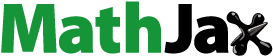
ABSTRACT
Elites’ skepticism of scientific consensus presents a formidable challenge in addressing critical issues like climate change and global pandemics. While extensive research has explored the capacity of events related to these challenges to act as “exogenous shocks,” motivating the general public to reassess their risk perceptions, our understanding of how elites similarly respond to such shocks remains limited. In this article, we investigate whether COVID-19 infections influenced US lawmakers’ support for COVID-19 containment measures, focusing on expressed opposition to COVID-19 policies on social media and in press releases throughout the first two years of the pandemic. Employing a staggered difference-in-differences design and matrix completion methods, our analysis reveals that COVID-19 infections caused a reduction of approximately 30% in legislators’ expressions of opposition to COVID-19 policies on social media. These findings underscore that elites are indeed responsive to policy shocks – even in highly polarized contexts – when they are personally affected by an issue.
Introduction
From natural disasters to pandemics, leaders worldwide confront unprecedented challenges that demand urgent responses. One critical factor influencing the timely and effective resolution of emergent policy issues in advanced democracies is the alignment between public perceptions and elite stances on these matters (Bisgaard & Slothuus, Citation2018; Merkley & Stecula, Citation2021; Pink et al., Citation2021). Indeed, extensive scholarly inquiry underscores the significant role of elite cues in shaping public attitudes toward policy problems (Bisbee & Da in Lee, Citation2022; Kousser & Tranter, Citation2018; Tappin, Citation2022), even in cases where scientific consensus overwhelmingly supports a particular stance. Recognizing the mounting influence of elite discourse in shaping public perceptions, it becomes imperative to deepen our understanding of how elites themselves develop attitudes toward pressing policy challenges. In this context, our research investigates the following question: How do personal experiences with a prominent policy issue influence the attitudes of elites? To answer this question, we focus on the attitudes of US Congress members toward the COVID-19 pandemic and explore how firsthand experiences of COVID-19 infections among members correspond to shifts in their expressed policy positions, as evident in social media engagement and official press releases.
Since the onset of the COVID-19 pandemic, governments worldwide have implemented stringent protective measures, ranging from stay-at-home orders to school closures and travel restrictions. Despite their significant impact on civil liberties and the ensuing criticism, these measures garnered increasing public support as essential strategies to combat the pandemic (Amat et al., Citation2020; Fetzer et al., Citation2020; Unan et al., Citation2023). Governments that enforced such measures experienced a surge in popularity, attributed to rally-around-the-flag effects (Bol et al., Citation2021; De Vries et al., Citation2021). However, amidst the perceived necessity and benefits of these protective actions, anti-science sentiments and skepticism toward state intervention emerged as prominent themes, particularly championed by populist and anti-establishment figures (Kreps & Kriner, Citation2020; Rutjens et al., Citation2021). Notably, political discourse surrounding the pandemic became increasingly polarized, fueled in part by the proliferation of conspiracy theories associated with populist rhetoric (Debus & Tosun, Citation2021; Eberl et al., Citation2021; Rao et al., Citation2021; Stecula & Pickup, Citation2021).
One such country where the debate about the pandemic and its consequences has been extremely polarized is the United States (Engel-Rebitzer et al., Citation2022; Pennycook et al., Citation2020). Since the early stages of the pandemic’s spread in 2020, then-President Donald Trump propagated numerous false or misleading assertions (Paz, Citation2020; Wolfe & Dale, Citation2020). Concurrently, Trump and congressional Republicans sought to downplay the virus’s threat by questioning the accuracy of COVID-19 case counts and dismissing the experiences of healthcare professionals (LeBlanc, Citation2020). However, this stance left little room for acknowledgment of the potential personal susceptibility to infection among the President and many of his supporters.
Previous studies have demonstrated that exposure to the adverse effects of politicized phenomena, such as extreme weather events within the context of climate change, can influence individuals’ perceptions of the severity of such phenomena (Baccini & Leemann, Citation2021), and may even prompt changes in political behavior (Visconti, Citation2022). In this article, we contribute to this body of literature by investigating the impact of COVID-19 infection on legislators’ communication. The analysis starts by collecting the universe of US Congress members’ social media messages on Twitter (now X) and official press releases. After identifying all COVID-related content using keyword searches, we then fine-tuned a pre-trained large language model (LLM) to classify the text according to whether the author expressed opposition or support for a given COVID-19 policy or measure. Leveraging data from GovTrack (Citation2022) on the times at which different legislators were infected with COVID-19 allowed us to adopt a staggered difference-in-differences design to identify the effects of infection on opposition to COVID-19 measures. We then employ matrix completion and interactive fixed effects specifications to show that legislators who were infected with COVID-19 reduced their expressed opposition to COVID-19 policies such as mask mandates, social distancing, and vaccine mandates on social media and in press releases in the weeks following infection. After showing that these results are robust to several alternative explanations, we conclude that infected legislators reduced the number of opposition messages shared on social media by approximately 30%, suggesting that personal experience with the virus can lead to a change in policy attitudes among political elites.
The findings of the article therefore add to our understanding of how elites respond to policy shocks when they are personally affected. Namely, our findings suggest that elites may reconsider their prior policy positions when they are potentially impacted by said positions. Our findings also contribute to the literature on the effects of exogenous events on political behavior (Baccini & Leemann, Citation2021; Hersh, Citation2013; Newman & Hartman, Citation2019; Visconti, Citation2022). Extending the focus to political elites, our findings provide additional evidence that external shocks can influence political communication and behavior. In the next section, we highlight the literature on policy shocks and COVID-19 attitudes. The third section provides the research design, and the fourth section presents the results. We then outline a number of robustness checks that confirm the substantive findings of the primary analysis, before a discussion on the wider implications of our analysis.
COVID-19 Opposition and Elite Cues
The sizable literature on socio-psychological correlates of COVID-19 perceptions demonstrates that various factors are associated with attitudes toward the pandemic and protection measures, including psychological traits, trust, risk attitudes and reliance on intuition (Esaiasson et al., Citation2021; Fetzer et al., Citation2020; Steffen & Cheng, Citation2021; Xu & Cheng, Citation2021). Extant research shows that individuals tend to underestimate health risks associated with COVID-19, are influenced considerably by framing effects in relation to the pandemic, and display what came to be known as “acceptance bias’’ when encountering misinformation related to the pandemic (Newman et al., Citation2022; Olmastroni et al., Citation2021; Pearce & Cooper, Citation2021; Rachev et al., Citation2021; Steffen & Cheng, Citation2021). Individuals also greatly underestimated their likelihood of contracting and carrying the virus, showing “unrealistic optimism” for themselves and close others (Salgado & Berntsen, Citation2021). Once infected, however, they may quickly update their perceptions; some – especially those in high-risk groups – may even go so far as to overestimate the degree to which they share similar levels of risk with others, as predicted by false consensus bias (Ross et al., Citation1977). Taken together, such biases in the human cognitive architecture help explain why lockdowns, face masks and other protection measures became polarizing issues in various political contexts.
Although politicians might be expected to be more welcoming of stringent protection measures, as certain traits such as education and proximity to expert knowledge mitigate the acceptance and persistence of misinformation in relation to COVID-19, a small but growing literature on misperceptions among political elites points to similar cognitive biases that are prevalent also among the public (Broockman & Skovron, Citation2018; Hertel-Fernandez et al., Citation2019; Sheffer et al., Citation2018). Kertzer (Citation2022), for instance, directly challenges the notion of elite exceptionalism – the idea that political elites are remarkably different than the public when it comes to decision making – by convincingly showing that elites and masses respond to treatments in experiments in a strikingly similar way.
Not only can political elites have distorted perceptions of constituency opinion (Broockman & Skovron, Citation2018; Pereira, Citation2021), but they may also show resistance to becoming more knowledgeable about constituency matters, and they tend to downplay or discount the opinions with which they disagree, even despite electoral pressures (Broockman & Skovron, Citation2018; Kalla & Porter, Citation2021; Pereira & Öhberg, Citation2020). Studies examining elites’ dispositions and leadership skills from an evolutionary perspective argue that elites are particularly prone to exhibiting displays of overconfidence (see Van Vugt, Citation2006; Sheffer & Loewen, Citation2019 for an overview). These studies lend strong support for theories of decision-making that assume universalistic cognitive biases that apply to both elites and masses (e.g., Jones, Citation2001; Simon, Citation1957; Tversky & Kahneman, Citation1974).
While there is little to no empirical research as to whether and to what extent politicians differ from individuals in the broader population in perceptions of the COVID-19 pandemic, the literature cited above provides some initial clues. Studies based on different political contexts, including North America, Britain and France, show that individuals greatly underestimated the risk of COVID-19 infection (Asimakopoulou et al., Citation2020; Attema et al., Citation2021; Wise et al., Citation2020). Moreover, it is hard to deny the realities of growing ideological polarization in contemporary American politics and its role in structuring beliefs about science. Recent research shows that political ideology is strongly correlated with science skepticism in general (Rutjens et al., Citation2021) and COVID-19 attitudes in particular (Debus & Tosun, Citation2021; Evans & Hargittai, Citation2020). World leaders such as Jair Bolsonaro, Rodrigo Duterte, and Donald Trump, previous heads of state in Brazil, the Philippines and the US, respectively, have publicly downplayed the health-related risks associated with the pandemic on numerous occasions (Lasco, Citation2020). Indeed, Kavakli's (Citation2020) cross-national analysis of government responses to the pandemic confirms that COVID-19 measures adopted by governments are strongly correlated with government ideology, with populist governments being sluggish in adopting drastic measures. In line with this view, Bayerlein et al. (Citation2021) theorize that both citizens and political elites in a populist and highly polarized political environment are more likely to underestimate the consequences of the pandemic.
Perhaps intuitively, skepticism and outright opposition may be mitigated by exogenous events that require an individual to grapple with an issue they are skeptical of or an event that causes personal ramifications for the individual. This proposition has been demonstrated in studies on the effects of extreme weather events on attitudes about climate change. For example, Baccini and Leemann (Citation2021) find that Swiss voters increase support for pro-climate policies by up to 20% following a personal experience with a flood. Also examining the influence of floods, Visconti (Citation2022) explores the extent to which Chilean voters shift their voting preferences in the direction of pro-climate politicians. The author exploits variation in exposure to flood severity to find that individuals who experience more severe flooding damage increasingly prefer politicians who similarly view climate change as a greater threat.
A personal experience with an event that causes harm or damage to an individual can have profound effects. In the context of COVID-19, research has shown that unlike social pressure or the advice of medical professionals, personal perceptions of risk shape the behavior of individuals (Sakakibara & Ozono, Citation2020; Wise et al., Citation2020). Wise et al. (Citation2020) document that the perceived probability of personally being infected is a strong predictor of compliance with protection measures. Latkin et al. (Citation2021) report a similar finding: people who endorse COVID-19 skepticism statements are less likely to believe that they and close others would die from COVID-19. One implication of this is that elected officials – just like individuals in the public with whom they share similar cognitive characteristics (Arceneaux et al., Citation2018; Sheffer et al., Citation2018) – likely underestimate the risk of COVID-19 infection and express opposition to stringent protection measures, especially given how polarized the American public is regarding the issue of pandemic policies (Gadarian et al., Citation2021).
Consequently, when there are low perceptions of risk from being infected with COVID-19, it is easier to downplay the virus and ignore its potential effects. However, once that risk is realized – either in the case of experiencing an extreme weather event or with being infected by COVID-19 – individuals often reassess the threat level of the risk. We apply this perspective to outline our theoretical expectations for the effects of COVID-19 infection on legislators’ revealed preferences regarding opposition to COVID-19 policies. We hypothesize that there are three co-constitutive factors at play that result from COVID-19 infection. First, infection may reduce opposition to COVID-19 policies by causing legislators to update their perceptions of the level of risk involved. Namely, legislators who downplayed the risks of the virus may do so because their reasoning is shaped by their partisan affiliation (Taber & Lodge, Citation2006), and they may not expect to face tangible personal repercussions from their policy positions on COVID-19. Once infected, legislators may find it more challenging to maintain the partisan illusion that COVID-19 does not pose a substantive threat to their own and others’ well-being, and therefore may update their risk perceptions.
Second, legislators may reduce their expression of opposition to COVID-19 measures due to personal strategic considerations. Policy reversals are costly (i.e. “flip-flopping”), so legislators face strong electoral incentives to maintain their stance as part of an attempt to preserve an image of competence (Andreottola, Citation2021). This effect was likely further magnified because journalists were quick to point out the alleged hypocrisy of representatives whose behavior differed from their partisan claims about COVID-19 measures (LeBlanc, Citation2020). Yet, not all policy reversals are costly. When representatives change positions to move in the direction of public opinion, the benefits of the reversal can outweigh the costs associated (Doherty et al., Citation2016). Consequently, when representatives experience a shock in the form of COVID-19 infection, they may take the opportunity to strategically shift to be more in line with public opinion on the matter, as well as to avoid further scrutiny from both the media and voters. With that said, remaining at odds with cues from the party leadership and in-partisan voters for an extended period of time might harm the electoral prospects of the legislator. This implies that legislators who get infected by COVID-19 may return to their initial stance on pandemic measures after temporarily reducing their opposition.
Additionally, it is certainly possible that some legislators, despite being fully aware of the risks associated with COVID-19, may have strategically chosen to downplay the risks for perceived advantages, namely, to align with the vote base in the regional constituency or with the party leadership. Consequently, the shift in their stance following infection may be less attributable to an altered risk assessment or partisan-motivated reasoning and more indicative of a necessity to mitigate the perceived implications of having contracted the virus after downplaying its severity. Upon being infected with the virus, legislators may tone down their expressions of skepticism about the virus and protection measures with the aim of reducing negative attention.
Another possibility is that legislators who are infected with COVID-19 may be asymptomatic and therefore may not experience the same effects as those who experience more severe symptoms. Nonetheless, we still expect these legislators to update their risk perceptions for two reasons. First, even if they are asymptomatic, legislators are likely to be excluded from representative duties and therefore may still experience the same social pressure regardless of severity. Second, asymptomatic infection still carries with it the possibility of infecting the legislator’s colleagues, family members and constituents. Therefore, asymptomatic individuals, or individuals that have only mild cases of COVID-19, may still update their prior beliefs about the chances of future infection for themselves and those around them.
Research Design
Since the beginning of the pandemic, COVID-19 infection has been common among elected officials from around the world, including high profile politicians such as Boris Johnson, Joe Biden, Donald Trump, Emmanuel Macron, and Jair Bolsonaro, just to name a few. US legislators were no exception. Within three months of the first confirmed case in the US on January 21, 2020, 53 members of the US Congress had been infected with the virus (GovTrack, Citation2022). By the end of 2020, that number would rise to 118 congress members. In total, from the time between January 2020 and May 2022, 230 legislators were infected 258 times (several members were infected multiple times). We focus on this time period in our analysis.
We rely on data from GovTrack (Citation2022) for the dates at which legislators were infected with COVID-19. The GovTrack team compiled data on legislators’ COVID-19 infections using a number of social and traditional media sources, and has produced high-quality legislative data in other domains as well. presents the dates at which legislators were infected with COVID-19. In the figure, new cases are presented in orange (first y-axis) and cumulative cases are presented in blue (second y-axis).
Figure 1. COVID-19 Infections among US legislators.
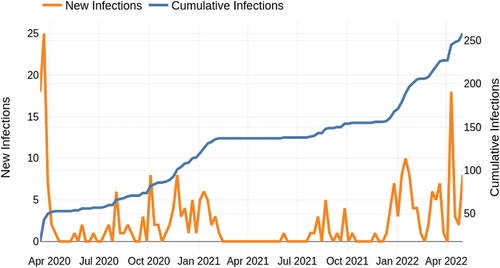
Although GovTrack made a concerted effort to identify all legislators who were infected with COVID-19 and provides sources for each observation, it is possible that some legislators were infected and did not report their infection or did not show symptoms and were unaware. In the latter scenario, we do not expect an effect because COVID-19 itself would not have led to a change in the individual’s environment. However, the former scenario presents an opportunity for bias if there are systematic differences between legislators who hid infection and legislators who did not. There are a few reasons why we do not expect systematic biases in reporting about COVID-19 infection among US legislators. First, legislators were required to be tested during several periods (Bresnahan & Caygle, Citation2020) in order to attend events such as the State of the Union Address (NPR, Citation2022). Second, hiding COVID-19 infection is likely to be a challenge for US legislators given a combination of age and public status. Individuals who are over 50 are at a significantly greater risk of hospitalization and mortality from COVID-19 (Biswas et al., Citation2021), and previous research suggests that older individuals are aware of such risks (Bruine de Bruin & Isaacowitz, Citation2021). Given that the average age of a US legislator is close to 60 (Cillizza, Citation2021), it would likely be challenging to avoid requiring medical attention – along with the publicity that entails – in the case that legislators were hiding COVID-19 infection. While we proceed in our primary analysis under the assumption that the GovTrack data on legislators’ COVID-19 infections are accurate, we return to examine how systematic differences in reporting may affect our results in the Alternative Explanations section below.
Measuring Opposition to COVID-19 Policies
We refer to legislators’ communication on Twitter (now called X) to understand legislators’ opposition to policies aimed at reducing the spread of COVID-19. Communication on social media, and Twitter in particular, can be a good measure of dynamic political attitudes and preferences of elites given the frequency with which they share their views online. Twitter also likely provides legislators more leeway to dissent from their party’s position without facing the kinds of repercussions associated with breaking with the party in the legislature. Moreover, legislators regularly use Twitter to discuss political issues and communicate with constituents. For instance, Russell (Citation2021) argues that legislators value Twitter because it offers “a birds-eye view” of national public attention, offering US representatives the opportunity to use Twitter to build policy reputations with constituents. Additionally, Twitter messages sent from politicians are a strong source for understanding elites’ political interests and issue priorities due to the level of conciseness required in a short message. Elites are also over-represented on Twitter – nearly every US legislator has an active Twitter account and engages on the platform.
To understand elites’ individual preferences on COVID-19 containment policies, we identified related messages sent by elected representatives in the US House of Representatives and the Senate. We first collected every message sent from US MCs during the time period from January 2020–May 2022. The messages were then filtered using keyword searches pertaining to COVID-19. We used the keywords “covid,” ”corona“, “sars-cov-2,” ”vaccine“, “virus,” ”mask“, “lockdown,” and ”social distanc.”Footnote1 The aim of the keyword search was simply to identify messages that explicitly concerned measures aimed at COVID-19 related policies. After the keyword search, there were 84,206 messages that were about COVID-19 and/or a policy aimed at reducing its impact.
The next step was to classify tweets according to whether they expressed opposition to COVID-19 related policies. For this, we fine-tuned a large language model (LLM) based on the BERT architecture (Devlin et al., Citation2018). The BERT transformer architecture allows for training and fine-tuning language models and achieves state-of-the-art performance on a number of different tasks. For our task, we used the BERTtweet model (Nguyen et al., Citation2020), which was pre-trained on a corpus of 850 million English tweets, and then fine-tuned on an additional 23 million tweets related to COVID-19. Using this model as our base model, we then further fine-tuned the model on an additional 10,000 tweet sample from our dataset of COVID-19-related tweets. We hand-coded this sample of 10,000 tweets according to whether each message expressed opposition to a COVID-19 policy or procedure. We then used the fine-tuned model for inference on the remaining tweets. This method was impressively effective, and our model achieved an F1 score of 0.95 on a held-out test set. Additional evaluation criteria and validation for the model are provided in Supplementary Materials A.
One of the most important steps in creating a supervised language model for classification is ensuring that the data used to train the model are accurate. The tweets varied widely and ranged from messages that recommended outright refusal to wear masks, to false claims about the intention of government policies aimed at curbing COVID-19. The vast majority of the tweets were easy to label; however, a few were more challenging. For instance, a clear example of a tweet from the dataset that did not express opposition to COVID-19 policies includes the following, sent from Rep. Rob Wittman on May 28, 2020:
I will continue to lead our commonwealth as we navigate the federal, state, and local response to the health and economic impacts to the coronavirus. We must continue to practice social distancing to keep our nation and communities safe.
This instance clearly shows no indication of opposition to COVID-19 restrictions, and in fact encourages the public to take recommended precautions. The majority of messages about COVID-19 fell into this category. An example that we labeled as expressing opposition to COVID-19 measures includes the following, sent from Rep. Bob Good on July 28, 2021:
The House has reinstated its draconian mask mandate once again. My amendment would end taxpayer funding for mask mandates in the Capitol complex. Follow the science – no more mask mandates!
From the message above, it is clear that Rep. Good opposes the mask mandates. This message was coded as expressing opposition.
We also provide an example of the type of messages that our model did not correctly classify. Although the model achieves a high level of accuracy, there are still some cases in which errors are made. To illustrate such an error, the following message from Thomas Massie in August 2021 is an example of a tweet that our model incorrectly predicted as not expressing opposition:
On the subject of mandatory vaccines imposed by private employers… if this is a matter of private contracts, and not a matter of criminal law, what’s the penalty for providing false vaccine information to an employer? termination? the same as refusing to take the vaccine?
Although Rep. Massie does not explicitly challenge vaccine policies for private employers, he implies that individuals who find themselves in a situation in which they do not want to take the vaccine face the same risk (termination) regardless of whether they lie about taking the vaccine or outright refuse to take it. Implicit opposition to COVID-19 measures is harder to capture using text alone here, which is likely why the model misclassified the tweet and did not identify it as expressing opposition.
To add confidence in our classifications and to minimize the possibility that downstream statistical estimates are based on classification errors, we re-classified all the messages using GPT-3.5 – the model underlying OpenAI’s ChatGPT (OpenAI, Citation2022). Previous studies have found that GPT-3.5 out-performs human annotators on a number of different annotation tasks (Gilardi et al., Citation2023), which makes the model especially useful for our objectives. Using the OpenAI API, we used the model as a zero-shot classifier to classify each of the tweets in our dataset on the same opposition – support dimension as the BERTtweet model. We then compared the predictions of our fine-tuned BERTtweet model with the predictions of GPT-3.5. In all cases of discordance between GPT-3.5 and our model’s predictions, we manually labeled the message. In total, 94.1% of the messages were similarly labeled by GPT-3.5 and our model.Footnote2
After each of the messages were classified according to whether they expressed opposition to COVID-related policies, we created a new dataset with bi-weekly measurements of tweets sent in opposition to COVID-19 restrictions, as well as bi-weekly measurements of the total number of tweets sent about COVID-19 in general. Measurements were made at the individual legislator level for every elected legislator who sent a tweet about a COVID-19 measure at any point between January 2020 and May 2022.
In order to provide a sense of our measure of opposition over time, presents dynamic opposition to COVID-19 policies by political party. The figure indicates that opposition to COVID-19 policies has been relative stable for Democrats, but has increased dramatically for Republicans, especially around the time Joe Biden took office in January 2021.
highlights the fact that opposition to COVID-19 policies is highly partisan. This is not surprising given the highly polarized nature of the COVID-19 pandemic in the US (Gadarian et al., Citation2021). We return to further consider partisan asymmetries in opposition to COVID-19 policies in robustness checks in the Alternative Explanations section.
Identification Strategy
We adopt a staggered difference-in-differences design to recover an estimate for the effects of COVID-19 infection on legislators’ expressed opposition to COVID-19 policies. We rely on variation in the timing of infection to identify the effects of COVID-19 infection. Specifically, we exploit sharp discontinuity in the four weeks right before and four weeks directly after COVID-19 infections. Our design therefore takes into consideration the possibility that legislators may have been infected with COVID-19 multiple times, while also still taking into consideration the dynamic nature of COVID-19 symptoms, which tend to have a duration of approximately 3–4 weeks (Mizrahi et al., Citation2020).
The primary identifying assumption of our research design that is required in order to recover a causal estimate is the parallel trends assumption. In order for there to be parallel trends in the outcome variable, the specific times (e.g. dates) at which legislators are infected with COVID-19 must be as-good-as random. In other words, legislators cannot select the dates on which they become infected with COVID-19. It is worth noting that our assumption is not that COVID-19 infections are exogenous, as infection is almost certainly correlated with attitudes about COVID-19. Moreover, we do not assume that the times of infection are exogenous in the wider population, which is also not likely to hold. Rather, we assume that conditional on being infected with COVID-19, expressed opposition to COVID-19 policies in the four weeks before infection should not systematically differ from opposition in the four weeks after infection, in the absence of infection. This assumption – also commonly referred to as the parallel trends assumption – is standard in difference-in-differences design (Card & Krueger, Citation1993; Cunningham, Citation2021), and we provide several pieces of evidence to support this crucial assumption.
First, we perform pre-treatment equivalence tests in order to test for trends in the pre-treatment periods (Liu et al., Citation2022). These tests examine the degree to which trends in the outcome variable (opposition) differs between the infected and not-yet-infected legislators in the pre-treatment period (i.e. before infection). We find no support for the hypothesis that pre-trend differences between the two groups exist. These results are presented in Supplementary Materials C. Second, we present coefficient estimates for the time period before COVID-19 infection, which allows for visual inspection of the pre-treatment trends in the outcome variable. In the case that the times at which infections occur was correlated with an unobserved variable, we would expect that the coefficient estimates could be statistically differentiable from zero in the time before the treatment. We find no evidence that the pre-treatment coefficients are statistically distinguishable from zero in the pre-infection period and present those estimates below in in the Results section.
Figure 3. Effects of COVID-19 Infection on opposition to COVID-19 policies.
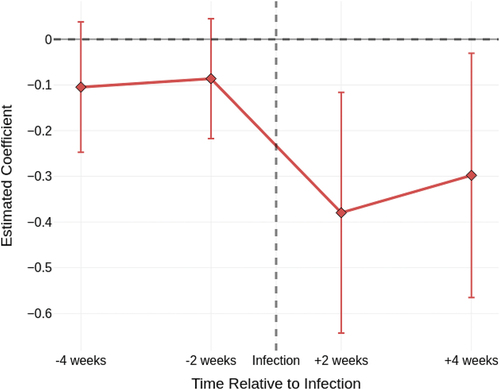
Estimation
Our estimation strategy requires accommodating several features of the data. Namely, our data includes staggered treatment timing in which legislators can be infected with COVID-19 at different time periods. Additionally, legislators can be re-infected multiple times, and we expect that the effects of infection should be more apparent in the weeks following infection compared to much later. Traditional approaches to estimation in similar settings have relied on two-way fixed effects specifications in which time and unit effects are fixed. However, the recent econometrics literature has demonstrated that two-way fixed effects regressions can produce biased estimates in staggered settings (Baker et al., Citation2022; Brantly & Sant’anna, Citation2021; Sun & Abraham, Citation2021). We therefore rely on matrix completion methods to estimate the effects of infection (Athey et al., Citation2021).
Matrix completion methods are a class of causal estimators for panel data settings that estimate counterfactual outcomes for treated units by imputing missing values in the outcome variable. In our case, matrix completion methods estimate the counterfactual outcomes for each legislator in the weeks following infection (while under “treatment”). The difference between the observed and imputed values are then used to calculate an individualistic treatment effect. Each of these individual effects are then aggregated to produce estimates for the effect of the intervention – in our case, the average treatment effect on the treated for COVID-19 infection. Importantly, matrix completion methods provide more reliable causal estimates than two-way fixed effects models in the case of heterogeneous treatment effects or when there are potential time-varying confounders that are unobserved (Liu et al., Citation2022).Footnote3
Matrix completion is especially relevant for our research design because it accommodates staggered treatments, as well as multiple treatments that switch on and off for the same unit. Moreover, the method allows for the inclusion of unit and time fixed effects, which allow us to control for time-invariant characteristics of legislators such as partisan and gender differences.
In order to recover causal estimates from the matrix completion estimator and other counterfactual estimators like the synthetic control method (Abadie et al., Citation2010), treated units must not anticipate treatment. In other words, infected legislators must not anticipate infection. This is indeed a strong assumption to make in our observed data given that legislators may not report COVID-19 infection immediately after becoming aware. Should this be the case, it would work against our hypothesis that COVID-19 infection reduces opposition by biasing our estimates toward zero. It is therefore possible that the effects of infection are greater than what we report.
In all estimations, we include unit and time fixed effects, and we report estimates with and without COVID-19 cases as a control variable (measured in the US state associated with the legislator’s constituency). In all estimates, the treatment indicator variable is a binary variable that takes the value of 1 in the four weeks after each COVID-19 infection and is 0 in all other cases. Our estimand is therefore the average treatment effect on the treated (ATT) of COVID-19 infection on legislators’ opposition to COVID-19 policies over the four weeks following infection. All estimations are performed using the fect library in R (Liu et al., Citation2022).
Results
Our theoretical expectations outlined above suggest that COVID-19 infection may lead legislators to reduce their expressed opposition to COVID-19 mitigation measures following personal experience with symptoms of the virus. presents the ATT estimates for the four weeks before and after infection. In the figure, the vertical line represents the date of infection. The first two coefficient estimates from left to right are the ATT estimates for four and two weeks before infection, respectively. These estimates are presented with 95% confidence intervals, and suggest that the level of expressed opposition in the four weeks before infection is relatively stable and is not statistically distinguishable from zero, which provides support for the parallel trends assumption.
The two coefficient estimates after infection are the ATT estimates for two and four weeks after infection, respectively, for the entire population of infections among lawmakers. In line with our theoretical expectations, these estimates are statistically distinguishable from zero, and suggest that expressed opposition to COVID-19 policies decreased by approximately 0.3–0.4 messages over the following four weeks. In other words, legislators who were infected with COVID-19 sent approximately 0.3–0.4 fewer messages expressing opposition to COVID-19 policies in the four weeks after infection than they did in the four weeks before infection.
We additionally consider the cumulative effects over the duration of infection. In , we present the cumulative effects of all infections on opposition to COVID-19 policies using several different specifications. Model 1 uses an inverse hyperbolic sine transformation of the outcome variable, which is a common transformation used in count models. Model 2 uses the logarithmic transformation of the outcome variable, which is also a common transformation used in count models. Model 3 includes the number of COVID-19 cases per day in each legislator’s constituency state. Model 4 includes the number of new cases and new deaths in each legislator’s constituency state.
Table 1. Cumulative effects (ATT) of COVID-19 Infection on Opposition to COVID-19 Policies.
Across each of the specifications, the results are consistent with our theoretical expectations. In all models, the coefficient estimate for the ATT is negative and significant at the 95% confidence level. The coefficient estimates for the ATT range from −0.327 to −0.329, which suggests that legislators who were infected with COVID-19 sent approximately 32% fewer messages expressing opposition to COVID-19 policies in the four weeks after infection than they did in the four weeks before infection. The results also appear to be robust to the inclusion of COVID-19 cases and deaths in the legislator’s constituency state.
How Long Do the Effects Last?
The results suggest that legislators respond to COVID-19 infection by reducing opposition to COVID-19 policies in the four weeks after infection. However, it is unclear how long the effects of infection on opposition last beyond the four-week period. We therefore consider the duration of the effects by estimating the effects of infection after the four-week duration following infection. Although we find limited evidence that the effect lasts after “exiting” the treatment (e.g. after recovering from COVID-19 following a 4-week infection), the figure provides suggestive evidence that lawmakers do not return right back to expressing the same level of opposition. Rather, the effect is still somewhat evident approximately two months after infection.
Nonetheless, the exit effects presented in suggest that infection reduces opposition for only a short period of time, with opposition returning fully to mean levels after approximately 12 weeks. This result indeed provides further support for the hypothesized effect of infection on opposition, but it also highlights the limitations of infection and the ability to create fundamental changes in lawmakers’ policy positions toward COVID-19.
Figure 4. Carry-over effects of COVID-19 infection on opposition to COVID-19 policies.
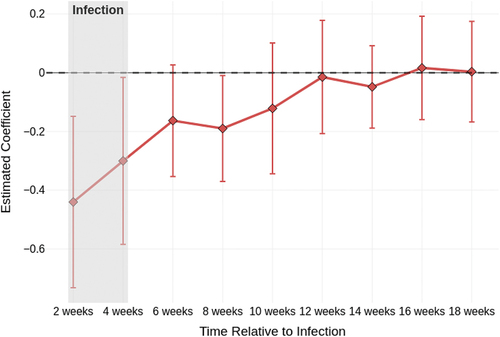
Robustness Checks
Our findings throughout the analysis thus far suggest that COVID-19 infection reduces legislators’ expressed opposition to COVID-19 policies. However, there are several alternative scenarios that may potentially explain the results we observe. In this section, we consider several alternative explanations for the results to test the robustness of our findings.
Party Differences
One potential scenario that may threaten our interpretation of the findings is that there are systematic differences in how legislators from different political parties reported COVID-19 infection. For instance, it may be possible that Republican lawmakers were more likely to hide their infections than Democratic lawmakers.
We therefore focus only on legislators who reported COVID-19 infection during the time period of analysis. The logic behind this check is that some legislators may hide their infection from the public. For instance, Republican lawmakers may feel pressured not to report that they were infected with COVID-19 if the party position is that COVID-19 is not a serious threat. Therefore, we subset the data to include only legislators who reported infection. We then used the subset to re-estimate each of the four primary specifications. The results, presented in Supplementary Materials E, confirm the substantive conclusions of the primary analysis and indicate nearly identical effect sizes when considering only legislators who reported infection as the control group.
We additionally estimated the conditional average treatment effects for Democratic and Republican legislators. The results, presented in Supplementary Materials D, suggest that the treatment effects are indeed greater for Republican lawmakers. These differences are statistically significant at conventional levels, suggesting that Republican legislators reduced their opposition to COVID-19 policies more so than Democratic legislators following infection. This is likely a result of the fact that Republicans were much more likely to express opposition to COVID-19 policies in the first place, which was illustrated in .
Total Tweets
Another potential scenario that may threaten our interpretation of the findings is that legislators who were infected with COVID-19 may send fewer tweets in a more general sense when they are infected with COVID-19. For example, legislators may spend less time on Twitter while recovering from the virus, and therefore the reduction in opposition to COVID-19 policies that we observe may simply be a function of fewer total tweets sent. To test this possibility, we re-estimate each of the main specifications using the total number of tweets sent by each legislator as the dependent variable. The results, presented in Supplementary Materials F, actually suggest that legislators tend to increase their activity on Twitter in the four weeks after infection. Given these results, we conclude that it is unlikely that the reduction in opposition to COVID-19 policies that we observe is simply a function of fewer tweets sent.
Legislative Press Releases
As a fourth robustness check, we consider the possibility that our reliance on data from Twitter does not translate to other, more official forms of communication. For this check, we collected additional data on legislators’ communication by referring to press releases during the same time period analyzed in the primary analysis. Specifically, we collected every press release sent by a US legislator via the ProPublica Recent Congressional Statements API (ProPublica, Citation2022). The API provides access to press releases issued directly by members of Congress, and includes the date of the press release, the title, and the full text of the press release. After collecting nearly 200,000 press releases in total, we filtered the press releases according to the same COVID-19 related keywords employed in the main analysis. After narrowing to 17,255 COVID-19 related press releases, we effectively replicated the primary analysis using the press releases to measure opposition. The only exception to this procedure was that we used the GPT-3.5 API (OpenAI, Citation2022) to classify all the press releases rather than training a new model ourselves to classify the messages according to whether they expressed opposition to COVID-19 measures.
The results of the analysis using the press releases and the same estimation strategy confirm the substantive conclusions drawn in the primary analysis with messages from Twitter. In press releases, legislators who were infected with COVID-19 reduce their opposition to COVID-19 policies by approximately 2% in the four weeks after infection compared to the four weeks before infection. The estimates are statistically significant across each of the specifications used in the primary analysis. We also demonstrate parallel trends in the pre-treatment period before infection in Supplementary Materials G.
Compared to the primary analysis using Twitter data, the effects sizes are much smaller in magnitude. This likely reflects the differing costs associated with distributing a press release compared to sending a tweet, as the amount of time and effort required to distribute a press release is greater in relation to sending a message on Twitter. In Supplementary Materials G, we present the full results and descriptive statistics from the press release analysis.
Alternative Estimators
We rely on matrix completion methods for estimation throughout the article. Despite recent studies that show that matrix completion methods outperform two-way fixed effects models and other methods that rely on assumptions of unconfoundedness (Athey et al., Citation2021), there are certain scenarios in which factor augmented models such as interactive fixed effects specifications may produce more reliable estimates (Liu et al., Citation2022). We therefore replicate the findings of the primary analysis and the robustness checks using interactive fixed effects models (e.g. factor-augmented models) (Bai, Citation2009; Gobillon & Magnac, Citation2016). The results, which are presented in Supplementary Materials H, confirm the substantive conclusions drawn in the primary analysis and the robustness checks.
Taken in total, the results from the robustness checks provide greater assurance that the results from the primary analysis are not driven by systematic party differences in reporting, in how infections may affect social media behavior (e.g. that the reduction in opposition we observe is not driven by a reduction in social media messages more generally), the medium of legislators’ communication, or the choice of estimation strategy. In each of the separate analyses, the results are consistent with the primary analysis, and suggest that legislators who were infected with COVID-19 reduce their expressed opposition to COVID-19 policies following infection.
Discussion
As the world must increasingly grapple with the effects of crises such as climate change and pandemics, the electorate, as well as our political leaders, must update their worldviews according to new information. As with climate change denial, skepticism and outright refusal of compliance with public health guidelines could be witnessed at nearly every position in government throughout the pandemic. Despite social and political pressure to take the virus more seriously, American legislators broadcast thousands of messages that expressed opposition to policies intended to slow the spread of COVID-19.
One explanation for why legislators downplayed the potential threat posed by the virus, we argued, is partisan motivated reasoning. Motivated reasoning plagues elites in the same way it might affect any other individual. Elites, like all of us, have a tendency to underestimate information perceived to be politically or personally damaging (Broockman & Skovron, Citation2018). Moreover, many of the most divisive issues become polarized in a similar fashion. Elites and more politically sophisticated individuals – both of whom are more polarized and more likely to reach conclusions that align with their partisan identities than the general electorate – articulate distinct conclusions about the same issue (Bayes & Druckman, Citation2021; Drummond & Fischhoff, Citation2017). As directions are set and in an effort to ensure that they are on the correct side of the argument, information that supports a certain viewpoint is amplified while conflicting information that challenges said viewpoint is simultaneously and systematically downplayed (Bayes & Druckman, Citation2021; Broockman & Skovron, Citation2018; Hart & Nisbet, Citation2012).
The most recent and salient iteration of this top-down partisan process played out on the issue of COVID-19. From the time of the initial spread of COVID-19 in early 2020, then-President Donald Trump unleashed a number of false or misleading claims about the virus (Paz, Citation2020; Wolfe & Dale, Citation2020). At various points, Trump and fellow Republicans blamed Mexico, China, Democrats, immigrants, and the “mainstream media” for the spread of COVID-19 (LeBlanc, Citation2020; Paz, Citation2020; Wolfe & Dale, Citation2020). When the buck could no longer be passed, Republicans, led by Trump, went to great lengths to downplay the threat of COVID-19, often sharing misinformation and conflicting public health advice from government officials (Cook & Choi, Citation2020; Gollust et al., Citation2020). Trump’s rhetoric and position on COVID-19 set the agenda for the broader Republican party, with many members emphasizing a similar sentiment (Cormack & Meidlinger, Citation2021). With this in mind, Republicans in Congress had strong incentives to continue on the same path of emphasizing opposition to COVID-19 measures and downplaying the risks of the virus.
Moreover, at the time of the initial COVID-19 outbreak, Donald Trump and fellow Republicans were gearing up for the 2020 election with one of the lowest unemployment rates in nearly a century and a stock market that was at all-time highs (MarketWatch, Citation2022; Federal Reserve Bank, Citation2022). Republicans likely imagined translating these two predictors of incumbent success, as well as other positive elements of the economy at the time, into a 2020 electoral victory. Consequently, when faced with the dire impact that the COVID-19 pandemic would bring to the economy, Republicans were deeply opposed to measures to contain its spread because they saw such measures as a threat to their electoral futures. Therefore, the chosen pathway forward was to deny the potential risk posed by the virus to maintain their favorable electoral position. Yet, denying the severity of a potential risk becomes harder once that risk is realized, which occurred when legislators were infected with COVID-19.
In conclusion, this article makes two key contributions to the literature on elites’ responsiveness to policy shocks. First, our findings suggest that exogenous shocks that are personally realized can incentivize reassessing a prior position. Moreover, this re-assessment process not only exists in an environment as polarized as the US, but for an issue as salient as COVID-19 and for individuals who are likely to be the most polarized – political elites in Congress. This finding is both encouraging and discouraging at the same time. While it is illuminating that elites change their views on salient matters when they are personally afflicted, it is discouraging that infection with a deadly virus in this instance may be the point at which positions are reassessed.
Second, the article speaks to the research on the role of exogenous events and their influence on political communication and behavior. Past studies have found conflicting results on the effects of extreme weather events and beliefs about climate change. Yet, there is evidence that climate events may change the political behavior of individuals (Baccini & Leemann, Citation2021). Our study contributes to this research by showing that a behavioral change may occur even before individuals are willing to share that they have changed their attitudes. While our data do not allow us to explicitly test whether infection changed legislators’ personal beliefs about the virus, there is an argument to be made that behavioral change in the form of a reduction in opposition is more important than changes in preferences.
While the evidence we present robustly demonstrates that infection reduced expressed opposition, our analysis also illustrates that the effects are relatively short lived, and legislators infected by the virus tend to return to their initial stances after about 2–3 months. We argue that this is mainly due to in-partisan voters’ skepticism about the pandemic measures. However, it is important to note that the persistence of the effect of personal contact with an external policy shock arguably depends heavily on the nature of the problem. For most people, COVID-19 infection does not lead to known long-term health consequences, and this might foster encouragement for skeptical views. However, personal contact with more persistent or more costly exogenous shocks, such as natural disasters, may induce a more enduring behavioral change among voters and political elites alike.
As with any study, there are several limitations to the findings of this research. First, the extent to which social media messages reflect the true preferences of elites is unclear. Although we additionally included an analysis of Congress members’ press releases, it is still possible that elites may say one thing while believing another. With that said, such words have real world effects, so the extent to which they are genuine may be less important than the fact that they are said. Future research should continue to explore the extent to which elite communication – and communication on social media in particular – reflects the true preferences of elites.
Second, while we consider several potential mechanisms for the relationship between COVID-19 infection and reduced opposition to protection measures, we are unable to disentangle how these mechanisms collectively explain shifts in attitudes toward protection measures. Recognizing that personal risk assessment and motivated reasoning might influence opposition to COVID-19 measures differently for different legislators, we argue that distorted risk assessments likely interact with motivated reasoning and strategic position-taking to shape legislators’ public stance on and attitudes toward external policy shocks. More research on cognitive distortions in elites should be welcomed. It is commonly accepted that elites are better informed about the nature of policy problems and hold a higher status in society than the wider public. These factors appear to paradoxically create a lower incentive for elites to update their views about the world (Tetlock, Citation2017), and past studies indicate that elites’ expertise acts as a constraint on their ability to adequately respond to changing conditions (Pereira & Öhberg, Citation2020). With this in mind, greater focus on the ways in which cognitive biases and motivated reasoning may be remedied in elites is a challenging yet important path for future research.
Supplemental Material
Download PDF (396.1 KB)Acknowledgments
Previous versions of this paper were presented at the 2022 ECPR General Conference and the 2023 Midwest Political Science Association Conference. This paper has benefited from helpful comments and feedback from Michael Patrick Olson and Sara Hobolt. The authors also thank three anonymous reviewers and the editorial team of Political Communication for constructive feedback.
Disclosure Statement
No potential conflict of interest was reported by the author(s).
Data Availability Statement
All materials required for replication of this analysis are available on https://github.com/z-dickson/covid_legislators_replication.
Supplemental data
Supplemental data for this article can be accessed on the publisher’s website at https://doi.org/10.1080/10584609.2024.2364072
Additional information
Notes on contributors
Zachary P. Dickson
Zachary P. Dickson is a Research Fellow in Quantitative Methodology at the London School of Economics and an affiliate with the LSE Data Science Institute. His research interests are in political behavior and computational methods, with past substantive work focusing on identity politics and the radical right.
Tevfik Murat Yildirim
Tevfik Murat Yildirim is an Associate Professor of Political Science at the University of Stavanger, Norway, and an affiliate with the “Politics of Inequality” cluster at the University of Konstanz. His research focuses on elite behavior, structural inequalities and biases in politics (primarily related to gender), and representation.
Notes
1. We used “social distanc” to capture both ”social distance” and “social distancing.” URLs were removed from messages before conducting the keyword searches.
2. We provide full details of the classification procedures in Supplementary Materials A and present descriptive statistics in Supplementary Materials B.
3. For a full overview of this method, see Athey et al. (Citation2021) or Liu et al. (Citation2022).
References
- Abadie, A., Diamond, A., & Hainmueller, J. (2010). Synthetic control methods for comparative case studies: Estimating the effect of California’s tobacco control program. Journal of the American Statistical Association, 105(490), 493–505. https://doi.org/10.1198/jasa.2009.ap08746
- Amat, F., Arenas, A., Falcó-Gimeno, A., & Muñoz, J. (2020). Pandemics meet democracy. Experimental evidence from the covid-19 crisis in Spain. SocArXiv papers, 6, 1–31.
- Andreottola, G. (2021). Flip-flopping and electoral concerns. The Journal of Politics, 83(4), 1669–1680. https://doi.org/10.1086/715070
- Arceneaux, K., Dunaway, J., Soroka, S., & Wilson, R. K. (2018). Elites are people, too: The effects of threat sensitivity on policymakers’ spending priorities. PLOS one, (4), e0193781. https://doi.org/10.1371/journal.pone.0193781
- Asimakopoulou, K., Hoorens, V., Speed, E., Coulson, N. S., Antoniszczak, D., Collyer, F., Deschrijver, E., Dubbin, L., Faulks, D., Forsyth, R., Goltsi, V., Harsløf, I., Larsen, K., Manaras, I., Olczak‐Kowalczyk, D., Willis, K., Xenou, T., & Scambler, S. (2020). Comparative optimism about infection and recovery from Covid-19; implications for adherence with lockdown advice. Health Expectations, 23(6), 1502–1511. https://doi.org/10.1111/hex.13134
- Athey, S., Bayati, M., Doudchenko, N., Imbens, G., & Khosravi, K. (2021). Matrix completion methods for causal panel data models. Journal of the American Statistical Association, 116(536), 1716–1730. https://doi.org/10.1080/01621459.2021.1891924
- Attema, A. E., L’haridon, O., Raude, J., Seror, V., & Group, C. (2021). Beliefs and risk perceptions about covid-19: Evidence from two successive French representative surveys during lockdown. Frontiers in Psychology, 12, 619145. https://doi.org/10.3389/fpsyg.2021.619145
- Baccini, L., & Leemann, L. (2021). Do natural disasters help the environment? How voters respond and what that means. Political Science Research and Methods, 9(3), 468–484. https://doi.org/10.1017/psrm.2020.25
- Bai, J. (2009). Panel data models with interactive fixed effects. Econometrica, 77(4), 1229–1279.
- Baker, A. C., Larcker, D. F., & Wang, C. C. (2022). How much should we trust staggered difference-in-differences estimates? Journal of Financial Economics, 144(2), 370–395. https://doi.org/10.1016/j.jfineco.2022.01.004
- Bayerlein, M., Boese, V.A, Gates, S., Kamin, K., & Mansoob Murshed, S.M. (2021). Populism and covid-19: How populist governments (Mis) handle the pandemic. Journal of Political Institutions and Political Economy, 2(3), 389–428. https://doi.org/10.1561/113.00000043
- Bayes, R., & Druckman, J. N. (2021). Motivated reasoning and climate change. Current Opinion in Behavioral Sciences, 42, 27–35. https://doi.org/10.1016/j.cobeha.2021.02.009
- Bisbee, J., & Da in Lee, D. (2022). Objective facts and elite cues: Partisan responses to covid-19. The Journal of Politics, 84(3), 1278–1291. https://doi.org/10.1086/716969
- Bisgaard, M., & Slothuus, R. (2018). Partisan elites as culprits? How party cues shape partisan perceptual gaps. American Journal of Political Science, 62(2), 456–469. https://doi.org/10.1111/ajps.12349
- Biswas, M., Rahaman, S., Kumar Biswas, T., Haque, Z., & Ibrahim, B. (2021). Association of sex, age, and comorbidities with mortality in covid-19 patients: A systematic review and meta-analysis. Intervirology, 64(1), 36–47. https://doi.org/10.1159/000512592
- Bol, D., Giani, M., Blais, A., & John Loewen, P. (2021). The effect of COVID-19 lockdowns on political support: Some good news for democracy? European Journal of Political Research, 60(2), 497–505. https://doi.org/10.1111/1475-6765.12401
- Brantly, C., & Sant’anna, P. H. (2021). Difference-in-differences with multiple time periods. Journal of Econometrics, 225(2), 200–230. https://doi.org/10.1016/j.jeconom.2020.12.001
- Bresnahan, J., & Caygle, H. (2020). Pelosi announces big expansion of COVID testing for lawmakers. Politico. November 2020. https://www.politico.com/news/2020/11/05/pelosi-covid-testing-congress-434399
- Broockman, D. E., & Skovron, C. (2018). Bias in perceptions of public opinion among political elites. American Political Science Review, 112(3), 542–563. https://doi.org/10.1017/S0003055418000011
- Bruine de Bruin, W., & Isaacowitz, D. (2021). Age differences in COVID-19 risk perceptions and mental health: Evidence from a National US survey conducted in March 2020. The Journals of Gerontology: Series B, 76(2), e24–29. https://doi.org/10.1093/geronb/gbaa074
- Card, D., & Krueger, A. B. (1993). Minimum wages and employment: A case study of the fast food industry in new jersey and Pennsylvania. National bureau of economic research working paper 4509.
- Cillizza, C. (2021). Two charts that show just how old this congress actually is. CNNPolitics January 2021. https://edition.cnn.com/2021/01/29/politics/congress-age/index.html
- Cook, N., & Choi, M. (2020). Trump rallies his base to treat coronavirus as a ‘Hoax’. Politico. 2020. https://www.politico.eu/article/trump-rallies-his-base-to-treat-coronavirus-as-a-hoax/
- Cormack, L., & Meidlinger, K. (2021). Congressional communication in a pandemic: ‘Follow the leader’ politics and responsive representation. Congress & the Presidency, 49(3), 1–26. https://doi.org/10.1080/07343469.2021.1955037
- Cunningham, S. (2021). Causal inference: The mixtape. Yale University Press.
- Debus, M., & Tosun, J. (2021). Political ideology and vaccination willingness: Implications for policy design. Policy Sciences, 54(3), 477–491. https://doi.org/10.1007/s11077-021-09428-0
- Devlin, J., Chang, M.-W., Lee, K., & Toutanova, K. (2018). Bert: Pre-training of deep bidirectional transformers for language understanding. Arxiv Preprint Arxiv:1810.04805.
- De Vries, C. E., Bakker, B. N., Hobolt, S. B., & Arceneaux, K. (2021). Crisis signaling: How Italy’s coronavirus lockdown affected incumbent support in other European Countries. Political Science Research and Methods, 9(3), 451–467. https://doi.org/10.1017/psrm.2021.6
- Doherty, D., Dowling, C. M., & Miller, M. G. (2016). When is changing policy positions costly for politicians? Experimental evidence. Political Behavior, 38(2), 455–484. https://doi.org/10.1007/s11109-015-9321-9
- Drummond, C., & Fischhoff, B. (2017). Individuals with greater science literacy and education have more polarized beliefs on controversial science topics. Proceedings of the National Academy of Sciences, 114(36), 9587–9592. https://doi.org/10.1073/pnas.1704882114
- Eberl, J.-M., A Huber, R., & Greussing, E. (2021). From populism to the ‘Plandemic’: Why populists believe in covid-19 conspiracies. Journal of Elections, Public Opinion and Parties, 31(sup1), 272–284. https://doi.org/10.1080/17457289.2021.1924730
- Engel-Rebitzer, E., Stokes, D. C., Meisel, Z. F., Purtle, J., Doyle, R., & Buttenheim, A. M. (2022). Partisan differences in legislators’ discussion of vaccination on twitter during the COVID-19 era: Natural language processing analysis. Jmir Infodemiology, 2(1), e32372. https://doi.org/10.2196/32372
- Esaiasson, P., Sohlberg, J., Ghersetti, M., & Johansson, B. (2021). How the coronavirus crisis affects citizen trust in institutions and in unknown others: Evidence from ‘the Swedish experiment’. European Journal of Political Research, 60(3), 748–760. https://doi.org/10.1111/1475-6765.12419
- Evans, J. H., & Hargittai, E. (2020). Who doesn’t trust Fauci? The public’s belief in the expertise and shared values of scientists in the covid-19 pandemic. Socius: Sociological Research for a Dynamic World, 6, 2378023120947337. https://doi.org/10.1177/2378023120947337
- Federal Reserve Bank. (2022). Unemployment rate (UNRATE) FRED St. Louis Fed. St. Louis Fed: Economic data. August 2022. https://fred.stlouisfed.org/series/UNRATE
- Fetzer, T., Witte, M., Hensel, L., Jachimowicz, J. M., Haushofer, J., Ivchenko, A., Caria, S., Reutskaja, E., Roth, C., Fiorin, S. & Gomez, M. (2020). Global behaviors and perceptions in the covid-19 pandemic. National Bureau of Economic Research. http://www.nber.org/papers/w27082
- Gadarian, S. K., Wallace Goodman, S., Pepinsky, T. B., & Lupu, N. (2021). Partisanship, health behavior, and policy attitudes in the early stages of the COVID-19 pandemic. Public Library of Science ONE, 16(4), e0249596. https://doi.org/10.1371/journal.pone.0249596
- Gilardi, F., Alizadeh, M., & Kubli, M. (2023). Chatgpt outperforms crowd-workers for text-annotation tasks. Proceedings of the National Academy of Sciences, 120(30). https://doi.org/10.1073/pnas.2305016120 arXiv Preprint arXiv:2303.15056.
- Gobillon, L., & Magnac, T. (2016). Regional policy evaluation: Interactive fixed effects and synthetic controls. Review of Economics and Statistics, 98(3), 535–551. https://doi.org/10.1162/REST_a_00537
- Gollust, S. E., Nagler, R. H., & Franklin Fowler, E. (2020). The emergence of COVID-19 in the us: A public health and political communication crisis. Journal of Health Politics, Policy and Law, 45(6), 967–981. https://doi.org/10.1215/03616878-8641506
- GovTrack. (2022). COVID-19 in congress. GovTrack. 2022. https://www.govtrack.us/covid-19
- Hart, P. S., & Nisbet, E. C. (2012). Boomerang effects in science communication: How motivated reasoning and identity cues amplify opinion polarization about climate mitigation policies. Communication Research, 39(6), 701–723. https://doi.org/10.1177/0093650211416646
- Hersh, E. D. (2013). Long-term effect of September 11 on the political behavior of victims’ families and neighbors. Proceedings of the National Academy of Sciences, 110(52), 20959–20963. https://doi.org/10.1073/pnas.1315043110
- Hertel-Fernandez, A., Stokes, L. C., & Stokes, L. C. (2019). Legislative staff and representation in congress. American Political Science Review, 113(1), 1–18. https://doi.org/10.1017/S0003055418000606
- Jones, B. D. (2001). Politics and the architecture of choice: Bounded rationality and governance. University of Chicago Press.
- Kalla, J. L., & Porter, E. (2021). Correcting bias in perceptions of public opinion among American elected officials: Results from two field experiments. British Journal of Political Science, 51(4), 1792–1800. https://doi.org/10.1017/S0007123419000711
- Kavakli, K. C. (2020). Did populist leaders respond to the covid-19 pandemic more slowly? Evidence from a global sample. Unpublished Manuscript. Milano: Covid crisis Lab, Bocconi University
- Kertzer, J. D. (2022). Re-assessing elite-public gaps in political behavior. American Journal of Political Science, 66(3), 539–553. https://doi.org/10.1111/ajps.12583
- Kousser, T., & Tranter, B. (2018). The influence of political leaders on climate change attitudes. Global Environmental Change, 50, 100–109. https://doi.org/10.1016/j.gloenvcha.2018.03.005
- Kreps, S. E., & Kriner, D. L. (2020). Model uncertainty, political contestation, and public trust in science: Evidence from the COVID-19 pandemic. Science Advances, 6(43), eabd4563. https://doi.org/10.1126/sciadv.abd4563
- Lasco, G. (2020). Medical populism and the COVID-19 pandemic. Global Public Health, 15(10), 1417–1429. https://doi.org/10.1080/17441692.2020.1807581
- Latkin, C. A., Dayton, L., Moran, M., Strickland, J. C., & Collins, K. (2021). Behavioral and psychosocial factors associated with covid-19 skepticism in the United States. Current Psychology, 41(11), 7918–7926. https://doi.org/10.1007/s12144-020-01211-3
- LeBlanc, P. (2020). Republican lawmakers who downplayed coronavirus concerns face backlash over early vaccinations. CNN. CNNPolitics. 2020. Retrieved: January 6, 2022, from. https://edition.cnn.com/2020/12/21/politics/vaccine-lawmakers-backlash/index.html
- Liu, L., Wang, Y., & Xu, Y. (2022). A practical guide to counterfactual estimators for causal inference with time-series cross-sectional data. American Journal of Political Science, 68(1), 160–176. https://doi.org/10.1111/ajps.12723
- MarketWatch. (2022). DJIA: Dow jones industrial average overview. Dow Jones Industrial Average. August. 2022. https://www.marketwatch.com/investing/index/djia
- Merkley, E., & Stecula, D. A. (2021). Party cues in the news: Democratic elites, republican Backlash, and the dynamics of climate skepticism. British Journal of Political Science, 51(4), 1439–1456. https://doi.org/10.1017/S0007123420000113
- Mizrahi, B., Shilo, S., Rossman, H., Kalkstein, N., Marcus, K., Barer, Y., Keshet, A., Shamir-Stein, N., Shalev, V., Zohar, A. E., Chodick, G., & Segal, E. (2020). Longitudinal symptom dynamics of COVID-19 infection. Nature Communications, 11(1), 6208. https://doi.org/10.1038/s41467-020-20053-y
- Newman, B. J., & Hartman, T. K. (2019). Mass shootings and public support for gun control. British Journal of Political Science, 49(4), 1527–1553. https://doi.org/10.1017/S0007123417000333
- Newman, D., Lewandowsky, S., & Mayo, R. (2022). Believing in nothing and believing in everything: The underlying cognitive paradox of anti-covid-19 vaccine attitudes. Personality and Individual Differences, 189, 111522. https://doi.org/10.1016/j.paid.2022.111522
- Nguyen, D. Q., Vu, T., & Tuan Nguyen, A. (2020). Bertweet: A pre-trained language model for English tweets. Proceedings of the 2020 Conference on Empirical Methods in Natural Language Processing: System Demonstrations (pp. 9–14). Association for Computational Linguistics, Online. https://doi.org/10.18653/v1/2020.emnlp-demos.2
- NPR. (2022). State of the Union attendees needed a COVID test. 6 legislators tested positive. Npr. March. 2022. https://www.npr.org/2022/03/01/1083845000/state-of-the-union-covid
- Olmastroni, F., Guidi, M., Martini, S., & Isernia, P. (2021). Framing effects on the COVID-19 see-saw. Swiss Political Science Review, 27(2), 257–270. https://doi.org/10.1111/spsr.12453
- OpenAI. (2022). GPT-3.5 language model. 2022. https://platform.openai.com/models/gpt-3.5
- Paz, C. (2020). All the president’s lies about the coronavirus. The Atlantic. 2020. https://www.theatlantic.com/politics/archive/2020/11/trumps-lies-about-coronavirus/608647/
- Pearce, L., & Cooper, J. (2021). Fostering COVID-19 safe behaviors using cognitive dissonance. Basic and Applied Social Psychology, 43(5), 267–282. https://doi.org/10.1080/01973533.2021.1953497
- Pennycook, G., McPhetres, J., Bago, B., & Rand, D. G. (2020). Predictors of attitudes and misperceptions about covid-19 in Canada, the UK, and the USA. PsyArXiv Preprints, 10, 1–25.
- Pereira, M. M. (2021). Understanding and reducing biases in elite beliefs about the electorate. American Political Science Review, 115(4), 1308–1324. https://doi.org/10.1017/S000305542100037X
- Pereira, M. M., & Öhberg, P. (2020, October). The expertise curse: How policy expertise can hinder responsiveness. OSF Preprints. https://doi.org/10.31219/osf.io/74kma
- Pink, S. L., Chu, J., Druckman, J. N., Rand, D. G., & Willer, R. (2021). Elite party cues increase vaccination intentions among republicans. Proceedings of the National Academy of Sciences, 118(32), e2106559118. https://doi.org/10.1073/pnas.2106559118
- ProPublica. (2022). Recent congressional statements. 2022. https://projects.propublica.org/represent/statements
- Rachev, N. R., Han, H., Lacko, D., Gelpı́, R., Yamada, Y., Lieberoth, A., & Aimone, J. A. (2021). Replicating the disease framing problem during the 2020 COVID-19 pandemic: A study of stress, worry, trust, and choice under risk. PLOS one, 16(9), e0257151. https://doi.org/10.1371/journal.pone.0257151
- Rao, A., Morstatter, F., Hu, M., Chen, E., Burghardt, K., Ferrara, E., & Lerman, K. (2021). Political partisanship and antiscience attitudes in online discussions about covid-19: Twitter content analysis. Journal of Medical Internet Research, 23(6), e26692. https://doi.org/10.2196/26692
- Ross, L., Greene, D., & House, P. (1977). The ‘false consensus effect’: An egocentric bias in social perception and attribution processes. Journal of Experimental Social Psychology, 13(3), 279–301. https://doi.org/10.1016/0022-1031(77)90049-X
- Russell, A. (2021). Senate representation on twitter: National policy reputations for constituent communication. Social Science Quarterly, 102(1), 301–323. https://doi.org/10.1111/ssqu.12904
- Rutjens, B. T., van der Linden, S., & van der Lee, R. (2021). Science skepticism in times of covid-19. Group Processes & Intergroup Relations, 24(2), 276–283. https://doi.org/10.1177/1368430220981415
- Sakakibara, R., & Ozono, H. (2020). Psychological research on the covid-19 crisis in Japan: Focusing on infection preventive behaviors, future prospects, and information dissemination behaviors. Psyarxiv Preprints, 1–19. https://doi.org/10.31234/OSF.IO/97ZYE
- Salgado, S., & Berntsen, D. (2021). ‘It won’t happen to us’: Unrealistic optimism affects covid-19 risk assessments and attitudes regarding protective behaviour. Journal of Applied Research in Memory and Cognition, 10(3), 368–380. https://doi.org/10.1016/j.jarmac.2021.07.006
- Sheffer, L., John Loewen, P., Soroka, S., Walgrave, S., & Sheafer, T. (2018). Nonrepresentative representatives: An experimental study of the decision making of elected politicians. American Political Science Review, 112(2), 302–321. https://doi.org/10.1017/S0003055417000569
- Sheffer, L., & Loewen, P. (2019). Electoral confidence, overconfidence, and risky behavior: Evidence from a study with elected politicians. Political Behavior, 41(1), 31–51. https://doi.org/10.1007/s11109-017-9438-0
- Simon, H. A. (1957). Models of Man, Social and Rational: Mathematical Essays on Rational Human Behavior in Society Setting. New York, NY: Wiley.
- Stecula, D. A., & Pickup, M. (2021). How populism and conservative media fuel conspiracy beliefs about covid-19 and what it means for covid-19 behaviors. Research & Politics, 8(1), 2053168021993979. https://doi.org/10.1177/2053168021993979
- Steffen, J., & Cheng, J. (2021). The influence of gain-loss framing and its interaction with political ideology on social distancing and mask wearing compliance during the covid-19 pandemic. Current Psychology, 42, 8028–8038. https://doi.org/10.1007/s12144-021-02148-x
- Sun, L., & Abraham, S. (2021). Estimating dynamic treatment effects in event studies with heterogeneous treatment effects. Journal of Econometrics, 225(2), 175–199. https://doi.org/10.1016/j.jeconom.2020.09.006
- Taber, C. S., & Lodge, M. (2006). Motivated skepticism in the evaluation of political beliefs. American Journal of Political Science, 50(3), 755–769. https://doi.org/10.1111/j.1540-5907.2006.00214.x
- Tappin, B. M. (2022). Estimating the between-issue variation in party elite cue effects. Public Opinion Quarterly, 86(4), 862–885. https://doi.org/10.1093/poq/nfac052
- Tetlock, P. E. (2017). Expert political judgment. Princeton University Press.
- Tversky, A., & Kahneman, D. (1974). Judgment under uncertainty: Heuristics and biases: Biases in judgments reveal some heuristics of thinking under uncertainty. Science, 185(4157), 1124–1131. https://doi.org/10.1126/science.185.4157.1124
- Unan, A., Klüver, H., Hobolt, S., & Rodon, T. (2023). The political effects of government communication during crises. OSF Preprints August, 30.
- Van Vugt, M. (2006). Evolutionary origins of leadership and followership. Personality and Social Psychology Review, 10(4), 354–371. https://doi.org/10.1207/s15327957pspr1004_5
- Visconti, G. (2022). After the flood: Disasters, ideological voting and electoral choices in chile. Political Behavior, 44(4), 1985–2004. https://doi.org/10.1007/s11109-022-09814-1
- Wise, T., Zbozinek, T. D., Michelini, G., Hagan, C. C., & Mobbs, D. (2020). Changes in risk perception and self-reported protective behaviour during the first week of the COVID-19 pandemic in the United States. Royal Society Open Science, 7(9), 200742. https://doi.org/10.1098/rsos.200742
- Wolfe, D., & Dale, D. (2020). All of the times president trump said covid-19 will disappear. Cnn. October 2020. https://edition.cnn.com/interactive/2020/10/politics/covid-disappearing-trump-comment-tracker/
- Xu, P., & Cheng, J. (2021). Individual differences in social distancing and mask-wearing in the pandemic of covid-19: The role of need for cognition, self-control and risk attitude. Personality and Individual Differences, 175, 110706. https://doi.org/10.1016/j.paid.2021.110706