ABSTRACT
Police departments around the world implement algorithmic systems to enhance various policing tasks. Ensuring such innovations take place responsibly – with public values upheld – is essential for public organisations. This paper analyses how public values are safeguarded in the case of MONOcam, an algorithmic camera system designed and used by the Netherlands police. The system employs artificial intelligence to detect whether car drivers are holding a mobile device. MONOcam can be considered a good example of value-sensitive design; many measures were taken to safeguard public values in this algorithmic system. In pursuit of responsible implementation of algorithms, most calls and literature focus on such value-sensitive design. Less attention is paid to what happens beyond design. Building on 120+ hours of ethnographic observations as well as informal conversations and three semi-structured interviews, this research shows that public values deemed safeguarded in design are re-negotiated as the system is implemented and used in practice. These findings led to direct impact, as MONOcam was improved in response. This paper thus highlights that algorithmic system design is often based on an ideal world, but it is in the complexities and fuzzy realities of everyday professional routines and sociomaterial reality that these systems are enacted, and public values are renegotiated in the use of algorithms. While value-sensitive design is important, this paper shows that it offers no guarantees for safeguarding public values in practice.
1. Introduction
Police departments across the globe increasingly implement algorithms to support law enforcement and enhance efficiency (Bennett Moses and Chan Citation2018, Hendrix et al. Citation2019, Ozbaran and Tasgin Citation2019, Brayne Citation2020, Kuziemski and Misuraca Citation2020, Ratcliffe et al. Citation2020). The Netherlands police are no exception (Waardenburg et al. Citation2018, Meijer et al. Citation2021, Schuilenburg and Soudijn Citation2023, Wessels Citation2023). Much academic work on algorithms in police settings focuses on predictive policing models, while only limited attention is paid to other applications. This paper investigates one of these other applications, specifically a camera system to support law enforcement by traffic police.
Dutch National law prohibits holding a mobile device while driving.Footnote1 Such distraction is considered one of the main causes of traffic accidents in the Netherlands. The fine is one of the highest for traffic offences in the Netherlands. Traffic police have long had difficulty enforcing this law. Available methods were inefficient; offenders had to be halted and fined manually, so large-scale controls cost a lot of valuable policing time and resources. As early as August 2017, the Netherlands police considered ways to increase efficiency and effectiveness using electronic means to enforce this law. Their efforts resulted in MONOcam, an algorithmic camera system using artificial intelligence to detect mobile phone usage in cars. Similar camera systems are increasingly tested and adopted in international police contexts, and the Dutch MONOcam system was shared with German police.Footnote2
By July 2021 MONOcam was officially rolled-out to all traffic police units in the Netherlands. ArthurFootnote3, my main contact at the Netherlands Police for this research, stood at the centre of these developments. He was part of the team that developed the software, algorithms, and the camera prototype. They ran the pilot and continued to develop MONOcam until it was ready for implementation. Even now that another team is officially responsible, Arthur is still involved in fine-tuning the system, and is regarded as an expert on MONOcam within the Netherlands Police. I first met Arthur in November 2021, determined to pitch MONOcam as a research case for my dissertation. My goal was to get access to investigate how patrol officers managed algorithmic systems and whether the algorithm was implemented responsibly. The Netherlands Police decided to grant me the required access to MONOcam developers and users as part of their commitment to responsible innovation.
Responsible implementation of algorithms can be achieved through value-sensitive design, an approach where the values of various direct and indirect stakeholders are taken into account in the design of a technology (Friedman et al. Citation2008, Riebe et al. Citation2023). Rather than stakeholder values, for public organisations such as the Netherlands Police, it can be argued that public values should take centre stage (Meijer et al. Citation2021, Meijer and Grimmelikhuijsen Citation2021). Public values are those qualities that are important in public governance, such as non-discrimination, privacy, inclusivity or preventing harm. They contribute to, or are essential for, ‘good’ public governance, specifying citizens’ rights and obligations as well as the principles on which we should base governments and policies. These values are often anchored in ‘good governance’ codes and ethical frameworks (Beck Jørgensen and Sørensen Citation2012, de Graaf et al. Citation2014, Beck Jørgensen and Bozeman Citation2007). As straightforward as this seems in theory – after all, nobody disagrees directly with most public values – practice is more complex. Public values depend on societal dynamics. People, cultures, or societies may consider different values to be relevant in a spatiotemporal context, and for different reasons.
Values also depend on technological developments, and different technologies bring out different sets of values (Friedman et al. Citation2008). Further, public values are often incompatible or incommensurable, some being inherently conflicting. When different relevant values are found to be conflicting, choices must be made to pursue one at the expense of another. This weighing of public values is a common and core responsibility in all layers of public professions, but it often happens implicitly (de Graaf et al. Citation2014, de Graaf and Meijer Citation2019). In this paper, I rely on CODIO, a code for good digital public governance serving as an appendix to the Dutch good governance code. CODIO focuses on the question in what ways governments and government organisations should be using digital technologies and how they can negotiate public values that warrant their use. The framework identifies three fundaments derived from the Dutch good governance code; democracy, rule of law, and administrative power. A total of 30 relevant public values is listedFootnote4 for each fundament (Meijer and Ruijer Citation2021).
I use the CODIO framework as a sensitising point of departure to investigate public values in the MONOcam algorithmic system. The intertwinement of policing, data and technology is nothing new (Chan, Citation2001, Manning Citation2008, Stol and Strikwerda Citation2017, Hendrix et al. Citation2019, Brayne Citation2020, Riebe et al. Citation2023), and simple algorithmic systems like automated number plate readers (ANPR) have been around since the early 2000s (Hendrix et al. Citation2019, Lum et al. Citation2019a). However, the introduction of more advanced algorithmic technologies like MONOcam is relatively recent (Ozbaran and Tasgin Citation2019). Such technologies have been found to transform police organisations and policing in unexpected ways (Waardenburg et al. Citation2018, Terpstra et al. Citation2019, Lum et al. Citation2019b, Brayne Citation2020, Willis et al. Citation2020, Guzik et al. Citation2021, Lorenz et al. Citation2021, Meijer et al. Citation2021, Meijer and Thaens Citation2021).
Many scholars have demonstrated that algorithmic technologies are value-laden (Gillespie Citation2014, Ananny Citation2016, Kitchin Citation2017, Bennett Moses and Chan Citation2018). Algorithmic systems have been found capable of (re)producing bias and discriminatory practices as well as inflicting harm, thus having serious real-world consequences (Stahl and Wright Citation2018, Meijer et al. Citation2019, Wieringa Citation2020, Meijer and Grimmelikhuijsen Citation2021, Wessels Citation2023). Camera systems in particular are often found to be invasive and are subject of societal concerns (e.g. Glancy Citation2004, Snijders et al. Citation2019), whilst there is no consensus whether algorithmic cameras are effective in deterring unwanted behaviour (Lum et al. Citation2011, Taylor et al. Citation2012, Ozbaran and Tasgin Citation2019). I had thus prepared for my meeting with Arthur assuming I would find similar patterns in MONOcam. After all, I was trained to look for public values and value-weighing – patterns that often come to light as ‘risks’.
I left the meeting disappointed and thoroughly impressed: good practice after good practice came to light as Arthur told me about designing MONOcam. Not only had they performed a Data Protection Impact Assessment (DPIA), which was not mandatory or expected at the time, they had submitted this DPIA to the Dutch Data Protection Agency before collecting citizen data and training the algorithms. Training data were collected in a large variety of situations (e.g. weather conditions). When COVID-19 suddenly hit, the models were tested with new data of people wearing facemasks in the car.Footnote5 MONOcam came equipped with built-in human final control, using a four-eyes principle.Footnote6 Data were transferred securely, and photos of non-offenders were immediately deleted. Passengers and other cars not related to the offender were made unidentifiable by deleting pixels. The design-and-testing team had even organised a session with the Public Prosecution Service to determine what makes a ‘hit’, and criteria for this were added in KIOSK, an interactive manual built into the software. What struck me most, was that they had separated tenders for the camera design and its production. This separation ensures police ownership over the design and decreasing dependency on the commercial company building the camera and doing maintenance. The software and algorithms, as well as the laptops are owned and maintained by the Netherlands Police.
MONOcam can be considered a best-practice when it comes to value-sensitive design in the Netherlands Police. As worded by a data protection officer in an e-mail (2019, after the DPIA had been pre-approved): ‘Congratulations with this result!! As far as I know, you’re the first to use a preliminary assessment, and with a positive outcome.’ I was left wondering whether this would be an interesting case for my research. In pursuit of responsible implementation of algorithms, most calls and literature focus on exactly the kind of value-sensitive design showcased in MONOcam. However, much less attention is paid to what happens beyond design, after a system is implemented and used in an organisation. Here, a grey area emerges, where it is not clear what happens to those public values deemed safeguarded in design.
Despite increasing calls to study the grey area between design and implementation in professional routines (e.g. Christin Citation2017, Kitchin Citation2017), research in this area remains limited. In this paper, I set out to map this grey area for MONOcam, and to uncover the role of public values in the MONOcam algorithmic system in use. In doing so, this paper adds to existing literature and practices of responsible algorithmisation (Meijer and Grimmelikhuijsen Citation2021) the insight that understanding system design intentions is not sufficient, actual use needs to be considered as well.
This paper adds to policing scholarship on algorithms and AI, where the majority of research in this area has focused on predictive policing applications (Bennett Moses and Chan Citation2018, Brayne Citation2020, Lorenz et al. Citation2021) Whilst some research on algorithmic camera systems has been conducted in the context of traffic policing, this has focused mostly on number plate recognition (Hendrix et al. Citation2019, Ozbaran and Tasgin Citation2019, Lum et al. Citation2019a). I am not aware of any research on a system similar to MONOcam. In fact this use of AI-driven cameras appears mostly unchallenged as it is increasingly adopted internationally. This is particularly problematic as camera systems are known to be relatively invasive (Glancy Citation2004, Snijders et al. Citation2019). As police forces continue to innovate, it is important to conduct critical research. The current study shows that research in this area needs to take into account the design as well as phases beyond design, when such technologies are implemented and used in practice The ethnographic approach taken in this research may prove fruitful for other police researchers working on similar topics.
Beyond these academic contributions, the paper provides insights for policing practice. The Dutch MONOcam system was adapted in response to these findings, and officers were given extra instruction. While the findings are specific to the Dutch policing context, it is plausible similar patterns emerge in other contexts of use. As AI-driven camera systems are increasingly used across the globe to enforce road safety, the paper offers practical insight into the risks and advantages of such systems as they are implemented and used in practice. It also sketches some cautionary measures police forces may explore to safeguard public values beyond design.
I posit that responsible implementation of algorithmic systems cannot be achieved through value-sensitive design alone. It is in the complexities and fuzzy realities of everyday policing that MONOcam’s real value becomes manifest.
1.1. Method
It is argued that algorithms, are ‘(…) inert, meaningless machines (…)’ (Gillespie Citation2014) until they are regarded in relation to their sociomaterial contexts (Orlikowski and Scott Citation2015, Citation2008, Kitchin Citation2017, Wieringa Citation2020). A key conviction in sociomateriality is that the social and the material are inseparable, rather than just being interconnected. Algorithmic systems, then, are enacted through practices that are both deeply social and material (Mol Citation2002, Orlikowski and Scott Citation2008, Leonardi Citation2011, Introna Citation2016, Seaver Citation2017). According to this strand of literature, algorithms have no determinate boundaries, properties or meanings prior to their incorporation and enactment in specific sociomaterial practices (Orlikowski and Scott Citation2015, Introna Citation2016, Bucher Citation2018). Following Wieringa, we speak of algorithmic systems rather than algorithms to include not only the technical but also the sociomaterial (Wieringa Citation2020).
To study MONOcam as an algorithmic system in use, I thus needed to take into account this sociomaterial nature of the system. I found ethnography to be a particularly useful method, as it allows to focus on situated practices of MONOcam patrol officers in contrast to the intended practices as conceptualised during MONOcam design (Schwartz-Shea and Yanow Citation2012, Kitchin Citation2017, Van Hulst Citation2020). ‘Following’ the MONOcam algorithmic system allowed investigation of the interconnected sociomaterial aspects that converge in the enactments of MONOcam beyond design (Seaver, Citation2017, Christin Citation2020, Citation2017). In line with these research traditions and methodologies, please note that my observations cannot be regarded as completely separate from myself as a researcher. Rather than presenting my research as neutral and objective, I have continuously reflected on my role and influence on my research findings.
My insights are grounded in combined ethnographic data from (a) in-the-field observations with MONOcam patrol officers across the Netherlands, (b) observations of presentations and demonstrations of MONOcam and (c) informal conversations and semi-structured interviews with people involved with the design, development, implementation and management of MONOcam. Whilst the Netherlands Police is a national organisation, responsibilities for enforcing traffic laws are shared between ten regional traffic teams and one national traffic team. There is much variation between teams. Some focus on a single city environment while others encompass up to three provinces. Each team owns at least one MONOcam system, with some of the larger teams having two systems available to them. Planning and organisation practices also vary, e.g. in some teams a single officer conducts a MONOcam control, while at others controls take place in pairs. In some teams, patrol officers have free reign of the location they set up MONOcam while in other teams they are ordered to go to a specific spot. There is also variation in the patrol officer population, some patrol officers have successfully completed police training while others did not receive such training. All patrol officers have taken an oath of office and are held to laws and the professional code of the Netherlands Police.
The observational data comprised over 120 h collected at 10 out of 11 MONOcam teams. Observations were turned into written fieldnotes, and the semi-structured interviews (three total) were transcribed verbatim. Throughout the research, I integrated initial findings with ongoing fieldwork, discussing findings and enhancing interactions during the observations. This iterative process allowed me to refine my argumentation whilst conducting observations. As part of this iterative approach, I conducted multiple rounds of open coding in NVIVO, but the majority of the selective coding process was conducted on paper. All research data were pseudonymised, the names in this article are fictional. Data were gathered between November 2021 and September 2022. Approval to conduct interviews and observations was granted by the Ethical Committee of Utrecht University (FETC-REBO ‘Value-sensitive algorithmization in the Netherlands Police’). When observing or interacting with participants, intentions, affiliation and research interest were clearly stated. Data were stored on YODA, a secure research data management service provided by Utrecht University.
In the remainder of this article, I take readers along on my observations sharing stories from the field (Van Hulst Citation2020). In each of the following sections, we uncover sociomaterial enactments of MONOcam in practice in which different public values are presented, weighed, or found to be conflicting. Sociomateriality will be an inevitable point of departure in each of the stories, any distinction between the two is made for purely analytical purposes. Section 2 introduces the MONOcam system, section 3 focuses on material aspects, section 4 introduces emergent functions of the algorithmic system and section 5 focuses on human discretion in the organisational context. Due to the situational nature of this research, these results are neither completely representative nor exhaustive, but they do show at least some ways in which the values of MONOcam in use differ from its design.
2. MONOcam set-up
The MONOcam system is mobile. It can be set up over an overpass in 15 min or less (see ). MONOcam consists of several hardware components, all with their own specifications and limitations. A camera is mounted on a pan-tilt unit for computerised control of camera movement, both of which are set up on a tripod. The tripod is manually adjusted by the patrol officer on site to align the camera properly. Data and power are transferred from the camera setup via a power over ethernet (PoE) cable which is 50 m long. This cable connects to a laptop in a police vehicle. The laptop is supervised by the patrol officer and runs the MONOcam software.
Figure 1. (left) A patrol officer setting up the MONOcam camera on a highway overpass. (right) MONOcam looking out over a highway overpass at incoming cars.
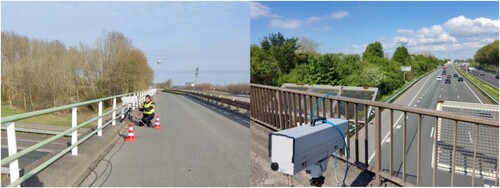
The software includes a total of five deep-learning models that locate and read licence plates, locate windscreens and detect drivers’ mobile phone use. Deep learning is a type of artificial intelligence that imitates neural networks to learn. These models function out of sight of the patrol officer, the laptop only shows a user interface (). The user interface allows the police officer on-site to adjust camera settings. As the camera system is running, photographs of all passing vehicles are shown in the bottom right corner. Showing these is necessary to determine photo quality and adjust settings accordingly.
Figure 2. Screenshot of the MONOcam software interface as shown on the laptop. The top left shows a livestream of incoming video, the top right shows the photographed car, with evidence of movement. The bottom right shows photographs of all passing vehicles. Potential hits are added to the list on the bottom left for further manual inspection by the patrol officer. Non-hits are deleted.
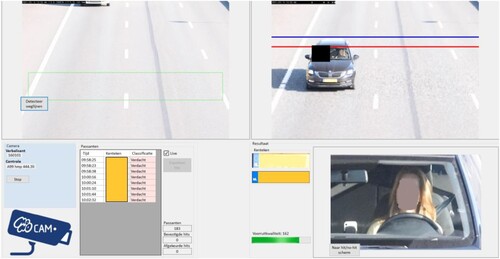
Photographs labelled as potential ‘hits’ by the system are listed. As a police officer clicks one of these hits, they are shown the photograph of the driver, a photograph of the licence plate and the licence plate as it has been ‘read’ by the software. The patrol officer must manually determine whether the photograph shows an offence, and whether the licence plate has been ‘read’ correctly. Rejected photographs are deleted, while accepted photographs are kept in the list as ‘confirmed hits’. Before closing the software, the patrol officer must judge all remaining potential hits and export them through a secured API gateway to the ‘DigiBon’ software for processing police reports.
Within DigiBon, the patrol officer manually assigns all confirmed hits to a colleague, taking at least seven mouse clicks per hit. The colleague rechecks all hits, and rejects any that do not conform to their standards. Sometimes both patrol officers discuss their interpretations of the photograph to reach consensus. All hits that are confirmed by both officers are processed into police reports by the first police officer. Most text in these reports is automatically generated using embedded data, but the officer may add or change things. Completed reports are sent to the Central Judicial Collection Agency (CJIB: Centraal Justitieel Incassobureau), which is part of the Ministry of Justice and Security in the Netherlands. Fines are processed by and paid to the CJIB directly. Despite falling under the same ministry, this is a separate organisation from the Netherlands Police. The two patrol officers retain access to the report and photograph in DigiBon until the data are deleted conforming to privacy law.
3. On windscreens, weather and wipers
MONOcam has a difficult job. It is to take a photo of a reflective object – a windscreen – that is moving at high speed. While the system manages to take 8 photos per second, the quality of these photos is not always guaranteed. If quality was found to be lacking, the patrol officer was unable to judge the photograph.
Early into my fieldwork, I noticed that some cars showed up with blue, purple or orange-coloured windshields, as can be seen in . I remember one session on a sunny afternoon where there seemed to be particularly many such cars. Geert, the police officer next to me, explains ‘these are coated windows’. He explains that expensive brands often add heat treatment in fabrication, which causes the colouration on our photographs. We made a ‘game’ out of spotting such cars for the rest of that session. ‘BMW!’, ‘Mercedes!’, ‘Was that an Alfa Romeo?’ No, that was a Porsche. Quite a few Teslas as well. Despite making this specific session that much more entertaining, more often than not, colourful windscreens make for useless MONOcam photographs. Even in cases where drivers were likely using their phones, the photo was often not clear enough to fine them. In this case, a very material aspect (heat treatment on windscreen) directly impacts public values such as fairness, non-discrimination and equal treatment. I should note that not every luxury car brand, not every Tesla, Porsche etc. showed this distortion.
Figure 3. Example of a MONOcam photo taken of a car with a coated windscreen. This particular car is an older model of the BMW-7 series, the most luxury sedan the brand produced at that time. The purple-and-blue glare partially obscures the driver in this photograph.
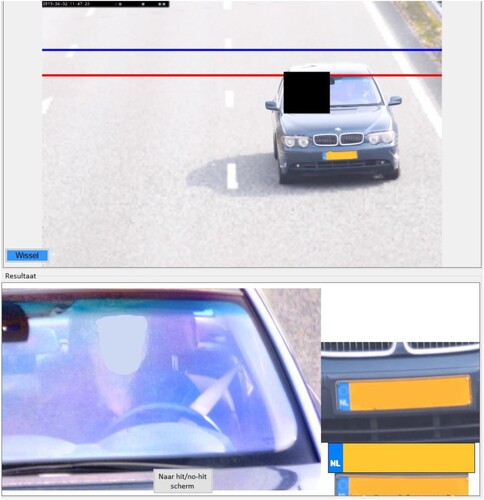
As noted above, it was a sunny day. Good weather conditions are key to obtaining high-quality photographs. Specific weather conditions can be considered material factors, as something need not have a physical form to be considered material (Moura and Bispo Citation2020). Because MONOcam has to make photographs of reflective objects, its functioning is highly dependent on the strength and direction of (sun)light. ‘Good MONOcam-weather’ is a phrase frequently used by traffic police officers throughout the country.
In fact, many officers did not even bother going out with the system if the weather was not ‘agreeable’ to the system. My MONOcam session with Jarno, for example, had to be rescheduled due to snowfall. Too much sunlight could cause reflections or underlit photo’s if it was not coming from the right direction. Lack of sunlight made the image very grainy and unrecognisable. Rain meant potential issues with autofocus, and extra work for the patrol officer on site as the wipers were sometimes recognised by the system as phones. MONOcam, thus, lies dormant for a significant part of the year.
In the grey area beyond design, we thus find external material aspects to affect the functioning of the MONOcam algorithmic system. Weather conditions have a significant impact on photo quality, posing implicit risks to fairness and equal treatment since drivers using their phones in the rain have a more limited chance of being fined. Weather conditions further impact the efficiency and effectiveness of the MONOcam system, which is unusable for a substantial portion of the year. Similarly, windscreen shape, angle and treatment also impact photo quality, posing the same implicit risks to fairness and equal treatment; the type of car you drive influences your chances of being fined. While these material aspects were already considered in the design, e.g. by including various weather conditions in the training data, some effects could only be detected after implementation. It is in daily routine practices, beyond the design team’s conceptualisations or the technical algorithm, where these material aspects significantly impact the algorithmic system.
4. A multiplicity of functions
MONOcam observations were rather uncomfortable. Long hours sitting in a van, watching a computer screen with no opportunity for bathroom breaks (). Of course, I had company, a luxury most MONOcam patrol officers don’t have. While some officers use this time to catch up on administrative work or watch movies, occasionally working through the list of potential hits, others found ways to entertain themselves with the system.
Figure 4. MONOcam setup inside a police vehicle. The bottom right of the laptop screen displays all incoming photographs made by the system.
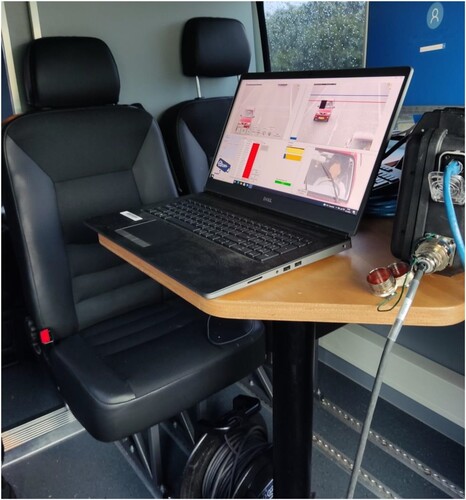
Particularly entertaining was the constant stream of incoming photos, designed for inspecting photo quality and adjusting settings as needed. Drivers pass by unassumingly, reduced to a small square in the bottom right corner of the laptop screen. MONOcam patrol officers make up stories about these drivers, who can be grouped into categories. There are drivers who point at the camera, sing and dance in their car or seem disturbingly sleepy. These types appear in almost every MONOcam session. Pointing them out has become a fun game to some patrol officers. Some drivers seemingly take pictures of the camera stationed on the highway overpass (resulting in a fine, much to the officers’ amusement) or give middle fingers. Sometimes citizen-drivers take a more positive approach to the patrol set-up by giving a thumbs up. There are many ‘very charming’ photographs as well, e.g. people picking their noses. In a way, MONOcam offers its patrol officers a gateway into the cars on the road underneath them. The small square in the lower right-hand corner of that laptop screen in their van becomes an officer’s connection to a whole new world.
Entertainment can also be found in recognising specific drivers. During our conversation, Aad suddenly proclaims ‘Hey! That’s my auntie Anna!’ Upon asking, he tells me this is no unique occurrence; he has seen other family members and colleagues through MONOcam. Do they also use their phones? ‘No, of couuuurse not’, Aad laughs. His auntie Anna would never do such a thing. His colleagues, on the other hand, turn MONOcam into a game. If they know where Aad is located, they purposely pass by MONOcam, making silly faces. Aad shows me a picture on his phone.
Finding entertainment in these working conditions is understandable. Working with MONOcam gets boring and repetitive, I found entertainment helped me stay alert and engaged with the system. It could have similar positive effects for patrol officers, potentially increasing their performance. Only when entertainment begins taking precedence over other values may it become a cause of concern. In the example above, the patrol officer took a photo of the system with his mobile phone, therefore taking data collected by MONOcam out of its secure and private environment, for entertainment purposes. In this case, the drivers involved were colleagues, but during my observations I have come across instances where non-offending drivers were recognised. For instance, when a MONOcam patrol officer spotted somebody who looked like one of their colleagues in the MONOcam photo. In this case, the photograph of a nonoffending driver was sent to the colleague with WhatsApp. While the phones of police officers are secure, this is not necessarily the case for photos that have been forwarded, and there is no control on how long photos are saved.Footnote7
Such enactment of MONOcam as an entertainment system could be regarded as function creep, a common term in scholarship about technologies and algorithms. Defined by Koops as ‘(…) an imperceptibly transformative and therewith contestable change in a data-processing system’s proper activity’, function creep denotes a transformation or expansion of technology usage (Koops Citation2021, p. 53). Key to this definition is the way in which such transformation takes place: imperceptibly. As a result, there is no perceived need for discussion about the desirability of the transformations or expansions taking place. In becoming a system of entertainment for patrol officers on duty, MONOcam is functioning in an unexpected way, not anticipated or considered by the designers who conceived it purely as an enforcement system. The lack of discussion about the entertainment properties of MONOcam constitutes a clear risk that an emergent MONOcam in practice may function in undesirable or even unacceptable ways. As we see in the story above, its new function causes a direct risk for privacy and data security.
After our MONOcam session, Huub and I return to the office. I am ready to leave. We have exchanged our goodbyes, and all that is left is for him to escort me to the door, which I cannot open myself. As he begins to stand up, his phone rings, and Huub settles back down on his chair. I follow his example. I can hear a voice asking ‘I understand you have access to the MONOcam photos, is that so?’ Huub confirms. The person explains they would like to see a particular photo. There has been an incident involving a firearm, and a vehicle was involved. The system showed the same vehicle had been fined through MONOcam, and the colleague on the phone would like to know who was driving it. Was it a man or a woman? And was this person the same as the registered owner of the vehicle? Huub asks for some additional data, but quickly finds out he was not personally involved in this MONOcam during his shift so he cannot provide the photograph. He proposes to call back next Sunday – when the colleagues are in. The person on the phone agrees, there’s no rush, they say, and the conversation is concluded. Although this is the only time I experience such a scene, Huub informs me that requests like this come up occasionally, and not just with MONOcam. He considers it a very good development.
In the example above, we see MONOcam enacted not as a system of entertainment, nor as a system of enforcement, but as a system to gather information for investigations. In this case, detective work, an altogether different branch of policing. Some MONOcam patrol officers also use the information gathered by MONOcam for other traffic police tasks. Aad is one such officer. During a MONOcam session he clicks a potential hit. The person in the photo is holding up both middle fingers. Clearly not a hit, but Aad says ‘let me run it’. Unmoved by the crude yet common gesture, Aad tells me he always likes to check whether something else can be found on this person. He takes out his mobile phone and opens MEOS – an application that allows police patrol officers to access information. He enters the licence plate and looks at his screen. ‘No insurance’, he says, and he opens a ticket, adding the MONOcam photo as evidence that this car was spotted on the road. The man can expect a big fine. Aad clicks a few more time in MEOS. ‘Is the driver also the owner?’ he questions out loud. Apparently not, the owner is a woman, but there is a man living at the same address. Aad clicks a few more times. Mugshots of the man are available, but it seems to be someone other than the MONOcam hit. He shows me the mugshots before putting away his phone and clicking ‘no hit’ in the MONOcam software. Although fining drivers for lack of car insurance is part of Aad’s traffic police responsibilities, it lies outside the scope of tasks MONOcam was intended for.
In the grey area beyond design, MONOcam is found to have a multiplicity of functions. Intended to be a system to enforce a specific law (prohibition to drive a vehicle while holding a mobile device), in practice it can be a source of entertainment or information, and the system can be used for other purposes, such as fining drivers for other violations. As these functions beyond those intended in design are not discussed and made public, this constitutes function creep. Emergent ways of using the system in practice might be found to be undesirable or unacceptable, e.g. they could cause issues concerning suitability or violate the juridical principles of proportionality and subsidiarity. In case photo images are taken out of the protected system, e.g. with the use of the mobile phone, we find a risk to privacy and data security; and the lack of discussion about how MONOcam is used expansively in police routine checks could harm the values of explainability and transparency of the system. After all, how can one be open about a system if it is unclear how the system functions in practice?
5. Mind the gap; patrol officer discretion in the absence of infrastructure
During one of my MONOcam sessions I am watching William. Every time a few new potential hits come in, William clicks and judges them. One potential hit, clearly a false positive, catches his interest. ‘It says 2, but there’s something in front of that.. what is that?’ he says. He is talking about the licence plate; something seems to be stuck in front of it. Although William remains unsure of what this is, he states it should be a 1, not a 2. ‘See’, he says, pointing at the screen, ‘there’s a corner of the 1’. After his explanation I can see it too. Satisfied, William clicks the ‘no-hit’ button. ‘I usually also check all the license plates before I close the software’, he says, ‘but now that I’m saying that, I don’t think I have checked any of the other license plates either’. He runs through the lists, but all the licence plates have been read by the system correctly. ‘That really makes you lazy’, William says. ‘It’s only good that the camera makes mistakes sometimes’, he jokes, ‘that means you really have to keep paying attention’.
Contrary to the license plate recognition algorithms, paying attention when judging potential hits in MONOcam does not seem to be a problem for patrol officers. Almost every patrol officer I observed with MONOcam has uttered some variation of the statement ‘when in doubt, throw it out’, a testament to their critical attitude. Patrol officers feel a responsibility to fine only those drivers who are indeed, without any shred of doubt, offenders. The exact meaning of these words, however, varies greatly. I often found myself comparing judgements between one observation and the next. Some MONOcam patrol officers were very strict while others would OK photos in which the supposed ‘phone’ might just as well have been a pen.
At one point, I am given the opportunity to join Johan and Jarno in the field. Johan has recently learned how to work with MONOcam, while Jarno is an experienced user. At one point a photo shows up on the screen, and Johan expresses doubt. ‘That’s a hit’, Jarno replies. A hand is clearly visible, and while the phone is difficult to see, to Jarno the posture and direction of the driver’s gaze are reason enough to label it as a hit. Johan, still in doubt, rebuts, saying that the hand is very small, it is not even a full finger. ‘When in doubt, throw it out’, Jarno concludes, and the photo is deleted.
Officers could rely on the criteria noted in the KIOSK manual, which included e.g. statements on the number of finger sections that should be visible, or to look out for a logo on the back of a phone. However, these criteria still left a lot of room for interpretation and discretion on the part of the patrol officer. This is particularly interesting, as discretion was traditionally central to the work of street-level police officers (e.g. Lipsky Citation1980, Tummers and Bekkers Citation2014), but this discretion was thought to be curbed through algorithmisation (Bovens and Zouridis Citation2002, Bullock Citation2019, Zouridis et al. Citation2020, de Boer and Raaphorst Citation2021). My observations indicate much discretion remains even after algorithms are implemented. With this discretion come clear risks to fairness and equal treatment, as different officers hold citizens to different standards. In part, this incongruency is related to officers’ varied knowledge levels. For one, there is great variation in frequency of use; some patrol officers use MONOcam almost daily during spring and summer season (when the weather is great), whilst others use it for a few days each month, making it more difficult to remember the finer details of their training.
Second, only select ‘ambassadors’ (one per team) received formal training with MONOcam, the other patrol officers learned the system second-hand, from their local ‘ambassador’, reminiscent of a game of telephone. At least two of the regional traffic police teams have appointed new ambassadors in the duration of my fieldwork, and no new training sessions were organised for them. In addition to a lack of formal training, no mechanisms are in place for sharing knowledge and best practices amongst patrol officers. MONOcam patrol officers may take initiative to fill the void left by an absence of official training or knowledge-sharing mechanisms. There is a willingness to learn and increase MONOcam proficiency common amongst those patrol officers I have observed.
The check by a second police officer under the four-eyes-principle provides another opportunity for patrol officers to discuss and compare opinions and set regional standards. Despite the common motto, some officers forward ambiguous photos for the sole purpose of discussing the case with the second officer. In some teams, non-offending photos are also labelled as ‘hit’ when they are perceived as useful for learning and sharing knowledge. These teams attempt to converge perceptions of what constitutes a hit and create a common knowledge base, thus alleviating risks to fairness and equal treatment. These ‘training’ photographs are downloaded from the system. Whilst innocent citizens are not being fined, privacy risks emerge when photos are taken out of the protected software. In this case, equal treatment seems to take precedence over privacy, even if the police officer in question is not aware that they are weighing these values.
A second perceived organisational gap that prompts patrol officers to apply discretion concerns citizens directly. Per the General Administrative Law Act (Algemene Wet Bestuursrecht: Awb) citizens have a right to access their own data and the right to appeal to a fine. As contestability is an important public value, this was safeguarded in the design. Fined drivers would be able to access their pictures through an online portal. This approach was mentioned in the very first preliminary DPIA and was expected to be implemented in 2019. No problems were foreseen, as a similar system already exists for camera-based speeding tickets.
When I started my fieldwork three years later, however, this was not yet implemented.Footnote8 Arthur explains the citizen portal is not owned by the police. Implementing the new functionality thus depends on external organisations. He attributes the delay to interorganisational politics between the police and those external organisations. In the absence of a technological alternative, the traffic police used their discretion to provide this service, a testimony to their ‘solution-orientedness’ (Meijer and Ruijer Citation2021). During this time, fined drivers’ requests for photographic evidence of their violation landed with the patrol officers operating MONOcam. This assigned responsibility to manage appeals can be attributed to the system’s technological design, as only those two officers linked to a certain ticket are able to access the photograph (a measure taken to promote privacy and data security). The following observation shows how a police officer was eager to help when approached by a fined driver.
I am sitting next to Menno in a police vehicle. It is a warm day, and the heat in the van is making me tired. At times our conversation runs dry, and Menno takes this opportunity to call a local police office in another province in the Netherlands. He explains he made an appointment on behalf of a fined driver hoping to see their photograph. After two attempts, the phone is finally answered by a police employee. Apparently, the citizen is already waiting in the lobby. Menno asks his colleague on the phone for their name and uses the outlook address book on his laptop to look up their e-mail address. He then forwards the citizen’s photograph, which he has downloaded in advance from the DigiBon application for police reports. The person on the phone confirms having received the e-mail and checks the identity of the driver before showing the photograph. As the phone is handed to the fined driver, they accept the fine after seeing the photograph. Menno is thanked for his efforts, and the conversation ends. I remember Menno had told me a few weeks earlier that ‘you can’t just send these photos to anyone’, referring to privacy issues. To Menno, sending the photos to police colleagues forms a safety net, improvising a substitute for the lacking online portal by means of service to the citizen. There is also a reference to efficiency: Menno believes helping citizens in this way prevents extra workload of official appeals, even if the method is much less efficient than an online portal would be.
Jarno sees things differently. I explicitly ask how he deals with people hoping to see their photographs. ‘Tell them to start an official objection procedure’, Jarno answers. There is no other way, he explains. As we talk, his frustration with the situation becomes clear. Citizens are provided faulty information and sent from pillar to post, he says. According to Jarno, this is not a good way to treat citizens. Jarno hopes the online portal will be implemented soon and does not understand why it takes this long. His only solution is explaining what the photo looks like over the phone. In some cases that is sufficient. If not, the citizen will have to start an official procedure. ‘Not that that helps, necessarily’, he adds, explaining these procedures can take up to a year, and as they are waiting for the outcome, people often neglect to pay the fine. When they refuse payment, the fine becomes increasingly expensive. ‘People can get into serious debt because of this’, Jarno says. He is clearly affected, feeling that public values such as human dignity and contestability are negatively affected by the lack of the online portal. But in contrast to Menno, Jarno perceives this as being outside of his control.
There is a clear difference in how Menno and Jarno weigh public values. In the case of Menno photos are downloaded and sent through e-mails, no longer being contained in the privacy-secured digital environment they were built in, as contestability and transparency are considered more important. Rather, privacy and data security are ensured through a manual ID-check and the presence of a name in the Outlook address book, flagging someone as a police colleague, and therefore trustworthy. If a citizen happens to pass by a MONOcam elsewhere in the Netherlands, they might not be able to see their photo at all. There appears to be a threat to equal treatment across geographical locations, as users and teams are divided by geographical location and sometimes weigh public values differently.
In the grey area beyond design, patrol officer discretion and variation in knowledge levels play a role in the direct effects of the MONOcam algorithmic system on citizens. As (observed and fined) drivers are held to different standards and have different rights, depending on the patrol officer executing the control, public values such as fairness and equal treatment are affected. Organisational infrastructure and interorganisational dynamics play a role as well. When my fieldwork came to an end in August 2022, the online portal was still not implemented, and no further training or knowledge sharing was facilitated. As patrol officers use their discretion to overcome these gaps, divergent routines emerge for initiating learning or providing service to citizens – a service which is a citizen right. Inevitably, new risks to privacy and data security emerge. Although design-measures such as the original training, the four-eyes principle and the KIOSK guidelines are expected to safeguard values, these cannot function without necessary organisational infrastructures and support.
6. Conclusions and discussion
In this article, I have provided insights into the design and use of the MONOcam system by the Netherlands police. MONOcam is an algorithmic camera system meant for spotting and fining mobile phone users while driving a vehicle on a public road. The design of MONOcam was value-sensitive and can be regarded as a best practice when it comes to responsible design of algorithmic systems in the Netherlands police. Such sensitivity to public values is regarded as one potential road towards the responsible implementation of algorithmic systems (Meijer et al. Citation2021, Meijer and Grimmelikhuijsen Citation2021, Riebe et al. Citation2023). I set out to investigate what lies beyond, in the phase between design and implementation in professional routines and practices, and subject to materiality. My aim was to examine the role of public values in MONOcam as an algorithmic system in use.
In this grey area beyond design, public values that were deemed ‘safeguarded’ through design practices were found to be renegotiated and weighed (de Graaf et al. Citation2014, de Graaf and Meijer Citation2019). This takes place in a variety of ways, and different factors play a role in renegotiating public values in use. In the case of MONOcam, most renegotiation of public values takes place not in the algorithm in its narrow, technical definition, but rather in the wider algorithmic system (Wieringa Citation2020). Due to the situational nature of this research, these results are neither completely representative nor exhaustive, but they do show at least some ways in which the values of MONOcam in use differ from its design.
I have shown how external material aspects such as weather conditions or windscreen properties may play a role in the functioning of the system. Such factors are only found in practice and lie beyond the designers’ influence and control. Although prototyping and testing can be expected to bring to light some of these material properties, this often takes place in a (partially) controlled context, with lots of support and small groups of patrol officers.
Like many systems, MONOcam suffers from function creep (Koops Citation2021), e.g. in emergent entertainment or investigation functions that are not explicitly discussed. Preventing function creep is in part the responsibility of patrol officers, who decide how to use the system at a given time. But allocating all responsibility to MONOcam’s patrol officers disregards the affordances of the technology and the organisation it is embedded in (Leonardi Citation2011).
Organisational infrastructure plays a role when it comes to providing training, standards and mechanisms for sharing knowledge beyond a local team. Such measures could help to create an equal knowledge base and professional practice for MONOcam patrol officers. The online portal that would provide fined drivers access to their photograph, had not been implemented by the time the system was in use. With the lack of such fundamental organisational infrastructure, patrol officers apply their discretion to fill these gaps.
So, what does this mean for safeguarding public values in the use of MONOcam? It can be argued that the system offers great potential to improve policing efficiency, effectiveness and to increase road safety, therefore providing great public benefit (Taylor et al. Citation2012, Lum et al. Citation2017, c.f. debates in 2011, Ozbaran and Tasgin Citation2019). During one of my observations with MONOcam, I was invited to split my day between MONOcam control and an ‘analogue’ control for mobile phone use. Without a doubt, MONOcam was more efficient; it catches more offenders with less resources. During the session I attended, the MONOcam control by a single patrol officer resulted in 14 hits, while a total of 22 patrol officers doing the analogue control caught 29 offenders. For some idea of the scale of this difference; had these patrol officers all been as efficient as MONOcam, 322 offenders would have been caught that morning. MONOcam also allows for relatively low-effort controls. Coordinating an analogue MONO patrol, in contrast, requires much planning and coordination between several local teams. I have been told these analogue controls can be organised at most a few times a year.
However, as a governmental organisation, and particularly as an enforcement organisation, the Netherlands Police has an obligation towards society to implement algorithmic systems in a responsible and ethical manner. This means that public values should not only be safeguarded in the design (as the police have clearly done) but also in the use of the system. While I found no indications of severe negative consequences, this research showed that the use of the system may result in unequal treatment of drivers and in infringement on citizen privacy.
Although this research has focused particularly on the Netherlands Police, the findings may be indicative of other policing contexts worldwide, where systems similar to MONOcam are widely tested and implemented. This paper shows that value-sensitive design is important but offers no guarantees for safeguarding public values in practice. Public values are renegotiated and weighed in each new situation of use. Design is often based on an ideal world, but it is in the complexities and fuzzy realities of everyday professional routines and sociomaterial reality that algorithmic systems such as MONOcam are enacted, and public values are renegotiated. The influence and power of design decrease as an algorithmic system is deployed in a reality where diverse actors, natural phenomena, organisational infrastructure and societal dynamics impact how it functions.
These findings merit a more practice-based scholarship on value-sensitivity in algorithmisation, expanded to include system implementation and use rather than merely system design. They also point out the need for more policing research towards algorithmic applications that do not fit the label of predictive policing. Although predictive policing technologies have justifiably received much academic consideration, this focus has resulted in less critical scholarship on more general technologies such as algorithmic camera systems. The current research shows that such technologies are not unproblematic and warrant critical research, as public values can be at risk.
Further, these findings have practical implications for responsible algorithmisation practices in policing worldwide, and provide valuable insight for public sector organisations implementing algorithmic systems (Friedman et al. Citation2008, Meijer and Grimmelikhuijsen Citation2021). Explicit political and organisational attention is needed for safeguarding public values not only in the design phase but also in the phase of algorithmic system use. If public values are found to be at risk in the phase of algorithm use, additional measures regarding the use of algorithms or even re-design of certain elements of the algorithm may be required.
6.1. Insight to impact
The insights presented in this paper were presented to the Netherlands Police. They have since taken action to further improve their practices and alleviate some of the risks presented in this paper. The Netherlands Police expressed they have gained new awareness on responsible implementation of algorithmic systems beyond technical design. Through their actions, and their support of open and transparent communication about my findings, the Netherlands Police has shown itself to be capable of reflection and committed to responsible implementation of innovative technologies such as the MONOcam algorithmic system, even when that means facing uncomfortable truths. I am happy to report on some of the measures taken, and hope these actions inspire a conversation on how responsible algorithmisation can be organised in complex public organisations. I further hope they can offer practical insights for other policing organisations adopting similar technologies and the designers of these algorithmic systems.
Under section 3 I discussed how some cars have coated windscreens, obscuring the driver from the MONOcam photograph. Due to the nature of my research, however, I could not quantify this finding. I thus advised the Police to conduct quantitative research into this phenomenon. They have deployed a MONOcam system specifically equipped to investigate (a) how many cars have such a coating and (b) what types of cars have such a coating. Final results are not yet in, but preliminary results with a small dataset offer a positive outlook. There seems to be a greater distribution of car brands that have a coating than expected based on my research.
Secondly, the Netherlands Police have taken measures against the function creep of the MONOcam algorithmic system as reported under section 4. Some technical changes have been made to the MONOcam software. A disclaimer has been added to the start-screen of the software. MONOcam patrollers now have to acknowledge that they are aware they are working with police data, and misuse is against the law. Additionally, photos of non-offending citizens are now visible for only three seconds. Previously, these photos were visible until another vehicle passed. The timeframe of three seconds allows the MONOcam patroller to adjust settings, but is short enough to impair their ability to take a photograph of the screen. The Police have also taken measures to increase awareness, e.g. a communication to traffic team chefs that the MONOcam software is not to be used for purposes other than what it was designed for.
In section 5, I mentioned risks of patrol officer discretion, particularly relating to differences in knowledge levels and experience. The Police acknowledges this discretion and is looking into the possibility of organising additional training for key users. Periodically, key users are invited for a plenary session. The target is to have such a session at least four times a year. During the last session, it has been emphasised how important the four-eyes principle is to alleviate some of the risks by discretion. It has also been reiterated that detailed criteria do exist and can be found in the KIOSK manual. Finally, as of March 28th, 2023, offenders have direct online access to the MONOcam photographs of the offence via the Citizen Portal, which alleviates the MONOcam patrollers from providing work-around procedures.
Disclosure statement
No potential conflict of interest was reported by the author(s).
Additional information
Funding
Notes
1 The law specifically concerns holding mobile electronic devices that could be used for communication or information processing. See ‘Reglement verkeersregels en verkeerstekens 1990’ and ‘Wet administratiefrechtelijke handhaving verkeersvoorschriften’, as per 24-06-2019.
2 Newspaper articles of similar technologies at various stages of implementation and use have been found for the United States, United Kingdom, Australia, France and Spain. I conducted observations during an exchange where German officers were trained by the Dutch developers to test the MONOcam system.
3 Please note that all names in this document are fictional – the dataset has been pseudonymized.
4 Whilst the authors list public values, they note that values are also context-dependent and emergent, the list is thus not regarded as complete or definite.
5 Information about the training data was collected in an interview with ‘Daan’, a data scientist who worked on these models. This interview took place prior to my meeting with Arthur.
6 The term ‘four-eyes principle’ prescribes that at least two different people assert human control, in the case of MONOcam this entails at least two officers independently judged the photographs.
7 The police have since tackled this issue. See conclusion.
8 The citizen portal is now implemented and has been operational since March 28th, 2023.
References
- Ananny, M., 2016. Toward an ethics of algorithms: convening, observation, probability, and timeliness. Science, Technology, & Human Values, 41, 93–117. doi:10.1177/0162243915606523.
- Beck Jørgensen, T., and Sørensen, D.-L., 2012. Codes of good governance: national or global public values? Public Integrity, 15, 71–96. doi:10.2753/PIN1099-9922150104.
- Bennett Moses, L., and Chan, J., 2018. Algorithmic prediction in policing: assumptions, evaluation, and accountability. Policing and Society, 28, 806–822. doi:10.1080/10439463.2016.1253695.
- Bovens, M., and Zouridis, S., 2002. From street-level to system-level bureaucracies: how information and communication technology is transforming administrative discretion and constitutional control. Public Administration Review, 62, 174–184. doi:10.1111/0033-3352.00168.
- Brayne, S., 2020. Predict and surveil: data, discretion, and the future of policing. New York: Oxford University Press.
- Bucher, T.. 2018. 'The Multiplicity of Algorithms', If...Then: Algorithmic Power and Politics, Oxford Studies in Digital Politics (New York, 2018; online edn, Oxford Academic, 19 July 2018), doi:10.1093/oso/9780190493028.003.0002.
- Bullock, J.B., 2019. Artificial intelligence, discretion, and bureaucracy. The American Review of Public Administration, 49, 751–761. doi:10.1177/0275074019856123.
- Chan, J.B.L., 2001. The technological game: how information technology is transforming police practice. Criminal Justice, 1, 139–159. doi:10.1177/1466802501001002001.
- Christin, A., 2017. Algorithms in practice: Comparing web journalism and criminal justice. Big Data & Society, 4, 1–14. doi:10.1177/2053951717718855.
- Christin, A., 2020. The ethnographer and the algorithm: beyond the black box. Theory and Society, 49, 897–918. doi:10.1007/s11186-020-09411-3.
- de Boer, N., and Raaphorst, N., 2021. Automation and discretion: explaining the effect of automation on how street-level bureaucrats enforce. Public Management Review, 25 (1), 42–62. doi:10.1080/14719037.2021.1937684.
- de Graaf, G., Huberts, L., and Smulders, R., 2014. Coping with public value conflicts. Administration & Society, 48, 1101–1127. doi:10.1177/0095399714532273.
- de Graaf, G., and Meijer, A., 2019. Social media and value conflicts: an explorative study of the Dutch Police. Public Admin Rev, 79, 82–92. doi:10.1111/puar.12914.
- Friedman, B., Kahn Jr., P.H., and Borning, A., 2008. Value sensitive design and information systems. In: K.E. Himma and H.T. Tavani, eds. The handbook of information and computer ethics. Hoboken, New Jersey: John Wiley, 69–101.
- Gillespie, T., 2014. The relevance of algorithms. In: T. Gillespie and P.J. Boczkowski, eds. Media technologies: Essays on Communication, Materiality, and Society. Cambridge: MA: The MIT Press, 167–194. doi:10.7551/mitpress/9780262525374.003.0009.
- Glancy, D., 2004. Privacy on the Open Road. Ohio Northern Law Review, 30, 295.
- Guzik, K., et al., 2021. Making the material routine: a sociomaterial study of the relationship between police body worn cameras (BWCs) and organisational routines. Policing and Society, 31, 100–115. doi:10.1080/10439463.2019.1705823.
- Hendrix, J.A., et al., 2019. Strategic policing philosophy and the acquisition of technology: findings from a nationally representative survey of law enforcement. Policing and Society, 29, 727–743. doi:10.1080/10439463.2017.1322966.
- Introna, L.D., 2016. Algorithms, governance, and governmentality: on governing academic writing. Science, Technology, & Human Values, 41, 17–49. doi:10.1177/0162243915587360.
- Jørgensen, T.B., and Bozeman, B., 2007. Public values: an inventory. Administration & Society, 39, 354–381. doi:10.1177/0095399707300703.
- Kitchin, R., 2017. Thinking critically about and researching algorithms. Information, Communication & Society, 20, 14–29. doi:10.1080/1369118X.2016.1154087.
- Koops, B.-J., 2021. The concept of function creep. Law, Innovation and Technology, 13, 29–56. doi:10.1080/17579961.2021.1898299.
- Kuziemski, M., and Misuraca, G., 2020. AI governance in the public sector: three tales from the frontiers of automated decision-making in democratic settings. Telecommunications Policy, 44, 101976. doi:10.1016/j.telpol.2020.101976.
- Leonardi, 2011. When flexible routines meet flexible technologies: affordance, constraint, and the imbrication of human and material agencies. MIS Quarterly, 35, 147. doi:10.2307/23043493.
- Lipsky, M., 1980. Street-level bureaucracy: dilemmas of the individual in public services. 1st ed. New York: Russell Sage Foundation.
- Lorenz, L., Meijer, A., and Schuppan, T., 2021. The algocracy as a new ideal type for government organizations: Predictive policing in Berlin as an empirical case. Information Polity, 26, 71–86. doi:10.3233/IP-200279.
- Lum, C., et al., 2011. License plate reader (LPR) police patrols in crime hot spots: an experimental evaluation in two adjacent jurisdictions. J Exp Criminol, 7, 321–345. doi:10.1007/s11292-011-9133-9.
- Lum, C., et al., 2019a. The rapid diffusion of license plate readers in US law enforcement agencies. PIJPSM, 42, 376–393. doi:10.1108/PIJPSM-04-2018-0054.
- Lum, C., et al., 2019b. Research on body-worn cameras: what we know, what we need to know. Criminology & Public Policy, 18, 93–118. doi:10.1111/1745-9133.12412.
- Lum, C., Koper, C.S., and Willis, J., 2017. Understanding the limits of technology’s impact on police effectiveness. Police Quarterly, 20, 135–163. doi:10.1177/1098611116667279.
- Manning, P. 2008. The technology of policing: crime mapping, information technology, and the rationality of crime control. The Technology of Policing: Crime Mapping, Information Technology, and the Rationality of Crime Control. 1–322.
- Meijer, A., and Grimmelikhuijsen, S.A., 2021. Responsible and accountable algorithmization: how to generate citizen trust in governmental usage of algorithms. In: M. Schuilenburg and R. Peeters, eds. The algorithmic society: technology, power, and knowledge. London: Routledge, 53–66.
- Meijer, A., Lorenz, L., and Wessels, M., 2021. Algorithmization of bureaucratic organizations: using a practice lens to study how context shapes predictive policing systems. Public admin rev, 81, 837–846. doi:10.1111/puar.13391.
- Meijer, A., and Ruijer, E., 2021. Code Goed Digitaal Openbaar Bestuur (CODIO): Borgen van waarden bij de digitalisering van openbaar bestuur. Utrecht: USBO Advies.
- Meijer, A., Schäfer, M.T., and Branderhorst, M., 2019. Principes voor goed lokaal bestuur in de digitale samenleving: Een aanzet tot een normatief kader. Bestuurswetenschappen, 73, 8–23. doi:10.5553/Bw/016571942019073004003.
- Meijer, A., and Thaens, M., 2021. The dark side of public innovation. Public performance & management review, 44, 136–154. doi:10.1080/15309576.2020.1782954.
- Mol, A., 2002. The body multiple: ontology in medical practice. Durham: Duke University Press. doi:10.2307/j.ctv1220nc1.
- Moura, E.O.d., and Bispo, M.d.S., 2020. Sociomateriality: theories, methodology, and practice. Can J Adm Sci, 37, 350–365. doi:10.1002/cjas.1548.
- Orlikowski, W.J., and Scott, S.V., 2008. 10 Sociomateriality: challenging the separation of technology, work and organization. ANNALS, 2, 433–474. doi:10.5465/19416520802211644.
- Orlikowski, W.J., and Scott, S.V., 2015. Exploring material-discursive practices: exploring material-discursive practices. Journal of Management Studies, 52, 697–705. doi:10.1111/joms.12114.
- Ozbaran, Y., and Tasgin, S., 2019. Using cameras of automatic number plate recognition system for seat belt enforcement a case study of Sanliurfa (Turkey). PIJPSM, 42, 688–700. doi:10.1108/PIJPSM-07-2018-0093.
- Ratcliffe, J.H., Taylor, R.B., and Fisher, R., 2020. Conflicts and congruencies between predictive policing and the patrol officer’s craft. Policing and Society, 30, 639–655. doi:10.1080/10439463.2019.1577844.
- Riebe, T., et al., 2023. Values and value conflicts in the context of OSINT technologies for cybersecurity incident response: a value sensitive design perspective. Comput Supported Coop Work, 33, 205–251. doi:10.1007/s10606-022-09453-4.
- Schuilenburg, M., and Soudijn, M., 2023. Big data policing: the use of big data and algorithms by the Netherlands Police. Policing: A Journal of Policy and Practice, 17, paad061. doi:10.1093/police/paad061.
- Schwartz-Shea, P., and Yanow, D., 2012. Interpretive research design: concepts and processes, Routledge series on interpretive methods. New York: Routledge.
- Seaver, N., 2017. Algorithms as culture: Some tactics for the ethnography of algorithmic systems. Big Data & Society, 4, 205395171773810. doi:10.1177/2053951717738104.
- Snijders, D., et al., 2019. Burgers en sensoren: Acht spelregels voor de inzet van sensoren voor veiligheid en leefbaarheid. Den Haag: Rathenau Instituut.
- Stahl, B.C., and Wright, D., 2018. Ethics and privacy in AI and Big Data: implementing responsible research and innovation. IEEE Secur. Privacy, 16, 26–33. doi:10.1109/MSP.2018.2701164.
- Stol, W., and Strikwerda, L., 2017. 5.5.2. Nieuwe ICT-hulpmiddelen. In: Strafrechtspleging in Een Digitale Samenleving. Den Haag: Boomjuridisch, 272–280.
- Taylor, B., Koper, C., and Woods, D., 2012. Combating vehicle theft in Arizona: a randomized experiment with license plate recognition technology. Criminal Justice Review, 37, 24–50. doi:10.1177/0734016811425858.
- Terpstra, J., Fyfe, N.R., and Salet, R., 2019. The abstract police: a conceptual exploration of unintended changes of police organisations. The Police Journal, 92, 339–359. doi:10.1177/0032258X18817999.
- Tummers, L., and Bekkers, V., 2014. Policy implementation, street-level bureaucracy, and the importance of discretion. Public Management Review, 16, 527–547. doi:10.1080/14719037.2013.841978.
- Van Hulst, M., 2020. Ethnography and narrative. Policing and society, 30, 98–115. doi:10.1080/10439463.2019.1646259.
- Waardenburg, L., Sergeeva, A., and Huysman, M., 2018. Hotspots and blind spots: a case of predictive policing in practice. In: U. Schultze, M. Aanestad, M. Mähring, C. Østerlund, and K. Riemer, eds. Living with monsters? Social implications of algorithmic phenomena, hybrid agency, and the performativity of technology, IFIP advances in information and communication technology. Cham: Springer, 96–109. doi:10.1007/978-3-030-04091-8_8.
- Wessels, M., 2023. Algorithmic policing accountability: eight sociotechnical challenges. Policing and Society, 34 (3), 124–138. doi:10.1080/10439463.2023.2241965.
- Wieringa, M., 2020. What to account for when accounting for algorithms: a systematic literature review on algorithmic accountability. In: Proceedings of the 2020 Conference on Fairness, Accountability, and Transparency. Presented at the FAT* ‘20: Conference on Fairness, Accountability, and Transparency, ACM. Barcelona, 1–18. doi:10.1145/3351095.3372833.
- Willis, J.J., Koper, C.S., and Lum, C., 2020. Technology use and constituting structures: accounting for the consequences of information technology on police organisational change. Policing and Society, 30, 483–501. doi:10.1080/10439463.2018.1557660.
- Zouridis, S., van Eck, M., and Bovens, M., 2020. Automated discretion. In: T. Evans and P. Hupe, eds. Discretion and the quest for controlled freedom. Cham: Springer, 313–329. doi:10.1007/978-3-030-19566-3_20.