ABSTRACT
ChatGPT has revolutionized the travel industry. This study employs the stimulus-organism-response (SOR) model to develop and validate a conceptual model for ChatGPT acceptance. Social influence and perceived value emerge as key determinants of user cognitive appraisals of ChatGPT’s expertise, trustworthiness, and emotional connections through parasocial interaction. These factors subsequently influence traveler acceptance of ChatGPT for travel-related services. Findings reveal that social influence is the most potent predictor of ChatGPT acceptance, while perceived trust directly impacts user acceptance during the cognitive process. These insights advance research on parasocial interaction, while providing valuable guidance for implementing ChatGPT in tourism services.
Introduction
The integration of artificial intelligence (AI) in the tourism and travel industry has become commonplace in recent years, primarily driven by its potential for reducing labour costs and improving service efficiency (Hwang et al., Citation2022; Lv et al., Citation2022). The use of applications such as AI-powered chatbots has become widespread in travel services, from simple hotel reservations to personalized travel recommendations (Zhang et al., Citation2024; Y. Zhu et al., Citation2023). Statista (Citation2023) reported that the percentage of revenue generated by AI-influenced travel companies is expected to increase from 21% to 32% by 2024. In particular, recent development of advanced natural language processing (NLP) models, such as ChatGPT (Generative Pre-trained Transformer), developed by OpenAI in November 2022, has attracted attention for its potential applications in the travel and tourism domain (Demir & Demir, Citation2023).
ChatGPT, a large language model (LLM) built on a transformer-based neural network and trained on massive text, is revolutionizing the travel and tourism industry by enabling human-like text generation from input prompt (Haleem et al., Citation2022; Lecler et al., Citation2023). ChatGPT-powered chatbots are becoming increasingly popular with hotels, airlines, and online travel agencies (OTAs), with one of the most significant applications being 24/7 automated customer service (Bulchand-Gidumal, Citation2022; Lv et al., Citation2022). These chatbots can respond promptly to customer inquiries, thereby improving customer satisfaction and reducing labour costs (Calvaresi et al., Citation2021). For example, Trip.com, a Singapore-based OTA, launched TripGen in February 2023, a chatbot powered by OpenAI that recommends flights, hotels, and other travel services to its mobile app users. By providing personalized recommendations anywhere and at anytime, optimizing customer service, and improving trip planning efficiency, ChatGPT has the potential to reshape the overall tourist experience and facilitate better travel decision-making process (Dwivedi, Pandey, et al., Citation2023). Despite the high potential of ChatGPT for application across the tourist decision journey and benefit to travel-related services, empirical research from the tourist perspective regarding consumer acceptance and use of ChatGPT remains limited. As Gursoy et al. (Citation2023) noted, research on ChatGPT is still at an early stage, and more empirical studies are needed to understand how consumers accept ChatGPT applications for travel-related services.
Driven by the surge of AI innovations such as service robots, autonomous driving and virtual tour guide, extensive research has explored consumers’ acceptance of these technologies within the travel and tourism industry (Au & Tsang, Citation2022; Cai et al., Citation2022; H. Kim et al., Citation2023; Ribeiro et al., Citation2022; J. Wang et al., Citation2023). Studies underscore various factors influencing the adoption of new technology, including trust (Chi et al., Citation2021; Seo & Lee, Citation2021), perceived usefulness (Ariza-Montes et al., Citation2023; Tavitiyaman et al., Citation2022), perceived ease of use (Pillai & Sivathanu, Citation2020; Pillai et al., Citation2020), perceived value-cocreation (Demir & Demir, Citation2023; Sthapit et al., Citation2023), perceived intelligence (Pillai & Sivathanu, Citation2020), anthropomorphism (Cai et al., Citation2022; Roy et al., Citation2020), social influence (Ribeiro et al., Citation2022; Solakis et al., Citation2022) and perceived risk (Habib & Hamadneh, Citation2021; Ribeiro et al., Citation2022). Notably, research indicate that emotional responses elicited through AI interactions (e.g. human-robot interaction) significantly impact tourists’ long-term intention to use AI technologies for travel and tourism purpose (Au & Tsang, Citation2022; Yang et al., Citation2022).
While existing research on consumer acceptance of AI technologies in travel and tourism emphasizes utilitarian factors such as perceived usefulness and perceived ease of use, the nuanced differences across diverse AI technologies and their distinct embodiments necessitates technology-specific investigation (Ribeiro et al., Citation2022). AI-consumer interactions span a spectrum, from basic chatbots with limited convers ability to sophisticated service robots. ChatGPT represents a new class of conversational agent enabled by advanced natural language processing and deep learning. Compared to previous chatbots and service robots, ChatGPT facilitates more anthropomorphic, context-adaptive and fluent dialogues. Consequently, consumers may prioritize the value brought by ChatGPT for travel information retrieval, planning and, translation over the perceived usefulness of physical service robots or the ease of use of traditional chatbots. This study thus focuses more on exploring the broad value of ChatGPT in understanding consumer acceptance. Moreover, AI-powered agents like ChatGPT exhibit capabilities beyond simply answering user queries. They can leverage their interactive nature to not only provide solutions, but also recognize and respond to user emotions. This “emotional intelligence” foster parasocial interaction, where users subconsciously develop rapport with the chatbot, perceiving it as a human travel consultant. The emotional connections induced by ChatGPT may play a mediating role in consumer acceptance behaviour. Given ChatGPT’s innovative attributes, technology-specific investigation is required to provide a comprehensive explanation of tourist attitudes and behaviours towards this novel AI system. Specifically, this study aims to answer the following research questions:
RQ 1:
What factors influence tourists’ acceptance and adoption of ChatGPT for travel-related services?
RQ 2:
How does parasocial interaction with ChatGPT mediate the relationship between perceived intelligence/competence and intention to adopt ChatGPT for travel-related services?
This study employs the Stimulus-Organism-Response (SOR) framework, which provides a broader perspective to addresses how external stimuli influence users’ internal psychological states to elicit responses and behaviours. While established theories such as the Technology Acceptance Model (TAM), Unified Theory of Acceptance and Use of Technology (UTAUT), AI Device Acceptance Model (AIDUA), and Theory of Planned Behaviour (TPB) have been widely applied to study consumer acceptance of new technologies, they have some limitations in explaining acceptance of emerging AI technologies such as ChatGPT. A key aspect is that these theoretical frameworks were developed to explain adoption of more productivity-oriented technologies (e.g. smartphone apps) rather than technologies like ChatGPT that may elicit emotional responses through human-AI model interaction, which are not captured in these frameworks. Furthermore, key constructs such as perceived ease of use and behavioural control may not apply as well to AI systems like ChatGPT that require minimal effort for users to engage with. Therefore, drawing upon on the SOR framework, this study proposes a context- specific ChatGPT acceptance model that elucidates the psychological factors influencing tourists’ adoption of ChatGPT for travel services. The proposed model explains how external factors (e.g. perceived value, social influence) interact with tourists’ cognitive appraisals (e.g. perceived expertise, trust in ChatGPT information) and emotional responses (e.g. parasocial interaction) to determine tourists’ behaviour. By extending SOR theory’s application to the novel context of predicting tourist use ChatGPT for travel-related services. This study aims to provide both theoretical and practical insights.
This study’s finding will contribute to the literature on AI technologies adoption. The results will also provide valuable practical insights within travel and tourism industry, AI developers, and policymakers by elucidating the key factors influencing consumer adoption of ChatGPT within the travel and tourism domain. Moreover, the findings can assist destination management organizations, governments and tourism business to develop strategies for integrating AI systems like ChatGPT more effectively. Overall, the theoretical and practical contributions will be valuable for both tourism practice and social science research investigating attitudes, decision-making, and behaviour in light of emerging AI technologies and models.
Theoretical background and hypotheses development
ChatGPT in tourism
ChatGPT revolutionizes tourists’ travel information search and decision-making by providing personalized and interactive assistance across all travel stages (Gursoy et al., Citation2023). Specifically, in the pre-trip planning stage, ChatGPT transforms how tourists explore destination options, obtain personalized recommendations, and acquire detailed travel information about attractions, accommodations, and transportation options. For example, consider a tourist planning a 7-day trip to Thailand for two adults and two children, with a budget of $3,000 USD and Phuket as one of their destinations. As illustrated in , ChatGPT can rapidly generate relevant responses, supplemented by appropriate travel guidance, based on the tourist’s requirements and set conditions. By leveraging its capabilities for contextual understanding and learning from vast data, ChatGPT profoundly transforms how tourists search for travel-related information and make travel plans, substantially enhancing the travel information searching experience and reducing the need for offline travel consultants.
During the trip, ChatGPT can serve as an intelligent virtual assistant, providing a wide range of on-site support, including real-time navigation, localized recommendations, and facilitation of communication with local service providers. It can also function as virtual tour guide, providing information on local attractions, history, and traditions (Gursoy et al., Citation2023). Additionally, ChatGPT can assist with unexpected trip challenges by quickly providing relevant details, such as the locations of key services such as a children’s hospital and police stations. Notably, ChatGPT can bridge language and cultural barriers by conversing with tourists in their preferred languages and tailoring advice to their needs. Such interactive nature of ChatGPT significantly enhances tourists’ travel experiences by enabling them to make informed decisions. shows ChatGPT recommending activities suitable for Chinese teens aged 10–15 years old in Phuket Island and providing responses in Chinese, English, and Thai languages. ChatGPT’s recommendations, including Thai addresses, help tourists better understand local information and communicate more effectively with local drivers, thereby bridging communication gaps between tourists and residents, reducing trip-related inconveniences, and enhancing their travel experience and satisfaction (Carvalho & Ivanov, Citation2023). While the post-trip stage is traditionally not considered as part of the travel decision-making process, ChatGPT enables tourists to share post-trip experiences, which helps it improve future recommendations and enables others to discover new destinations and activities (Carvalho & Ivanov, Citation2023; Gursoy et al., Citation2023).
Figure 2. ChatGPT recommendations for Chinese teens in three languages.
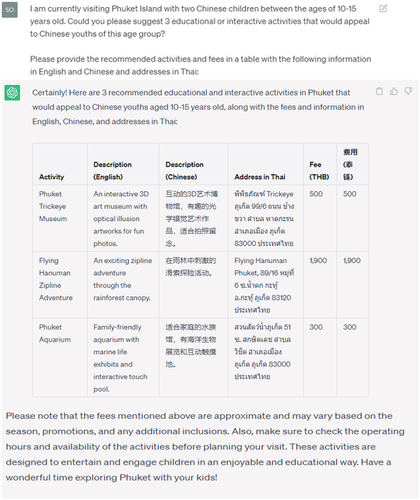
ChatGPT’s potential to trigger parasocial bonds between individuals and the AI-powered language model is another notable characteristic that has attracted significant attention from tourism scholars and practitioners (Demir & Demir, Citation2023; Zhang et al., Citation2024). As travellers repeatedly interact with ChatGPT during the journey, they may begin to perceive it as a trusted virtual companion rather than just an AI tool, leading to increased dependence and emotional attachment. This connection can significantly influence tourists’ acceptance and willingness to use the AI model as a travel agent. While previous tourism research has explored human-robot interactions (Chi et al., Citation2021; Murphy et al., Citation2019), limited research has been directed towards elucidating how tourists interact with AI-powered language models like ChatGPT that lack physical forms. Therefore, more work is needed to better understand their emergence and impact on tourists’ intentions to accept and use such AI models in the tourism and travel domain.
Stimulus-organism-response models
The stimulus-organism-response (SOR) paradigm developed by Mehrabian and Russell (Citation1974) forms the theoretical foundation for this study. The SOR framework outlines three components: stimulus, which refer to external triggers; an organism, representing an individual’s internal cognitive and affective evaluations of those stimuli; and responses, or the behavioural outcomes (Mehrabian & Russell, Citation1974; Tung & Tse, Citation2023). Grounded in environmental psychology, SOR theory posits that external cues and social influences elicit mental and emotional reactions, subsequently driving behaviour. Stimuli encompass object stimuli, linked to tangible product/service attributes like price and functionality, and social-psychological stimuli, tied to peer norms and pressures (S. Lee et al., Citation2011). In this study on ChatGPT adoption for travel or tourism purposes, perceived value constitutes a key object stimulus and social influence represents a salient social-psychological stimulus. Although SOR model has been extensively employed by tourism researchers to examine how various external stimuli predict consumer responses and behaviours in information technology contexts, including travel app experience (S.-E. Kim & Jung, Citation2022; Wu et al., Citation2021), virtual reality tourism experience (Chua et al., Citation2023; Shin & Jeong, Citation2022), robot service and among other areas (Tung & Tse, Citation2023; Y. Zhu et al., Citation2023), it has rarely been extended to encompass AI-powered technologies, such as ChatGPT. Therefore, the present study aims to expand the model’s scope by incorporating ChatGPT.
The SOR framework guides this study in exploring relationships between external environmental influences (stimulus), users’ internal evaluations (organism), and acceptance behaviours (response) in the context of using ChatGPT for travel information and travel- related solutions. Specifically, perceived value and social are positioned as external that may motivate users to consult ChatGPT. In the organism phase, perceived trust in ChatGPT’s information, perceived expertise of ChatGPT, and parasocial interaction with ChatGPT represent internal variables that reflect how a user cognitively and emotionally evaluates ChatGPT. Finally, at the response phase, tourist acceptance behaviour toward using ChatGPT for travel-related services is examined as the key outcome. illustrates the fundamental variables associated with each phase of the SOR model.
Social influence and perceived value
Within the context of tourism technology, the stimulus component of the SOR framework can be categorized into two main areas: 1) social influence, and 2) perceived value of the technology. Social influence refers to the extent to which a particular attitude or behaviour is considered important by an individual’s social group (Ma & Li, Citation2022; Solakis et al., Citation2022). In the context of this study, social influence pertains to how tourists’ social groups, including friends, family, and social networks, perceive the use of ChatGPT for travel-related or tourism-related purposes such as travel planning, information search, and translation, in alignment with their social group norms (Venkatesh et al., Citation2012). If members of a tourist’ social group positively evaluate ChatGPT’s usefulness in enhancing travel experiences and recommend its adoption, this social influence can shape tourists’ own perceptions of the value of ChatGPT. Researchers have acknowledged that travellers’ adherence to their social groups’ norms plays a key role in the early stages of new technology acceptance (Taylor & Todd, Citation1995) and user adoption intention (López-Nicolás et al., Citation2008; Ribeiro et al., Citation2022). Several studies have identified the significant influence of social groups on tourist behavioural intentions, including acceptance of mobile services in mature tourism market (Bader et al., Citation2012), travel blogging for travel-related service (H.-Y. Wang, Citation2012), adopting autonomous vehicles (Park et al., Citation2021; Ribeiro et al., Citation2022), and online hotel bookings (Confente & Vigolo, Citation2018; Ma & Li, Citation2022). Social influence is therefore predicted to be a crucial stimulus influencing tourists’ acceptance of ChatGPT for tourism and travel services.
H1:
Social influence will positively influence tourists’ acceptance of using ChatGPT for travel-related purposes.
Perceived value refers to users’ assessments of the benefits a new technology offers, weighted against costs and risks (M. Choi et al., Citation2023; X. Wang et al., Citation2022). In the context of conversational AI, perceived value refers to travellers’ appraisals of ChatGPT’s potential functional benefits such as its 24/7 availability to provide quick answers to trip planning questions, tailored to individuals’ preferences, and multi-lingual translation and information search (Carvalho & Ivanov, Citation2023; Demir & Demir, Citation2023). Tourists who recognize and believe in its value, usefulness, convenience, personalization, and problem-solving capabilities are more likely to be motivated to try and accept ChatGPT for travel and tourism purposes. Previous studies suggest that tourists’ perceived value can directly and strongly impact users’ acceptance and usage of technology (M. Choi et al., Citation2023; Sarkady et al., Citation2021). For example, de Kervenoael et al. (Citation2020) found perceived value positively influenced tourists’ acceptance of social robots in tourism services. J. Wang et al. (Citation2023) found that the perceived value of cashless payment positively related to usage intention during the COVID-19 pandemic. Therefore, it is reasonable to hypothesize that tourists’ perceived value of ChatGPT serves as a significant stimulus influencing its acceptance for tourism and travel services.
H2:
Perceived value of ChatGPT will positively influence acceptance of using ChatGPT for travel-related purposes.
Perceived trust and perceived expertise
Tourists’ willingness to rely on AI-powered ChatGPT recommendations is influenced by two key organism variables: perceived trust and perceived expertise. Perceived trust refers to tourists’ confidence in ChatGPT’s ability to provide truthful, accurate, and timely travel information (Palos-Sanchez et al., Citation2021). Percevied expertise refers to tourists’ assessment of ChatGPT’s competence and skills in delivering high-quality travel information (Ohanian, Citation1990). Perceived trust and expertise are essential dimensions of source credibility that influence information acceptance (Y. Choi et al., Citation2021; Jang et al., Citation2021), especially for information provided by novel technologies like ChatGPT, where limited user familiarity and experience may pose risks (Pavlou, Citation2003). Tourists who doubt ChatGPT’s trustworthiness or expertise will likely avoid relying on it for travel-related services (Dwivedi, Kshetri, et al., Citation2023).
Social influence enhances perceptions of reliability and expertise in tourism, as individuals seek social recognition, approval, and belongingness (G. Zhu et al., Citation2017). Social information processing theory suggests people rely on trusted friends or peers to evaluate new technologies (Fulk et al., Citation1987). Early ChatGPT adopters in tourism may lack personal experience and rely heavily on others’ opinions to form initial perceptions of its competence. For example, if experienced travel bloggers express confidence that conversational AI like ChatGPT can offer useful personalized recommendations and practical trip planning advice, this positive evaluation from important referents may enhance tourist users’ own beliefs in ChatGPT’s expertise, ultimately motivating intentions to adopt ChatGPT. Previous studies have shown that social influence plays a vital role in fostering consumers’ perceived trust and expertise in various contexts, such as UGC websites, online banking services, and service robots (Chaouali et al., Citation2016; Della Corte et al., Citation2023; Hazari et al., Citation2017).
Perceived value is a critical antecedent of perceived trust and expertise, particularly when adopting new technologies (B. Hu et al., Citation2023; Uzir et al., Citation2021). This is because users who perceive new technologies as valuable are more motivated to overcome risks and trust them (Xie et al., Citation2021). The large language model like ChatGPT, still in their infancy in tourism, may raise concerns among potential users due to limited familiarity (B. Hu et al., Citation2023; Xie et al., Citation2021). However, recognizing ChatGPT’s functional benefits and added value can enhance tourists’ beliefs in its ability to deliver reliable and professional information that meets their travel needs. Moreover, rational decision-making theory suggests that individuals perform a mental cost-benefit analysis and are motivated to maximize perceived gains when evaluating new innovations (Kleijnen et al., Citation2007). Thus, when tourists believe ChatGPT can offer value-added services exceeding traditional search engines and real-person travel consultants, this high perceived value can boost their confidence in ChatGPT’s competence and qualifications to give helpful and accurate travel recommendations (Gursoy et al., Citation2023). Based on above discussion, the following hypotheses are proposed:
H3:
Social influence will positively influence perceived expertise in information provided by ChatGPT.
H4:
Social influence will positively influence perceived trust in information provided by ChatGPT.
H5:
Percevied value will positively influence perceived expertise in information provided by ChatGPT.
H6:
Percevied value will positively influence perceived trust in information provided by ChatGPT.
H7:
Percevied expertise will positively influence tourists’ acceptance of ChatGPT for travel-related purposes.
H8:
Percevied trust will positively influence tourists’ acceptance of ChatGPT for travel-related purposes.
Parasocial interaction
Parasocial interaction (PSI) is a one-sided emotional intimacy relationship that audiences develop with fictional or media personalities (Horton & Richard Wohl, Citation1956). Parasocial relationship often emerge between readers and fictional literary characters (Giles, Citation2002). In the era of social media, PSI has become increasingly prevalent as audiences have more opportunities to interact with Youtubers, TikTok creators and social media influencers (Rasmussen, Citation2018). Though these parasocial relationships, people tend to image media characters as if they are real-life friends (Giles, Citation2002; M. Lee & Park, Citation2022; Haobin Ye et al., Citation2021). While the specific targets of parasocial interaction may differ, the nature of parasocial interaction remains the same: these relationships appear bidirectional but are actually one-sided, with the target character is unaware of the other people’s parasocial relationships (Gómez-Morales et al., Citation2022; Jarzyna, Citation2021). Parasocial interaction theory has been extensively used to explain the effect of various types of target characters, such as video bloggers (Sakib et al., Citation2020; Yousaf, Citation2022), film celebrities (Bonus et al., Citation2021; Tucker, Citation2020) and social media influencers (Yang & Ha, Citation2021; Yuan & Lou, Citation2020), on consumers’ attitudes towards brand marketing and consumer purchasing decision. These studies support that parasocial relationships play an important role in shaping people’s attitudes and behaviours.
User interaction with ChatGPT contribute to its development of parasocial relationships can be categorized into three key aspects. First, ChatGPT adapt its language style to user-defined roles, enhancing users’ perceived expertise in information it provides and facilitating relational bounds (Klaus & Zaichkowsky, Citation2020). For example, users may request ChatGPT act as a tourism ambassador, providing personalized recommendations tailored to their travel preference. When responding to user queries, ChatGPT not only provides trusted information and practical solutions, but it may also address users as “honoured guests” or “distinguished guests” to elicit positive emotional responses.
Second, ChatGPT can provide great emotional value to users through its supportive and affirming interaction style. Self-determination theory posits that relatedness is a key need individuals require when accepting a new technology (Zhang et al., Citation2024). A sense of relatedness is the desire to feel cared for and connected with others (Ruan & Mezei, Citation2022). Unlike a human tourism consultant, ChatGPT is designed without any self-interest, so it does not exhibit impatience in the face of constant questions and requests from the users. Moreover, ChatGPT often initiates conversations with affirmations or expressions of appreciation, such as “certainly,” “of course,” or “you are right” to convey empathy and understanding towards users.
Third, ChatGPT’s advanced memory function allows it to learn a user’s viewpoints, preferences and other personal characteristics through frequent conservations. As users disclose more information, ChatGPT can tailor its conversational style to align with users’ perspectives and preferences. Social penetration theory suggests that relationships progress through gradually increasing self-disclosure (Altman & Taylor, Citation1973). This cumulative process of self-disclosure may enable users immerse themselves in their chosen conversation roles and believe they are cognitively and emotionally engaged in a two-way dialogue (Labrecque, Citation2014; Pentina et al., Citation2023). Such parasocial interactions can further develop to the point where consumers begin to view ChatGPT more as a trusted friend or a professional travel assistant rather than a virtual AI model (Pentina et al., Citation2023; Stern et al., Citation2007). Consequently, users may become more willing to rely on ChatGPT for travel-related services. In summary, this study posits that parasocial interaction plays a mediating role between tourists’ perceptions of trustworthiness and expertise in the information provided by ChatGPT and their acceptance of ChatGPT for travel-related services. Based on above discussion, the following hypotheses are proposed:
H9:
Perceived information expertise provided by ChatGPT will positively influence parasocial interaction.
H10:
Perceived trust in the information provided by ChatGPT will positively influence parasocial interaction.
H11:
Parasocial interaction will positively influence acceptance of ChatGPT for travel-related purposes.
Methodology
Participants and procedure
To understand whether users accept ChatGPT for travel-related services and how parasocial interaction influences their acceptance, tourists with a minimum of six months of experience using ChatGPT were recruited as participants. In order to obtain more accurate results with a larger sample size, an online questionnaire was utilized to collect data for this study. The questionnaire was distributed via Wenjuanxing (www.wenjuanxing.com), the largest online survey platform in China, from April to July 2023. The target respondents were those who had utilized ChatGPT for travel-related purposes, so submissions were only collected from users who passed the two screening questions (i.e. had used ChatGPT for over six months and had ever used ChatGPT for travel-related purposes). Online surveys are commonly employed in existing technology acceptance investigations (e.g. Chi et al., Citation2023; Gursoy et al., Citation2019) as they can approach much broader users and provide a more representative sample than traditional on-site data collection methods (Buhrmester et al., Citation2011).
Before the main study, a pilot on-site survey was conducted at three universities in Macau to test the questionnaire’s reliability and validity. The target respondents were university students, teachers, and staff who had experience using ChatGPT for travel-related purposes. This population was selected based on their forward-thinking mindset and recognition of the value of technology in both professional and personal aspects. Given these characteristics, they were considered more likely to adopt ChatGPT for travel-related services. The questionnaire was a Likert-type survey in which responses were measured on a 5-point scale ranging from 1 (strongly disagree) to 5 (strongly agree). As a result, the pilot survey yielded 117 valid questionnaires from respondents meeting the screening criteria (50.4% female, 58.12% with a master’s degree, and 76.24% in the 18–30 age group). Next, the pilot data were analysed to ensure the reliability of the selected measures. Results demonstrated acceptable Cronbach’s alpha value exceeding .70 for all measures, indicating adequate internal consistency for broader investigations with more diverse populations (Taber, Citation2018).
After the pilot study, the questionnaire was distributed online. Two screening questions were still included at the beginning of the online survey: “Have you ever used ChatGPT over six months?” and “Have you ever used ChatGPT for travel-related purposes?” to filter respondents. Only respondents who answered “Yes” to both questions were considered eligible to participate. In total, 450 responses were recorded, of which 331 responses were retained after excluding those with short answer times (less than 10 minutes) or pattern answers. This yielded an effective response rate of 73.56% for data analysis. This study employed 25 question items to evaluate the proposed framework. Hair et al. (Citation2012) recommends a sample-to-variable ratio of 5–10, suggesting a minimum sample size of 125–250. With 313 valid responses, our study exceeds this ratio, ensuring sufficient data for reliable and valid results. Participants worked in a variety of occupations, including managerial (37.5%), student (32%), professional (19.6%), and sales (9.7%). The detailed demographic characteristics are presented in .
Table 1. Profile of survey respondents (n = 331).
Measures
To ensure our measures’ initial reliability and validity, all scales used in this study have previously been validated in earlier studies. Social influence was measured using a 5-item scale adapted from Ribeiro et al. (Citation2022). The four measures for perceived value were adapted from de Kervenoael et al. (Citation2020) and Lalicic and Weismayer (Citation2021). Expertise and trust were each measured with 4 items adapted from Muda and Hamzah (Citation2021). Parasocial interaction was assessed using a 4-item scale from I. Kim and Kim (Citation2017). Finally, acceptance of ChatGPT for travel services was evaluated with a scale adapted from Ivanov et al. (Citation2017), Melián-González et al. (Citation2021) and Ribeiro et al. (Citation2022). All the constructs were measured on 5-point Likert scales (1=strongly disagree to 5=strongly agree). Since all scales were derived from English literature, a rigorous translation and back-translation process was performed to accurately translate them into Chinese. shows the variable used to test the model.
Table 2. Reliability and validity indicators, and scale items of the model.
Data analysis
Structural equation modelling (SEM) was utilized to test the hypothesized relationships and validate the proposed conceptual model (See ). Partial least squares SEM (PLS-SEM) was conducted using SmartPLS (version 4.0.9.2) to estimate the measurement and structural models. PLS-SEM was selected as the variance-based approach is better suited for predicting key target constructs and testing exploratory relationships (J. Hair et al., Citation2023).
The two-stage PLS-SEM method outlined by Henseler (Citation2017) was applied. In the first stage, the measurement (outer) model was examined by assessing item loadings, internal consistency reliability, convergent validity, and discriminant validity. Item loadings, Cronbach’s α, and composite reliability (CR) were used to evaluate internal consistency reliability. Convergent validity was assessed based on average variance extracted (AVE) exceeding 0.50 (J. F. Hair et al., Citation2013). Discriminant validity was established using the Fornell-Larcker criterion by comparing the square root of the AVE values with inter-construct correlations (Fornell & Larcker, Citation1981). In the second stage, the structural (inner) model was analysed to test the hypothesized relationships between constructs. Bootstrapping with 5,000 subsamples was used to generate standard errors and t-values (J. Hair et al., Citation2023).
Common method variance (CMV) was also assessed prior to analysing the PLS-SEM model since this study relied on self-reported data collected through a single questionnaire, which have the potential to result in inflated correlations between focal constructs (Podsakoff et al., Citation2003). To mitigate this issue, both procedural and statistical remedies were implemented to control for CMV. Procedurally, confidentiality, anonymity, and no right or wrong answers were emphasized in the online survey introduction, which are recognized methods for minimizing CMV (Podsakoff et al., Citation2003). Statistically, Harman’s single factor was performed (Min et al., Citation2016). The results indicated that the first factor explained only 38.52% of the variation in each measured item without rotation, which is below the accepted threshold of 50% (J. Hair et al., Citation2023). Based on these results as not a significant concern in this study.
Results
Measurement model
Reliability
Data normality was confirmed prior to evaluating the measurement model. Kurtosis and skewness values ranged from −0.50 to 1.50 across all items, indicating approximate normality (J. F. Hair et al., Citation2013). To evaluate the internal consistency reliability of the scales, both Cronbach’s alpha and composite reliability (CR) values were examined (J. F. Hair et al., Citation2013). While Cronbach’s alpha assesses the lower bound estimate of reliability, CR represents the upper-bound value. Generally, values exceeding 0.70 for Cronbach’s alpha and 0.80 for CR indicate satisfactory reliability (Nunnally & Bernstein, Citation1994). The overall scale demonstrated strong internal consistency, with a Cronbach’s alpha of 0.939. The Cronbach’s alpha for each individual construct also exhibited good reliability (See ): social influence (α = .878), perceived value (α = .783), expertise (α = .789), trust (α = .789), parasocial interaction (α = .716) and acceptance behaviour (α = .772). Furthermore, CR values ranged from 0.824 to 0.911, also exceeding the recommended level of 0.80 (J. F. Hair et al., Citation2013). Thus, these results provide evidence for the credibility and internal consistency of the scales used in this study.
Evaluation of the measurement model fit
The PLS-SEM assesses model fit by examining the standardized root mean square residual (SRMR) values using a bootstrapping procedure. According to J. F. Hair et al. (Citation2013) and L.-T. Hu and Bentler (Citation1998), a SRMR value less than 0.1, or more conservatively 0.08, indicates acceptable fit. Besides, Henseler (Citation2017) suggest a SRMR value below the 95% bootstrap quantile (HI95 of SRMR) and dULS value below 95% bootstrap quantile (HI95 of dULS) are indicative of good model fit. In the current study, these requirements for adequate model fit are satisfied as the obtained SRMR value is 0.065, below the acceptable 0.08 threshold (Henseler, Citation2017). Specifically, the dULS value of 1.364 is below the 95% bootstrap quantile value of 1.386 obtained through 5000 resamples.
Convergent validity
Convergent validity was assessed by examining the outer loadings of the indicators and the average variance extracted (AVE) values (J. F. Hair et al., Citation2013). Indicator loadings were significant (p < .001) and exceeded the recommended threshold value of 0.7, with values ranging from 0.742 to 0.846 (See ). Additionally, the AVE values for all constructs surpassed the suggested cutoff value of 0.5, ranging from 0.540 to 0.671 (Fornell & Larcker, Citation1981; J. F. Hair et al., Citation2013). These results confirm that the indicators effectively captured variance in their respective constructs, demonstrating adequate convergent validity.
Discriminant validity
Discriminant Validity was assessed using the heterotrait-monotrait ratio (HTMT) technique (Henseler, Citation2017). HTMT values between all reflective construct pairs were below the conservative threshold value of 0.85, ranging from 0.342 to 0.729 (See ). Furthermore, the Fornell-Larcker criterion analysis revealed that the square root of the AVE for each construct exceeded inter-construct correlations (Fornell & Larcker, Citation1981). Together, these findings provide evidence that the constructs measure distinct concepts, establishing satisfactory discriminant validity.
Table 3. Discriminant validity.
Hypothesis testing
Hypotheses were tested using 5000 bootstrap subsamples. The results revealed support for most hypotheses, except for H1 and H7 (See and ). Contrary to our predictions, social influence did not directly affect adoption behaviour (β = −0.022, p > .005), rejecting H1. However, there was a significant direct effect between perceived value and ChatGPT adoption for travel (β = 0.265, p < .001), supporting H2. This suggests that tourists’ perceived value of ChatGPT is a strong predictor with their adoption of ChatGPT for travel purposes. Moreover, the results indicated a significant positive relationship between the two stimuli cues of social influence (β = 0.297, p < .001) and perceived value (β = 0.527, p < .001) with expertise in Chat GPT provided information, supporting H3 and H5. Social influence (β = 0.345, p < .001) and perceived value (β = 0.458, p < .001) were both positively related to user trust in ChatGPT information, supporting H4 and H6. While trust directly and significantly influenced intention to adopt Chat GPT for travel purposes (β = 0.264, p < .001), supporting H8; the direct effect of expertise was not significant (β = 0.069, p > .005), rejecting H7. Finally, higher perceived expertise (β = 0.379, p < .001) and trust (β = 0.380, p < .001) in Chat GPT information yielded a higher level of parasocial interaction with Chat GPT, and intention to adopt ChatGPT for travel purposes (β = 0.181, p < .001), supporting H9, H10, and H11.
Table 4. Path estimates and hypothesis testing.
The predictive power of the model was assessed using the coefficient of determination R2. The R2 value indicates the proportion of variance in an endogenous construct explained by its predictor variables. In this study, the R2 values demonstrated that the model accounted for a substantial amount of variance in perceived expertise (56.8%), trust (53.2%), parasocial interaction (49.9%), and intention to adopt Chat GPT for travel purposes (44.9%). Overall, these results provide evidence that the key variables in proposed model have good explanatory power for the endogenous constructs.
Discussion
Grounded in S-O-R theory, this study developed and tested a conceptual model elucidating the mechanisms driving tourists’ acceptance of utilizing ChatGPT for travel and tourism services. The model identified social influence and perceived value as two key external stimuli that activate tourists’ perceived trust and expertise in the travel information provided by ChatGPT (cognitive process) and parasocial interaction with ChatGPT (psychological process) and determine tourists’ adoption intentions and behaviour towards ChatGPT.
Firstly, the results suggest that social influence is a key external stimulus that positively shapes the tourists’ perceived trust and expertise in the travel information provided by ChatGPT. This finding is consistent with prior technology acceptance research (Ladeira et al., Citation2023; Lu et al., Citation2019), which highlights the importance of group norms and social approval in users’ appraisals of an AI-powered language models. Specifically, when tourists perceive that their important social groups (e.g. family and friends) encourage using ChatGPT for tourism and travel-related service, they likely develop more favourable evaluations of ChatGPT’s capabilities. However, social influence does not directly affect tourists’ intentions to use ChatGPT for travel-related services. This suggests that while social group can help to shape tourists’ perceptions of ChatGPT’s expertise and trust, additional factors beyond expertise and trust are needed to determine adoption behaviour. This aligns with dual-process theory (Frankish, Citation2010; McCabe et al., Citation2016), whereby both implicit social influences and explicit deliberative evaluations jointly shape individual decisions and behaviours.
Secondly, the results indicate that perceived value is the most influential determinant of adoption of ChatGPT for travel-related purposes. It was found to affect ChatGPT acceptance both directly and indirectly. The direct impact of perceived value on behaviour is consistent with previous consumer research emphasizing its importance in adoption decisions (M. Choi et al., Citation2023; Shaw & Sergueeva, Citation2019). This direct effect suggests that tourists perform a rational cost-benefit analysis when evaluating ChatGPT for travel-related services. Specifically, when functional, economic, or emotional benefits of using ChatGPT for travel-related service are perceived to outweigh costs, tourists exhibit a stronger willingness to adopt. On the other hand, the perceived value also indirectly influenced tourists’ acceptance intentions by enhancing their perception of ChatGPT’s expertise and trust. This finding reveals that when travellers perceive greater overall value in using ChatGPT, they develop more favourable evaluations of its recommendations and provided information.
Thirdly, the results reveal that perceived trust and expertise in the information provided by ChatGPT are important factors that influence travellers’ parasocial interactions with ChatGPT and their willingness to use it for travel-related services. Specifically, beyond perceived functional or economic benefits, when travellers view ChatGPT as a trusted and professional virtual advisor, they are more likely to anthropomorphize ChatGPT and develop one-sided emotional bonds with it. This highlights the significance of parasocial phenomena in shaping users’ acceptance and use of ChatGPT. In essence, despite realizing ChatGPT was not human, travellers engage with it parasocially as if it were a trusted travel expert. Our study thus extends previous academic findings, which have focused more narrowly on audience and media characters relationship (S. Choi et al., Citation2019; Haobin Ye et al., Citation2021), to human-AI-powered language model interaction. Additionally, this study found that perceived trust in information provided by ChatGPT directly influenced travellers’ adoption and usage of ChatGPT for travel and tourism purposes. In contrast, perceived expertise in ChatGPT’s information did not directly affect traveller’s adoption behaviours. This discrepancy highlights the unique importance of trust in shaping AI-powered language model adoption, like ChatGPT, for travel-related services.
Theoretical implications
This study makes significant theoretical contributions by advancing the understanding of tourist acceptance for emerging conversational AI like ChatGPT in travel and tourism. Addressing a crucial gap where established models (e.g. TAM, UTAUT) often fall short in capturing nuances of human-computer interaction, the current study employs SOR theory as a foundation. Building upon it, this study proposes and validate a conceptual framework tailored specifically to ChatGPT acceptance for travel-related purposes. This framework incorporates key factors unique to this context, including social influence, perceived value, trust, expertise, and parasocial interaction, providing a more nuanced understanding of user acceptance.
Secondly, the findings suggest that fostering positive perceptions of trust and expertise in information provided by ChatGPT is crucial for enhancing tourists’ parasocial interaction with ChatGPT and their willingness to utilize ChatGPT for travel and tourism purposes. To augment the AI model’s knowledge base in the context of travel and tourism, destination managers can establish channels for tourists to provide feedback on the accuracy and relevance of information delivered by ChatGPT. This feedback can then be relayed to AI model developers, who can utilize it to consider specialized training for the AI model. This will ensure that tourists can make informed decisions based on reliable information. Furthermore, the research findings indicate that the perceived trustworthiness of information provided by ChatGPT exerts a stronger direct influence on tourists’ acceptance of ChatGPT. Consequently, destination managers should prioritize effectively communicating the trustworthiness and reliability of ChatGPT to users, fostering tourists’ confidence in relying on ChatGPT for travel advice or other tourism-related services.
Thirdly, this research expands the boundaries of parasocial interaction theory, extending its applicability to the novel realm of human-AI interaction. This study empirically demonstrate that tourists develop parasocial bonds with ChatGPT, akin to human friendship (Perse & Rubin, Citation1989). The current study provides empirical evidence that people can form parasocial bounds with AI model similar to human friendships. Just as audiences build imaginary relationships with media influencers they perceive as a friend, the results of this study indicate that tourists develop parasocial bonds with ChatGPT, treating it as a helpful travel assistant. Moreover, this study reveals tourists’ perceptions of ChatGPT’s expertise and trustworthiness increase their parasocial engagement with the AI. This reinforces in human-AI model relationship, parasocial interaction theory’s emphasis on perceived expertise and trust as key antecedents of imaginary relationships (Horton & Richard Wohl, Citation1956).
Finally, the proposed theoretical framework comprehensively explains how functional and social factors interact to influence users’ adoption of ChatGPT for travel and tourism purposes. The results indicated both the objective qualities of AI-provided information as well as subjective psychological engagement determine the likelihood of adoption. This study thus advances knowledge and enriches theories of AI adoption by examining these cognitive-psychological factors behind ChatGPT’s adoption.
Practical implications
The findings of this research offer several valuable managerial insights for various stakeholders, including destination managers, AI model developers and policy makers. Firstly, to boost the adoption of AI models like ChatGPT application for travel purposes, destination manager should recognize that perceived value of ChatGPT, compared to social influence, is a key driver of adoption. Therefore, they can focus on promotions and conveying the practical benefits and advantages of using such systems. For example, they can place a ChatGPT plugin on the official destination website and encourage tourists to use it to find relevant information, plan their itineraries, or ask for personalized recommendations. As perceived value has a strong and direct impact on tourists’ acceptance of ChatGPT for travel-related purposes, this is a critical step. Additionally, as AI technology is continually evolving, AI model developers should make continuous improvements and regular updates and enhancements based on user feedback. This will help to ensure that ChatGPT is more effective application in tourism domain.
Secondly, the research findings suggest that fostering positive perceptions of trust and expertise in information provided by ChatGPT is crucial for enhancing tourists’ parasocial interaction with ChatGPT and their willingness to utilize ChatGPT for travel and tourism purposes. To augment the AI model’s knowledge base in the context of travel and tourism, destination managers can establish channels for tourists to provide feedback on the accuracy and relevance of information delivered by ChatGPT. This feedback can then be relayed to AI model developers, who can utilize it to consider specialized training for the AI model. This will ensure that tourists can make informed decisions based on reliable information. Furthermore, the research findings indicate that the perceived trustworthiness of information provided by ChatGPT exerts a stronger direct influence on tourists’ acceptance of ChatGPT. Consequently, destination managers should prioritize effectively communicating the trustworthiness and reliability of ChatGPT to users, fostering tourists’ confidence in relying on ChatGPT for travel advice or other tourism-related services.
Thirdly, the findings underscore the significance of parasocial interaction as crucial mediating factor in tourists’ acceptance of ChatGPT for travel and tourism purposes. Destination marketers can strategically identify scenarios that encourage tourists to adopt ChatGPT for relevant services, thereby enhancing their comfort and facilitating a strong connection between tourists and AI-driven conservational models. For example, destination managers can actively encourage tourists to adopt ChatGPT for routine itinerary recommendations. By deploying ChatGPT with features such as multi-language support and 7/24 availability, it can reinforce tourists parasocial interaction with AI, thereby reducing the operation cost of tourism company. However, it is essential to recognize that ChatGPT may not be the ideal choice for scenario such as emergency situation or guiding outdoor adventures, where safety is a major concern due to heightened risks associated with such activities. In such scenarios, ChatGPT’s inability to provide detailed and real-time information can not only diminish parasocial interaction but also potentially pose safety risks. Therefore, the selection of suitable scenarios for ChatGPT interaction with tourists is crucial to ensure both their satisfaction and safety during travels.
On the other hand, while fostering parasocial interaction with ChatGPT can significantly enhance tourists’ acceptance of AI-powered language model in tourism and travel services, it also raises ethical considerations. Tourism policymakers must collaborate with stakeholders to establish comprehensive ethical guidelines for the use of AI-driven conservational models like ChatGPT in the tourism domain. These guidelines should encompass various aspects, including safeguarding data privacy during AI interactions, ensuring transparency in AI’s role within the destination’s services, and addressing potential biases in AI algorithms. Additionally, tourists should be informed that while AI can provide valuable assistance, connecting with real people and immersing oneself in the local culture remain essential for a more enriching and authentic travel experience. Therefore, responsible AI-model deployment, ethical considerations, and transparency are crucial aspects that should be integrated into the fabric of AI integration in the travel and tourism industry.
Limitations
This study acknowledges some limitations that present opportunities for future research. Firstly, the data was solely collected from tourists in China through an online survey. This limits the generalizability of the findings to tourists from other cultural backgrounds. Future research could employ a cross-country approach to investigate how travellers from diverse cultures interact with and perceive ChatGPT for travel and tourism services. Examining the impact of parasocial interaction across cultures would significantly enrich this area of study. Secondly, this study relied on a single survey methodology. Future research could employ a mix of complementary methodologies for a more comprehensive investigation. Thirdly, this study tested a model with a limited set of variables explaining AI-powered language model acceptance in tourism and travel domain. Building on this study’s findings, future studies could incorporate additional factors like perceived usefulness, risk, and privacy concerns to provide a more comprehensive understanding of tourists’ AI model adoption decisions. Lastly, this research exclusively focused on ChatGPT acceptance. As new AI-powered language models emerge, such as Microsoft Bing, Claude 2, and Google Bard, researchers should explore acceptance of these alternative AI models. Comparing perceptions of different AI models would provide a more complete picture of AI models’ evolving role in the travel and tourism domain.
Disclosure statement
No potential conflict of interest was reported by the author(s).
References
- Altman, I., & Taylor, D. A. (1973). Social penetration: The development of interpersonal relationships. Holt, Rinehart & Winston.
- Ariza-Montes, A., Quan, W., Radic, A., Yu, J., & Han, H. (2023). Human values and traveler behaviors: Metaverse for conferences and meetings. Journal of Travel & Tourism Marketing, 40(6), 490–511. https://doi.org/10.1080/10548408.2023.2263766
- Au, W. C. W., & Tsang, N. K. (2022). What makes a destination smart? An intelligence-oriented approach to conceptualizing destination smartness. Journal of Travel & Tourism Marketing, 39(4), 448–464. https://doi.org/10.1080/10548408.2022.2116627
- Bader, A., Baldauf, M., Leinert, S., Fleck, M., & Liebrich, A. (2012). Mobile tourism services and technology acceptance in a mature domestic tourism market: The case of Switzerland. In M. Fuchs, F. Ricci, & L. Cantoni (eds.), Information and communication technologies in tourism 2012 (pp. 296–307). Springer.
- Bonus, J. A., Matthews, N. L., & Wulf, T. (2021). The impact of moral expectancy violations on audiences’ parasocial relationships with movie heroes and villains. Communication Research, 48(4), 550–572. https://doi.org/10.1177/0093650219886516
- Buhrmester, M., Kwang, T., & Gosling, S. D. (2011). Amazon’s Mechanical Turk: A new source of inexpensive, yet high-quality, data? Perspectives on Psychological Science, 6(1), 3–5. https://doi.org/10.1177/1745691610393980
- Bulchand-Gidumal, J. (2022). Impact of artificial intelligence in travel, tourism, and hospitality. In Z. Xiang, M. Fuchs, U. Gretzel, & W. Höpken (eds.), Handbook of e-Tourism (pp. 1943–1962). Springer.
- Cai, D., Li, H., & Law, R. (2022). Anthropomorphism and OTA chatbot adoption: A mixed methods study. Journal of Travel & Tourism Marketing, 39(2), 228–255. https://doi.org/10.1080/10548408.2022.2061672
- Calvaresi, D., Ibrahim, A., Calbimonte, J.-P., Fragniere, E., Schegg, R., & Schumacher, M. I. (2021). Leveraging inter-tourists interactions via chatbots to bridge academia, tourism industries and future societies. Journal of Tourism Futures, 9(3), 211–337. https://doi.org/10.1108/JTF-01-2021-0009
- Carvalho, I., & Ivanov, S. (2023). ChatGPT for tourism: Applications, benefits and risks. Tourism Review, 79(2), 290–303. https://doi.org/10.1108/TR-02-2023-0088
- Chaouali, W., Yahia, I. B., & Souiden, N. (2016). The interplay of counter-conformity motivation, social influence, and trust in customers’ intention to adopt internet banking services: The case of an emerging country. Journal of Retailing and Consumer Services, 28, 209–218. https://doi.org/10.1016/j.jretconser.2015.10.007
- Chi, O. H., Chi, C. G., Gursoy, D., & Nunkoo, R. (2023). Customers’ acceptance of artificially intelligent service robots: The influence of trust and culture. International Journal of Information Management, 70, 102623. https://doi.org/10.1016/j.ijinfomgt.2023.102623
- Chi, O. H., Jia, S., Li, Y., & Gursoy, D. (2021). Developing a formative scale to measure consumers’ trust toward interaction with artificially intelligent (AI) social robots in service delivery. Computers in Human Behavior, 118, 106700. https://doi.org/10.1016/j.chb.2021.106700
- Choi, M., Choi, Y., Nosrati, S., Hailu, T. B., & Kim, S. (2023). Psychological dynamics in the metaverse: Evaluating perceived values, attitude, and behavioral intention in metaverse events. Journal of Travel & Tourism Marketing, 40(7), 602–618. https://doi.org/10.1080/10548408.2023.2276435
- Choi, S., Kim, I., Cha, K., Suh, Y.-K., & Kim, K.-H. (2019). Travelers’ parasocial interactions in online travel communities. Journal of Travel & Tourism Marketing, 36(8), 888–904. https://doi.org/10.1080/10548408.2019.1657053
- Choi, Y., Lee, J., & Choe, Y. (2021). Online travel information filtering: Role of commercial cues in trust and distrust mechanisms. Journal of Travel & Tourism Marketing, 38(7), 710–724. https://doi.org/10.1080/10548408.2021.1985037
- Chua, B.-L., Kim, S., Hailu, T. B., Yu, J., Moon, H., & Han, H. (2023). Emerging armchair travel: Investigation of authentic armchair travel experiences and their effect on armchair travel stickiness. Journal of Travel & Tourism Marketing, 40(3), 203–220. https://doi.org/10.1080/10548408.2023.2236652
- Confente, I., & Vigolo, V. (2018). Online travel behaviour across cohorts: T he impact of social influences and attitude on hotel booking intention. International Journal of Tourism Research, 20(5), 660–670. https://doi.org/10.1002/jtr.2214
- de Kervenoael, R., Hasan, R., Schwob, A., & Goh, E. (2020). Leveraging human-robot interaction in hospitality services: Incorporating the role of perceived value, empathy, and information sharing into visitors’ intentions to use social robots. Tourism Management, 78, 104042. https://doi.org/10.1016/j.tourman.2019.104042
- Della Corte, V., Sepe, F., Gursoy, D., & Prisco, A. (2023). Role of trust in customer attitude and behaviour formation towards social service robots. International Journal of Hospitality Management, 114, 103587. https://doi.org/10.1016/j.ijhm.2023.103587
- Demir, M., & Demir, Ş. Ş. (2023). Is ChatGPT the right technology for service individualization and value co-creation? evidence from the travel industry. Journal of Travel & Tourism Marketing, 40(5), 383–398. https://doi.org/10.1080/10548408.2023.2255884
- Dwivedi, Y. K., Kshetri, N., Hughes, L., Slade, E. L., Jeyaraj, A., Kar, A. K., Baabdullah, A. M., Koohang, A., Raghavan, V., Ahuja, M., Albanna, H., Albashrawi, M. A., Al-Busaidi, A. S., Balakrishnan, J., Barlette, Y., Basu, S., Bose, I., Brooks, L., Buhalis, D., … Wirtz, J. (2023). Opinion paper: “so what if ChatGPT wrote it?” multidisciplinary perspectives on opportunities, challenges and implications of generative conversational AI for research, practice and policy. International Journal of Information Management, 71, 102642. https://doi.org/10.1016/j.ijinfomgt.2023.102642
- Dwivedi, Y. K., Pandey, N., Currie, W., & Micu, A. (2023). Leveraging ChatGPT and other generative artificial intelligence (AI)-based applications in the hospitality and tourism industry: Practices, challenges and research agenda. International Journal of Contemporary Hospitality Management, 36(1), 1–12. https://doi.org/10.1108/IJCHM-05-2023-0686
- Fornell, C., & Larcker, D. F. (1981). Structural equation models with unobservable variables and measurement error: Algebra and statistics. Journal of Marketing Research, 18(3), 382–388. https://doi.org/10.2307/3150980
- Frankish, K. (2010). Dual‐process and dual‐system theories of reasoning. Philosophy Compass, 5(10), 914–926. https://doi.org/10.1111/j.1747-9991.2010.00330.x
- Fulk, J., Steinfield, C. W., Schmitz, J., & Power, J. G. (1987). A social information processing model of media use in organizations. Communication Research, 14(5), 529–552. https://doi.org/10.1177/009365087014005005
- Giles, D. C. (2002). Parasocial interaction: A review of the literature and a model for future research. Media Psychology, 4(3), 279–305. https://doi.org/10.1207/S1532785XMEP0403_04
- Gómez-Morales, B., Nieto-Ferrando, J., & Sánchez-Castillo, S. (2022). (Re) visiting game of thrones: Film-induced tourism and television fiction. Journal of Travel & Tourism Marketing, 39(1), 73–86. https://doi.org/10.1080/10548408.2022.2044971
- Gursoy, D., Chi, O. H., Lu, L., & Nunkoo, R. (2019). Consumers acceptance of artificially intelligent (AI) device use in service delivery. International Journal of Information Management, 49, 157–169.
- Gursoy, D., Li, Y., & Song, H. (2023). ChatGPT and the hospitality and tourism industry: An overview of current trends and future research directions. Journal of Hospitality Marketing & Management, 32(5), 579–592. https://doi.org/10.1080/19368623.2023.2211993
- Habib, S., & Hamadneh, N. N. (2021). Impact of perceived risk on consumers technology acceptance in online grocery adoption amid COVID-19 pandemic. Sustainability, 13(18), 10221. https://doi.org/10.3390/su131810221
- Hair, J., Hair, J. F., Jr., Sarstedt, M., Ringle, C. M., & Gudergan, S. P. (2023). Advanced issues in partial least squares structural equation modeling. Sage Publications.
- Hair, J. F., Ringle, C. M., & Sarstedt, M. (2013). Partial least squares structural equation modeling: Rigorous applications, better results and higher acceptance. Long Range Planning, 46(1–2), 1–12. https://doi.org/10.1016/j.lrp.2013.01.001
- Hair, J. F., Sarstedt, M., Pieper, T. M., & Ringle, C. M. (2012). The use of partial least squares structural equation modeling in strategic management research: A review of past practices and recommendations for future applications. Long range planning, 45(5–6), 320–340.
- Haleem, A., Javaid, M., & Singh, R. P. (2022). An era of ChatGPT as a significant futuristic support tool: A study on features, abilities, and challenges. BenchCouncil Transactions on Benchmarks, Standards and Evaluations, 2(4), 100089. https://doi.org/10.1016/j.tbench.2023.100089
- Haobin Ye, B., Fong, L. H. N., & Luo, J. M. (2021). Parasocial interaction on tourism companies’ social media sites: Antecedents and consequences. Current Issues in Tourism, 24(8), 1093–1108. https://doi.org/10.1080/13683500.2020.1764915
- Hazari, S., Bergiel, B. J., & Sethna, B. N. (2017). Hedonic and utilitarian use of user-generated content on online shopping websites. Journal of Marketing Communications, 23(6), 572–591. https://doi.org/10.1080/13527266.2016.1143383
- Henseler, J. (2017). Partial least squares path modeling. In Peter S. H. Leeflang, Jaap E. Wieringa, Tammo H. A. Bijmolt, & Koen H. Pauwels (eds.), Advanced methods for modeling markets (pp. 361–381). Springer.
- Horton, D., & Richard Wohl, R. (1956). Mass communication and para-social interaction: Observations on intimacy at a distance. Psychiatry, 19(3), 215–229. https://doi.org/10.1080/00332747.1956.11023049
- Hu, L.-T., & Bentler, P. M. (1998). Fit indices in covariance structure modeling: Sensitivity to underparameterized model misspecification. Psychological Methods, 3(4), 424–453. https://doi.org/10.1037/1082-989X.3.4.424
- Hu, B., Liu, Y.-L., & Yan, W. (2023). Should I scan my face? The influence of perceived value and trust on Chinese users’ intention to use facial recognition payment. Telematics and Informatics, 78, 101951. https://doi.org/10.1016/j.tele.2023.101951
- Hwang, J., Kim, H. M., Joo, K.-H., & Kim, J. J. (2022). The antecedents and consequences of brand authenticity in the restaurant industry: Robot service employees versus human service employees. Journal of Travel & Tourism Marketing, 39(2), 256–270. https://doi.org/10.1080/10548408.2022.2061678
- Ivanov, S. H., Webster, C., & Berezina, K. (2017). Adoption of robots and service automation by tourism and hospitality companies. Revista Turismo & Desenvolvimento, 27(28), 1501–1517.
- Jang, W., Kim, J., Kim, S., & Chun, J. W. (2021). The role of engagement in travel influencer marketing: The perspectives of dual process theory and the source credibility model. Current Issues in Tourism, 24(17), 2416–2420. https://doi.org/10.1080/13683500.2020.1845126
- Jarzyna, C. L. (2021). Parasocial interaction, the COVID-19 quarantine, and digital age media. Human Arenas, 4(3), 413–429. https://doi.org/10.1007/s42087-020-00156-0
- Kim, S.-E., & Jung, S. (2022). Exploring virtual race participants’ perceptions of event mobile apps and behavioral intention: The stimulus-organism-response approach. Journal of Convention & Event Tourism, 23(4), 365–383. https://doi.org/10.1080/15470148.2022.2102559
- Kim, I., & Kim, J. J. (2017). Older adults’ parasocial interaction formation process in the context of travel websites: The moderating role of parent-child geographic proximity. Tourism Management, 63, 399–416. https://doi.org/10.1016/j.tourman.2017.07.012
- Kim, H., Kim, S.-E., Park, K., & Tennessee, S. (2023). Exploring the role of flow experience and telepresence in virtual reality (VR) concerts. Journal of Travel & Tourism Marketing, 40(7), 568–582. https://doi.org/10.1080/10548408.2023.2276437
- Klaus, P., & Zaichkowsky, J. (2020). AI voice bots: A services marketing research agenda. Journal of Services Marketing, 34(3), 389–398. https://doi.org/10.1108/JSM-01-2019-0043
- Kleijnen, M., De Ruyter, K., & Wetzels, M. (2007). An assessment of value creation in mobile service delivery and the moderating role of time consciousness. Journal of Retailing, 83(1), 33–46. https://doi.org/10.1016/j.jretai.2006.10.004
- Labrecque, L. I. (2014). Fostering consumer–brand relationships in social media environments: The role of parasocial interaction. Journal of Interactive Marketing, 28(2), 134–148. https://doi.org/10.1016/j.intmar.2013.12.003
- Ladeira, W., Perin, M. G., & Santini, F. (2023). Acceptance of service robots: A meta-analysis in the hospitality and tourism industry. Journal of Hospitality Marketing & Management, 32(6), 694–716. https://doi.org/10.1080/19368623.2023.2202168
- Lalicic, L., & Weismayer, C. (2021). Consumers’ reasons and perceived value co-creation of using artificial intelligence-enabled travel service agents. Journal of Business Research, 129, 891–901. https://doi.org/10.1016/j.jbusres.2020.11.005
- Lecler, A., Duron, L., & Soyer, P. (2023). Revolutionizing radiology with GPT-based models: Current applications, future possibilities and limitations of ChatGPT. Diagnostic and interventional imaging, 104(6), 269–274.
- Lee, S., Ha, S., & Widdows, R. (2011). Consumer responses to high-technology products: Product attributes, cognition, and emotions. Journal of Business Research, 64(11), 1195–1200. https://doi.org/10.1016/j.jbusres.2011.06.022
- Lee, M., & Park, J. S. (2022). Do parasocial relationships and the quality of communication with AI shopping chatbots determine middle‐aged women consumers’ continuance usage intentions? Journal of Consumer Behaviour, 21(4), 842–854. https://doi.org/10.1002/cb.2043
- López-Nicolás, C., Molina-Castillo, F. J., & Bouwman, H. (2008). An assessment of advanced mobile services acceptance: Contributions from TAM and diffusion theory models. Information & Management, 45(6), 359–364. https://doi.org/10.1016/j.im.2008.05.001
- Lu, L., Cai, R., & Gursoy, D. (2019). Developing and validating a service robot integration willingness scale. International Journal of Hospitality Management, 80, 36–51. https://doi.org/10.1016/j.ijhm.2019.01.005
- Lv, L., Huang, M., Guan, D., & Yang, K. (2022). Apology or gratitude? The effect of communication recovery strategies for service failures of AI devices. Journal of Travel & Tourism Marketing, 39(6), 570–587. https://doi.org/10.1080/10548408.2022.2162659
- Ma, J., & Li, F. (2022). Effects of psychological distance and social influence on tourists’ hotel booking preferences. Journal of Travel & Tourism Marketing, 39(4), 394–411. https://doi.org/10.1080/10548408.2022.2116628
- McCabe, S., Li, C., & Chen, Z. (2016). Time for a radical reappraisal of tourist decision making? Toward a new conceptual model. Journal of Travel Research, 55(1), 3–15. https://doi.org/10.1177/0047287515592973
- Mehrabian, A., & Russell, J. A. (1974). An approach to environmental psychology. The MIT Press.
- Melián-González, S., Gutiérrez-Taño, D., & Bulchand-Gidumal, J. (2021). Predicting the intentions to use chatbots for travel and tourism. Current Issues in Tourism, 24(2), 192–210. https://doi.org/10.1080/13683500.2019.1706457
- Min, H., Park, J., & Kim, H. J. (2016). Common method bias in hospitality research: A critical review of literature and an empirical study. International Journal of Hospitality Management, 56, 126–135. https://doi.org/10.1016/j.ijhm.2016.04.010
- Muda, M., & Hamzah, M. I. (2021). Should I suggest this YouTube clip? The impact of UGC source credibility on eWOM and purchase intention. Journal of Research in Interactive Marketing, 15(3), 441–459. https://doi.org/10.1108/JRIM-04-2020-0072
- Murphy, J., Gretzel, U., & Pesonen, J. (2019). Marketing robot services in hospitality and tourism: The role of anthropomorphism. Journal of Travel & Tourism Marketing, 36(7), 784–795. https://doi.org/10.1080/10548408.2019.1571983
- Nunnally, J. C., & Bernstein, I. H. (1994). Psychometric theory (3rd ed.). McGraw-Hill.
- Ohanian, R. (1990). Construction and validation of a scale to measure celebrity endorsers’ perceived expertise, trustworthiness, and attractiveness. Journal of Advertising, 19(3), 39–52. https://doi.org/10.1080/00913367.1990.10673191
- Palos-Sanchez, P., Saura, J. R., & Correia, M. B. (2021). Do tourism applications’ quality and user experience influence its acceptance by tourists? Review of Managerial Science, 15(5), 1205–1241. https://doi.org/10.1007/s11846-020-00396-y
- Park, J., Hong, E., & Le, H. T. (2021). Adopting autonomous vehicles: The moderating effects of demographic variables. Journal of Retailing and Consumer Services, 63, 102687. https://doi.org/10.1016/j.jretconser.2021.102687
- Pavlou, P. A. (2003). Consumer acceptance of electronic commerce: Integrating trust and risk with the technology acceptance model. International Journal of Electronic Commerce, 7(3), 101–134.
- Pentina, I., Hancock, T., & Xie, T. (2023). Exploring relationship development with social chatbots: A mixed-method study of replika. Computers in Human Behavior, 140, 107600. https://doi.org/10.1016/j.chb.2022.107600
- Perse, E. M., & Rubin, R. B. (1989). Attribution in social and parasocial relationships. Communication Research, 16(1), 59–77. https://doi.org/10.1177/009365089016001003
- Pillai, R., & Sivathanu, B. (2020). Adoption of AI-based chatbots for hospitality and tourism. International Journal of Contemporary Hospitality Management, 32(10), 3199–3226. https://doi.org/10.1108/IJCHM-04-2020-0259
- Pillai, R., Sivathanu, B., & Dwivedi, Y. K. (2020). Shopping intention at AI-powered automated retail stores (AIPARS). Journal of Retailing and Consumer Services, 57, 102207. https://doi.org/10.1016/j.jretconser.2020.102207
- Podsakoff, P. M., MacKenzie, S. B., Lee, J.-Y., & Podsakoff, N. P. (2003). Common method biases in behavioral research: A critical review of the literature and recommended remedies. Journal of Applied Psychology, 88(5), 879–903. https://doi.org/10.1037/0021-9010.88.5.879
- Rasmussen, L. (2018). Parasocial interaction in the digital age: An examination of relationship building and the effectiveness of YouTube celebrities. Journal of Social Media in Society, 7(1), 280–294.
- Ribeiro, M. A., Gursoy, D., & Chi, O. H. (2022). Customer acceptance of autonomous vehicles in travel and tourism. Journal of Travel Research, 61(3), 620–636. https://doi.org/10.1177/0047287521993578
- Roy, P., Ramaprasad, B. S., Chakraborty, M., Prabhu, N., & Rao, S. (2020). Customer acceptance of use of artificial intelligence in hospitality services: An Indian hospitality sector perspective. Global Business Review, 25(3), 832–851. https://doi.org/10.1177/0972150920939753
- Ruan, Y., & Mezei, J. (2022). When do AI chatbots lead to higher customer satisfaction than human frontline employees in online shopping assistance? Considering product attribute type. Journal of Retailing and Consumer Services, 68, 103059. https://doi.org/10.1016/j.jretconser.2022.103059
- Sakib, M. N., Zolfagharian, M., & Yazdanparast, A. (2020). Does parasocial interaction with weight loss vloggers affect compliance? The role of vlogger characteristics, consumer readiness, and health consciousness. Journal of Retailing and Consumer Services, 52, 101733. https://doi.org/10.1016/j.jretconser.2019.01.002
- Sarkady, D., Neuburger, L., & Egger, R. (2021). Virtual reality as a travel substitution tool during COVID-19. Information and communication technologies in tourism 2021: Proceedings of the ENTER 2021 eTourism conference, January 19-22, 2021 (pp. 452–463). Springer.
- Seo, K. H., & Lee, J. H. (2021). The emergence of service robots at restaurants: Integrating trust, perceived risk, and satisfaction. Sustainability, 13(8), 4431. https://doi.org/10.3390/su13084431
- Shaw, N., & Sergueeva, K. (2019). The non-monetary benefits of mobile commerce: Extending UTAUT2 with perceived value. International Journal of Information Management, 45, 44–55. https://doi.org/10.1016/j.ijinfomgt.2018.10.024
- Shin, H. H., & Jeong, M. (2022). Does a virtual trip evoke travelers’ nostalgia and derive intentions to visit the destination, a similar destination, and share?: Nostalgia-motivated tourism. Journal of Travel & Tourism Marketing, 39(1), 1–17. https://doi.org/10.1080/10548408.2022.2044972
- Solakis, K., Katsoni, V., Mahmoud, A. B., & Grigoriou, N. (2022). Factors affecting value co-creation through artificial intelligence in tourism: A general literature review. Journal of Tourism Futures, 10(1), 116–130. https://doi.org/10.1108/JTF-06-2021-0157
- Statista. (2023). AI-influenced revenue share of travel companies worldwide 2018-2024. [Online] https://www.statista.com/statistics/1378046/ai-revenue-share-travel-companies-worldwide/
- Stern, B. B., Russell, C. A., & Russell, D. W. (2007). Hidden persuasions in soap operas: Damaged heroines and negative consumer effects. International Journal of Advertising, 26(1), 9–36. https://doi.org/10.1080/02650487.2007.11072994
- Sthapit, E., Garrod, B., Stone, M. J., Björk, P., & Song, H. (2023). Value co-destruction in tourism and hospitality: A systematic literature review and future research agenda. Journal of Travel & Tourism Marketing, 40(5), 363–382. https://doi.org/10.1080/10548408.2023.2255881
- Taber, K. S. (2018). The use of Cronbach’s alpha when developing and reporting research instruments in science education. Research in Science Education, 48(6), 1273–1296. https://doi.org/10.1007/s11165-016-9602-2
- Tavitiyaman, P., Zhang, X., & Tsang, W. Y. (2022). How tourists perceive the usefulness of technology adoption in hotels: Interaction effect of past experience and education level. Journal of China Tourism Research, 18(1), 64–87. https://doi.org/10.1080/19388160.2020.1801546
- Taylor, S., & Todd, P. (1995). Assessing it usage: The role of prior experience. MIS Quarterly, 19(4), 561–570. https://doi.org/10.2307/249633
- Tucker, M. (2020). Parasocial relationships among film consumers: Can film celebrities influence purchase intentions?. Syracuse University.
- Tung, V. W. S., & Tse, S. W. T. (2023). A patterns perspective of customers’ brand experiences with robotics in hospitality. Journal of Travel & Tourism Marketing, 40(1), 75–89. https://doi.org/10.1080/10548408.2023.2215268
- Uzir, M. U. H., Al Halbusi, H., Thurasamy, R., Hock, R. L. T., Aljaberi, M. A., Hasan, N., & Hamid, M. (2021). The effects of service quality, perceived value and trust in home delivery service personnel on customer satisfaction: Evidence from a developing country. Journal of Retailing and Consumer Services, 63, 102721. https://doi.org/10.1016/j.jretconser.2021.102721
- Venkatesh, V., Thong, J. Y., & Xu, X. (2012). Consumer acceptance and use of information technology: Extending the unified theory of acceptance and use of technology. MIS Quarterly, 36(1), 157–178. https://doi.org/10.2307/41410412
- Wang, H.-Y. (2012). Investigating the determinants of travel blogs influencing readers’ intention to travel. The Service Industries Journal, 32(2), 231–255. https://doi.org/10.1080/02642069.2011.559225
- Wang, J., Nguyen, N., Jiang, X., Nguyen, H. V., & Saleem, M. A. (2023). Consumers’ perceived value and use intention of cashless payment in the physical distancing context: Evidence from an Asian emerging market. Asia Pacific Journal of Marketing & Logistics, 35(6), 1513–1531. https://doi.org/10.1108/APJML-05-2022-0408
- Wang, X., Yu, Y., Zhu, Z., & Zheng, J. (2022). Visiting intentions toward theme parks: Do short video content and tourists’ perceived playfulness on TikTok matter? Sustainability, 14(19), 12206. https://doi.org/10.3390/su141912206
- Wu, S., Wong, I. A., & Lin, Z. C. (2021). Understanding the role of atmospheric cues of travel apps: A synthesis between media richness and stimulus–organism–response theory. Journal of Hospitality & Tourism Management, 49, 226–234. https://doi.org/10.1016/j.jhtm.2021.09.014
- Xie, J., Ye, L., Huang, W., & Ye, M. (2021). Understanding FinTech platform adoption: Impacts of perceived value and perceived risk. Journal of Theoretical & Applied Electronic Commerce Research, 16(5), 1893–1911. https://doi.org/10.3390/jtaer16050106
- Yang, Y., & Ha, L. (2021). Why people use TikTok (Douyin) and how their purchase intentions are affected by social media influencers in China: A uses and gratifications and parasocial relationship perspective. Journal of Interactive Advertising, 21(3), 297–305. https://doi.org/10.1080/15252019.2021.1995544
- Yang, Y., Liu, Y., Lv, X., Ai, J., & Li, Y. (2022). Anthropomorphism and customers’ willingness to use artificial intelligence service agents. Journal of Hospitality Marketing & Management, 31(1), 1–23. https://doi.org/10.1080/19368623.2021.1926037
- Yousaf, S. (2022). Food vloggers and parasocial interactions: A comparative study of local and foreign food vlogs using the SOR paradigm. International Journal of Contemporary Hospitality Management, 34(9), 3525–3549. https://doi.org/10.1108/IJCHM-09-2021-1090
- Yuan, S., & Lou, C. (2020). How social media influencers foster relationships with followers: The roles of source credibility and fairness in parasocial relationship and product interest. Journal of Interactive Advertising, 20(2), 133–147. https://doi.org/10.1080/15252019.2020.1769514
- Zhang, J., Chen, Q., Lu, J., Wang, X., Liu, L., & Feng, Y. (2024). Emotional expression by artificial intelligence chatbots to improve customer satisfaction: Underlying mechanism and boundary conditions. Tourism Management, 100, 104835. https://doi.org/10.1016/j.tourman.2023.104835
- Zhu, G., So, K. K. F., & Hudson, S. (2017). Inside the sharing economy: Understanding consumer motivations behind the adoption of mobile applications. International Journal of Contemporary Hospitality Management, 29(9), 2218–2239. https://doi.org/10.1108/IJCHM-09-2016-0496
- Zhu, Y., Zhang, R., Zou, Y., & Jin, D. (2023). Investigating customers’ responses to artificial intelligence chatbots in online travel agencies: The moderating role of product familiarity. Journal of Hospitality & Tourism Technology, 14(2), 208–224. https://doi.org/10.1108/JHTT-02-2022-0041