emission budget for building the required infrastructure in developing countries assuming current level of technologies (Müller et al. Citation2013). To get the global construction sector on track to achieving the goals of this agreement, building and infrastructure decarbonisation commitments all over the world need to increase in both scale and pace.
Such commitment includes also the structural engineering profession. A number of different strategies, methods and tools for the environmental sustainability assessment of structures have been developed in the last decades (Ding Citation2008, Pomponi and Moncaster Citation2016, Eberhardt, Birkved, and Birgisdottir Citation2020, KC and Gautam Citation2021), often related to Life Cycle Assessment (LCA). However, in spite of significant standardisation efforts over the last decades, e.g. CEN (Citation2010, Citation2011), and the availability of Environmental Product Declarations (EPD), several doubts on the practical implementation of LCA procedures and their associated uncertainties remain (Dong et al. Citation2018, Hawkins et al. Citation2021, De Wolf et al. Citation2020, Saxe et al. Citation2020), which hamper their acceptance and application on a broader level. A more important problem is that LCA standards prescribe methods and procedures for environmental sustainability assessments, while they disregard their interpretation and value judgements. Indeed, decision making within the scope of LCA is mainly limited to comparative studies of different decision alternatives at the conceptual design stage, e.g. decisions regarding the choice of the structural system, its constitutive members and principle materials, without, however, addressing the question if the decision outcome may be judged to be ‘sufficiently sustainable’. Decisions on structural performance are subject to manifold requirements and constraints, including safety, functionality, economy and environmental performance among others. A trade-off between these attributes is sought to identify optimal design decisions that comply with societal preferences on the allocation of available financial and environmental resources. Addressing this challenge clearly falls outside the purview and scope of current LCA procedures and standards.
Structural design codes and standards provide a broader perspective in this regard. Created and developed jointly by different stakeholders including scientists, manufacturers, regulators and users (), these standards contain the compulsory decision rules for the detailed design of structures and their constitutive members (e.g. cross-section design). Since these rules prescribe the flow of structural materials throughout our entire built environment, their careful formulation is an essential means for addressing sustainable development. The Eurocodes (CEN Citation2002a, Citation2020), for instance, provide specific decision rules for verifying compliance with requirements to safety, serviceability and durability. However, optimisation principles, crucial for sustainable development, have only marginally been considered in the establishment of these rules, and only limited to economic considerations. For assuring an optimal structural material use with minimised greenhouse gas emissions, well defined adjustments to the decision strategies and rules for structural design are imperative (GLOBE Citation2022, Holický and Sýkora Citation2021). Conveniently, such developments should be based on risk-informed approaches, which due to their high level of detail regarding the representation of both uncertainties and consequences involved in structural limit state problems, contribute to resource efficient decision making (Köhler and Baravalle Citation2019).
Figure 1. Regulation of the construction sector by codes, which should represent the societal preferences for sustainable development.
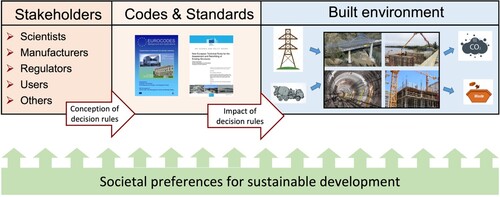
On the grounds of a literature review (Section 2), the paper provides a broad overview on the governing influences and mitigation opportunities of the environmental impact in current structural design practice, with focus on greenhouse gas emissions embodied in building structures. The review also addresses the establishment of corresponding emission benchmarks as well as sources and magnitudes of associated uncertainties. While the review shows that current strategies for savings of resources and emissions mainly address the conceptual structural design stage, the paper subsequently explores the up-to date non-quantified potential for such savings through modifications of the decision strategies and rules for detailed structural member design. Section 3 reviews existing approaches for this purpose and discusses their aptitude in regard to sustainable development. Subsequently, Section 4 illustrates, by means of a case study – the design of steel beams in building structures – the resource- and emission saving potential of risk-informed decisions compared to conventional structural design, based on standardised decision rules. On this basis, Section 5 identifies necessary future developments with the aim to improve the sustainability performance of structures. The paper closes in Section 6 with a summary of the most relevant conclusions.
2. Embodied energy and carbon emissions of structures
2.1. Overview
Greenhouse gas emissions due to human activities are mainly associated with the production and consumption of energy. The energy demand of the built environment can be divided into two principal contributions, respectively, operational and embodied energy (EE), e.g. Sartori and Hestnes (Citation2007), Aye et al. (Citation2012), and Cabeza et al. (Citation2014). The former addresses the energy used during the use phase of buildings and other infrastructure, for purpose of heating, cooling, ventilation, lightning, etc., see stage B1 in , which shows the classification of life cycle stages of buildings according to CEN (Citation2011). The EE refers to the energy required for the product stage (A1–A3), maintenance, repair/replacement/refurbishment processed during the use stage (B2–B5) and the end of life management of structures including demolition, waste processing and final disposal (C1–C4), see . A life cycle assessment of the EE and the associated greenhouse gas emissions, termed ECOe (CO
-equivalent quantities) throughout this paper, may also account for the potentially large benefits related to recycling of materials or reuse of structural components or entire systems (stage D in ), see e.g. Andersen et al. (Citation2020), De Wolf et al. (Citation2020), Eberhardt, Birgisdottir, and Birkved (Citation2019), and Eberhardt, Birkved, and Birgisdottir (Citation2020).
Figure 2. Life cycle stages according to CEN (Citation2011).
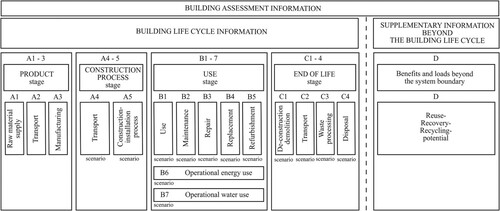
The contribution of EE to the total energy consumption of buildings is strongly dependent on the energy efficiency of the building, see e.g. Chaudhary and Piracha (Citation2013). Excluding high energy efficient designs, such as passive and net zero buildings, the share of EE is generally less than 20%, see e.g. Acree Guggemos and Horvath (Citation2005), Sartori and Hestnes (Citation2007), Dimoudi and Tompa (Citation2008), Gustavsson and Joelsson (Citation2010), and Vukotic, Fenner, and Symons (Citation2010). Moreover, only a certain portion of the total EE of a building is associated with the load-bearing structure (beams, columns, slabs, foundations, etc.), whereas the rest is attributable to non-structural components (e.g. suspended ceiling, lining, cladding, etc.). A review of different studies (Buchanan and Honey Citation1994, Eaton and Amato Citation1998, Dimoudi and Tompa Citation2008, Kaethner and Burridge Citation2012, Clark Citation2013), reveals that the contribution of structures to the total EE and ECOe of buildings ranges between 30 and 80%. An illustrating example for this given in for 30 office buildings investigated by Davis Langdon (Clark Citation2013). The breakdown of their total ECO
e into the contribution of the structure, the facade, internal non-load-bearing components and installations (M&E), reveals that the (mainly steel-framed) structures accounts on average for around 60%. Generally, however, the share of structure-embodied CO
e in buildings is strongly case-dependent. The main influence parameters are the type and geometry of both building and structural systems, their constitutive components and main construction materials (see Section 2.2).
Figure 3. Percentage contribution of different components to total ECOe in 30 office buildings (S = steel framed, C = concrete framed, M = mixed; numbers = no. of storeys). Figure reprinted from Clark (Citation2013) with permission.
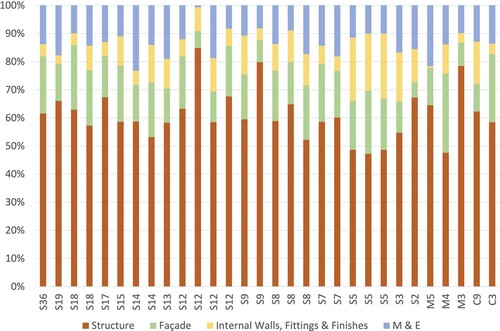
Although to be treated with caution since affected by manifold and large uncertainties, the evaluation of real-world databases (De Wolf et al. Citation2016, Sarkisian and Shook Citation2014) reveals central estimates for cradle-to-gate embodied COe in building structures of the order of 300-400
of floor area (see Section 2.3). Taking Germany as an example, where the 10 year (2012–2021) average annual useful floor area in new buildings (residential and non-residential) amounts to around 57 million m
(Bundesamt Citation2021), this implies an annual amount of approximately 20 million tons of ECO
e in structural materials for buildings. Adding a 20–30% average contribution of life cycle stages beyond the production stage (Sarkisian and Shook Citation2014, Hart, D'Amico, and Pomponi Citation2021) and up-scaling the result from the useful (UFA) to the total building floor area (TFA) assuming a corresponding ratio of UFA/TFA=0.7, inferred from data in Kalusche and Herke (Citation2020), leads to a roughly estimated 38 million tons of annually embodied CO
e in German building structures during the last decade. In comparison to the total German annual average emissions (across all sectors) in this decade (Bundesregierung Citation2022), this corresponds to a significant share of 4 to 5%. In developing countries with comparatively higher construction rates, this share can be expected to be significantly higher.
The indicated numbers refer to buildings only. Infrastructure for energy supply, management of hydraulic resources and transport offer additional significant potential for savings of EE and associated ECO. Especially bridges are promising in this regard, not only because they are generally abundant, but also because they exhibit a very high proportion of structural materials (Gervásio and Silva Citation2008, Zhang, Amaduddin, and Canning Citation2011, Nahangi et al. Citation2021). A recently published extensive data survey concluded with an order of 1 to 7 tons of ECO
e per m
of bridge area (Collings Citation2022), what exceeds by around one order of magnitude the above indicated ECO
e/m
footprint of buildings. Also tunnels embody large amounts of emissions, with certain scope for possible reductions. In Norway, for example, the greenhouse gases embodied in the national road tunnel stock amount to more than eight million tons of CO
e, equal to 83% of the total national direct annual CO2e emissions due to road traffic (in 2011) (Huang et al. Citation2015).
2.2. Influences and mitigation through ‘engineering’
Structural performance, e.g. in terms of load-bearing capacity, is, generally speaking, a function of the demands on a structure, the properties of its constitutive materials and its geometrical characteristics. Both material and geometry have also a relevant impact on the environmental performance of structures and corresponding decisions should be adopted carefully. Depending on the particular circumstances of a structural project, designers can influence such decisions to certain extent by good engineering practices. The opportunities for this are highest at the conceptual structural design stage (Feria and Amado Citation2019, Vilutiene et al. Citation2020), which mainly consists in selecting the type, layout and main dimensions of the load-bearing system, its main constitutive members and most prominent details, as well as the appropriate materials, as reported in Sections 2.2.1 and 2.2.2 below.
In comparison to the conceptual design stage, the subsequent detailed member design (e.g. dimensioning of cross-sections) offers only limited opportunities to designers for savings of materials and EE and ECOe. Detailed member design is subject to the constraints imposed by the compulsory decision rules defined in structural codes and standards, for which compliance must be demonstrated. Potential research-based modifications to these rules, with the aim to improve the sustainability performance of structures, are discussed in Sections 3–5.
2.2.1. Structural system and components
An appropriate choice of the structural system's layout and geometry can be an effective strategy for mitigating EE and ECOe of structures. Low rise buildings, for instance, require a comparatively lower amount of structural material per area of building floor. An increasing building height does not only call for an enhanced material demand of columns and walls required for transmission of gravity loads, but also for members counteracting lateral wind or seismic loads (Khan and Rankine Citation1981, Moon Citation2008), although more recently it has been concluded that the so-called ‘premium for height’ is not substantial (Foraboschi, Mercanzin, and Trabucco Citation2014). Further opportunities for the efficient use of materials are offered by the choice of appropriate span lengths in buildings or bridges. Shorter spans often entail lower emissions (García-Segura et al. Citation2015, Yepes, Martí, and García-Segura Citation2015, Ferreiro-Cabello et al. Citation2016), but might conflict with aesthetic requirements to structures. Shaping structures to follow the flow of internal forces might resolve such incompatibility (Tanner, Bellod, and Sanz Citation2018).
Further potential for saving of material quantities is provided by a proper selection of structural system components. In office buildings, for instance, the design of waffle slabs instead of solid concrete slabs, was found to reduce the cradle-to-grave life cycle greenhouse gas emissions by around 30% (Hájek, Fiala, and Kynčlová Citation2011). The use of post-tensioned concrete slab systems may constitute another environmentally efficient option (Miller and Doh Citation2015, Miller, Doh, and Mulvey Citation2015). An example in the context of transport infrastructure is given in Sakai (Citation2005), where the seismic performance of a railway viaduct was assured by increasing the bearing capacity of the reinforced concrete piles by steel tubes. In comparison to a solution based on the design of massive transverse footing beams, this approach reduced ECOe emissions by 28%.
2.2.2. Materials
The most significant potential for reducing EE and ECOe emissions of structures at the conceptional design stage is provided through the choice of the main constitutive materials. Among the first to investigate into this were Buchanan and Honey (Citation1994) who arrived at the conclusion that timber structures for buildings embody far less EE and ECO
e than functionally identical structures with reinforced concrete or steel members. Later studies supported this finding (Gustavsson, Pingoud, and Sathre Citation2006, Gustavsson and Joelsson Citation2010, Vukotic, Fenner, and Symons Citation2010, Skullestad, André Bohne, and Lohne Citation2016, Lu, Hanandeh, and Gilbert Citation2017), which may be partially attributed to a comparatively lower energy demand for manufacturing of wood-based components. Moreover, ECO
e in timber structures may account for the amounts of CO
sequestered from the atmosphere during tree growth, see e.g. Buchanan and Honey (Citation1994), Arehart et al. (Citation2021), and Hawkins et al. (Citation2021). There is a generally large variation in the way biogenic carbon is treated, with highly sensitive conclusions depending on the methods applied (Arehart et al. Citation2021, Hawkins et al. Citation2021). The replacement of fossil fuels through energy recovery by incineration of by-products, e.g. from the manufacturing stage, or of the timber structure itself following its end of life, may also be accounted for in the carbon emission balance of timber structures, e.g. Gustavsson, Pingoud, and Sathre (Citation2006), Gustavsson and Joelsson (Citation2010), and Niu et al. (Citation2022) (see stage D, ).
While there appears to be a general consensus on the environmental benefits of timber as structural material, the performance of concrete and steel structures with regard to EE and ECOe are subject to controversy. Several studies point to a comparatively larger impact of steel structures, what is often attributed to the more energy intensive processes involved in the material manufacturing process as compared to concrete production (Buchanan and Honey Citation1994, Acree Guggemos and Horvath Citation2005, Aye et al. Citation2012, Kim et al. Citation2013). Conversely, other studies arrived at the opposite conclusion (Jönsson, Björklund, and Tillman Citation1998, Lu, Hanandeh, and Gilbert Citation2017, Oladazimi, Mansour, and Hosseinijou Citation2020, Hawkins et al. Citation2021). The reasons for this are various. Site-related differences seem to play a mayor role: Industrial processes and associated energy demand and fuel types used for manufacturing of materials and construction activities may vary widely between countries, e.g. Gustavsson and Joelsson (Citation2010), Aye et al. (Citation2012), and Skullestad, André Bohne, and Lohne (Citation2016). Site-specific influences may also influence the recycling opportunities of materials. While in most countries steel production incorporates significant amounts of recycled material, with a corresponding large environmental benefit (Vukotic, Fenner, and Symons Citation2010, Gan et al. Citation2017, Hawkins et al. Citation2021), recycling of concrete waste (e.g. of the aggregates) is not yet standard practice. Not rarely, concrete residues are fully destined to landfill, with a detrimental impact on the ecosystem (Lu, Hanandeh, and Gilbert Citation2017, Oladazimi, Mansour, and Hosseinijou Citation2020). See Section 2.3 for further discussion on material-related influence on ECO
e.
2.3. Benchmarking and uncertainties
In order to deduce benchmarks for the ECOe of buildings and to quantify the associated uncertainties, different studies have developed and evaluated databases containing real-world constructions (Sarkisian and Shook Citation2014, De Wolf et al. Citation2016, Simonen, Rodriguez, and Wolf Citation2017, Röck et al. Citation2020, Wiik et al. Citation2020, Andersen et al. Citation2021). The results of two of these investigations (Sarkisian and Shook Citation2014, De Wolf et al. Citation2016), both with particular emphasis on ECO
e in the structures of the buildings, are compared in more detail below. In addition, the comparison includes the findings of a systematic study of hypothetical, but realistic building structures (Hart, D'Amico, and Pomponi Citation2021).
De Wolf et al. (Citation2016, Citation2020) surveyed the ECOe of more than 500 building structures all over the globe, collected in the ‘database of embodied Quantity outputs’ (deQo) (deQo Citation2021). The ECO
e (in
of building floor area) were computed as the product of structural material quantities (SMQ) (in kg/m
) and the embodied carbon emission intensities (in kgCO
e/kg of material; in the following referred to as CEI), associated with the production stage (). The cited study offers a statistical representation of the calculated ECO
e by means of box-plots. summarises the results dependent on the main constitutive structural material. In line with the observations in Section 2.2, timber structures show generally the lowest emissions, followed by masonry. In comparison to this, the ECO
e of composite, (reinforced) concrete and steel structures, are significantly higher. The order of magnitude of the corresponding medians, which do not differ largely (
), are in reasonable agreement with the findings from another large data collection on ECO
e, compiled by Sarkisian and Shook (Citation2014) from over 200 building structures in the U.S.. The ECO
e of these structures was assessed using a unique LCA tool and inventory, assuring thereby uniformity of the results with respect to procedural aspects, such as the estimation of the SMQ and the CEI. plots the average ECO
e (mean values) corresponding to the cradle-to-gate life cycle obtained by Sarkisian and Shook (Citation2014), which is of the order of around
, irrespective of the material (only concrete, steel and composite structures were considered).
Figure 4. Statistical description of different data sets for ECOe of structures of different (main) constitutive materials (cradle-to-gate): DeQo database by De Wolf et al. (Citation2016), database by Sarkisian and Shook (Citation2014) and dataset representative of frame structures in British cities by Hart, D'Amico, and Pomponi (Citation2021).
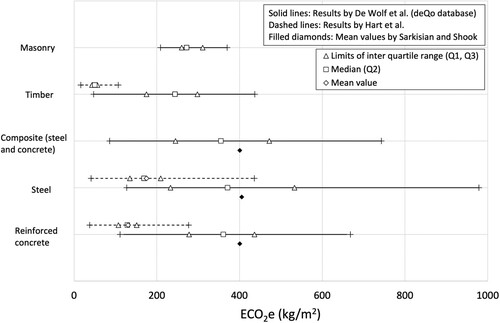
further shows that the deQo data is highly scattered, depending largely on the main constitutive material. The lowest variability is obtained for masonry structures, as indicated by a relatively low range (max – min) and inter-quartile range IQR (Q – Q
), of around 161 and 50 kgCO
e/m
, respectively. In contrast to this, these values soar to about 852 and 300 kgCO
e/m
when steel structures are concerned. The observed variability in the ECO
e can be attributed to several sources, among them variations of the SMQ depending on the characteristics of the buildings included in the database (e.g. building use category and geometry, type of structural system and main components, uncertainties in the differentiation of structural and non-structural building components), but also to procedural differences in the estimation of both the SMQ and the CEI. Since the latter were case-specifically introduced in the deQo database (De Wolf et al. Citation2016), they are most likely subject to a wide scatter, which may have manifold explanations. Among these are different scopes regarding the specific life-cycle stages considered (), material-specific differences (e.g. cement type in concrete structures or the amount of recycled scrap in steel), production-related influences (e.g. energy demand for material production or construction activities) or time dependencies. Generally, CEI tend to decrease over time as a result of more efficient production processes and decarbonisation of the electricity grid, e.g. Lausselet et al. (Citation2021). For further discussion of CEI and their governing influences the reader is referred to Hammond and Jones (Citation2008).
For sake of comparison, the results from De Wolf et al. (Citation2016) and Sarkisian and Shook (Citation2014) are compared to those obtained by Hart, D'Amico, and Pomponi (Citation2021), who, instead of dealing with an arbitrary set of real buildings, systematically generated a set of archetypes of building frame structures representative of multi-storey buildings in British cities, designed according to Eurocode regulations (CEN Citation2002a). summarises the results corresponding to the cradle-to-gate life cycle. Consistent with the deQo data, steel structures show the generally highest ECOe, followed by RC and timber. However, the respective central estimates (Q
, μ) are around 55–65% lower than the corresponding values obtained by De Wolf et al. (Citation2016) or (Sarkisian and Shook Citation2014). Also the scatter is observed to be significantly lower than compared to the results from De Wolf et al. (Citation2016). In addition to site and time-specific influences, as discussed above, these differences are attributable to the fact that Hart, D'Amico, and Pomponi (Citation2021) considered only the frame structure (beams and columns) and the floor slabs in their computations, whereas the structures compiled in the cited real-world databases include also other structural components, such as foundations, basement walls or lateral load-bearing components (e.g. shear walls, cores), which due to their comparatively large volumes, may embody significant amounts of ECO
e. In general terms, this comparison demonstrates that any conclusions or recommendations regarding suitable benchmark values derived from real-world databases as by Sarkisian and Shook (Citation2014) or De Wolf et al. (Citation2016), shall not be positioned within anything but wide bounds. Increasing the degree of representativeness of this data would call for larger sample sizes and/or more narrowly defined system boundaries, e.g. with regard to the type of structures and constitutive members, their geometries, geographic location or the year of construction.
3. Approaches to decision making in structural engineering
In Section 2.2 several opportunities for mitigation of emissions through practitioners, i.e. practicing engineers or architects, were described, including an appropriate choice of the structural system, of its members, geometry and materials. However, decisions on the environmental sustainability performance of constructions are often at the mercy of the client (Miller and Doh Citation2015). The client's interests, in turn, are mainly governed by cost-efficiency, usually pursuing short-term profit by minimising the initial investment (construction costs). Environmental concerns are often disregarded in such thinking (Sakai et al. Citation2016). The fundamental question in this context is how a decision-maker can be persuaded to invest in achieving specific sustainability objectives if this affects his immediate financial gratification (Ellingwood and Lee Citation2016). Solutions to this problem call for environmental sustainability performance requirements at the systemic level, i.e. implemented in the rules which control most structural engineering decisions and the corresponding use of materials.
Decision-making procedures in the context of structural engineering are generally associated with large uncertainties concerning the demands on the structure (e.g. the loads) and its capacity (e.g. the resistance). Consequently, any structural design solution resulting from such procedures is associated with a finite risk. The term risk is understood here as a function of the occurrence probability of a specific non-satisfactory structural performance, e.g. a collapse, and its consequences. While structure-related risks cannot be totally avoided, they can be assessed and managed in the public interest. Three different levels of detail of decision analysis are distinguished (ISO Citation2015, Köhler and Baravalle Citation2019): risk-informed decision making, reliability based design (see e.g. Rodriguez-Nikl, Christiansen, and Walters Citation2012) and semi-probabilistic design, see . As further explained below, the higher level approaches provide the basis for the establishment of requirements for the more simplified and generalised lower level formats.
Figure 5. Different approaches to decision making in structural engineering according to ISO (Citation2015) and Köhler and Baravalle (Citation2019).
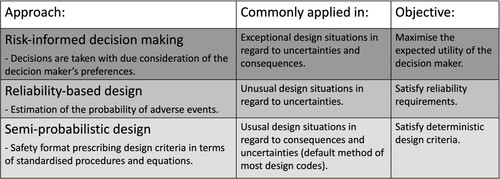
3.1. Decision support by codes and standards
3.1.1. Safety format
In daily practice, decisions concerning structural safety and other essential requirements (e.g. serviceability, durability), are largely regulated by structural design codes and standards (). Most codes, such as the Eurocode (CEN Citation2002a), have adopted the partial factor method (PFM) to account for structure-related risk in an implicit manner. For convenience of code users, the underlying semi-probabilistic concept () is reflected in simple decision rules suited for routine applications. Structural safety, which is the most important performance attribute of structures, is verified through a comparison of design values for resistance () and load (effect) variables (
), as expressed by Equation (Equation1
(1)
(1) ). Formulated in terms of characteristic values for these variables and associated reliability elements (e.g. partial safety factors, load combination factors), the design values are usually defined such that the solution complies, within reasonable bounds, with a specific nominal reliability requirement. This is in general referred to as reliability-based code calibration, e.g. Sørensen, Kroon, and Faber (Citation1994), Faber and Sørensen (Citation2003), and Köhler and Baravalle (Citation2019).
(1)
(1)
3.1.2. Lack of efficiency
The main challenge of such a code-calibration procedure lies in the appropriate choice of the underlying assumptions and models. This is commonly done on the basis of risks experienced and apparently accepted in the past. The Eurocodes, for instance, recognise that the design rules and requirements provided in the code are derived on the basis of a ‘calibration to a long experience of building tradition’ (CEN Citation2002a), along with the appreciation that risks associated with structural failure are nowadays reasonably low (Calgaro and Gulvanessian Citation2001). However, while the overall level of risks associated with civil engineering structures designed to current Eurocode practices appears to be generally acceptable, previous studies have shown that such risks diverge widely, pointing to non-optimal design decisions and, hence, to inefficient allocation of societal resources, e.g. Tanner and Hingorani (Citation2015). In part, the significant scatter observed may be attributed to a lack of a consistent calibration of the standardised design rules based on optimisation principles. The necessary generalisations and simplifications introduced in these rules to facilitate their straightforward application in daily practice (Calgaro and Gulvanessian Citation2001, SAKO Citation1999), which might be interpreted as a systemic suboptimality, constitute a further, inevitable source of this scatter.
3.1.3. Parametric design tools
With the aim to optimise the economic and environmental performance of structures within the bounds of the existing PFM-based design rules in current design codes and standards (Section 3.1.1), a number of parametric design tools have been developed in the recent past and applied to both buildings, e.g. Paya-Zaforteza et al. (Citation2009), Camp and Huq (Citation2013), Kaveh (Citation2017), Eleftheriadis et al. (Citation2018), D'Amico and Pomponi (Citation2018), Ženíšek et al. (Citation2020), Ismail and Mueller (Citation2021) – some of them with focus on seismic loads (Mergos Citation2018, Kaveh, Izadifard, and Mottaghi Citation2020, Mottaghi, Izadifard, and Kaveh Citation2021) – and bridges, e.g. García-Segura et al. (Citation2015), García-Segura and Yepes (Citation2016), and Yepes, Martí, and García-Segura (Citation2015). The cited studies show that, in comparison to traditional and more straight-forward structural design methods, parametric design tools can contribute to significant savings of both financial and environmental resources. These tools are able to deal with relatively complex optimisation problems characterised by multiple decision parameters and imposed constraints, the latter given by structural safety, service ability or durability design rules and requirements, as defined in codes and standards. However, since these rules and requirements have never been consistently calibrated (3.1.2), parametric design tools as described ignore a large potential for mitigation of material consumption and ECOe. Efforts to improve this should be based on resource-efficient risk-informed approaches, as outlined in the following sections.
3.2. Risk-informed decision support
3.2.1. Economic optimisation framework
Given its ability to explicitly account not only for the uncertainties and consequences, but also for the associated safety costs, risk analysis has been identified as a powerful approach for consistent decision making related to the design of structures, e.g. Rackwitz (Citation2000), Rackwitz, Lentz, and Faber (Citation2005), Fischer et al. (Citation2019), and Köhler and Baravalle (Citation2019). Supported by standardised guidance and recommendations for practical applications (ISO Citation2015), these developments facilitate optimised structural design such that a balance is achieved between the costs and benefits associated with a specific structural engineering decision. Normally, the benefits may be considered independent of the decision parameter p (e.g. a cross-section dimension) and the optimisation problem simplifies to the determination of the argument (arg) , which minimises the expected total cost (
) function, as expressed by Equation (Equation2
(2)
(2) ) and illustrated in . The total cost
is constituted by the sum of the expected safety- (
) and failure- (
) cost, see (a). The former are defined as the sum of the construction cost,
and the expected obsolescence cost,
. Costs related to periodic inspection, repair or maintenance are omitted in this representation, see e.g. Nishijima, Faber, and Straub (Citation2007a Citation2007b), Ellingwood and Lee (Citation2016), and Adhikari et al. (Citation2020) for consideration of such costs in optimisation frameworks.
(2)
(2)
(3)
(3)
(4)
(4)
(5)
(5) In Equation (Equation3
(3)
(3) ) the construction cost
are defined as the sum of one part,
, that is proportional to the decision parameter p, and one part,
, that is independent of p. The expected failure cost
(Equation (Equation4
(4)
(4) )) and the expected obsolescence cost
(Equation (Equation5
(5)
(5) )) are estimated based on the assumption that structures are continuously renewed either after failure of after becoming obsolete respectively. A discussion and justification of this assumption can be found in Rackwitz (Citation2000). Correspondingly, the conditional failure cost (reconstruction costs
plus non-structural failure cost H) are multiplied by the yearly failure rate
and result in expected yearly failure costs. Similarly, the conditional obsolescence costs (the reconstruction cost
plus demolition costs D) are multiplied by the yearly obsolescence-rate ω resulting in the expected yearly obsolescence costs. The net present value of the sum of annual expectations with the annual interest rate i is computed by utilising the asymptotic solution:
.
Figure 6. (a) Illustration of cost optimisation principle and of deviations and
from the minimum total cost (
) and optimal decision parameter (
), respectively (left); (b) Illustration of relationship between failure probability (
) and p and of acceptable range for
limited by life-safety (
) and environmental (
) requirements, respectively (right).
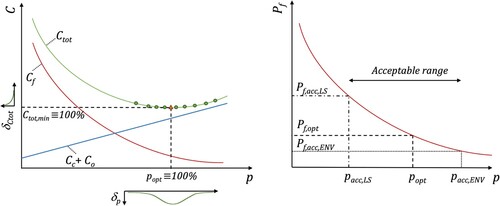
The objective function given by Equation (Equation2(2)
(2) ) ensures that resources are expended optimally from the financial point of view. But it does not guarantee that the optimum decision
is consistent with the societal preferences in regard to life safety. This can be assured by imposing a corresponding acceptance criteria
((b)), e.g. based on the marginal life saving costs principle, which ensures that the societal resources are allocated to efficient risk-reduction measures, i.e. measures that can save one additional life at a cost that the society is willing (or able) to pay, see e.g. ISO (Citation2015) and Fischer et al. (Citation2019).
3.2.2. Monetary valuation of environmental impacts
An economic optimisation framework as described under the previous Section 3.2.1 should account for the monetised environmental impact and benefit of structures. Although subject to ethical concerns and controversy, e.g. regarding the adoption of suitable discount rates, the socioeconomic consequences of ECOe may be monetised, see e.g. Nordhaus (Citation2017), Pindyck (Citation2017), Pindyck (Citation2017), and Tol (Citation2019). This enables their incorporation into the construction cost function given by Equation (Equation3
(3)
(3) ). Monetized carbon emissions allocated to End-of-Life processes (), such as waste processing might also be accounted for if relevant. In case of timber structures, the benefits associated with energy recovery due to the incineration process, including the direct economic benefit (e.g. exported heat and power used for district heating and electricity) and the monetised environmental benefit through replacement of fossil fuels can be considered, see (Niu et al. Citation2022). Future revisits of risk-informed decision frameworks should also address the benefits of reuse and recycling of structural components and materials.
3.2.3. Inter-generational discounting
Current frameworks for risk-informed decision analysis of structures consider economic discounting for establishing the net present value of costs related to periodical structural renewal, as well as to potential structural failure, see Equations (Equation4(4)
(4) ) and (Equation5
(5)
(5) ). A constant discount rate (i), inferred from consumption surveys, is commonly used for this purpose, what might imply disproportional economic efforts by future generations. However, structural optimisation frameworks should generally support sustainable and equitable decisions over extended time-frames. Existing proposals in this direction include time-declining discount rates (Lee and Ellingwood Citation2015, Ellingwood and Lee Citation2016) or an equivalent discount rate, which pursues decisions with potential implications for future generations to be based on an equal weighting of the preferences of present and future decision makers (Faber and Rackwitz Citation2004, Nishijima, Faber, and Straub Citation2007a Citation2007b).
3.2.4. Environmental sustainability potential of cost-optimised structural design
The cost-optimisation framework described in Section 3.2.1 allows for identification of optimal decisions in terms of expected costs. The corresponding saving potential in relation to the sub-optimal decisions based on the standardised design rules defined in structural codes and standards (Section 3.1) is illustrated in (a). By definition, these suboptimal decisions, represented by the dots, entail a certain deviation () from their respective optimum,
, represented by the triangle (normalised to 100%). In regard to environmentally sustainable development, it is further of interest, how the suboptimal solutions deviate from the optimum in terms of the decision parameter p, since the choice of p is representative of the material consumption at the structural design stage, and hence of related embodied energies and greenhouse gas emissions. In contrast to
, this deviation (
) can occur on both sides of the optimum (
), see (a). To date, this deviation has not been quantified, however – the case study presented in Section 4 addresses this.
Another question which has not been investigated until now is if economically optimum decisions according to the described framework can be judged to be sufficiently sustainable from an environmental perspective. As opposed to the life-safety acceptance criterion , which provides a lower bound to material consumption (
), such an investigation should envisage the derivation of a corresponding upper bound criterion (
), based on the (acceptable) environmental impact of structures from a socio-economic point of view, as illustrated in (b). As what concerns greenhouse gas emissions, such a criterion should take basis in scientifically agreed global emission budgets, duly broken down to admissible emissions, and the corresponding material consumption, related to a particular structural design decision. Further considerations regarding this complex issue are out of scope of the present paper.
3.2.5. Environmentally optimal design
An alternative to the described approach in the previous section, which quantifies the environmental sustainability potential of an economic optimisation framework, is to formulate the objective function in terms of a specific environmental impact indicator instead of costs. In the context of global warming potential, such a function is given by Equation (Equation6(6)
(6) ), which, in equivalency to Equation (Equation2
(2)
(2) ), aims at establishing decision parameter
such that it minimises the total embodied carbon emissions
of a specific structural engineering decision.
(6)
(6) Several studies addressing multi-objective parametric structural design (see Section 3.1.3) suggest that economically optimal solutions show also a relatively satisfactory environmental performance in terms of
. Indeed, this seems reasonable as both optimisation objectives, costs and emissions, are correlated to large extent through the amount of material consumed. However, for the time being it has not been analysed if this conclusion holds also in a risk-based optimisation framework, where the indirect failure costs (H in Equation (Equation4
(4)
(4) )) and emissions might influence the optimum to a different extent and where unlike discounting strategies might apply for cost and environmental impact minimisation, respectively, see e.g. Weikard and Zhu (Citation2005) and Gollier (Citation2010). Future studies should address such aspects.
4. Resource and emission-saving potential of risk-informed decisions – a case study
4.1. Objective
In order to assess the resource- and emission-saving potential of explicit risk-informed decision making in structural design procedures, a case study is conducted based on the economic optimisation framework given by Equations (Equation2(2)
(2) ) to (Equation5
(5)
(5) ) and the results compared to the findings of a strict design (
) according to the semi-probabilistic PFM defined in the Eurocodes (CEN Citation2002a) (Section 3.1).
4.2. Methods and assumptions
4.2.1. Structural system and loads
The case study addresses frequently encountered and simple structural engineering decisions in practice – the design of steel beams in residential and office buildings. The simply supported members of span L provide support to reinforced concrete slabs (RCS) spanning over length D in the direction perpendicular to the beam orientation ().
Figure 7. Sketch of the analysed floor system consisting of steel beams supporting one-way RC slabs.
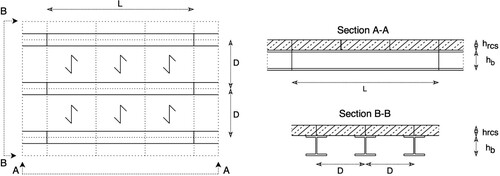
Permanent loads g acting on the beams include the structural self weight , with contributions of both the beam,
, and the RCS,
. In addition, g accounts for the weight of non-structural permanent loads,
, including the flooring and roofing system (). Moreover, the beams sustain a uniformly distributed imposed load q.
4.2.2. Limit state function and decision parameter
Bending failure at mid-span of the beams is investigated. The limit state function is given by Equation (Equation7(7)
(7) ), representing equilibrium between acting and resisting bending moments at mid-span of the beams, where
and
denote the corresponding model uncertainties. Variable
represents the uncertainties associated with the imposed load model and
the steel yield strength.
(7)
(7) Decision parameter p is represented by the plastic cross-section modulus
– assuming class 1 or 2 cross-sections (CEN Citation2005). Note that p is functionally related to the beam's material volume V. Equation (Equation8
(8)
(8) ) provides a close estimate of the relationship between V and p, where
represents the cross-section depth of rolled steel profiles with I or H cross-section (
is assumed).
(8)
(8)
4.2.3. Characteristic values and probabilistic models
With the aim to define a representative set of design situations, the parameters most relevant to the design of the steel beams are varied within reasonable ranges. Three spans (L) are distinguished (6, 12 and 18 m) in addition to four different spacings D between members (L/1, L/2, L/3, L/4) (). Steel grades S235 () and S355 (
) are assumed.
A characteristic value for the variable load of is adopted, situated between the recommended values for residential (
) and office buildings (
), respectively, according to CEN (Citation2002b). A wide range of ratios
of variable (
) to total loads (
) between 0.05 and 0.95 is considered, with an interval size
. In addition, three ratios
of self weight (
) to total permanent loads (
), ranging between 0.6 and 1.0 are adopted (
). Based on these assumptions, the characteristic values of the permanent loads
and
are computed. The self-weight,
, can be further broken down into the contribution of the beam (
) and of the RCS (
). The depth of the latter,
, is then inferred under the assumption of a typical density
. In order to ensure only practically relevant design situations to be included in the study, maximum and minimum ratios of depth
to span (D) of the RCS, of respectively, 1/20 and 1/30, are imposed in addition to a minimum depth of
. By imposing these constraints, the number of design situations is limited to a total of 77 cases, distributed within
and
– cases with
beyond these thresholds do not comply with the described constraints to
/D and
. in Appendix A provides an overview of the basic variables involved in the case study and their characteristic values.
The probabilistic models for the loads (,
, q), material strength (
) and model uncertainties (
,
,
) are adopted from CEN (Citation2022). All other variables in Equation (Equation7
(7)
(7) ) (L, D, p) are assumed as deterministic quantities, see (Appendix A).
4.2.4. Costs
Construction costs – see Equation (Equation9
(9)
(9) ) – are based on indicated price benchmarks for steel frame structures in Germany, which according to Bauforumstahl (Citation2021) oscillate between 1.6 and 2.2 €/kg (for rolled profiles) and account for the three primary components of the total installed cost of a steel frame, i.e. material (mat), fabrication (fab) and installation (inst) costs (Barg, Flager, and Fischer Citation2018). Taking this range as a basis, a representative value of
= 2 €/
is adopted in the present study. However, costs for steel construction works are not entirely proportional to their weight, what holds especially for fabrication and installation costs (Barg, Flager, and Fischer Citation2018). For sake of simplicity, this study assumes a linear relationship between the structural mass M (
, where
) and the material costs. In addition, 50% of the fabrication costs are assumed to be linearly correlated with M. According to Bauforumstahl (Citation2021), indicative shares for material and fabrication costs of the total installed cost of steel frames are 36% and 34%, respectively, hence
in Equation (Equation9
(9)
(9) )
€/
. Costs for installation
are assumed entirely independent of the weight of the structure (hence of p) and are disregarded due to their negligible influence on the results.
Although current carbon emission pricing rates are generally low in comparison to the costs for material, fabrication and erection of the structure, they should principally not be ignored (Niu et al. Citation2022). It might be expected that carbon prices are going to increase substantially in the future and hence their impact on the outcome of this and similar studies. The present study considers a carbon emission price €/
, what corresponds to the 2022 maximum value according to the EU emissions trading system (European Comission Citation2021a). A CEI of
is assumed, corresponding to cradle-to-gate emissions (stages A1–A3, see ) of structural steel products of German producers (IBU Citation2018).
(9)
(9) The indirect failure costs H in Equation (Equation4
(4)
(4) ) should consider compensation for potential fatalities in case of member collapse. This involves affronting the difficult task of assigning an economic value to human life. Virguez and Faber (Virguez and Faber Citation2011) and Annex E of ISO 2394 (ISO Citation2015) suggest the adoption of the Societal Value of a Statistical Life (SVSL) for assessing the monetary impact of loss of life in the context of economic optimisation frameworks. The SVSL can be inferred from the same basis (the Life Quality Index) as the Societal Willingness to Pay (SWTP) (Fischer and Faber Citation2012), which provides the basis for deriving human safety acceptance criteria
in structural decision problems according to the marginal life saving principle (see (b)). The present study considers a SVSL of 2.775E06 € converted from the value indicated in ISO2394 for Germany (based on an annual discount rate i = 3%) by adjusting the average GDP per capita from U.S.$ 33668 in 2008 (ISO Citation2015) to 43292 € in 2021 (Bundesamt Citation2022).
The SVSL is then multiplied with the expected number of fatalities due to beam collapse () according to Equation (Equation10
(10)
(10) ), estimated as a function of the probability of occurrence of a fatal collapse event,
=
, the expected area affected by such a collapse,
, and a consequence class-dependent model constant CC, which is related to the number of persons at risk per unit of
(CC = 30 for residential and office buildings). In accordance with the definition of the structural system (), the collapse of a beam is assumed to affect an area
(floor area supported by and below the collapsing beam). See (Hingorani et al. Citation2020) for further details on the consequence model and the corresponding assumptions.
The calculation of expected obsolescence costs according to Equation (Equation5
(5)
(5) ) assumes an annual obsolescence rate
. Demolition costs (D) are neglected. The considered annual discount rate i is 3%. All cost-related modelling assumptions are summarised in (Appendix A).
(10)
(10)
4.3. Results
(a) compares the annual () reliability index (
(
), where Φ = standard normal distribution),
(crosses) and
(dots) corresponding to the optimum and Eurocode design, respectively. The latter are found to vary largely with the variable load ratio
(
/(
)), shown on the horizontal axis. Except for small
, the
decrease with increasing
due to the dominating influence of the imposed load model, as observed in other studies, e.g. Meinen and Steenbergen (Citation2018). In contrast to this, the
are far less scattered and almost independent of
. Except for
, the
, situated between 4.6 and 5.0, are below their
counterparts, which range between 4.8 and 5.5. The ratios of the corresponding annual failure probabilities,
to
, vary between 0.7 and 65, see (b). It should be noted that the
are likely to fall below the life-safety requirement
based on the marginal life saving cost principle ((b)).
Figure 8. (a) Annual reliability index (crosses) and
(dots) corresponding to optimum and Eurocode design, respectively (left) and (b) ratio of annual failure probabilities
to
(right) vs. load ratio
.
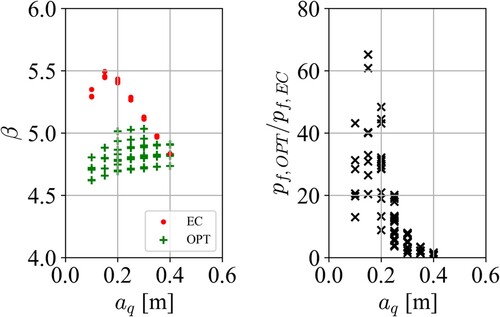
The resource-saving potential of the optimisation is illustrated in . (a) shows the difference between the minimised, expected total costs
and the solution based on the Eurocode design, while (b) plots the corresponding difference for the member material volume
, which is equal to the deviation in terms of the decision parameter p,
(see (a)). Deviation
has been determined under the assumption of constant cross-section dimensions (corresponding to the mid-span cross-section) along the longitudinal axis of the beams. The figure depicts a strong correlation between the saving potential and the ratio
to
, plotted on the abscissa. Generally, the higher this ratio, the higher
and
, although the increment of both δ clearly diminishes with increasing
/
. The former (
) attains maxima of around 8%, the latter (
) of about 11%. For
< 1,
approaches 0 and
< 0, i.e. the Eurocode design consumes less material at the design stage than the optimal solution (
<
). (b) reveals that this occurs for comparatively large
.
4.4. Discussion
4.4.1. Saving potential
The presented results appear to be generally plausible: Less investment of resources into structural safety than compared to current Eurocode practice, as represented by positive and
, entails comparatively higher failure probabilities. Nevertheless, all obtained
are close to or above the (overly conservative) nominal Eurocode target,
. Hence, the optimised solutions can by no means be judged as unsafe. They reflect a more efficient use of money and materials at the structural design stage, as well as a reduction of CO
emissions. For the here investigated 77 design situations, the average reduction
is around 7% (see Baseline scenario in ). Given the large amount of structures erected every day all over the globe, the case study underlines the, in absolute terms, large resource- and emission saving potential of risk-informed optimisation procedures. However, the results reveal also certain limitations of the approach. Situations characterised by larger
lead to only irrelevant or even negative resource savings. Since variable loads are governing many design situations in practice, the results might be less favourable (smaller
and
) when widening the scope of the study to other member types and/or load scenarios.
Table 1. Mean (μ), min and max (all numbers in [%]) of savings and
obtained under different scenarios.
The reasons for this are to be sought in the unlike relationship between and, respectively,
and
((a)). On one hand,
generally tends to increase with rising
due to the dominant influence of the comparatively uncertain variable load, as observed for Eurocode results,
. On the other, larger
cause higher expected failure costs, which play a dominant role in the economic optimisation of the beams due to the comparatively high (in relation to other costs) indirect costs, i.e. human fatality compensation costs. Higher failure costs, in turn, cause decision parameter p to increase, hence the optimum
to decrease. This balancing effect explains the almost constant, even slightly increasing, optimum reliability level (
) visible in (a), and hence continuously decreasing material/emission savings at the design stage
as
further rises. One inference of this is that an emission-based optimisation of the beams (see Section 3.2.5), where the indirect failure component H is expected to have a far less dominant influence on the optimum
, would most likely reveal a significantly lower sensitivity of such savings with respect to
. Future studies should validate this assumption.
4.4.2. Sensitivity with regard to assumptions
The large influence of the member loading conditions on the results merits a closer look into the corresponding probabilistic modelling assumptions. Current models for imposed loads are outdated and might not adequately represent current building use conditions (Honfi Citation2014). In addition, more research is needed to improve the models for the representation of load effect uncertainties ( in Equation (Equation7
(7)
(7) )). Only small changes in these models can have a substantial impact on the structural failure probabilities and hence on the here investigated resource and emission saving potential. To illustrate this, the coefficient of variation for
has been reduced from 0.1, as suggested in CEN (Citation2022), to a value of 0.075, recommended in the current draft of the future fib Model Code (fib Citation2022). While the effect of this apparently minor change on
is small, it substantially increases
(especially in the range of small
). Consequently, both
and
increase with respect to the values obtained in the Baseline scenario, attaining mean/maximum values of around 9/14% and 12/17%, respectively – see (Reduced load effect model uncertainty scenario).
In addition to the probabilistic models, the results are to some extent sensitive to the cost model parameters. Among these, the metric used for monetising human fatalities should be highlighted. A comparison of several such metrics reveals quite different results (Fischer and Faber Citation2012). Following the recommendation in ISO2394 (ISO Citation2015), the SVSL was adopted in the Baseline scenario analysed in this study. However, the cited standard also points to the fact that different legal systems in different countries lead to different compensation practices. In order to gauge the sensitivity of the results with respect to such differences, the SVSL in Equation (Equation4(4)
(4) ) is replaced by the so-called Societal Human Capital (SHC), proposed by Lentz (Citation2007) as a lower bound for compensation costs and which monetises fatalities in terms of lost future earnings due to the premature death of a person. In this case, a SHC of around 1.968E06 € is estimated as the product of German average income for 2021 and an age-average life expectancy of 40 years (Lentz Citation2007), which is around 30% lower than the SVSL estimate used in the Baseline scenario. Lower failure costs imply lower
, hence both
and
increase with respect to the Baseline scenario. However, as shows, the order of magnitude of the obtained δ remains the same. Variations of other cost modelling assumptions within reasonable limits, e.g. assumptions on the steel price or on the relationship between the decision parameter p and the different construction cost components (material, fabrication, installation), lead to the same conclusion.
4.4.3. Discounting strategies
As stated in Section 3.2.3, the use of a constant economic discount rate based on market interest rates is questionable from the perspective of inter-generational equity, especially for longer structural life times. Alternative approaches aim at a trade-off between investments of today's generation in structural design and the cost of maintenance or replacement of structures for future generations (Faber and Rackwitz Citation2004, Nishijima, Faber, and Straub Citation2007a Citation2007b, Lee and Ellingwood Citation2015, Ellingwood and Lee Citation2016). In comparison to the traditional discounting method, these approaches assign higher resource use and monetary efforts at the time the decision is made as compared to the costs which are postponed to the future. In the context of this study, these approaches would lead to comparatively higher realisations of decision parameter than obtained under the traditional discounting scheme used. This, in turn, would imply higher financial efforts at the design stage and, conversely, lower expected failure costs due to comparatively higher reliability levels. However, as discussed above, comparatively larger
are equivalent to higher material consumption and hence greenhouse gas emissions. In turn, resource consumption and emissions associated with future structural maintenance or repair might be lower. Larger initial investments into safety could also reflect in longer structural service lifetimes (given a specific maintenance scheme) and hence in lower financial and environmental burdens for future generations. These considerations indicate the complexity associated with the choice of an appropriate discounting method in the context of sustainable development in general, and climate change mitigation in particular. Is it reasonable to allow for larger emissions now in exchange for lower emissions in the future? Or should we, given the apparent urgency of the matter, put more weight on emission reduction at present? It is clear that such decisions should, in addition to structural engineers, be informed by other relevant stakeholders such as e.g. climate scientists, economists and representatives of those affected by the decisions. Further discussion of this matter is out of scope of this study.
5. Required developments
The development of sustainable built environment systems (e.g. buildings, infrastructure), in general, and for the loadbearing part of such systems (i.e. structures), in particular, within a framework of limited resources and a strong need for mitigation of greenhouse gas emissions, requires substantial modifications at the systemic level of the rules and regulations, which implicitly control most structural engineering decisions (Section 3.1). Its high level of detail in regard to the representation of the decision problem and the associated ability to identify optimal design solutions, makes explicit risk-informed decision analysis an appropriate tool for this purpose (Sections 3.2 and 4). However, their inherent complexity hampers a wide application of risk-based approaches across the structural engineering community. Besides from enhancing knowledge of such approaches through education, there are generally two possibilities to deal with this problem, as outlined below.
5.1. Risk-based calibration
The first of these possibilities is a consistent, risk-based calibration of the PFM-based decision rules. Equation (Equation11(11)
(11) ) specifies one possible objective function for this purpose. The function aims at finding the set of optimal partial factors
, which minimises the sum of the total costs
(see Equation (Equation2
(2)
(2) )) over a specific set of design situations j. As an alternative to the minimum cost, the risk-based calibration should also envisage environmental objectives (Section 3.2.5), always taking due account of human safety boundaries.
(11)
(11) Subscript w in
indicates the need for weights in order to consider a representative set of design situations that are regulated by the calibrated design rules. Specific attention should be paid to the definition of the scope of this set. Generally, the broader the spectra of design situations covered by a specific array of calibrated partial factors, the larger is the expected deviation from the optimum. An illustration of this is provided in a recent calibration study carried out in the context of the Ad-hoc group Reliability background of the Eurocodes (CEN Citation2022), which showed that the large scatter affecting the reliability levels of structures designed according to the Eurocode (Section 4.1) can be significantly reduced when assigning individual partial factors to snow, wind and imposed loads, instead of a common factor
for all variable loads as under the current approach. A differentiation of imposed load models depending on the building use entails further potential scope for optimisation, as would the updating of these models (Honfi Citation2014).
5.2. Explicit risk-based design enabled though digital tools
A risk-based calibration of the rules for structural design can reduce the degree of suboptimality affecting the underlying decisions. However, a significantly higher degree of optimisation could be achieved by directly applying risk-based decision analysis to individual structural design projects. Digital tools offer an innovative perspective on how this could be efficiently implemented on a broad scale. Moving away from the current ‘analog’ approach to structural decision making based on codified rules and the corresponding coarse use of information, the disposal of user-friendly and operational IT-tools would bring the ability of flexible risk-based design to identify optimal solutions within the reach of routine applications in daily engineering practice. If appropriately standardised, such tools could also form the grounds for enhancing the decision scope of structural design codes from the detailed member design stage, to the conceptual design stage. Such a holistic approach to risk-informed optimisation would most likely entail the largest resource saving potential in structural design problems. Existing studies on structural optimisation based on parametric design tools underline this potential, see Section 3.1.3. The general shortfall of these studies is that they optimise within the bounds of the implicit structural safety and serviceability requirements as defined in structural design codes. The potential of risk-informed optimisation in connection with parametric design tools has not been explored to date.
6. Conclusions
Engineering structures play a fundamental role in the consumption of financial and environmental resources and contribute significantly to (embodied) greenhouse gas emissions (ECOe) of constructions. A literature survey has shown that up to 80% of the overall embodied emissions in buildings may be attributed to the load-bearing structural members, a share which is even higher in other types of infrastructure (e.g. bridges or tunnels). The choice of the main construction material was identified as the main governing influence on structure-related ECO
e, with clear benefits of timber over concrete or steel. Other significant opportunities for reduction of ECO
e at the conceptual structural design stage are the choice of the structural system, its geometry and constitutive members.
The large variety of parameters influencing ECOe of structures hampers a straightforward establishment of corresponding benchmark values. An evaluation of different data sets revealed that ECO
e of building structures is highly scattered. In addition to the diversity of structure-specific parameters (e.g. system, member and material type, geometry, loading conditions), both time and site-specific influences on the carbon emission intensities (CEI – amount of carbon per unit weight of a specific material) contribute to this scatter. Among these are e.g. the share of fossil fuels among the energy mix or the energy demand in material production or construction activities. Also the specific life cycle stages considered in the determination of the CEI should be carefully considered when evaluating and comparing emissions embodied in structures.
While there are several possibilities for mitigation of ECOe at the conceptual structural design stage, supported by standardised guidance on life cycle assessments (LCA), these are only seldom realised. Mainly due to lack of both knowledge and financial incentives, sustainability objectives are not efficiently accounted for in most practical structural projects. Solutions to this problem call for an implicit consideration of such objectives in the compulsory decision rules applied in daily structural design practice, which largely control structural material use. Only then, it seems possible to reduce structure-related ECO
e at a large scale.
The decision rules in structural design codes, such as the Eurocodes, are highly generalised and simplified, as the ‘ease of use’ was one of the most important criteria for their development. Moreover, these rules do not contain any account of objectives related to environmental sustainability. This translates into a lack of efficiency in regard to the desired optimal balance between structure-inherent safety levels and the resources allocated to this end. Opposed to this, risk-informed approaches, facilitate a consistent way to identify an optimum out of different decision alternatives, as illustrated by a case-study. With respect to design based on Eurocode decision rules, economic optimisation applied to different design situations involving steel beams in buildings entailed reductions in expected costs and material use at the design stage of up to approximately 10%. The results were found to depend strongly on the ratio of variable to total loads, however. Larger ratios led to minor or even negative material savings. To a minor extend, the saving potential was found to be sensitive to the probabilistic and cost modelling assumptions.
Risk-informed decision making provides a rational basis for calibration of the simplified decision rules in structural design codes, thereby reducing the degree of suboptimality with respect to structural engineering decisions under current practice. Such a calibration is subject of ongoing studies. Future developments should also envisage the possibility for risk-informed, parametric structural design based on digital tools in order to exploit in a holistic approach the full resource- and emission saving potential of both the conceptual and detailed structural design stage.
Disclosure statement
No potential conflict of interest was reported by the author(s).
Additional information
Funding
References
- Acree Guggemos, A., and A. Horvath. 2005. “Comparison of Environmental Effects of Steel- and Concrete-Framed Buildings.” Journal of Infrastructure Systems 11 (2): 93–101. https://doi.org/10.1061/(ASCE)1076-0342(2005)11:2(93).
- Adhikari, P., H. Mahmoud, A. Xie, K. Simonen, and B. Ellingwood. 2020. “Life-Cycle Cost and Carbon Footprint Analysis for Light-Framed Residential Buildings Subjected to Tornado Hazard.” Journal of Building Engineering 32: 101657. https://www.sciencedirect.com/science/article/pii/S2352710220308482.
- Andersen, C. E., K. Kanafani, R. Kjær Zimmermann, F. Nygaard Rasmussen, and H. Birgisdóttir. 2020. “Comparison of GHG Emissions from Circular and Conventional Building Components.” Buildings and Cities 1 (1): 379–392. https://doi.org/10.5334/bc.55.
- Andersen, C. E., F. N. Rasmussen, G. Habert, and H. Birgisdóttir. 2021. “Embodied GHG Emissions of Wooden Buildings–Challenges of Biogenic Carbon Accounting in Current LCA Methods.” Frontiers in Built Environment 7: 120. https://www.frontiersin.org/article/10.3389/fbuil.2021.729096.
- Arehart, Jay H., Jim Hart, Francesco Pomponi, and Bernardino D'Amico. 2021. “Carbon Sequestration and Storage in the Built Environment.” Sustainable Production and Consumption 27: 1047–1063. https://www.sciencedirect.com/science/article/pii/S235255092100066X.
- Aye, L., T. Ngo, R. H. Crawford, R. Gammampila, and P. Mendis. 2012. “Life Cycle Greenhouse Gas Emissions and Energy Analysis of Prefabricated Reusable Building Modules.” Energy and Buildings47: 159–168. https://www.sciencedirect.com/science/article/pii/S0378778811005950.
- Barg, Steve, Forest Flager, and Martin Fischer. 2018. “An Analytical Method to Estimate the Total Installed Cost of Structural Steel Building Frames During Early Design.” Journal of Building Engineering 15: 41–50. https://www.sciencedirect.com/science/article/pii/S235271021730503X.
- Bauforumstahl. 2021. “Kosten im Stahlbau 2021, Basisinformationen zur Kalkulation.”
- Buchanan, A. H., and B. G. Honey. 1994. “Energy and Carbon Dioxide Implications of Building Construction.” Energy and Buildings 20 (3): 205–217. https://www.sciencedirect.com/science/article/pii/0378778894900248.
- Bundesamt, DESTATIS. Statistisches. 2021. “Bautätigkeit und Wohnungen. Fachserie 5 / Reihe 1. Bautätigkeit.” https://www.destatis.de.
- Bundesamt, DESTATIS. Statistisches. 2022. “Volkswirtschaftliche Gesamtrechnungen, Bruttoinlandsprodukt (BIP).” https://www.destatis.de/DE/Themen/Wirtschaft/Volkswirtschaftliche-Gesamtrechnungen-Inlandsprodukt/Tabellen/bip-bubbles.html.
- Bundesregierung. 2022. Klimaschutzbericht 2022. Report. Bundesministerium für Wirtschaft und Klimaschutz.
- Cabeza, L. F., L. Rincón, V. Vilariño, G. Pérez, and A. Castell. 2014. “Life Cycle Assessment (LCA) and Life Cycle Energy Analysis (LCEA) of Buildings and the Building Sector: A Review.” Renewable and Sustainable Energy Reviews 29: 394–416. https://www.sciencedirect.com/science/article/pii/S1364032113005777.
- Calgaro, J. A., and H. Gulvanessian. 2001. “Management of Reliability and Risk in the Eurocode System.” In Safety, Risk and Reliability – Trends in Engineering: International Conference, Malta, 155–160. Zurich: International Association for Bridge and Structural Engineering (IABSE).
- Camp, C. V., and F. Huq. 2013. “CO2 and Cost Optimization of Reinforced Concrete Frames Using a Big Bang-Big Crunch Algorithm.” Engineering Structures 48: 363–372. https://www.sciencedirect.com/science/article/pii/S0141029612004828.
- CEN. 2002a. “EN1990. Eurocode – Basis of Structural Design.”
- CEN. 2002b. “EN1991-1-1. Eurocode 1: Actions on Structures – Part 1-1: General Actions – Densities, Self-Weight, Imposed Loads for Buildings.”
- CEN. 2005. “EN1993-1-1. Eurocode 3: Design of Steel Structures – Part 1-1: General Rules and Rules for Buildings.”
- CEN. 2010. “EN15643-1:2010. Sustainability of Construction Works – Sustainability Assessment of Buildings – Part 1: General Framework.”
- CEN. 2011. “EN15978:2011. Sustainability of Construction Works – Assessment of Environmental Performance of Buildings – Calculation Method.”
- CEN. 2020. “prEN1990. Eurocode – Basis of Structural and Geotechnical Design.”
- CEN. 2022. Draft JRC Report – Reliability Background of the Eurocodes – 2022-02-11. Report. CEN/TC 250/ SC10 N 5553.
- Chaudhary, Tariq, and Awais Piracha. 2013. “Effective Contribution of Structural Engineers to Green Buildings and Sustainability.” Canadian Journal of Civil Engineering 40 (1): 97–100. doi:10.1139/cjce-2012-0154.
- CIB. 1999. Agenda 21 on Sustainable Construction. Report. International Council for Research and Innovation in Building and Construction (CIB), Comission W81.
- Clark, D. H. 2013. What Colour is Your Building? Measuring and Reducing the Energy and Carbon Footprint of Buildings: Information Paper 12 – Embodied Carbon Case Studies for Office Buildings. Positive Zero Pty Limited and Cundall Johnston and Partners LLP. https://www.positivezero.com.au/wciyb.
- Collings, David. 2022. “The Carbon Footprint of Bridges.” Structural Engineering International 32 (4): 501–506. https://doi.org/10.1080/10168664.2021.1917326.
- D'Amico, Bernardino, and Francesco Pomponi. 2018. “Accuracy and Reliability: A Computational Tool to Minimise Steel Mass and Carbon Emissions at Early-Stage Structural Design.” Energy and Buildings168: 236–250. https://www.sciencedirect.com/science/article/pii/S0378778817336198.
- deQo. 2021. “Database of Embodied Quantity Outputs (deQo).” https://www.carbondeqo.com; accessed in 09/2021.
- De Wolf, C., E. Hoxha, A. Hollberg, C. Fivet, and J. Ochsendorf. 2020. “Database of Embodied Quantity Outputs: Lowering Material Impacts Through Engineering.” Journal of Architectural Engineering 26 (3): 04020016. https://ascelibrary.org/doi/abs/10.1061/.
- De Wolf, C., F. Yang, D. Cox, A. Charlson, A. S. Hattan, and J. Ochsendorf. 2016. “Material Quantities and Embodied Carbon Dioxide in Structures.” Proceedings of the Institution of Civil Engineers – Engineering Sustainability 169 (4): 150–161. doi:10.1680/ensu.15.00033.
- Dimoudi, A., and C. Tompa. 2008. “Energy and Environmental Indicators Related to Construction of Office Buildings.” Resources, Conservation and Recycling 53 (1): 86–95. https://www.sciencedirect.com/science/article/pii/S0921344908001481.
- Ding, Grace K. C. 2008. “Sustainable Construction – the Role of Environmental Assessment Tools.” Journal of Environmental Management86 (3): 451–464. https://www.sciencedirect.com/science/article/pii/S0301479706004270.
- Dong, Yan, Simona Miraglia, Stefano Manzo, Stylianos Georgiadis, Hjalte Jomo Danielsen Sørup, Elena Boriani, Tine Hald, Sebastian Thöns, and Michael Z. Hauschild. 2018. “Environmental Sustainable Decision Making – the Need and Obstacles for Integration of LCA into Decision Analysis.” Environmental Science & Policy 87: 33–44. https://www.sciencedirect.com/science/article/pii/S1462901118302776.
- Eaton, K. J., and A. Amato. 1998. “A Comparative Life Cycle Assessment of Steel and Concrete Framed Office Buildings.” Journal of Constructional Steel Research 46 (1-3): 286–287. doi:10.1016/S0143-974X(98)00074-1.
- Eberhardt, Leonora Charlotte Malabi, Harpa Birgisdottir, and Morten Birkved. 2019. “Life Cycle Assessment of a Danish Office Building Designed for Disassembly.” Building Research and Information47 (6): 666–680. doi:10.1080/09613218.2018.1517458.
- Eberhardt, Leonora Charlotte Malabi, Morten Birkved, and Harpa Birgisdottir. 2020. “Building Design and Construction Strategies for a Circular Economy.” Architectural Engineering and Design Management 18: 93–113. https://doi.org/10.1080/17452007.2020.1781588.
- Eleftheriadis, S., P. Duffour, P. Greening, J. James, B. Stephenson, and D. Mumovic. 2018. “Investigating Relationships Between Cost and CO2 Emissions in Reinforced Concrete Structures Using a BIM-Based Design Optimisation Approach.” Energy and Buildings 166: 330–346. https://www.sciencedirect.com/science/article/pii/S0378778817320133.
- Ellingwood, B. R., and J. Y. Lee. 2016. “Life Cycle Performance Goals for Civil Infrastructure: Intergenerational Risk-Informed Decisions.” Structure and Infrastructure Engineering 12 (7): 822–829. https://doi.org/10.1080/15732479.2015.1064966.
- European Comission. 2021a. https://climate.ec.europa.eu/eu-action/eu-emissions-trading-system-eu-ets.
- European Comission. 2021b. “Emissions Database for Global Atmospheric Research (EDGAR), Release EDGAR v6.0 (1970–2018).” https://edgar.jrc.ec.europa.eu.
- Faber, M. H., and R. Rackwitz. 2004. “Sustainable Decision Making in Civil Engineering.” Structural Engineering International 14 (3): 237–242. https://doi.org/10.2749/101686604777963919.
- Faber, M. H., and J. D. Sørensen. 2003. “Reliability-Based Code Calibration: The JCSS Approach.” In Applications of Statistics and Probability in Civil Engineering, edited by A. D. Kiureghian, M. Samer, and J. M. Pestana, Vol. 2, 927–935. Rotterdam: Millpress.
- Feria, Margarida, and Miguel Amado. 2019. “Architectural Design: Sustainability in the Decision-Making Process.” Buildings 9 (5): 135. https://www.mdpi.com/2075-5309/9/5/135.
- Ferreiro-Cabello, Javier, Esteban Fraile-Garcia, Eduardo Martinez de Pison Ascacibar, and Francisco Javier Martinez de Pison Ascacibar. 2016. “Minimizing Greenhouse Gas Emissions and Costs for Structures with Flat Slabs.” Journal of Cleaner Production 137: 922–930. https://www.sciencedirect.com/science/article/pii/S0959652616310496.
- fib. 2022. “fib Model Code, Chapter 12: Principles of Structural Design and Assessment, Draft 2022.”
- Fischer, K., and M. H. Faber. 2012. “The LQI Acceptance Criterion and Human Compensation Costs for Monetary Optimization – A Discussion Note.” In LQI Symposium in Kgs. Lyngby, Denmark: Joint Committee on Structural Safety (JCSS).
- Fischer, K., C. Viljoen, J. Köhler, and M. H. Faber. 2019. “Optimal and Acceptable Reliabilities for Structural Design.” Structural Safety 76: 149–161. https://www.sciencedirect.com/science/article/pii/S0167473017302394.
- Foraboschi, Paolo, Mattia Mercanzin, and Dario Trabucco. 2014. “Sustainable Structural Design of Tall Buildings Based on Embodied Energy.” Energy and Buildings 68: 254–269. https://www.sciencedirect.com/science/article/pii/S0378778813005653.
- GABC. 2020. 2020 Global Status Report for Buildings and Construction. Report. Global Alliance for Buildings and Construction. United Nations Environment Programme.
- Gan, Vincent J. L., Jack C. P. Cheng, Irene M. C. Lo, and C. M. Chan. 2017. “Developing a CO2-e Accounting Method for Quantification and Analysis of Embodied Carbon in High-rise Buildings.” Journal of Cleaner Production 141: 825–836. https://www.sciencedirect.com/science/article/pii/S0959652616314676.
- García-Segura, T., and V. Yepes. 2016. “Multiobjective Optimization of Post-Tensioned Concrete Box-Girder Road Bridges Considering Cost, CO2 Emissions, and Safety.” Engineering Structures 125: 325–336. https://www.sciencedirect.com/science/article/pii/S0141029616303406.
- García-Segura, T., V. Yepes, J. Alcalá, and E. Pérez-López. 2015. “Hybrid Harmony Search for Sustainable Design of Post-Tensioned Concrete Box-Girder Pedestrian Bridges.” Engineering Structures 92: 112–122. https://www.sciencedirect.com/science/article/pii/S0141029615001510.
- Gervásio, H., and L. S. da Silva. 2008. “Comparative Life-Cycle Analysis of Steel-Concrete Composite Bridges.” Structure and Infrastructure Engineering 4 (4): 251–269. https://doi.org/10.1080/15732470600627325.
- GLOBE. 2022. “Joint Committee on the Global Consensus on Sustainability in the Built Environment.” Decarbonizing Global Construction – Draft, September. https://www.rilem.net/globe.
- Gollier, Christian. 2010. “Ecological Discounting.” Journal of Economic Theory 145 (2): 812–829. Judgment Aggregation, https://www.sciencedirect.com/science/article/pii/S002205310900115X.
- Gustavsson, L., and A. Joelsson. 2010. “Life Cycle Primary Energy Analysis of Residential Buildings.” Energy and Buildings 42 (2): 210–220. https://www.sciencedirect.com/science/article/pii/S0378778809002102.
- Gustavsson, L., K. Pingoud, and R. Sathre. 2006. “Carbon Dioxide Balance of Wood Substitution: Comparing Concrete- and Wood-Framed Buildings.” Mitigation and Adaptation Strategies for Global Change 11 (3): 667–691. https://doi.org/10.1007/s11027-006-7207-1.
- Hájek, P., C. Fiala, and M. Kynčlová. 2011. “Life Cycle Assessments of Concrete Structures – a Step Towards Environmental Savings.” Structural Concrete 12 (1): 13–22. https://onlinelibrary.wiley.com/doi/abs/10.1002/suco.201000026.
- Hammond, G. P., and C. I. Jones. 2008. “Embodied Energy and Carbon in Construction Materials.” Proceedings of the Institution of Civil Engineers – Energy and Buildings 161 (2): 87–98. doi:10.1680/ener.2008.161.2.87.
- Hart, Jim, Bernardino D'Amico, and Francesco Pomponi. 2021. “Whole-Life Embodied Carbon in Multistory Buildings: Steel, Concrete and Timber Structures.” Journal of Industrial Ecology 25 (2): 403–418. https://onlinelibrary.wiley.com/doi/abs/10.1111/jiec.13139.
- Hawkins, W., S. Cooper, S. Allen, J. Roynon, and T. Ibell. 2021. “Embodied Carbon Assessment Using a Dynamic Climate Model: Case-Study Comparison of a Concrete, Steel and Timber Building Structure.” Structures 33: 90–98. https://www.sciencedirect.com/science/article/pii/S2352012420307323.
- Hingorani, Ramon, Peter Tanner, Miguel Prieto, and Carlos Lara. 2020. “Consequence Classes and Associated Models for Predicting Loss of Life in Collapse of Building Structures.” Structural Safety 85: 101910. https://www.sciencedirect.com/science/article/pii/S0167473019303741.
- Holický, M., and M. Sýkora. 2021. “Reliability Approaches Affecting the Sustainability of Concrete Structures.” Sustainability 13 (5) 2627. doi:10.3390/su13052627.
- Honfi, D. 2014. “Serviceability Floor Loads.” Structural Safety 50: 27–38. https://www.sciencedirect.com/science/article/pii/S0167473014000228.
- Huang, L., R. A. Bohne, A. Bruland, P. D. Jakobsen, and J. Lohne. 2015. “Life Cycle Assessment of Norwegian Road Tunnel.” The International Journal of Life Cycle Assessment 20 (2): 174–184. https://doi.org/10.1007/s11367-014-0823-1.
- Huang, L., G. Krigsvoll, F. Johansen, Y. Liu, and X. Zhang. 2018. “Carbon Emission of Global Construction Sector.” Renewable and Sustainable Energy Reviews 81: 1906–1916. doi:10.1016/j.rser.2017.06.001.
- IBU, Institut Bauen und Umwelt e.V. 2018. “Environmental Product Declaration as per /ISO 14025/ and /EN 15804. Structural Steel: Sections and Plates. Declaration Number: EPD-BFS-20180116-IBG2-EN.” https://www.ibu-epd.com.
- Ismail, Mohamed A., and Caitlin T. Mueller. 2021. “Minimizing Embodied Energy of Reinforced Concrete Floor Systems in Developing Countries Through Shape Optimization.” Engineering Structures 246: 112955. https://www.sciencedirect.com/science/article/pii/S0141029621010993.
- ISO. 2015. “ISO 2394. General Principles on Reliability for Structures, 4th Edition.”
- Jönsson, A., T. Björklund, and A. M. Tillman. 1998. “LCA of Concrete and Steel Building Frames.” The International Journal of Life Cycle Assessment 3 (4): 216–224. https://doi.org/10.1007/BF02977572.
- Kaethner, S. C., and J. A. Burridge. 2012. “Embodied CO2 of Structural Frames.” Structural Engineer90 (5): 33–40. https://www.istructe.org/journal/volumes/volume-90-(2012)/issue-5/embodied-co2-of-structural-frames/
- Kalusche, W., and S. Herke. 2020. BKI Baukosten 2020 Neubau. Statistische Kostenkennwerte für Gebäude. Stuttgart: BKI, Baukosteninformationszentrum.
- Kaveh, A. 2017. Cost and CO2 Emission Optimization of Reinforced Concrete Frames Using Enhanced Colliding Bodies Optimization Algorithm, 319–350. Cham: Springer International Publishing. https://doi.org/10.1007/978-3-319-48012-1_17.
- Kaveh, A., R. A. Izadifard, and L. Mottaghi. 2020. “Optimal Design of Planar RC Frames Considering CO2 Emissions Using ECBO, EVPS and PSO Metaheuristic Algorithms.” Journal of Building Engineering28: 101014. https://www.sciencedirect.com/science/article/pii/S2352710219316341.
- KC, S., and D. Gautam. 2021. “Progress in Sustainable Structural Engineering: A Review.” Innovative Infrastructure Solutions 6: 68. doi:10.1007/s41062-020-00419-3.
- Khan, F. R., and J. Rankine. 1981. Tall Building Systems and Concepts. New York: American Society of Civil Engineers (ASCE).
- Kim, S., J.-H. Moon, Y. Shin, G.-H. Kim, and D.-S. Seo. 2013. “Life Comparative Analysis of Energy Consumption and CO2 Emissions of Different Building Structural Frame Types.” The Scientific World Journal 2013: 175702. https://doi.org/10.1155/2013/175702.
- Köhler, Jochen, and Michele Baravalle. 2019. “Risk-Based Decision Making and the Calibration of Structural Design Codes – Prospects and Challenges.” Civil Engineering and Environmental Systems36 (1): 55–72. https://doi.org/10.1080/10286608.2019.1615477.
- Kriegler, E., K. Riahi, N. Petermann, V. Bosetti, P. Capros, D. P. van Vuuren, P. Criqui, et al. 2014. Assessing Pathways Toward Ambitious Climate Targets at the Global and European Levels. A Synthesis of Results from the AMPERE Project. Report. https://ampere-project.eu.
- Lausselet, Carine, Johana Paola Forero Urrego, Eirik Resch, and Helge Brattebø. 2021. “Temporal Analysis of the Material Flows and Embodied Greenhouse Gas Emissions of a Neighborhood Building Stock.” Journal of Industrial Ecology 25 (2): 419–434. https://onlinelibrary.wiley.com/doi/abs/10.1111/jiec.13049.
- Lee, J. Y., and B. R. Ellingwood. 2015. “Ethical Discounting for Civil Infrastructure Decisions Extending Over Multiple Generations.” Structural Safety 57: 43–52. https://www.sciencedirect.com/science/article/pii/S0167473015000466.
- Lentz, A. 2007. “Acceptability of Civil Engineering Decisions Involving Human Consequences.” Thesis.
- Lu, H. R., A. El Hanandeh, and B. P. Gilbert. 2017. “A Comparative Life Cycle Study of Alternative Materials for Australian Multi-Storey Apartment Building Frame Constructions: Environmental and Economic Perspective.” Journal of Cleaner Production 166: 458–473. https://www.sciencedirect.com/science/article/pii/S095965261731781X.
- McKinsey. 2017. “Reinventing Construction: A Route to Higher Productivity.” Press Release, February 2017.
- Meinen, N. E., and R. D. J. M. Steenbergen. 2018. “Reliability Levels Obtained by Eurocode Partial Factor Design – a Discussion on Current and Future Reliability Levels.” Heron 63 (3): 243–302.
- Mergos, P. E. 2018. “Contribution to Sustainable Seismic Design of Reinforced Concrete Members Through Embodied CO2 Emissions Optimization.” Structural Concrete 19 (2): 454–462. https://onlinelibrary.wiley.com/doi/abs/10.1002/suco.201700064.
- Miller, D., and J.-H. Doh. 2015. “Incorporating Sustainable Development Principles into Building Design: A Review from a Structural Perspective Including Case Study.” The Structural Design of Tall and Special Buildings 24 (6): 421–439. https://onlinelibrary.wiley.com/doi/abs/10.1002/tal.1172.
- Miller, D., J.-H. Doh, and M. Mulvey. 2015. “Concrete Slab Comparison and Embodied Energy Optimisation for Alternate Design and Construction Techniques.” Construction and Building Materials80: 329–338. https://www.sciencedirect.com/science/article/pii/S0950061815001051.
- Moon, K. S. 2008. “Sustainable Structural Engineering Strategies for Tall Buildings.” The Structural Design of Tall and Special Buildings 17 (5): 895–914. https://onlinelibrary.wiley.com/doi/abs/10.1002/tal.475.
- Mottaghi, L., R. A. Izadifard, and A. Kaveh. 2021. “Factors in the Relationship Between Optimal CO2 Emission and Optimal Cost of the RC Frames.” Periodica Polytechnica Civil Engineering 65 (1): 1–14. https://pp.bme.hu/ci/article/view/16790.
- Müller, Daniel B., Gang Liu, Amund N. Løvik, Roja Modaresi, Stefan Pauliuk, Franciska S. Steinhoff, and Helge Brattebø. 2013. “Carbon Emissions of Infrastructure Development.” Environmental Science and Technology 47 (20): 11739–11746. https://doi.org/10.1021/es402618m.
- Nahangi, Mohammad, Gursans Guven, Bolaji Olanrewaju, and Shoshanna Saxe. 2021. “Embodied Greenhouse Gas Assessment of a Bridge: A Comparison of Preconstruction Building Information Model and Construction Records.” Journal of Cleaner Production 295: 126388. doi:10.1016/j.jclepro.2021.126388.
- Nishijima, K., M. H. Faber, and D. Straub. 2007a. “Inter-Generational Distribution of the Life-Cycle Cost of an Engineering Facility.” Journal of Reliability of Structures and Materials 1 (3): 33–46.
- Nishijima, K., M. H. Faber, and D. Straub. 2007b. “Sustainable Decisions for Life-Cycle Based Design and Maintenance.” Australian Journal of Civil Engineering 4 (1): 59–72. https://doi.org/10.1080/14488353.2007.11463928.
- Niu, Y., R. Hingorani, G. Fink, and J. Köhler. 2022. “Design Concept for the Sustainable Use of Timber in Structures.” In ICOSSAR 2021–22, 13th International Conference on Structural Safety and Reliability. Shanghai: International Association for Structural Safety and Reliability (IASSAR).
- Nordhaus, W. 2017. “Revisiting the Social Cost of Carbon.” Proceedings of the National Academy of Sciences 114 (7): 1518. https://www.pnas.org/content/114/7/1518.abstract.
- Oladazimi, A., S. Mansour, and S. A. Hosseinijou. 2020. “Comparative Life Cycle Assessment of Steel and Concrete Construction Frames: A Case Study of Two Residential Buildings in Iran.” Buildings 10 (3): 54. doi:10.3390/buildings10030054.
- Paya-Zaforteza, I., V. Yepes, A. Hospitaler, and F. González-Vidosa. 2009. “CO2-Optimization of Reinforced Concrete Frames by Simulated Annealing.” Engineering Structures 31 (7): 1501–1508. https://www.sciencedirect.com/science/article/pii/S0141029609000996.
- Pindyck, R. S. 2017. “Coase Lecture–Taxes, Targets and the Social Cost of Carbon.” Economica 84 (335): 345–364. https://doi.org/10.1111/ecca.12243.
- Pindyck, R. S. 2017. “The Use and Misuse of Models for Climate Policy.” Review of Environmental Economics and Policy 11 (1): 100–114. https://doi.org/10.1093/reep/rew012.
- Pomponi, Francesco, and Alice Moncaster. 2016. “Embodied Carbon Mitigation and Reduction in the Built Environment – What Does the Evidence Say?” Journal of Environmental Management 181: 687–700. https://www.sciencedirect.com/science/article/pii/S0301479716305746.
- Rackwitz, R. 2000. “Optimization – the Basis of Code-Making and Reliability Verification.” Structural Safety 22 (1): 27–60. https://www.sciencedirect.com/science/article/pii/S0167473099000375.
- Rackwitz, R., A. Lentz, and M. Faber. 2005. “Socio-Economically Sustainable Civil Engineering Infrastructures by Optimization.” Structural Safety 27 (3): 187–229. https://www.sciencedirect.com/science/article/pii/S0167473004000487.
- Röck, Martin, Marcella Ruschi Mendes Saade, Maria Balouktsi, Freja Nygaard Rasmussen, Harpa Birgisdottir, Rolf Frischknecht, Guillaume Habert, Thomas Lützkendorf, and Alexander Passer. 2020. “Embodied GHG Emissions of Buildings – the Hidden Challenge for Effective Climate Change Mitigation.” Applied Energy 258: 114107. https://www.sciencedirect.com/science/article/pii/S0306261919317945.
- Rodriguez-Nikl, T., J. W. Christiansen, and K. Walters. 2012. “Reliability-Based Life Cycle Assessment of Green Concrete Structures.” In ACI Symposium, Vol. 289, 1–13. American Concrete Institute (ACI)
- Sakai, K. 2005. “Environmental Design for Concrete Structures.” Journal of Advanced Concrete Technology 3 (1): 17–28. doi:10.3151/jact.3.17.
- Sakai, K., T. Shibata, A. Kasuga, and H. Nakamura. 2016. “Sustainability Design of Concrete Structures.” Structural Concrete17 (6): 1114–1124. https://onlinelibrary.wiley.com/doi/abs/10.1002/suco.201600069.
- SAKO. 1999. Basis of design of structures. Proposals for Modification of Partial Safety Factors in Eurocodes. Report. Joint Committee of Nordic Committee on Building Regulations (NKB) and Nordic Standardization in the Construction Field (INSTA-B).
- Sarkisian, M., and D. Shook. 2014. “Embodied Carbon in Structures and Cities.” In IABSE Symposium Madrid: Engineering for Progress, Nature and People, 3166–3173. Zurich: International Association for Bridge and Structural Engineering (IABSE).
- Sartori, I., and A. G. Hestnes. 2007. “Energy Use in the Life Cycle of Conventional and Low-energy Buildings: A Review Article.” Energy and Buildings 39 (3): 249–257. https://www.sciencedirect.com/science/article/pii/S0378778806001873.
- Saxe, Shoshanna, Gursans Guven, Lucas Pereira, Alessandro Arrigoni, Tamar Opher, Adrien Roy, Aldrick Arceo, et al. 2020. “Taxonomy of Uncertainty in Environmental Life Cycle Assessment of Infrastructure Projects.” Environmental Research Letters 15 (8): 083003. https://dx.doi.org/10.1088/1748-9326/ab85f8.
- Simonen, K., B. Rodriguez, and C. De Wolf. 2017. “Benchmarking the Embodied Carbon of Buildings.” Technology, Architecture + Design 1 (2): 208–218. doi:10.1080/24751448.2017.1354623.
- Skullestad, Julie Lyslo, Rolf André Bohne, and Jardar Lohne. 2016. “High-Rise Timber Buildings as a Climate Change Mitigation Measure – a Comparative LCA of Structural System Alternatives.” Energy Procedia 96: 112–123. Sustainable Built Environment Tallinn and Helsinki Conference SBE16, https://www.sciencedirect.com/science/article/pii/S1876610216307512.
- Sørensen, J. D., I. B. Kroon, and M. H. Faber. 1994. “Optimal Reliability-Based Code Calibration.” Structural Safety 15 (3): 197–208. https://www.sciencedirect.com/science/article/pii/016747309490040X.
- Tanner, P., J. L. Bellod, and D. Sanz. 2018. “Paper and Pencil in the Age of BIM.” Structural Engineering International 28 (4): 396–407. https://doi.org/10.1080/10168664.2018.1496782.
- Tanner, P., and R. Hingorani. 2015. “Acceptable Risks to Persons Associated with Building Structures.” Structural Concrete 16 (3): 314–322. https://onlinelibrary.wiley.com/doi/pdf/10.1002/suco.201500012.
- Tol, R. S. J. 2019. “A Social Cost of Carbon for (Almost) Every Country.” Energy Economics 83: 555–566. https://www.sciencedirect.com/science/article/pii/S014098831930218X.
- Vilutiene, Tatjana, Gvidas Kumetaitis, Arvydas Kiaulakis, and Darius Kalibatas. 2020. “Assessing the Sustainability of Alternative Structural Solutions of a Building: A Case Study.” Buildings 10 (2): 36. doi:10.3390/buildings10020036.
- Virguez, E., and M. H. Faber. 2011. “Supporting Decisions on Global Health and Life Safety Investments.” In Proceedings of the 11th International Conference on Applications of Statistics and Probability in Civil Engineering (ICASP), 434–443. Zurich: CRC Press/Balkema.
- Vukotic, L., R. A. Fenner, and K. Symons. 2010. “Assessing Embodied Energy of Building Structural Elements.” Proceedings of the Institution of Civil Engineers – Engineering Sustainability 163 (3): 147–158. https://www.icevirtuallibrary.com/doi/abs/10.1680/ensu.2010.163.3.147.
- Webb, D., and B. M. Ayyub. 2017. “Sustainability Quantification and Valuation. I: Definitions, Metrics, and Valuations for Decision Making.” ASCE-ASME Journal of Risk and Uncertainty in Engineering Systems, Part A: Civil Engineering 3 (3): E4016001. https://doi.org/10.1061/AJRUA6.0000893.
- Weikard, Hans-Peter, and Xueqin Zhu. 2005. “Discounting and Environmental Quality: When Should Dual Rates be Used?” Economic Modelling 22 (5): 868–878. https://www.sciencedirect.com/science/article/pii/S0264999305000453.
- Wiik, M. K., E. Selvig, M. Fuglseth, C. Lausselet, E. Resch, I. Andresen, H. Brattebø, and U. Hahn. 2020. “GHG Emission Requirements and Benchmark Values for Norwegian Buildings.” In IOP Conference Series: Earth and Environmental Science. Vol. 588, 022005. IOP Publishing Ltd. doi:10.1088/1755-1315/588/2/022005.
- Yepes, V., J. V. Martí, and T. García-Segura. 2015. “Cost and CO2 Emission Optimization of Precast–Prestressed Concrete U-Beam Road Bridges by a Hybrid Glowworm Swarm Algorithm.” Automation in Construction 49: 123–134. https://www.sciencedirect.com/science/article/pii/S0926580514002246.
- Ženíšek, M., J. Pežta, M. Tipka, V. Kočí, and P. Hájek. 2020. “Optimization of RC Structures in Terms of Cost and Environmental Impact–Case Study.” Sustainability 12 (20): 8532. https://www.mdpi.com/2071-1050/12/20/8532.
- Zhang, Chao, Muhammad Amaduddin, and Lee Canning. 2011. “Carbon Dioxide Evaluation in a Typical Bridge Deck Replacement Project.” Proceedings of the Institution of Civil Engineers – Energy 164 (4): 183–194. doi:10.1680/ener.2011.164.4.183.
Appendix A.
Assumptions for the case study (Section 4)
Table A1. Characteristic values for geometry, material and load variables.
Table A2. Probabilistic models; Source: (CEN Citation2022).
Table A3. Assumptions related to the cost model.