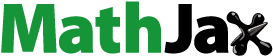
Abstract
Personalized nutrition (PN) has gained much attention as a tool for empowerment of consumers to promote changes in dietary behavior, optimizing health status and preventing diet related diseases. Generalized implementation of PN faces different obstacles, one of the most relevant being metabolic characterization of the individual. Although omics technologies allow for assessment the dynamics of metabolism with unprecedented detail, its translatability as affordable and simple PN protocols is still difficult due to the complexity of metabolic regulation and to different technical and economical constrains. In this work, we propose a conceptual framework that considers the dysregulation of a few overarching processes, namely Carbohydrate metabolism, lipid metabolism, inflammation, oxidative stress and microbiota-derived metabolites, as the basis of the onset of several non-communicable diseases. These processes can be assessed and characterized by specific sets of proteomic, metabolomic and genetic markers that minimize operational constrains and maximize the information obtained at the individual level. Current machine learning and data analysis methodologies allow the development of algorithms to integrate omics and genetic markers. Reduction of dimensionality of variables facilitates the implementation of omics and genetic information in digital tools. This framework is exemplified by presenting the EU-Funded project PREVENTOMICS as a use case.
Introduction
Personalized nutrition (PN) has gained great attention in the last decade and is currently one of the top trends in nutritional sciences (Callahan Citation2022). PN offers the possibility of adapting eating behavior to personal needs and preferences, but mainly to harness optimal benefits. From the consumer’s point of view, PN represents a natural path to empowerment, facilitating decision-taking processes impacting on very different domains of their lives, such as physical activity performance, mental and physical wellbeing, or overall health improvement. Moreover, PN is also gaining relevance from the perspective of healthcare systems, since a wide array of highly prevalent non-communicable diseases (NCDs) that put a high socio-economic burden on society, are directly linked with dietary patterns and eating behavior (Afshin et al. Citation2019; Cena and Calder Citation2020; Forouhi and Unwin Citation2019; Moszak, Szulińska, and Bogdański Citation2020). A well-known example is obesity and obesity-related diseases, which are tightly linked to unbalanced behaviors in terms of physical activity, mental distress, and eating patterns.
Despite the acceptance in the last decades of the benefits of healthy dietary habits for promoting health and wellbeing by the scientific community as a whole, it has been repeatedly demonstrated that general nutrition recommendations, even those adopted in national and international polices, are not sufficient to promote the required changes in the eating behavior of individuals. This is highlighted by large cohort studies such as the Global Burden of Disease (GBD) study, reporting steady increases in diet-associated metabolic risks such as a high body mass index (BMI) and a high fasting glucose concentration accompanied by no substantial changes in diet quality (Chew et al. Citation2023; Murray et al. Citation2020). Whilst regulatory policies and taxations have had remarkable results for tobacco and lead exposure, the application of similar initiatives in domains related to diet and nutrition is underutilized (Gakidou et al. Citation2017; Martini et al. Citation2021; Sacks, Kwon, and Backholer Citation2021). Thus, according to GBD, since 1990 the health loss has shifted toward a growing burden from NCDs and away from communicable, maternal, neonatal, and nutrient deficiency diseases, the latter linked to specific nutrient deficiencies, but not to metabolic alterations associated with unbalanced dietary habits. In this scenario, PN arises as an opportunity to develop new tools for promoting long lasting changes in dietary behaviors, paving the way to new strategies for improving metabolic alterations with a relevant impact on health, understood by the World Health Organization as “a state of complete physical, mental and social well-being and not merely the absence of disease or infirmity” (Huber et al. Citation2011). Complementary to this definition of health, in 1943, French physician Georges Canguilhem proposed to understand health as the ability to adapt to one’s environment: it varies for every individual, depending on their circumstances (The Lancet Citation2009). This latter definition aligns with the concept of personalization.
Recent advances in technology, from information and communication technologies (ICT) to cutting edge analytical technologies, are key allies to PN. Advances in wearables and biosensors for personal monitoring provide a better understanding of the individual, enabling adaptation of recommendations to the immediate needs of the user and an optimal communication pathway for delivering these recommendations together with strategies for promoting behavioral change (Kim and Seo Citation2021; Mandracchia et al. Citation2019; Sempionatto et al. Citation2021). In parallel, analytical techniques in the field of omics sciences provide the necessary tools to understand the physiological state of the individual in relation to genetic background, enabling the elaboration of recommendations for the mid- and long-term. Likely PN will be most successful if it specifically addresses metabolic deviations that can be nutritionally targeted by specific dietary choices, with the outcomes measured and made visible to the consumer. In this process, specific genetically determined susceptibilities and risks may be taken into account, together with the phenotypic characterization of the individual. Despite these advances might facilitate and boost deployment of PN in society, it must be taken into account that a reliable PN system should target key elements of the food environment of the consumer, which is the context that influences and drives the decision making process for food choices (Nutrition and Food Systems Division Citation2016). This involves development of simple, flexible PN systems able to interoperate with different components of the consumers’ food environment, such as nutrition professionals, retailers, food producers, and even policy makers.
An strategy to simplify PN is based in metabotyping, which allows classification of individuals according to their metabolic signature, which in turn can be associated to health status (Cirulli et al. Citation2019), dietary patterns and response to interventions (Garcia-Perez et al. Citation2020; Posma et al. Citation2020). In this approach to PN, individuals are grouped in an unbiased manner to smaller, relatively homogenous groups according to their metabotype, as functional groups for personalized nutritional recommendations. This concept can be further extended to the concept of precision nutrition, in which the individual characteristics are the main driver of the recommendations (Ordovas et al. Citation2018; Picó et al. Citation2019; Tebani and Bekri Citation2019). Nevertheless, although metabolic markers can be currently applied to obtain a precise overview of the state of metabolic health of the person at the metabolite level, nutritional recommendations are still far away from the precision that is needed to fulfill the specific requirements of everyone. This apparent gap is a consequence of the lack of knowledge on the effects of individual eating behaviors on specific elements of metabolic health, also because the complexity of food itself is further confounded by the complex processes of digestion, absorption, and metabolism, and interactions and signaling properties of food components, which in turn are dependent on individual’s intrinsic and environmental exposure factors (Barabási, Menichetti, and Loscalzo Citation2019). Although the generation of knowledge to establish causal links between specific foods and health can be seen as a long-time journey, the state of the art already allows to propose some novel strategies to tailor nutritional recommendations to functional, metabo-phenotypically relative homogenous groups, placed between generic concepts of nutrition based on population groups and individuality, the latter requiring precision nutrition.
In this context, although omics technologies allow for assessment the dynamics of metabolism and characterization of the individual with unprecedented detail, the use as an effective, affordable and simple PN tool is still difficult due to the complexity of metabolic regulation and to the various technical and economical constrains. Therefore, we here provide a conceptual framework on how omics biomarkers could be implemented for a practical effective PN advice. In section “Personalization based on overarching processes,” this framework is developed taking constraints, such as reliability of biomarkers according to the state of the art and feasibility of analysis in terms of benefit-cost ratio, into account. Section “Biomarkers for assessing the status of core health processes” then reviews various metabolic and proteomics biomarkers, detectable in accessible biofluids such as blood and urine, compliant with the above premises and this section proposes a methodology for their integration in accord with current state of the art. Since individual genetic variation impacts susceptibility to health derailment as well as effectiveness of dietary recommendations for health improvement, section “Genetic markers to accommodate the susceptibility of the individual” reviews genetic markers that might be considered to complement the information provided by proteomics and metabolomic biomarkers described previously. In section “Practical implementation of the personalized nutrition framework: the PREVENTOMICS project as a use case,” a concrete implementation example is provided. It describes, as one possible translation option, how this approach is used in the EU-funded project PREVENTOMICS, which was conceived as a proof of concept from selecting biomarkers, as described above, to tangible tools for integration and for empowerment of the consumer. The latter is envisioned as an app that provides dietary, shopping or cooking advise, guided by the individual’s aggregated biomarker profile and genetic susceptibility. The last section (section “Other omics with potential applications”) considers consumption biomarkers and gut microbiome dynamics as other aspects to be considered in this PN strategy and sketches future directions.
Personalization based on overarching processes
Current PN based on metabolic profiling relies on the association of metabolic footprints with specific phenotypes. This approach faces two main challenges. The first one is related to the concept of health itself. Associating a metabolic profile with the presence or absence of a disease is feasible and different approaches and applications can be found in the literature (Cirulli et al. Citation2019; Garcia-Perez et al. Citation2020; Posma et al. Citation2020). While this strategy has been proven successful in the case of clinically relevant metabolic alterations, it still lacks a wider applicability in the general population for prevention of disease and maintaining physiological homeostasis, that is, the correct performance of the different processes that can buffer the deleterious consequences of daily stressors on metabolism. These stressors can be understood as environmental factors such as psychological stress, pathogens, pollution, allergens, smoking or diet, among others, as well as internal factors such as inborn alterations or genetically-driven up/down-regulation of some metabolic processes. A decade ago, Van Ommen et al. proposed that many diseases arise from the imbalanced homeostasis of overarching processes due mainly to four stressors; metabolic stress, inflammatory stress, oxidative stress and psychological stress (van Ommen et al. Citation2009). Accordingly, a good health is not the absence of disease alone but requires the maintenance and support of these overarching processes controlling health status. This concept provides a basis for quantifying health.
The second challenge of PN is the current lack of available biomarkers that describe deviations from homeostatic balance, especially biomarkers that would be able to capture early deviations from a completely optimal health state (Elliott et al. Citation2007). This is due to the fact that a functional, even suboptimal, homeostasis tends to maintain the levels of circulating molecules (i.e. hormones, cytokines, metabolites) within a certain range of values (Picó et al. Citation2019; van Ommen et al. Citation2008; van Ommen et al. Citation2014). During the basal (fasting) state, these circulating molecules fall outside boundaries of normality only when the homeostatic capacity of the individual has been exceeded due to a physiological insult or, worse, a disease. Therefore, in a non-diseased individual quantification of biomarkers that are indicative of their health status likely associate with disease onset and progression or are, at most, risk indexes. Under this scenario, measuring the health status of the individual becomes a challenge, because, without extensively stressing the individual, it is not possible to measure how far a process is from the point of disruption of homeostasis. In other words, capturing alterations in the overarching processes is currently a challenge, but it would open up a wide array of possibilities for personalization strategies toward an improved health status.
It has been proposed that challenging homeostasis through different experimental approaches based on punctual application of stressors might be useful to measure the buffering capacity of the individual counteracting the effects of the given stressor (namely phenotypic flexibility) (Broek et al. Citation2017; Hoevenaars et al. Citation2020; van Ommen et al. Citation2008; van Ommen et al. Citation2014). Deviations of this capacity are interpreted as altered homeostatic robustness that can result in metabolic derailments in the future, leading to the onset of disease (van Ommen et al. Citation2009). Nevertheless, translating such an approach into a tool for individual characterization would involve (a battery of) functional tests that are difficult to scale and deploy in current healthcare systems or to apply in the context of PN. In particular, it is not realistic for participants to perform “stress-tests” at home, because standardization is required and often specialized, large or expensive equipment or ethical or safety constraints apply for sampling. An applicable approach should be relatively simple in terms of what the individual has to do and should involve limited sampling or even be noninvasive. Such an alternative approach may be feasible when based on the idea that the overarching processes indicated above are associated to core metabolic pathways and physiological processes (core health processes). We propose that five core health processes, that is, carbohydrate metabolism, lipid metabolism, oxidative stress, systemic inflammation, and microbiota status, recapitulate, at least in significant part, the overarching processes governing health. A functional characteristic of these core health processes is that they can be assessed and quantified by means of biomarkers measured by omics technologies, mainly metabolomics and proteomics. Therefore, we propose that a practical approach could consist of determining measurable alterations of the core health processes under a fixed condition, such as after an overnight fast, assuming that this, at least in part, captures the state of the overarching processes. In doing this it should be realized that large interindividual variation in biomarkers exists (Hageman et al. Citation2019; Janssen et al. Citation2023). Moreover, several studies have shown a marked within person variability in the glycemic response (Matthan et al. Citation2016; Vega-López et al. Citation2007). To standardize analysis conditions and to reduce the person variability, overnight fasting is well-accepted to reduce the differences observed in the post-absorptive state, acknowledging that the impact of fasting may impact carbohydrate metabolism significantly (Meng et al. Citation2017). Further standardization may be achieved by also applying a standardized meal on the evening before sampling and by refraining from heavy physical activity at least 24 h before sampling (Janssen et al. Citation2023). This type of additional standardization has to be weighed against ease of implementation and level of adherence.
The main characteristic of the core health processes (lipid metabolism, carbohydrate metabolism, systemic inflammation, oxidative stress, and microbiome status (Hou et al. Citation2022) is that each one recapitulates different and complementary aspects of metabolism and that they can be conceived as relatively independent clusters of different metabolites and proteins biomarkers. Some of these biomarkers are currently recognized as established clinical biomarkers or are in advanced research stages (i.e. meta-analyses of clinical studies) as indicators and predictors of specific conditions or metabolic alterations. These emerging and consolidated biomarkers, combined by algorithms assisted by data analysis and machine learning techniques, can provide a measurement of the state of each core health process. The main advantage of this approach is that each core health process can be captured (functionally represented) by a combination of different biomarkers, with each of the markers associated with a slightly different aspect of metabolism. For example, in case of inflammation, blood C-reactive protein (CRP) concentration is indicative of systemic inflammatory state, whilst levels of monocyte chemoattractant protein-1 (MCP1) can be understood as an inflammatory response of the adipose tissue, at least in an obesogenic background (Choe et al. Citation2016). Moreover, N-acetylglycosylated proteins, a new type of composite biomarker discussed below, offer information related with low grade chronic inflammation (Chiesa et al. Citation2022). Thus, in terms of physiology and health-to-disease progression, the information provided by these biomarkers is complementary. Combining biomarkers by taking into consideration both the relevance for health-to-disease progression and blood concentrations might sensitively capture changes in homeostasis of inflammation. Therefore, we propose that subtle changes in a metabolic process, undetectable when biomarkers are considered separately, might become detectable if different biomarkers are considered as a single composite biomarker. The combination of markers thus provides a health signature, corresponding to the core health processes. In this, different weights should be applied to the markers, to allow that important changes in a single, but relevant biomarker of the signature is taken into account, even in the unlikely situation that other biomarkers of the same signature remain unaltered.
Another fundamental characteristic of this design is that core health processes can be associated, at least to some extent, with dietary advice according to the current knowledge on dietary patterns, food bioactive compounds and dietary supplements (Guasch-Ferré and Willett Citation2021; Jayedi et al. Citation2018; Jiang, Liu, and Li Citation2021; Milajerdi et al. Citation2020; Ni et al. Citation2022; Papakonstantinou et al. Citation2022; Shivappa et al. Citation2017; Xu et al. Citation2021). For example, when the core health process lipid metabolism, but not the other four health processes carbohydrate metabolism, systemic inflammation, oxidative stress or microbiome status, is affected, it can be targeted specifically. Moreover, nutritional recommendations might be aligned with current regulations on the use of nutrition and health claims. Thus, according to European regulation (EC) 1924/2006, health claims made on food should not be used unless scientific evidence has been approved by expert panels (EUR-Lex Citation2014). Health claims have been grouped in six different domains, namely: appetite ratings, weight management, and blood glucose concentrations (EFSA Panel on Dietetic Products. Nutrition and Allergies (NDA) Citation2012a); antioxidants, oxidative damage and cardiovascular health (Turck, Bresson, et al. Citation2018); bone, joints, skin, and oral health (EFSA Panel on Dietetic Products. Nutrition and Allergies (NDA) Citation2012b); nervous system, including psychological functions (EFSA Panel on Dietetic Products. Nutrition and Allergies (NDA) Citation2012c); muscle function and physical performance (Turck, Castenmiller, et al. Citation2018); immune system, the gastrointestinal tract and defence against pathogenic microorganisms (EFSA Panel on Dietetic Products. Nutrition and Allergies (NDA) Citation2016). Therefore, although not fully aligned, the modularity of the PN system proposed herein allows to adapt dietary recommendations at different levels to the physiological characteristics of the person.
The last critical point is to define a set of biomarkers that could be easily implemented in an affordable PN strategy. Determinants are reproducibility and repeatability, but also affordability. In this sense, despite a wide array of analytical technologies being currently available, only a few display the dual principle of technical reliability and affordability. One methodology that is exemplary is proton nuclear magnetic resonance (1H-NMR). 1H-NMR is a rapid method of analysis, which allows for measurement of many metabolites in one (small volume) sample (Hernandez-Baixauli et al. Citation2020), with easy sample handling and being highly reproducible. It is therefore easily scalable. Despite the fact that setting up the methodology for a specific metabolite can be time consuming and sometimes is expensive due to the need for specific standards, once developed, the costs for measuring one analyte or hundreds of analytes is similar. 1H-NMR is currently established in biomedical research for large clinical studies and is steadily being adopted by industry for different production purposes (Emwas et al. Citation2019). In the PN strategy proposed here, selection of metabolite-based biomarkers has been restricted to those that can be quantified in human biofluids by 1H-NMR and, in turn, are of plausible metabolic significance. Blood is not only a rich source of metabolites and cells, but it is also a rich source of proteins. Omics analysis of proteins, proteomics, is another technology that might benefit from the advances in different platforms that allow simultaneous quantification of different molecules in a single biological sample. Many biomarkers that were traditionally determined individually by ELISA can be now simultaneously analyzed by flow cytometry or multiplex technologies (Smith and Gerszten Citation2017; Vashist Citation2021), see also (Correa Rojo et al. Citation2021). Implementation of robust, omics-based analysis of circulating proteins will make an even wider array of biomarkers available for PN strategies, but effective implementation of such markers will require standardization and appropriate quality assurance and control measures (Beger et al. Citation2019).
Biomarkers for assessing the status of core health processes
Determination of the various signatures, informing on the status of the previously introduced core health processes (a selection of measurable elements telling about the state of overarching processes relevant for maintaining an optimal health status), requires informative biomarkers. In this section, we propose a selection of such biomarkers, with sufficient scientific underpinning, reliability, and affordability. Individual biomarkers or sets of biomarkers form the components that together inform on a specific (metabolic) signature.
Components of the carbohydrate metabolism signature
Insulin resistance has become a worrying condition for a considerable part of the population (Freeman and Pennings Citation2022). It is one of the features of the metabolic syndrome (MetS) and is mainly caused by an altered metabolism of carbohydrates. Insulin resistance can often lead to type 2 Diabetes Mellitus (T2DM), and accounts for approximately 90% of T2DM cases worldwide, which also increases the risk for cardiovascular disease (CVD). Analysis of early deviations is possible by considering metabolites involved in various aspects of carbohydrate metabolism by metabolomics approaches (Goldberg et al. Citation2019; Roberts, Koulman, and Griffin Citation2014; Zhao et al. Citation2016). It is plausible to hypothesize that the assessment of novel biomarkers with predictive capabilities together with clinically accepted gold standard markers, could enable a better characterization of metabolic disturbances at early stages of the onset of carbohydrate-related diseases.
Glucose, insulin, HOMA-IR, leptin, adiponectin
High circulating glucose levels could be the consequence of insulin resistance in peripheral tissues and of pancreatic β-cell alterations which, if untreated, will consequently progress to T2DM (Brereton et al. Citation2016), making glucose a key marker for the diagnosis of this disease (Rawat et al. Citation2019), together with glycated hemoglobin (HbA1c) (Use of Glycated Haemoglobin (HbA1c) in the Diagnosis of Diabetes Mellitus Abbreviated Report of a WHO Consultation Citation2011) and insulin. In addition, the HOMA-IR index is the most frequently used index to calculate insulin resistance state based on fasting blood levels of glucose and insulin (van der Aa et al. Citation2017); according to the formula [HOMA-IR= (insulin × glucose)/22.5] (Matthews et al. Citation1985). The protein hormones leptin and adiponectin have opposing effects on insulin sensitivity, subclinical inflammation, endothelial function, and atherosclerosis. Elevated levels of leptin contribute to the development of insulin resistance and chronic inflammation, whereas adiponectin exerts insulin sensitizing, anti-inflammatory and cardioprotective effects (Finucane et al. Citation2009; López-Jaramillo et al. Citation2014).
Hydroxybutyrate and lactate
Acetoacetate, 3-hydroxybutyrate and acetone are ketone bodies, emerging as crucial regulators of metabolic health. Ketone bodies are produced in the liver from fatty acids and serve as a circulating substrate for cellular energy generation in situations of glucose deprivation (Philip C Calder Citation2017). Ketone bodies have a characteristic smell, which can easily be detected in the breath of persons with ketosis and ketoacidosis (Newman and Verdin Citation2014). Levels of 3-hydroxybutyrate (or β-hydroxybutyrate) increase over three orders of magnitude during prolonged fasting (Newman and Verdin Citation2014). Moreover, 3-hydroxybutyrate is described as a beneficial metabolite by conferring substantial protection against oxidative stress, via inhibition of deacetylases (Shimazu et al. Citation2013), which may explain, at least partially, the therapeutic effects of very low-carbohydrate and ketogenic diets. However, T2DM patients present increased 3-hydroxybutyrate levels (Shearer et al. Citation2008). Therefore, 3-hydroxybutyrate is an important marker, especially for early metabolic derailment, but it should be interpreted with caution.
Pyruvate is the end-product of glycolysis, a cytoplasmic ATP-generating process. Pyruvate can enter mitochondria and, after being converted to acetyl-CoA, is either oxidized (via the citric acid cycle and oxidative phosphorylation) or used as a substrate for fatty acid biosynthesis. Alternatively, pyruvate can be converted to lactate in the cytoplasm and be excreted from the cell. Thus, lactate reflects the balance between glycolytic and mitochondrial oxidative energy metabolism. Alterations in carbohydrate metabolism are also reflected in circulating lactate levels (Würtz et al. Citation2012). Indeed, altered metabolism in pancreatic β-cells results in abnormal accumulation of lactate in urine, blood and cerebrospinal fluid (Abu Bakar et al. Citation2015). Different clinical studies assessed lactate levels in patients with an altered metabolic profile, demonstrating it was increased in serum from patients with higher risk for MetS (Gogna et al. Citation2015; Pasanta et al. Citation2019; Würtz et al. Citation2012). Lactic acid plays a role in several biochemical processes and is produced in the muscles during intense physical activity, being subsequently cleared by the liver. Lactate is also an end-product of bacterial fermentation. Elevations in lactate have been consistently associated with T2DM and obesity. Changes in blood lactate during glucose tolerance tests are inversely correlated with fasting insulin (Berhane et al. Citation2015; Choi et al. Citation2002; Crawford et al. Citation2010; Gonzalez-Franquesa et al. Citation2016; Lovejoy et al. Citation1992; Rossi et al. Citation2018; Zhao et al. Citation2016).
Acylcarnitines
Acylcarnitines are esters of L-carnitine and fatty acids and facilitate transport of fatty acids into the mitochondrial matrix for β-oxidation, as a major source of energy for cellular activities. The function of acylcarnitines in intermediary metabolism is essential to maintain cellular bioenergetics (Reuter and Evans Citation2012). Disruption in β-oxidation results in elevated acylcarnitine concentrations (Koves et al. Citation2005). Although acylcarnitines are measured by liquid chromatography/mass spectrometry (LC/MS), and not by 1H-NMR, these metabolites inform well on lipid oxidation and overall substrate (lipid and carbohydrate) catabolism, as well as specific lipid and carbohydrate metabolism alterations. The profile of large spectrum of acylcarnitines, usually together with amino acids, can be accurately determined by LC/MS, which is a major attraction of using acylcarnitines as biomarker (Saito et al. Citation2017). Concentrations of some acylcarnitines were associated with MetS, obesity and pre-diabetes (Ha et al. Citation2012; Huffman et al. Citation2009; Mihalik et al. Citation2010; Zhang et al. Citation2014). Although the mechanistic link with mitochondrial dysfunction has yet to be fully elucidated (Beger et al. Citation2018), acylcarnitines have been associated to a pre-disease state. Among the different types of acylcarnitines, propionylcarnitine is the most promising short chain acylcarnitine as a pre-disease biomarker. In general, the levels of blood acylcarnitines reflect inadequate tissue acylcarnitine metabolism (Schooneman et al. Citation2014; van der Hoek et al. Citation2020), but propionylcarnitine seems to be an exception (Lagerwaard et al. Citation2021). In some studies of short-chain carnitine esters, propionylcarnitine has been positively associated with T2DM risk and insulin resistance (Bene et al. Citation2020). On the other hand, the combination of propionylcarnitine with other metabolites of interest, such as branched chain amino acids (BCAAs), glutamate/glutamine and methionine, was most robust in differentiating metabolically lean from obese patients (Dorcely et al. Citation2017; Gonzalez-Franquesa et al. Citation2016). In another clinical study, twenty-four acylcarnitines were measured in more than 1,000 subjects who were grouped by normal glucose tolerance, isolated impaired fasting glycemia, impaired glucose tolerance, or T2DM (Mai et al. Citation2013). Serum levels of propionylcarnitine differed significantly among the groups, highlighting its relevance as a robust biomarker of early stages of carbohydrate metabolism disorders (Mai et al. Citation2013). Thus, determination of propionyl carnitine seems to allow a precise view into the early development of metabolic diseases.
Glutamine and glutamate
Glutamine plays a crucial role in various cellular processes, such as energy balance, apoptosis, and cell proliferation. Its deprivation can activate fatty acid β-oxidation (Carlessi et al. Citation2019; Long et al. Citation2016) with a strong inverse association with the risk to develop T2DM (Abdul Ghani et al. Citation2019; Marta Guasch-Ferré et al. Citation2016). Moreover, meta-analysis of nutritional interventions conclude that glutamine supplementation significantly ameliorates elevated fasting plasma glucose and CRP levels (Hasani et al. Citation2021). Glutamine is part of the glutamate-glutamine cycle, and glutamate was reported to be directly related to disruption of glucose metabolism (Tulipani, Palau-Rodriguez, Miñarro Alonso, et al. 2016). Glutamate is produced in the first step of BCAA catabolism (Bhagavan and Ha Citation2011). Different authors have proposed glutamate as a stimulus for glucagon release, increasing transamination of pyruvate to alanine, which strongly promotes gluconeogenesis in obesity (Sookoian and Pirola Citation2012). Glutamate levels varied depending on weight, being higher in young patients with obesity than those without (Htun et al. Citation2021). Furthermore, circulating glutamate was positively related to visceral obesity and development of MetS (Araujo et al. Citation2019; Maltais-Payette et al. Citation2018). It has been proposed that glutamate is elevated in patients with obesity due to an increased need for α-ketoglutarate in the citric acid cycle to compensate for decreased glucose uptake due to insulin resistance (Tulipani, Palau-Rodriguez, Minarro Alonso, et al. Citation2016).
Branched chain amino acids and aromatic amino acids
Leucine, isoleucine, and valine are branched chain amino acids (BCAAs). High levels of circulating BCAA have been associated with increased T2DM risk and insulin resistance, and plasma levels are a predictor of T2DM risk (Bloomgarden Citation2018; Dorcely et al. Citation2017; Gannon, Schnuck, and Vaughan Citation2018; C. Jang et al. Citation2016; Siomkajło and Daroszewski Citation2019). Circulating levels of BCAAs are considered in metabolic assessment, as amino acids participate in the regulation of glucose, lipid, and protein synthesis, among other functions. Particularly, altered levels of BCAAs are associated with disruption of carbohydrate metabolism, informing about poor glycaemic control in healthy adults and in patients with pre-diabetes, T2DM, and gestational diabetes mellitus (‘t Hart et al. 2018; Chen et al. Citation2016; Gogna et al. Citation2015; Jiang et al. Citation2020; Würtz et al. Citation2012). In addition, phenylalanine and tyrosine are aromatic amino acids (AAAs). High levels of these amino acids have been significantly associated with insulin resistance and increased T2DM. Fasting concentrations of AAAs are already elevated very early in the natural history of T2DM (Dorcely et al. Citation2017; Gonzalez-Franquesa et al. Citation2016; Marta Guasch-Ferré et al. Citation2016; Newgard Citation2017)
Uric acid
Uric acid has a key role in glucose metabolism. Not surprisingly, circulating uric acid levels are positively associated with incidence of T2DM (Jia et al. Citation2013; Kodama et al. Citation2009). High levels of this biomarker are independent of other established risk factors, especially MetS components (Barragan et al. Citation2019; Cox et al. Citation2012; Lv et al. Citation2013). Furthermore, uric acid levels are also increased by fructose ingestion, which could be useful as a predictor of further metabolic disorders, since Western diets can be fructose-rich (Johnson et al. Citation2018).
Components of the lipid metabolism signature
Dyslipidemia is one of the metabolic disruptions with high prevalence and is a feature of MetS and a CVD risk factor. Dyslipidemia is currently assessed by plasma lipid profile: total cholesterol (TC), low-density lipoprotein (LDL)-cholesterol, non-HDL-cholesterol, high-density lipoprotein (HDL)-cholesterol, and triglycerides (TGs). Elevated fasting plasma TGs, high LDL-cholesterol and low HDL-cholesterol are risk factors for CVD (Weverling-Rijnsburger et al. Citation2003). In addition, some lipoproteins, such as HDL, have important roles in the acute phase response, due their anti-inflammatory properties reducing cell membrane free cholesterol and lipid raft content, thus attenuating pro-inflammatory signaling pathways (Jahangiri Citation2010). Similarly Lipoprotein A, which can carry oxidized lipids, has been associated with cardiovascular disease and well as inflammation (Blalock et al. 2023). In obesity, enhanced lipolysis in adipose tissue, elevated plasma free fatty acid (FFA) levels and high levels of lipid metabolites in non-adipose tissues act as metabolic mediators of insulin resistance and inflammation, which, in turn induce altered lipoprotein metabolism in the liver (Longo et al. Citation2019); the production of very low-density lipoproteins (VLDLs) is increased and their catabolism diminished, while the catabolism of HDL is increased leading to low HDL-cholesterol levels. Increased accumulation of fat (TGs but also other lipid metabolites) in the liver is associated with increased lipotoxicity and represents the primary liver insult in the pathogenesis of nonalcoholic fatty liver disease (NAFLD) and steatosis (Klop, Elte, and Cabezas Citation2013; Perla et al. Citation2017; Suárez et al. Citation2017).
Fatty acids
Fatty acids (FAs) are a family of molecules with a wide variety of functions and associations with health and disease. Although extensive research is still needed to fully characterize functions and health effects of different FAs, current knowledge allows to design dietary recommendations based on the FA signature, targeting different aspects of health to disease progression. In this sense, polyunsaturated fatty acids (PUFAs) stand out. PUFAs are more beneficial than saturated fatty acids (SFAs), given that their consumption generally promotes antioxidant and anti-inflammatory responses (Djuricic and Calder Citation2021). N-3 PUFAs include alpha linolenic acid, eicosapentaenoic acid (EPA), docosahexaenoic acid (DHA) and their derivatives, while n-6 PUFAs include linoleic acid (LA), arachidonic acid (AA), and their derivatives. Dietary PUFAs have beneficial effects on human health. For example, n-3 PUFAs consumption have been shown to be inversely correlated with coronary heart disease incidence (Philip C Calder Citation2017; Hammad, Pu, and Jones Citation2016; Zock et al. Citation2016). DHA is a n-3 PUFA found in oily fish and fish oil supplements. It is capable of partly inhibiting many aspects of inflammation, including leucocyte chemotaxis, adhesion molecule expression and leucocyte–endothelial adhesive interactions, production of eicosanoids like prostaglandins and leukotrienes from the n-6 fatty acid AA, as well as production of pro-inflammatory cytokines (Philip C Calder Citation2017). In contrast to the overall beneficial effects of PUFAs, increased SFAs are detected in the circulation of patients with diabetes, compared to non-diabetic controls, underpinning the close relationship between lipid and carbohydrate metabolism (Gogna et al. Citation2015; Pasanta et al. Citation2019). In agreement with a large body of evidence, the American Heart Association claimed in 2017 that current evidence supports that replacement of saturated fats by polyunsaturated vegetable oils reduces CVD incidence by a 30% and that replacement by polyunsaturated and monounsaturated fats decreases all-cause mortality while replacement by carbohydrates, mostly refined, does not have an impact (Sacks et al. Citation2017).
As for all fatty acids, monounsaturated fatty acids (MUFAs) are used as substrates for the synthesis of TGs, cholesteryl esters and membrane phospholipids. The saturated to monounsaturated fatty acid ratio affects membrane phospholipid composition and thereby its fluidity, and alterations in this ratio have been implicated in a variety of disease states including CVD, obesity, and T2DM (Miyazaki and Ntambi Citation2003). Numerous beneficial physiological effects have been attributed to MUFAs, including protection from obesity, T2DM, cancer, and atherosclerosis (Hammad, Pu, and Jones Citation2016; Zock et al. Citation2016). Oleic acid is the predominant dietary MUFA, accounting for up to 92% of all dietary MUFAs. Dietary MUFA consumption has been suggested to result in a 20% reduction in the risk of cardiovascular events, as evidenced by a large body of prospective cohort studies (Hammad, Pu, and Jones Citation2016).
In addition to unsaturation level, FA chain length is also an important functional characteristic of FAs. Oleic acid with an aliphatic tail of 18 carbons belongs to the long chain fatty acids (LCFAs; C13-C21) as does EPA (C20), while DHA (C22) is categorized as a very long chain fatty acid (VLCFA; C22 or more). VLCFAs have important structural and functional roles, especially as components of membrane lipids (Kihara Citation2012). High levels of saturated VLCFAs in enterocytes have been associated with atherogenic lipid profiles in MetS (Matsumori et al. Citation2013). On the other hand, high circulating VLCFA levels are especially markers for peroxisomal disorders (Stradomska et al. Citation2020), since VLCFAs require peroxisomal degradation to LCFAs before they can be oxidized in mitochondria. Medium chain fatty acids (MCFAs; C6-C12) modulate mitochondrial function and are thought to improve insulin resistance (Augustin et al. Citation2018). Of note, high levels of circulating MCFAs, especially C9, also have been associated with normoalbuminuria in T1DM (Moon et al. Citation2021), making circulating MCFAs relevant targets for further exploration as biomarkers. Dietary short chain fatty acids (SCFAs; C2-C5) are mostly released in the stomach and, via the portal vein, directly reach the liver, with preclinical indications for beneficial effects against fatty liver (Gart et al. Citation2021). SCFAs are also major products of microbial fermentation of fibers in the colon. Indeed, butyrate (C4) is the unique SCFA used as an important energy source for colonocytes supporting intestinal barrier integrity. Butyrate may also induce oxidation of pyruvate (Bekebrede et al. Citation2021), serve signaling functions and modulate metabolic tissue function, potentially improving cardiometabolic health (Canfora, Jocken, and Blaak Citation2015). Butyrate supplementation was shown to improve nonalcoholic steato-hepathosis in a translational model (Gart et al. Citation2021; Mayorga-Ramos et al. Citation2022). However, the use of SCFAs as biomakers is complicated by their high volatility.
Interestingly, when assessed by 1H-NMR, FA signals are influenced by their carbon structure and by the nature of bonds, allowing to differentiate between saturated, mono- and the various polyunsaturated fatty acids. In addition, the 1H-NMR has the potential to detect circulating fatty acids integrated into different structures such as triacylglycerides and lipoproteins (Khakimov et al. Citation2022). Within PUFAs, 1H-NMR allows to differentiate DHA and EPA from other PUFAs. Together with clinical markers of lipid metabolism, blood FA composition stands out as a key element when delivering dietary advice. The interplay between different FA families and variety of effects on health, both positive and negative, opens the door to a wide array of possibilities in PN.
Lysophospholipids and choline
Lysophospholipid metabolism is impaired in obesity, which has been associated with significantly lower concentrations of most species of lysophosphatidylcholine (LPC) (Del Bas et al. Citation2016). LPCs are signaling molecules produced from phospholipid hydrolysis. Circulating LPCs have also been linked to inflammation, although whether they exert pro- or anti-inflammatory actions is still under debate. The signaling action of lysophospholipids is related to inflammation, insulin production and insulin sensitivity through interaction with G protein-coupled receptors. Thereby, lysophospholipids may be key molecules in the onset and progression of obesity related disorders such as nonalcoholic fatty liver disease (NAFLD), steatohepatitis, T2DM and MetS (Morze et al. Citation2022; Surendran et al. Citation2021; Willis et al. Citation2021). Individuals with elevated concentrations of circulating choline have a higher risk for CVD compared to those with low concentrations (Heianza et al. Citation2017). Additionally, an alteration of choline metabolism has been associated with obesity (Palau-Rodriguez et al. Citation2015). Choline is involved in the mobilization of fat from liver, being negatively associated with hepatic fat accumulation. Choline levels were diminished in serum or urine of patients with obesity (Du et al. Citation2017; Htun et al. Citation2021).
Components of oxidative stress signature
Oxidative stress results from an imbalance between oxidant production and antioxidant defenses, which is translated into accumulation of reactive oxygen species (ROS) and related damage, which is thought to play a key role in the development of a range of metabolic disorders such as obesity, insulin resistance, T2DM, hepatic steatosis and CVD (Le Lay et al. Citation2014).
8-iso-PGF2α, 8-OHdG and LDLox
F2-isoprostane 8-iso-prostaglandin F2α (8-iso-PGF2α) is a product of free radical-mediated oxidation of the n-6 PUFA arachidonic acid, and is altered in TD2M, hypercholesterolemia, hypertension and MetS (Il’yasova, Scarbrough, and Spasojevic Citation2012; Milne, Musiek, and Morrow Citation2005). Urinary 8-iso-PGF2α is considered as a gold standard marker of oxidative stress by regulatory authorities such as EFSA, although quantification is suggested under very specific conditions, such as in 24 hour urine and analysis by chromatographic techniques coupled to mass spectrometry (Djuricic and Calder Citation2021). Together with isoprostanes, 8-hydroxy-2′-deoxyguanosine (8-OHdG) is a widely-used biomarker of oxidative stress, providing information about DNA damage. It is altered in TD2M, hypertension and in patients with CVD (Di Minno et al. Citation2016; Il’yasova, Scarbrough, and Spasojevic Citation2012).
Regarding the diagnostic value of oxidative stress biomarkers, 8-iso-PGF2α and 8-OHdG, together with oxidized LDL (LDLox), might be considered as the more robust biomarkers based on the large amount of evidence collected in meta-analyses and clinical studies (Di Minno et al. Citation2016; Kim et al. Citation2012; Kroese and Scheffer Citation2014; van’t Erve 2018). To note, whilst 8-iso-PGF2α captures oxidative events on lipidic molecules, 8-OHdG is a marker for nucleic acid damage and LDLox is related with the oxidation of apolipoproteins embedded in lipoprotein particles. Therefore, these three biomarkers are indicators of complementary processes leading to physiological alterations. This is especially relevant for capturing different aspects of the same core health process as a single composite biomarker, since current knowledge on nutrition does not allow yet to deliver dietary recommendations based on specific oxidation products. Oxidized lipids of red blood cell membranes are regarded as diet amendable markers of oxidative stress (Jauregibeitia et al. Citation2020; Jauregibeitia et al. Citation2021) and individualized dietary targeting strategies have been proposed (Jauregibeitia et al. Citation2020). However, their analysis requires dedicated analysis approaches (Ferreri et al. Citation2016).
Components of gut microbiota metabolic signature
The human gut microbiota is a large and complex community with more than a thousand species that contribute to human metabolism by supplementing enzymes that are not encoded by the human genome (Rowland et al. Citation2018). These enzymatic capacities are critical for metabolism of several dietary components, taking part in the anabolism of essential molecules (i.e. vitamins) and in the catabolism of poorly digestible diet components (i.e. polysaccharides and polyphenols) (Rowland et al. Citation2018). Human gut dysbiosis can alter the metabolite balance that is in part provided by microbial fermentation of dietary components, which may have an important role in the etiology of various metabolic diseases. To date, knowledge on the link between microbiota and metabolic diseases is still scarce, and using gut microbial populations as a marker of cardiometabolic diseases is not yet accepted. In part this may be because the attention has been focused on the health effects of the presence, absence and balance between individual bacterial species. The newest sequencing capabilities allow the characterization of the microbial community as a whole (metagenomics), without culturing, and are thought to provide more solid microbiota-health relationships (MacCaferri, Biagi, and Brigidi Citation2011). Nevertheless, already now some circulating and urinary metabolites associated to key metabolic actions are known to be modulated by both microbiota activity and host endogenous processes. Imbalances in these metabolites can be interpreted as the result of a dual action driven by microbiota and host metabolism. Therefore, interventions addressed to improve microbiota status (i.e. prebiotic and probiotic-based interventions) might have a positive impact in the initial step of the imbalance (Simó and García-Cañas Citation2020). In this scenario, assessment of different metabolites representative of this microbiota-host interaction might provide a surrogate indicator of general microbiota status.
TMAO, TMA, DMA, choline, and betaine
Different meta-analyses conclude that blood trimethylamine-N-oxide (TMAO) is associated with development of different NCDs (Dehghan et al. Citation2020; Ge et al. Citation2020; Guasti et al. Citation2021; D. Li et al. Citation2022; Yao et al. Citation2020; Zeng et al. Citation2021). This evidence is supported by mechanistic studies showing that TMAO has a dual origin in microbiota and host metabolism (Loo et al. Citation2022). The precursor of TMAO, trimethylamine (TMA), is produced from dietary choline, carnitine, betaine, ergothionase and γ-butyrobetaine by enteric bacteria and further oxidized by hepatic flavin containing monooxigenases (FMMO) and commensal bacteria to TMAO (Djuricic and Calder Citation2021). In turn, gut bacteria can utilize TMAO to produce, among other metabolites, TMA and dimethylamine (DMA) (Loo et al. Citation2022) which can be detected by 1H-NMR in human urine (Laíns et al. Citation2019). Despite the metabolic link between these biomarkers, a recent meta-analysis did not support an association of the precursors choline and betaine with CVD (Meyer and Shea Citation2017). Nevertheless, simultaneous assessment of these metabolites by 1H-NMR allows to capture changes in different elements of this complex network of interactions to inform decisions about dietary changes intended to modulate either the microbiota or intake of TMAO precursors, as has been proposed elsewhere (Simó and García-Cañas Citation2020). In any case, it is important to not only measure metabolites, but also determine the dietary habits of the subjects, because some fish and seafood contain high levels of TMAO, and may induce a transient elevation in TMAO levels in some individuals (Wang et al. Citation2022) which might not link to CVD.
Other microbiota-related biomarkers
Evidence supports the role of bacterial lipopolysaccharide (LPS) as a signaling molecule that triggers different systemic inflammatory responses after translocation from gut lumen to the circulation through the intestinal epithelium (Mohammad and Thiemermann Citation2020). Increased LPS translocation has been described in gut diseases, but also as a response of microbiota to certain components of diet, such as saturated fat, in both normal-weight and overweight individuals (Erlanson-Albertsson and Stenkula Citation2021). Since the mechanisms underlying increased microbiota-derived LPS in blood are well known, quantification of LPS or its surrogate LPS-binding protein (LBP) has been extensively used as a marker of microbiota-derived endotoxemia (Marti et al. Citation2021). Nevertheless, the role of endotoxemia as a trigger of metabolic diseases is still under debate, as well as the cause-effect relationship between circulating LPS and disease progression (Mohammad and Thiemermann 2021). In this scenario, using these molecules as biomarkers of health to metabolic disease progression must be taken with caution. Nevertheless, interventions targeting gut health in general, and microbiota stabilization in particular, have been shown to decrease LPS translocation into blood stream (Beam, Clinger, and Hao Citation2021; Mohammad and Thiemermann 2021). Therefore, quantification of circulating LPS or LBP stands out as key biomarker of microbiota performance when designing dietary interventions targeting gut and microbiome health optimization.
SCFAs are major microbiota-derived fermentation metabolites, as indicated above. During the last years, different association and preclinical mechanistic studies have highlighted potential roles of SCFAs in different human metabolic processes ranging from inflammation to modulation of the gut-brain axis. Nevertheless, although associations between SFCAs and obesity have been found in different human studies, conclusive proof of health effects is still lacking. Moreover, the microbiota-dependent origin of these metabolites restricts most current studies to measurements of SCFAs in fecal samples. In fact, acetate, propionate and butyrate absorption has been estimated in 36%, 9%, and 2%, respectively, and they are mainly excreted via the lungs after oxidation whilst urinary concentration represents less than the 0.05% of total absorbed SCFAs (Beam, Clinger, and Hao Citation2021). Thus, although quantification of these metabolites might represent a source of invaluable information about gut microbiota status, blood or urine are not adequate samples.
Low grade inflammation and its signature components
Inflammation presents the two sides of a coin; it is critical in the response against infection and tissue damage, but a chronic high or low-grade inflammatory state is a pathologic characteristic of a wide range of chronic conditions (Minihane et al. Citation2015). The chronic low-grade inflammatory state has been proposed as a risk factor, characteristic of the early stages of development for a number of chronic diseases, such as MetS, NAFLD, T2DM, and CVD (Minihane et al. Citation2015). Although causal links between inflammation and metabolic alterations are supported by a large body of evidence from both preclinical and human studies, the complexity of the immune/inflammatory system together with a wide diversity of environmental and endogenous inflammatory triggers (i.e. diet, gut microbiota, tissue microenvironment among others) represent an obstacle to fully understand the mechanisms underlying the different strong associations found so far (Wu and Ballantyne Citation2020). Nevertheless, different meta-analyses of observational and intervention studies support a key role of diet in modulating inflammatory markers (Aleksandrova, Koelman, and Rodrigues Citation2021; Custodero et al. Citation2018; Motamedi et al. Citation2022; Mukherjee et al. Citation2022), supporting the idea that assessment of inflammation might be useful for designing more personalized dietary interventions.
CCL2 and ICAM1 as biomarkers of tissue-mediated inflammation
Metabolic inflammation can be understood as the overall result of different insults of different origins. Adipose tissue has been proposed as a relevant source of inflammatory molecules. Thus, in obesity, adipose tissue expansion is accompanied by increased release of C-C motif chemokine ligand 2 (CCL2, also known as MCP1). CCL2 is a potent chemoattractant triggering the recruitment of monocytes/macrophages from the blood stream into the tissue (Gschwandtner, Derler, and Midwood Citation2019). Adipose tissue from obese animals or humans is characterized by an increased number of activated macrophages (in a pro-inflammatory state). Once activated, these macrophages secrete cytokines such as tumor necrosis factor alpha (TNF) and interleukin 6 (IL6), which act as signaling molecules inducing different inflammatory and metabolic responses at different levels and in different tissues. Assessment of these mediators, especially CCL2, in blood might provide information about inflammatory status related with adipose tissue function (Gschwandtner, Derler, and Midwood Citation2019).
Intercellular adhesion molecule 1 (ICAM1) is a molecule continuously present in low concentrations in the membranes of leukocytes and endothelial cells. Upon cytokine (i.e. IL1 and TNF) stimulation, the concentrations greatly increase. It is mainly expressed in vascular endothelium, macrophages and lymphocytes, and promotes vascular adhesion and activation of inflammatory cells (Sprague and Khalil Citation2009). Thus, ICAM1 is currently an accepted biomarker of endothelial function. Different studies have shown dose-dependent association of ICAM1 with T2DM (Qiu et al. Citation2019), and different nutritional interventions have demonstrated beneficial effects on circulating ICAM1 levels concomitant with other inflammatory markers (Schwingshackl and Hoffmann Citation2014).
In the view of this evidence, both CCL2 and ICAM1 could be proposed as biomarkers of inflammation. Nevertheless, both are recapitulating inflammatory events impacting different specific tissues and combining both might allow to capture different but complementary deviations.
Biomarkers of systemic inflammation; CRP, interleukins and GlycA and GlycB
Among the different biomarkers of inflammation, CRP (hsCRP if assessed using highly sensitive technologies) stands out as a gold standard with a demonstrated high association with CVD (Ridker et al. Citation2003). Nevertheless, other biomarkers have been proposed for capturing systemic inflammation, such as IL6 and TNF as previously discussed, and interleukin 10 (IL10). IL10 is especially interesting due to its key role in inhibiting proinflammatory responses that might harm tissues during response to pathogens (Ouyang and O’Garra Citation2019). From the metabolic inflammation perspective, IL10 is significantly decreased in obesity, insulin resistance and inflammatory bowel disease (Charles et al. Citation2011; Leon-Cabrera et al. Citation2015; Meng, Liang, and Guo Citation2019), being a relevant candidate to be included as an indicator of inflammatory status in different metabolic conditions due to its master regulatory role. However, it is important to remember that infectious processes or acute injury can increase the levels of inflammation biomarkers many times more than the increases observed due to metabolic derangements, with the CRP response as prime example (Araújo et al. Citation2022; P C Calder et al. Citation2013).
GlycA and Glyc B, referring to glycosylated serum proteins, are novel biomarkers related to systemic inflammation. Contrary to other biomarkers, GlycA and GlycB are not molecules, but signals that can be assessed only by 1H-NMR and correspond to N-acetyl methyl group protons within N-acetyl glucosamine residues (for GlycA) or sialic acid (for GlycB) on the glycan portions of acute-phase proteins such as α1-acid glycoprotein, haptoglobin, α1-antitrypsin and α1-antichymotrypsin (Connelly et al. Citation2017). Therefore, both GlycA and GlycB can be proposed as a composite biomarker. Although blood levels of GlycA are associated to concentrations of inflammatory biomarkers such as IL6, TNF and CRP, among others, it has been proposed as a more sensitive biomarker than CRP when assessing inflammation in the context of metabolic diseases such as CVD or T2DM (Connelly et al. Citation2016; Connelly et al. Citation2017; McGarrah et al. Citation2017).
For optimal performance, combinations of different biomarkers related to systemic inflammation as a composite biomarker have been proposed (Hopkins, Flanders, and Bostick Citation2012; Morrison et al. Citation2016; Nagrani et al. Citation2022). This allows to better capture overall deviations caused by slight alterations in complementary processes. Using this approach with the above proposed biomarkers might provide an accurate overview of the inflammatory state of the individual to better adapt dietary recommendations.
Integrating metabolomics and proteomics biomarkers as signatures of core health processes
The approach to PN presented herein is based on the integration of metabolic biomarkers to obtain information on the state of core health processes to describe the metabolic state of the individual. The results can then be used as inputs for dietary advice. Several approaches can be undertaken for integrating metabolomics, and proteomic markers by means of data analysis technologies and artificial intelligence.
A plausible approach is integrating classical clinical biomarkers with new metabolic biomarkers. In order to incorporate the latest scientific insights and developments, incorporation of biomarkers that have not yet been widely accepted and/or lack established thresholds is a key feature if they may contribute to differentiate between health and disease or altered metabolic states. This implies that an individual is not (and cannot) be classified in absolute or binary terms of presence or absence of a phenotype. Therefore, a characterization of individuals with a continuous scoring system needs to be applied. This might be done by defining the overall distribution of a biomarker in the general population in order to, subsequently, determine whether an individual falls in higher, middle or lower ranges. Since associations between a given biomarker and altered health states are known, such an approach provides initial information of whether that biomarker points to a higher risk of developing a given phenotype. This approach is inherently imprecise and involves the need for defining the values for the biomarker in a wide population representative of as many different health states as possible, which is a significant effort and an expensive undertaking. Nevertheless, nowadays the number of large cohorts in observational studies is steadily growing and currently it is possible to access sufficient data or samples in a limited number of health conditions.
The combination of different biomarkers involves an approach based on algorithms able to calculate quantitative values for the five core health processes. This approach has been proposed before, for assessing systemic inflammation or to develop a continuous scoring system for MetS (Hopkins, Flanders, and Bostick Citation2012; Jang et al. Citation2021; Klisic et al. Citation2021; Morrison et al. Citation2016; Nagrani et al. Citation2022; Soldatovic et al. Citation2016). In our case, core health processes are represented by five clusters of biomarkers that might be combined by five different algorithms. To develop these algorithms, two relevant points should be considered. First, objective variables of biomarkers such as concentration in biofluids can be combined in the algorithm as a sum of z-scores. Second, the importance of each biomarker relative to others in the same cluster should be taken into consideration when designing the algorithm. For example, when considering the carbohydrates core health process, glucose should have a higher weight than BCAAs, since glucose is considered the main biomarker for dysfunctional carbohydrate metabolism. For other biomarkers though, the differences in importance may not be readily evident. The contribution, or weight, of each biomarker to the overall outcome of each core health process signature can be quantified by a data-driven approach. In this approach, different data analysis tools, including artificial intelligence-based tools, can be used to calculate the weight of each biomarker. Thus, different gold standards can be defined for each core health process signature. For example, to analyze the importance of biomarkers when defining the signature of the carbohydrates core health process, the presence of T2DM might be used as the independent variable to be described by all biomarkers in this signature, except glucose. Subsequently, different machine learning methods, including regression (Partial Least-Squares Regression (PLSR) or weighted quantile sum (WQS) and classification algorithms (Support Vector Machine (SVM), Random Forest (RF) or conditional independence maps), can be applied to define the contribution of each biomarker to the classification, providing a quantitative measure of the importance in prediction, which can be further implemented in the final algorithms as the weight. This approach can be used either with different gold-standards or different target phenotypes, such as obesity or MetS, among others. As a result, a value can be defined for the weight of each biomarker depending on the intended target.
Ultimately, every individual can be represented by a metabolic score for each of the five core health processes as illustrated in Equation (1). Again, the scores for each signature does not provide a measure of disease and should not be used for diagnostic or diagnosis purposes. Instead, the scores represent the deviation from the average, or from a healthy state if sufficient data are available. Higher scores indicate a higher deviation from the average. The deviation from the average in the unhealthy direction can then be used to provide dietary advice for health improvement. Nutritional recommendations can be provided accordingly on an individual basis, with each core health process (or the one that deviates most, taking the other processes into account) targeted toward a healthier or healthy state.
Equation (1) metabolic scoring function. Given the set of metabolic clusters c = {Carbohydrate, Lipid, Inflammation, Microbiota, and Oxidative Stress} is the vector of the values of the user’s biomarkers associated to cluster c and
is the vector containing the weights for each of these biomarkers chosen in such a way that
where
is the total number of biomarkers included in cluster c.
An advantage of such a scoring system is that its modularity allows for adaptation of clusters and their interpretation to specific needs. A limitation of the scoring system is that reference values or thresholds to differentiate between health and disease cannot be set. This would require a well characterized representative population of sufficient size, including a sufficient number of healthy individuals and individuals deviating toward disease in the different directions of the core health processes.
Genetic markers to accommodate the susceptibility of the individual
Susceptibility to disease, but as well to nutritional interventions for health improvement, is also dependent on the genetics of the individual. In the previous section, the use of metabolomics and proteomics biomarkers to assess the individual’s core health processes is described. In this section, we add a genetic layer to enhance effectiveness, both to improve the typing of the individual and to improve the potential effectiveness of the resulting dietary advice. PN based on genetic background to modulate the impact of diet is not new and has been employed for decades (Peña-Romero et al. Citation2018). Based on genetic variation, specific recommendations can be defined, which is efficiently used for people suffering from celiac disease, lactose intolerance or phenylketonuria, among a number of well characterized metabolic diseases that have a clear genetic component (Chaudhary et al. Citation2021). Completion of the 1000 Genomes Project has provided a comprehensive catalogue of human genetic variations, as diverse individuals from multiple populations have been analyzed (https://www.internationalgenome.org). Since then, several follow up studies have contributed to further delineation of the role of genetic variations in human metabolism as well as their interaction with nutrients and other external factors that modulate human health. Considering that metabolic diseases have a multifactorial origin and are the result of complex interactions among many genes and external cues, including diet and lifestyle (Chen, Michalak, and Agellon Citation2018), only a holistic approach is able to bring the management of these diseases forward. Genotype constitutes one category of information that can be introduced into personalized dietary advice and it should be integrated with other relevant information such as sex, age, phenotypic variables, health status, and metabolic biomarkers. The integration of genetic information with metabolic biomarkers provides an additional layer of personalization. This section reviews genetic markers that can be incorporated in the individual’s health profile as a susceptibility layer. Methods for integration of genetic information with metabolic biomarkers is discussed in section “Practical implementation of the personalized nutrition framework: the PREVENTOMICS project as a use case.”
Genetic animal models provide the basis to mechanistically explain the role of specific genes in the etiology of chronic diseases (Suleiman, Mohamed, and Bakar Citation2020). Such models help to dissect specific aspects of multifaceted conditions, such as obesity, especially in combination with human nutrigenetic studies. Effectiveness of dietary treatment and metabolic disease prevention strategies focusing on counteracting specific metabolic disturbances, like those associated with low-grade inflammation, oxidative stress and imbalance or disturbances of lipid or carbohydrate metabolism and even the microbiome are likely dependent on interindividual differences in genetic makeup. The degree of response to treatment may be different in individuals as may be their response to diet, food and nutrients. Nutrigenetics is therefore and indispensable element in the implementation of PN as a strategy for disease prevention and to enhance long-lasting and sustainable health (Mullins et al. Citation2020). As mentioned above, evidence-based guidelines are likely most effective when the genetic makeup is included in stratification of individuals, allowing improved prediction of the best diets for each individual. It should be mentioned that scientific advances in the field of nutrition have revealed that epigenetic markers associated with nutrients can modulate gene expression and are involved with health as well as disease (Li et al. Citation2019; Lorenzo et al. Citation2022). However, epigenetic regulation has not been included in this review, because changes may reflect the current metabolic situation, but epigenetic marks can also be generated long before the time of analysis, even in previous generations (Portha et al. Citation2014).
Genetic markers for overarching process-based dietary recommendations
Progress in DNA sequencing and related techniques allowing the simultaneous analyses of large numbers of genetic variants, has permitted the characterization of genetic biomarkers as a tool to assess predisposition to health/metabolic disease (Loos and Yeo Citation2022; Robinette et al. Citation2012). Specifically, genome-wide association studies (GWAS) in large populations have documented the association of phenotypic traits with common genetic variations – generally, single nucleotide polymorphisms (SNPs) (Robinette et al. Citation2012). This, coupled with the increasing level of scientific understanding of gene-nutrient associations and their influence on metabolism, supports the scope of genetic assessment of metabolic health with greater precision and increases the potential for its application in individuals (Floris et al. Citation2020). Currently, specific genetic information can be used to cover three potential domains of application. That is, to assess the influence of the genetic makeup of the individual on its health status; to characterize the impact of individual’s genetics on metabolic biomarkers; and to provide guidelines for a more PN in view of genetically determined dietary requirement and response differences.
Assessment of metabolic health through genetic predisposition scores
GWAS and large association studies have made it possible to identify several loci related to the predisposition to develop metabolic disease associated alterations. This is of particular relevance in complex diseases that may be defined by up to hundreds of polymorphisms, each of them having a small effect. This has led the development of polygenic risk scores, an approach aiming to aggregate the main loci involved in predicting the individual’s phenotype. This is a tool that contributes to stratify the population by aggregating the effects conferred by the relevant variants that may predispose to higher susceptibility of the disease under study, as can be the case for obesity (Loos and Yeo Citation2022). In this context, the analysis of specific genotypes related to obesity allows estimation of an index of predisposition to obesity and to stratify tendency to adiposity in subclasses that could receive more focused and personalized advice (Goodarzi Citation2018; Khera et al. Citation2019; Loos Citation2012).
In this context, the involvement of common variants in FTO (fat mass and obesity-associated gene) is well documented for the influence on obesity risk and related alterations, such as TD2M (Frayling et al. Citation2007; Loos et al. Citation2008; Speliotes et al. Citation2010; Thorleifsson et al. Citation2009; Willer et al. Citation2009). The presence of FTO risk variants has been correlated with increases of 2.5 kg in body fat mass (Pausova et al. Citation2009) and 0.39 kg·m−2 in BMI in Europeans (Speliotes et al. Citation2010). Beyond FTO variants, obesity predisposition also depends on variants located on other genes, but with less impact on BMI. For example, variants in Melanocortin 4 receptor (MC4R) or Brain-derived neurotrophic factor (BDNF) coding genes, have been associated with 0.33 and 0.19 kg·m−2 of BMI increases per risk allele, respectively (Speliotes et al. Citation2010). Consequently, the inclusion in the genetic assessment of these and other main variants associated with a specific trait, obesity in this case, contributes to estimate the genetic component of the individual’s phenotype. Here the application of polygenic risk scores comes into play. Polygenic risk scores integrate combinations of genetic variants that influence specific biological functions or are associated with the same phenotypic trait (Hüls et al. Citation2017). Estimation of the Performance of Polygenic Scores (PPS) has more predictive potential than the interpretation of isolated variants (Abadi et al. Citation2017; Khera et al. Citation2019; Shabana, Shahid, and Hasnain Citation2018; Udler et al. Citation2019), can be very sensitive, and can contribute to define the impact of genetics on metabolic health, even from birth (Khera et al. Citation2019). It should be noted, that only a small number of cases of obesity (5%) result from mutations in specific genes (González Jiménez Citation2011), which should be taken into account to assess the impact of genetic mutations in the development of obesity. The same is likely true of other nutrition and lifestyle associated metabolic diseases.
The genetic variants identified can nevertheless be used for the calculation of PPS to provide information on tendency to cope with metabolic disorders like obesity, visceral obesity, T2DM, dyslipidemia, metabolic inflammation, and hypertension. Accordingly, the estimation of the PPS associated with different metabolic traits allows to identify and inform about the predisposition to metabolic alterations that are most likely to develop in an individual. This type of knowledge opens the road to prevention and will facilitate personalized strategies to improve health (de Toro-Martín et al. Citation2017; Laddu and Hauser Citation2019; Udler et al. Citation2019). PPS are not only relevant in relation to disease risk, but may also contribute to better characterize (and understand) other functions, such as eating behavior. As an example, a genetic variant in the ANKK1 gene may influence the satiating effect of meals, since subjects having the variant are prone to experience hunger less frequently (Lek, Ong, and Say Citation2018). In turn this may affect adherence to a nutritional intervention. Similarly, the presence of a variant in BDNF gene could alter adherence to a diet, as it has been associated with higher tendency to experience pleasure in response to binge-eating (Bumb et al. Citation2021). Understanding the impact of the genetic makeup on the eating behavior of individuals facilitates anticipating to altered eating behaviors and may help to find solutions to deal with it satisfactorily.
Genetic markers for overarching process-based health signature interpretation
GWAS also provides a scientific rationale for the impact of the individual’s genetic makeup on inter-individual differences in levels of metabolic biomarkers in biological fluids (Hysi et al. Citation2022; Shin et al. Citation2014; Yin et al. Citation2022). This has been well documented for plasma lipids, which in addition to being influenced by dietary intake, also show a relevant heritable component (CitationTabassum et al. 2019). Therefore, the incorporation and analysis of targeted genetic information related to specific metabolic biomarkers may provide greater sensitivity to the metabolite-based clustering of participants. To some extent, evidence allows an estimate of the variability in levels of biomarkers that would be explained by the presence of genetic variants. This approach allows less focus on metabolites whose (altered) levels may be dependent, to some extent, on genetics and, consequently may be more difficult to improve by dietary strategies. A brief cluster-by-cluster explanation about how genetics can contribute to the individualized characterization of core health signatures is outlined below.
Lipid metabolism core health signature
As mentioned above (see section “Components of the lipid metabolism signature”), biomarkers related to lipid metabolism provide a signature of health status. Current scientific evidence supports that at least 14 of these parameters can be modulated by genetics and part of the variability in their concentration can be explained by genetics (Supplementary Table 2). Therefore, 18 genetic variants, generally located in lipoprotein coding and/or key lipid metabolism genes, are genetic influences to be taken into account in dietary health improvement strategies.
In this context, polymorphism rs174547, located on the FADS1 gene, encoding the Fatty Acid Desaturase 1 enzyme, which catalyzes the final step of EPA and AA production, may play a major role in blood lipid modulation (Wang et al. Citation2021), influencing the concentration of, at least, five traits: TGs (Kathiresan et al. Citation2009), TC (Kulminski et al. Citation2018), PUFA (Kettunen et al. Citation2016), LPCs (Draisma et al. Citation2015) and LA (Dorajoo et al. Citation2015). In addition, rs7412 (a Arg176Cys exchange variant of Apolipoprotein E (APOE) impacting on HDL, TC, and LDL levels (Hoffmann et al. Citation2018) and rs12272004 (on the Apolipoprotein A-V gene (APOA5) influencing TG, TC and LDL levels (Aulchenko et al. Citation2009) have a role in determining the blood lipid profile with a potential modulation of at least three phenotypic traits per variant. Moreover, rs326 (on Lipoprotein lipase (LPL), influencing HDL and TG levels (Hoffmann et al. Citation2018)), rs12678919 (on Tissue inhibitor of metalloproteinase 3 (TIMP3), influencing HDL and TG levels (Hoffmann et al. Citation2018), rs780093 (on Glucokinase regulatory protein (GCKR), associated with leptin (Kilpeläinen et al. Citation2016) and SFA (Wu et al. Citation2013) plasma levels), rs1800562 (on homeostatic iron regulator (HFE), influencing TC and LDL (Hoffmann et al. Citation2018) and rs409224 (on Cut-like homeobox 1 (CUX1), associated with plasma PUFA, specifically DHA levels (Dorajoo et al. Citation2015) are examples of genetic variants associated with different levels of parameters linked with lipid metabolism. Besides, other additional SNPs have been linked to specific lipid biomarkers: rs3811444 (Tripartite motif-containing 58; TRIM58), rs182052 (Adiponectin, C1Q and collagen domain containing; ADIPOQ), rs8396 (peptidylprolyl isomerase D (cyclophilin D); PPID), rs1171614 (solute carrier family 16 (monocarboxylic acid transporters), member 9; SLC16A9) affect levels of oleic acid (Tintle et al. Citation2015), adiponectin (Dastani et al. Citation2012) and MUFAs (Illig et al. Citation2010) and the acylcarnitine profile (Draisma et al. Citation2015), respectively. Therefore, consideration of the role of these variants may contribute to provide deeper assessment on the impact of genetics on lipid metabolism.
Carbohydrate metabolism core health signature
Following the same procedure, a set of SNPs previously associated with plasma/serum biomarkers of carbohydrate metabolism have been selected. Two genetic polymorphisms (rs1260326 and rs7903146) are especially relevant because of the remarkable number of metabolites that each influence (Supplementary Table 2). The first polymorphism encodes a missense variant in the Glucokinase regulatory protein (GCKR) and this is associated with circulating levels of glucose (Shin et al. Citation2014), lactate (Tin et al. Citation2016) and BCAAs (valine, leucine and isoleucine) (Lotta et al. Citation2016). The second is a common variant in the Transcription factor 7 like 2 (TCF7L2) gene that has been associated with risk for alterations in carbohydrate metabolism, and also affects glucose and insulin levels (Manning et al. Citation2012).
Other genetic markers are added to refine the impact of genetics on the carbohydrate cluster. SNPs in the Fatty acid desaturase 1 (FADS1) gene (Wessel et al. Citation2015) and in the SLC2A2 gene, encoding for the glucose transporter GLUT2 (Nagy et al. Citation2017), may contribute to explain part of the variability found in glucose levels. Furthermore, plasma leptin is potentially modulated by rs780093 (GCKR) and rs10487505 (leptin; LEP) (Kilpeläinen et al. Citation2016); insulin by rs1801282 (Peroxisome proliferator activated receptor gamma; PPARG) (Mahajan et al. Citation2015); while glutamine, phenylalanine and tyrosine are influenced by rs2657879 (near the Glutaminase 2 (GLS2) and the SPRY domain containing 4 (SPRYD4) genes) (Shin et al. Citation2014), rs17450122 (Achaete-scute family bHLH transcription factor 1; ASCL1) (Imaizumi et al. Citation2019) and rs14399 (SLC16A10) (Kettunen et al. Citation2016) variants, respectively. The consideration of a PPS taking the potential action of these genetic variants into account constitutes the core of the assessment of the influence of genetics on the levels of biomarkers analyzed in relation to the carbohydrate health signature.
Oxidative stress core health signature
Concerning genetic factors modulating oxidative stress, up-to-date evidence has associated specific genetic variants with the buffering capacity of oxidative species. Analysis of rs4680 (Catechol O-methyltransferase; COMT), rs1695 (Glutathione S-Transferase Pi 1; GSTP1), rs1801133 (methylenetetrahydrofolate reductase; MTHFR), rs838133 (fibroblast growth factor 21; FGF21), rs4880 (Superoxide dismutase 2; SOD2) and rs715 (carbamoyl-phosphate synthetase 1; CPS1) can been used to estimate the impact of genetics on the oxidative stress cluster.
The minor rs4680 allele, a variant located on the COMT gene, has been related with lower enzyme activity (Shin et al. Citation2014), lower production of endogenous antioxidants (Hysi et al. Citation2019) and, probably as a consequence, increased susceptibility to coronary artery disease (Mir et al. Citation2018). GSTP1 plays a fundamental role in xenobiotic metabolism detoxification by catalyzing the conjugation of several compounds with glutathione, although its increased expression has been associated with increased oxidative stress (Li et al. Citation2013). In this context, rs1695 (on GSTP1) is associated with higher expression of this gene, which could contribute to a more oxidative status (Sun et al. Citation2018). Similarly, the minor variant of rs838133 of the FGF21 gene is associated with increased plasma homocysteine and coronary artery disease risk (van Meurs et al. Citation2013), consequently, it may also be considered as a marker of oxidative status. The rs1801133 variant, which comprises an Ala222Val substitution in the MTHFR gene, involved in folate metabolism, is associated with lower efficiency in folic acid processing, contributing to increased homocysteine and, lower vitamin B12 and folate plasma concentrations (Boccia et al. Citation2009; van Meurs et al. Citation2013). Consequently, that associated-phenotype could lead to greater susceptibility to oxidative profile-based diseases (da Silva et al. Citation2017). Furthermore, the rs4880 variant in the SOD2 gene has been mechanistically associated with lower capacity to buffer superoxide radicals generated by mitochondrial activity (Sutton et al. Citation2005). This SNP and T allele presence is associated with a reduced cellular free radical scavenging efficiency (Sutton et al. Citation2005), in addition to higher levels of protein and lipid oxidative by-products (Jerotic et al. Citation2019). Finally, GWAS evidence shows the influence of rs715 on the CPS1 gene on blood betaine concentrations (Hartiala et al. Citation2016). Bearing in mind that elevated betaine levels are considered a biomarker of oxidative signatures (see section “Components of oxidative stress signature”), the rs715 genotype could also be considered a relevant genetic marker for predisposition to a pro-oxidative state. The aforementioned genetic markers may support the assessment of genetics impact on the inter-individual capacity to buffer oxidative stress and a PPS can be defined to evaluate the individual’s plasma oxidative profile.
Inflammatory core health signature
Current scientific evidence supports the genetic influence of at least nine biomarkers related with the core inflammatory health signature. At least five parameters included in the lipid cluster are also commonly known for their relationship with an inflammatory profile (SFAs, PUFAs, DHA, LPCs, and LA). Particularly, the polymorphism rs174547 (FADS1) which is included in the lipid metabolism cluster, would also have a considerable impact on the inflammatory status associated with its relevant influence on lipid profile.
Beyond these lipid cluster genes, the inflammatory signature is defined by biomarkers of systemic inflammation and the activity of components of the immune system. rs429358 (on APOE) and rs1260326 (GCKR) have been described to modulate CRP levels (Ligthart et al. Citation2018). Similarly, rs1800795, a genetic variant in the interleukin 6 (IL6) gene promoter, influences IL6 levels (Ljungman et al. Citation2009). Likewise, rs12075 (near the cell adhesion molecule 3 (CADM3) and arylsulfatase B (ARSB) genes) influences CCL2 (MCP1) levels (Comuzzie et al. Citation2012) and rs5498 (ICAM1) has been associated with interindividual variability in the plasma concentrations of ICAM-1 (Sun et al. Citation2018). Together these genetic variants can be considered to constitute a PPS aiming to estimate the genetic predisposition to modify plasma inflammatory biomarkers.
Microbiome core health signature
The microbiome is evidently influenced by diet, but long-term the genetic make-up of the host is also a key determinant in its microbiome composition (Dąbrowska and Witkiewicz Citation2016) and relates to, for example, cardiovascular health (L. Chen et al. Citation2021), Evidently, in the near future distinct genetic determinants will be elucidated that will allow incorporation of the individual’s genetic predisposition affecting its microbiome composition in relation to its influences on the core health processes and the response to diet.
Practical implementation of the personalized nutrition framework: The PREVENTOMICS project as a use case
In previous sections, a conceptual framework based on the analysis of metabolic signatures associated to core health processes has been discussed, with an extensive list of metabolomic and proteomic biomarkers with sufficient scientific evidence for a disease relationship that can be robustly and affordably analyzed. Next an approach for integration of such biomarkers is proposed as a description of genetic biomarkers to be added as a layer of disease and intervention susceptibility. To make the hurdle toward practical implementation tangible, we now describe, as one exemplifying translation option, how this approach is implemented in the EU-funded project ‘Empowering consumers to PREVENT diet-related diseases through OMICS sciences’ (PREVENTOMICS) (https://preventomics.eu). This project was conceived as a proof of concept from selecting biomarkers, as described above, to tangible tools for integration, taking consumption biomarkers and gut microbiome dynamics into account, and for empowerment of the consumer. The latter is envisioned as an app that provides dietary, shopping or cooking advise, guided by the individual’s aggregated biomarker profile and genetic susceptibility. PREVENTOMICS thus aims to develop an omics-based PN system and tools that shapes the food environment of the person toward empowering consumers and nutrition professionals in decision-making processes related with dietary habits and nutrition. The project follows the approach described in the previous sections, and therefore is based in the functional categorization in core health processes of reliable metabolic and genetic biomarkers that can be further integrated to obtain information about the metabolic status of the individual. By analysis of metabolic and genetic markers representative of overarching processes, individuals were characterized and the results were used as inputs for dietary advice. The samples were collected locally in different European countries and analyzed centrally using ‘omics technologies. The above-described biomarker levels were fed into a tangible tool to provide personalized dietary recommendations based on the signature of core health processes that are represented by the biomarkers.
Other layers of personalization were added, such as adherence to Mediterranean Diet, dietary preferences and restrictions and personality traits to adapt dietary recommendations. A personalized behavior change program was incorporated to aid the user in the process of changing their dietary habits. The tool developed during the PREVENTOMICS project was deployed in different business models focused on end-users, such as recommendations during shopping in a supermarket e-commerce portal and suggestions when ordering foods for delivery or adaptation of delivered meals to the individual’s recommendations, and, last but not least, it will provide nutrition professionals with key information in their own language for delivering appropriate dietary recommendations to consumers.
Integration of omics and genetic markers in PREVENTOMICS
In PREVENTOMICS, the approach discussed in section “Integrating metabolomics and proteomics biomarkers as signatures of core health processes” has been translated into a tangible analytical platform. Three hundred twenty nine biobank samples from Spain and Denmark were used to initially set up the personalized nutrition tool. Then 275 samples from healthy individuals from the Maastricht Study (Schram et al. Citation2014), MCAGE (Lagerwaard et al. Citation2020) (age) and BMCORE (Janssen et al. Citation2021; Janssen et al. Citation2023) (high and low physical active) were added to provide a wider range of healthy individuals. In addition, four intervention studies in four different European countries were performed, with a total number of 764 individuals.
Once the metabolomics and proteomics have been integrated to obtain the score for each core health process, the next step is to integrate the information provided by the genetic background information with the metabolic information. The need for this is particularly exemplified when one considers nutrient requirements. These have been established to cover daily needs of a reference population, but these may not fit the requirements of specific individuals. Therefore, dietary recommendations adapted to individual backgrounds have been proposed in order to promote health in a more accurate way. For example, the modulating influence of the genetic load is taken into account in the characterization of the anti-inflammatory potential of omega-3 fatty acids, and how the bioavailability of vitamins, minerals and ergogenic aids is configured by the presence of genetic variants (De Caterina and El-Sohemy Citation2016; Galmés et al. Citation2019; Galmés, Palou, and Serra Citation2020; Galmés, Serra, and Palou Citation2018; Gkouskou et al. Citation2021; Niforou, Konstantinidou, and Naska Citation2020; Schwartz Citation2014; Southward et al. Citation2018). Following a similar approach, taking the modifying effects of genetic variation on metabolic disease susceptibility, biomarker behavior and response to the diet into account, the PREVENTOMICS project has incorporated current knowledge on nutrigenetics, i.e. incorporated genetic variation that likely impacts the health cluster signatures, to give comprehensive nutrition and lifestyle advice to participants.
Based on genetics a scoring system was designed to define the individual’s specific nutritional needs. Then, lifestyle messages were prepared to give indications on how to fulfill these needs. To avoid overwhelming the participants, the output to the individuals was implemented in the form of tips, short sentences focused on preventing food-related diseases in a personalized approach or even with a pictorial code to get the focus on what is theoretically the best practice. Examples of nutrigenetic information provided to PREVENTOMICS participants, so that they could benefit more from specific guidelines, are as follows: type of diet that would be more effective in minimizing the predisposition to metabolic alterations (Galmés et al. Citation2019) or to promote more weight reduction in case of obesity (Ramos-Lopez, Milton-Laskibar, and Martínez Citation2021); susceptibility to poor satiety control (Panduro et al. Citation2017); genotype-recommend bioactive nutrients with greater expected potential of enhancing health; genetic predisposition to gluten problems (van Heel et al. Citation2007) or lactose intolerance (Lukito et al. Citation2015), low sensitivity to oral fat perception or food preferences (Keller Citation2012); genetic influences on metabolism, transport or handling of micronutrients that could support immune health (Galmés, Serra, and Palou Citation2020).
The genetics were also incorporated in the overall dietary advice resulting from the PREVENTOMICS tool. The individual’s score obtained by the equation depicted in Equation (1) provides a continuous read of the information from metabolic biomarkers, both metabolomic and proteomics. As has been explained above, at the individual level, metabolic performance is highly influenced by genetics. In the PREVENTOMICS project, the influence of genetics on each cluster of biomarkers has been considered as a factor that might modify the metabolic score depending on the specific genetic background of the user. Thus, a genetic score has been developed and integrated as depicted in Equation (2).
Equation (2). Cluster scoring function where the metabolic score defined in Equation (1) is adjusted by the genetic risk function, obtaining the final score for each cluster c.
Finally, in PREVENTOMICS, it was chosen to target the most deviating overarching process, that is, the cluster obtaining the higher cluster score (Equation (3)). Hence, in the intervention studies individuals were allocated to different dietary plans accordingly.
Equation (3). Altered cluster function. c refers to one single cluster, while C comprises all 5 clusters.
Application of personalization based in overarching processes into tangible tools
In the PREVENTOMICS project, the approach presented in this critical review was implemented as a personalized nutrition tool, interoperable with different frontends representative of important elements of the food environment of consumers, such a lifestyle, shopping, dietary and meal advice as well as recipes. To this end, the information provided by omics and genetic biomarkers, together with behavioral, dietary and phenotypic information, was used to feed a recommender system (). The resulting personalized recommendations were stored in a decision support system that can be accessed by different digital applications (), such as a software for nutrition professionals, an application simulating an e-commerce portal and, implemented in the workflow of a catering company (Aldubayan et al. Citation2022). All these implementations have been successfully developed and were further tested by means of nutritional interventions during the project. Although most data are still being analyzed, proof of concept is evidenced by the publication of the results of one study (Aldubayan et al. Citation2022). The precedent of PREVENTOMICS as a proof of concept project indicates that implementing personalization at a population-wide level is in principle feasible. Nutrition interventions are required to assess the effectiveness of such systems.
Figure 1. Alterations of overarching processes that sustain health can be considered as a key feature of the onset of non-communicable, multifactorial diseases (Van Ommen Citation2009). Core health processes, that is, carbohydrate metabolism, lipid metabolism, inflammation, oxidative stress and microbiota status, are proposed as measurable elements of these overarching processes. The status of core health processes can be assessed by omics profiling, and further characterized by integrating genetic markers that are informative of complementary aspects. Information provided by assessment of core health processes status can be further used to deliver nutritional recommendations based in dietary patterns with sufficient scientific basis in order to modulate behavior and dietary habits affecting overarching processes.
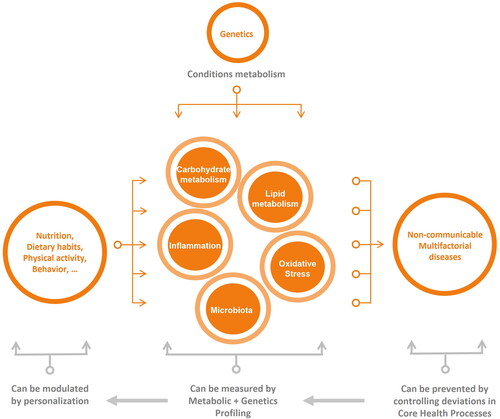
Figure 2. The approach proposed in this review was implemented in a tangible personalized nutrition system in the project PREVENTOMICS. (A) The system combined metabolomic, proteomic and genetic data together with results of data analysis for scoring the five core health processes. (B) The information on core health processes scores was further combined with behavioral information within a decision support system. (C) The decision support system was accessed by different softwares that could retrieve specific information about the user to elaborate personalized recommendations.
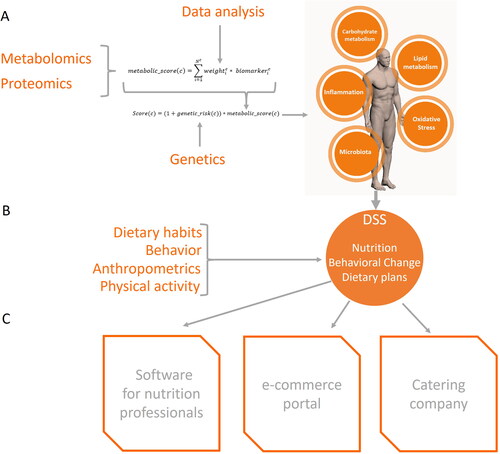
Other omics with potential applications
The present work reviews metabolomics, proteomics and genetic markers with application in feasible PN systems that are compliant with the current constrains. This is, well characterized, science substantiated omics and genetic markers that can be robustly analyzed at a feasible cost in terms of benefit-cost ratio. Other fields of research in biomedical and nutrition research to be incorporated in PN applications in the near future include food intake biomarkers and gut microbiome markers. Although the knowledge in these fields is rapidly expanding, its application in affordable PN systems still needs further development. Below we provide the current perspective on these fields in the context of PN, with an eye on the core health processes.
Food intake biomarkers to improve personalization
Dietary habits are among the most relevant factors determining alterations in individuals’ metabolic status (Murray et al. Citation2020). In addition to metabolic and genetic markers, food consumption data can provide information on individuals’ dietary patterns allowing to investigate the association between nutrition and health status (González-Domínguez, Jáuregui, et al. Citation2020). The current assessment of dietary intake, that relies on food frequency questionnaires, dietary recalls, or food diaries, is almost invariably affected by misreporting and bias, due to the subjective nature of these methods. Moreover, these instruments are intrinsically based on food composition tables that can lead to errors in nutrient estimation (Garcia-Aloy et al. Citation2017). Recently, research has moved toward establishing more objective measures of dietary intake, by identification and validation of biomarkers of food intake in biological samples (Dragsted et al. Citation2018; Maruvada et al. Citation2020; Ulaszewska et al. Citation2018) aiming to overcome the limitations associated with traditional dietary assessment approaches (Garcia-Aloy et al. Citation2017).
Food intake biomarkers are compounds derived from the metabolism of constituents of specific foods or food groups that can be found in especially biological fluids, like plasma or urine, and are reflective of the intake of their precursors present in the food matrix (Garcia-Aloy et al. Citation2017). A good biomarker of food intake must be specific for a certain foodstuff/food group, and its level in plasma or urine must increase as a consequence of the increased consumption of the foodstuff/food group (D’Angelo et al. Citation2019). Already, several comprehensive “panels” of biomarkers able to reflect a person’s diet more globally have been proposed, potentially allowing the assessment of dietary patterns (Acar et al. Citation2019; Playdon et al. Citation2017). As there are hundreds of metabolites from the diet, metabolomics represents the best modern approach to measure the majority of them (González-Domínguez, Urpi-Sarda, et al. Citation2020; González-Domínguez, Jáuregui, et al. Citation2020). Nevertheless, the number of fully validated biomarkers is so far quite low and still inadequate to properly characterize dietary patterns. However, already in the near future they could be taken into consideration together with metabolic and genetic markers to more fully characterize individuals and their metabolic status. Within the PREVENTOMICS project, food intake biomarkers determined by means of targeted LC/MS-MS, a technique more qualitative than quantitative, because it identifies specific fragments of a molecule rather than measuring their abundance (Bantscheff et al. Citation2012; Olsen and Mann Citation2004), will be evaluated, together with traditional methods, to assess whether effective shifts have occurred in the dietary habits of the study participants. This will also help to improve biomarker validity and to establish accurate associations between food intake and the main cardiometabolic outcomes considered in PREVENTOMICS.
Gut microbiota and personalization
The human gut microbiota is responsive to many different environmental factors. Although the gut microbiome composition is subject to the individual’s genetic makeup, diet is considered the main driver able to modify the composition and function of the gut microbiome (Donovan Citation2017; Fernández-Calleja et al. Citation2018; Gentile and Weir Citation2018). For this reason, personalized diets have the potential to modify the composition of the host microbiota on an individual basis. However, the role of personalized diets and the impact on the microbiome, and the subsequent relation to health promotion, has not been studied in depth.
The main challenge to exploiting the potential of microbiome-based PN is to identify how the host, microbiota and dietary exposures interact in shaping dietary responses. Recently, the role of the gut microbiota in the response to personalized interventions has been highlighted in different studies. For example, the glycemic response based on the intake of different types of bread has been predicted based on the microbiota composition (Korem et al. Citation2017). Also, interindividual microbiome responses to obesity-related dietary interventions have been found to be associated with specific bacterial species based on the initial composition of the microbiome in both men and women (Korpela et al. Citation2014). Similarly, another study has successfully predicted host response to a diet for obese patients based on the initial composition of their microbiota, with especially Bacteroidaceae bacteroides as a good predictor (Zhang et al. Citation2021). These and other data are part of a growing body of evidence suggesting that dietary response patterns may be driven, to some extent, by individualized microbial compositions. However, microbial metabolic activity is a complex process that involves a network of metabolic pathways and interactions between different microbial species in a community (Lam and Ye Citation2022). This is likely not well reflected by quantifying individual species and it is increasingly realized that functional role of the microbiota-as-a-whole may have to be considered. Meta-transcriptomic approaches can examine the transcripts expressed by the entire microbiota community (Qin et al. Citation2012), and may thus provide a more comprehensive view of the metabolic activities and alterations therein of the microbiota. During the PREVENTOMICS project, the microbiota from the participants in the personalized dietary interventions have been sampled and are being characterized. The gut microbiota characterization will allow to better understand the association between microbiota, metabolites, genetics, food markers and, importantly, the personalized interventions.
Concluding remarks
The combined application of metabolomics, proteomics and genetics, together with anthropometry and clinical biomarkers, will provide signatures for core health processes; the harmonized integration of metabolic signatures together with genetic susceptibility thus results in a score for each of these processes. Together with food intake data and other information such as habits, mainly dietary habits and physical activity, personality traits, preferences, food allergies or intolerances, among other variables, will provide an aggregated nutritional phenotype. Likely, gadgets to monitor the individual’s behavior and health related parameters will provide relevant additional information to be incorporated in the individual’s nutritional phenotype in the near future. Furthermore, ethnicity-related differences in metabolomic and genetic risk markers may be included. These and other developments, imply a modular approach for any PN system to be successful on the longer term. A PN system should also contemplate psychology, since behavioral change is critical for adherence to recommendations (Broers et al. Citation2020). Therefore, a successful PN system needs to consider the different dimensions of the individual relation with food.
In the critical review, we have proposed a framework that builds on sets of science-based metabolic and proteomics biomarkers, that with different weights, together provide a signature for each of the core health processes. Genetic information is integrated reflecting the individual’s susceptibility to health impairment and diet. The combined signatures of integrated metabolic and genetic information are open to adaption and thus flexible, and the use of information technology tools, such as AI, facilitate optimization. Also new sets of biomarkers may be added, reflecting, for example, microbiota profiling or food consumption. The combined signatures reflect overarching processes and together with phenotypic, lifestyle, dietary and behavioral information provide a nutritional phenotype and facilitate individual personal advice. End users can be provided with personal advice, in which behavior traits and psychological factors are integrated. The PREVENTOMICS project as a use case shows that the implementation of the framework is feasible in practice. Our hope is that, after future optimization, this framework, in the form used in PREVENTOMICS or in another, will empower actors in the food system, but especially consumers to make their own science based PN choices to improve their health.
Disclosure agreement
Patent application “Method for the selection of diet for a subject”. Application number EP22382965.6.
Supplemental Material
Download PDF (411 KB)Additional information
Funding
References
- Abadi, A., A. Alyass, S. Robiou Du Pont, B. Bolker, P. Singh, V. Mohan, R. Diaz, J. C. Engert, S. Yusuf, H. C. Gerstein, et al. 2017. Penetrance of polygenic obesity susceptibility loci across the body mass index distribution. American Journal of Human Genetics 101 (6):925–38. doi: 10.1016/j.ajhg.2017.10.007.
- Abdul Ghani, Z. D. F., A. H. Ab Rashid, K. Shaari, and Z. Chik. 2019. Urine NMR metabolomic study on biochemical activities to investigate the effect of P. betle extract on obese rats. Applied Biochemistry and Biotechnology 189 (2):690–708. doi: 10.1007/s12010-019-03042-w.
- Abu Bakar, M. H., M. R. Sarmidi, K. K. Cheng, A. Ali Khan, C. Lee Suan, H. Zaman Huri, and H. Yaakob. 2015. Metabolomics – The complementary field in systems biology: A review on obesity and type 2 diabetes. Molecular bioSystems 11 (7):1742–74. doi: 10.1039/c5mb00158g.
- Acar, E., G. Gürdeniz, B. Khakimov, F. Savorani, S. K. Korndal, T. M. Larsen, S. B. Engelsen, A. Astrup, and L. O. Dragsted. 2019. Biomarkers of individual foods, and separation of diets using untargeted LC-MS-based plasma metabolomics in a randomized controlled trial. Molecular Nutrition & Food Research 63 (1):e1800215. doi: 10.1002/mnfr.201800215.
- Afshin, A., P. J. Sur, K. A. Fay, L. Cornaby, G. Ferrara, J. S. Salama, E. C. Mullany, K. Hassen Abate, C. Abbafati, Z. Abebe, et al. 2019. Health effects of dietary risks in 195 countries, 1990–2017: A systematic analysis for the global burden of disease study 2017. The Lancet 393 (10184):1958–72. Elsevier:1958–1972. doi: 10.1016/S0140-6736(19)30041-8.
- Aldubayan, M. A., K. Pigsborg, S. M. Gormsen, F. Serra, M. Palou, P. Mena, M. Wetzels, A. Calleja, A. Caimari, J. Del Bas, et al. 2022. Empowering consumers to PREVENT Diet-related diseases through OMICS sciences (PREVENTOMICS): Protocol for a parallel double-blinded randomised intervention trial to investigate biomarker-based nutrition plans for weight loss. BMJ Open 12 (3):e051285. doi: 10.1136/BMJOPEN-2021-051285.
- Aleksandrova, K., L. Koelman, and C. E. Rodrigues. 2021. Dietary patterns and biomarkers of oxidative stress and inflammation: A systematic review of observational and intervention studies. Redox Biology 42 (June):101869. doi: 10.1016/j.redox.2021.101869.
- Araújo, R., L. F. N. Bento, T. A. H. Fonseca, C. P. Von Rekowski, B. R. da Cunha, and C. R. C. Calado. 2022. Infection biomarkers based on metabolomics. Metabolites 12 (2):92. doi: 10.3390/metabo12020092.
- Araujo, T. R., J. A. da Silva, J. F. Vettorazzi, I. N. Freitas, C. Lubaczeuski, E. A. Magalhaes, J. N. Silva, E. S. Ribeiro, A. C. Boschero, E. M. Carneiro, et al. 2019. Glucose intolerance in monosodium glutamate obesity is linked to hyperglucagonemia and insulin resistance in alpha cells. Journal of Cellular Physiology 234 (5):7019–31. doi: 10.1002/jcp.27455.
- Augustin, K., A. Khabbush, S. Williams, S. Eaton, M. Orford, J. H. Cross, S. J. Heales, M. C. Walker, and R. S. Williams. 2018. Mechanisms of action for the medium-chain triglyceride ketogenic diet in neurological and metabolic disorders. The Lancet Neurology 17 (1):84–93. doi: 10.1016/S1474-4422(17)30408-8.
- Aulchenko, Y. S., S. Ripatti, I. Lindqvist, D. Boomsma, I. M. Heid, P. P. Pramstaller, B. W. J. H. Penninx, A. C. J. W. Janssens, J. F. Wilson, T. Spector, et al. 2009. Loci influencing lipid levels and coronary heart disease risk in 16 European Population Cohorts. Nature Genetics 41 (1):47–55. doi: 10.1038/ng.269.
- Bantscheff, M., S. Lemeer, M. M. Savitski, and B. Kuster. 2012. Quantitative mass spectrometry in proteomics: Critical review update from 2007 to the present. Analytical and Bioanalytical Chemistry 404 (4):939–65. doi: 10.1007/s00216-012-6203-4.
- Barabási, A.-L., G. Menichetti, and J. Loscalzo. 2019. The unmapped chemical complexity of our diet. Nature Food 1 (1):33–7. doi: 10.1038/s43016-019-0005-1.
- Barragan, M., V. Luna, J. M. Vargas-Morales, C. Aradillas-Garcia, and M. Teran-Garcia. 2019. Uric acid: An overlooked, inexpensive biomarker of metabolic syndrome (P10-068-19). Current Developments in Nutrition 3 (Supplement_1):nzz034.P10-068-19. doi: 10.1093/cdn/nzz034.P10-068-19.
- Beam, A., E. Clinger, and L. Hao. 2021. Effect of diet and dietary components on the composition of the gut microbiota. Nutrients 13 (8):2795. doi: 10.3390/nu13082795.
- Beger, R. D., S. Bhattacharyya, P. S. Gill, and L. P. James. 2018. Acylcarnitines as translational biomarkers of mitochondrial dysfunction. Mitochondrial Dysfunction Caused by Drugs and Environmental Toxicants 1–2 (Cpt 2):383–93. doi: 10.1002/9781119329725.ch24.
- Beger, R. D., W. B. Dunn, A. Bandukwala, B. Bethan, D. Broadhurst, C. B. Clish, S. Dasari, L. Derr, A. Evans, S. Fischer, et al. 2019. Towards quality assurance and quality control in untargeted metabolomics studies. Metabolomics: Official Journal of the Metabolomic Society 15 (1):4. doi: 10.1007/s11306-018-1460-7.
- Bekebrede, A. F., T. van Deuren, W. J. J. Gerrits, J. Keijer, and V. C. J. de Boer. 2021. Butyrate alters pyruvate flux and induces lipid accumulation in cultured colonocytes. International Journal of Molecular Sciences 22 (20):10937. doi: 10.3390/ijms222010937.
- Bene, J., A. Szabo, K. Komlosi, and B. Melegh. 2020. Mass spectrometric analysis of l-carnitine and its esters: Potential biomarkers of disturbances in carnitine homeostasis. Current Molecular Medicine 20 (5):336–54. doi: 10.2174/1566524019666191113120828.
- Berhane, F., A. Fite, N. Daboul, W. Al-Janabi, Z. Msallaty, M. Caruso, M. K. Lewis, Z. Yi, M. P. Diamond, A. Badi Abou-Samra, et al. 2015. Plasma lactate levels increase during hyperinsulinemic euglycemic clamp and oral glucose tolerance test. Journal of Diabetes Research 2015:1–7. doi: 10.1155/2015/102054.
- Bhagavan, N. V., and C.-E. Ha. 2011. Chapter 15 – Protein and amino acid metabolism. In Essentials of Medical Biochemistry Ha, eds. N. V. Bhagavan and C.-E. Ha, 169–90. San Diego: Academic Press. doi: 10.1016/B978-0-12-095461-2.00015-1.
- Blalock, W., Lampsas, S., M. Xenou, E. Oikonomou, P. Pantelidis, A. Lysandrou, S. Sarantos, A. Goliopoulou, K. Kalogeras, V. Tsigkou, A. Kalpis, et al. 2023. Lipoprotein(a) in atherosclerotic diseases: From pathophysiology to diagnosis and treatment. Molecules 28 (3):969. doi: 10.3390/molecules28030969.
- Bloomgarden, Z. 2018. Diabetes and branched-chain amino acids: What is the link? Journal of Diabetes. John Wiley and Sons Inc 10 (5):350–2. doi: 10.1111/1753-0407.12645.
- Boccia, S., P. Boffetta, P. Brennan, G. Ricciardi, F. Gianfagna, K. Matsuo, C. M. van Duijn, and R. J. Hung. 2009. Meta-analyses of the methylenetetrahydrofolate reductase C677T and A1298C polymorphisms and risk of head and neck and lung cancer. Cancer Letters 273 (1):55–61. doi: 10.1016/j.canlet.2008.07.026.
- Brereton, M. F., M. Rohm, K. Shimomura, C. Holland, S. Tornovsky-Babeay, D. Dadon, M. Iberl, M. V. Chibalina, S. Lee, B. Glaser, et al. 2016. Hyperglycaemia induces metabolic dysfunction and glycogen accumulation in pancreatic β-cells. Nature Communications 7 (1):13496. doi: 10.1038/ncomms13496.
- Broek, T. J., G. C. M. Bakker, C. M. Rubingh, S. Bijlsma, J. H. M. Stroeve, B. van Ommen, M. J. van Erk, and S. Wopereis. 2017. Ranges of phenotypic flexibility in healthy subjects. Genes & Nutrition 12 (1):32. doi: 10.1186/s12263-017-0589-8.
- Broers, E. R., G. Gavidia, M. Wetzels, V. Ribas, I. Ayoola, J. Piera-Jimenez, J. W. Widdershoven, and M. Habibović. 2020. Usefulness of a lifestyle intervention in patients with cardiovascular disease. The American Journal of Cardiology 125 (3):370–5. doi: 10.1016/J.AMJCARD.2019.10.041.
- Bumb, J. M., P. Bach, M. Grosshans, X. Wagner, A. Koopmann, S. Vollstädt-Klein, R. Schuster, K. Wiedemann, and F. Kiefer. 2021. BDNF influences neural cue-reactivity to food stimuli and food craving in obesity. European Archives of Psychiatry and Clinical Neuroscience 271 (5):963–74. doi: 10.1007/s00406-020-01224-w.
- Calder, P. C. 2017. Omega-3 fatty acids and inflammatory processes: From molecules to man. Biochemical Society Transactions 45 (5):1105–15. doi: 10.1042/BST20160474.
- Calder, P. C., N. Ahluwalia, R. Albers, N. Bosco, R. Bourdet-Sicard, D. Haller, S. T. Holgate, L. S. Jönsson, M. E. Latulippe, A. Marcos, et al. 2013. A consideration of biomarkers to be used for evaluation of inflammation in human nutritional studies. British Journal of Nutrition 109 (S1):S1–S34. doi: 10.1017/S0007114512005119.
- Callahan, E. A. 2022. Challenges and opportunities for precision and personalized nutrition: proceedings of a workshop. In Challenges and opportunities for precision and personalized nutrition, April. National Academies Press. doi: 10.17226/26299.
- Canfora, E. E., J. W. Jocken, and E. E. Blaak. 2015. Short-chain fatty acids in control of body weight and insulin sensitivity. Nature Reviews. Endocrinology 11 (10):577–91. doi: 10.1038/NRENDO.2015.128.
- Carlessi, R., J. Rowlands, G. Ellison, H. Helena de Oliveira Alves, P. Newsholme, and C. Mamotte. 2019. Glutamine deprivation induces metabolic adaptations associated with beta cell dysfunction and exacerbate lipotoxicity. Molecular and Cellular Endocrinology 491:110433. doi: 10.1016/j.mce.2019.04.013.
- Cena, H., and P. C. Calder. 2020. Defining a healthy diet: Evidence for the role of contemporary dietary patterns in health and disease. Nutrients 12 (2):334. doi: 10.3390/nu12020334.
- Charles, B. A., A. Doumatey, H. Huang, J. Zhou, G. Chen, D. Shriner, A. Adeyemo, and C. N. Rotimi. 2011. The roles of IL-6, IL-10, and IL-1RA in obesity and insulin resistance in African-Americans. The Journal of Clinical Endocrinology and Metabolism 96 (12):E2018–E2022. doi: 10.1210/jc.2011-1497.
- Chaudhary, N., V. Kumar, P. Sangwan, N. Chandra Pant, A. Saxena, S. Joshi, and A. Nath Yadav. 2021. Personalized nutrition and -omics. In Comprehensive foodomics, 495–507. doi: 10.1016/B978-0-08-100596-5.22880-1.
- Chen, Y., M. Michalak, and L. B. Agellon. 2018. Importance of nutrients and nutrient metabolism on human health. The Yale Journal of Biology and Medicine 91 (2):95–103.
- Chen, T., Y. Ni, X. Ma, Y. Bao, J. Liu, F. Huang, C. Hu, G. Xie, A. Zhao, W. Jia, et al. 2016. Branched-chain and aromatic amino acid profiles and diabetes risk in chinese populations. Scientific Reports 6 (1):20594. doi: 10.1038/srep20594.
- Chen, L., D. Wang, S. Garmaeva, A. Kurilshikov, A. Vich Vila, R. Gacesa, T. Sinha, E. Segal, R. K. Weersma, C. Wijmenga, et al. 2021. The Long-term genetic stability and individual specificity of the human gut microbiome. Cell 184 (9):2302–15.e12. doi: 10.1016/J.CELL.2021.03.024.
- Chew, N. W. S., C. H. Ng, D. J. H. Tan, G. Kong, C. Lin, Y. H. Chin, W. H. Lim, D. Q. Huang, J. Quek, C. E. Fu, et al. 2023. The global burden of metabolic disease: Data from 2000 to 2019. Cell Metabolism 35 (3):414–28.e3. doi: 10.1016/J.CMET.2023.02.003.
- Chiesa, S. T., M. Charakida, G. Georgiopoulos, J. D. Roberts, S. J. Stafford, C. Park, J. Mykkänen, M. Kähönen, T. Lehtimäki, M. Ala-Korpela, et al. 2022. Glycoprotein acetyls: A novel inflammatory biomarker of early cardiovascular risk in the young. Journal of the American Heart Association 11 (4):24380. doi: 10.1161/JAHA.121.024380.
- Choe, S. S., J. Y. Huh, I. J. Hwang, J. I. Kim, and J. B. Kim. 2016. Adipose tissue remodeling: Its role in energy metabolism and metabolic disorders. Frontiers in Endocrinology 7 (April):30. doi: 10.3389/fendo.2016.00030.
- Choi, C. S., Y. B. Kim, F. N. Lee, J. M. Zabolotny, B. B. Kahn, and J. H. Youn. 2002. Lactate induces insulin resistance in skeletal muscle by suppressing glycolysis and impairing insulin signaling. American Journal of Physiology-Endocrinology and Metabolism 283 (2):E233–E240. doi: 10.1152/ajpendo.00557.2001.
- Cirulli, E. T., L. Guo, C. Leon Swisher, N. Shah, L. Huang, L. A. Napier, E. F. Kirkness, T. D. Spector, C. T. Caskey, B. Thorens, et al. 2019. Profound perturbation of the metabolome in obesity is associated with health risk. Cell Metabolism 29 (2):488–500.e2. doi: 10.1016/j.cmet.2018.09.022.
- Comuzzie, A. G., S. A. Cole, S. L. Laston, V. S. Voruganti, K. Haack, R. A. Gibbs, and N. F. Butte. 2012. Novel genetic loci identified for the pathophysiology of childhood obesity in the hispanic population. PloS One 7 (12):e51954. doi: 10.1371/journal.pone.0051954.
- Connelly, M. A., E. G. Gruppen, J. Wolak-Dinsmore, S. P. Matyus, I. J. Riphagen, I. Shalaurova, S. J. Bakker, J. D. Otvos, and R. P. Dullaart. 2016. GlycA, a marker of acute phase glycoproteins, and the risk of incident type 2 diabetes mellitus: PREVEND study. Clinica Chimica Acta; International Journal of Clinical Chemistry 452 (January):10–7. doi: 10.1016/j.cca.2015.11.001.
- Connelly, M. A., J. D. Otvos, I. Shalaurova, M. P. Playford, and N. N. Mehta. 2017. GlycA, a novel biomarker of systemic inflammation and cardiovascular disease risk. Journal of Translational Medicine 15 (1):17. doi: 10.1186/s12967-017-1321-6.
- Correa Rojo, A., D. Heylen, J. Aerts, O. Thas, J. Hooyberghs, G. Ertaylan, and D. Valkenborg. 2021. Towards building a quantitative proteomics toolbox in precision medicine: A mini-review. Frontiers in Physiology 12:723510. doi: 10.3389/fphys.2021.723510.
- Cox, C. L., K. L. Stanhope, J. M. Schwarz, J. L. Graham, B. Hatcher, S. C. Griffen, A. A. Bremer, L. Berglund, J. P. McGahan, N. L. Keim, et al. 2012. Consumption of fructose- but not glucose-sweetened beverages for 10 weeks increases circulating concentrations of uric acid, retinol binding protein-4, and gamma-glutamyl transferase activity in overweight/obese humans. Nutrition & Metabolism 9 (1):1. doi: 10.1186/1743-7075-9-68.
- Crawford, S. O., R. C. Hoogeveen, F. L. Brancati, B. C. Astor, C. M. Ballantyne, M. I. Schmidt, and J. H. Young. 2010. Association of blood lactate with type 2 diabetes: The atherosclerosis risk in communities carotid MRI study. International Journal of Epidemiology 39 (6):1647–55. doi: 10.1093/ije/dyq126.
- Custodero, C., R. T. Mankowski, S. A. Lee, Z. Chen, S. Wu, T. M. Manini, J. Hincapie Echeverri, C. Sabbà, D. P. Beavers, J. A. Cauley, et al. 2018. Evidence-based nutritional and pharmacological interventions targeting chronic low-grade inflammation in middle-age and older adults: A systematic review and meta-analysis. Ageing Research Reviews 46 (September):42–59. doi: 10.1016/J.ARR.2018.05.004.
- da Silva, D. G. H., E. Belini Junior, L. de Souza Torres, J. V. Okumura, W. Marcel Barberino, R. Garcia de Oliveira, V. Urbinatti Teixeira, C. Lopes de Castro Lobo, E. Alves de Almeida, and C. R. Bonini-Domingos. 2017. Impact of genetic polymorphisms in key enzymes of homocysteine metabolism on the pathophysiology of sickle cell anemia. Free Radical Biology & Medicine 106 (May):53–61. doi: 10.1016/j.freeradbiomed.2017.02.019.
- Dąbrowska, K., and W. Witkiewicz. 2016. Correlations of host genetics and gut microbiome composition. Frontiers in Microbiology 7 (Aug):1357. doi: 10.3389/FMICB.2016.01357/BIBTEX.
- D’Angelo, S., I. C. Gormley, B. A. McNulty, A. P. Nugent, J. Walton, A. Flynn, and L. Brennan. 2019. Combining biomarker and food intake data: Calibration equations for citrus intake. The American Journal of Clinical Nutrition 110 (4):977–83. doi: 10.1093/ajcn/nqz168.
- Dastani, Z., M.-F. Hivert, N. Timpson, J. R. B. Perry, X. Yuan, R. A. Scott, P. Henneman, I. M. Heid, J. R. Kizer, L.-P. Lyytikäinen, et al. 2012. Novel loci for adiponectin levels and their influence on type 2 diabetes and metabolic traits: a multi-ethnic meta-analysis of 45,891 individuals. Edited by Peter M. PLoS Genetics 8 (3):e1002607. doi: 10.1371/journal.pgen.1002607.
- De Caterina, R., and A. El-Sohemy. 2016. Moving towards specific nutrigenetic recommendation algorithms: Caffeine, genetic variation and cardiovascular risk. Journal of Nutrigenetics and Nutrigenomics 9 (2-4):106–15. doi: 10.1159/000446801.
- de Toro-Martín, J., B. J. Arsenault, J. P. Després, and M. C. Vohl. 2017. Precision nutrition: A review of personalized nutritional approaches for the prevention and management of metabolic syndrome. Nutrients 9 (8):913. doi: 10.3390/nu9080913.
- Dehghan, P., M. A. Farhangi, L. Nikniaz, Z. Nikniaz, and M. Asghari-Jafarabadi. 2020. Gut microbiota-derived metabolite trimethylamine N-oxide (TMAO) potentially increases the risk of obesity in adults: An exploratory systematic review and dose-response meta-analysis. Obesity Reviews 21 (5): e12993. doi: 10.1111/obr.12993.
- Del Bas, J. M., A. Caimari, M. I. Rodriguez-Naranjo, C. E. Childs, C. Paras Chavez, A. L. West, E. A. Miles, L. Arola, and P. C. Calder. 2016. Impairment of lysophospholipid metabolism in obesity: Altered plasma profile and desensitization to the modulatory properties of n-3 polyunsaturated fatty acids in a randomized controlled trial. The American Journal of Clinical Nutrition 104 (2):266–79. doi: 10.3945/ajcn.116.130872.
- Di Minno, A., L. Turnu, B. Porro, I. Squellerio, V. Cavalca, E. Tremoli, and M. N. D. Di Minno. 2016. 8-Hydroxy-2-deoxyguanosine levels and cardiovascular disease: A systematic review and meta-analysis of the literature. Antioxidants & Redox Signaling 24 (10):548–55. doi: 10.1089/ars.2015.6508.
- Djuricic, I., and P. C. Calder. 2021. Beneficial outcomes of omega-6 and omega-3 polyunsaturated fatty acids on human health: An update for 2021. Nutrients 13 (7):2421. doi: 10.3390/nu13072421.
- Donovan, S. M. 2017. Introduction to the special focus issue on the impact of diet on gut microbiota composition and function and future opportunities for nutritional modulation of the gut microbiome to improve human health. Gut Microbes 8 (2):75–81. doi: 10.1080/19490976.2017.1299309.
- Dorajoo, R., Y. Sun, Y. Han, T. Ke, A. Burger, X. Chang, H. Q. Low, W. Guan, R. N. Lemaitre, C.-C. Khor, et al. 2015. A genome-wide association study of n-3 and n-6 plasma fatty acids in a Singaporean Chinese population. Genes & Nutrition 10 (6):53. doi: 10.1007/s12263-015-0502-2.
- Dorcely, B., K. Katz, R. Jagannathan, S. S. Chiang, B. Oluwadare, I. J. Goldberg, and M. Bergman. 2017. Novel biomarkers for prediabetes, diabetes, and associated complications. Diabetes, Metabolic Syndrome and Obesity: Targets and Therapy 10 (August):345–61. doi: 10.2147/DMSO.S100074.
- Dragsted, L. O., Q. Gao, A. Scalbert, G. Vergères, M. Kolehmainen, C. Manach, L. Brennan, L. A. Afman, D. S. Wishart, C. A. Lacueva, et al. 2018. Validation of biomarkers of food intake—Critical assessment of candidate biomarkers. Genes & Nutrition 13 (1):14. doi: 10.1186/s12263-018-0603-9.
- Draisma, H. H. M., R. Pool, M. Kobl, R. Jansen, A.-K. Petersen, A. A. M. Vaarhorst, I. Yet, T. Haller, A. Demirkan, T. Esko, et al. 2015. Genome-wide association study identifies novel genetic variants contributing to variation in blood metabolite levels. Nature Communications 6 (June):7208. doi: 10.1038/ncomms8208.
- Du, S., S. Sun, L. Liu, Q. Zhang, F. Guo, C. Li, R. Feng, and C. Sun. 2017. Effects of histidine supplementation on global serum and urine 1H NMR-based metabolomics and serum amino acid profiles in obese women from a randomized controlled study. Journal of Proteome Research 16 (6):2221–30. doi: 10.1021/acs.jproteome.7b00030.
- EFSA Panel on Dietetic Products. Nutrition and Allergies (NDA). 2012a. Guidance on the scientific requirements for health claims related to appetite ratings, weight management, and blood glucose concentrations. EFSA Journal 10 (3):2604. doi: 10.2903/j.efsa.2012.2604.
- EFSA Panel on Dietetic Products. Nutrition and Allergies (NDA). 2012b. Guidance on the scientific requirements for health claims related to bone, joints, skin, and oral health. EFSA Journal 10 (5):2702. doi: 10.2903/J.EFSA.2012.2702.
- EFSA Panel on Dietetic Products. Nutrition and Allergies (NDA). 2012c. Guidance on the scientific requirements for health claims related to functions of the nervous system, including psychological functions. EFSA Journal 10 (7): 2816. doi: 10.2903/J.EFSA.2012.2816.
- EFSA Panel on Dietetic Products. Nutrition and Allergies (NDA). 2016. Guidance on the scientific requirements for health claims related to the immune system, the gastrointestinal tract and defence against pathogenic microorganisms. EFSA Journal 14 (1): 4369. doi: 10.2903/J.EFSA.2016.4369.
- Elliott, R., C. Pico, Y. Dommels, I. Wybranska, J. Hesketh, and J. Keijer. 2007. Nutrigenomic approaches for benefit-risk analysis of foods and food components: Defining markers of health. The British Journal of Nutrition 98 (6):1095–100. doi: 10.1017/S0007114507803400.
- Emwas, A. H., R. Roy, R. T. McKay, L. Tenori, E. Saccenti, G. A. N. Gowda, D. Raftery, F. Alahmari, L. Jaremko, M. Jaremko, et al. 2019. NMR spectroscopy for metabolomics research. Metabolites 9 (7):123. doi: 10.3390/metabo9070123.
- Erlanson-Albertsson, C., and K. G. Stenkula. 2021. The importance of food for endotoxemia and an inflammatory response. International Journal of Molecular Sciences 22 (17):9562. doi: 10.3390/ijms22179562.
- EUR-Lex. 2014. Regulation (EC) No 1924/2006 of the European Parliament and of the Council of 20 December 2006 on Nutrition and Health Claims Made on Foods.
- Fernández-Calleja, J. M., P. Konstanti, H. J. Swarts, L. M. Bouwman, V. Garcia-Campayo, N. Billecke, A. Oosting, H. Smidt, J. Keijer, and E. M. van Schothorst. 2018. Non-invasive continuous real-time in vivo analysis of microbial hydrogen production shows adaptation to fermentable carbohydrates in mice. Scientific Reports 8 (1):1–16. doi: 10.1038/s41598-018-33619-0.
- Ferreri, C., A. Masi, A. Sansone, G. Giacometti, A. V. Larocca, G. Menounou, R. Scanferlato, S. Tortorella, D. Rota, M. Conti, et al. 2016. Fatty acids in membranes as homeostatic, metabolic and nutritional biomarkers: recent advancements in analytics and diagnostics. Diagnostics 7 (1):1. doi: 10.3390/diagnostics7010001.
- Finucane, F. M., J. Luan, N. J. Wareham, S. J. Sharp, S. O’Rahilly, B. Balkau, A. Flyvbjerg, M. Walker, K. Højlund, J. J. Nolan, et al. 2009. Correlation of the leptin: Adiponectin ratio with measures of insulin resistance in non-diabetic individuals. Diabetologia 52 (11):2345–9. doi: 10.1007/s00125-009-1508-3.
- Floris, M., A. Cano, L. Porru, R. Addis, A. Cambedda, M. L. Idda, M. Steri, C. Ventura, and M. Maioli. 2020. Direct-to-consumer nutrigenetics testing: An overview. Nutrients 12 (2):566. doi: 10.3390/nu12020566.
- Forouhi, N. G., and N. Unwin. 2019. Global diet and health: Old questions, fresh evidence, and new horizons. The Lancet 393 (10184):1916–8. doi: 10.1016/S0140-6736(19)30500-8.
- Frayling, T. M., N. J. Timpson, M. N. Weedon, E. Zeggini, R. M. Freathy, C. M. Lindgren, J. R. B. Perry, K. S. Elliott, H. Lango, N. W. Rayner, et al. 2007. A common variant in the FTO gene is associated with body mass index and predisposes to childhood and adult obesity. Science (New York, N.Y.) 316 (5826):889–94. doi: 10.1126/science.1141634.
- Freeman, A. M., and N. Pennings. 2022. Insulin resistance. Treasure Island (FL).
- Gakidou, E., A. Afshin, A. A. Abajobir, K. H. Abate, C. Abbafati, K. M. Abbas, F. Abd-Allah, A. M. Abdulle, S. F. Abera, V. Aboyans, et al. 2017. Global, regional, and national comparative risk assessment of 84 behavioural, environmental and occupational, and metabolic risks or clusters of risks, 1990–2016: A systematic analysis for the global burden of disease study 2016. The Lancet 390 (10100):1345–422. doi: 10.1016/S0140-6736(17)32366-8.
- Galmés, S., M. Cifre, A. Palou, P. Oliver, and F. Serra. 2019. A genetic score of predisposition to low-grade inflammation associated with obesity may contribute to discern population at risk for metabolic syndrome. Nutrients 11 (2):298. doi: 10.3390/nu11020298.
- Galmés, S., A. Palou, and F. Serra. 2020. Increased risk of high body fat and altered lipid metabolism associated to suboptimal consumption of vitamin a is modulated by genetic variants Rs5888 (Scarb1), Rs1800629 (Ucp1) and Rs659366 (Ucp2). Nutrients 12 (9):2588. doi: 10.3390/nu12092588.
- Galmés, S., F. Serra, and A. Palou. 2018. Vitamin E metabolic effects and genetic variants: A challenge for precision nutrition in obesity and associated disturbances. Nutrients 10 (12):1919. doi: 10.3390/nu10121919.
- Galmés, S., F. Serra, and A. Palou. 2020. Current state of evidence: Influence of nutritional and nutrigenetic factors on immunity in the COVID-19 pandemic framework. Nutrients 12 (9):2738. doi: 10.3390/nu12092738.
- Gannon, N. P., J. K. Schnuck, and R. A. Vaughan. 2018. BCAA metabolism and insulin sensitivity – dysregulated by metabolic status? Molecular Nutrition & Food Research 62 (6):1700756. doi: 10.1002/mnfr.201700756.
- Garcia-Aloy, M., M. Rabassa, P. Casas-Agustench, N. Hidalgo-Liberona, R. Llorach, and C. Andres-Lacueva. 2017. Novel strategies for improving dietary exposure assessment: Multiple-data fusion is a more accurate measure than the traditional single-biomarker approach. Trends in Food Science & Technology 69 (November):220–9. doi: 10.1016/j.tifs.2017.04.013.
- Garcia-Perez, I., J. M. Posma, E. S. Chambers, J. C. Mathers, J. Draper, M. Beckmann, J. K. Nicholson, E. Holmes, and G. Frost. 2020. Dietary metabotype modelling predicts individual responses to dietary interventions. Nature Food 1 (6):355–64. doi: 10.1038/s43016-020-0092-z.
- Gart, E., W. van Duyvenvoorde, K. Toet, M. P. Caspers, L. Verschuren, M. J. Nielsen, D. J. Leeming, E. Souto Lima, A. Menke, R. Hanemaaijer, et al. 2021. Butyrate protects against diet-induced NASH and liver fibrosis and suppresses specific non-canonical TGF-β signaling pathways in human hepatic stellate cells. Biomedicines 9 (12):1954. doi: 10.3390/biomedicines9121954.
- Ge, X., L. Zheng, R. Zhuang, P. Yu, Z. Xu, G. Liu, X. Xi, X. Zhou, and H. Fan. 2020. The gut microbial metabolite trimethylamine N-oxide and hypertension risk: A systematic review and dose-response meta-analysis. Advances in Nutrition 11 (1):66–76. doi: 10.1093/advances/nmz064.
- Gentile, C. L., and T. L. Weir. 2018. The gut microbiota at the intersection of diet and human health. Science (New York, N.Y.) 362 (6416):776–80. doi: 10.1126/science.aau5812.
- Gkouskou, K. K., M. G. Grammatikopoulou, I. Vlastos, D. Sanoudou, and A. G. Eliopoulos. 2021. Genotype-guided dietary supplementation in precision nutrition. Nutrition Reviews 79 (11):1225–35. doi: 10.1093/nutrit/nuaa132.
- Gogna, N., M. Krishna, A. M. Oommen, and K. Dorai. 2015. Investigating correlations in the altered metabolic profiles of obese and diabetic subjects in a South Indian Asian Population using an NMR-based metabolomic approach. Molecular bioSystems 11 (2):595–606. doi: 10.1039/C4MB00507D.
- Goldberg, R. B., G. A. Bray, S. M. Marcovina, K. J. Mather, T. J. Orchard, L. Perreault, and M. Temprosa. 2019. Non-traditional biomarkers and incident diabetes in the diabetes prevention program: comparative effects of lifestyle and metformin interventions. Diabetologia 62 (1):58–69. doi: 10.1007/s00125-018-4748-2.
- González Jiménez, E. 2011. Genes and obesity: A cause and effect relationship. Endocrinologia y Nutricion: organo de la Sociedad Espanola de Endocrinologia y Nutricion 58 (9):492–6. doi: 10.1016/j.endonu.2011.06.004.
- González-Domínguez, R., O. Jáuregui, P. Mena, K. Hanhineva, F. J. Tinahones, D. Angelino, and C. Andrés-Lacueva. 2020. Quantifying the human diet in the crosstalk between nutrition and health by multi-targeted metabolomics of food and microbiota-derived metabolites. International Journal of Obesity (2005) 44 (12):2372–81. doi: 10.1038/S41366-020-0628-1.
- González-Domínguez, R., M. Urpi-Sarda, O. Jáuregui, P. W. Needs, P. A. Kroon, and C. Andrés-Lacueva. 2020. Quantitative dietary fingerprinting (QDF)-a novel tool for comprehensive dietary assessment based on urinary nutrimetabolomics. Journal of Agricultural and Food Chemistry 68 (7):1851–61. doi: 10.1021/acs.jafc.8b07023.
- Gonzalez-Franquesa, A., A. M. Burkart, E. Isganaitis, and M.-E. Patti. 2016. What have metabolomics approaches taught us about type 2 diabetes? Current Diabetes Reports 16 (8):74. doi: 10.1007/s11892-016-0763-1.
- Goodarzi, M. O. 2018. Genetics of obesity: What genetic association studies have taught us about the biology of obesity and its complications. The Lancet. Diabetes & Endocrinology 6 (3):223–36. doi: 10.1016/S2213-8587(17)30200-0.
- Gschwandtner, M., R. Derler, and K. S. Midwood. 2019. More than just attractive: How CCL2 influences myeloid cell behavior beyond chemotaxis. Frontiers in Immunology 10(December):2759. doi: 10.3389/FIMMU.2019.02759/BIBTEX.
- Guasch-Ferré, M., A. Hruby, E. Toledo, C. B. Clish, M. A. Martínez-González, J. Salas-Salvadó, and F. B. Hu. 2016. Metabolomics in prediabetes and diabetes: A systematic review and meta-analysis. Diabetes Care 39 (5):833–46. doi: 10.2337/dc15-2251.
- Guasch-Ferré, M., and W. C. Willett. 2021. The Mediterranean diet and health: A comprehensive overview. Journal of Internal Medicine 290 (3):549–66. doi: 10.1111/JOIM.13333.
- Guasti, L., S. Galliazzo, M. Molaro, E. Visconti, B. Pennella, G. V. Gaudio, A. Lupi, A. M. Grandi, and A. Squizzato. 2021. TMAO as a biomarker of cardiovascular events: A systematic review and meta-analysis. Internal and Emergency Medicine 16 (1):201–7. doi: 10.1007/s11739-020-02470-5.
- Ha, C. Y., J. Y. Kim, J. K. Paik, O. Y. Kim, Y.-H. Paik, E. J. Lee, and J. H. Lee. 2012. The association of specific metabolites of lipid metabolism with markers of oxidative stress, inflammation and arterial stiffness in men with newly diagnosed type 2 diabetes. Clinical Endocrinology 76 (5):674–82. doi: 10.1111/j.1365-2265.2011.04244.x.
- Hageman, J. H. J., A. G. Nieuwenhuizen, S. M. van Ruth, J. A. Hageman, and J. Keijer. 2019. Application of volatile organic compound analysis in a nutritional intervention study: differential responses during five hours following consumption of a high- and a low-fat dairy drink. Molecular Nutrition & Food Research 63 (20):e1900189. doi: 10.1002/mnfr.201900189.
- Hammad, S., S. Pu, and P. J. Jones. 2016. Current evidence supporting the link between dietary fatty acids and cardiovascular disease. Lipids 51 (5):507–17. doi: 10.1007/s11745-015-4113-x.
- Hartiala, J. A., W. H. Wilson Tang, Z. Wang, A. L. Crow, A. F. R. Stewart, R. Roberts, R. McPherson, J. Erdmann, C. Willenborg, S. L. Hazen, et al. 2016. Genome-wide association study and targeted metabolomics identifies sex-specific association of CPS1 with coronary artery disease. Nature Communications 7 (1):10558. doi: 10.1038/ncomms10558.
- Hasani, M., A. Mansour, H. Asayesh, S. Djalalinia, A. Mahdavi Gorabi, F. Ochi, and M. Qorbani. 2021. Effect of glutamine supplementation on cardiometabolic risk factors and inflammatory markers: A systematic review and meta-analysis. BMC Cardiovascular Disorders 21 (1): 190. doi: 10.1186/s12872-021-01986-8.
- Heianza, Y., W. Ma, J. A. E. Manson, K. M. Rexrode, and L. Qi. 2017. Gut microbiota metabolites and risk of major adverse cardiovascular disease events and death: A systematic review and meta-analysis of prospective studies. Journal of the American Heart Association 6 (7):4947. doi: 10.1161/JAHA.116.004947.
- Hernandez-Baixauli, J., S. Quesada-Vázquez, R. Mariné-Casadó, K. Gil Cardoso, A. Caimari, J. M. Del Bas, X. Escoté, and L. Baselga-Escudero. 2020. Detection of early disease risk factors associated with metabolic syndrome: A new era with the NMR metabolomics assessment. Nutrients 12 (3):806. doi: 10.3390/nu12030806.
- Hoevenaars, F., J. W. van der Kamp, W. D. van den Brink, and S. Wopereis. 2020. Next generation health claims based on resilience: The example of whole-grain wheat. Nutrients 12 (10):2945. doi: 10.3390/nu12102945.
- Hoffmann, T. J., E. Theusch, T. Haldar, D. K. Ranatunga, E. Jorgenson, M. W. Medina, M. N. Kvale, P.-Y. Kwok, C. Schaefer, R. M. Krauss, et al. 2018. A large electronic-health-record-based genome-wide study of serum lipids. Nature Genetics 50 (3):401–13. doi: 10.1038/s41588-018-0064-5.
- Hopkins, M. H., W. D. Flanders, and R. M. Bostick. 2012. Associations of circulating inflammatory biomarkers with risk factors for colorectal cancer in colorectal adenoma Patients. Biomarker Insights 7:143–50. doi: 10.4137/BMI.S10092.
- Hou, K., Z.-X. Wu, X.-Y. Chen, J.-Q. Wang, D. Zhang, C. Xiao, D. Zhu, J. B. Koya, L. Wei, J. Li, et al. 2022. Microbiota in health and diseases. Signal Transduction and Targeted Therapy 7 (1):135. doi: 10.1038/s41392-022-00974-4.
- Htun, K. T., J. Pan, D. Pasanta, M. Tungjai, C. Udomtanakunchai, S. Chancharunee, S. Kaewjaeng, H. J. Kim, J. Kaewkhao, and S. Kothan. 2021. Identification of metabolic phenotypes in young adults with obesity by 1H NMR metabolomics of blood serum. Life 11 (6):574. doi: 10.3390/life11060574.
- Huber, M., J. A. Knottnerus, L. Green, H. Horst, A. R. Jadad, D. Kromhout, B. Leonard, K. Lorig, M. I. Loureiro, J. W. M. Meer, et al. 2011. How should we define health? BMJ (Clinical Research Ed.) 343 (jul26 2):d4163–d4163. doi: 10.1136/bmj.d4163.
- Huffman, K. M., S. H. Shah, R. D. Stevens, J. R. Bain, M. Muehlbauer, C. A. Slentz, C. J. Tanner, M. Kuchibhatla, J. A. Houmard, C. B. Newgard, et al. 2009. Relationships between circulating metabolic intermediates and insulin action in overweight to obese, inactive men and women. Diabetes Care 32 (9):1678–83. doi: 10.2337/dc08-2075.
- Hüls, A., U. Krämer, C. Carlsten, T. Schikowski, K. Ickstadt, and H. Schwender. 2017. Comparison of weighting approaches for genetic risk scores in gene-environment interaction studies. BMC Genetics 18 (1):115. doi: 10.1186/s12863-017-0586-3.
- Hysi, P. G., A. P. Khawaja, C. Menni, B. Tamraz, N. Wareham, K.-T. Khaw, P. J. Foster, L. Z. Benet, T. D. Spector, and C. J. Hammond. 2019. Ascorbic acid metabolites are involved in intraocular pressure control in the general population. Redox Biology 20 (January):349–53. doi: 10.1016/j.redox.2018.10.004.
- Hysi, P. G., M. Mangino, P. Christofidou, M. Falchi, E. D. Karoly, A. M. Mohney, T. D. Valdes, C. Spector, and Menni, R. P. 2022. Metabolome genome-wide association study identifies 74 novel genomic regions influencing plasma metabolites levels. Metabolites 12 (1):61. doi: 10.3390/metabo12010061.
- Il’yasova, D., P. Scarbrough, and I. Spasojevic. 2012. Urinary biomarkers of oxidative status. Clinica Chimica Acta 413 (19-20):1446–53. doi: 10.1016/j.cca.2012.06.012.
- Illig, T., C. Gieger, G. Zhai, W. Römisch-Margl, R. Wang-Sattler, C. Prehn, E. Altmaier, G. Kastenmüller, B. S. Kato, H.-W. Mewes, et al. 2010. A genome-wide perspective of genetic variation in human metabolism. Nature Genetics 42 (2):137–41. doi: 10.1038/ng.507.
- Imaizumi, A., Y. Adachi, T. Kawaguchi, K. Higasa, Y. Tabara, K. Sonomura, T.-A. Sato, M. Takahashi, T. Mizukoshi, H.-O. Yoshida, et al. 2019. Genetic basis for plasma amino acid concentrations based on absolute quantification: A genome-wide association study in the Japanese population. European Journal of Human Genetics: EJHG 27 (4):621–30. doi: 10.1038/s41431-018-0296-y.
- Jahangiri, A. 2010. High-density lipoprotein and the acute phase response. Current Opinion in Endocrinology, Diabetes, and Obesity 17 (2):156–60. doi: 10.1097/MED.0b013e328337278b.
- Jang, Y. N., J. H. Lee, J. S. Moon, D. R. Kang, S. Y. Park, J. Cho, J. Y. Kim, and J. H. Huh. 2021. Metabolic syndrome severity score for predicting cardiovascular events: A nationwide population-based study from Korea. Diabetes & Metabolism Journal 45 (4):569–77. doi: 10.4093/DMJ.2020.0103.
- Jang, C., S. F. Oh, S. Wada, G. C. Rowe, L. Liu, M. C. Chan, J. Rhee, A. Hoshino, B. Kim, A. Ibrahim, et al. 2016. A branched-chain amino acid metabolite drives vascular fatty acid transport and causes insulin resistance. Nature Medicine 22 (4):421–6. doi: 10.1038/nm.4057.
- Janssen, J. J. E., B. Lagerwaard, A. G. Nieuwenhuizen, X. Escoté, N. Canela, J. M. Del Bas, V. C. J. de Boer, and J. Keijer. 2023. Single and joined behaviour of circulating biomarkers and metabolic parameters in high-fit and low-fit healthy females. International Journal of Molecular Sciences 24 (4):4202. doi: 10.3390/ijms24044202.
- Janssen, J. J. E., B. Lagerwaard, A. G. Nieuwenhuizen, S. Timmers, V. C. J. de Boer, and J. Keijer. 2021. The effect of a single bout of exercise on vitamin B2 status is not different between high- and low-fit females. Nutrients 13 (11):4097. doi: 10.3390/nu13114097.
- Jauregibeitia, I., K. Portune, I. Rica, I. Tueros, O. Velasco, G. Grau, L. Castaño, F. Di Nolfo, C. Ferreri, and S. Arranz. 2021. Potential of erythrocyte membrane lipid profile as a novel inflammatory biomarker to distinguish metabolically healthy obesity in children. Journal of Personalized Medicine 11 (5):337. doi: 10.3390/jpm11050337.
- Jauregibeitia, I., K. Portune, I. Rica, I. Tueros, O. Velasco, G. Grau, N. Trebolazabala, L. Castaño, A. V. Larocca, C. Ferreri, et al. 2020. Fatty acid profile of mature red blood cell membranes and dietary intake as a new approach to characterize children with overweight and obesity. Nutrients 12 (11):3446. doi: 10.3390/nu12113446.
- Jayedi, A., A. Rashidy-Pour, M. Parohan, M. Sadat Zargar, and S. Shab-Bidar. 2018. Dietary antioxidants, circulating antioxidant concentrations, total antioxidant capacity, and risk of all-cause mortality: A systematic review and dose-response meta-analysis of prospective observational studies. Advances in Nutrition (Bethesda, Md.) 9 (6):701–16. doi: 10.1093/ADVANCES/NMY040.
- Jerotic, D., M. Matic, S. Suvakov, K. Vucicevic, T. Damjanovic, A. Savic-Radojevic, M. Pljesa-Ercegovac, V. Coric, A. Stefanovic, J. Ivanisevic, et al. 2019. Association of Nrf2, SOD2 and GPX1 polymorphisms with biomarkers of oxidative distress and survival in end-stage renal disease patients. Toxins 11 (7):431. doi: 10.3390/toxins11070431.
- Jia, Z., X. Zhang, S. Kang, and Y. Wu. 2013. Serum uric acid levels and incidence of impaired fasting glucose and type 2 diabetes mellitus: A meta-analysis of cohort studies. Diabetes Research and Clinical Practice 101 (1):88–96. doi: 10.1016/j.diabres.2013.03.026.
- Jiang, S., H. Liu, and C. Li. 2021. Dietary regulation of oxidative stress in chronic metabolic diseases. Foods 10 (8):1854. doi: 10.3390/foods10081854.
- Jiang, R., S. Wu, C. Fang, C. Wang, Y. Yang, C. Liu, J. Hu, and Y. Huang. 2020. Amino acids levels in early pregnancy predict subsequent gestational diabetes. Journal of Diabetes 12 (7):503–11. doi: 10.1111/1753-0407.13018.
- Johnson, R. J., S. E. Perez-Pozo, J. L. Lillo, F. Grases, J. D. Schold, M. Kuwabara, Y. Sato, A. A. Hernando, G. Garcia, T. Jensen, et al. 2018. Fructose increases risk for kidney stones: Potential role in metabolic syndrome and heat stress. BMC Nephrology 19 (1):1–7. doi: 10.1186/s12882-018-1105-0.
- Kathiresan, S., C. J. Willer, G. M. Peloso, S. Demissie, K. Musunuru, E. E. Schadt, L. Kaplan, D. Bennett, Y. Li, T. Tanaka, et al. 2009. Common variants at 30 loci contribute to polygenic dyslipidemia. Nature Genetics 41 (1):56–65. doi: 10.1038/ng.291.Common.
- Keller, K. L. 2012. Genetic influences on oral fat perception and preference. Journal of Food Science 77 (3):S143–S147. doi: 10.1111/j.1750-3841.2011.02585.x.
- Kettunen, J., A. Demirkan, P. Würtz, H. H. M. Draisma, T. Haller, R. Rawal, A. Vaarhorst, A. J. Kangas, L.-P. Lyytikäinen, M. Pirinen, et al. 2016. Genome-wide study for circulating metabolites identifies 62 loci and reveals novel systemic effects of LPA. Nature Communications 7 (March):11122. doi: 10.1038/ncomms11122.
- Khakimov, B., H. C. J. Hoefsloot, N. Mobaraki, V. Aru, M. Kristensen, M. V. Lind, L. Holm, J. L. Castro-Mejía, D. S. Nielsen, D. M. Jacobs, et al. 2022. Human blood lipoprotein predictions from (1)H NMR spectra: Protocol, model performances, and cage of covariance. Analytical Chemistry 94 (2):628–36. doi: 10.1021/acs.analchem.1c01654.
- Khera, A. V., M. Chaffin, K. H. Wade, S. Zahid, J. Brancale, R. Xia, M. Distefano, O. Senol-Cosar, M. E. Haas, A. Bick, et al. 2019. Polygenic prediction of weight and obesity trajectories from birth to adulthood. Cell 177 (3):587–96.e9. doi: 10.1016/j.cell.2019.03.028.
- Kihara, A. 2012. Very long-chain fatty acids: Elongation, physiology and related disorders. Journal of Biochemistry 152 (5):387–95. doi: 10.1093/JB/MVS105.
- Kilpeläinen, T. O., J. F. M. Carli, A. A. Skowronski, Q. Sun, J. Kriebel, M. F. Feitosa, Å. K. Hedman, A. W. Drong, J. E. Hayes, J. Zhao, et al. 2016. Genome-wide meta-analysis uncovers novel loci influencing circulating leptin levels. Nature Communications 7 (February):10494. doi: 10.1038/ncomms10494.
- Kim, J.-Y., J.-W. Lee, Y.-J. Youn, M.-S. Ahn, S.-G. Ahn, B.-S. Yoo, S.-H. Lee, J. Yoon, and K.-H. Choe. 2012. Urinary levels of 8-iso-prostaglandin F2α and 8-hydroxydeoxyguanine as markers of oxidative stress in patients with coronary artery disease. Korean Circulation Journal 42 (9):614–7. doi: 10.4070/kcj.2012.42.9.614.
- Kim, G. Y., and J.-S. Seo. 2021. A new paradigm for clinical nutrition services in the era of the fourth industrial revolution. Clinical Nutrition Research 10 (2):95–106. doi: 10.7762/cnr.2021.10.2.95.
- Klisic, A., N. Kavaric, S. Vujcic, V. Spasojevic-Kalimanovska, J. Kotur-Stevuljevic, and A. Ninic. 2021. Factorial analysis of the cardiometabolic risk influence on redox status components in adult population. Oxidative Medicine and Cellular Longevity 2021:1–9. doi: 10.1155/2021/6661940.
- Klop, B., J. W. F. Elte, and M. C. Cabezas. 2013. Dyslipidemia in obesity: Mechanisms and potential targets. Nutrients 5 (4):1218–40. doi: 10.3390/nu5041218.
- Kodama, S., K. Saito, Y. Yachi, M. Asumi, A. Sugawara, K. Totsuka, A. Saito, and H. Sone. 2009. Association between serum uric acid and development of type 2 diabetes. Diabetes Care 32 (9):1737–42. doi: 10.2337/dc09-0288.
- Korem, T., D. Zeevi, N. Zmora, O. Weissbrod, N. Bar, M. Lotan-Pompan, T. Avnit-Sagi, N. Kosower, G. Malka, M. Rein, et al. 2017. Bread affects clinical parameters and induces gut microbiome-associated personal glycemic responses. Cell Metabolism 25 (6):1243–53.e5. doi: 10.1016/j.cmet.2017.05.002.
- Korpela, K., H. J. Flint, A. M. Johnstone, J. Lappi, K. Poutanen, E. Dewulf, N. Delzenne, W. M. de Vos, and A. Salonen. 2014. Gut microbiota signatures predict host and microbiota responses to dietary interventions in obese individuals. PloS One 9 (6):e90702. doi: 10.1371/journal.pone.0090702.
- Koves, T. R., P. Li, J. An, T. Akimoto, D. Slentz, O. Ilkayeva, G. L. Dohm, Z. Yan, C. B. Newgard, and D. M. Muoio. 2005. Peroxisome proliferator-activated receptor-gamma co-activator 1alpha-mediated metabolic remodeling of skeletal myocytes mimics exercise training and reverses lipid-induced mitochondrial inefficiency. The Journal of Biological Chemistry 280 (39):33588–98. doi: 10.1074/jbc.M507621200.
- Kroese, L. J., and P. G. Scheffer. 2014. 8-hydroxy-2′-deoxyguanosine and cardiovascular disease: A systematic review. Current Atherosclerosis Reports 16 (11): 452. doi: 10.1007/s11883-014-0452-y.
- Kulminski, A. M., J. Huang, Y. Loika, K. G. Arbeev, O. Bagley, A. Yashkin, M. Duan, and I. Culminskaya. 2018. Strong impact of natural-selection-free heterogeneity in genetics of age-related phenotypes. Aging 10 (3):492–514. doi: 10.18632/aging.101407.
- Laddu, D., and M. Hauser. 2019. Addressing the nutritional phenotype through personalized nutrition for chronic disease prevention and management. Progress in Cardiovascular Diseases 62 (1):9–14. doi: 10.1016/j.pcad.2018.12.004.
- Lagerwaard, B., A. G. Nieuwenhuizen, V. C. de Boer, and J. Keijer. 2020. In vivo assessment of mitochondrial capacity using NIRS in locomotor muscles of young and elderly males with similar physical activity levels. GeroScience 42 (1):299–310. doi: 10.1007/S11357-019-00145-4.
- Lagerwaard, B., M. D. van der Hoek, J. Hoeks, L. Grevendonk, A. G. Nieuwenhuizen, J. Keijer, and V. C. J. de Boer. 2021. Propionate hampers differentiation and modifies histone propionylation and acetylation in skeletal muscle cells. Mechanisms of Ageing and Development 196 (April):111495. doi: 10.1016/j.mad.2021.111495.
- Laíns, I., D. Duarte, A. S. Barros, A. S. Martins, T. J. Carneiro, J. Q. Gil, J. B. Miller, M. Marques, T. S. Mesquita, P. Barreto, et al. 2019. Urine nuclear magnetic resonance (NMR) metabolomics in age-related macular degeneration. Journal of Proteome Research 18 (3):1278–88. doi: 10.1021/ACS.JPROTEOME.8B00877/SUPPL_FILE/PR8B00877_SI_001.PDF.
- Lam, T. J., and Y. Ye. 2022. Meta-analysis of microbiome association networks reveal patterns of dysbiosis in diseased microbiomes. Scientific Reports 12 (1):17482. doi: 10.1038/s41598-022-22541-1.
- Le Lay, S., G. Simard, M. C. Martinez, and R. Andriantsitohaina. 2014. Oxidative stress and metabolic pathologies: From an adipocentric point of view. Oxidative Medicine and Cellular Longevity 2014:1–18. doi: 10.1155/2014/908539.
- Lek, F.-Y., H.-H. Ong, and Y.-H. Say. 2018. Association of dopamine receptor D2 gene (DRD2) Taq1 polymorphisms with eating behaviors and obesity among Chinese and Indian Malaysian university students. Asia Pacific Journal of Clinical Nutrition 27 (3):707–17. doi: 10.6133/apjcn.092017.09.
- Leon-Cabrera, S., Y. Arana-Lechuga, E. Esqueda-León, G. Terán-Pérez, A. Gonzalez-Chavez, G. Escobedo, and J. Velázquez Moctezuma. 2015. Reduced systemic levels of {IL}-10 are associated with the severity of obstructive sleep apnea and insulin resistance in morbidly obese humans. Mediators of Inflammation 2015:493409. doi: 10.1155/2015/493409.
- Ligthart, S., A. Vaez, U. Võsa, M. G. Stathopoulou, P. S. de Vries, B. P. Prins, P. J. Van der Most, T. Tanaka, E. Naderi, L. M. Rose, et al. 2018. Genome analyses of >200,000 individuals identify 58 loci for chronic inflammation and highlight pathways that link inflammation and complex disorders. American Journal of Human Genetics 103 (5):691–706. doi: 10.1016/j.ajhg.2018.09.009.
- Li, D., Y. Lu, S. Yuan, X. Cai, Y. He, J. Chen, Q. Wu, D. He, A. Fang, Y. Bo, et al. 2022. Gut microbiota-derived metabolite trimethylamine-n-oxide and multiple health outcomes: An umbrella review and updated meta-analysis. The American Journal of Clinical Nutrition 116 (1):230–43. doi: 10.1093/ajcn/nqac074.
- Li, G., T. D. Petkova, E. Laritsky, N. Kessler, M. S. Baker, S. Zhu, and R. A. Waterland. 2019. Early postnatal overnutrition accelerates aging-associated epigenetic drift in pancreatic islets. Environmental Epigenetics 5 (3):dvz015. doi: 10.1093/eep/dvz015.
- Li, T., X.-P. Zhao, L.-Y. Wang, S. Gao, J. Zhao, Y.-C. Fan, and K. Wang. 2013. Glutathione S-transferase P1 correlated with oxidative stress in hepatocellular carcinoma. International Journal of Medical Sciences 10 (6):683–90. doi: 10.7150/ijms.5947.
- Ljungman, P., T. Bellander, F. Nyberg, E. Lampa, B. Jacquemin, M. Kolz, T. Lanki, J. Mitropoulos, M. Müller, S. Picciotto, et al. 2009. DNA variants, plasma levels and variability of interleukin-6 in myocardial infarction survivors: results from the AIRGENE study. Thrombosis Research 124 (1):57–64. doi: 10.1016/j.thromres.2008.10.009.
- Long, B., R. Muhamad, G. Yan, J. Yu, Q. Fan, Z. Wang, X. Li, A. Purnomoadi, J. Achmadi, and X. Yan. 2016. Quantitative proteomics analysis reveals glutamine deprivation activates fatty acid β-oxidation pathway in HepG2 cells. Amino Acids 48 (5):1297–307. doi: 10.1007/s00726-016-2182-7.
- Longo, M., F. Zatterale, J. Naderi, L. Parrillo, P. Formisano, G. A. Raciti, F. Beguinot, and C. Miele. 2019. Adipose tissue dysfunction as determinant of obesity-associated metabolic complications. International Journal of Molecular Sciences 20 (9):2358. doi: 10.3390/ijms20092358.
- Loo, R. L., Q. Chan, J. K. Nicholson, and E. Holmes. 2022. Balancing the equation: A natural history of trimethylamine and trimethylamine-N-oxide. Journal of Proteome Research 21 (3):560–89. doi: 10.1021/acs.jproteome.1c00851.
- Loos, R. J. 2012. Genetic determinants of common obesity and their value in prediction. Best Practice & Research. Clinical Endocrinology & Metabolism 26 (2):211–26. doi: 10.1016/j.beem.2011.11.003.
- Loos, R. J. F., C. M. Lindgren, S. Li, E. Wheeler, J. H. Zhao, I. Prokopenko, M. Inouye, R. M. Freathy, A. P. Attwood, J. S. Beckmann, et al. 2008. Common variants near MC4R are associated with fat mass, weight and risk of obesity. Nature Genetics 40 (6):768–75. doi: 10.1038/ng.140.
- Loos, R. J., and G. S. Yeo. 2022. The genetics of obesity: From discovery to biology. Nature Reviews Genetics 23 (2):120–33. doi: 10.1038/s41576-021-00414-z.
- López-Jaramillo, P., D. Gómez-Arbeláez, J. López-López, C. López-López, J. Martínez-Ortega, A. Gómez-Rodríguez, and S. Triana-Cubillos. 2014. The role of leptin/adiponectin ratio in metabolic syndrome and diabetes. Hormone Molecular Biology and Clinical Investigation 18 (1):37–45. doi: 10.1515/hmbci-2013-0053.
- Lorenzo, P. M., A. G. Izquierdo, G. Rodriguez-Carnero, A. Fernández-Pombo, A. Iglesias, M. C. Carreira, C. Tejera, D. Bellido, M. A. Martinez-Olmos, R. Leis, et al. 2022. Epigenetic effects of healthy foods and lifestyle habits from the Southern European Atlantic diet pattern: A narrative review. Advances in Nutrition (Bethesda, Md.) 13 (5):1725–47. doi: 10.1093/advances/nmac038.
- Lotta, L. A., R. A. Scott, S. J. Sharp, S. Burgess, J. Luan, T. Tillin, A. F. Schmidt, F. Imamura, I. D. Stewart, J. R. B. Perry, et al. 2016. Genetic predisposition to an impaired metabolism of the branched-chain amino acids and risk of type 2 diabetes: A Mendelian randomisation analysis. PLoS Medicine 13 (11):e1002179. doi: 10.1371/journal.pmed.1002179.
- Lovejoy, J., F. D. Newby, S. S. Gebhart, and M. DiGirolamo. 1992. Insulin resistance in obesity is associated with elevated basal lactate levels and diminished lactate appearance following intravenous glucose and insulin. Metabolism: clinical and Experimental 41 (1):22–7. doi: 10.1016/0026-0495(92)90185-D.
- Lukito, W., S. G. Malik, I. S. Surono, and M. L. Wahlqvist. 2015. From “lactose intolerance” to “lactose nutrition. Asia Pacific Journal of Clinical Nutrition 24 (Suppl 1):S1–S8. doi: 10.6133/apjcn.2015.24.s1.01.
- Lv, Q., X. F. Meng, F. F. He, S. Chen, H. Su, J. Xiong, P. Gao, X. Juan Tian, J. S. Liu, Z. Hua Zhu, et al. 2013. High serum uric acid and increased risk of type 2 diabetes: A systemic review and meta-analysis of prospective cohort studies. PLoS One. 8 (2):e56864. doi: 10.1371/journal.pone.0056864.
- MacCaferri, S., E. Biagi, and P. Brigidi. 2011. Metagenomics: Key to human gut microbiota. Digestive Diseases (Basel, Switzerland) 29 (6):525–30. doi: 10.1159/000332966.
- Mahajan, A., X. Sim, H. J. Ng, A. Manning, M. A. Rivas, H. M. Highland, A. E. Locke, N. Grarup, H. K. Im, P. Cingolani, et al. 2015. Identification and functional characterization of G6PC2 coding variants influencing glycemic traits define an effector transcript at the G6PC2-ABCB11 locus. PLoS Genetics 11 (1):e1004876. doi: 10.1371/journal.pgen.1004876.
- Mai, M., A. Tönjes, P. Kovacs, M. Stumvoll, G. M. Fiedler, and A. Benedikt Leichtle. 2013. Serum levels of acylcarnitines are altered in prediabetic conditions. PLoS One. 8 (12):e82459. doi: 10.1371/journal.pone.0082459.
- Maltais-Payette, I., M.-M. Boulet, C. Prehn, J. Adamski, and A. Tchernof. 2018. Circulating glutamate concentration as a biomarker of visceral obesity and associated metabolic alterations. Nutrition & Metabolism 15 (1):78. doi: 10.1186/s12986-018-0316-5.
- Mandracchia, F., E. Llauradó, L. Tarro, J. del Bas, R. Valls, A. Pedret, P. Radeva, L. Arola, R. Solà, and N. Boqué. 2019. Potential use of mobile phone applications for self-monitoring and increasing daily fruit and vegetable consumption: A systematized review. Nutrients 11 (3):686. doi: 10.3390/nu11030686.
- Manning, A. K., M.-F. Hivert, R. A. Scott, J. L. Grimsby, N. Bouatia-Naji, H. Chen, D. Rybin, C.-T. Liu, L. F. Bielak, I. Prokopenko, et al. 2012. A genome-wide approach accounting for body mass index identifies genetic variants influencing fasting glycemic traits and insulin resistance. Nature Genetics 44 (6):659–69. doi: 10.1038/ng.2274.
- Marti, A., I. Martínez, A. Ojeda‐rodríguez, and M. C. Azcona‐sanjulian. 2021. Higher lipopolysaccharide binding protein and chemerin concentrations were associated with metabolic syndrome features in pediatric subjects with abdominal obesity during a lifestyle intervention. Nutrients 13 (2):289. doi: 10.3390/nu13020289.
- Martini, D., M. Tucci, J. Bradfield, A. Di Giorgio, M. Marino, C. Del Bo’, M. Porrini, and P. Riso. 2021. Principles of sustainable healthy diets in worldwide dietary guidelines: Efforts so far and future perspectives. Nutrients 13 (6):1827. doi: 10.3390/nu13061827.
- Maruvada, P., J. W. Lampe, D. S. Wishart, D. Barupal, D. N. Chester, D. Dodd, Y. Djoumbou-Feunang, P. C. Dorrestein, L. O. Dragsted, J. Draper, et al. 2020. Perspective: Dietary biomarkers of intake and exposure-exploration with omics approaches. Advances in Nutrition (Bethesda, Md.) 11 (2):200–15. doi: 10.1093/advances/nmz075.
- Matsumori, R., T. Miyazaki, K. Shimada, A. Kume, Y. Kitamura, K. Oshida, N. Yanagisawa, T. Kiyanagi, M. Hiki, K. Fukao, et al. 2013. High levels of very long-chain saturated fatty acid in erythrocytes correlates with atherogenic lipoprotein profiles in subjects with metabolic syndrome. Diabetes Research and Clinical Practice 99 (1):12–8. doi: 10.1016/J.DIABRES.2012.10.025.
- Matthan, N. R., L. M. Ausman, H. Meng, H. Tighiouart, and A. H. Lichtenstein. 2016. Estimating the reliability of glycemic index values and potential sources of methodological and biological variability. The American Journal of Clinical Nutrition 104 (4):1004–13. doi: 10.3945/ajcn.116.137208.
- Matthews, D. R., J. P. Hosker, A. S. Rudenski, B. A. Naylor, D. F. Treache, and D. R. Turner. 1985. Homeostasis model assessment: Insulin resistance and beta-cell function from fasting plasma glucose and insulin concentrations in man. Diabetologia 28 (7):412–9. doi: 10.1007/BF00280883.
- Mayorga-Ramos, A., C. Barba-Ostria, D. Simancas-Racines, and L. P. Guamán. 2022. Protective role of butyrate in obesity and diabetes: new insights. Frontiers in Nutrition 9:1067647. doi: 10.3389/fnut.2022.1067647.
- McGarrah, R. W., J. P. Kelly, D. M. Craig, C. Haynes, R. C. Jessee, K. M. Huffman, W. E. Kraus, and S. H. Shah. 2017. A novel protein glycan-derived inflammation biomarker independently predicts cardiovascular disease and modifies the association of HDL subclasses with mortality. Clinical Chemistry 63 (1):288–96. doi: 10.1373/clinchem.2016.261636.
- Meng, D., L. Liang, and X. Guo. 2019. Serum interleukin-10 level in patients with inflammatory bowel disease: A meta-analysis. European Journal of Inflammation 17 (January):205873921984340. doi: 10.1177/2058739219843405.
- Meng, H., N. R. Matthan, L. M. Ausman, and A. H. Lichtenstein. 2017. Effect of prior meal macronutrient composition on postprandial glycemic responses and glycemic index and glycemic load value determinations. The American Journal of Clinical Nutrition 106 (5):1246–56. doi: 10.3945/ajcn.117.162727.
- Meyer, K. A., and J. W. Shea. 2017. Dietary choline and betaine and risk of CVD: A systematic review and meta-analysis of prospective studies. Nutrients 9 (7):711. doi: 10.3390/nu9070711.
- Mihalik, S. J., B. H. Goodpaster, D. E. Kelley, D. H. Chace, J. Vockley, F. G. Toledo, and J. P. Delany. 2010. Increased levels of plasma acylcarnitines in obesity and type 2 diabetes and identification of a marker of glucolipotoxicity. Obesity 18 (9):1695–700. doi: 10.1038/oby.2009.510.
- Milajerdi, A., S. M. Mousavi, A. Sadeghi, A. Salari-Moghaddam, M. Parohan, B. Larijani, and A. Esmaillzadeh. 2020. The effect of probiotics on inflammatory biomarkers: A meta-analysis of randomized clinical trials. European Journal of Nutrition 59 (2):633–49. doi: 10.1007/S00394-019-01931-8.
- Milne, G. L., E. S. Musiek, and J. D. Morrow. 2005. F2-isoprostanes as markers of oxidative stress in vivo: An overview. Biomarkers 10 (Suppl 1):S10–S23. doi: 10.1080/13547500500216546.
- Minihane, A. M., S. Vinoy, W. R. Russell, A. Baka, H. M. Roche, K. M. Tuohy, J. L. Teeling, E. E. Blaak, M. Fenech, D. Vauzour, et al. 2015. Low-grade inflammation, diet composition and health: Current research evidence and its translation. British Journal of Nutrition 114 (7):999–1012. doi: 10.1017/S0007114515002093.
- Mir, R., M. Bhat, J. Javid, C. Jha, A. Saxena, and S. Banu. 2018. Potential impact of COMT-Rs4680 G > A gene polymorphism in coronary artery disease. Journal of Cardiovascular Development and Disease 5 (3):38. doi: 10.3390/jcdd5030038.
- Miyazaki, M., and J. M. Ntambi. 2003. Role of stearoyl-coenzyme a desaturase in lipid metabolism. Prostaglandins, Leukotrienes, and Essential Fatty Acids 68 (2):113–21. doi: 10.1016/S0952-3278(02)00261-2.
- Mohammad, S., and C. Thiemermann. 2020. Role of metabolic endotoxemia in systemic inflammation and potential interventions. Frontiers in Immunology 11 (January):594150. doi: 10.3389/fimmu.2020.594150.
- Moon, S., J. J. Tsay, H. Lampert, Z. I. Md Dom, A. D. Kostic, A. Smiles, and M. A. Niewczas. 2021. Circulating short and medium chain fatty acids are associated with normoalbuminuria in type 1 diabetes of long duration. Scientific Reports 11 (1): 8592. doi: 10.1038/s41598-021-87585-1.
- Morrison, L., J. A. Laukkanen, K. Ronkainen, S. Kurl, J. Kauhanen, and A. T. Toriola. 2016. Inflammatory biomarker score and cancer: A population-based prospective cohort study. BMC Cancer 16 (1):80. doi: 10.1186/s12885-016-2115-6.
- Morze, J., C. Wittenbecher, L. Schwingshackl, A. Danielewicz, A. Rynkiewicz, F. B. Hu, and M. Guasch-Ferre. 2022. Metabolomics and type 2 diabetes risk: an updated systematic review and meta-analysis of prospective cohort studies. Diabetes Care 45 (4):1013–24. doi: 10.2337/DC21-1705.
- Moszak, M., M. Szulińska, and P. Bogdański. 2020. You are what you eat—the relationship between diet, microbiota, and metabolic disorders—A review. Nutrients. MDPI AG 12 (4):1096. doi: 10.3390/nu12041096.
- Motamedi, A., M. Askari, H. Mozaffari, R. Homayounfrar, A. Nikparast, M. L. Ghazi, M. M. Nejad, and S. Alizadeh. 2022. Dietary inflammatory index in relation to type 2 diabetes: A meta-analysis. International Journal of Clinical Practice 2022 (February):9953115. doi: 10.1155/2022/9953115.
- Mukherjee, M. S., C. Y. Han, S. Sukumaran, C. L. Delaney, and M. D. Miller. 2022. Effect of anti-inflammatory diets on inflammation markers in adult human populations: A systematic review of randomized controlled trials. Nutrition Reviews 81 (1):55–74. doi: 10.1093/nutrit/nuac045.
- Mullins, V. A., W. Bresette, L. Johnstone, B. Hallmark, and F. H. Chilton. 2020. Genomics in personalized nutrition: can you “eat for your genes”? Nutrients 12 (10):3118. doi: 10.3390/nu12103118.
- Murray, C. J. L., A. Y. Aravkin, P. Zheng, C. Abbafati, K. M. Abbas, M. Abbasi-Kangevari, F. Abd-Allah, A. Abdelalim, M. Abdollahi, I. Abdollahpour, et al. 2020. Global burden of 87 risk factors in 204 countries and territories, 1990–2019: A systematic analysis for the global burden of disease study 2019. The Lancet 396 (10258):1223–49. doi: 10.1016/S0140-6736(20)30752-2.
- Nagrani, R., R. Foraita, M. Wolters, S. De Henauw, S. Marild, D. Molnár, L. A. Moreno, P. Russo, M. Tornaritis, T. Veidebaum, et al. 2022. Longitudinal association of inflammatory markers with markers of glycaemia and insulin resistance in European Children. Diabetes/Metabolism Research and Reviews 38 (3):e3511. doi: 10.1002/DMRR.3511.
- Nagy, R., T. S. Boutin, J. Marten, J. E. Huffman, S. M. Kerr, A. Campbell, L. Evenden, J. Gibson, C. Amador, D. M. Howard, et al. 2017. Exploration of haplotype research consortium imputation for genome-wide association studies in 20,032 generation scotland participants. Genome Medicine 9 (1):1. doi: 10.1186/s13073-017-0414-4.
- Newgard, C. B. 2017. Metabolomics and metabolic diseases: Where do we stand? Cell Metabolism 25 (1):43–56. doi: 10.1016/j.cmet.2016.09.018.
- Newman, J. C., and E. Verdin. 2014. β-Hydroxybutyrate: Much more than a metabolite. Diabetes Research and Clinical Practice 106 (2):173–81. doi: 10.1016/j.diabres.2014.08.009.
- Ni, C., Q. Jia, G. Ding, X. Wu, and M. Yang. 2022. Low-glycemic index diets as an intervention in metabolic diseases: A systematic review and meta-analysis. Nutrients 14 (2):307. doi: 10.3390/nu14020307.
- Niforou, A., V. Konstantinidou, and A. Naska. 2020. Genetic variants shaping inter-individual differences in response to dietary intakes—A narrative review of the case of vitamins. Frontiers in Nutrition 7 (December): 558598. doi: 10.3389/fnut.2020.558598.
- Nutrition and Food Systems Division. 2016. Influencing food environments for healthy diets. Edited by Food and agriculture organization of the United Nations.
- Olsen, J. V., and M. Mann. 2004. Improved peptide identification in proteomics by two consecutive stages of mass spectrometric fragmentation. Proceedings of the National Academy of Sciences of the United States of America 101 (37):13417–22. doi: 10.1073/pnas.0405549101.
- Ordovas, J. M., L. R. Ferguson, E. S. Tai, and J. C. Mathers. 2018. Personalised Nutrition and Health. BMJ (Online) 361:bmj.k2173. doi: 10.1136/bmj.k2173.
- Ouyang, W., and A. O’Garra. 2019. IL-10 family cytokines IL-10 and IL-22: From basic science to clinical translation. Immunity 50 (4):871–91. doi: 10.1016/J.IMMUNI.2019.03.020.
- Palau-Rodriguez, M., S. Tulipani, M. Isabel Queipo-Ortuño, M. Urpi-Sarda, F. J. Tinahones, and C. Andres-Lacueva. 2015. Metabolomic insights into the intricate gut microbial-host interaction in the development of obesity and type 2 diabetes. Frontiers in Microbiology 6:1151. doi: 10.3389/fmicb.2015.01151.
- Panduro, A., I. Rivera-Iñiguez, M. Sepulveda-Villegas, and S. Roman. 2017. Genes, emotions and gut microbiota: The next frontier for the gastroenterologist. World Journal of Gastroenterology 23 (17):3030–42. doi: 10.3748/wjg.v23.i17.3030.
- Papakonstantinou, E., C. Oikonomou, G. Nychas, and G. D. Dimitriadis. 2022. Effects of diet, lifestyle, chrononutrition and alternative dietary interventions on postprandial glycemia and insulin resistance. Nutrients 14 (4):823. doi: 10.3390/nu14040823.
- Pasanta, D., S. Chancharunee, M. Tungjai, H. J. Kim, and S. Kothan. 2019. Effects of obesity on the lipid and metabolite profiles of young adults by serum (1)H-NMR spectroscopy. PeerJ. 7:e7137. doi: 10.7717/peerj.7137.
- Pausova, Z., C. Syme, M. Abrahamowicz, Y. Xiao, G. T. Leonard, M. Perron, L. Richer, S. Veillette, G. D. Smith, O. Seda, et al. 2009. A common variant of the FTO Gene is associated with not only increased adiposity but also elevated blood pressure in french Canadians. Circulation. Cardiovascular Genetics 2 (3):260–9. doi: 10.1161/CIRCGENETICS.109.857359.
- Peña-Romero, A. C., D. Navas-Carrillo, F. Marín, and E. Orenes-Piñero. 2018. The future of nutrition: Nutrigenomics and nutrigenetics in obesity and cardiovascular diseases. Critical Reviews in Food Science and Nutrition 58 (17):3030–41. doi: 10.1080/10408398.2017.1349731.
- Perla, F., M. Prelati, M. Lavorato, D. Visicchio, and C. Anania. 2017. The role of lipid and lipoprotein metabolism in non‐alcoholic fatty liver disease. Children 4 (6):46. doi: 10.3390/children4060046.
- Picó, C., F. Serra, A. M. Rodríguez, J. Keijer, and A. Palou. 2019. Biomarkers of nutrition and health: New tools for new approaches. Nutrients. MDPI AG 11 (5):1092. doi: 10.3390/nu11051092.
- Playdon, M. C., S. C. Moore, A. Derkach, J. Reedy, A. F. Subar, J. N. Sampson, D. Albanes, F. Gu, J. Kontto, C. Lassale, et al. 2017. Identifying biomarkers of dietary patterns by using metabolomics. The American Journal of Clinical Nutrition 105 (2):450–65. doi: 10.3945/ajcn.116.144501.
- Portha, B., A. Fournier, M. D. A. Kioon, V. Mezger, and J. Movassat. 2014. Early environmental factors, alteration of epigenetic marks and metabolic disease susceptibility. Biochimie 97 (February):1–15. doi: 10.1016/j.biochi.2013.10.003.
- Posma, J. M., I. Garcia-Perez, G. Frost, G. S. Aljuraiban, Q. Chan, L. Van Horn, M. Daviglus, J. Stamler, E. Holmes, P. Elliott, et al. 2020. Nutriome–metabolome relationships provide insights into dietary intake and metabolism. Nature Food 1 (7):426–36. doi: 10.1038/s43016-020-0093-y.
- Qin, J., Y. Li, Z. Cai, S. Li, J. Zhu, F. Zhang, S. Liang, W. Zhang, Y. Guan, D. Shen, et al. 2012. A metagenome-wide association study of gut microbiota in type 2 diabetes. Nature 490 (7418):55–60. doi: 10.1038/nature11450.
- Qiu, S., X. Cai, J. Liu, B. Yang, M. Zügel, J. M. Steinacker, Z. Sun, and U. Schumann. 2019. Association between circulating cell adhesion molecules and risk of type 2 diabetes: A meta-analysis. Atherosclerosis 287 (August):147–54. doi: 10.1016/J.ATHEROSCLEROSIS.2019.06.908.
- Ramos-Lopez, O., I. Milton-Laskibar, and J. A. Martínez. 2021. Precision nutrition based on phenotypical traits and the (epi)genotype: Nutrigenetic and nutrigenomic approaches for obesity care. Current Opinion in Clinical Nutrition and Metabolic Care 24 (4):315–25. doi: 10.1097/MCO.0000000000000754.
- Rawat, A., G. Misra, M. Saxena, S. Tripathi, D. Dubey, S. Saxena, A. Aggarwal, V. Gupta, M. Y. Khan, and A. Prakash. 2019. 1H NMR based serum metabolic profiling reveals differentiating biomarkers in patients with diabetes and diabetes-related complication. Diabetes & Metabolic Syndrome 13 (1):290–8. doi: 10.1016/j.dsx.2018.09.009.
- Reuter, S. E., and A. M. Evans. 2012. Carnitine and acylcarnitines. Clinical Pharmacokinetics 51 (9):553–72. doi: 10.1007/BF03261931.
- Ridker, P. M., J. E. Buring, N. R. Cook, and N. Rifai. 2003. C-reactive protein, the metabolic syndrome, and risk of incident cardiovascular events: An 8-Year follow-up of 14 719 initially healthy American women. Circulation 107 (3):391–7. doi: 10.1161/01.CIR.0000055014.62083.05.
- Roberts, L. D., A. Koulman, and J. L. Griffin. 2014. Towards metabolic biomarkers of insulin resistance and type 2 diabetes: Progress from the metabolome. The Lancet Diabetes and Endocrinology. Lancet Publishing Group 2 (1):65–75. doi: 10.1016/S2213-8587(13)70143-8.
- Robinette, S. L., E. Holmes, J. K. Nicholson, and M. E. Dumas. 2012. Genetic determinants of metabolism in health and disease: From biochemical genetics to genome-wide associations. Genome Medicine 4 (4):30. doi: 10.1186/gm329.
- Rossi, A., M. Ruoppolo, P. Formisano, G. Villani, L. Albano, G. Gallo, D. Crisci, A. Moccia, G. Parenti, P. Strisciuglio, et al. 2018. Insulin-resistance in glycogen storage disease type Ia: Linking carbohydrates and mitochondria? Journal of Inherited Metabolic Disease 41 (6):985–95. doi: 10.1007/s10545-018-0149-4.
- Rowland, I., G. Gibson, A. Heinken, K. Scott, J. Swann, I. Thiele, and K. Tuohy. 2018. Gut Microbiota functions: Metabolism of nutrients and other food components. European Journal of Nutrition 57 (1):1–24. doi: 10.1007/s00394-017-1445-8.
- Sacks, G., J. Kwon, and K. Backholer. 2021. Do taxes on unhealthy foods and beverages influence food purchases? Current Nutrition Reports 10 (3):179–87. doi: 10.1007/s13668-021-00358-0.
- Sacks, F. M., A. H. Lichtenstein, J. H. Wu, L. J. Appel, M. A. Creager, P. M. Kris-Etherton, M. Miller, E. B. Rimm, L. L. Rudel, J. G. Robinson, et al. 2017. Dietary fats and cardiovascular disease: A presidential advisory from the American Heart Association. Circulation 136 (3):e1–e23. doi: 10.1161/CIR.0000000000000510.
- Saito, N., T. Saito, T. Yamazaki, Y. Fujimine, and T. Ihara. 2017. Establishment of an analytical method for accurate purity evaluations of acylcarnitines by using quantitative 1H NMR spectroscopy. Accreditation and Quality Assurance 22 (4):171–8. doi: 10.1007/s00769-017-1263-y.
- Schooneman, M. G., N. Achterkamp, C. A. Argmann, M. R. Soeters, and S. M. Houten. 2014. Plasma acylcarnitines inadequately reflect tissue acylcarnitine metabolism. Biochimica et Biophysica Acta (BBA) - Molecular and Cell Biology of Lipids 1841 (7):987–94. doi: 10.1016/j.bbalip.2014.04.001.
- Schram, M. T., S. J. S. Sep, C. J. van der Kallen, P. C. Dagnelie, A. Koster, N. Schaper, R. M. A. Henry, and C. D. A. Stehouwer. 2014. The Maastricht study: An extensive phenotyping study on determinants of type 2 diabetes, its complications and its comorbidities. European Journal of Epidemiology 29 (6):439–51. doi: 10.1007/s10654-014-9889-0.
- Schwartz, B. 2014. New criteria for supplementation of selected micronutrients in the era of nutrigenetics and nutrigenomics. International Journal of Food Sciences and Nutrition 65 (5):529–38. doi: 10.3109/09637486.2014.898258.
- Schwingshackl, L., and G. Hoffmann. 2014. Mediterranean dietary pattern, inflammation and endothelial function: A systematic review and meta-analysis of intervention trials. Nutrition, Metabolism, and Cardiovascular Diseases: NMCD 24 (9):929–39. doi: 10.1016/J.NUMECD.2014.03.003.
- Sempionatto, J. R., V. R.-V. Montiel, E. Vargas, H. Teymourian, and J. Wang. 2021. Wearable and mobile sensors for personalized nutrition. ACS Sensors 6 (5):1745–60. doi: 10.1021/acssensors.1c00553.
- Shabana, S. U. Shahid, and S. Hasnain. 2018. Use of a gene score of multiple low-modest effect size variants can predict the risk of obesity better than the individual SNPs. Lipids in Health and Disease 17 (1):155. doi: 10.1186/s12944-018-0806-5.
- Shearer, J., G. Duggan, A. Weljie, D. S. Hittel, D. H. Wasserman, and H. J. Vogel. 2008. Metabolomic profiling of dietary-induced insulin resistance in the high fat-fed C57BL/6J mouse. Diabetes, Obesity & Metabolism 10 (10):950–8. doi: 10.1111/j.1463-1326.2007.00837.x.
- Shimazu, T., M. D. Hirschey, J. Newman, W. He, K. Shirakawa, N. Le Moan, C. A. Grueter, H. Lim, L. R. Saunders, R. D. Stevens, et al. 2013. Suppression of oxidative stress by β-hydroxybutyrate, an endogenous histone deacetylase inhibitor. Science (New York, N.Y.) 339 (6116):211–4. doi: 10.1126/science.1227166.
- Shin, S. Y., E. B. Fauman, A. K. Petersen, J. Krumsiek, R. Santos, J. Huang, M. Arnold, I. Erte, V. Forgetta, T. P. Yang, et al. 2014. An atlas of genetic influences on human blood metabolites. Nature Genetics 46 (6):543–50. doi: 10.1038/ng.2982.
- Shivappa, N., J. R. Hebert, M. Kivimaki, and T. Akbaraly. 2017. Alternative Healthy Eating Index 2010, dietary inflammatory index and risk of mortality: Results from the Whitehall II cohort study and meta-analysis of previous dietary inflammatory index and mortality studies. The British Journal of Nutrition 118 (3):210–21. doi: 10.1017/S0007114517001908.
- Simó, C., and V. García-Cañas. 2020. Dietary bioactive ingredients to modulate the gut microbiota-derived metabolite TMAO. New opportunities for functional food development. Food & Function 11 (8):6745–76. doi: 10.1039/D0FO01237H.
- Siomkajło, M., and J. Daroszewski. 2019. Branched chain amino acids: Passive biomarkers or the key to the pathogenesis of cardiometabolic diseases? Advances in Clinical and Experimental Medicine 28 (9):1263–9. doi: 10.17219/acem/104542.
- Smith, J. G., and R. E. Gerszten. 2017. Emerging affinity-based proteomic technologies for large-scale plasma profiling in cardiovascular disease. Circulation 135 (17):1651–64. doi: 10.1161/CIRCULATIONAHA.116.025446.
- Soldatovic, I., R. Vukovic, D. Culafic, M. Gajic, and V. Dimitrijevic-Sreckovic. 2016. SiMS Score: Simple method for quantifying metabolic syndrome. Plos ONE 11 (1):e0146143. doi: 10.1371/journal.pone.0146143.
- Sookoian, S., and C. J. Pirola. 2012. Alanine and aspartate aminotransferase and glutamine-cycling pathway: Their roles in pathogenesis of metabolic syndrome. World Journal of Gastroenterology 18 (29):3775–81. doi: 10.3748/wjg.v18.i29.3775.
- Southward, K., K. Rutherfurd-Markwick, C. Badenhorst, and A. Ali. 2018. The role of genetics in moderating the inter-individual differences in the ergogenicity of caffeine. Nutrients 10 (10):1352. doi: 10.3390/nu10101352.
- Speliotes, E. K., C. J. Willer, S. I. Berndt, K. L. Monda, G. Thorleifsson, A. U. Jackson, H. Lango Allen, C. M. Lindgren, J. Luan, R. Mägi, et al. 2010. Association analyses of 249,796 individuals reveal 18 new loci associated with body mass index. Nature Genetics 42 (11):937–48. doi: 10.1038/ng.686.
- Sprague, A. H., and R. A. Khalil. 2009. Inflammatory cytokines in vascular dysfunction and vascular disease. Biochemical Pharmacology 78 (6):539–52. doi: 10.1016/j.bcp.2009.04.029.
- Stradomska, T. J., M. Syczewska, E. Jamroz, A. Pleskaczyńska, P. Kruczek, E. Ciara, and A. Tylki-Szymanska. 2020. Serum very long-chain fatty acids (VLCFA) levels as predictive biomarkers of diseases severity and probability of survival in peroxisomal disorders. PloS One 15 (9):e0238796. doi: 10.1371/JOURNAL.PONE.0238796.
- Suárez, M., N. Boqué, J. M. Del Bas, J. Mayneris-Perxachs, L. Arola, and A. Caimari. 2017. Mediterranean diet and multi-ingredient-based interventions for the management of non-alcoholic fatty liver disease. Nutrients 9 (10):1052. doi: 10.3390/nu9101052.
- Suleiman, J., M. Mohamed, and A. Bakar. 2020. A systematic review on different models of inducing obesity in animals: Advantages and limitations. Journal of Advanced Veterinary and Animal Research 7 (1):103–14. doi: 10.5455/javar.2020.g399.
- Sun, B. B., J. C. Maranville, J. E. Peters, D. Stacey, J. R. Staley, J. Blackshaw, S. Burgess, T. Jiang, E. Paige, P. Surendran, et al. 2018. Genomic atlas of the human plasma proteome. Nature 558 (7708):73–9. doi: 10.1038/s41586-018-0175-2.
- Surendran, A., N. Atefi, H. Zhang, M. Aliani, and A. Ravandi. 2021. Defining acute coronary syndrome through metabolomics. Metabolites 11 (10):685. doi: 10.3390/metabo11100685.
- Sutton, A., A. Imbert, A. Igoudjil, V. Descatoire, S. Cazanave, D. Pessayre, and F. Degoul. 2005. The manganese superoxide dismutase Ala16Val dimorphism modulates both mitochondrial import and MRNA stability. Pharmacogenetics and Genomics 15 (5):311–9. doi: 10.1097/01213011-200505000-00006.
- ‘t Hart, L. M., N. Vogelzangs, D. O. Mook-Kanamori, A. Brahimaj, J. Nano, A. A. W. A. van der Heijden, K. Willems van Dijk, R. C. Slieker, E. W. Steyerberg, M. A. Ikram, et al. 2018. Blood metabolomic measures associate with present and future glycemic control in type 2 diabetes. The Journal of Clinical Endocrinology and Metabolism 103 (12):4569–79. doi: 10.1210/jc.2018-01165.
- Tabassum, R., J. T. Rämö, P. Ripatti, J. T. Koskela, M. Kurki, J. Karjalainen, P. Palta, S. Hassan, J. Nunez-Fontarnau, T. T. J. Kiiskinen, et al. 2019. Genetic architecture of human plasma lipidome and its link to cardiovascular disease. Nature Communications 10 (1):4329. doi: 10.1038/s41467-019-11954-8.
- Tebani, A., and S. Bekri. 2019. Paving the way to precision nutrition through metabolomics. Frontiers in Nutrition 6:41. doi: 10.3389/fnut.2019.00041.
- The Lancet. 2009. What is health? The ability to adapt. Lancet (London, England) 373 (9666):781. doi: 10.1016/S0140-6736(09)60456-6.
- Thorleifsson, G., G. B. Walters, D. F. Gudbjartsson, V. Steinthorsdottir, P. Sulem, A. Helgadottir, U. Styrkarsdottir, S. Gretarsdottir, S. Thorlacius, I. Jonsdottir, et al. 2009. Genome-wide association yields new sequence variants at seven loci that associate with measures of obesity. Nature Genetics 41 (1):18–24. doi: 10.1038/ng.274.
- Tin, A., P. Balakrishnan, T. H. Beaty, E. Boerwinkle, R. C. Hoogeveen, J. H. Young, and W. H. Kao. 2016. GCKR and PPP1R3B identified as genome-wide significant loci for plasma lactate: The atherosclerosis risk in communities (ARIC) study. Diabetic Medicine: A Journal of the British Diabetic Association 33 (7):968–75. doi: 10.1111/dme.12971.
- Tintle, N. L., J. V. Pottala, S. Lacey, V. Ramachandran, J. Westra, A. Rogers, J. Clark, B. Olthoff, M. Larson, W. Harris, et al. 2015. A genome-wide association study of saturated, mono- and polyunsaturated red blood cell fatty acids in the framingham heart offspring study. Prostaglandins, Leukotrienes, and Essential Fatty Acids 94 (March):65–72. doi: 10.1016/j.plefa.2014.11.007.
- Tulipani, S., M. Palau-Rodriguez, A. Minarro Alonso, F. Cardona, A. Marco-Ramell, B. Zonja, M. Lopez de Alda, A. Munoz-Garach, A. Sanchez-Pla, F. J. Tinahones, et al. 2016. Biomarkers of morbid obesity and prediabetes by metabolomic profiling of human discordant phenotypes. Clinica Chimica Acta; International Journal of Clinical Chemistry 463 (December):53–61. doi: 10.1016/j.cca.2016.10.005.
- Turck, D., J. Castenmiller, S. De Henauw, K. I. Hirsch-Ernst, J. Kearney, H. K. Knutsen, A. Maciuk, I. Mangelsdorf, H. J. McArdle, A. Naska, et al. 2018. Guidance on the scientific requirements for health claims related to muscle function and physical performance: (Revision 1). EFSA Journal 16 (10): 5434. doi: 10.2903/j.efsa.2018.5434.
- Turck, D., J. L. Bresson, B. Burlingame, T. Dean, S. Fairweather-Tait, M. Heinonen, K. I. Hirsch-Ernst, I. Mangelsdorf, H. J. McArdle, A. Naska, et al. 2018. Guidance for the scientific requirements for health claims related to antioxidants, oxidative damage and cardiovascular health: (revision 1). EFSA Journal 16 (1):. 136 10.2903/J.EFSA.2018.5136.
- Udler, M. S., M. I. McCarthy, J. C. Florez, and A. Mahajan. 2019. Genetic risk scores for diabetes diagnosis and precision medicine. Endocrine Reviews 40 (6):1500–20. doi: 10.1210/er.2019-00088.
- Ulaszewska, M., N. Vázquez-Manjarrez, M. Garcia-Aloy, R. Llorach, F. Mattivi, L. O. Dragsted, G. Praticò, and C. Manach. 2018. Food intake biomarkers for apple, pear, and stone fruit. Genes & Nutrition 13 (1):29. doi: 10.1186/s12263-018-0620-8.
- Use of Glycated Haemoglobin (HbA1c) in the Diagnosis of Diabetes Mellitus Abbreviated Report of a WHO Consultation. 2011.
- van der Aa, M. P., C. A. J. Knibbe, A. D. Boer, and M. M. J. van der Vorst. 2017. Definition of insulin resistance affects prevalence rate in pediatric patients: A systematic review and call for consensus. Journal of Pediatric Endocrinology & Metabolism: JPEM 30 (2):123–31. doi: 10.1515/jpem-2016-0242.
- van der Hoek, M. D., A. G. Nieuwenhuizen, O. Kuda, P. Bos, V. Paluchová, L. Verschuren, A. M. van den Hoek, R. Kleemann, N. J. G. M. Veeger, F. R. van der Leij, et al. 2020. Intramuscular short-chain acylcarnitines in elderly people are decreased in (pre-)frail females, but not in males. FASEB Journal: Official Publication of the Federation of American Societies for Experimental Biology 34 (9):11658–71. doi: 10.1096/fj.202000493R.
- van Heel, D. A., L. Franke, K. A. Hunt, R. Gwilliam, A. Zhernakova, M. Inouye, M. C. Wapenaar, M. C. N. M. Barnardo, G. Bethel, G. K. T. Holmes, et al. 2007. A genome-wide association study for celiac disease identifies risk variants in the region harboring IL2 and IL21. Nature Genetics 39 (7):827–9. doi: 10.1038/ng2058.
- van Meurs, J. B. J., G. Pare, S. M. Schwartz, A. Hazra, T. Tanaka, S. H. Vermeulen, I. Cotlarciuc, X. Yuan, A. Mälarstig, S. Bandinelli, et al. 2013. Common genetic loci influencing plasma homocysteine concentrations and their effect on risk of coronary artery disease. The American Journal of Clinical Nutrition 98 (3):668–76. doi: 10.3945/ajcn.112.044545.
- van Ommen, B., J. Keijer, S. G. Heil, and J. Kaput. 2009. Challenging homeostasis to define biomarkers for nutrition related health. Molecular Nutrition & Food Research 53 (7):795–804. doi: 10.1002/mnfr.200800390.
- van Ommen, B., J. Keijer, R. Kleemann, R. Elliott, C. A. Drevon, H. McArdle, M. Gibney, and M. Müller. 2008. The challenges for molecular nutrition research 2: Quantification of the nutritional phenotype. Genes & Nutrition 3 (2):51–9. doi: 10.1007/s12263-008-0084-3.
- van Ommen, B., J. van der Greef, J. M. Ordovas, and H. Daniel. 2014. Phenotypic flexibility as key factor in the human nutrition and health relationship. Genes & Nutrition 9 (5):423. doi: 10.1007/s12263-014-0423-5.
- Van’t Erve, T. J. 2018. Strategies to decrease oxidative stress biomarker levels in human medical conditions: A meta-analysis on 8-iso-prostaglandin F2α. Redox Biology 17 (July):284–96. doi: 10.1016/j.redox.2018.05.003.
- Vashist, S. K. 2021. Trends in multiplex immunoassays for in vitro diagnostics and point-of-care testing. Diagnostics 11 (9):1630. doi: 10.3390/diagnostics11091630.
- Vega-López, S., L. M. Ausman, J. L. Griffith, and A. H. Lichtenstein. 2007. Interindividual variability and intra-individual reproducibility of glycemic index values for commercial white bread. Diabetes Care 30 (6):1412–7. doi: 10.2337/dc06-1598.
- Wang, Y., Y. Tang, Y. Ji, W. Xu, N. Ullah, H. Yu, Y. Wu, and L. Xie. 2021. Association between FADS1 Rs174547 and levels of long-chain PUFA: A meta-analysis. The British Journal of Nutrition 126 (8):1121–9. doi: 10.1017/S0007114520005103.
- Wang, Z., W. H. W. Tang, T. O’Connell, E. Garcia, E. J. Jeyarajah, X. S. Li, X. Jia, T. L. Weeks, and S. L. Hazen. 2022. Circulating trimethylamine N-oxide levels following fish or seafood consumption. European Journal of Nutrition 61 (5):2357–64. doi: 10.1007/s00394-022-02803-4.
- Wessel, J., A. Y. Chu, S. M. Willems, S. Wang, H. Yaghootkar, J. A. Brody, M. Dauriz, M.-F. Hivert, S. Raghavan, L. Lipovich, et al. 2015. Low-frequency and rare exome chip variants associate with fasting glucose and type 2 diabetes susceptibility. Nature Communications 6 (January):5897. doi: 10.1038/ncomms6897.
- Weverling-Rijnsburger, A. W., I. J. Jonkers, E. Van Exel, J. Gussekloo, and R. G. Westendorp. 2003. High-density vs low-density lipoprotein cholesterol as the risk factor for coronary artery disease and stroke in old age. Archives of Internal Medicine 163 (13):1549–54. doi: 10.1001/archinte.163.13.1549.
- Willer, C. J., E. K. Speliotes, R. J. F. Loos, S. Li, C. M. Lindgren, I. M. Heid, S. I. Berndt, A. L. Elliott, A. U. Jackson, C. Lamina, et al. 2009. Six new loci associated with body mass index highlight a neuronal influence on body weight regulation. Nature Genetics 41 (1):25. doi: 10.1038/NG.287.
- Willis, S. A., S. J. Bawden, S. Malaikah, J. A. Sargeant, D. J. Stensel, G. P. Aithal, and J. A. King. 2021. The role of hepatic lipid composition in obesity-related metabolic disease. Liver International: Official Journal of the International Association for the Study of the Liver 41 (12):2819–35. doi: 10.1111/LIV.15059.
- Wu, H., and C. M. Ballantyne. 2020. Metabolic inflammation and insulin resistance in obesity. Circulation Research 126 (11):1549–64. doi: 10.1161/CIRCRESAHA.119.315896.
- Wu, J. H. Y., R. N. Lemaitre, A. Manichaikul, W. Guan, T. Tanaka, M. Foy, E. K. Kabagambe, L. Djousse, D. Siscovick, A. M. Fretts, et al. 2013. Genome-wide association study identifies novel loci associated with concentrations of four plasma phospholipid fatty acids in the de novo lipogenesis pathway: Results from the cohorts for heart and aging research in genomic epidemiology (CHARGE) Consortiu. Circulation. Cardiovascular Genetics 6 (2):171–83. doi: 10.1161/CIRCGENETICS.112.964619.
- Würtz, P., M. Tiainen, V.-P. Mäkinen, A. J. Kangas, P. Soininen, J. Saltevo, S. Keinänen-Kiukaanniemi, P. Mäntyselkä, T. Lehtimäki, M. Laakso, et al. 2012. Circulating metabolite predictors of glycemia in middle-aged men and women. Diabetes Care 35 (8):1749–56. doi: 10.2337/dc11-1838.
- Xu, B., J. Fu, Y. Qiao, J. Cao, E. C. Deehan, Z. Li, M. Jin, X. Wang, and Y. Wang. 2021. Higher intake of microbiota-accessible carbohydrates and improved cardiometabolic risk factors: A meta-analysis and umbrella review of dietary management in patients with type 2 diabetes. The American Journal of Clinical Nutrition 113 (6):1515–30. doi: 10.1093/AJCN/NQAA435.
- Yao, M. E., P. D. Liao, X. J. Zhao, and L. Wang. 2020. Trimethylamine-N-oxide has prognostic value in coronary heart disease: A meta-analysis and dose-response analysis. BMC Cardiovascular Disorders 20 (1):7. doi: 10.1186/s12872-019-01310-5.
- Yin, X., L. S. Chan, D. Bose, A. U. Jackson, P. VandeHaar, A. E. Locke, C. Fuchsberger, H. M. Stringham, R. Welch, K. Yu, et al. 2022. Genome-wide association studies of metabolites in finnish men identify disease-relevant loci. Nature Communications 13 (1):1644. doi: 10.1038/s41467-022-29143-5.
- Zeng, Y., M. Guo, X. Fang, F. Teng, X. Tan, X. Li, M. Wang, Y. Long, and Y. Xu. 2021. Gut Microbiota-derived trimethylamine N-oxide and kidney function: A systematic review and meta-analysis. Advances in Nutrition (Bethesda, Md.) 12 (4):1286–304. doi: 10.1093/advances/nmab010.
- Zhang, S., P. Wu, Y. Tian, B. Liu, L. Huang, Z. Liu, N. Lin, N. Xu, Y. Ruan, Z. Zhang, et al. 2021. Gut Microbiota serves a predictable outcome of short-term low-carbohydrate diet (LCD) intervention for patients with obesity. Microbiology Spectrum 9 (2):e0022321. doi: 10.1128/Spectrum.00223-21.
- Zhang, X., C. Zhang, L. Chen, X. Han, and L. Ji. 2014. Human serum acylcarnitine profiles in different glucose tolerance states. Diabetes Research and Clinical Practice 104 (3):376–82. doi: 10.1016/j.diabres.2014.04.013.
- Zhao, X., X. Gang, Y. Liu, C. Sun, Q. Han, and G. Wang. 2016. Using metabolomic profiles as biomarkers for insulin resistance in childhood obesity: A systematic review. Journal of Diabetes Research 2016:1–12. doi: 10.1155/2016/8160545.
- Zock, P. L., W. A. M. Blom, J. A. Nettleton, and G. Hornstra. 2016. Progressing insights into the role of dietary fats in the prevention of cardiovascular disease. Current Cardiology Reports 18 (11): 111. doi: 10.1007/s11886-016-0793-y.