ABSTRACT
The teaching of communication skills is a labour-intensive task because of the detailed feedback that should be given to learners during their prolonged practice. This study investigates to what extent our FILTWAM facial and vocal emotion recognition software can be used for improving a serious game (the Communication Advisor) that delivers a web-based training of communication skills. A test group of 25 participants played the game wherein they were requested to mimic specific facial and vocal emotions. Half of the assignments included direct feedback and the other half included no feedback. It was investigated whether feedback on the mimicked emotions would lead to better learning. The results suggest the facial performance growth was found to be positive, particularly significant in the feedback condition. The vocal performance growth was significant in both conditions. The results are a significant indication that the automated feedback from the software improves learners’ communication performances.
1. Introduction
In the last few decades, communication skills have become much more important in a wide variety of jobs’ portfolios (e.g. Brantley & Miller, Citation2008). This pattern can be easily explained as a consequence of today’s networked world of digital technologies, which have considerably altered the nature of professional work. Increasingly, professional labour has become knowledge-driven and requires extensive collaboration and communication between professionals. For instance, a few decades ago engineers could more or less work independently without intensive communication with fellow engineers. But, as products and processes have become increasingly complex, diverse specialists from a wide range of disciplines have to work closely together in order to develop or support such sophisticated products and services.
Nowadays, communication skills’ training is mostly arranged in face-to-face contexts. But such training is not without problems. Communication courses require intensive skilled tutoring by teachers, preferably at a one-to-one personalized level, which puts heavy loads on tutoring capacity. Moreover, because of this heavy tutoring load, communication courses are expensive, or even worse; they are less effective because of a teacher bandwidth problem (Hager, Hager, & Halliday, Citation2006; Vorvick, Avnon, Emmett, & Robins, Citation2008). Another issue is the required teacher quality: providing proper feedback in communication training is a challenging and demanding task that is only mastered after appropriate training (Cantillon & Sargeant, Citation2008). The shortage of well-qualified teachers is problematic (Hager et al., Citation2006). In addition, face-to-face training is usually arranged within fixed schedules and time slots, which severely affects the learners’ flexibility to do their exercises. Prolonged practice, which is an essential condition for truly mastering communication skills, also seems to be hampered by the limitations of face-to-face training. Online approaches to communication skills training would provide a more flexible training context, allowing learners to do their exercises whenever they want and wherever they are. Digital serious games developed for learning purposes facilitate such flexibility in an attractive way. Serious games are part of the wider area of the game-based learning. Abt (Citation1970) introduced the term “serious games” to indicate games for job training, such as the training of army personnel or insurance salesmen. Moreover, the effectiveness of serious games for learning has been acknowledged in several studies (Connolly, Boyle, MacArthur, Hainey, & Boyle, Citation2012; Wouters, Van Nimwegen, Van Oostendorp, & Van der Spek, Citation2013).
The current study focuses on the empirical validation of our emotion recognition approach described in previous studies (Bahreini, Nadolski, & Westera, Citation2014, Citation2015, Citation2016a, Citation2016b) within the practical context of a serious game for communication skills training (the Communication Advisor). Our basic research question is to what extent facial and vocal emotion recognition software can be used for improving communication skills training. The Communication Advisor allows learners to practice and improve their skills for emotion expression. The Communication Advisor has been developed based on the EMERGO game engine.Footnote1 The Communication Advisor offers learners a number of separate assignments that require them to respond to a specific problem situation by displaying pre-defined emotions. The manifested emotions of the learners are captured with the computer’s webcam and microphone and are fed into the FILTWAM face and voice emotion recognition technologies. The FILTWAM software assesses the manifested emotions for both facial expression and voice intonation. The outcome is then used for presenting direct feedback to the learners about the correctness of their performances. Importantly and in contrast with physiological sensor-based emotion recognition technologies, the FILTWAM software allows for the unobtrusive in-game assessment of learners’ manifested emotions, as it only captures and analyses webcam and microphone signals. Moreover, FILTWAM evaluates the observed emotion in real time, whereby it can be used for giving direct feedback. For this validation study, we used a within-subjects experimental design with 25 participants playing the Communication Advisor. Besides collecting the players’ performance data, we collected qualitative data comprising the players’ appreciations and judgements about the game as a learning tool.
We first provide a brief overview of previous research in emotion recognition used in computer-based learning. After a brief explanation of the FILTWAM framework, we describe the research methodology and the research findings. We conclude the paper by discussing the findings and making suggestions for future research.
2. Related work
It is commonly acknowledged that emotions are a significant influential factor in the process of learning, as they affect memory and action (Pekrun, Citation1992). The influence of emotions on learning is traditionally well recognized in classroom teaching practice (Bower, Citation1981). More recently, emotions have also received attention in the domain of intelligent tutoring systems (ITS) (Sarrafzadeh, Alexander, Dadgostar, Fan, & Bigdeli, Citation2008). An ITS is a computer-based system that is capable of providing immediate and personalized instruction and feedback to learners (Psotka & Mutter, Citation1988). The general premise is that extending ITS with emotion recognition capabilities would lead to better conditions for learning, as it allows for adjusting its interventions to the emotional states of the user. Although there are many studies reported in the wider domains of emotion recognition and ITS, to our knowledge no study has yet been conducted that specifically combines automatic facial and vocal emotion recognition in communication skills training.
An important success factor in classroom learning is the capability of a teacher to timely recognize and respond to the affective states of their learners. For this, teachers continuously adjust their teaching behaviour by observing and evaluating the behaviour of the learners, including their facial expressions, body movements, and other signals of overt emotions. In e-learning, just as with classroom learning, it is not only about cognition and learning, but also about the interdependency of cognition and emotion. These relationships between learners’ cognition and emotion are influenced by the electronic learning environment, which mediates the communication between participants (teacher, learner, and his peers) and contains or refers to e-learning materials (e.g. text, photos, audios and videos, and animations). Contemporary, instructional approaches increasingly address emotional dimensions by accommodating challenges, excitement, ownership, and responsibility, among other things, in the learning environment. Software systems for e-learning (e.g. ITS, serious games, personal learning environments) could better foster learning if they also adapt the instruction and feedback to the emotional state of the learner (Sarrafzadeh et al., Citation2008). Within the scope of ITS, Feidakis, Daradoumis, and Caballe (Citation2011) categorized emotion measurement into three types of tools, which have been described in several previous studies: (1) psychological (Wallbott, Citation1998), (2) physiological (Kramer, Citation1991), and (3) motor-behavioural (Leventhal, Citation1984). Psychological tools are self-reporting tools for capturing the subjective experience of emotions of users. Physiological tools comprise sensors that capture an individual’s physiological responses. Motor-behaviour tools for emotion extraction use special software to measure behavioural movements captured by PC cameras, mouse, or keyboard. Most of these emotion recognition tools suffer from limited reliability and unfavourable conditions of use, which hampered successful implementation of so-called affective tutoring systems (ATS). But more recently, there has been a growing body of research on ATS that recommends emotion recognition technologies based on facial expressions (Ben Ammar, Neji, Alimi, & Gouardères, Citation2010; Wu, Huang, & Hwang, Citation2015) and vocal expressions (Rodriguez, Beck, Lind, & Lok, Citation2008; Zhang, Hasegawa-Johnson, & Levinson, Citation2003).
Communication skills’ training typically involves expressing specific emotions at the right point and time; such training can become tedious, as it requires prolonged practice. Serious games offer a challenging and dynamic learning context that seamlessly combine emotion and cognition (Westera, Nadolski, Hummel, & Wopereis, Citation2008). Such games are characterized by timely feedback to cater for skills learning from prolonged practice and are praised for their motivational affordances (Van Eck, Citation2010). Note that as online communication is inherently truncated communication, which tends to strip messages from their emotional dimensions (Westera, Citation2013), emotion recognition is an emerging field in human–computer interaction as this would be a promising next step in enhancing the quality of online interaction and communication. Unfortunately, only a few studies address emotion recognition in digital serious games. A study by Hyunjin, Sang-Wook, Yong-Kwi, and Jong-Hyun (Citation2013) investigated whether a simple brain computer interface with a few electrodes can recognize emotions in more natural settings such as playing a game. They invited 42 participants to play a brain-controlled video game wearing a headset with single electrode brain computer interface and provided a self-assessed arousal feedback at the end of each round. By analysing the data obtained from the self-evaluated questionnaires and the recordings from the brain computer interfaces device, they proposed an automatic emotion recognition method that classifies four emotions with the accuracy of about 66%. Some studies address adaptation in games based on the measurement of user’s emotions, motivation, and flow (Pavlas, Citation2010; Tijs, Brokken, & IJsselsteijn, Citation2009). In the study conducted by Tijs et al., the researchers investigated the relations among game mechanics, a player’s emotional state, and the associated emotional data. The researchers manipulated speed as a game mechanic in the experimental sessions. They requested players to provide their emotional state for valence, arousal, and boredom–frustration–enjoyment. Moreover, they measured a number of physiology-based emotional data features. Then, they compared the previous approaches and found correlations between the valence/arousal self-assessment and the emotional data features. Finally, they found that there are seven emotional data features, such as keyboard pressure and skin conductance that can distinguish among boring, frustrating, and enjoying game modes.
Other studies have shown that it is possible to measure facial and vocal emotions with considerable reliability in real time, both separately and in combination (Bahreini et al., Citation2015, Citation2016a, Citation2016b). Taking this previous research into account, our approach will use common low-cost computer webcams and microphones rather than dedicated sensor systems for emotion detection. First, emotion detection could be used for tracking the learner’s moods during their learning, which could inform the pedagogical intervention strategies to be applied for achieving optimal learning outcomes. Second, when emotions are part of the learning content, which is the case in communication skills training, emotion recognition could be used for measuring the learners’ mastery of emotions and providing feedback. In this study, we focus on the latter usage of the facial and the vocal emotion recognition technologies. Furthermore, we will revert to the emotion classification suggested by Ekman and Friesen (Citation1978), which is widely used in psychological research and practice. This classification comprises six basic emotions: happiness, sadness, surprise, fear, disgust, and anger. In addition, we will include the complement, neutral emotion.
3. The FILTWAM framework
The FILTWAM framework enables the real-time recognition of emotions, either from facial expressions or from vocal intonations. The use of the FILTWAM framework in a learning situation is shown in . It includes five layers and a number of components within the layers. The first FILTWAM layer refers to the learner, who is the subject using the computer for accessing learning materials for personal development or preparing for an exam. The device layer reflects the equipment of the learner, whether a personal computer, a laptop, or a smart device. It is supposed to include a webcam and a microphone for collecting user data. The web interface runs a serious game (or any other online training) and allows the learner to interact with the game components. The learner will receive the feedback/content through the Internet. The web service client in the device layer uses the affective computing tool and calls the web service in the application layer. It reads the affective data and broadcasts the live stream including the facial emotion recognition expression and the vocal emotion recognition of the learner through the Internet to the web service. The affective computing tool processes facial expressions and vocal intonations data of the learner. The component “emotion recognition from facial features” extracts facial features from the face and classifies emotions. It leads to the recognition and categorization of a specific emotion. The process of emotion recognition from facial features starts at the face detection. Then, the facial feature extraction extracts a sufficient set of feature points of the learner. Finally, the facial emotion classification analyses video sequences and extracts an image of each frame for its analysis and compares the image with the data set. Its development is based on the FaceTracker software (Saragih, Lucey, & Cohn, Citation2011). It supports the classification of six basic emotions (Ekman & Friesen, Citation1978) plus the neutral emotion, but can in principle also recognize other or more detailed face expressions when required. The data layer physically stores the facial and the vocal corpus of the emotions. The network layer uses the Internet to broadcast a live stream (cut in pieces, digitized, and sent) of the learner. The application layer consists of an e-learning environment and its two subcomponents. In our case, the e-learning environment was a game. The e-learning environment uses the live stream of the facial and the vocal data of the learner to deliver new content. The web service receives emotional data from the web service client. The rules engine component in the game manages didactical rules and triggers the relevant rules for providing feedback as well as tuned training content to the learner via the device. This component uses some decision algorithms to provide feedback. At this stage, learners can receive a feedback based on their facial and vocal emotion expressions. For voice data, the process is largely similar. A detailed description for voice emotion recognition is available in a previous study (Bahreini et al., Citation2016b).
4. Methodology
The Communication Advisor is the web-based serious game that was used in our study. All participants were offered 3 rounds of the same 28 assignments within the game. The assignments were presented in the same order in each round. Each assignment required the participant to respond in a pre-defined way to a specific problem situation. This situation was briefly represented in a video clip. Participants’ manifested emotions (i.e. performance as expressed facial or vocal emotion) in response to the 28 assignments were recorded by the emotion recognition software and assessed as being correct or incorrect.
4.1. Participants
Twenty-five participants, all employees from the Welten Institute (16 males, 9 females; mean age = 44, standard deviation = 15), volunteered to participate in the study. Participants were non-actors. The participants were invited to do a communication skills training that constituted of a completion of all assignments within the Communication Advisor. By signing an agreement form, the participants allowed us to record all their data that could be gathered by the game, including their facial expressions and their vocal intonations. For participating in this experiment, no specific background knowledge was required.
4.2. Materials
The Communication Advisor is a web-based serious game for learning. It (1) deals with authentic real-life tasks (challenge and real-world relevance), (2) uses video to establish a real-life setting (context that enables transfer of learning), (3) can provide immediate and frequent feedback (guides learning), (4) offers a score mechanism (quantifiable outcomes that guide learning), and (5) presents many small assignments (challenges) that require user input (interactivity).
The Communication Advisor includes several components and a number of buttons at the graphical user interface (GUI) level. represents the main components (indicator, text description, video, task, and instruction) and the two buttons (refresh and microphone) of the Communication Advisor. , represents the main components (indicator, text description, video, task, and instruction), the feedback component, and the navigation button of the Communication Advisor. The three components on the top of are the indicator components. They simply indicate the round number, the group number, the task number within the group, and the task number of the whole tasks within each round. Every single assignment in the game included a text description of a real-life situation. These texts are included in the text description component. The video component plays a video of a communication partner related to the specific task, while the task component displays a sentence with a requested emotion that is to be expressed by the learner. The instruction component shows the learner how to proceed in the game. The refresh button will replay the video of the communication partner again. The microphone button will allow the learner to record his facial emotion expressions and his vocal emotion intonations. The feedback component gives the learner his emotional feedback based on his facial expressions and his vocal intonations. The navigation button will forward the learner to the next task. After the third round is finished, the Communication Advisor provides an overview of the learners’ performances on the rounds and tasks.
Figure 3. The game components and the navigation button in an assignment with direct feedback on vocal and facial emotions of a participant in the communication advisor.
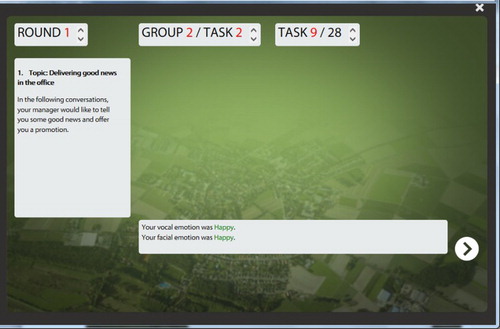
The assignments in the game aimed at helping the participants to understand and improve their facial expressions and vocal intonations. After the first round, the same assignments were then again presented in rounds 2 and 3 (cf. ). Every single assignment included a text description of a real-life situation, a video of a communication partner (see ), and a sentence with a requested emotion that was to be expressed. The participants were asked to mimic the facial expressions while looking at the communication partner, speak aloud and produce the required voice emotions. After each video, the participant was asked to deliver the pre-defined response, which was then captured and analysed by the FILTWAM software. The detected correctness (or incorrectness) of the expressed emotion was then fed back directly to the game and presented on the screen. Each assignment addressed one out of seven basic facial and vocal expressions (happy, sad, surprise, fear, disgust, anger [Ekman & Friesen, Citation1978], and neutral). The assignments covered diverse themes: an employment situation, a visit to the dentist, a visit to a restaurant, and a traffic accident. For instance, one of the assignments deals with a bad-news conversation in the employment situation: your manager tells you that you will not be promoted to a managerial position. We used transcripts and instructions for the good-news and bad-news conversations from an existing OUNL training course (Lang & Van der Molen, Citation2008) and a communication book (Van der Molen & Gramsbergen-Hoogland, Citation2005).
4.3. Design
The experiment was arranged in a within-subjects (repeated measures) design, with two experimental conditions: (1) assignments without feedback and (2) assignments with feedback. First, in a within-subjects design, all participants are exposed to all conditions, which means that differences between conditions are not blurred by individual differences in, for example, personality, acting skills, or emotional intelligence. Second, for practical reasons, the number of participants had to be restricted; therefore, it was not feasible to arrange two or more trial groups that would allow for a between groups comparison. The Communication Advisor included 3 consecutive rounds; each round (28 assignments) included 4 blocks of 7 assignments. In each of the blocks, all 7 basic emotions were addressed. displays the structure.
Given this structure, in total 84 face expressions and 84 voice intonations of each participant were gathered. The two experimental conditions are alternated across the blocks of 7 assignments: the assignments in the odd blocks are without feedback and the assignments in the even blocks are with feedback. Given the similarities across the assignments, we assume that the difficulty levels of the assignments, or rather of the blocks, are comparable, as to allow for a comparison between experimental conditions.
4.4. Procedure
Participants individually performed all assignments in a single session of about 120 minutes. There were short breaks between each round for avoiding fatigue. The sessions were conducted in a silent room with good lighting conditions. During the sessions, a moderator was present in the room. The moderator gave a short instruction at the beginning of the session and asked the participants to mimic the seven basic emotions to calibrate the face emotion recognition software. For voice emotion recognition, no such calibration was needed. The instruction included the request to show real expressions that are moderate and not too intensive. The performance was checked by face and voice emotion recognition software and assessed as being correct or incorrect. In the even blocks, the participants received direct feedback on the screen about the correctness or incorrectness of their expressed emotions (cf. ). After the sessions, the participants were asked to fill out an online questionnaire about their opinions and appreciations. Finally, the participants were requested not to talk to each other about the experiment in between sessions so that they would not influence each other.
4.5. Test environment
All assignments in the game were performed on a single web browser on a standard Windows 7 PC with integrated webcam and microphone. The EMERGO game engine was performed on a Windows server machine. The emotion recognition software applications were performed on a Mac OS computer. In principle, the experiment can also be carried out on a single computer. The data communications between the computers were performed through the web services described in the FILTWAM framework section. An external 1080HD camera was used for recording the facial and the vocal expression of the participants and their interactions with the screen. Such data were needed for post-processing by human raters (see below), in order to be able to assess the accuracy of the emotion recognition software during the sessions.
4.6. Measurement instruments
The participants’ performance levels of face and voice emotions were calculated both at the block level and round level as a percentage of correct performances. In addition, we have developed an online questionnaire to collect participants’ opinions and appreciations about the training sessions, their performances, and the feedback they received. All participants’ data were collected using a 7-point Likert scale format (1 = completely disagree, 7 = completely agree). Participants’ opinions about their assignments were gathered for: (1) difficulty to mimic the requested emotions, (2) quality of the given feedback, (3) self-confidence for being able to mimic the requested emotions, (4) clarity of the instructions, (5) attractiveness of the assignments, (6) relevancy of the assignments, (7) the GUI of the game, (8) their concentration on the given assignments, (9) their acting skills, (10) their comfortableness after receiving the feedback on their performance, (11) their preference to receive feedback on their performance by a real person instead of by a computer, (12) their trust in the judgment of a real person for giving feedback more than the judgement of the computer, and (13) usefulness of the assignments to improve their communication skills. Furthermore, two questions were asked the participants to report their opinion in the descriptive format: (14) their suggestions to use such training in a real usage context and (15) their suggestions to improve the training.
4.7. Human raters
For being able to assess the accuracy of the emotion recognition software, two expert raters individually rated the facial and the vocal emotion of the participants in the recorded video and audio files. Both raters have an academic level psychology background in emotion detection/recognition. Both raters are familiar and skilled with face, voice, and speech analysis. The same procedure as in our previous studies was followed to determine the accuracy of the emotion recognition system by the raters. Hence, the raters were asked to categorize and rate the recorded video and audio files of the participants for facial expressions and for vocal intonations. For supporting the rating process, the raters used the ELAN tool,Footnote2 which is a professional tool for making complex annotations on video and audio resources.
First, the raters received an instruction package for doing the ratings of the emotions based on recorded video and audio. Second, both raters participated in a training session where ratings of the participant were discussed to identify possible issues with the rating task and to improve common understanding of the rating categories. Third, raters assigned their individual ratings of participants’ emotions for the complete set of recorded files. Fourth, they participated in a negotiation session where all ratings were discussed to check whether negotiation about dissimilar ratings could lead to similar ratings or to sustained disagreement. Finally, the final ratings resulting from this negotiation session were contrasted with the software results for the further analysis by the main researcher. The data that the raters assigned during the initial training session were also included in the final analysis. The raters received: (1) a user manual, (2) 25 video and audio files of all the participants, (3) an instruction guide on how to use ELAN, and (4) an excel file with 25 data sheets; each of which corresponded with 1 participant. The raters rated the facial expressions and the vocal intonations of the participants in the form of categorical labels covering the six basic emotions (happiness, sadness, surprise, fear, disgust, and anger) suggested by Ekman and Friesen (Citation1978), as well as the neutral emotion.
5. Results and findings
First, we will explain the system’s reliability by contrasting the FILTWAM software output and the raters’ judgements of the participants’ performances. Then, we will present the performances of the participants in the three rounds and analyse the differences between the two conditions (feedback and no-feedback). Finally, we will present the outcomes of the questionnaire.
5.1. Raters’ results vs. FILTWAM software
5.1.1. Face
The interrater reliability of the human raters was found to yield kappa = 0.894 (p < .001). Therefore, an almost perfect agreement among human raters was obtained, a qualification that holds for kappa values larger than 0.8 (Landis & Koch, Citation1977). The overall accuracy turned out to be 90%, which confirms the high quality of raters, as from the literature we know that the accuracy of human emotion recognition is around 80% (Burkhardt, Paeschke, Rolfes, Sendlmeier, & Weiss, Citation2005). Furthermore, we contrasted the face software output and the human ratings using the raters’ agreement about the displayed emotions as a reference. Most agreement between FILTWAM and the raters is obtained for the emotion category of happiness (kappa = 0.855, p < .001) followed by neutral 0.805, anger 0.805, disgust 0.783, sadness 0.744, surprise 0.727, and fear 0.623. The overall interrater reliability between the software and the human raters was kappa = 0.776 (p < .001). According to Landis and Koch (Citation1977), this reflects substantial agreement, because kappa is between 0.6 and 0.8. The overall accuracy of the face software was 70%.
5.1.2. Voice
The interrater reliability of the two human raters was kappa = 0.881 (p < .001), which is an almost perfect agreement (Landis & Koch, Citation1977). The overall accuracy of their voice ratings was 89%. By contrasting the voice software output and the human ratings, we found that most agreement is obtained for the emotion category of anger (Kappa = 0.856, p < .001) followed by happiness 0.813, neutral 0.740, sadness 0.731, surprise 0.658, disgust 0.585, and fear 0.575. The overall interrater reliability between the software and the human raters was kappa = 0.727 (p < .001), which is to be qualified as substantial agreement (Landis & Koch, Citation1977). The overall accuracy of the voice software was 61.5%.
5.2. Performances
5.2.1. Facial expression performance
Facial performances in the three consecutive rounds are presented in .
Table 1. Means, standard deviations, and standard errors of the face performances of the participants at each round.
A repeated measures ANOVA shows that the face performances are significantly different across rounds, F(2, 48) = 6.48, p < .025. The data in show a gradual increase of performance. Pairwise comparisons between three rounds with the Bonferroni corrections show that there are significant differences between rounds 2 and 3 (p = .04), and between rounds 1 and 3 (p = .007). The required assumption of sphericity of the data was established by Mauchly’s test, x2(2) = 0.073, p = .964.
Overall, the performance of the participants showed a significant and steady increase of 11% for the facial emotion expression.
5.2.2. Vocal expression performance
We have followed the same procedure for voice as we have done for face. shows the voice performances of the participants at each round.
Table 2. Means, standard deviations, and standard errors of the voice performances of the participants at each round.
The data in show a gradual increase of voice performance. The repeated measures ANOVA shows that the voice performances are significantly different across rounds, F(1.8, 42) = 20.6, p < .025. Pairwise comparisons of the three rounds with the Bonferroni corrections show that differences between all rounds are significant: between rounds 1 and 2 (p = .007), between rounds 2 and 3 (p = .002), and between rounds 1 and round 3 (p = .001). The required assumption of sphericity of the data was established by Mauchly’s test, x2(2) = 5.562, p = .062. The entire performance of the participants showed a significant and steady increase of 33% for the vocal emotion expression.
5.2.3. Facial feedback versus no-feedback
We aggregated the even blocks in each round to calculate the average performance in the feedback condition per round. Likewise, the odd blocks were used to calculate the average performance in each round in the no-feedback condition. represents the average facial performances in the three rounds, the no-feedback condition and the feedback condition.
Overall, the figure suggests a better performance in the feedback condition as compared to the no-feedback condition. However, the error bars are substantial. Paired-samples t-tests between the two conditions for each round did not reveal significant differences. Likewise, the overall performances in three combined rounds did not show significant differences between the no-feedback and the feedback data.
5.2.4. Vocal feedback versus no-feedback
Paired-samples t-tests, between the no-feedback and feedback conditions in each round, show that vocal performances differ significantly (see ). The data show a significantly higher vocal performance in the feedback condition. The data also suggest a faster growth of performance in the feedback condition.
Table 3. Paired-samples t-tests between the no-feedback and feedback conditions for voice performance.
5.2.5. Facial performance growth across rounds
Repeated measures ANOVA for the no-feedback condition between rounds did not show significant differences, that is, the performance growth in the no-feedback condition cannot be confirmed (see ).
Table 4. Means and standard errors of the face performances of the participants at each round for no-feedback condition.
In the feedback condition, however, we found a significant result (F(5.4), p = .005). Paired-samples t-test showed that the observed facial performances in rounds 1 and 3 are significantly different, growing from 0.64 to 0.72 (p = .02) ().
Table 5. Means and standard errors of the face performances of the participants at each round for feedback condition.
5.2.6. Vocal performance growth across rounds
A comparison of vocal performances between rounds shows significant results for both conditions. In the no-feedback condition, we found a significantly statistical performance growth between all rounds (see ). In round 2, performance goes up from 0.45 to 0.51 (p = .04); in round 3 performance rises to 0.58 (p = .01).
Table 6. Means and standard errors of the voice performances of the participants at each round for no-feedback condition.
For the feedback condition, we also found significant differences between all rounds. shows the vocal performance growth in round 2 from 0.50 to 0.60 (p = .002), and in round 3 from 0.60 to 0.67 (p = .007).
Table 7. Means and standard errors of the voice performances of the participants at each round for feedback condition.
It should be noted that, although the Bonferroni corrections have been applied throughout the analysis, the repeated use of the dataset in Sections 5.2.1, 5.2.3, 5.2.5 and 5.2.2, 5.2.4, 5.2.6, respectively, would require additional corrections for the significance threshold (0.017 rather than 0.05). Although few of the results are then disqualified, the overall trends and conclusions persist.
5.3. Post-practice questionnaire
We follow Norman’s (Citation2010) approach to allow parametric statistics for the ordinal Likert scale data. This approach quantifies Likert scale scores, be it conditional to normality checks, and allows the scores to be represented with the arithmetical mean and standard deviation, respectively. We transformed our 7-point Likert data into a linear metric at the interval [0.0, 1.0], with the value of 0.5 as the reference of a neutral response.
First, participants did not consider themselves as particularly good actors (mean = 0.39; standard deviation = 0.30). With respect to the experimental conditions, they were very positive about the quality of the instructions (mean = 0.88; standard deviation = 0.22), the user interface (mean = 0.90; standard deviation = 0.20), and the arrangement of the experiment (mean = 0.78; standard deviation = 0.18). With respect to the communication training, participants were moderately positive about the attractiveness of assignments (mean = 0.66; standard deviation = 0.20), the appropriateness of the assignments for training the communication skills (mean = 0.61; standard deviation = 0.17), the relevancy of contents (mean = 0.64; standard deviation = 0.21), the confidence to mimic the requested emotions (mean = 0.60; standard deviation = 0.22), the helpfulness of the feedback (mean = 0.67; standard deviation = 0.23), and the comfortability while receiving feedback (mean = 0.69; standard deviation = 0.27). They reported that they were neutral about the ease of mimicking requested emotions (mean = 0.53; standard deviation = 0.23), trusting human judgments better or worse than judgements by the computer (mean = 0.53; standard deviation = 0.25), and receiving feedback from a real person or a computer (mean = 0.56; standard deviation = 0.22).
6. Discussion
In a within-subjects experiment, it was investigated whether feedback on the mimicked emotions would lead to better learning. Facial performance growth during the game was found to be positive, particularly significant in the feedback condition. The vocal performance growth was significant in both conditions, while the growth is stronger when feedback is provided. The performance of the participants showed a significant and steady increase of 11% for facial emotion expression and 33% for vocal emotion expression. This establishes the game’s role as a tool for learning. These results suggest a positive contribution of the automated feedback to the process of mastery.
A principal requirement for the successful use of emotion recognition software is its accuracy. While using the judgement of human raters as a reference, the accuracies of our emotion recognition software turned out to be 70% for facial emotions and 61.5% for vocal emotions. Although these accuracies are lower than those of the human raters involved (90% for facial emotions and 89% for vocal emotions), the result is consistent with previous studies (Bahreini et al., Citation2016a, Citation2016b; Busso, Deng, & Yildirim, Citation2004; Jaimes & Sebe, Citation2007). One may wonder if the FILTWAM emotion recognition software will work better for some emotions than for other ones. This is actually a follow-up research question that is beyond the scope of this study.
For investigating the impact of automated feedback on performance, we compared the performances in the feedback condition with those in the no-feedback condition in each of the rounds. For facial emotions, the performances were found to be systematically higher in the feedback condition, but the differences with the no-feedback condition were not statistically significant. A similar pattern was found for vocal emotions, be it that the differences were statistically significant. The vocal performances of participants were found to be significantly higher in the feedback condition as compared to the no-feedback condition. These results suggest a positive contribution of the automated feedback to the process of mastery.
In both conditions, the facial performance growth across rounds was found to be positive, be it only significant in the feedback condition. The vocal performance growths across rounds are significant in both conditions: also the growth is stronger when feedback is provided. Again this is a significant indication of the effectiveness of automated feedback.
As can be concluded from the above, both feedbacks on facial emotion expression and on vocal emotion expression result in increased performances. Yet, in some cases, particularly regarding facial emotions, we were not able to demonstrate significant effects. Although the total number of observations was high (2100 facial and 2100 vocal), the sample size of participants was only 25. A larger sample could have demonstrated more significant impact, but this was not feasible within the context of this study.
Although the 28 assignments share the same setup, a block-wise calculation of performance scores (each round comprises 4 blocks) shows a jagged pattern rather than a gradual increase (cf. ). This may be an indicator of unequal complexity of the assignments, which would affect the validity of the study, while favouring one condition above the other.
In a separate analysis, we have cancelled out the effects of potential complexity differences of the assignments by using the four blocks of round 1 as a reference, that is, the performance in the blocks of rounds 2 and 3 are presented as a measure relative to performances of the corresponding blocks in round 1. shows the relative performances of both face emotions and voice emotions.
Although in both graphs of the curves are slightly smoothened, the variability remains considerable. Statistical comparison of the no-feedback and the feedback conditions in each round did not produce significant results. Hence, correcting for complexity differences of assignments does not improve the outcomes.
As a general trend, the data suggest that vocal emotion performance is structurally better that facial emotion performance. As a first explanation, this may be accounted to the better accuracy of the vocal emotion recognition software: indeed, receiving correct feedback may be expected to help improve performance. Another explanation may be in the intrinsic textual setup of the assignments: reading out aloud pre-defined texts is a quite artificial task, which leaves little room for free (facial) expression, while in contrast the vocal expression is less restricted and would allow for more spontaneous performances. So far, these explanations are suppositions that could not be verified.
The results of the questionnaire indicate that the participants were ready to use a serious game to improve their communication skills instead of taking lessons from a human teacher. The results indicate that the participants were moderately positive about the helpfulness of the feedback. The participants’ scores for attractiveness, appropriateness, and contents of the assignments indicate room for improvement of the Communication Advisor.
The findings obtained from this study could affect educational practice in various respects. First, it enables reliable real-time emotion detection and adaptation in e-learning. Second, it can cope with the fact that teachers are not always available to monitor the progress of learners towards mastery, for example in the case of communication skills training. Third, the findings seem to indicate that prolonged practice without loss of motivation is possible. Finally, the findings indicate that it is possible to integrate the Communication Advisor with ITS to achieve an ATS-based serious game environment.
In addition, the post-practice questionnaire indicates some limitations of our study that imply challenges for future research. Further improvements are possible in the area of technology development, game development, more reliable systems, and more accurate software applications. The accuracy of emotion recognition can be further improved by combining other sensory data to the FILTWAM framework. Further improvements are required to extend the FILTWAM framework for more reliable and mature exploitation of real-time emotion recognition technologies in e-learning. This would offer an innovative approach for applying emotion recognition in affective e-learning (Bahreini et al., Citation2016a; Sebe, Citation2009). New software applications and serious games with emotion recognition technology could strongly influence e-learning and gaming. The current feedback mechanism in the Communication Advisor is sufficient, but restricted; therefore, an improved feedback mechanism is suggested. The feedback mechanism should offer a solution, by which the learner can learn how to correct his/her mistaken facial and vocal emotional expressions. The feedback mechanism should preferably include or refer to e-learning materials (e.g. text, photos, audios and videos, and animations).
Furthermore, more ecologically valid circumstances are needed to test similar systems like the Communication Advisor with a sufficient number of participants so as to achieve full-fledged serious games. Besides the aforementioned technical challenges, legal issues, ethical issues, and essentially psychological issues also pose substantial challenges. We know that the relationship between emotion and learning is a highly complex relationship when it concerns human learning (Bower, Citation1981; Schwarz, Citation1990). The full integration and exploitation of emotion recognition techniques in e-learning environments deserve extensive investigation of that relationship.
7. Conclusion
The research presented in this study has shown that facial and vocal emotion recognition software can be successfully used in a serious game for communication skills training. The results indicate that, when learners repeatedly receive feedback on their assignments, their performances will improve possibly faster (as in the case of voice emotion) than when no feedback is given.
The FILTWAM emotion recognition technology connected to the Communication Advisor allowed providing real-time feedback on the learners’ facial and vocal emotion expression performances. Although FILTWAM was using domestic devices (standard webcam and microphone), its accuracy was sufficient for guiding learners to better perform. Herewith the study provides a proof of concept that would allow for a wider use of the approach. Although we have considered only seven basic emotions in this study, the FILTWAM framework can be easily extended to include more detailed emotion categories. In principle, the successful validation of FILTWAM paves the way for a structural inclusion of affective computing technologies in electronic learning environments.
Acknowledgements
We thank our colleagues at the Welten Institute of the Open University Netherlands who participated in this study. We likewise thank the two raters who helped us to rate the recorded video files. We are grateful to Hub Kurvers for designing the Communication Advisor serious game in the EMERGO environment, to Charlotte Wolff for revision of the game contents, to Jeroen Berkhout for producing the image contents and recording the video files, to Marcel Vos and Mat Heinen for technical support for video recording, to Jason Saragih and his colleagues for permission to develop the facial emotion recognition software application based on their FaceTracker software (Saragih, Lucey, & Cohn, 2011), to Setareh Habibzadeh for data entry and data visualization, and to Mieke Haemers for proofreading the manuscript.
Disclosure statement
The authors have no potential conflict of interest to declare.
Notes on contributors
Kiavash Bahreini is a computer scientist with an interest in affective computing, human computer interaction, machine learning, real-time applications, data analytics, and e-learning applications; he is a Post Doctoral researcher in the Welten Institute, Research Centre for Learning, Teaching and Technology at the Open University of the Netherlands.
Rob Nadolski is an educational technologist with an interest in enhancing learner support facilities, e-learning applications, complex cognitive skills; he is an Assistant Professor in the Welten Institute, Research Centre for Learning, Teaching and Technology at the Open University of the Netherlands.
Wim Westera is an educational media researcher with an interest in serious gaming and simulation; he is a Full Professor in the Welten Institute, Research Centre for Learning, Teaching and Technology at the Open University of the Netherlands.
Additional information
Funding
Notes
References
- Abt, C. (1970). Serious games. New York, NY: Viking Press.
- Bahreini, K., Nadolski, R., & Westera, W. (2014, September 16–19). Multimodal emotion recognition for assessment of learning in a game-based communication skills training. In For and In Serious Games, Joint workshop of the GALA network of excellence and the LEA’s BOX Project at EC-TEL 2014, Graz, Austria, pp. 22–25.
- Bahreini, K., Nadolski, R., & Westera, W. (2015). Improved multimodal emotion recognition for better game-based learning. In A. De Gloria (Ed.), Games and learning alliance (pp. 107–120). Springer. doi:10.1007/978-3-319-22960-7_11
- Bahreini, K., Nadolski, R., & Westera, W. (2016a). Towards multimodal emotion recognition in e-learning environments. Interactive Learning Environments, 24(3), 590–605. doi:10.1080/10494820.2014.908927
- Bahreini, K., Nadolski, R., & Westera, W. (2016b). Towards real-time speech emotion recognition for affective e-learning. Education and Information Technologies, 21(5), 1367–1386. doi:10.1007/s10639-015-9388-2
- Ben Ammar, M., Neji, M., Alimi, A. M., & Gouardères, G. (2010). The affective tutoring system. Expert Systems with Applications, 37(4), 3013–3023. doi:10.1016/j.eswa.2009.09.031
- Bower, G. H. (1981). Mood and memory. American Psychologist, 36, 129–148. doi: 10.1037/0003-066X.36.2.129
- Brantley, C. P., & Miller, M. G. (2008). Effective communication for colleges. Mason: Thomson Higher Education.
- Burkhardt, F., Paeschke, A., Rolfes, M., Sendlmeier, W., & Weiss, B. (2005). A database of German emotional speech. Proceedings of the inter speech, Lissabon, pp. 1517–1520.
- Busso, C., Deng, Z., & Yildirim, S. (2004, October 13–15). Analysis of emotion recognition using facial expressions, speech and multimodal information. Proceedings of the 6th international conference on multimodal Interfaces, ICMI 2004, State College, PA, USA. ACM.
- Cantillon, P., & Sargeant, J. (2008). Giving feedback in clinical settings. BMJ, 337(a1961). doi:10.1136/bmj.a1961
- Connolly, T. M., Boyle, E. A., MacArthur, E., Hainey, T., & Boyle, J. M. (2012). A systematic literature review of empirical evidence on computer games and serious games. Computers & Education, 59(2), 661–686. doi:10.1016/j.compedu.2012.03.004
- Ekman, P., & Friesen, W. V. (1978). Facial action coding system: Investigator’s guide. Douglas, AZ: A Human Face.
- Feidakis, M., Daradoumis, T., & Caballe, S. (2011). Emotion measurement in intelligent tutoring systems: What when and how to measure (pp. 807–812). Third international conference on intelligent networking and col-laborative systems, Fukuoka, Japan.
- Hager, P. J., Hager, P., & Halliday, J. (2006). Recovering informal learning: Wisdom judgment and community. Lifelong learning book series. Dordrecht: Springer.
- Hyunjin, Y., Sang-Wook, P., Yong-Kwi, L., & Jong-Hyun, J. (2013, October). Emotion recognition of serious game players using a simple brain computer interface. International Conference on ICT Convergence (ICTC), pp. 783–786. doi:10.1109/ICTC.2013.6675478
- Jaimes, A., & Sebe, N. (2007). Multimodal human–computer interaction: A survey, computer vision and image understanding. Special Issue on Vision for Human-Computer Interaction, 108(1–2), 116–134.
- Kramer, A. F. (1991). Physiological metrics of mental workload: A review of recent progress. In D. L. Damos (Ed.), Multiple-task-performance (pp. 329–360). London: Taylor & Francis.
- Landis, J. R., & Koch, G. G. (1977). The measurement of observer agreement for categorical data. Biometrics, 33, 159–174. doi: 10.2307/2529310
- Lang, G., & Van der Molen, H. T. (2008). Psychologische gespreksvoering book. Heerlen: Open University of the Netherlands.
- Leventhal, H. (1984). A perceptual motor theory of emotion. In K. R. Scherer & P. Ekman (Eds.), Approaches to emotion (pp. 271–291). Hillsdale, NJ: Lawrence Erlbaum Associates.
- Norman, G. (2010). Likert scales, levels of measurement and the “laws” of statistics. Advances in Health and Science Education, 15(5), 625–632. doi:10.1007/s10459-010-9222-y
- Pavlas, D. (2010). A model of flow and play in game-based learning: The impact of game characteristics, player traits, and player states (Ph.D. dissertation). University of Central Florida, Orlando, FL.
- Pekrun, R. (1992). The impact of emotions on learning and achievement: Towards a theory of cognitive/motivational mediators. Journal of Applied Psychology, 41, 359–376. doi:10.1111/j.1464-0597.1992.tb00712.x
- Psotka, J., & Mutter, S. A. (1988). Intelligent tutoring systems: Lessons learned. Hillsdale, NJ: Lawrence Erlbaum Associates.
- Rodriguez, H., Beck, D., Lind, D., & Lok, B. (2008). Audio analysis of human/virtual-human interaction. In H. Prendinger, J. C. Lester, & M. Ishizuka (Eds.), IVA 2008. LNCS (LNAI), 5208 (pp. 154–161). Heidelberg: Springer.
- Saragih, J., Lucey, S., & Cohn, J. F. (2011). Deformable model fitting by regularized landmark mean-shift. International Journal of Computer Vision (IJCV), 91(2), 200–215. doi: 10.1007/s11263-010-0380-4
- Sarrafzadeh, A., Alexander, S., Dadgostar, F., Fan, C., & Bigdeli, A. (2008). How do you know that I don’t understand? A look at the future of intelligent tutoring systems. Computers in Human Behavior, 24(4), 1342–1363. doi:10.1016/j.chb.2007.07.008
- Schwarz, N. (1990). Feeling as information. Informational and motivational functions of affective states. In E. T. Higgins & R. Sorrentino (Eds.), Handbook of motivation and cognition. Foundations of social behavior (pp. 527–560). New York, NY: Guilford Press.
- Sebe, N. (2009). Multimodal interfaces: Challenges and perspectives. Journal of Ambient Intelligence and Smart Environments, 1(1), 23–30.
- Tijs, T., Brokken, D., & IJsselsteijn, W. (2009). Creating an emotionally adaptive game. In S. M. Stevens & S. Saldamarco (Eds.), Entertainment computing - ICEC 2008. 7th international conference, Pittsburgh, PA, USA, September 25–27, 2008, Proceedings (pp. 122–133). Berlin: Springer.
- Van der Molen, H. T., & Gramsbergen-Hoogland, Y. H. (2005). Communication in organizations: Basic skills and conversation models. New York, NY: Psychology Press.
- Van Eck, R. (2010). Interdisciplinary models and tools for serious games: Emerging concepts and future directions. Hershey, PA: IGI Global.
- Vorvick, L., Avnon, T., Emmett, R., & Robins, L. (2008). Improving teaching by teaching feedback. Medical Education, 42, 540–543. doi: 10.1111/j.1365-2923.2008.03069.x
- Wallbott, H. G. (1998). Bodily expression of emotion. European Journal of Social Psychology, 28(6), 879–896. doi: 10.1002/(SICI)1099-0992(1998110)28:6<879::AID-EJSP901>3.0.CO;2-W
- Westera, W. (2013). The digital turn. How the internet transforms our existence. Bloomington, IN: Author house.
- Westera, W., Nadolski, R., Hummel, H. G. K., & Wopereis, I. (2008). Serious games for higher education: A framework for reducing design complexity. Journal of Computer Assisted Learning, 24(5), 420–432. doi: 10.1111/j.1365-2729.2008.00279.x
- Wouters, P., Van Nimwegen, C., Van Oostendorp, H., & Van der Spek, E. D. (2013). A meta-analysis of the cognitive and motivational effects of serious games. Journal of Educational Psychology, 105(2), 249–265. doi: 10.1037/a0031311
- Wu, C. H., Huang, Y. M., & Hwang, J. P. (2015). Review of affective computing in education/learning: Trends and challenges. British Journal of Educational Technology. Advance online publication. doi:10.1111/bjet.12324
- Zhang, T., Hasegawa-Johnson, M., & Levinson, S. E. (2003). Mental state detection of dialogue system users via spoken language. ISCA/IEEE workshop on spontaneous speech processing and recognition (SSPR), Kyoto, Japan.