Abstract
Ruminant animals, such as dairy cattle, produce CH4, which contributes to global warming emissions and reduces dietary energy for the cows. While the carbon foot print of milk production varies based on production systems, milk yield and farm management practices, enteric fermentation, and manure management are major contributors togreenhouse gas emissions from dairy cattle. Recent emerging evidence has revealed the existence of genetic variation for CH4 emission traits among dairy cattle, suggests their potential inclusion in breeding goals and genetic selection programs. Advancements in high-throughput sequencing technologies and analytical techniques have enabled the identification of potential metabolic biomarkers, candidate genes, and SNPs linked to methane emissions. Indeed, this review critically examines our current understanding of carbon foot print in milk production, major emission sources, rumen microbial community and enteric fermentation, and the genetic architecture of methane emission traits in dairy cattle. It also emphasizes important implications for breeding strategies aimed at halting methane emissions through selective breeding, microbiome driven breeding, breeding for feed efficiency, and breeding by gene editing.
Introduction
The world’s 1.4 billion cattle population both contributes to the agricultural greenhouse gas (GHG) emissions and suffers from high ambient temperatures combined with humidity.Citation1,Citation2 With the ongoing global population growth, increasing income levels, and urbanization, there is an anticipated rise in the demand for animal products. The projected increases in demand for various animal products necessitate a corresponding increase in production efficiency for animal species. Despite improvements in production efficiency, the commensurate increase in GHG emissions from livestock systems remains a significant challenge.Citation3 The Intergovernmental Panel on Climate Change (IPCC) highlighted the substantial contribution of agriculture, predominantly by livestock, to the increase in atmospheric CH4 emissions.Citation4 Among the livestock species, cattle are the primary contributor to GHG emissions, accounting for ∼62% of all livestock emissions.Citation3 When considering emission sources within the livestock sector, the emissions of CH4 from enteric fermentation account for more than half (54%) of total (78%) livestock emissions, of which, cattle contribute approximately three-quarter (76%) of the GHG emissions from enteric fermentation as well as around one-third (33%) from manure management.Citation3 Each cow generates between 70 and 120 kg of CH4 annually.Citation5
The potential consequences of CH4 emissions from ruminants pose significant concerns for both environmental sustainability and energy efficiency in the dairy industry. To address this issue, various strategies have been proposed to reduce ruminant CH4 emissions, including adjusting management practices and implementing nutritional treatments.Citation6 However, these approaches are usually not cost-effective for farmers and can have a significant impact on CH4 production, thus, finding an effective and sustainable solution to the problem remains challenging.Citation7,Citation8 Genetic selection for low CH4-emitting animals, particularly dairy cows, is proposed as the best mitigation strategy that is cost-effective, permanent, and cumulative across generations.Citation9–11 However, the success of this approach relies on the existence of genetic variation in CH4 production and favorable genetic associations of CH4 traits with other breeding goal traits.Citation7 Several studies have reported the genetic control of CH4 emission traits and the existence of sizable genetic variation in CH4 emission among dairy cattle.Citation9,Citation12–16 While, methane emission traits in dairy cattle, such as daily methane production, methane yield, and methane intensity, are heritable and can be targeted through genetic selection programs aimed at mitigating GHG emissions,Citation7,Citation17 it is crucial to acknowledge that multiple genes contribute to the phenotype, making it a complex trait. Consequently, genetic progress through conventional genetic selection methods is slow. Therefore, gaining a better understanding of the genetics involved in CH4 emissions can generate valuable insights for genomic prediction and achieve rapid genetic progress for reduction in CH4 emission in dairy cattle. This prompts the need to identify genomic variants associated with different CH4 emission traits, thus contributing an additional feature in the headway of genomic selection of low CH4 emitter dairy cows. In this regard, genome-wide association studies (GWAS) have identified several genomic regions and variants affecting CH4 emission traits in dairy cattle, thus providing an additional attribute to improve genetic gain in the process of genomic selection.Citation7,Citation11,Citation18,Citation19 Since genome-wide significant single nucleotide polymorphisms (SNPs) have become affordable, genomic selection has been tested and would be of great value for selecting low emitter dairy animals with a desirable genetic makeup, as they would facilitate the selection of true causal variants and genes controlling complex traits. However, the economic value of an identified quantitative trait nucleotide (QTN) in the selection program will depend on the effects of the QTN on the traits included in the selection index, gene interactions, interactions of the QTL with other genes, and the frequency of the QTL alleles in the commercial population.Citation20 Indeed, this review aims to undertake a comprehensive search for the carbon foot print (CF) of milk production, major emission hotspots, rumen microbiota and enteric methane emissions, and methane reducing cow feed additives. Additionally, it explores the genetic basis of CH4 emission traits in dairy cattle. Given its significance for future breeding programs, a holistic breeding strategy to halt CH4 emissions in dairy cows are also discussed.
Carbon footprint of milk production in dairy cattle
The carbon footprint (CF) is an environmental indicator, that measures the direct and indirect GHG emissions released into the atmosphere during the entire life cycle of a product within the production chain.Citation21 It is typically expressed as kilogram of CO2 equivalent (CO2e) per kilograms of product.Citation22 To develop effective GHG mitigation strategies for milk production, it is important to understand the CF in terms of CO2e per kilogram of fat and protein corrected milk (CO2e/kg FPCM) specific to different regions.
Several studies () have suggested that the major GHG contributing to the CF of milk on a dairy farm are CH4 from enteric fermentation and nitrous oxide from manure management and feed production.Citation23–26,Citation29,Citation34,Citation36,Citation40 It is worth mentioning that, the estimated emissions for producing 1 kg of fat and protein corrected milk (FPCM) amounted to 1.23 kg of CO2e, with enteric methane accounting for 46% and manure management accounting for 32.7% of these emissions.Citation23 In countries where milk production is predominantly based on pasture systems, over 50% of the CF can be attributed to CH4 emissions from enteric fermentation.Citation29 Gollnow et al.Citation39 reported that the largest share of the CF comes from enteric fermentation (57%) and manure (9%) deposited directly into pastures during grazing. Furthermore, Jayasundara et al.Citation31 claim that enteric fermentation (44%) and the production and supply of feed (36%) contribute the most to the CF of milk.
Table 1. Summary of recent studies on carbon footprint (CF) and milk production in dairy cattle.
It is evident that CH4 emission intensity varies between production systemsCitation27,Citation29,Citation40,Citation41 and per kg output (FPCM) per lactationCitation25 and farm management practices.Citation24,Citation30 According to Gerber et al.,Citation42 GHG emissions increase with higher yield, but emissions per kg FPCM decrease significantly as animal productivity increases. It is shown that the CF of milk production is more than 2-fold higher when milk yield is below 3500 kg a year per lactating cow.Citation25 Depending on the production system, CF for milk has been estimated to be as low as 0.75 and as high as 1.21, and even over 5.0 kg of CO2e/kg energy corrected milk (ECM) or FPCM.Citation29,Citation41 Likewise, the CF of milk ranged from 0.74 (New Zealand) to 5.99 (Tanzania) kg CO2e per kg−1 FPCM.Citation29 In the Peruvian Amazon, silvo-pastoral dairy production systems have an average milk CF of 2.26 kg CO2e/kg FPCM,Citation26 while smallholder dairy farms in Ethiopia have a milk CF of 2.35 kg CO2e/kg FPCM.Citation27 A more recent investigation revealed that housed-farms exhibited elevated CF compared to mixed and grazing farms, with farm management practices accounting for up to 79% of the variability in CF.Citation24 In this context, increasing the length of the grazing season and improving annual milk production per hectare and per cow can reduce the CF of milk and increase farm profit.Citation43
Nevertheless, promoting balanced feed rations and adjusting concentrate feeding depending on cows’ needs throughout the lactation cycle is suggested as an alternative strategy to increase milk production and reduce CF per milk output on smallholder farms.Citation32 It is worth noting that farms with superior feed quality, higher production levels, and a greater percentage of lactating animals tended to have lower milk CF values (1.76 vs. 3.09 kg CO2e/kg FPCM).Citation26 On the other hand, improving milking cow productivity and increasing the proportion of milking cows, as well as implementing various manure management systems and encouraging dairy farmers to return manure to nearby crop lands, were identified as promising measures to decrease environmental impacts.Citation37 Therefore, farm systems that optimize milk production have the lowest environmental impact.Citation34 It has been determined that the calculated emissions of CO2e per kg of FPCM produced vary between 0.8 and 3.5 kg CO2e/kg FPCM (). Different production systems and feeding strategies exert diverse impacts on the CF, where intensive systems exhibit elevated emissions compared to pasture-based or smallholder farms (). Summarized studies focusing on CF and milk production in dairy cattle have pinpointed enteric fermentation, manure management, and feed production as primary contributors to emissions (). Within this framework, the proportionate contribution of various emission sources varies between countries, with enteric fermentation emerging as a significant contributor in the majority of cases. Overall, these studies underscore the need for implementing sustainable practices in dairy cattle production to curtail carbon emissions and alleviate environmental repercussions.
Genetic basis of methane emission traits in dairy cattle
Methane emission traits are partially influenced by genetics, with the surrounding environment being the most important factor.Citation10 Genetic selection for traits related to CH4 emission has the potential to bring about permanent and cumulative changes. The literature () highlights the presence of sizable genetic variation in CH4 emission traits among dairy cattle, offering valuable insight for inclusion in genetic selection programs.Citation12–14,Citation44,Citation52 One way to evaluate the nature and extent of genetic variation for methane emissions is to genetically evaluate traits, such as CH4 intensity (MeI: methane per unit of product), CH4 yield (MeY: methane per unit of feed intake), CH4 production (MeP: the total amount of CH4 emitted by cows in grams per day), and residual methane traits corrected for dry matter intake and milk yield in dairy cattle.
Table 2. Summary of recent studies on estimates of heritability for methane emission traits in dairy cattle.
In an attempt to perform a meta-analysis to estimate genetic parameters for methane emission traits (MeY, MeI, and MeP), Hossein-ZadehCitation44 confirmed the presence of modest genetic variation for methane emission traits with heritability estimates ranging from 0.180 to 0.244 in dairy cows. Recently, Richardson et al.Citation53 reported heritability estimates of 0.16 for MeP, 0.23 for MeY, and 0.33 for MeI in Australian Holstein cows. Similarly, Lassen and LøvendahlCitation13 reported a heritability of 0.21 for both CH4 production and CH4 intensity recorded over a week in Danish Holstein cows from multiple commercial herds. However, Pszczola et al.Citation15 reported higher heritability estimates for daily MeP with values ranging from 0.23 to 0.30 across lactation in two herds. Using longitudinal data, Difford et al.Citation54 reported heritability estimates that varied from low (0.15) to moderate (0.26) for MeC in Dutch and Danish Holstein cows, respectively. In contrast, Manzanilla-Pech et al.Citation19 reported heritability estimates of 0.14 for MeC and 0.15 for MeP in Danish Holstein population. Furthermore, heritability estimates of 0.12 were reported for CH4/CO2Citation50 and methane concentrationCitation48 in dairy cattle. For various definitions of residual methane, Richardson et al.Citation53 reported heritability estimates ranging from 0.11 to 0.21 in Australian Holstein cattle. Considering predicted methane yields as CH4 traits, heritability estimates varied from 0.12 to 0.44 in Dutch Holstein-Friesian cows, thus, indicating that response to selection would be expected to reduce predicted CH4 emission (PME).Citation55 Kamalanathan et al.Citation17 reported heritability estimates of 0.16 for daily MeP, 0.27 for daily MeY, and 0.21 for daily MeI in dairy cattle, indicating that there is room to reduce CH4 emission in Holstein cattle through genetic selection.
On the other hand, there is evidence of genetic control of microbiota composition through changes in physical and physiological conditions in the rumen that promote the growth of specific microbes.Citation13,Citation56 It is of note that, the specific functional capacity of the ruminal microbiome is heritable with heritability estimates ranging between 0.13 and 0.61 for the comprehensive set of microbial genes involved in a variety of CH4 metabolism pathways and ribosomal biosynthesis.Citation57 Likewise, heritability estimates for the relative abundance of rumen microbes ranged between 0.08 and 0.48,Citation48 which opens up opportunities to consider a new source of genetic variation that could be utilized in the dairy cattle genetic selection program. This suggests that gaining insights into the genetic basis of the microbiota and its composition with CH4 emissions holds great importance.
When considering genetic correlations, it is important to note that traits like milk production, composition, body weight, and health status of cows are genetically linked to CH4 production.Citation7 Previous studies have shown unfavorable associations between CH4 production and milk production,Citation13,Citation44,Citation54,Citation58 dry matter intake,Citation54,Citation58 and residual feed intakeCitation12 in dairy cattle. Therefore, caution should be advised when selecting for reduced CH4 production to avoid a decrease in milk yield. Several other studies have examined correlations between CH4 emissions and other breeding goal traits, and have found that selecting for reduced CH4 emissions is likely to have minimal consequences on other traits, such as reproduction and health.Citation7,Citation59
Diet, rumen microbial community, and emissions from enteric methane in dairy cattle
It is widely acknowledged that diet plays a significant role in shaping the composition of the rumen microbial community.Citation60–62 In ruminants, the rumen, one of their stomachs, stands out for harboring a diverse community of bacteria, ciliate protozoa, and anaerobic fungi that work together to break down feed material (). In this process, complex dietary carbohydrates, proteins, and lipids are converted into volatile fatty acids (VFAs), microbial proteins and vitamins, while CO2, H2, and other compounds are released.Citation57,Citation63 Among the VFAs, acetate and butyrate are produced through methanogenic fermentation, which spares H2, while propionate is glucogenic, utilizes H2 during enteric fermentation.Citation64–68 This indicates that increased acetate and butyrate production during enteric fermentation leads to higher production of H2 and CO2 by methanogenic bacteria that cannot be utilized by dairy cows, as a result, CH4 is generated as a by-product, which the cow expels through eructation.Citation69 Eructated CH4 not only significantly contributes to global warming, but also represents a 5–7% loss of dietary energy.Citation70 It is estimated to account for between 2% and 12% of net energy intake, which has a profound impact on dairy cattle productivityCitation12. As suggested by Gerber et al.,Citation42 when low-quality feed spends a long time in the rumen, it leads to high enteric methane emission and emission intensity, resulting in low animal productivity. For instance, the inclusion of a high percentage of forage in the ration (58.6%) explains the relatively increased emissions from enteric fermentation.Citation23 Therefore, changes in feeding practices, such as the use of concentrates and high-quality fodders like legumes and high-starch forages, have been associated with reduced CH4 emission in ruminant digestive systems.Citation71,Citation72 Indeed, the intricate relationship between rumen microbiota, their functional composition and H2 is further influenced by various factors, such as diet, host genetics, physiological status, dietary composition, and environmental conditions.Citation61,Citation73 These factors are crucial in determining animal performance and efficiency.Citation74
Figure 1. An overview of animal feed, rumen microbiota and enteric methane emissions in dairy cattle.
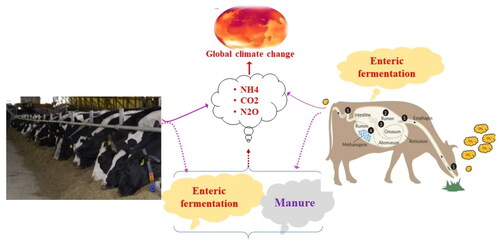
Implementing balanced feeding strategies to reduce CH4 emissions from dairy cows is of great importance from both an environmental and economic perspective. One way to achieve this is improving the efficiency of dairy cattle in converting feed to milk, rather than wasting energy as enteric methane.Citation11 It is important to note that, altering the diets fed to ruminants leads to changes in CH4 emissions and alterations in the VFA proportions.Citation75 In this context, modulating the rumen microbiome to improve feed efficiency and reduce enteric CH4 emissions from ruminants in general and dairy cattle in particular, without altering other traits, such as production and health is desirable as a strategy to decarbonize the dairy industry and improve its productive efficiency.
Rumen metabolites, such as VFAs indicators, have the potential to be used as proxies (indirect indicators/traits) for CH4 emissions, mostly because the fermentation of feed in the rumen into VFAs is connected to the release of H2.Citation76 Diets that promote propionate dominated fermentation in the rumen have been linked to decreased CH4 emissions, while diets that favor acetate/butyrate dominated fermentation are associated with increased CH4 production.Citation77 On the other hand, supplementing ruminants with dietary lipids can act as a toxic agent against methanogens and protozoa, leading to a change in the rumen environment, resulting in increased propionate production, and reduced enteric CH4 emissions.Citation71 Adding lipids to the diet can reduce absolute CH4 emissions by 19%.Citation3 It is important to note that diet composition and dry matter intake are key factors that explain the variation in CH4 emissions. Among the main VFAs, propionate is generally associated with diets that have lower CH4 emissions, while butyrate and acetate are correlated with diets that have higher CH4 yield.Citation67 CH4 emissions are also generally related to the ratio of acetate to propionate (A:P), with decreasing CH4 production associated with a decreasing A:P ratio.Citation67 Numerous studies have also shown that lowering the dietary CP content resulted in a significant reduction in N2 excretion of dairy cattle, which subsequently lowers ammonia (NH3) and nitrous oxide (N2O) emissions related to energy-corrected milk and DM intake.Citation78–81 Therefore, comprehensive feeding strategies, such as begin-of-pipe feeding mitigation measures must be developed and implemented to mitigate the harmful effects of GHG emissions.Citation81 In this regard, efforts are underway to develop and implement the possibilities of CP-optimized feeding strategies for dairy cows.Citation82 While Alfalfa is widely recognized as the predominantly used forage crop for dairy cattle and is considered a substantial carbon sink owing to its high productivity, extensive root systems, and nitrogen-fixation capacity, the emissions N2O can notably diminish its potential in mitigating climate change.Citation83
Methane-reducing cow feed additives
Several novel and interesting feed additives for cattle have been suggested to reduce enteric methane emissions. For instance, methane inhibitors, such as monensin, 3-Nitrooxypropanol (3-NOP), and bromoform-containing seaweed species have shown promise in reducing emissions in cattle.
Evidences shows that 3-NOP effectively reduces the formation of enteric CH4 in dairy cows by inhibiting the enzyme methyl-coenzyme M reductase.Citation73 Studies have demonstrated that supplementing high yielding dairy cows with 3-NOP, a powerful CH4 inhibitor, can lead to a significant decrease of 23–37% in CH4 emissions.Citation84,Citation85 Supporting these findings, Melgar et al.Citation86 have consistently shown that administering 3-NOP through the total mixed ration (TMR) can reduce CH4 emissions by 22–40% in lactating dairy cows. This reduction is attributed to the high solubility and rapid metabolism of 3-NOP in the rumen, resulting in decreased enteric MeP. Importantly, the use of 3-NOP reduce CH4 emissions in cows does not have a negative impact on animal feed intake or productivity.Citation71,Citation87,Citation88 However, it does show a linear relationship with an increase in both milk fat concentration and yield.Citation86 In line with this, a meta-analysis conducted at Pennsylvania State University, which included studies involving dairy cows fed the CH4 inhibitor 3-NOP, consistently demonstrated a 28–32% reduction in daily CH4 emissions yield and intensity.Citation89 This reduction was observed without any effect on DMI, milk production, and body weight, but there was a slight increase in milk fat concentration (0.19 percentage units) and yield.Citation89 A recent meta-analysis of models by Kebreab et al.Citation90 observed that a 3-NOP dose of 70.5 mg/kg DM resulted in decreases in MeP (g/day), MeY (g/kg DMI), and MeI (g/kg energy-corrected milk) of 32.7, 30.9, and 32.6%, respectively. Moreover, evidence suggests that early-life dietary intervention in dairy calves, such as supplementing their diets with 3-NOP, can lead to a long-term reduction in CH4 emissions. This indicates that changes in microbial colonization of the rumen before weaning may imprint the rumen microbiome and have lasting effects on phenotypes later in life.Citation91 According to Vrancken et al.,Citation92 several companies have started manufacturing commercial feed additives of this kind, which may become available in the market in the next few years.
Regarding of the use of macroalgae, the incorporation of Asparagopsis taxiformis (AT) into the diets of dairy cows and steers can significantly reduce enteric MeP by 9–98%.Citation71,Citation93 In another study by Ramin et al.,Citation94 AT supplementation in dairy cow diets resulted in a 61% reduction in enteric MeP. However, the widespread use of seaweed feed additives to mitigate livestock CH4 emissions poses certain concerns. One argument suggests that to have a substantial global impact, there would need to be massive production and sale of seaweed supplements, considering the large worldwide population of dairy cattle. Harvesting enough seaweed for incorporation into their feed would therefore be logistically impossible. To use seaweed as a feed additive on a large scale, cultivation in aquaculture operations would thus be necessary.Citation40 Another challenge is the palatability of seaweed to cows, as researchers have found that cows do not enjoy the taste of seaweed. Additionally, concerns have been raised about the long-term effects of seaweed, such as the presence of bromoform in Asparagopsis taxiformis which can be excreted in milk and urine, and potentially cause inflammation in the rumen wall. These concerns raise questions about the safety of seaweed for humans and the environment.Citation3,Citation95 In a nutshell, these additives are now undergoing testing for application in dairy cows due to their considerable potential. For instance, companies like CH4 Australia are commercially introducing Asparagopsis seaweed to address climate change.
On the other hand, when dairy cows were supplemented with garlic and citrus extract, rumen fermentation and microbiota, were altered, leading to decreased MeP and MeI without affecting dry matter intake or milk production in dairy cows.Citation96
Genomic regions and candidate genes associated with methane emission traits
Understanding the genetic basis of methane emission traits is crucial for implementing sustainable breeding strategies aimed at reducing GHG emissions from livestock. Quantitative traits, including CH4 emission traits in farm animals, are intricately linked to polygenes located at QTLs, distributed widely throughout the genome. Within these QTLs, pinpointing a handful of markers that adequately explain a substantial proportion of genetic variance poses a considerable challenge. Consequently, the candidate gene approach has proven notably unsuccessful in the realm of commercial breeding for quantitative traits. Despite the fact that CH4 emissions are a complex trait influenced by multiple genetic loci acting together, only a limited number of QTLs associated with CH4 emissions are currently listed on the QTLdb website, primarily due to the limited routine measurement of CH4 emissions in dairy cattle.Citation11 This underscores the pressing need for comprehensive identification of genome-wide loci and/or variants that are associated with the complex phenotypes of CH4 emissions through the use of GWAS and transcription wide association studies (TWAS).
Given recent developments in high-throughput sequencing techniques and availability of high-resolution sequence data, it is now feasible to detect numerous significant genomic variants that impact CH4-emission traits in dairy cattle. However, the identification of genome-wide SNPs and genomic regions associated with CH4 emission traits in dairy cattle through GWAS is still in its early stages. Nevertheless, it is gaining momentum due to the increasing challenges posed by climate change, as evidenced by the recent surge in published studies ().Citation7,Citation11,Citation18,Citation19,Citation49 For example, Atashi et al.Citation49 identified several genes, namely LY6D, GML, CYHR1, PPP1R16A, ARHGAP39, ZNF7, OPLAH, MAF1, and SPATC1 located on chromosome 14, as potential candidate genes associated with predicted daily CH4 emissions and log-transformed predicted MeI. Similarly, Pszczola et al.Citation7 reported potential QTL regions and their respective candidate genes CYP51A1 (BTA4: 9,306,414–9,323,252), PPP1R16B (BTA13: 68,258,627–68,366,080), NTHL1 (BTA25: 1,590,252–1,595,934), TSC2 (BTA25: 1,596,730–1,626,967), and PKD1 (BTA 25: 1,627,978–1,666,088) affecting CH4 production located within QTLs that are related to feed efficiency, milk-related traits, body size and health status in dairy cattle. Importantly, PKD1 was identified as one of the most promising genes associated with digestive development and potentially involved in GHG emissions, including not only CH4 but also nitrogen-related emissions.Citation7 Manzanilla-Pech et al.Citation19 reported the presence of strong association signals on BTA13 and 26 for MeC and MeP in Danish Holstein cattle. Several previous studies have also confirmed significant effects of QTL regions on BTA1, 3, 13, and 20 that are strongly associated with MeP in dairy crossbred-dual purpose Mexican cows. Furthermore, Manzanilla-Pech et al.Citation19 reported significant regions on chromosomes 3, 6, and 13 for MeP, MeY, and MeI in Australian Holstein cows. Van EngelenCitation55 reported strong associations for MeP and MeY with the SNPs identified as the ones coding for DGAT1 on chromosome 14. There appears to be stronger evidence, suggesting a reliable association on chromosome 13 for MeP in several populations of Holstein cows in different countries.Citation7,Citation18,Citation19 On the other hand, Sarghale et al.Citation11 identified the most significant (p < 5 × 10−8) SNPs and regions associated with predicted CH4 emission per kg milk (15: 25,797,132) and per kg fat (19: 24,494,923 and 28: 21,771,233) in dairy cattle that are in close proximity to the QTLs. Additionally, promising candidate genes (ARPC1A and TRRAP) related to residual feed intakeCitation99,Citation100 were identified for valeric acid.Citation11 The ARPC1A gene is involved in regulating branched actin networks, whereas TRRAP is an adaptor protein found in multiprotein chromatin complexes with histone acetyltransferase activity, which adds a specific tag for epigenetic transcription activation.Citation11
Table 3. Candidate genes and SNPs in significant regions associated with various methane emission traits in dairy cattle.
Most GWAS studies on CH4 traits in dairy cattle have generally had small number of genotyped animals, with sample sizes ranging from fewer than 300 cows to <2000 cows. This is far below the optimal power for detecting QTL explaining at least 5% of the genetic variance, so care should be taken when considering for reported candidate variants. According to Gebreyesus et al.Citation101 who performed a power detection test on several Holstein populations, as a function of sample size and proportion of explained variance by a QTL, a population of 2,880 animals could have a detection power of 0.97, whereas a population of 1566 animals could have a detection power of 0.57 and a population of 614 animals explaining 5% of the genetic variance. This indicates more CH4 data are required to achieve higher power detection in GWAS for QTL associated with CH4 traits with the corresponding genetic variance associated with it.
Several studies have emphasized the importance of ruminal VFAs as metabolic biomarkers for CH4 emissions in dairy cattle. Interestingly, most of the SNPs that have a strong association with valeric acid (BTA5, 11, and 25) and isovaleric acid (BTA9 and 28) in dairy cattle are found near QTLs for milk production, its components, body weight, and RFI.Citation11 These metabolic biomarkers, such as valeric acid and isovaleric acid, can assist dairy farmers in improving the availability of these acids in the rumen for ruminal cellulolytic bacteria through genetic selection.
There is increasing evidence that cow host genetics play a role in shaping the composition of the rumen microbiota.Citation5,Citation102 Understanding how host genetics influence variation in the ruminal microbiota and their combined effects on CH4 emissions is crucial for breeding dairy cows that produce less CH4 .Citation5,Citation48,Citation57,Citation66,Citation98,Citation102 The ruminal bacterial community is believed to be influenced by a large number of genes, making it a polygenic trait. However, the complex relationship between cow genes and microbial relative abundance in the rumen is not yet well documented. Transcriptome-wide and GWAS can be used to pinpoint host chromosomal regions and causal genes that affect the composition and function of the rumen microbiome. Recent advancements in high-throughput sequencing techniques and advanced analytical methods have provided opportunities to unravel the rumen biochemical network involved in CH4 production in cattle. In a recent GWAS conducted by Abbas et al.,Citation103 several loci on different chromosomes (2, 6, 9, 19, 23, and 27) were found to be associated with the rumen microbiota. Notably, Prevotella, a dominant genus in the rumen that is involved in protein and energy metabolism, showed associations with these loci. The study also revealed a connection between the CLDN18 gene on chromosome 1, within the 132–133 Mb region, and Succiniclasticum ruminis, a common rumen inhabitant, that specializes in converting succinate to propionate for primary energy production. Abbas et al.Citation103 also highlighted specific genetic associations between the genes GMDS (chromosome 23), A4GNT (chromosome 1), and ANXA5 (chromosome 6), and Succiniclasticum ruminis and Prevotella. These genes are involved in endocytotic and exocytotic pathways, suggesting their role in modulating host rumen microbes to enhance energy absorption. The candidate genes DNAH9, ABS4, and DNAJC10 have been identified as potentially influencing and modifying the composition of ruminal microbial communities, making them potential genetic markers in cows.Citation98 The abundance of TSTA3 was host-genomically found to be positively correlated with CH4 emissions and could be a valuable biomarker for rumen pH, CH4 metabolism, and potentially for host-microbiome interactions to enhance saliva production.Citation57
Notably, understanding the specific molecular and biological pathways that regulate CH4 production is crucial for investigating the mechanisms of reduced CH4 emissions in dairy cattle. These pathways may be connected to energy metabolism, feed efficiency, and nutrient utilization, which are important factors to consider. To develop effective genetic selection strategies that address both CH4 emissions and dairy cattle productivity, it is essential to have a comprehensive understanding of these pathways. Candidate genes identified through GWAS are often associated with specific molecular pathways and biological functions, which further emphasizes the importance of studying them.
Breeding strategies to reduce CH4 emissions in dairy cattle
The future production environment is anticipated to undergo changes as the modern dairy production system heavily relies on expensive inputs, such as grain. Factors, such as ongoing competition for grain for human consumption, particularly in most agriculture dependent countries, and the impact of climate change on grain production are expected to drive grain prices higher. These economic considerations may lead to alterations in dairy production systems and subsequently influence the desired genetic attributes. Given the unpredictability of the future, genetic improvement systems that can swiftly adapt to changes in breeding objectives are deemed necessary.Citation104 A more enduring solution might come through gene editing combined with selective breeding, which could provide a long-term solution for methane emissions.Citation1 A more reasonable attention should be placed on a holistic breeding approach, including incorporating feed conversion efficiency and methane emission traits into the breeding goal traits (). This approach should be implemented to confront future emerging challenges, and ensure sustainable dairy production.
Figure 2. Brief overview of integrating omics data collection and selection with genomic best linear unbased prediction (GBLUP) and microbiome best linear unbiased prediction (MBLUP) methods, and holistic breeding strategies to achieve optimal genetic progress for low methane emission in dairy cattle.
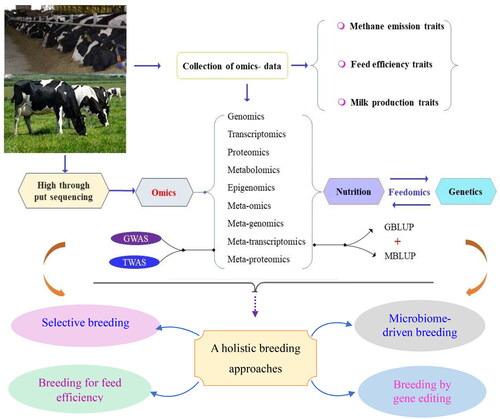
Selective breeding
Genetic selection stands out as a viable approach to address CH4 emissions, given the considerable genetic variation observed in both CH4 emissions and production (g/day) traits in cattle. The heritability of CH4 exhibits substantial genetic variation, with values ranging from 0.12 to 0.45.Citation12–14,Citation19 The strategy to reduce CH4 production involves selection for CH4 traits, either through direct CH4 measurements or by breeding based on correlated traits, such as residual feed intake.Citation93 Although breeding for increased milk production has already contributed to a reduction in emission intensity, more rapid decreases in emission levels could be achieved by selecting traits more strongly correlated with CH4 emissions than with production.Citation104 A compelling recent investigation by Zira et al.Citation105 revealed that when cows are selected based on resilience traits, the CF per unit of milk and meat product is reduced compared to those selected under the current breeding goal, particularly in situations of constrained feeding conditions. Their conclusion emphasizes the significance of development of breeding goals that address resilience to disturbances arising from climate changes as a crucial aspect of promoting sustainable dairy production. However, CH4 emission traits do not take into account in the current dairy breeding goal traits in dairy cattle. If this trend persists, CH4 production is projected to increase by 13% by 2050.Citation3 Therefore, the inclusion of CH4 traits into national breeding programs, alongside other breeding goal, holds the potential to reduce CH4 production. However, a tradeoff may exist, potentially leading to a decline in the genetic trend for milk productionCitation9 and reduced fertility and body condition score,Citation106 necessitate more profound adjustments in current indexes.Citation107 A practical example comes from Spanish Holstein cattle, where a feasible 20% reduction in CH4 emissions over a decade was achieved, with a corresponding reduction in gain for production traits ranging from 6% to 18%.Citation108 Consequently, the inclusion of CH4 emission traits in commercial selection indices may have consequences on other economically important traits in dairy cattle. When constructing these indices, careful consideration of the implications of GHG emissions is essential, as different sub-indexes can have varying effects on emissions.Citation109 Nevertheless, additional research is required to assess the downstream and upstream net GHG emissions impacts of selecting based on CH4 measurements.Citation93 Likewise, further investigation is needed to understand the relationship between CH4 emissions, feed intake, and production traits.
Breeding for reduced CH4 production offers a long-term solution that will define the breeds pursued for this approach indefinitely. However, the pursuit of lower CH4 emissions is not governed by a single gene; rather, the phenotype could be conferred by the interaction of several genes, each exerting a small effect. The elucidation of the genetic architecture of CH4 emission traits is now possible through genomics and provides a robust tool to address these challenges. In this regard, genomic selection is often preferred due to its ability to capture the polygenic nature of these traits. Indeed, the prospects of developing dairy cattle with minimal CH4 emission are possible through genomic selection. Genomic selection, employing genome-wide DNA markers, holds an advantage over conventional selection methods, particularly for selecting animals early in life and for traits that are challenging or expensive to measure, such as CH4 emissions and feed conversion efficiency in dairy cattle. To date, there is a lack of reports on genomic selection targeting lower CH4 emissions while concurrently maintaining production levels in dairy cattle.
Microbiome-driven breeding
Apart from studying traits associated with genome-wide genetic variations, it is crucial to acknowledge that livestock harbor various symbiotic organisms, including bacteria and protozoa, in their digestive tract.Citation104 Profiling the rumen microbiome has historically been challenging due to difficulties in culturing many rumen bacterial species. However, advances in high-throughput-sequencing technology and improvements in analytical capabilities are now facilitating the profiling of the rumen microbiome in animals. It is worth mentioning that researchers are currently investigating QTL in hosts that influence the composition of the gut microbiome in dairy cattle. Consequently, the selection of dairy cattle could involve selecting animals with a desirable rumen microbial ecosystem, and directly influencing these microbes could improve host efficiency.
The literature suggests that the abundance of microbial communities, including their genes and interactions, serves as effective biomarkers for predicting phenotypes associated with CH4 emissions.Citation57 In addition, there is evidence indicating genetic control over the composition of microbiota in the rumen, influenced by changes in both physical and physiological conditions that favor the growth of specific microbes.Citation13,Citation56,Citation103 In this context, Martínez-Álvaro et al.Citation57 have provided evidence of how genomic selection can modify the communities and functions of the rumen microbiome to develop animals with lower CH4 emissions. Their findings highlighted that specific microbial gene abundances offer more valuable information for breeding purposes compared to specific taxonomies. This observation is supported by the notion that a higher number of microbial genes, rather than genera, exhibit host-genomic correlations with CH4 emissions. By employing genome-resolved metagenomics, Roehe et al.Citation110 identified 20 microbial gene abundances primarily linked to the methanogenesis pathway, elucidating 81% of the variance in CH4 emissions in cattle.Citation57 The authors emphasized that selection based on the abundances of 30 microbial genes demonstrated a mitigation potential of 17% in mean CH4 emissions per generation. This mitigation potential surpassed the impact of direct genomic selection based on precisely measured CH4 emissions using respiration chambers (13%), highlights the potential effectiveness of a microbiome-driven breeding strategy in collectively reducing CH4 emissions and contributing to climate change mitigation.Citation57
With recent omics-based advancements, regulatory ncRNAs expression has been studied in cattle, and it was found that miRNA expression shows site-specificity along the gut in cattle.Citation111,Citation112 Most tissue-specific miRNAs for ruminants can be found in the rumen.Citation111 A study on early rumen development in neonatal dairy calves revealed that almost half of the expressed miRNAs are responsive to microbial metabolites,Citation113 indicating their significant role as responders to the intestinal microbiome during early life stages. Manipulating early-life microbiomes may offer opportunities for long-term interventions aimed at improving cow CH4 emissions; however, there is still limited understanding of the regulatory mechanisms behind these crucial host-microbiome interactions during early-life stage. In summary, numerous factors including genetics, diet, age, and time of day contribute toward variations observed within rumen microbiomes among dairy cattle populations, which presents challenges for applying microbiome driven breeding strategies in this context.
Breeding for feed efficiency
There is growing evidence supporting the idea that modifying the diet of dairy cows can effectively lower CH4 emissions. Pryce and Haile-MariamCitation2 have suggested that direct selection for feed efficiency presents an opportunity for rapid genetic progress in emission reduction. Notably, feed conversion efficiency confirmed a negative correlation with CF in dairy cattle.Citation32 De Haas et al.Citation12 proposed that by selecting more efficient cows, a 26% reduction could be achieved over a 10-year period. It has been observed that more efficient cows can produce milk without an increase in the amount of feed consumed. An international team of scientists conducted a recent meta-analysis of global dataCitation87 and proposed various product-based mitigation strategies. These include improving forage quality, specifically improving digestibility, and incorporating more concentrate feeds into the diet. These measures decreased enteric methane emissions in ruminants by 12–32%.
Breeding by genome-editing
Genome editing is a powerful tool in genomics that has the potential to revolutionize animal production. In cattle, the main cause of CH4 emissions is the symbiotic microbes present in their digestive tract, rather than the cattle themselves. By directly manipulating the genome of methanogenic archaea, it is possible to engineer these microbes and explore new ways to reduce CH4 emissions from their rumen. It is possible to identify the specific genes involved in MeP in the rumen of cattle, it becomes easier to use gene editing techniques to either eliminate the bacteria that contribute high MeP or increase the expression of those that promote low-methane species,Citation1 while maintaining proper digestion. This allows researchers to target specific genes involved in CH4 production and generate mutant methanogenic archaea that produce less CH4, which can then be used to populate the rumen of cattle. Previous research has demonstrated the feasibility of deleting the genomic sequence of Methanosarcina acetivorans and introducing multiple deletions causing Cas9-mediated genome editing.Citation114 The genome sequence of Methanobrevibacter ruminantium is also available,Citation115 and the functions of some predicted gene clusters have been established.Citation116 Further research is needed on rumen methanogens to gain insights into the genes that contribute to MeP in the rumen. There are various actions that can expedite the implementation of gene-edited solutions for climate benefits, although the extent of their contribution is not yet clear.Citation1 Innovative projects, such as the $70-million TED’s Audacious Project in California, aim to use CRISPR genome-editing on gut microbes in cattle to reduce emissions and halt climate change. These ground breaking initiatives demonstrate the potential for impactful research that will have a positive global impact. Addressing climate change through CRISPR genome editing and metagenomics research on microbiomes is crucial, as even the smallest organisms can provide valuable solutions to major challenges. Although the research in this field is ongoing, the rapid progress in gene editing has raised ethical concerns, regulatory framework, and public acceptance regarding its application in different animals. To address these concerns, involving community members in dairy farming programs can help make the approach less controversial compared to other gene drive designs.Citation117
Conclusions and future prospects
This review critically discusses current understandings of the carbon foot print of milk production, diet, and emissions from enteric fermentation in dairy cattle. It further explores the genetic mechanisms involved in CH4 emission traits and their implications for breeding approaches aimed at addressing or halting CH4 emissions from dairy cattle. As the effect of global climate change intensifies, this knowledge holds the potential to open up new avenues for mitigating the effects of CH4 from dairy cattle on global climate change. The literature indicates that genetic methods could be employed to breed dairy cows with reduced CH4 emissions, and incorporating traits, such as feed conversion efficiency into the current breeding goals. Given the complexity of identifying low-emitting cows and the tradeoffs involved, this review emphasizes the adoption of holistic breeding approaches. These include selective breeding, microbiome-driven breeding, breeding for feed efficiency, and breeding by genome editing to ensure sustainable dairy production in the face of global warming. The literature also underscores the importance of DNA methylation (DNAm) in shaping the microbial community in the rumen and suggests exploring methods, such as modifying the epigenetic landscape as an alternative approach to reduce CH4 emissions. The need for further research on genome-wide DNAm profiles for this purpose is highlighted. In summary, this review provides valuable insights for the dairy breeding industry, offering strategies to reduce CH4 emissions while maintaining milk production traits.
Disclosure statement
No potential conflict of interest was reported by the author(s).
Additional information
Funding
References
- Giddings L, Robert R, David MH. Gene editing for the climate: biological solutions for curbing greenhouse emissions. Washington, DC: Information Technology and Innovation Foundation (ITIF); 2020.
- Pryce JE, Haile-Mariam M. Symposium review: genomic selection for reducing environmental impact and adapting to climate change. J Dairy Sci. 2020;103(6):1–20.
- FAO. Pathways towards lower emissions – a global assessment of the greenhouse gas emissions and mitigation options from livestock agrifood systems. Rome: FAO; 2023.
- IPCC. Climate change 2021–the physical science basis: working group I contribution to the sixth assessment report of the intergovernmental panel on climate change. 1st ed. Cambridge: Cambridge University Press; 2021.
- Wallace RJ, Sasson G, Garnsworthy PC, et al. A heritable subset of the core rumen microbiome dictates dairy cow productivity and emissions. Sci Adv. 2019;5(7). 1–12.
- Knapp JR, Laur GL, Vadas PA, Weiss WP, Tricarico JM. Invited review: enteric methane in dairy cattle production: quantifying the opportunities and impact of reducing emissions. J Dairy Sci. 2014;97(6):3231–3261.
- Pszczola M, Strabel T, Mucha S, et al. Genome-wide association identifies methane production level relation to genetic control of digestive tract development in dairy cows. Sci Rep. 2018;8:15164.
- Silpa MV, König S, Sejian V, et al. Climate-resilient dairy cattle production: applications of genomic tools and statistical models. Front Vet Sci. 2021;8:625189.
- De Haas Y, Veerkamp RF, De Jong G, Aldridge MN. Selective breeding as a mitigation tool for methane emissions from dairy cattle. Animal. 2021;15 Suppl 1:100294.
- Lassen J, Difford GF. Review: genetic and genomic selection as a methane mitigation strategy in dairy cattle. Animal. 2020;14(S3):s473–s483.
- Sarghale AJ, Moradi Shahrebabak M, Moradi Shahrebabak H, et al. Genome-wide association studies for methane emission and ruminal volatile fatty acids using Holstein cattle sequence data. BMC Genet. 2020;21(1):1–14.
- De Haas Y, J, Windig M, Calus, et al. Genetic parameters for predicted methane production and potential for reducing enteric emissions through genomic selection. J Dairy Sci. 2011;94(12):6122–6134.
- Lassen J, Løvendahl P. Heritability estimates for enteric methane emissions from Holstein cattle measured using non-invasive methods. J Dairy Sci. 2016;99(3):1959–1967.
- Negussie E, de Haas Y, Dehareng F, et al. Invited review: large-scale indirect measurements for enteric methane emissions in dairy cattle: a review of proxies and their potential for use in management and breeding decisions. J Dairy Sci. 2017;100(4):2433–2453.
- Pszczola M, Rzewuska K, Mucha S, Strabel T. Heritability of methane emissions from dairy cows over a lactation measured on commercial farms. J Anim Sci. 2017;95(11):4813–4819.
- Yin T, Pinent T, Brügemann K, Simianer H, König S. Simulation, prediction, and genetic analyses of daily methane emissions in dairy cattle. J Dairy Sci. 2015;98(8):5748–5762.
- Kamalanathan S, Houlahan K, Miglior F, et al. Genetic analysis of methane emission traits in Holstein dairy cattle. Animals. 2023;13(8):1308.
- Calderón-Chagoya R, Hernandez-Medrano JHH, Ruiz-López FJJ, et al. Genome-wide association studies for methane production in dairy cattle. Genes. 2019;10(12):995.
- Manzanilla-Pech CIV, Difford GF, Sahana G, Romé H, Løvendahl P, Lassen J. Genome-wide association study for methane emission traits in Danish Holstein cattle. J Dairy Sci. 2022;105(2):1357–1368.
- Ron M, Weller JI. From QTL to QTN identification in livestock-winning by points rather than knock-out: a review. Anim Genet. 2007;38(5):429–439.
- Vistoso E, Salazar F, Gonzáles S. Estimación de la huella de carbono para la producción deleche en zona sur de Chile. XXXVIII Congreso Sociedad Chilena de Producción Animal (SOCHIPA). AgrovetMARKET; 2015.
- IPCC. Glossary. In: Refinement to the 2006 IPCC Guidelines for National Greenhouse Gas Inventories; 2019.
- Naranjo AM, Sieverding H, Clay D, Kebreab E. Carbon footprint of South Dakota dairy production system and assessment of mitigation options. PLOS One. 2023;18(3):e0269076.
- Sorley M, Casey I, Styles D, et al. Factors influencing the carbon footprint of milk production on dairy farms with different feeding strategies in western Europe. J Cleaner Prod. 2023;435:140104.
- Mech A, Devi GL, Sivaram M, et al. Assessment of carbon footprint of milk production and identification of its major determinants in smallholder dairy farms in Karnataka. J Dairy Sci. 2023;106(12):8847–8860.
- Ruiz-Llontop D, Velarde-Guillén J, Fuentes E, Prudencio M, Gómez C. Milk carbon footprint of silvo-pastoral dairy systems in the Northern Peruvian Amazon. Trop Anim Health Prod. 2022;54(4):227.
- Feyissa AA, Senbeta F, Diriba D, Tolera A. Understanding variability in carbon footprint of smallholder dairy farms in the central highlands of Ethiopia. Trop Anim Health Prod. 2022;54(6):411.
- Hospers J, Kuling L, Modernel P, et al. The evolution of the carbon footprint of Dutch raw milk production between 1990 and 2019. J Cleaner Prod. 2022;380:134863.
- Mazzetto AM, Falconer S, Ledgard S. Mapping the carbon footprint of milk production from cattle: a systematic review. J Dairy Sci. 2022;105(12):9713–9725.
- Ledgard SF, Falconer SJ, Abercrombie R, Philip G, Hill JP. Temporal, spatial, and management variability in the carbon footprint of New Zealand milk. J Dairy Sci. 2020;103(1):1031–1046.
- Jayasundara S, Worden D, Weersink A, et al. Improving farm profitability also reduces the carbon footprint of milk production in intensive dairy production systems. J Cleaner Prod. 2019;229:1018–1028.
- Wilkes A, Shimels W, Charles O, Simon F, Suzanne VD. Variation in the carbon footprint of milk production on smallholder dairy farms in central Kenya. J Cleaner Prod. 2020;265:121780.
- Woldegebriel D, Udo H, Viets T, Van der Harst E, Potting J. Environmental impact of milk production across an intensification gradient in Ethiopia. Livest Sci. 2017;206:28–36.
- Galloway C, Conradie B, Prozesky H, Esler K. Opportunities to improve sustainability on commercial pasture-based dairy farms by assessing environmental impact. Agric Syst. 2018;166:1–9.
- Morais TG, Teixeira RFM, Rodrigues NR, Domingos T. Carbon footprint of milk from pasture-based dairy farms in Azores, Portugal. Sustainability. 2018;10(10):3658.
- Sejian V, Prasadh RS, Lees AM, et al. Assessment of the carbon footprint of four commercial dairy production systems in Australia using an integrated farm system model. Carbon Manage. 2018;9(1):57–70.
- Wang X, Ledgard S, Lou J, et al. Environmental impacts and resource use of milk production on the North China Plain, based on life cycle assessment. Sci Total Environ. 2018;625:486–495.
- Zhao R, Xu Y, Wen X, Zhang N, Cai J. Carbon footprint assessment for a local branded pure milk product: a life-cycle based approach. Food Sci Technol. 2018;38:98–105.
- Gollnow S, Lundie S, Moore AD, et al. Carbon footprint of milk production from dairy cows in Australia. Int Dairy J. 2014;37(1):31–38.
- Hristov AN. Perspective: could dairy cow nutrition meaningfully reduce the carbon footprint of milk production? J Dairy Sci. 2023;106(11):7336–7340.
- Uddin ME, Tricarico JM, Kebreab E. Impact of nitrate and 3-nitrooxypropanol on the carbon footprints of milk from cattle produced in confined-feeding systems across regions in the United States: a life cycle analysis. J Dairy Sci. 2022;105(6):5074–5083.
- Gerber P, Vellinga T, Opio C, Steinfeld H. Productivity gains and greenhouse gas emissions intensity in dairy systems. Livest Sci. 2011;139(1–2):100–108.
- O’Brien D, Hennessy T, Moran B, Shalloo L. Relating the carbon footprint of milk from Irish dairy farms to economic performance. J Dairy Sci. 2015;98(10):7394–7407.
- Hossein-Zadeh NG. Estimates of the genetic contribution to methane emission in dairy cows: a meta-analysis. Sci Rep. 2022;12(1):12352.
- Lopes LSF, Schenkel FS, Houlahan K, et al. Estimates of genetic parameters for rumination time, feed efficiency, and methane production traits in first lactation Holstein cows. J Dairy Sci. 2024; 107: S0022-0302.
- López-Paredes J, Goiri I, Atxaerandio R, et al. Mitigation of greenhouse gases in dairy cattle via genetic selection: 1. Genetic parameters of direct methane using non-invasive methods and proxies of methane. J Dairy Sci. 2020;103(8):7199–7209.
- Van Breukelen AE, Veerkamp RF, de Haas Y, Aldridge MN. Genetic parameter estimates for methane emission during lactation from breath and potential inaccuracies in reliabilities assuming a repeatability versus random regression model. J Dairy Sci. 2024; 107: S0022-0302.
- Saborío‐Montero A, Gutiérrez‐Rivas M, García‐Rodríguez A, et al. Structural equation models to disentangle the biological relationship between microbiota and complex traits: methane production in dairy cattle as a case of study. J Animal Breed Genet. 2019;137(1):36–48.
- Atashi H, Wilmot H, Vanderick S, Hubin X, Soyeurt H, Gengler N. Genome-wide association study for mid-infrared methane predictions in Walloon dairy cows. Proceedings of 12th World Congress on Genetics Applied to Livestock Production (WCGALP); 2022:156–159.
- Uemoto Y, Tomaru T, Masuda M, et al. Exploring indicators of genetic selection using the sniffer method to reduce methane emissions from Holstein cows. Anim Biosci. 2024;37(2):173–183.
- Sitkowska B, Yüksel HM, Piwczyński D, Önder H. Heritability and genetic correlations of rumination time with milk-yield and milking traits in Holstein-Friesian cows using an automated milking system. Animal. 2024;18(3):101101.
- Pickering NK, Oddy VH, Basarab J, et al. Animal board invited review: genetic possibilities to reduce enteric methane emissions from ruminants. Animal. 2015;9(9):1431–1440.
- Richardson CM, Nguyen TTT, Abdelsayed M, et al. Genetic parameters for methane emission traits in Australian dairy cows. J Dairy Sci. 2021;104(1):539–549.
- Difford GF, Løvendahl P, Veerkamp RF, et al. Can greenhouse gases in breath be used to genetically improve feed efficiency of dairy cows? J Dairy Sci. 2020;103(3):2442–2459.
- Van Engelen S, Bovenhuis H, Dijkstra J, van Arendonk J, Visker M. Genetic study of methane production predicted from milk fat composition in dairy cows. J Dairy Sci. 2015;98(11):8223–8226.
- Gonzalez-Recio O, Zubiria I, García-Rodríguez A, Hurtado A, Atxaerandio R. Signs of host genetic regulation in the microbiome composition in 2 dairy breeds: Holstein and Brown Swiss. J Dairy Sci. 2018;101(3):2285–2292.
- Martínez-Álvaro M, Auffret MD, Duthie CA, et al. Bovine host genome acts on rumen microbiome function linked to methane emissions. Commun Biol. 2022;5(1):350.
- Breider IS, Wall E, Garnsworthy PC. Short communication: heritability of methane production and genetic correlations with milk yield and body weight in Holstein-Friesian dairy cows. J Dairy Sci. 2019;102(8):7277–7281.
- Zetouni L, Difford GF, Lassen J, Byskov MV, Norberg E, Løvendahl P. Is rumination time an indicator of methane production in dairy cows? J Dairy Sci. 2018;101(12):11074–11085.
- Anderson CL, Schneider CJ, Erickson GE, MacDonald JC, Fernando SC. Rumen bacterial communities can be acclimated faster to high concentrate diets than currently implemented feedlot programs. J Appl Microbiol. 2016;120(3):588–599.
- Henderson G, Cox F, Ganesh S, et al. Rumen microbial community composition varies with diet and host, but a core microbiome is found across a wide geographical range. Sci Rep. 2015;5:14567.
- Huws SA, Creevey CJ, Oyama LB, et al. Addressing global ruminant agricultural challenges through understanding the rumen microbiome: past, present and future. Front Microbiol. 2018;9:2161.
- Barrett K, Jensen K, Meyer AS, Frisvad JC, Lange L. Fungal secretome profile categorization of CAZymes by function and family corresponds to fungal phylogeny and taxonomy: example Aspergillus and Penicillium. Sci Rep. 2020;10:1–12.
- Danielsson R, Dicksved J, Sun L, et al. Methane production in dairy cows correlates with rumen methanogenic and bacterial community structure. Front Microbiol. 2017;8:226.
- Delgado B, Bach A, Guasch I, et al. Whole rumen metagenome sequencing allows classifying and predicting feed efficiency and intake levels in cattle. Sci Rep. 2019;9:11.
- Difford GF, Plichta DR, Løvendahl P, et al. Host genetics and the rumen microbiome jointly associate with methane emissions in dairy cows. PLOS Genet. 2018;14(10):e1007580.
- Haque MN. Dietary manipulation: a sustainable way to mitigate methane emissions from ruminants. J Anim Sci Technol. 2018;60(1):15.
- Tapio I, Snelling TJ, Strozzi F, Wallace RJ. The ruminal microbiome associated with methane emissions from ruminant livestock. J Anim Sci Biotechnol. 2017;8:7.
- Mizrahi I, Wallace RJ, Moraïs S. The rumen microbiome: balancing food security and environmental impacts. Nat Rev Microbiol. 2021;9(19):553–566.
- Arndt C, Powell JM, Aguerre MJ, Crump PM, Wattiaux MA. Feed conversion efficiency in dairy cows: repeatability, variation in digestion and metabolism of energy and nitrogen, and ruminal methanogens. J Dairy Sci. 2015;98(6):3938–3950.
- Beauchemin KA, Ungerfeld EM, Abdalla AL, et al. Invited review: current enteric methane mitigation options. J Dairy Sci. 2022;105(12):9297–9326.
- Hawkins J, Yesuf G, Zijlstra M, Schoneveld GC, Rufino MC. Feeding efficiency gains can increase the greenhouse gas mitigation potential of the Tanzanian dairy sector. Sci Rep. 2021;11(1):4190.
- Pitta DW, Indugu N, Melgar A, et al. The effect of 3-nitrooxypropanol, a potent methane inhibitor, on ruminal microbial gene expression profiles in dairy cows. Microbiome. 2022;10(1):146.
- Mizrahi I, Jami E. Review: the compositional variation of the rumen microbiome and its effect on host performance and methane emission. Anim Int J Anim Biosci. 2018;12:s220–s232.
- Borreani G, Tabacco E, Schmidt RJ, et al. Silage review: factors affecting dry matter and quality losses in silages. J Dairy Sci. 2018;101(5):3952–3979.
- Bica R, Palarea-Albaladejo J, Lima J, et al. Methane emissions and rumen metabolite concentrations in cattle fed two different silages. Sci Rep. 2022;12(1):5441.
- Beauchemin KA, Kreuzer M, O’Mara F, McAllister TA. Nutritional management for enteric methane abatement: a review. Aust J Exp Agric. 2008;48(2):21–27.
- Bougouin A, Leytem A, Dijkstra J, Dungan RS, Kebreab E. Nutritional & environmental effects on ammonia emissions from dairy cattle housing: a meta-analysis. J Environ Qual. 2016;45:1123–1132.
- Edouard N, Charpiot A, Robin P, Lorinquer E, Dollé JB, Faverdin P. Influence of diet and manure management on ammonia and greenhouse gas emissions from dairy barns. Animal. 2019;13(12):2903–2912.
- Edouard N, Hassouna M, Robin P, Faverdin P. Low degradable protein supply to increase nitrogen efficiency in lactating dairy cows and reduce environmental impacts at barn level. Animal. 2016;10(2):212–220.
- Schrade S, Zeyer K, Mohn J, Zähner M. Effect of diets with different crude protein levels on ammonia and greenhouse gas emissions from a naturally ventilated dairy housing. Sci Total Environ. 2023;896:165027.
- Federal Office for Agriculture. Research and advisory projects, evaluation projects and external studies.Bern, Switzerland: Federal Office for Agriculture, 2022.
- Anthony TL, Szutu DJ, Verfaillie JG, et al. Carbon-sink potential of continuous alfalfa agriculture lowered by short-term nitrous oxide emission events. Nat Commun. 2023;14:1926.
- Haisan J, Sun Y, Guan L, et al. The effects of feeding 3-nitro-oxypropanol at two doses on milk production, rumen fermentation, plasma metabolites, nutrient digestibility, and methane emissions in lactating Holstein cows. Anim Prod Sci. 2017;57(2):282–289.
- Van Wesemael D, Vandaele L, Ampe B, et al. Reducing enteric methane emissions from dairy cattle: two ways to supplement 3-nitrooxypropanol. J Dairy Sci. 2019;102(2):1780–1787.
- Melgar A, Harper MT, Oh J, et al. Effects of 3-nitrooxypropanol on rumen fermentation, lactational performance, and resumption of ovarian cyclicity in dairy cows. J Dairy Sci. 2020;103(1):410–432.
- Arndt C, Hristov AN, Price WJ, et al. Full adoption of the most effective strategies to mitigate methane emissions by ruminants can help meet the 1.5 °C target by 2030 but not 2050. Proc Natl Acad Sci USA. 2022;119(20):e2111294119.
- FAO. Methane emissions in livestock and rice systems-sources, quantification, mitigation and metrics. Rome: FAO; 2023.
- Hristov AN, Melgar A, Wasson D, Arndt C. Symposium review: effective nutritional strategies to mitigate enteric methane in dairy cattle. J Dairy Sci. 2022;105(10):8543–8557.
- Kebreab E, Bannink A, Pressman EM, et al. A meta-analysis of effects of 3-nitrooxypropanol on methane production, yield, and intensity in dairy cattle. J Dairy Sci. 2023;106(2):927–936.
- Meale SJ, Popova M, Saro C, et al. Early life dietary intervention in dairy calves results in a long-term reduction in methane emissions. Sci Rep. 2021;11(1):3003.
- Vrancken H, Suenkel M, Hargreaves PR, et al. Reduction of enteric methane emission in a commercial dairy farm by a novel feed supplement. Open J Anim Sci. 2019;9(3):286–296.
- Fouts JQ, Honan MC, Roque BM, Tricarico JM, Kebreab E. Enteric methane mitigation interventions. Transl Anim Sci. 2022;6(2):txac041.
- Ramin M, Chagas JCC, Pal Y, Danielsson R, Fant P, Krizsan SJ. Reducing methane production from stored faces of dairy cows by Asparagopsis taxiformis. Front Sustain Food Syst. 2023;7:1187838.
- Muizelaar W, Groot M, Van Duinkerken G, Peters R, Dijkstra J. Safety and transfer study: transfer of bromoform present in Asparagopsis taxiformis to milk and urine of lactating dairy cows. Foods. 2021;10(3):584.
- Khurana R, Tassilo B, Ilma T, Ali-Reza B. Effect of a garlic and citrus extract supplement on performance, rumen fermentation, methane production, and rumen microbiome of dairy cows. J Dairy Sci. 2023;106(7):4608–4621.
- Sypniewski M, Strabel T, Pszczola M. Genetic variability of methane production and concentration measured in the breath of polish Holstein-Friesian cattle. Animals. 2021;11(11):3175.
- Zhang Q, Difford G, Sahana G, et al. Bayesian modelling reveals host genetics associated with rumen microbiota jointly influence methane emission in dairy cows. ISME J. 2020;14(8):2019–2033.
- De Lima AO, de Oliveira PSN, Tizioto PC, et al. Association analyses pointed the TIPARP as a potential candidate gene influencing residual feed intake variation in Nelore Cattle. Jaboticabal: International Meeting of Advances in Animal Science; 2016.
- Salleh MS, Mazzoni G, Höglund JK, et al. RNA-Seq transcriptomics and pathway analyses reveal potential regulatory genes and molecular mechanisms in high-and low-residual feed intake in Nordic dairy cattle. BMC Genomics. 2017;18(1):258.
- Gebreyesus G, Buitenhuis AJ, Poulsen NA, et al. Combining multi-population datasets for joint genome-wide association and meta-analyses: the case of bovine milk fat composition traits. J Dairy Sci. 2019;102(12):11124–11141.
- Sasson G, Kruger BS, Seroussi E, Doron-Faigenboim A, Shterzer N, Yaacoby S, Mizrahi I . Heritable bovine rumen bacteria are phylogenetically related and correlated with the cow’s capacity to harvest energy from its feed. Mol Biol. 2017;8:1–12.
- Abbas W, Howard JT, Paz HA, et al. Influence of host genetics in shaping the rumen bacterial community in beef cattle. Sci Rep. 2020;10(1):15101.
- Hayes BJ, Lewin HA, Goddard ME. The future of livestock breeding: genomic selection for efficiency, reduced emissions intensity, and adaptation. Trends Genet. 2013;29(4):206–214.
- Zira S, Bouquet A, Rydhmer L, Kargo M, Puillet L. Carbon footprint based on lifetime productivity for future cows selected for resilience to climate-related disturbances. J Dairy Sci. 2023;106(12):8953–8968.
- Kandel PB, Vanderick S, Vanrobays ML, et al. Consequences of selection for environmental impact traits in dairy cows. Proceedings, 10th World Congress of Genetics Applied to Livestock Production; 2014.
- Kandel P, Vanderick S, Vanrobays M, Soyeurt H, Gengler N. Consequences of genetic selection for environmental impact traits on economically important traits in dairy cows. Anim Prod Sci. 2018;58(10):1966–1966.
- González-Recio O, López-Paredes J, Ouatahar L, et al. Mitigation of greenhouse gases in dairy cattle via genetic selection: 2. Incorporating methane emissions into the breeding goal. J Dairy Sci. 2020;103(8):7210–7221.
- Walmsley BJ. Consequences of using different economic selection index methods on greenhouse gas emissions in beef cattle. Proceedings of 12th World Congress on Genetics Applied to Livestock Production (WCGALP); 2022.
- Roehe R, Dewhurst RJ, Duthie C-A, et al. Bovine host genetic variation influences rumen microbial methane production with best selection criterion for low methane emitting and efficiently feed converting hosts based on metagenomic gene abundance. PLOS Genet. 2016;12(2):e1005846.
- Sun HZ, Chen Y, Guan LL. MicroRNA expression profiles across blood and different tissues in cattle. Sci Data. 2019;6(1):190013.
- Wang Y, Li D, Wang Y, et al. The landscape of circular RNAs and mRNAs in bovine milk exosomes. J Food Compos Anal. 2019;76:33–38.
- Malmuthuge N, Liang G, Guan LL. Regulation of rumen development in neonatal ruminants through microbial metagenomes and host transcriptomes. Genome Biol. 2019;20(1):172.
- Nayak DD, Metcalf WW. Cas9-mediated genome editing in the methanogenic archaeon Methanosarcina acetivorans. Proc Natl Acad Sci USA. 2017;114(11):2976–2981.
- Leahy SC, Kelly WJ, Altermann E, et al. The genome sequence of the rumen methanogen Methanobrevibacter ruminantium reveals new possibilities for controlling ruminant methane emissions. PLOS One. 2010;5(1):e8926.
- Bharathi M, Senthil Kumar N, Chellapandi P. Functional prediction and assignment of Methanobrevibacter ruminantium M1 operome using a combined bioinformatics approach. Front Genet. 2020;11:593990.
- Worku D, Hussen J, De Matteis G, Schusser B, Alhussien MN. Candidate genes associated with heat stress and breeding strategies to relieve its effects in dairy cattle: a deeper insight into the genetic architecture and immune response to heat stress. Front Vet Sci. 2023;10:1151241.