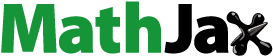
Abstract
This study tested unexpected vection and postural instability accounts of cybersickness by having 30 participants play a commercial virtual reality (VR) simulation (“Mission-ISS”), while wearing head-mounted displays (HMD) for up to 14 minutes, or until the first symptoms of sickness. Of these participants, 23 reported symptoms, while 7 did not. We found that the likelihood and severity of cybersickness increased when the vection experienced was unexpected. Spontaneous standing sway before entering VR was also found to differ depending on whether the participant became sick or remained well. In addition, the severity of their sickness appeared to be related to changes in their sway during VR. When these vection and sway-based predictors were examined together, unexpected vection emerged as the strongest predictor of cybersickness. Taken together with the findings of recent studies, the research shows that unexpected vection predicts experiences of cybersickness across a variety of different virtual environments, virtual navigation methods and types of simulated movement.
1. Introduction
Continuous advancements in Head-Mounted Display (HMD) technology have revolutionized our interaction with virtual reality (VR) systems, expanding their potential applications across diverse fields ranging from household entertainment to astronautic research (Bhagat et al., Citation2016; Burns et al., Citation2017; Lee et al., Citation2017; Liu et al., Citation2016; Pfandler et al, Citation2017). Despite these strides, the pervasive issue of cybersickness (also sometimes referred to as simulator sickness or VR sickness) remains a major deterrent to the further adoption of HMD-VR technology. The presentation and aetiology of cybersickness is complex (Lawson, Citation2014), and currently there is no universally agreed upon theory of this sickness (Palmisano et al., Citation2023). Thus far, sensory conflict and postural instability are the most widely cited causes of this motion sickness (Reason, Citation1978; Reason & Brand, Citation1975; Riccio & Stoffregen, Citation1991). In this paper, we will examine the postural instability theory of motion sickness (Riccio & Stoffregen, Citation1991), and further test our new sensory-conflict account of cybersickness during HMD-VR—the unexpected vection hypothesis (Teixeira et al., Citation2022).
1.1. The postural instability theory of motion sickness
According to the postural instability theory proposed by Riccio and Stoffregen (Citation1991—see also Stoffregen & Smart, Citation1998), motion sickness is more likely to occur when an individual’s ability to maintain their postural stability is compromised. The theory makes three main predictions: 1) individuals who are more unstable in their everyday life will also be more susceptible to cybersickness when they are placed into HMD-VR; 2) during VR, their cybersickness will be preceded by an increase in their postural instability; and 3) the symptoms of this sickness will persist until their postural stability is restored.
Numerous studies now support the proposal that an individual’s natural stability/instabilityFootnote1 can be used to predict the likelihood of them experiencing motion sickness (i.e., under provocative conditions—see Arcioni et al., Citation2019, Bonnet et al., Citation2006; Koslucher et al., Citation2015, Citation2016; Merhi et al., Citation2007; Munafo et al., Citation2017; Risi & Palmisano, Citation2019; Stoffregen & Smart, Citation1998; Stoffregen et al., Citation2000, Citation2008, Citation2010, Citation2014; Teixeira & Palmisano, Citation2021; Villard et al., Citation2008; Yokota et al., Citation2005). Most of these studies examined the visually-induced motion sickness (or VIMS) triggered by console video games, expansive moving rooms, and handheld devices. However, a few of the most recent ones have also examined the theory’s ability to explain cybersickness during HMD-VR. These studies found that differences in their participants’ spontaneous postural activity before entering VR could be used to predict which of them would become sick, or remain well, later during exposure, (Arcioni et al., Citation2019, Munafo et al., Citation2017; Risi & Palmisano, Citation2019; Teixeira & Palmisano, Citation2021). For example, Risi and Palmisano (Citation2019) found that the sway of their “sick” participants produced significantly larger eyes-open, and eyes-closed DFA αA/P values than that of their “well” participants.
It is important to note that the postural instability theory is focussed on identifying: 1) people who will become sick (as distinct from those who will remain well), and 2) situations which are more (or less) likely to induce sickness.
However, Arcioni et al. (Citation2019) also found evidence of scaling relationships between their participant’s spontaneous postural sway (before entering VR) and the severity of their cybersickness (during HMD-VR). Participants who swayed more before entering VR appeared to become sicker during their HMD exposure. However, such scaling relationships have not always been found. For example, Dennison and D’Zmura (Citation2017), failed to find significant relationships between participant sway and cybersickness (as measured by the Simulator Sickness Questionnaire)—possibly because they were focussed only on the spatial magnitude of the postural sway and did not also check on its temporal dynamics.
1.1.1. Predictions which require further testing
To date, most studies examining the relationship between postural instability and cybersickness in HMD-VR have primarily focused on pre-exposure measures of postural sway (in order to test the first prediction of that theory—see Arcioni et al., Citation2019; Chardonnet et al., Citation2017; Clifton & Palmisano, Citation2019; Cobb, Citation1999; Cobb & Nichols, Citation1998; Munafo et al., Citation2017; Nishiike et al., Citation2013; Palmisano et al., Citation2018; Risi & Palmisano, Citation2019). Only a handful of studies have measured their participants’ postural sway during HMD-VR (e.g., Akiduki et al., Citation2003; Dennison & D’Zmura, Citation2017; Merhi et al., Citation2007; Teixeira et al., Citation2023), which is necessary to test the second and third predictions of the theory. While their findings regarding these specific predictions have been inconclusive, the methodology could also have been improved on in some cases (Litleskare, Citation2021). To comprehensively test the postural instability theory of cybersickness, it is essential to assess the participant’s sway: 1) both prior to and during HMD-VR exposure; and 2) by considering both its temporal dynamics (how it evolves over time) and spatial magnitude (how large and variable it is). Teixeira et al. (Citation2023) recently attempted to do this by comparing individuals’ sway during the first and last 30 seconds of their HMD-VR gameplay. The fluctuations in their centre of foot pressure (CoP) were recorded while they flew a futuristic “aircar” for 14 min, or until they dropped out at the first signs of sickness. Consistent with the predictions of the postural instability theory, they found that the amount of change in (both the spatial magnitude and the temporal dynamics of) their participants’ sway correlated significantly with the severity of experienced cybersickness.
1.2. Sensory conflict and motion sickness
Sensory conflict theorists provide very different explanations for this motion sickness. For example, according to Reason (see Reason, Citation1978; Reason & Brand, Citation1975), the motion sickness could be the result of discrepancies in the information: 1) provided by the same sensory system (intrasensory conflicts); 2) between different sensory systems (intersensory conflicts); or 3) between what is being currently sensed and expected to be sensed based on past experience (expectancy violations). Below we will discuss the possible roles of expectancy violations and intersensory conflicts in motion sickness in more detail.
1.2.1. Role of expectancy violations in motion sickness
Reason’s sensory rearrangement theory (1978) predicts that individuals will be more likely to experience motion sickness when there is a neural mismatch between their currently perceived motion and their expected motion stimulation based on past experience. This theory, therefore, explains why motion sickness tends to fade with repeated exposures to initially provocative motion stimulation (as the perceived motion that it produces would initially be unexpected but become progressively more expected with each subsequent exposure—see also Nooij et al., Citation2021). According to Reason (Citation1978), sensory conflicts should only result in motion sickness if the person’s sensory stimulation deviates from what is expected based on their recent exposure history—highlighting the importance of expectations in understanding the aetiology of motion sickness (Nooij et al., Citation2021).
Thus far, only a few studies appear to have directly examined the possible links between expectations and motion sickness. Kuiper et al. (Citation2020) found that auditory cues about future motion directions reduced participant experiences of sickness during physical movements on a sled. Similarly, Feenstra et al. (Citation2011) found that sickness was reduced when anticipatory visual cues were provided about their participants’ physical motion. These findings suggest that being able to anticipate upcoming motions reduces both the likelihood and the severity of motion sickness. They also demonstrate the role that cognitive factors play in the development of motion sickness.
1.2.2. Role of sensory conflicts involving vection in motion sickness
Stoffregen and Riccio (Citation1991) have previously noted that “sensory conflict must be far more common than motion sickness, which is a problem for any claim that conflict causes motion sickness” (p. 161). In response to this, sensory conflict theorists have since sought to identify the specific types of conflict that might be responsible for VIMS and cybersickness. Some have argued that both types of sickness are in fact triggered by the visual-vestibular conflicts produced by visually induced illusions of self-motion. The idea that this vection (i.e., illusory self-motion—see Palmisano et al., Citation2015) might serve as a prerequisite for VIMS is typically attributed to Hettinger et al.’s (Citation1990) flight simulation study (where 9 of their 18 participants reported becoming sick, and 8 of those 9 “sick” participants also experienced vection). However, thus far, the empirical support for this vection conflict proposal has been quite mixed (see Keshavarz et al., Citation2015; Teixeira et al., Citation2022 for reviews of this research). Most studies attempting to test it have looked for evidence of positive scaling relationships between VIMS and vection.Footnote2 However, in their 2015 review of the VIMS literature, Keshavarz and colleagues noted that the relationships between vection strength and sickness severity appeared to differ from study to study—with some studies reporting positive relationships, others negative, and others still finding no significant relationships between them (Keshavarz et al., Citation2015).
In the last decade, research has also examined the possible relationships between vection and cybersickness during HMD-VR (e.g., Clifton & Palmisano, Citation2019; Palmisano et al., Citation2017; Pöhlmann et al., Citation2022; Risi & Palmisano, Citation2019; Teixeira & Palmisano, Citation2021). While some of these studies reported positive scaling relationships between vection strength and sickness severity (e.g., Clifton & Palmisano, Citation2019; Pöhlmann et al., Citation2022; Risi & Palmisano, Citation2019), others reported that the relationships between them were either negative (Palmisano et al., Citation2017) or non-significant (Teixeira & Palmisano, Citation2021).
The above mixed findings appear difficult to reconcile with the widespread belief that vection is always problematic for VIMS and cybersickness. Rather, they suggest that it might be difficult to predict a person’s experience of sickness based simply on the strength of their vection experience alone. If vection plays a causal role in the motion sickness, then information beyond whether it was induced (or not), and how strong it was, must also be important.
1.2.3. Cognitions, vection and motion sickness
Consistent with this idea, recent research suggests that higher-level cognitions about vection are important for understanding the development of both VIMS and cybersickness (see Nooij et al., Citation2021; Teixeira et al., Citation2022, Citation2023). One of these studies, by Nooij and colleagues (Citation2021), examined the experiences of vection and VIMS induced by an optokinetic drum apparatus. Participants sat on a motorised chair inside a large black and white striped drum—both the chair and the drum could be rotated independently about an earth vertical axis (resulting in physical and illusory self-motion respectively). They found: 1) when their seated participants believed that their illusory self-motions produced by the drum rotations were real, their inter-sensory conflicts did not trigger VIMS; 2) differences between the participants’ perceived self-motion and their beliefs about their actual motion accounted for 51% of the variance in their VIMS. Thus, in this study, motion sickness appeared to increase with the participants’ visually induced experience of self-motion, but only when they perceived it to be illusory (as opposed to being caused by actual self-motion).
Of particular relevance to the article are several recent HMD-VR studies which appear to highlight the importance of cognitions about vection for understanding cybersickness (Teixeira et al., Citation2022, Citation2023). Similar to Nooij et al.’s findings above, both studies found evidence that cybersickness increased with the strength of the users’ experiences of vection, but only when this self-motion was perceived to be unexpected and/or out of their control. The idea that expectancy violations involving vection might contribute to HMD user discomfort, disorientation, and motion sickness is not new (Bonato et al., Citation2009). However, Teixeira et al. (Citation2022) were the first to formalise this idea as a theory—their Unexpected Vection Hypothesis (which is described later).
1.2.4. The unexpected vection hypothesis
Teixeira and colleagues (Citation2022) combined the concepts of expectancy violations (Section 1.2.1) and vection conflict (Section 1.2.2) to produce a new sensory conflict-based account of the cybersickness produced by HMD-VR. According to this theory, cybersickness should only be triggered when the vection experienced during a VR simulation is unexpected. This unexpected vection is defined as occurring when: 1) the HMD user perceives self-motion despite being physically still, and 2) this sensation of movement happens in a way that is not anticipated or expected. The theory also proposes that the severity of their cybersickness should increase with the strength of any unexpected (but not expected) vection. Importantly, according to the theory, vection that is expected by the HMD user based on their past or current experiences and perceived to be under their control, should not lead to significant discomfort.
1.2.4.1 Support for the unexpected vection hypothesis
Thus far, support for this hypothesis has been provided by two HMD-VR studies with somewhat differing methodologies. In the first (Teixeira et al., Citation2022), participants interacted with the HMD-VR simulation “Mission: ISS” for 14 min, or until they were forced to dropout (due to significant sickness). During the simulation, they moved through various sections of the international space station under simulated microgravity conditions (by making thumb-based joystick deflections to jet, or tracked arm movements to push or pull, themselves along). While the self-reported “sick” and “well” participants in this study did not differ in terms of overall vection strength, those who became sick were significantly more likely to report experiences of unexpected/uncontrolled vection. Through machine learning analysis, unexpected vection also emerged as the primary predictor of their cybersickness.
More recently, Teixeira et al. (Citation2023) had their participants play a different HMD-VR game “Aircar,” where they flew a futuristic aircar along a pre-selected route for up to 14 min. In contrast to the earlier study, these participants were instructed to terminate the game at the first signs of cybersickness (regardless of severity). Again, the “sick” participants in this study were more likely to have experienced unexpected vection. While their sickness severity increased with the strength of the unexpected vection, no relationship was found between vection strength and cybersickness when vection was expected.
1.3. The current study
Teixeira et al.’s (Citation2023) recent “Aircar” study found some support for the predictions of both the unexpected vection hypothesis and the postural instability theory (without showing a strong preference for one theory over the other). The current study was aimed at both replicating and extending the findings of that study. As different gaming environments: 1) can be more/less provocative for cybersickness; 2) pose unique challenges in terms of the HMD user’s postural stability, and 3) often vary significantly in their simulated experiences of self-motion, it was deemed to be important to re-examine the predictions of both theories when playing a different commercially available HMD-VR game. In doing so, we aimed to tease apart any situational dependencies and assess the broader predictive power of these two cybersickness theories.
1.3.1. Replicating the effects of unexpected vection on cybersickness
Instead of the flying “Aircar” game, the current study instead used the “Mission: ISS” microgravity simulator. Based on findings of both prior studies (i.e., Teixeira et al., Citation2022, Citation2023), we predicted that: 1) participants who became sick would also be more likely to experience unexpected vection during “Mission: ISS” (compared to those who remained well); and 2) the severity of their sickness would increase with the strength of their unexpected vection. That is, we expected that unexpected vection would continue to play an important role in both the occurrence and the development of cybersickness in this new study—thereby replicating the vection–cybersickness findings of both of our recent HMD-VR studies.
1.3.2. Understanding how postural instability contributes to cybersickness
While our earlier “Mission: ISS” study (Teixeira et al., Citation2022) examined the role of unexpected vection in cybersickness, it did not test the postural instability theory. It also had participants persist with their symptoms of cybersickness until they were forced to drop out of the simulation (due to their severity). By contrast, in the current “Mission: ISS” study, we had participants drop out at the very first signs/symptoms of cybersickness (mirroring the approach taken by Teixeira et al.’s (Citation2023) more recent “Aircar” study). This design choice was important to determine the specific patterns of postural activity during the VR simulation which were responsible for initially triggering the cybersickness.
In line with the postural instability theory, we expected that: 1) more naturally unstable participants to be the most susceptible to cybersickness when playing “Mission: ISS”; 2) during gameplay, the onset of their cybersickness would be preceded by an increase in their postural instability (compared to their earlier sway, before experiencing cybersickness). Crucially, Teixeira et al.’s (Citation2023) recent “Aircar” study only provided clear support for the second (not the first) of these predictions. Recent research also shows that effects on cybersickness do not always generalise from one VR game to another (e.g., the effects of repeated exposure to VR games on the severity of the sufferer’s symptoms—see Palmisano & Constable, Citation2022). Thus, we felt that it was important to test the postural instability theory again, with its predictions placed in direct competition with those based on the unexpected vection hypothesis.
1.3.3. Testing the predictions of the unexpected vection and postural instability theories together
Until now, the unexpected vection and postural instability theories have only been tested together using the flying HMD-VR game “Aircar.” Postural measures and unexpected vection were both found to be somewhat effective in predicting the cybersickness experienced in that study. Compared to “Aircar,” “Mission: ISS” was expected to pose much greater postural challenges to our standing HMD users: 1) with its simulated microgravity conditions altering their perceptions of self-motion (e.g., promoting unique sensations like “floating”); and 2) by providing them with little visual information about where their “up” and “down” were supposed to beFootnote3 (Howard & Hu, Citation2001). Thus, the choice of this microgravity simulator was expected to favour the postural predictors of cybersickness in this study. However, the task requirements to move during these simulated microgravity conditions were also expected to increase the likelihood of unexpected vection (as well as user perceptions of a loss in control; ).
Table 1. Comparison of study characteristics: Teixeira et al. (Citation2022, Citation2023) vs. Current study.
2. Method
2.1. Participants
The study examined 30 participants (16 males and 14 females; age = 26 years ± 6.03 years) recruited from the general population. They all had normal or corrected-to-normal vision and no self-reported visual, vestibular, or neurological impairments. All reported feeling well at the start of the experiment. Two of these were excluded from some of the statistical analyses below as they came off the Bertec balance plate during gameplay. The study was approved by the UOW Ethics Committee, and each participant provided informed written consent prior to participating in the study.
2.2. Materials
2.2.1. Hardware and software
Exposure to the virtual environment was delivered via an Oculus Rift S HMD,Footnote4 and two Oculus Touch (hand) controllers. The HMD had a resolution of 1280 × 1440 pixels for each eye and a refresh rate of 80 Hz via Fast-Switch LCD technology. The virtual environment was generated by a Microsoft Windows 10 Dell Optiplex 7090 computer, which contained a Nvidia GeForce RTX3070 graphics card with the latest stable driver software installed and an Intel 11th generation CPU. The simulation software used in this study was “Mission: ISS” (https://www.oculus.com/experiences/rift/1178419975552187/?locale=en_US).
Participants were exposed to the simulator’s tutorial section, which simulated first-person exposure to microgravity inside a virtual model of the International Space Station. Within the parameters of the tutorial participants learned to move through the space station via “thrusting” (pushing the left joystick in the direction of desired movement and then accelerating in that direction) and by pulling themselves along the space station’s interior (using the Oculus Touch hand controllers to first grab, and then release, handles and other protrusions). Like Teixeira et al. (Citation2022) the experience and control of microgravity locomotion was somewhat alien to participants. It was thus expected that this software might be likely to induce unexpected types of vection, particularly due to perceived losses of control. However, the interior of the space station also provided little visual information about “up” and “down.” Beyond the interior’s visual frame cues, there was only some ambiguous intrinsic visual polarity information (provided by floating objects with identifiable “tops” and “bottoms”) and no useful extrinsic polarity information (which normally relies on one or more objects resting on/supporting another) to assist the HMD user in their orienting behaviour (see Howard & Hu, Citation2001 for a discussion). Thus, this simulated microgravity environment was also expected to pose significantly greater postural challenges to our HMD users (compared to the game “Aircar”; ).
Table 2. Comparative overview of VR environments: “Mission: ISS” vs. “Aircar.”
2.2.2 Postural measures
Before commencing the experiment, participant’s foot size, width, and arch lengths were measured using a Brannock Device. Their spontaneous postural sway (eyes-open) was then measured before the VR exposure trial. While standing in a quiet stance, a Bertec balance plate (http://bertec.com/products/balance-plates.html) recorded the spontaneous fluctuations in their anterior/posterior (A/P) and medio/lateral (M/L) centre of foot pressure (CoP) for two 60 s periods (sampled at 500 Hz).
To make the CoP data more manageable for subsequent analyses, the sample rate was reduced from 500 to 50 Hz. We then calculated the standard deviations of the CoP along their A/P and M/L axes (i.e., STDEV CoPA/P and STDEV CoPM/L) to estimate the spatial magnitudes of their sway. We also performed detrended fluctuation analyses (DFA) on these CoPA/P and CoPM/L data to investigate the temporal dynamics of their sway. The scaling exponents of these DFAs (i.e., DFA αA/P and DFA αM/L) provided indexes of the long-range auto correlations in the CoP data.
The balance plate was also used to measure our participants’ standing postural sway while they were playing HMD-VR. We examined how their sway changed across the HMD-VR exposure by comparing the spatial magnitudes and the temporal dynamics of their sway at the start and the end of their gameplay. Specifically, we calculated standard deviation and DFA α difference scores for the CoPA/P and CoPM/L data obtained in the first and last 30 s of their exposure (For an examination of the first and last 30s of these sway indices independently, see Appendix A).
2.2.3. Cybersickness severity and symptom profiling
At the end of their HMD-VR exposure participants were first asked whether they felt sick or well (their response to this question was used to classify them into “sick” and “well” groups). Before and after the HMD-VR exposure, their nausea, disorientation, and oculomotor symptoms were also assessed using Kennedy et al.’s (Citation1993) Simulator Sickness Questionnaire (SSQ). Based on Stanney et al. (Citation2014), it was assumed that SSQ scores greater than 20.1 represented functionally significant sickness during VR (see Lawson & Stanney, Citation2021).
2.2.4. Vection type and strength
The concept of vection was introduced to participants as the illusion of self-movement while physically stationary, typically elicited through visual stimuli (Kooijman et al., Citation2023; Palmisano et al., Citation2015). When contextualizing vection within the “Mission: ISS” VR environment, our explanations were tailored to the specific tasks that participants would perform, following guidelines for ensuring relevance and practicality in vection measurement (Kooijman et al., Citation2023). We described how, during the virtual experience of moving inside the ISS, participants might feel that they were physically moving through the modules despite being (mostly) stationary in the real world.
We further clarified our concepts by distinguishing between expected and unexpected vection using the same methods as Teixeira et al. (Citation2022, Citation2023). Expected vection was described as the perception of motion that aligns with what the participants see and anticipate in the VR environment. For instance, feeling a sense of floating or moving forward within the ISS when navigating through its interior. Conversely, unexpected vection was characterized as perceiving a motion that contradicts anticipatory visual cues or expectations. This could include sensations like feeling a drift in a direction opposite to where they are were trying to navigate towards or experiencing an unexpected change in the speed or direction of their simulated movements.
This detailed explanation was aimed at helping participants to not only understand the concept of vection but also differentiate between its expected and unexpected forms. We engaged participants actively in this process, encouraging them to discuss their understanding and relate it to their own experiences. This interactive approach was important in confirming that participants comprehended these concepts as they related to their unique experiences.
Directly after the simulation terminated, we obtained an overall rating of the strength of their vection experienced during the exposure (from 0 = “no vection” to 10 = “strong vection”). Participants were also then quizzed about the nature of their experience of vection. If they had reported being sick, they were asked:
“Did your feelings of being in control of your perceived self-movement change prior to sickness?”
“Was the type of your perceived self-movement more or less expected/anticipated prior to sickness?”
“Is there anything else you wanted to add? Do you have any insights about why you think you became sick?”
Alternatively, if they reported remaining well throughout the HMD-VR session, they were instead asked:
“Did you feel in control of your perceived self-movement during the simulation?”
“Were the types of perceived self-movement expected/anticipated during the simulation?”
“Is there anything else you wanted to add?”
During the debriefing at the end of the experiment, we revisited participants’ reported sensations of self-motion to clarify their reports and experiences of vection within the “Mission: ISS” VR environment. In addition to confirming that they were referring to whole-body self-motions relative to the virtual environment (rather than the movements of their limbs or their postural sway), we aimed to specifically identify and confirm instances of unexpected vection.
Feedback gathered during these sessions often involved participants reporting examples of vection (particularly unexpected vection), which participants found particularly memorable. For instance, many described that when they stopped making thrusting or pulling control movements, in order to avoid hitting a looming wall, ceiling, or floor, they continued to feel compelling sensations of movement towards these objects (Similar to original reports described in Teixeira et al., Citation2022). Additionally, reports of unexpected rotational vection were common, where participants experienced an unanticipated sense of turning or rotating as being particularly unpleasant. These checks served as a final confirmation of the participants’ understanding of vection, particularly unexpected vection in the study.
2.3. Procedure
Participants were given an information sheet that described the experiment, which they were asked to sign to indicate their consent. Their height and foot size were then recorded before they were instructed to step on a Bertec balance plate to assess their spontaneous postural instability (based on subsequent analyses of recorded fluctuations in their CoP). Their spontaneous postural activity was measured (eyes-opened, looking at a piece of paper attached to the laboratory wall 1 m in front of them) for two 60 s periods. Then, directly before their exposure to HMD-VR, they completed the pre-exposure sections of the SSQ to provide a baseline measure of their current sickness levels. Participants were next instructed to step back onto the balance plate, don the Oculus Rift S HMD and run through the tutorial section of “Mission: ISS” either for 14 minutes, or until they responded, “yes” to the question: “Do you feel sick?” During gameplay, postural stability indices were constantly recorded as participants actively explored the space station via “thrusting” (pushing the joystick in the direction of the desired locomotion and then accelerating in space) and by pushing or pulling themselves via the hand controllers along the space station’s interior. Directly after leaving HMD-VR, their post-exposure sickness status (sick or well), sickness severity and symptomology were assessed (using a “Yes/No” response to the question “do you feel sick?” and responses to the 16 items on the post-exposure SSQ). Participants also provided an overall (0-20) rating of the vection strength for the trial. Finally, they verbally responded to our three exploratory questions about the nature of their experiences of vection during HMD-VR ().
2.4. Data analysis
Data from Teixeira et al. (Citation2023) (which tested 40 participants) was first used to perform a power analysis for the current study using a resampling technique. The results of the analysis, which returned an observed statistical power (1 − β) of 91%, revealed that our sample size (28 participants after exclusions) should have been sufficient to detect true relationships of the same size involving cybersickness (on the assumption of an α level of 0.05).
We then validated our classification of participants into sick and well groups using a Yuen’s trimmed means testFootnote5 (jamovi 2.3, Walrus R package), bootstrapped at 1000 samples to assess differences in post-pre SSQ-T scores between these groups. To explore the relationships between vection and sickness, we employed several approaches: a Pearson’s chi-square test was used to assess differences in instances of unexpected vection between sick and well groups, and a Yuen’s t-test compared the average vection strength ratings between these groups. For predicting the participant’s Sick/Well group membership based on vection type, we utilized a logistic model (jamovi 2.3, GAMLj package). We also used a generalised linear model to test the hypothesis of a positive scaling relationship between vection strength and cybersickness severity for participants who experienced unexpected vection (Poisson distribution – jamovi 2.3, GAMLj package).
To examine possible relationships between sickness and sway, we followed similar methodology. A logistic model was used to predict participants’ Sick/Well group membership based on the fluctuations in their pre-exposure spontaneous CoP (its spatial magnitude and temporal dynamics). Another logistic model assessed whether an increase in postural instability during VR (as determined by changes in the summary measures of their CoP data for the first and last 30 s of exposure) preceded cybersickness. Additionally, a generalised linear model (Poisson distribution) explored potential scaling relationships between these CoP change measures and cybersickness severity. Finally, we investigated whether combined vection and CoP change measures significantly predicted cybersickness occurrence using a logistic model and assessed their combined contributions to cybersickness severity using a generalised linear model (Poisson distribution – jamovi 2.3, GAMLj package).
3. Results
3.1. Examining cybersickness symptomology & confirming sick/well groupings
The SSQ was used to assess our participants’ post-exposure nausea (SSQ-N), disorientation (SSQ-D) and oculomotor (SSQ-O) symptoms (see ). As expected for HMD-VR. We found that these post-exposure scores were higher on average for the SSQ-D (M = 31.1, SD = 26) than for the SSQ-N (M = 29.3, SD = 21.1) and the SSQ-O (M = 19.7, SD = 15.4) (SSQ-T M = 29.5, SD = 19.5).
Figure 2. Post-exposure SSQ-T (total), SSQ-D (disorientation), SSQ-N (nausea), and SSQ-O (oculomotor) scores split by “sick” and “well” participants.
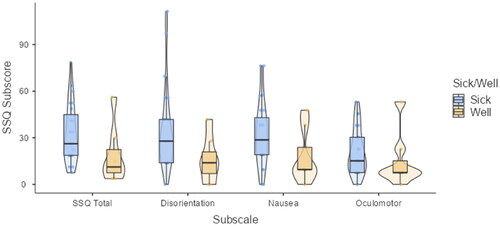
Our 30 participants were classified as either being “sick” or “well” based on their answers to the question: “Do you feel sick?”. The 23 “sick” participants responded “yes” to this question, while the remaining 7 “well” participants responded “no.” To check on the success of this classification, we conducted a Yuen’s trimmed means t-test, bootstrapped at 1000 samples to assess the difference between “sick” and “well” groups’ post-exposure total SSQ (SSQ-T) scores. “Sick” participants were found to have significantly higher post SSQ-T scores (M = 32.8, SD = 18.9) than “well” participants (M = 18.7, SD = 18.7, t(8.93) = 1.89, p = .049, ξ = .485). Based on Stanney et al. (Citation2014), the SSQ-T scores of our “sick” participants corresponded to “high” or “extreme” levels of sickness (please see Table 31.3 in that book chapter). As expected, our “well” participants did not on average reach the identified threshold for functionally significant sickness (i.e., SSQ-T > 20.1).
3.2. Characteristics of vection in “sick” and “well” groups
Before proceeding with further analyses, we examined the occurrence of vection among our participants, categorized into our “sick” and “well” groups. Among the “sick” group, a 91% (21 out of 23) reported experiencing unexpected vection during their HMD VR sessions. In contrast, within the “well” group, only 14% (1 out of 7) participants reported such experiences (see for a breakdown of these counts).
Figure 3. Numbers of “sick” and “well” participants who did (and did not) experience unexpected vection during HMD VR.
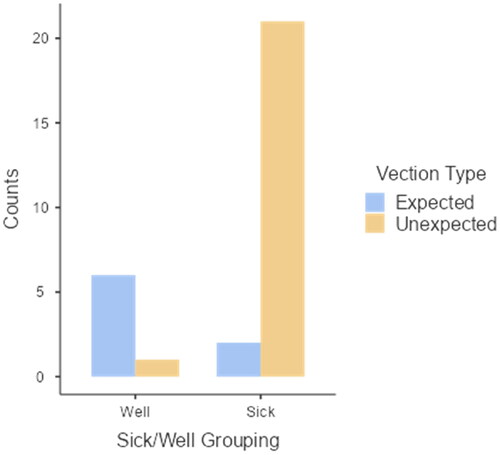
Following this, we assessed the average vection strength reported by both groups. Participants in the “sick” group, who largely experienced unexpected vection, reported a mean vection strength of 6.91 (out of a maximum possible score of ten), SD = 1.93. Conversely, the “well” group, which had fewer reports of unexpected vection, reported a lower average vection strength of 5.86, SD = 2.27 (see ).
3.4. Predicting the occurrence of sickness based on vection type
To investigate our hypothesis that “sick” participants in our study would be more likely to report unexpected vection (compared to those who were “well”) we used the following logistic model (jamovi 2.3, GAMLj package). We originally intended to model the interaction between vection strength and type. However, only one participant in the “well” group reported experiencing unexpected vection. This limitation in the data precluded a meaningful analysis of the interaction as initially planned, due to the insufficient variation in vection experiences among the “well” participants. Rather than examining the interaction, we instead focused on predicting participants’ Sick/Well group membership using only the type of vection that they had experienced (expected/unexpected) as a fixed effect:
We found a significant main effect of vection type (z = 2.54, p = .011, estimate = 5.134) on Sick/Well group membership, indicating that participants were more likely to be “sick” when their vection was unexpected (rather than expected).
3.5. Predicting the occurrence of sickness based on postural sway
3.5.1. Pre-exposure (spontaneous) postural activity and “sick/well” grouping
To examine whether more naturally unstable individuals would be more susceptible to sickness in HMD-VR (compared to those who remain well) we used the following logistic model (jamovi 2.3, GAMLj package), where participants’ Sick/Well group memberships were predicted by the spatial magnitude and temporal dynamics of their pre-exposure spontaneous sway as fixed effects:
We found a significant main effect of STDEV CoPA/P (z = −2.06, p = .039, estimate = 12.42) on Sick/Well group membership, indicating that participants with lower STDEV CoPA/P were more likely to report being sick than those with higher STDEV CoPA/P values.
3.5.2. Changes in sway during VR and “sick/well” grouping
To examine whether cybersickness was preceded by an increase in postural instability during VR, we used the following logistic model (jamovi 2.3, GAMLj package), where participants’ Sick/Well group memberships were predicted by the changes in the spatial magnitude and temporal dynamics of their sway (by calculating standard deviation and DFA α change scores based on the differences in the first and last 30 s of the exposureFootnote6) as fixed effects:
While the model converged, we did not find any significant effects of our change in sway variables on Sick/Well grouping.
3.6. Predicting the occurrence of sickness based on both vection and changes in sway
Next, we investigated whether vection and sway change measures contributed significantly to predicting the occurrence of cybersickness when they were both combined into a single model. We used the following logistic model (jamovi 2.3, GAMLj package), where participants’ Sick/Well group memberships were predicted by the changes in the spatial magnitude and temporal dynamics of their sway, as well as the type and strength of the vection they experienced (plus their interaction) as fixed effects:
When modelling these factors together however, the model failed to converge.
3.7. Predicting sickness severity based on vection
The above analyses all focussed on the occurrence of cybersickness (whether someone reported being “sick” instead of “well”). We next examined whether the severity of cybersickness could be predicted by our participants’ experience of vection. As with our other recent studies, we used a generalised linear model (Poisson distribution) to test our hypothesis that there would be a positive relationship between vection strength and cybersickness severity for participants who experienced unexpected types of vection. Sickness severity (as measured by the post-exposure SSQ-T) was modelled in terms of the vection strength (i.e., reported vection strength prior to dropout), vection type (whether it was expected/unexpected) and their interaction, as fixed effects:
As illustrated in , this model revealed a significant interaction between vection strength and vection type, z = 3.02, p =.003, estimate = 0.1951. Thus, the main effects of vection type and vection strength on SSQ-T ratings were not investigated further.
Figure 5. Non-linear relationships between vection strength and sickness severity (post SSQ-T) as a function of vection type (unexpected/expected). Shaded areas represent standard error.
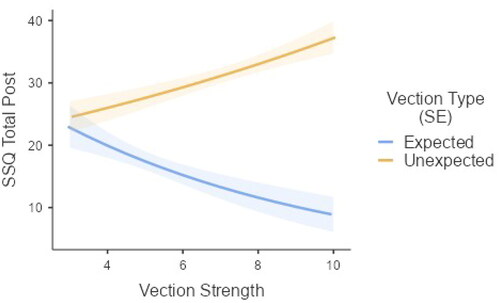
The simple effects of vection strength for each type of vection (expected vs unexpected, effects corrected for multiple comparisons, adjusted p-value threshold = 0.025) confirmed a significant positive relationship between vection strength and cybersickness severity for those who experienced unexpected vection (z = 2.77, p =.006, estimate = .0597), but not for those who experienced expected vection (z = −2.22, p = 0.026, estimate = −0.1354).
3.8. Predicting sickness severity based on changes in sway
We also used a generalised linear model (Poisson distribution) to explore whether there might be scaling relationships between our sway change measures and cybersickness severity (using jamovi 2.3, GAMLj package). Sickness severity (post-SSQ-T) was modelled in terms of the changes in our spatial magnitude and temporal dynamics sway measures (i.e., last 30 s – first 30 s):
As shown in , we found significant main effects of ΔDFA αM/L (z = −3.28, p = .001, estimate = −1.18) and ΔSTDEV CoPM/L (z = 4.64, p <.001, estimate = 29.5) on sickness severity. This indicates that larger Late-early DFA αM/L values were associated with lower SSQ-T scores, whereas larger Late-early STDEV CoPM/L values were associated with higher SSQ-T scores.
Figure 6. Non-linear relationships between spatial magnitude (late–early STDEV CoPA/P or STDEV CoPM/L)/temporal dynamics (late–early DFA αa/P or DFA αM/L) measures and sickness severity (post SSQ-T) for the “changes in sway model.” Shaded areas represent standard error.
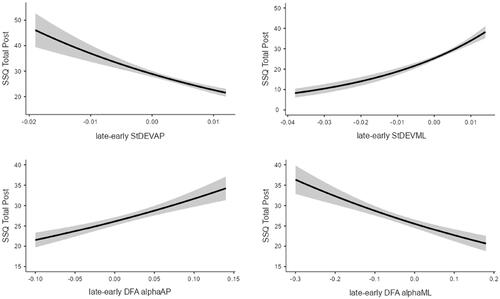
We also found significant main effects of ΔSTDEV COPA/P (z = −3.77, p <.001, estimate = −24.55) and ΔDFA αA/P (z = 3.06, p = .002, estimate = 1.93) on sickness severity. This suggests that larger Late-early STDEV COPA/P values were associated with lower SSQ-T scores and larger Late-early DFA αA/P values were associated with higher SSQ-T scores.
3.9. Predicting sickness severity based on both vection and changes in sway
Next, we investigated whether vection and sway-based measures both contributed significantly to predicting the severity of cybersickness when they were combined into a single model. We used a generalised linear model (Poisson distribution—jamovi 2.3, GAMLj package). Sickness severity (post SSQ-T) was modelled in terms of the changes in our spatial magnitude and temporal dynamics-based sway measures, as well as vection type, vection strength and their interaction, as fixed effects:
As shown in , we found significant main effects of ΔDFA αM/L (z = −2.904, p = .004, estimate = −1.0609) and ΔSTDEV CoPM/L (z = 5.424, p <.001, estimate = 51.8752) on sickness severity. This indicates that larger late–early DFA αM/L values were associated with lower SSQ-T scores and larger late–early STDEV CoPM/L were associated with higher SSQ-T scores. We also found significant main effects of ΔSTDEV COPA/P (z = −6.829, p <.001, estimate = −51.3572) and ΔDFA αA/P (z = 2.483, p = .013, estimate = 1.735) on sickness severity. This suggests that larger Late-early STDEV COPA/P values were associated with lower SSQ-T scores, while larger Late-early DFA αA/P values were associated with higher SSQ-T scores. As hypothesised, the interaction between vection strength and type was significant (z = 2.752, p = .006, estimate = 0.1876). When examining the simple effects (adjusted p-value threshold = 0.025), there was a significant positive relationship between vection strength and cybersickness severity for those who experienced unexpected vection (z = 3.22, p = .001, estimate = 0.0750), but not for those who only experienced expected vection (z = −1.71, p = .088; see ).
4. Discussion
In this HMD-VR study, we investigated sickness predictions based on both the unexpected vection hypothesis and the postural instability theory. Our analyses provided evidence that it was possible to predict cybersickness based on measures obtained before, during, and after VR exposure, with notable distinctions. Unexpected vection emerged as a robust predictor of cybersickness occurrence and severity across all models tested. In contrast, the hypotheses of the postural instability theory received mixed support. In this discussion we present the evidence for each theory individually, then compare them when assessed together.
4.1. Support for the unexpected vection hypothesis
In line with the predictions of the unexpected vection hypothesis and the findings of Teixeira et al. (Citation2022, Citation2023), our study demonstrated strong and reliable associations between experiences of unexpected vection and reports of cybersickness.
In terms of predicting the occurrence of cybersickness, we found that 91% of our “sick” participants had experienced unexpected vection, whereas this was only the case for 14% of our “well” participants.Footnote7 Thus, like Teixeira et al. (Citation2022, Citation2023), unexpected vection was found to significantly increase the probability of experiencing sickness, as vection type (expected/unexpected) significantly predicted our participants sick/well group membership. It is important to recognize though that there were isolated instances of reported sickness in the absence of unexpected vection.
We also hypothesised that cybersickness severity would increase with the strength of the participant’s experience of unexpected vection, but not with the strength of vection when it was expected. Consistent with Teixeira et al. (Citation2022, Citation2023), our results revealed: 1) significant positive relationships between the strength of unexpected vection and cybersickness severity (again, with a good fit provided by a non-linear model); and 2) no statistically significant relationships between the strength of expected vection and sickness severity.
Taken together, these findings support, and build upon those presented in our past studies (Teixeira et al., Citation2022, Citation2023). All three studies consistently demonstrate that the effects of unexpected vection on cybersickness are robust across various virtual reality contexts. Specifically, they persist despite differences in the virtual environment, the type/s of simulated locomotion, and the stage of sickness progression being examined.
4.2. Support for the postural instability theory
Like Teixeira et al. (Citation2023), our study also assessed sickness predictions based on the postural instability theory. Initially, we investigated if more naturally unstable individuals would also be more prone to experiencing cybersickness. Consistent with that proposal, we found that our self-reported “sick” and “well” participants differed significantly in terms of their spontaneous standing sway (i.e., their sway before entering HMD-VR). Intuitively, one might assume that unstable participants would sway more (as indicated by larger values of STDEV CoPA/P and STDEV CoPM/L). However, we found that the spontaneous sway of our self-reported “sick” participants had significantly smaller (not larger) STDEV CoPA/P than our self-reported “well” participants. These “sick” participants also did not appear to differ significantly from the “well” participants in terms of the temporal dynamics of their spontaneous sway.
One possible interpretation of our findings is that the simulated microgravity environment within the ISS markedly deviates from the typical VR conditions of most prior studies (such as driving vehicles). As such, these distinct features may have provoked postural adjustments not commonly observed in standard VR environments. Subjects with naturally lower standing-sway variability may have been inherently less capable of adapting to these novel challenges of navigating in a microgravity setting. Specifically, their lower sway variability along the anterior-posterior axis may reflect an intrinsic postural rigidity, or a lack of compensatory sway strategies that may be effective in more conventional or familiar settings seen in prior studies, but less so in the context of microgravity simulation, leading them more likely to become “sick.”
We next explored whether changes in our participants’ postural sway during HMD-VR exposure would predict the imminent onset of their experiences of cybersickness. However, contrary to the predictions of the postural instability theory, our data did not reveal significant associations between the changes in (the spatial magnitude or the temporal dynamics of) our HMD users’ standing sway and their sick/well group status.Footnote8
While not explicitly predicted by the postural instability theory (as outlined by Stoffregen & Smart, Citation1998), we also checked for possible relationships between our participants’ postural activity during HMD-VR and the severity of their sickness. Consistent with our recent findings (in Teixeira et al., Citation2023), we did find significant relationships between the changes in their sway and cybersickness severity ratings. Specifically, we found that increases in STDEV CoP M/L, and decreases in STDEV CoP A/P, over the course of the HMD-VR exposure were each associated with an increase in sickness severity ratings. Similarly, we also found that an increase in DFA αA/P values, and a decrease in DFA αM/L values, over the course of the HMD-VR exposure were each associated with an increase in sickness severity ratings. It should be noted that these results only partially supported the findings of Teixeira et al. (Citation2023) with “Aircar,” which found that sickness severity ratings increased as: 1) both STDEV CoPA/P and STDEV CoP M/L increased; and 2) both DFA αA/P and DFA αM/L values decreased over the course of the trial.
These inverse relationships might be a result of the natural gameplay conditions and the unique challenges posed by the microgravity simulation in “Mission: ISS.” Unlike more static VR tasks in previous studies (such as in “aircar”), the microgravity simulation in “Mission: ISS” requires users to physically engage with the virtual space in unconventional ways, potentially leading to distinct sway patterns. Specifically, the way participants physically interact with and navigate through the “Mission: ISS” environment—responding to visual cues and adapting to the lack of gravitational reference—might influence their postural stability differently across the M/L and A/P axes. For example, in response to sickening or disorienting scenarios, players might instinctively ‘grab’ virtual elements like the sides of the space station, increasing the variability along the mediolateral axis while reducing variability along the anterior posterior axis as a strategy to stabilize themselves or counteract unsettling motions encountered within the game.
It is important to note that postural instability researchers consider any change in a person’s standing sway before the onset of sicknessFootnote9 to be potentially indicative of provocative postural activity for motion sickness (see Keshavarz et al., Citation2014 for a discussion). Having said that, the relationships between our potential postural predictors and HMD user experiences of cybersickness appeared to be particularly complex in the current study. When taken together, the above discrepancies pose a question as to the degree to which the findings of our studies align with the postural instability theory. The differences across our studies suggest a need for more reliable methods to identify and measure postural instability in future research.
4.3. Comparing both theories of cybersickness
Following from the findings from Teixeira et al. (Citation2023), we also explored models that combined both vection and sway based measures to predict cybersickness. These models included the participants’ reported experiences of vection (its type and strength), as well as measures of the changes in their sway during the VR exposure (considering both its temporal dynamics and positional variability). However, when we modelled both types of measures together to predict the sick/well group membership of our participants, the combined model did not converge.Footnote10 When we instead attempted to model our participants’ ratings of cybersickness severity, both the vection and sway-based measures were found to significantly contribute to the model’s predictions. Again, a non-linear positive relationship was identified between vection strength and sickness severity, but only for those participants who experienced unexpected vection. Changes in our participants’ STDEV CoP and DFA α values over the course of the exposure also continued to be associated with cybersickness severity ratings (again in the same rather complex fashion described in Section 4.2).
When we compared the findings of this combined model with those of Teixeira et al. (Citation2023), unexpected vection emerged as a consistent and robust predictor of sickness occurrence and severity. In contrast, our attempt to integrate sway measures into the combined model faced convergence challenges, and some of the directional effects observed in Teixeira et al. (Citation2023) were not replicated in the current study. This suggests that unexpected vection exerts a more immediate and apparent influence on cybersickness ratings (in comparison to the specific sway-based predictor variables that we utilised in both studies—i.e., STDEV CoP and DFA α).
4.4. Implications and relevance of findings
Vection is still generally perceived to be a problem that should be avoided due to its potential association with cybersickness. This common view has caused reluctance in integrating compelling vection experiences into HMD-VR, consequently limiting the technology’s full potential and benefits. Our current findings, and those of Teixeira et al. (Citation2022, Citation2023), strongly challenge this notion. Unlike the mixed results of past studies examining the influence of vection in general, our studies have consistently demonstrated the influence of unexpected vection on cybersickness (across each of the various stages of sickness progression, in different novel virtual environments, and using a range of different methods of simulated self-locomotion).
While unexpected vection should be avoided, our studies have also shown that it is possible to experience compelling vection without any sickness (i.e., when that vection is expected and perceived to be under the HMD user’s control). Thus, our results emphasize the need to mitigate experiences of unexpected vection as a primary approach to reducing cybersickness. During game/app development, beta testing should be used to the modify simulated self-motions based on user feedback (i.e., to find the times/movements in the simulation which are more likely to generate unexpected vection, and once they are identified, then alter them). Incorporating “comfort” modes in these apps, which provide straightforward indicators of the future self-motions (via directional arrows or anticipatory animations), could also prepare users for them (even when these are simulated to be fast and continuous). Both approaches could maximise the benefits of providing vection during HMD-VR, while also ensuring that the simulated self-motions are expected and perceived to be under the user’s control. Future research will of course be required to demonstrate the effectiveness of such interventions.
While our findings appear to provide partial support for the postural instability theory, they also differed from those recently reported in Teixeira et al. (Citation2023). In contrast to that study, we did find that our “sick” and “well” participants differed in terms of the spatial magnitude of their spontaneous sway (although our “sick” participants were observed to sway less than our “well” participants before entering HMD-VR). Unlike the previous study, we did not observe obvious increases in participant sway before the onset of their sickness symptoms. However, we did find that the changes in their sway across the VR exposure were related to the severity of their sickness symptoms.
These results highlight the significant role that the context and nature of the VR environment play in the relationship between postural sway and cybersickness severity. As mentioned, the immersive experience of navigating a microgravity environment, such as that of the ISS in VR, may have introduced postural and responses not previously encountered in more conventional settings. While the primary goal of our study was to examine the effects of unexpected vection across various HMD contexts, our findings drive home the necessity of extending this approach to other theories of cybersickness as well. Future research should delve into the impact of diverse VR environments, particularly those simulating non-terrestrial conditions, on postural control and cybersickness, to broaden our understanding of these phenomena.
4.5. Limitations and future directions
We recognize the potential influence of demand characteristics on our study’s outcomes, particularly in evaluating expected and unexpected vection. However, we considered this risk minimal. To minimize it further, we employed two strategies: 1) limiting participants to a single HMD-VR exposure, and 2) questioning them about their vection experiences only after exposure, using the text specified in Section 2.2.4.
We also recognize the challenge in determining directionality in subjective experiences. Future research should therefore attempt to identify exactly when unexpected vection experiences occur (in addition to when the first symptoms of sickness are experienced). This would help to clarify the directionality of any relationships between vection type and the onset of sickness. To enhance the comprehensiveness of our findings, future studies could also benefit from integrating objective measures alongside our self-report data. Incorporating physiological indicators, such as tracking eye movements or other reactions of the vestibular and visual systems, would provide a more complete understanding of the experience of vection. Additionally, our study design did not include experimental manipulations specifically aimed at inducing expected or unexpected vection. Implementing such manipulations in future research could offer a clearer understanding of how different vection types are elicited and experienced within controlled settings.
Another potential limitation of our research to date is that all three unexpected vection studies have examined cybersickness during active gameplay. As a result, the contribution of unexpected vection to cybersickness during passive HMD-VR has yet to be explored. Investigations of the effects of vection and sway on cybersickness during passive VR gameplay are not only important because of its prevalence, but also because such conditions are inherently less predictable (due to the absence of any user control). Passive VR also provides researchers with unique opportunities in terms of experimental design and control—allowing them to present highly consistent and tightly controlled exposures of simulated self-motion to all their participants. Therefore, we suggest that future studies bridge these gaps by examining cybersickness during both active and passive VR gameplay—to gain a more comprehensive understanding of how unexpected vection and sway influence these VR experiences.
Finally, while our findings contribute to the understanding of unexpected vection and cybersickness in VR, they do not account for variations in participants’ inherent susceptibility to motion sickness. This omission might influence the strength and directionality of the observed relationships between vection type, vection strength, and cybersickness. Future research should include measures such as the MSSQ (Golding, Citation1998) to provide a more comprehensive analysis that accounts for these individual differences.
4.6. Conclusions
We compared the impact of unexpected vection and postural instability on cybersickness induced by a commercial HMD-VR simulation. Our results revealed that cybersickness was more likely and severe among participants who experienced unexpected (as opposed to expected) vection. While certain postural measures were also found to be significantly associated with cybersickness, only unexpected vection was found to reliably predict this sickness when both types of predictors were combined in the same model. These findings emphasize the robust nature of the effects of unexpected vection on cybersickness, which appear consistent regardless of differences in the virtual environment, simulated locomotion, or the stage of sickness progression being examined.
Data availability statement
Data will be made available upon reasonable request.
Disclosure Statement
No potential conflict of interest was reported by the author(s).
Additional information
Funding
Notes on contributors
Joel Teixeira
Joel Teixeira is a PhD candidate at the University of Wollongong. His research is centred around virtual reality, with a specific focus on VR cybersickness and vection.
Sebastien Miellet
Sebastien Miellet’s research focuses on dynamic scene perception and face processing within active vision. He develops new analysis and modeling approaches, facilitating a thorough comprehension of perception-action coordination. Moreover, he devises gaze-contingent methods for precise isolation of visual diagnostic data.
Stephen Palmisano
Stephen Palmisano is a Professor of Psychology at the University of Wollongong. He was awarded his PhD from the University of New South Wales in 1997. His primary research interests are self-motion perception (especially vection), stereoscopic depth perception and motion sickness.
Notes
1 i.e., their postural instability when they are attempting to stand still without any provocative motion stimulation.
2 It should, however, be noted that Hettinger et al. (Citation1990) did not actually propose that sickness severity should scale with the strength of the vection.
3 In “Mission: ISS” the interior of the space station provided minimal intrinsic, and no extrinsic, visual polarity cues to “up” and “down” (see Howard & Hu, Citation2001 for explanations of these cues). By contrast, “Aircar” provided extensive visual information about the orientations of the HMD user in their virtual aircar, and that car in the virtual world (provided by the interior cabin of the car and the external buildings, roads, waterways, and visible horizon respectively).
4 Since data collection, newer VR headsets with advanced features have surpassed the Rift S. This may limit the generalizability of our findings to current VR technology. Future research should use the latest headsets to explore their impact on vection and cybersickness.
5 We selected Yuen’s trimmed means t-test for our analysis because it accommodates the unequal sample sizes and variances often seen in studies like ours, with “sick” and “well” groups (Yuen, Citation1974). This test also trims extreme values, making it resilient against outliers and skewness, which is crucial given the variability in cybersickness symptoms (Wilcox, Citation2011).
6 e.g., ΔSTDEV CoPA/P = STDEV CoPA/P last 30 s – STDEV CoPA/P first 30 s.
7 Consistent with our expectations, the incidence of unexpected vection observed in the present study appeared to exceed that reported in Teixeira et al. (Citation2023)’s “Aircar” study, where only 79% of the “sick” participants and 6% of the “well” participants reported experiencing unexpected vection.
8 We had expected to find larger late-early differences in the sway of our “sick” (compared to “well”) participants. However, because sick participants dropped out at the very first signs of sickness, they stood for much less time than the ‘well’ participants (< 8 mins, as opposed to 14). To double check our prediction, we estimated the spatial magnitudes and temporal dynamics of the “well” participants’ sway just before the average dropout time for “sick” participants (3.61 minutes). Similar to our original late-early sway analyses, these later-early analyses revealed no significant differences in sway change for our “sick” and “well” participants (p >.05). This suggests that the varied exposure times and early withdrawal of “sick” participants did not undermine the validity of our original conclusions.
9 Whether it be an increase/decrease in sway, or an increase in the variability of that sway (Keshavarz et al., Citation2014).
10 This issue might have arisen due to the postural measures, which, given their non-significant effects when examined independently, could have adversely affected the model.
References
- Akiduki, H., Nishiike, S., Watanabe, H., Matsuoka, K., Kubo, T., & Takeda, N. (2003). Visual-vestibular conflict induced by virtual reality in humans. Neuroscience Letters, 340(3), 197–200. https://doi.org/10.1016/s0304-3940(03)00098-3
- Arcioni, B., Palmisano, S., Apthorp, D., & Kim, J. (2019). Postural stability predicts the likelihood of cybersickness in active HMD-based virtual reality. Displays, 58, 3–11. https://doi.org/10.1016/j.displa.2018.07.001
- Bhagat, K. K., Liou, W. K., & Chang, C. Y. (2016). A cost-effective interactive 3D virtual reality system applied to military live firing training. Virtual Reality, 20(2), 127–140. https://doi.org/10.1007/s10055-016-0284-x
- Bonato, F., Bubka, A., & Palmisano, S. (2009). Combined pitch and roll and cybersickness in a virtual environment. Aviation, Space, and Environmental Medicine, 80(11), 941–945. https://doi.org/10.3357/asem.2394.2009
- Bonnet, C. T., Faugloire, E., Riley, M. A., Bardy, B. G., & Stoffregen, T. A. (2006). Motion sickness preceded by unstable displacements of the center of pressure. Human Movement Science, 25(6), 800–820. https://doi.org/10.1016/j.humov.2006.03.001
- Burns, J. A., Adkins, L. K., Dailey, S., & Klein, A. M. (2017). Simulators for laryngeal and airway surgery. Otolaryngologic Clinics of North America, 50(5), 903–922. https://doi.org/10.1016/j.otc.2017.05.003
- Chardonnet, J.-R., Mirzaei, M. A., & Mérienne, F. (2017). Features of the postural sway signal as indicators to estimate and predict visually induced motion sickness in virtual reality. International Journal of Human–Computer Interaction, 33(10), 771–785. https://doi.org/10.1080/10447318.2017.1286767
- Clifton, J., & Palmisano, S. (2019). Effects of steering locomotion and teleporting on cybersickness and presence in HMD-based virtual reality. Virtual Reality, 24, 453–468. https://doi.org/10.1007/s10055-019-00407-8
- Cobb, S. V. (1999). Measurement of postural stability before and after immersion in a virtual environment. Applied Ergonomics, 30(1), 47–57. https://doi.org/10.1016/S0003-6870(98)00038-6
- Cobb, S. V., & Nichols, S. C. (1998). Static posture tests for the assessment of postural instability after virtual environment use. Brain Research Bulletin, 47(5), 459–464. https://doi.org/10.1016/S0361-9230(98)00104-X
- Dennison, M. S., & D'Zmura, M. (2017). Cybersickness without the wobble: Experimental results speak against postural instability theory. Applied Ergonomics, 58, 215–223. https://doi.org/10.1016/j.apergo.2016.06.014
- Feenstra, P. J., Bos, J., & Gent, R. N. H. W. (2011). A visual display enhancing comfort by counteracting airsickness. Displays, 32(4), 194–200. https://doi.org/10.1016/j.displa.2010.11.002
- Golding, J. F. (1998). Motion sickness susceptibility questionnaire revised and its relationship to other forms of sickness. Brain Research Bulletin, 47(5), 507–516. https://doi.org/10.1016/s0361-9230(98)00091-4
- Hettinger, L. J., Berbaum, K. S., Kennedy, R. S., Dunlap, W. P., & Nolan, M. D. (1990). Vection and simulator sickness. Military Psychology: The Official Journal of the Division of Military Psychology, American Psychological Association, 2(3), 171–181. https://doi.org/10.1207/s15327876mp0203_4
- Howard, I. P., & Hu, G. (2001). Visually induced reorientation illusions. Perception, 30(5), 583–600. https://doi.org/10.1068/p3106
- Kennedy, R. S., Lane, N. E., Berbaum, K. S., & Lilienthal, M. G. (1993). Simulator sickness questionnaire: An enhanced method for quantifying simulator sickness. The International Journal of Aviation Psychology, 3(3), 203–220. https://doi.org/10.1207/s15327108ijap0303_3
- Keshavarz, B., Hecht, H., & Lawson, B. D. (2014). Visually induced motion sickness: Causes, characteristics, and countermeasures. In K. S. Hale and K. M. Stanney (Eds.), Handbook of Virtual Environments: Design, Implementation, and Applications. (pp. 652–703). CRC Press. https://doi.org/10.1201/b17360-32
- Keshavarz, B., Riecke, B. E., Hettinger, L. J., & Campos, J. L. (2015). Vection and visually induced motion sickness: How are they related? Frontiers in Psychology, 6, 472. https://doi.org/10.3389/fpsyg.2015.00472
- Kooijman, L., Berti, S., Asadi, H., Nahavandi, S., & Keshavarz, B. (2023). Measuring vection: A review and critical evaluation of different methods for quantifying illusory self-motion. Behavior Research Methods, 1–19. https://doi.org/10.3758/s13428-023-02148-8
- Koslucher, F., Haaland, E., & Stoffregen, T. A. (2016). Sex differences in visual performance and postural sway precede sex differences in visually induced motion sickness. Experimental Brain Research, 234(1), 313–322. https://doi.org/10.1007/s00221-015-4462-y
- Koslucher, F., Haaland, E., Malsch, A., Webeler, J., & Stoffregen, T. A. (2015). Sex differences in the incidence of motion sickness induced by linear visual oscillation. Aerospace Medicine and Human Performance, 86(9), 787–793. https://doi.org/10.3357/amhp.4243.2015
- Kuiper, O. X., Bos, J. E., Diels, C., & Schmidt, E. A. (2020). Knowing what’s coming: Anticipatory audio cues can mitigate motion sickness. Applied Ergonomics, 85, 103068. https://doi.org/10.1016/j.apergo.2020.103068
- Lawson, B. (2014). Motion sickness symptomatology and origins. In Hale, K. S. & Stanney K. M. (Eds.). Handbook of virtual environments: Design, implementation, and applications (pp. 532–587). CRC Press. https://doi.org/10.1201/b17360-29
- Lawson, B., & Stanney, K. M. (2021). Editorial: Cybersickness in virtual reality and augmented reality. Frontiers in Virtual Reality, 2, 2759682. https://doi.org/10.3389/frvir.2021.759682
- Lee, A. Y., Fried, M. P., & Gibber, M. (2017). Improving rhinology skills with simulation. Otolaryngologic Clinics of North America, 50(5), 893–901. https://doi.org/10.1016/j.otc.2017.05.002
- Litleskare, S. (2021). The relationship between postural stability and cybersickness: It’s complicated–An experimental trial assessing practical implications of cybersickness etiology. Physiology & Behavior, 236, 113422. https://doi.org/10.1016/j.physbeh.2021.113422
- Liu, X., Liu, Y., Zhu, X., An, M., & Hu, F. (2016). Virtual reality-based navigation training for astronaut moving in a simulated space station. In Virtual, Augmented and Mixed Reality: 8th International Conference, VAMR 2016, Held as Part of HCI International 2016, Toronto, Canada, July 17-22, 2016. Proceedings. (Vol. 8, pp. 416–423). Springer International Publishing.
- Merhi, O., Faugloire, E., Flanagan, M., & Stoffregen, T. A. (2007). Motion sickness, console video games, and head-mounted displays. Human Factors, 49(5), 920–934. https://doi.org/10.1518/001872007X230262
- Munafo, J., Diedrick, M., & Stoffregen, T. A. (2017). The virtual reality head-mounted display Oculus Rift induces motion sickness and is sexist in its effects. Experimental Brain Research, 235(3), 889–901. https://doi.org/10.1007/s00221-016-4846-7
- Nishiike, S., Okazaki, S., Watanabe, H., Akizuki, H., Imai, T., Uno, A., Kitahara, T., Horii, A., Takeda, N., & Inohara, H. (2013). The effect of visual-vestibulosomatosensory conflict induced by virtual reality on postural stability in humans. The Journal of Medical Investigation: JMI, 60(3-4), 236–239. https://doi.org/10.2152/jmi.60.236
- Nooij, S. A., Bockisch, C. J., Bülthoff, H. H., & Straumann, D. (2021). Beyond sensory conflict: The role of beliefs and perception in motion sickness. PloS One, 16(1), e0245295. https://doi.org/10.1371/journal.pone.0245295
- Palmisano, S., & Constable, R. (2022). Reductions in sickness with repeated exposure to HMD-based virtual reality appear to be game-specific. Virtual Reality, 26(4), 1373–1389. https://doi.org/10.1007/s10055-022-00634-6
- Palmisano, S., Allison, R. S., Schira, M. M., & Barry, R. J. (2015). Future challenges for vection research: Definitions, functional significance, measures and neural bases. Frontiers in Psychology, 6, 193. https://doi.org/10.3389/fpsyg.2015.00193
- Palmisano, S., Allison, R. S., Teixeira, J., & Kim, J. (2023). Cybersickness in head-mounted displays is caused by differences in the user’s virtual and physical head pose. Virtual Reality, 27(2), 1293–1313. https://doi.org/10.3389/frvir.2020.587698
- Palmisano, S., Arcioni, B., & Stapley, P. J. (2018). Predicting vection and visually induced motion sickness based on spontaneous postural activity. Experimental Brain Research, 236(1), 315–329. https://doi.org/10.1007/s00221-017-5130-1
- Palmisano, S., Mursic, R., & Kim, J. (2017). Vection and cybersickness generated by head-and-display motion in the Oculus Rift. Displays, 46, 1–8. https://doi.org/10.1016/j.displa.2016.11.001
- Pfandler, M., Lazarovici, M., Stefan, P., Wucherer, P., & Weigl, M. (2017). Virtual reality-based simulators for spine surgery: A systematic review. The Spine Journal: official Journal of the North American Spine Society, 17(9), 1352–1363. https://doi.org/10.1016/j.spinee.2017.05.016
- Pöhlmann, K. M. T., Föcker, J., Dickinson, P., Parke, A., & O'Hare, L. (2022). The relationship between vection, cybersickness and head movements elicited by illusory motion in virtual reality. Multisensory Research, 71, 1–40. https://doi.org/10.1016/j.displa.2021.102111
- Reason, J. T. (1978). Motion sickness adaptation: A neural mismatch model. Journal of the Royal Society of Medicine, 71(11), 819–829. https://doi.org/10.1177/014107687807101109
- Reason, J. T., & Brand, J. J. (1975). Motion sickness. Academic press.
- Riccio, G. E., & Stoffregen, T. A. (1991). An ecological theory of motion sickness and postural instability. Ecological Psychology, 3(3), 195–240. https://doi.org/10.1207/s15326969eco0303_2
- Risi, D., & Palmisano, S. (2019). Effects of postural stability, active control, exposure duration and repeated exposures on HMD induced cybersickness. Displays, 60, 9–17. https://doi.org/10.1016/j.displa.2019.08.003
- Stanney, K. M., Kennedy, R. S., & Hale, K. S. (2014). Virtual environment usage protocols. In Hale, K. S. & Stanney, K. M. (Eds.), Handbook of virtual environments: Design, implementation and applications (pp 532–587.). CRC Press. https://doi.org/10.1201/b17360
- Stoffregen, T. A., & Riccio, G. E. (1991). An ecological critique of the sensory conflict theory of motion sickness. Ecological Psychology, 3(3), 159–194. https://doi.org/10.1207/s15326969eco0303_1
- Stoffregen, T. A., & Smart, L. J. Jr. (1998). Postural instability precedes motion sickness. Brain Research Bulletin, 47(5), 437–448. https://doi.org/10.1016/s0361-9230(98)00102-6
- Stoffregen, T. A., Chen, Y. C., & Koslucher, F. C. (2014). Motion control, motion sickness, and the postural dynamics of mobile devices. Experimental Brain Research, 232(4), 1389–1397. https://doi.org/10.1007/s00221-014-3859-3
- Stoffregen, T. A., Faugloire, E., Yoshida, K., Flanagan, M. B., & Merhi, O. (2008). Motion sickness and postural sway in console video games. Human Factors, 50(2), 322–331. https://doi.org/10.1518/001872008X250755
- Stoffregen, T. A., Hettinger, L. J., Haas, M. W., Roe, M. M., & Smart, L. J. (2000). Postural instability and motion sickness in a fixed-base flight simulator. Human Factors, 42(3), 458–469. https://doi.org/10.1518/001872000779698097
- Stoffregen, T. A., Yoshida, K., Villard, S., Scibora, L., & Bardy, B. G. (2010). Stance width influences postural stability and motion sickness. Ecological Psychology, 22(3), 169–191. https://doi.org/10.1080/10407413.2010.496645
- Teixeira, J., & Palmisano, S. (2021). Effects of dynamic field-of-view restriction on cybersickness and presence in HMD-based virtual reality. Virtual Reality, 25(2), 433–445. https://doi.org/10.1007/s10055-020-00466-2
- Teixeira, J., Miellet, S., & Palmisano, S. (2022). Unexpected vection exacerbates cybersickness during HMD-based virtual reality. Frontiers in Virtual Reality, 3, 43. https://doi.org/10.3389/frvir.2022.860919
- Teixeira, J., Miellet, S., & Palmisano, S. (2023). Unexpected vection predicts the likelihood and severity of sickness during HMD based virtual reality. Journal of Vision, 23(9), 5036–5036. [Vision Sciences Society Annual Meeting Abstract]. https://doi.org/10.1167/jov.23.9.5036
- Villard, S. J., Flanagan, M. B., Albanese, G. M., & Stoffregen, T. A. (2008). Postural instability and motion sickness in a virtual moving room. Human Factors, 50(2), 332–345. https://doi.org/10.1518/001872008X250728
- Wilcox, R. R. (2011). Introduction to robust estimation and hypothesis testing. Academic press.
- Yokota, Y., Aoki, M., Mizuta, K., Ito, Y., & Isu, N. (2005). Motion sickness susceptibility associated with visually induced postural instability and cardiac autonomic responses in healthy subjects. Acta Oto-Laryngologica, 125(3), 280–285. https://doi.org/10.1080/00016480510003192
- Yuen, K. K. (1974). The two-sample trimmed t for unequal population variances. Biometrika, 61(1), 165–170. https://doi.org/10.2307/2334299
Appendix A:
Figure A2. Non-linear relationships between Spatial Magnitude (STDEV CoPA/P or STDEV CoPM/L)/Temporal Dynamics (DFA αA/P or DFA αM/L) measures and sickness severity based on sway from the first 30s of Exposure. (Post SSQ-T). Shaded areas represent standard error.
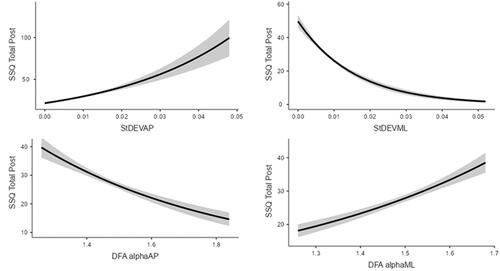
Figure A4. Non-linear relationships between Spatial Magnitude (STDEV CoPA/P or STDEV CoPM/L)/Temporal Dynamics (DFA αA/P or DFA αM/L) measures and sickness severity based on sway from the last 30s of Exposure. (Post SSQ-T). Shaded areas represent standard error.
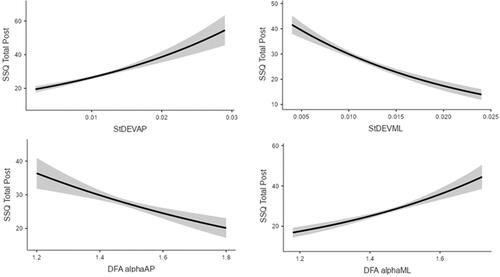
Table A1. Descriptives of the Spatial Magnitude (STDEV CoPA/P or STDEV CoPM/L)/Temporal Dynamics (DFA αA/P or DFA αM/L) measures obtained from the 1st 30s of the exposure.
Table A3. Descriptives of Spatial Magnitude (STDEV CoPA/P or STDEV CoPM/L)/Temporal Dynamics (DFA αA/P or DFA αM/L) measures obtained from the last 30s of the exposure.