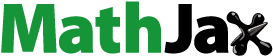
JSE Editorship
AR: Congratulations on beginning your term as editor of the Journal of Statistics Education, Jeff, and thanks for agreeing to this interview. I believe that you were also involved with the creation of JSE 25 years ago. Let me ask you to look back and then forward: What have been JSE’s primary contributions in the past 25 years, and what are your goals for the next three years?
JW: You are right that I was at the meeting, at North Carolina State, that resulted in JSE being created—and edited by Oberlin College alumna Jackie Dietz (who graduated long before I joined the Oberlin faculty). Our goal was to provide an outlet for sharing ideas about teaching, particularly at the undergraduate level (since Teacher’s Corner in The American Statistician was already providing an outlet for teaching ideas at the graduate level). I think that JSE has met that goal.
Early on, JSE articles often had the flavor of “Here is a cool idea.” (Remember that there weren’t blogs back then.) Over time, JSE articles moved more in the direction of “Here is some serious evidence about how and why something works.” I’m hoping that in the next three years we will continue to publish papers that address the scholarship of teaching and learning, while also giving teachers useful and classroom-tested ideas they can use. When I applied for the editorship I looked at data(!) on the numbers of views of JSE articles. The view tracking only goes back a few years, but in a sample of 30 articles I found overwhelming evidence that the most widely read articles are those that contain something a STAT 101 teacher could use immediately to change their course. I have a bias for such articles, and I like cool ideas. I respect the work of people who make fundamental contributions to statistics education with an eye on the long horizon; but I get really excited when I glance at the abstract of a paper that I think will tell me something I can do that will help the students I’ll be teaching in the coming semester or two.
I also told the editor-selection committee that I would like to see JSE include more in the area of “what to teach” in addition to “how to teach.” The “how to teach” papers are helpful (Should I flip my classroom? Should I use group projects? What about mnemonic devices to help students remember concepts?) but I also like to hear reasoned arguments about what to teach (Bootstrapping? Time series? Power and effect size?).
A big part of the “what to teach” picture is our new section on teaching Data Science. I’m delighted that Nick Horton has agreed to take the lead in guiding this part of the journal, which we hope will provide articles on how to teach, as well as what to teach. As a profession, we are far from settled on what data science is, what it should be, and how it should be taught. JSE has the chance to be a valuable resource for teachers here.
I’m also excited that Matt Hayat is going to continue an initiative he started before I came along on teaching statistics in the health sciences. ASA has a section by that name and they have sponsored some outstanding talks at JSM; now there will be a place to publish papers under that heading.
A Quarter-Century of Teaching
AR: I like your delineation of “what to teach” and “how to teach,” and I’m also liking the comparison of 25 years ago with today. So my next question is: How has what you teach changed between 25 years ago and today? (And I suspect that you already know what my next question will be.)
JW: Mostly, as I’ve become more experienced as a teacher my courses have moved farther away from mathematics and closer to the historical roots of statistics. Statistics is a blend of art and science. When I was younger I took a more mathematical view of statistics, favoring the science side, but now I do more to play up the art side when I teach. The art of statistics shows up most clearly when building a model, such as in a regression situation where there are lots of models that one might use but there is generally no “best” model. But even a t-test is a kind of model (although we don’t usually say that in STAT 101), and the way I have taught t-tests and related topics has always deviated some from the standard statistical catechism, but more so today than years ago.
I’m a Bayesian and always have been (I joined the Bayes club long before it was popular) so my courses have always had a bit of a Bayesian flavor to them, even when I’ve been teaching the frequentist paradigm (which has been most of the time). What I mean is that, for example, I’ve never been a big fan of hypothesis testing and have favored parameter estimation (which might include making a confidence interval—but if I make a CI, I’m not thinking that every value in the CI is as likely as any other to be the truth; unlike some statisticians, I’m favoring the point estimate and near neighbors over values at the edge of the CI, since I’m not in a black/white, reject/retain frame of mind). I point out to my students that in most situations we know that H0 can’t possibly be exactly true (Is it possible that the population mean is exactly 80? Not 80.0001 but 80?) but as George Box said, all models are wrong yet some are useful and it might be sensible to act as if H0 were true.
One of my father’s favorite lines was “We measure with a micrometer, draw the line with a piece of chalk, and make the cut with an axe.” Given the various uncertainties associated with most data collection and analysis projects, it is downright silly to report a p-value as 0.032874 or even as 0.0328. Something like “0.03 ± 0.01” would be better. Moreover, even if the model is perfect and we have no measurement error to worry about, etc., the p-value for a t-test varies a lot from one sample to the next—take a look at “Dance of the p Values” on YouTube, for example.
But the main idea, as a Bayesian would tell you, is to not treat a p-value as some kind of magical quantity, since it can differ from the probability that H0 is true by as much as an order of magnitude. If p = 0.03 then there might be a 30% chance that H0 is “true,” in the sense that a 50–50 prior on “theta is in the neighborhood of zero” versus “no, theta is far from zero” would lead to a posterior probability of 30% that theta is near zero. Not 3%, but 30%. Or, if you don’t like the idea of using prior distributions, then consider a simulation in which sometimes the null is true and sometimes it is false. Collect thousands of simulation results and look at the subset of those results for which the p-value is between 0.049 and 0.050. One might hope that in that subset the null would have been true 5% of the time; but it turns out that the null is true more than 22% of the time. OK, 22% is not an order of magnitude greater than 5%, but 22% is a lower bound on the fraction of simulations for which H0 is true when the p-value is 0.05. And all of this trouble with p-values this isn’t even taking into consideration the problem with using 0.05 as a threshold for statistical significance, as if 0.05 were engraved in stone. I have a much better appreciation for all of this today than I did 25 years ago, and that has informed my teaching.
When I was younger I said a few words about power, but I didn’t talk about effect size (to my eternal shame) as if “statistically significant” were more important that “practically significant.” Now I make effect size a key part of my courses. For example, the guidelines I give for student project reports now require that the report discuss effect size; but in my younger days I didn’t do this. (I haven’t yet written letters of apology to the hundreds of students I had in classes in which I didn’t discuss effect size.)
I now show my students logistic regression in STAT 101, although I don’t expect them to learn how to do it (i.e., “No, this won’t be on the final exam—but I want you to be aware of it.”) I also do more with data visualization—technology has come a long way since the 1980s. I always talked about the regression effect but now I make a bigger deal of it.
Of course, I didn’t teach bootstrapping or randomization testing 25 years ago. I knew what those things were, but I didn’t teach them because software back then didn’t allow for easy use of those methods. Again, the world of statistics has changed a lot over a quarter century. But that is probably better as an answer to “How has ‘how you teach’ changed over 25 years?”
One thing I haven’t yet done is add causal inference to my courses in any substantial way. I’ve just recently arrived at the causal inference party—you may have heard me plug Judea Pearl’s The Book of Why at JSM last summer—finally accepting Milo Schield’s long-standing invitation. I’m just learning how to dance to the music of causal inference. I love telling my students about Simpson’s Paradox, but for years I’ve only been telling part of the story. I see Danny Kaplan and a few others in the room, but there is a lot of open space on the dance floor and most statisticians are either outside, looking in through the window, or are outside and are intentionally looking the other way.
AR: Thanks for all of this food for thought that you’ve provided, which also leads to many possible follow-up questions. First, when you talk about effect size in STAT 101 and expect those students to report effect size, do you mean something more than a confidence interval for mu1 – mu2?
JW: Actually, I mean something different than a confidence interval for mu1 – mu2. If you think of a CI as the inversion of a test, then in that sense the CI gives the same information as the test. In the two-sample setting, the CI is the set of numbers such that “H0: mu1 – mu2 = theta” would be retained (with 95% confidence associated with a 5% test, e.g.). If n1 and n2 are large, then the CI will be narrow—and likely to not contain zero—and Statistician A will say that we can be highly confident that mu1 – mu2 is between 1 and 7, say, while Statistician B will say that we have statistically significant evidence that mu1 is not equal to mu2. But these aren’t satisfactory reports.
It isn’t enough to infer that mu1 differs from mu2. As I said in my previous answer, most of the time it is not believable that mu1 could exactly equal mu2. For practical purposes, we care how far apart they are. As statisticians we are always asking “Compared to what?” For mu1 – mu2, the “what” is the standard deviation. In STAT 101 I take the easy approach of defining (sample) effect size as —ybar1 – ybar2—/SD, where SD is the larger of s1 and s2. More formally, one might use the pooled SD here (and get Cohen’s D), but I’m trying to keep things simple (and if s1 and s2 are very different, then I question a comparison of means in the first place—but that’s an argument for another day).
So don’t just tell me that the difference in sample means was 4 and the margin of error was 3 and thus the CI is (1,7). Is a difference of 4 a big difference? If the inherent variability in the data is large and the SD is 40, then a difference of 4 is only one-tenth of a standard deviation, which is nothing, and even a difference of 7 is less than one-fifth of a standard deviation, which is a small effect.
A p-value might be small for two reasons: (1) there is a big effect or (2) we have large samples. For example, in the two-sample t-test setting if and the two sample SDs are equal then t = (sample effect size)*sqrt(n/2). Thus, when we have large samples we can expect a large test statistic and a small p-value even if there is a small difference in the means. The same thing happens with categorical data and with linear models: a large n means a small p-value. All statisticians know this and our students figure it out even if we forget to directly tell them. (There is the related question of whether it is more impressive to have a tiny p-value with large samples or a smallish p-value with small samples. But again, that’s a topic for another day.)
If I tell you that there is a statistically significant evidence against H0, I have told you very little. I would rather tell you “(a) Here is the effect size that I saw: the two sample means were separated by half of a standard deviation and (b) the sample sizes were large enough that this size of a sample effect is unlikely to arise by chance (p = 0.03).” You can think about whether a shift of half of an SD is important in the current context and you can ask yourself whether a p-value of 0.03, combined with your external understanding of the situation, is strong evidence of a real difference (recalling from my previous answer that p = 0.03 isn’t terribly strong evidence on its own—but maybe that’s a two-sided p-value and your prior experience allows you to cut it in half and get p = 0.015, since you allow the use of prior information in your frequentist world when you make the decision to use a directional alternative hypothesis.;-)
I show my students sets of overlapping normal curves to help them develop a sense of what an effect size is; sometimes I’ll also talk about the probability that an observation from group 2 will exceed the mean of group 1. I take a similar approach with ANOVA, looking at parallel dotplots to understand the different ways that an F-test might be significant: (1) large separation of means (large effect size) or (2) large n’s or (3) small within-group SDs.
By the way, one of my pet peeves (and I seem to have many…) is when statisticians(!) say “We have evidence that the (population) means are significantly different” when they should say “We have significant evidence that the (population) means are different.” Not that they are significantly different, but that they are different, period. I understand students using sloppy language, but we statisticians should be more careful here, given how confusing hypothesis testing is. HA doesn’t say that mu1 and mu2 are significantly different, HA says that mu1 and mu2 are different. The question is whether the sample means are far enough apart that we might infer that the population means are not identical. I quoted my father in answering the last question and I’ll quote Michael Hartoonian here: “The temple of reason is entered through the courtyard of habit.” I try to instill good habits, in myself and in my students, through the careful use of language. (And yet I try not to be pedantic. It is a delicate balance.) Don’t reject H0 and then say that you found the (population) means to be significantly different; say that you found them to be different.
AR: I agree that careful use of technical language is important, but I also think it’s important to be able to state things in everyday terms. Do you object to saying that the sample means differ significantly, if the test indicates strong evidence that the population means differ?
JW: Not at all. A small p-value says that the sample means differ by a statistically significant amount. What I object to is a conclusion such as “The t-test tells us that the drug is significantly better than the placebo.” That’s not a proper statement. It would be OK to say “The t-test tells us that the drug is better than the placebo” (implicitly referring to the two population means, and begging for a follow-up on effect size so that we know if “better” is a small or large amount) or “The t-test tells us that the drug was significantly better than the placebo in this experiment” (implicitly referring to the two sample means). My objection to “The t-test tells us that the drug is significantly better than the placebo” parallels my objection to a student writing “H0: ybar1 = ybar2,” which is something I sometimes see on exams, and which I mark as wrong (after wondering how I could have failed so badly as a teacher that my student would write such a thing).
AR: You mentioned that you do more with data visualization than you used to. Does this mean more than the typical graphs that one sees in Stat 101—histograms, boxplots, bar graphs, scatterplots?
JW: I show my students animated graphics such as at gapminder.org and I’ll spend part of a day having the students play around with the wide variety of data visualizations that Kari Lock Morgan has collected at http://www.personal.psu.edu/klm47/visualization.htm. During a computer lab session I’ll have students use the mPlot() command within the mosaic package in R, so that they can easily use color and faceting while working with scatterplots. This lets them deal with four variables at once and doesn’t require any coding ability, just the use of drop-down menus.
AR: About the causal inference party that you’ve recently joined: I’m guilty of trying to teach my Stat 101 students that causal conclusions can be drawn from randomized experiments but not from observational studies. I realize that this is overly simplistic, but I emphasize this theme repeatedly throughout my course in an effort to provide a lasting impact. Do you advise me and others to stop emphasizing this simplistic formulation and instead describe situations in which causal inference is warranted from observational data?
JW: Yes. Everyone knows that correlation does not imply causation and everyone forgets it, especially when forgetting is convenient. Statisticians do well in helping students understand the difference between correlation and causation and in being skeptical of causal conclusions drawn from correlations; but as a profession we have gone too far in that direction. Pearl’s The Book of Why explains a lot of that history, and how our unwillingness to think about causation, outside of randomized experiments, has hampered scientific and societal progress. You should continue to tell your students to beware of lurking variables and confounded effects and to celebrate double-blind, randomized, controlled experiments. But you should also tell your students that causal inference is possible with observational data.
Look, I can’t hold a candle to R.A. Fisher as a statistician, but he was quite wrong about smoking and lung cancer. Fisher, who smoked a pipe, refused to believe that smoking caused cancer and dismissed all evidence of a causal link by talking about alternative explanations. Jerome Cornfield considered the possibility of a confounding factor, such a gene that predisposed people to smoke and also to get lung cancer. He showed, mathematically, that if smokers are W times more likely to get lung cancer, then the confounding factor needs to be W times more common in smokers than in nonsmokers to explain the difference in cancer risk. Cornfield used W = 9 in his 1954 paper whereas a more recent estimate is W = 23, but even taking W = 9 leads to an implausible situation for someone like Fisher. As Pearl puts it “If 11 percent of nonsmokers have [the “smoking gene”], then 99 percent of the smokers would have to have it…if 12 percent…then it becomes mathematically impossible for the cancer gene to account fully for the association between smoking and cancer.” So yes, confounding might be an issue with observational data, but if we have enough evidence then we can rule out certain explanations. We can talk about causation with observational data.
But I’m just starting to bring this into my STAT 101 class. I’ve stopped telling my students that we can only think about causation if we have experimental data. I have many times shown students the three schematics, for causation, common response, and confounding, that David Moore put into his textbooks many years ago. (Moore also listed five “Hill criteria” for establishing causation.) I talk about spurious association, where controlling for Z eliminates an apparent link between X and Y. I have sometimes added a fourth schematic, for a chain relationship (X Z
Y).
A further step, which I have yet to take, is to add a schematic for what is called a collider (X Z ← Y) and to talk about how controlling for Z here can induce a link between X and Y. Borrowing from Pearl: Suppose A and B are independent diseases and neither A nor B will land someone in the hospital, but the combination of A and B will. Then if you look at people in the hospital, A and B are positively associated. If A alone or B alone will put you in the hospital, then hospitalized patients show a negative association between A and B. Google “Berkson’s paradox.” I have been teaching Simpson’s paradox for years, but I think I should add Berkson’s paradox as part of my discussion of causation.
My grandfather was a big fan of baseball and I remember him being puzzled about the fact that pitchers can’t hit. Why not? He wondered aloud, many times. Well, if X is pitching talent and Y is hitting talent and Z is being in the major leagues, then we have X Z ← Y. If we condition on Z (i.e., we only look at major league players) there is a strong negative correlation between pitching and hitting (although we almost never get data on the poor pitching ability of guys who play first base.) The thing is, if someone is low on X and on Y (“can’t pitch; can’t hit”) they don’t make it very far in baseball. We only see players for whom either X or Y is strongly positive—very good pitchers or very good hitters. My grandfather would see very good pitchers with terrible batting averages and wonder how such good athletes—the rare players who can make the big leagues as pitchers—were such lousy hitters.
I was only 10 years old when my grandfather died, but even when I was 50 years old I didn’t know how to use causal diagrams to clarify causal relationships. It is too late for me to help my grandfather understand what is happening in baseball, but it is not too late for me to help my students understand why, when they consider the people they have dated, there is a negative association between attractiveness and intelligence. If you only date people who are either attractive or intelligent or both, then you are conditioning on Z in X Z ← Y and you think that the correlation between X and Y is negative, when really it is zero.
Students take statistics courses for many reasons, one of which is that they want to understand the world. That means understanding causation and thinking about the conditions that make a cause-effect inference valid. Most data are observational, so if we tell students that it is only valid to think about cause-and-effect for data from randomized experiments, then we are severely limiting our impact. (This is kind of like the fact that a huge fraction of the typical STAT 101 course sits between 1.645 and 1.96. That region is important, but it is only part of the number line.)
AR: I was with you until your parenthetical comment at the end. Can you elaborate on what you mean there?
JW: Harry Roberts gave a talk at a conference in the 1990s about teaching statistics and he mentioned that in his introductory course he would spend perhaps a week on hypothesis testing. I was stunned. A STAT 101 course that did not devote at least half of the semester to hypothesis tests in various settings?! Yes, he said; he did not misspeak, he was just spending class time on things other than formal inference.
Judging by textbooks, most of us spend a lot of time on statistical inference, which means trying to figure out if a p-value is large, moderate, or small. Under normality, that means dividing the positive half of the number line into (0, 1.645), (1.645, 1.96), and (1.96, infinity), which correspond to , and p < 0.05 (if HA is nondirectional). If p > 0.10 or p < 0.05, then we don’t care much about the exact value, only that our results are “not significant” or are “significant.” If
then we have something to think about and we might say “marginally significant” and worry some about conditions and robustness, the effect of an outlier, one-sided versus two-sided alternatives, and related matters.
When I meet with consulting clients I always ask them “What are you trying to find out?” and often a simple graph is all that they need—unless they are trying to appease a journal editor or a supervisor and then they often need to jump through statistical inference hoops, not because this helps them answer their question but because non-statisticians tend to think that statistics is about figuring out the size of the p-value relative to 0.05 (or 0.10).
My parenthetical comment was a dig at overemphasis on statistical inference and under-emphasis on modeling, data collection, measurement error, graphics, causation, multivariate reasoning, etc.
AR: That’s a lot about your changes to what you teach. Now let me belatedly ask about changes in how you teach over the past 25 years.
JW: I’m going to go back a bit more than 25 years, if that’s OK. When I started teaching computers weren’t used much at the STAT 101 level. I remember the momentous decision to give up one lecture session per week and replace it with a computer lab session, rather than just having a few trips to the computer lab during the semester. I also remember adding a semester-long project to the intro course. Activity-Based Statistics first appeared in 1996—so that is within the past 25 years—and my work on that project affected my teaching, as I added a set of activities to my courses.
Those were big changes. A smaller, and more recent change, as that I require students in STAT 101 to take a short online reading quiz before each class. In the past I encouraged students to look over an assigned part of the textbook before each class, but now I force them to at least skim the assigned reading well enough to be able to answer a pair of multiple-choice questions. I think that the students find this even more onerous than I do; and I really don’t enjoy uploading all of those quizzes and then checking before class to see how everyone did. But sometimes several students make the same mistake on a question so I know what to talk about that day. I also have before/after data on exam performance, where I’ve asked the same question on exams a few years apart. Being the kind of data collector that I am, I can tell you that in 2012, on exam 2, question 5, the students scored 73%; and that when I asked that same question in 2016 the class scored 85%. Analyzing data across several such pairs of questions I have strong evidence that student performance improved after I started using the reading quizzes (or else my grading has gotten softer, but I doubt that).
Of course, I make much greater use of computing, particularly graphing, than I did years ago. Before working through an example of, say, a two-sample t-test I’ll graph the data, including tossing in normal quantile plots. The computer makes it easy to investigate conditions—to behave like a statistician.
AR: I’ve been told by others that it requires more thought to say what has not changed in your teaching rather than what has changed, so that’s my next question: What have you steadfastly maintained about your teaching over your career?
JW: That’s a hard question. I suppose that one constant is that I’ve always thought of myself as a statistician, even when I moved from a statistics department (my first stop after grad school was the University of Florida) to a mathematics department (I’ve been at Oberlin College for a long time). That means that I prefer an approximate answer to an exact question over an exact answer to an approximate question. That attitude probably came through in some of my earlier answers, such as not wanting to report a p-value to three decimal places when we know that our model is only approximate—but we hope it is approximating the right thing. Also, the consulting work I do informs my teaching much more than any research that I do. And I try to bring in examples from the news, or from my personal life. That aspect of my teaching hasn’t changed.
Career Path
AR: Let me ask some questions about your career path. Where were you when you were 18 years old, and what were your thoughts about career goals at that point?
JW: When I turned 18 I had just graduated from high school (in La Crosse, WI), but during my senior year I took more classes at the local university than at my high school so I was a third of the way through college, as a mathematics major. I liked the applied side of math but I hadn’t taken any statistics courses yet, only a probability course. I knew that graduate school was in my future, and probably a teaching career, but I didn’t know that I was going to become a statistician.
AR: What led you to statistics?
JW: The summer before my senior year of high school I took a course on probability at UW-La Crosse. I hadn’t learned calculus yet, but half of my classmates were high school math teachers working on MA degrees and a few of them taught me the parts of calculus that one needs for an introductory probability course. I loved the material and I found out that I have pretty good intuition about probability. A year later, after learning calculus, I took the follow-up course on mathematical statistics, taught by Jack Scheidt. The next semester Dr. Scheidt taught a course called “Industrial Statistics,” which really was a course on stochastic operations research, although I didn’t know that. I had enjoyed studying math stat with Dr. Scheidt so I signed up for this course and it really got me hooked, with my favorite topic being queueing theory. Dr. Scheidt was an applied probabilist, but he had a Ph.D. in statistics so I thought that statistics graduate school should be my next stop after college.
I applied to Minnesota and to Wisconsin (and a couple of other schools that I was less interested in). Minnesota immediately offered me a teaching assistantship, while Wisconsin didn’t, so I went to Minnesota to get my Ph.D. This worked out well for me in three ways. For one thing, before my family moved to La Crosse I had lived in the Madison area for several years, which might have made being a student at UW a little too comfortable for me. Minneapolis wasn’t much farther away from home than Madison was, but socially it was a new space for me. I hadn’t gone away to college, so going to Minnesota—and being a Packers fan living amidst Vikings fans!—was good for me.
The second thing is that by the time Wisconsin eventually offered me a teaching assistantship I had already made up my mind to go to Minnesota. I took it that Wisconsin thought I was a decent student but not strong enough to get one of the 10(?) TA offers they sent out in their first round. During the following four years, if I ever needed a little extra motivation while study late at night instead of sleeping, I reminded myself that the admissions committee at Wisconsin placed me on their waitlist. So in a way Wisconsin did me a favor by not offering me financial support right away.
The third thing is that I loved being at Minnesota, where the School of Statistics had a Department of Theoretical Statistics, on the Minneapolis campus, and a Department of Applied Statistics, on the St. Paul campus. There was only one program for graduate students but we took courses on each campus. [Side note: I was given a desk in a shared student office in St. Paul that was right next to the office of David Hinkley. This was just a few years after the publication of Theoretical Statistics by Cox and Hinkley, which has been called “the first modern treatment of the foundations and theory of statistics,” and yet Hinkley was in the Applied Statistics department. I guess that was because a faculty line was open in the Applied department when Minnesota had the chance to hire Hinkley.] I did well in the theoretical courses, and my advisor, Don Berry, was in that department, but the applied courses gave me an appreciation for statistics that shaped my career. I think in particular about the required course in statistical consulting, taught by Sandy Weisberg.
AR: Please tell us about Weisberg’s statistical consulting course.
JW: The Department of Applied Statistics ran a consulting center with students involved in two ways. (1) There was a small office staffed by statistics graduate students who accepted walk-in clients, who were mainly (entirely?) graduate students in other departments. Statistics faculty served as back-up for the grad students. (2) There was a required consulting course, in which some of those consulting cases, plus others, were discussed. But I didn’t actually take the course. For some reason (class scheduling?), I was assigned to work in the consulting center during my second year in lieu of enrolling in the course. What I remember is tremendous discomfort in feeling out of my depth when taking questions from a client, and then the even greater feeling of satisfaction when I was able to do something that was half-way correct and that helped the client.
One memory stands out clearly. I was in the consulting center one day when a graduate student from forestry (I think it was) came in and asked for help with doing an analysis of covariance. I didn’t know what that was, so I stalled and eventually the student scheduled a follow-up meeting and left. I then ran up two flights of stairs to Sandy’s office and asked him what analysis of covariance was. He wrote out a model and said, in so many words, “You can think of ANCOVA as multiple regression where the predictor variable of interest is categorical, as in ANOVA, but there is a continuous variable also in the model, called a covariate.” Relief poured over me like a refreshing rain. The scary question from the client was something I could actually handle, once Sandy explained to me how it related to things I knew.
AR: How did you find your way to Bayesian statistics?
JW: When I was an infant I wanted to communicate with my parents, so I learned to speak their language (English). Developing the necessary skills was an inductive, Bayesian process and it worked out pretty well for me, so I continued to use Bayesian reasoning. That is to say, I was born a Bayesian, as we all are;-).
Many years later, in graduate school I took a course on Bayesian statistics taught by Don Berry and my classmates remarked that I seemed to have unusually good intuition for Bayesian methods. That plus the fact that I’ve always been a non-conformist led me to work with Don as my thesis advisor on a Bayesian project. Don’s advisor was Jimmie Savage and I read Savage’s book The Foundations of Statistics, which reinforced my belief that we should promote and use Bayesian reasoning in statistical inference. Back then MCMC was only an idea, not something one could actually use given the slow computers of the day, so Bayesian applications were pretty much limited to the Bernoulli-Beta setting and the Normal-Normal setting. Still, the appeal of being coherent was strong and, like most people, I found the use a p-values to be problematic. I was a grad student when I first heard the quote of Harold Jeffreys “What the use of P implies, therefore, is that a hypothesis that may be true may be rejected because it has not predicted observable results that have not occurred. This seems a remarkable procedure.” Remarkable indeed.
AR: Was your interest in teaching kindled while in graduate school?
JW: My interest in teaching started when I was taking 10th grade geometry and Mr. Robarge had me go to the blackboard and explain something to the class. This happened a number of times during the year and I found that I really enjoyed explaining mathematical ideas to my classmates. That’s when I set my sights on becoming a professor. I had a lot of good teachers in college and in graduate school, but it was a high school mathematics teacher handing me a piece of chalk that set me on my career path.
AR: Thank you to Mr. Robarge. Let me modify my question: Did your teaching skills and interests develop while in graduate school, or not until later?
JW: When I was in graduate school I was pretty much focused on learning and research, not on teaching. After getting my Ph.D. I took a tenure-track job at the University of Florida, but after a couple of years there my wife and I decided to move back to the Midwest. I applied for jobs at research universities and one liberal arts college: Oberlin. I fell in love with Oberlin and have been here for over 30 years. I knew that the Oberlin job would mean more time in the classroom, but it also meant teaching really good students, which is great. I knew that to get tenure at Oberlin I would need to become a better teacher, but the rewards in teaching are deeply satisfying.
Dick Scheaffer was the chair of the department at Florida and he gave a talk one day about the Quantitative Literacy (QL) project, which was just getting started under his leadership. So in my first few years at Oberlin I signed on to help with leading QL workshops at John Carroll University, which were organized by the indefatigable Jerry Moreno. I was particularly intrigued by the QL approach to teaching what a confidence interval for a proportion is: the set of all population proportions that make the observed data likely. I knew, in theory, that one can think of a CI as the inversion of a hypothesis test, but the QL approach (which used manual simulation) really brought this home.
I’ve been presenting CIs in that way ever since; and I’ve been using the Activity Based Statistics (ABS) approach to introducing hypothesis testing for many years; and just last week I used the random rectangles activity from ABS; and I could go on. My involvement with the launch of JSE started with Jackie Dietz inviting me to give a talk at NCSU on “The Best Ideas I Know for Teaching Statistics” and many of those ideas came from the QL project or related work that ended up in ABS. So it was interacting the Dick Scheaffer, Ann Watkins, Gail Burrill, Jerry Moreno, and others that reoriented me as a teacher.
AR: I suspect that “random rectangles” from ABS is the most widely used activity for teaching statistics. Please tell us about another favorite of yours from ABS, perhaps one that is not as well known. I’ll also ask you to describe an activity that you use that’s not in ABS.
JW: I like the Dueling Dice activity, where an ordinary die competes against an altered die in a series of “roll-offs” and the altered die has the advantage of having two 5 s, rather than a 5 and a 2. Students aren’t told that one die has been altered and they almost never notice. There is a big “aha” moment when the data are analyzed, with a chi-square test giving evidence that the null hypothesis (of equal dice) is false. I have some hand-made dice that I use for the activity and the fact that they are not machine-crafted perfect cubes adds to the plausibility that one die might produce larger numbers than the other.
But the reason this activity is a favorite is that it reminds me of the day in 1994 that a group of us attending ICOTS in Marrakesh took a day trip to the town of Essaouira, on the coast of Morocco; you were part of that group. Robin Lock and I found a craftsman who was selling dice that either had pips made of wood (of a different color than the wood of the die) or mother-of-pearl. When we told him what we wanted he thought we were crazy, but we convinced him, across a language barrier, that we really did want dice with two 5 s and he made each of us a few dice altered in that way. Every time I conduct that activity I am reminded of a beautiful day on the coast of Morocco with friends; plus my father was part of the group. I cherish the memory.
For something not from ABS, I’ll mention Kari Lock Morgan’s collection of data visualizations at http://www.personal.psu.edu/klm47/visualization.htm. I click on a couple of links and show my students examples, but then I have them form pairs and I turn them loose to play around, exploring the variety of ways to display data. We then share examples they have found to be interesting. We could spend several days doing this, but I limit the activity to about half of a class period.
AR: I do remember the Essaouira trip well; that was my first ICOTS and my first conference outside the U.S. Closer to home, please tell us about statistics at Oberlin—what was the state of statistics (how’s that for an awkward phrase!) at Oberlin when you arrived, and how has that changed over the years?
JW: When I arrived, in 1986, Oberlin had two versions of introductory statistics, one with a calculus prerequisite and one without, plus we had a standard 300-level course in mathematical statistics (which followed a semester of probability theory, but since probability is not statistics that course doesn’t count, the opinions of some mathematicians notwithstanding). Neither intro course made much use of computing. We’ve gone through several ups and downs since 1986, many of which I would like to forget, to get to the current state of statistics, which includes having two statisticians rather than just one.
We now have two versions of intro stat but neither requires calculus. Instead, one is a general course and the other is a biostatistics course taken mostly by biology majors, neuroscience majors, and premed students. The two courses are similar in that they meet MWF for lecture with a computer lab session on Tuesday (using R) and they include a semester-long student project.
At the 200-level we have two versions of “Stat2,” one of which follows immediately from intro stat and the other of which blends Stat1 and Stat2 into a single course that is intended for students with AP statistics background or for mathematics majors or others with strong quantitative skill. (We don’t give credit for AP statistics because the AP syllabus isn’t close enough to what we do in Stat1.) We also have a course on Bayesian methods and a Data Visualization course that my colleague Colin Dawson created. At the upper level we have math stat, plus a machine learning course that Colin created.
We don’t have a statistics major, but we have a concentration in statistics and modeling that includes courses from many departments. I haven’t kept track, but my estimate is that almost half of the concentrators have been psychology majors, with the others coming from mathematics or economics or other departments.
AR: For anyone who might be considering a career as a statistician at a liberal arts college, what would you say are the primary pros and cons of such a career?
JW: The primary pro is that you get to work with interesting undergraduates and help shape their futures. A student who chooses a liberal arts college is preparing for a life of the mind and a future that might include many jobs and adventures. It is trite, but true, when we say “We don’t train you to do something, we prepare you to do anything.” Teaching at a small school, I interact with a wide variety of faculty and students. I do consulting work for people in biology, anthropology, psychology, physics, geology, environmental studies, and other fields. The mix of questions I’m asked by faculty and by research students and honors students keeps me on my toes.
The other side of the liberal arts college coin is that I’m not around a large group of research-oriented statisticians, so I often ask friends at other schools for help when a consulting question has me puzzled. I don’t have as much week-to-week interaction with people who are thinking about statistics teaching and research questions, but the internet has made a huge difference in that area, so any sense of isolation that I had in the past has largely abated. The teaching load is higher at a liberal arts college than at a research university, but that can be offset by smaller classes of better students.
Pop Quiz
AR: Let’s start what I call the “pop quiz” portion of the interview. First, please tell us about your family.
JW: I have been married for 38 years and have two sons, one in Chicago and one in LA. I am the son of two Wisconsinites (my father died 20 years ago, right after the two of us returned from ICOTS in Singapore, but my mother is still alive). I have a brother in Wisconsin and a sister who splits time between Minnesota and Texas, based on the seasons. (You can guess which months she spends in Texas.)
AR: What are some of your hobbies?
JW: My main hobby is analyzing data—much of which I have collected while playing golf. I play a lot of golf during the summer and for each hole I record my score plus a lot of other things. I record (1) whether my drive ended up in the fairway, the left rough, or the right rough; (2) whether or not I reached the green “in regulation,” (i.e., in two shots on a par 4); (3) the length of my first putt; (4) whether that putt ended up short, long, left, right, or in the cup (so nine possible combinations of length and accuracy—think of a 3 × 3 grid); (5) if I missed the first putt, whether I missed above the hole or below the hole; (6) how many “bad swings,” if any, I had on the hole; (7) the quality of each shot, on a 1–10 scale, and the type of club used (iron, hybrid, wedge, etc.). I also record how many minutes it took to play the round, how many people I played with, and how many steps my pedometer recorded.
When I get home I enter those data into my computer and from time to time I fit models. I can tell you that for the 89 rounds that I played in 2019 my average score was 83.1 and I broke 80 a dozen times, with my best score being a 75. I hit the fairway on 649 drives, I hit 262 drives into the left rough, and I hit 252 drives into the right rough. I averaged 4.9 greens in regulation, with my chance of getting onto the green in two shots on a par 4 being 34% if I found the fairway with my drive but only 12% if I hit my drive into the rough. I averaged 32.3 putts per round (including putts taken from off of the green) and for the year I missed 297 putts above the hole and 298 putts below the hole. Using a logistic regression model, I estimate my probability of hitting a 9-foot putt as 0.34. I missed putts to the left of the hole 32% of the time and to the right of the hole 27% of the time—so maybe I need to work on how I line up my putts, since ideally those two numbers would be equal. On average it took me over 3 hr to play 18 holes, but when playing alone I needed only 178 min to complete a round versus 254 min when playing in a foursome. In an average round I took 15,520 steps, but when playing in a foursome that number was 16,779.
Some people think that I am obsessed with collecting and analyzing golf data. I think that other people have too casual an approach to the game.;-)
I am the faculty advisor to the Women’s Lacrosse team at Oberlin and I do some data analysis for them. For example, I can tell you that high shots taken by our team go in about 46% of the time but low shots (e.g., shots that bounce at the feet of the goalkeeper) go in about 58% of the time.
Other hobbies include working on jigsaw puzzles and crossword puzzles. I always put together a couple of jigsaw puzzles around Christmas, after I’ve finished my grading for the fall semester, but I don’t have much time for puzzles during the rest of the year. I play Words with Friends against my son who lives in LA, and I usually lose to him. I play the “solo” version of the game quite a bit, since this is a game that can be played in 30 sec increments of time. The algorithm for the solo game doesn’t provide very tough competition, which is why I am in the midst of a 240 game winning streak that goes back to 2017.
AR: That’s an impressive winning streak, regardless of algorithm. More impressive is that you can cite the length of the streak so precisely. What are some recent books that you have read?
JW: I know the length of the streak because I record data on each game; e.g., my highest score was 512 and my average winning margin over the past 240 games is 55.3. As for reading, I don’t find time to read a lot of books (I spend more time reading blogs, e.g., by Joe Posnanski), and I have a strong preference for nonfiction. Recent books include Steve Pinker’s Enlightenment Now: The Case for Reason, Science, Humanism, and Progress; Steven Levingston’s Kennedy and King: The President, the Pastor, and the Battle Over Civil Rights; Charles Mann’s The Wizard and the Prophet, which is “an intellectual history of the clash between techno-optimists and environmentalists, but it’s also the very personal story of two thinkers, Norman Borlaug [the wizard] and William Vogt [the prophet];” and Erika Hewitt’s The Shared Pulpit: A Sermon for Lay People. This week I started reading Elusive Utopia: The Struggle for Racial Equality in Oberlin, Ohio by Gary Kornblith and Carol Lasser, two recently retired Oberlin history professors and long-time friends.
AR: What are some of your favorite places to have traveled, and what’s next on your travel “bucket list”?
JW: I’ve been to New Zealand twice and last week I was talking with a friend who was just back from a trip there and was exclaiming about the beauty of the country; I told her that I saw Sutherland Falls from a small airplane while flying from Milford Sound back to Queenstown and that it is probably the most gorgeous thing I’ve ever seen in nature. I have loved the three safaris I’ve taken, two in South Africa and one in Kenya. It is thrilling to be a few feet away from a lion in the wild or to see part of the wildebeest migration. And it was fun to watch the sunrise from the top of Mt Fuji after hiking to the summit last summer.
My wife and I want to visit Iceland and to see the northern lights from above the arctic circle in Finland. I would be happy to do that during the summer, but she is more interested in a winter trip, which might be next up for us.
AR: Now I’ll ask a fanciful question: You can have dinner anywhere in the world with three companions, but the dinner conversation must focus on teaching statistics. Who would you invite, and where would you eat?
JW: I’m having a hard time choosing three names from a long list, but I would invite David Moore, George Cobb, and Jerry Moreno. I’ve already learned a lot from each of them, but not enough, and buying them dinner would be a small repayment for what they’ve each done to help me over the years. We would eat at Dulini River Lodge in Sabi Sand Game Reserve in South Africa, where one can gaze upon wildlife in an open-air setting while enjoying great food.
AR: That sounds great. Now another fanciful question: Suppose that you could travel in time to observe what’s going on in the world for one day. What time would you travel to—in the past or the future—any why?
JW: I am curious about the future, of course, but I think I’ll go with a trip to Nazareth, a couple thousand years back. I would try to locate a guy named Jesus and have a conversation with him (although I don’t speak a word of Aramaic, but I presume that if time travel is possible, then universal translation is as well). What are his beliefs? If someone were to come along after he died and start a new religion in his name, how would he feel? Happy? Surprised? Confused? I have many other questions.
Leftovers
AR: I neglected to ask earlier about your work as a textbook author. How did you get involved with that?
JW: My first effort was a short (122 pages) book called Data Analysis: An Introduction that I wrote because in 1992 the only statistics courses we had at Oberlin were intro stat and math stat. Students who took math stat skipped intro stat, so they learned theory but not application. I converted some notes for them into a book that presents boxplots, smoothing of time series, the regression effect, capture/recapture, Simpson’s Paradox, and other topics. Almost no one bought the book, but I’m glad that I wrote it.
A few years later I had the pleasure of working with Dick Scheaffer, Mrudulla Gnanadesikan, and Ann Watkins, plus an advisory team, on the creation of Activity-Based Statistics. We collected lots of ideas and put them into a book—and this one was actually used, quite widely. We sold almost the same number of copies of the softcover Student Guide as we did of the hardbound Instructor Resources, not the 25:1 ratio the publisher might have expected. I infer that lots of faculty did what I did: I made photocopies of pages of activities rather than having students buy the book. What matters is that lots of students benefitted from the activities, so the project was a success.
Shortly after that, Myra and Steve Samuels came to visit their daughter, Ellen, who was a creative writing major at Oberlin. I was using Myra’s Statistics for the Life Sciences in the intro biostat course I had created and I enjoyed talking to her about the book. Sadly, Myra died of cancer not long after that and her book was in danger of going out of print. I agreed to take over as author for the second edition. We are now up to the fifth edition, having made quite a few changes to the book over the years. I say “we” because your colleague Andrew Schaffner has been my coauthor for the last two editions.
I’ve written a handful of other books, most recently the second edition of STAT2: Modeling with Regression and ANOVA, always with coauthors. I like working on a team and learning from others as I write.
AR: I confess that I had forgotten about your short Data Analysis book, but now I recall that I really liked it and used it for independent study with some students when I taught at Dickinson College. Another question that I meant to ask earlier (and I have one more to come): Please tell us about your time as an academic dean at Oberlin. What appealed to you about the position, and what was most challenging?
JW: My father was a university administrator for most of his life so I always valued and respected administrative work more than some faculty do. Nonetheless, when I was first asked to become an associate dean I said no. When I was asked again, I agreed to leave the classroom for what I thought would be three years, but my stint in the dean’s office turned out to last quite a bit longer. The deanship opened up when I was an associate dean (I’ll skip the long story) and I ended up being Acting Dean of Arts and Sciences for a total of three years (with a break in the middle). So it wasn’t that I set out to be a dean; instead, circumstances led to that adventure.
Statisticians work with students and faculty in many fields—as John Tukey said, we get to play in everyone else’s sandbox—so I knew more about the scholarly lives of a variety of faculty than is true for most of my colleagues. Due to their consulting work, statisticians learn to be good problem solvers, plus we tend to handle ambiguity a bit better than some people. I recognized that not many faculty have the disposition to be a dean and few have the interest, so I felt a kind of obligation to serve the College in that capacity.
Being a dean is difficult, but it is rewarding. The most challenging part of the job was probably just the many hours per week that are required. I almost never put in 80 hr in a week, but I rarely worked fewer than 65 hr. That didn’t leave much time for reading statistics articles or doing any writing. A particularly challenging part of the job is helping faculty, and their departments, deal with health problems and family issues that arise in mid-semester. Sometimes we had to scramble to cover the courses of a professor who suddenly needed to be away. That can be quite hard, but the goodwill and creativity of faculty in difficult circumstances can be a joy to experience.
On balance, being a dean was a positive part of my career, so I am only half-joking when I say that I was happy to be paroled more than a decade ago.
AR: Another leftover question that I meant to ask earlier: I know that you served on your local school board for many years. What motivated you to pursue that, and what did you learn about education from that experience?
JW: Well, a bunch of us were not happy with the Superintendent. We would go to School Board meetings and complain about how things were being done and make suggestions, but nothing changed. I figured that if I’m going to complain then I should be willing to work to make things better, so I ran for a seat on the Board, along with two friends. All three of us were elected, as a kind of team.
I spent a total of eight years on the Board (two years as President) and that time was filled with highs and lows—but somehow I remember the lows more clearly than the highs. I learned many things, including that I would not be a good principal or superintendent. It is one thing to lead college faculty and another thing to lead K-12 teachers. The way that faculty think about their roles within the world of education is different from how many K-12 teachers approach things.
Conclusions
AR: Before I ask my final two questions, let me ask if there is anything that you wish I had asked but haven’t.
JW: Given your penchant for the whimsical, I thought you might ask about acronyms. I remember the meeting at Ohio State that launched CAUSE, before it had a name. The meeting broke into groups to work on various tasks and you and I ended up as partners who finished our work early. We used the extra time to think about a name and we came up with Consortium to Advance Undergraduate Statistics Education, which we agreed was a worthy cause. Earlier I had come up with the name Science Education and Quantitative Literacy for a project that was a sequel to the Quantitative Literacy project. Later I was part of the group that worked on statistics guidelines and thought of calling the report Guidelines for Assessment and Instruction in Statistics Education, so that people gazing into the future might remember where to look for guidance. It seems that while some people are thinking deeply about serious matters, my mind wanders off into a mnemonic playground.
AR: Among your many accomplishments in statistics education, of which are you most proud?
JW: I’m going to point to the Isolated Statisticians. “Proud” and “accomplishment” aren’t really the right words here, but I’m probably happier about the development of this group than anything else. Back in 1991 Don Bentley and I put a note on the (physical) message board at JSM in Atlanta, inviting isolated statistics educators to meet us for an informal discussion one afternoon, in a room that we had reserved. I think there were 7 of us at that first meeting, and I’m not sure that everyone had an email address. Today there are 429 of us on the isostat listserv. We have a meeting each summer at JSM that doesn’t draw large numbers, but the listserv is fairly active. Despite the ubiquity of blogs and other internet resources, and despite many small colleges now having two or more statisticians (we have a very relaxed definition of “isolated”), the isostat listserv continues to be a useful resource for a lot of people. (If any statistics educator, at any size of school, with any number of local colleagues, wants to be added to the group they can write to me at [email protected].)
AR: I agree that the “isolated statisticians” group has been a very important contribution to the careers of many statistics teachers. I have greatly benefitted myself from that group.
Thanks very much for taking the time to answer all of these questions and for taking on the JSE editorship. My final question is: What advice do you have for JSE readers who are near the beginning of their careers in statistics education?
JW: Be prepared for change, and speak up when you see something that needs to change. Basically, I’m encouraging the reader to be a non-conformist. It is easier to go along with what the majority likes, and often something is a majority opinion because lots of smart people have looked at a situation and have reached the same conclusion about what to do. But sometimes we just repeat what was done in the past, not because it is best but because it is familiar.
For example, several years ago a few of us started a campaign to replace “assumption” with “condition” in the statistical lexicon, and I’ve seen some progress on this front, although I still see the word “assumption” being used. A mathematician might assume something at the start of a proof, but a statistician should never assume that the population is normal, for example. A t-test is valid if, among other things, the population is normal. We can investigate the normality condition, so let’s do that and let’s not call normality an assumption, as if we could simply assume it to be true. If we use proper language we are more likely to help our students think clearly. And in case there is a data scientist reading this (don’t ask me what a data scientist is), I’ll add that we should think about whether we have a random sample, which is a much more important condition than normality.
So my advice is to question, challenge, speak up, promote change, and continue to learn. But that’s advice on what to do and the more important advice might be about how to do it, so I’ll finish with one more quote, this one from Mark Patinkin: “Don’t chase success. If you chase excellence first, success will chase you.”
Additional information
Notes on contributors
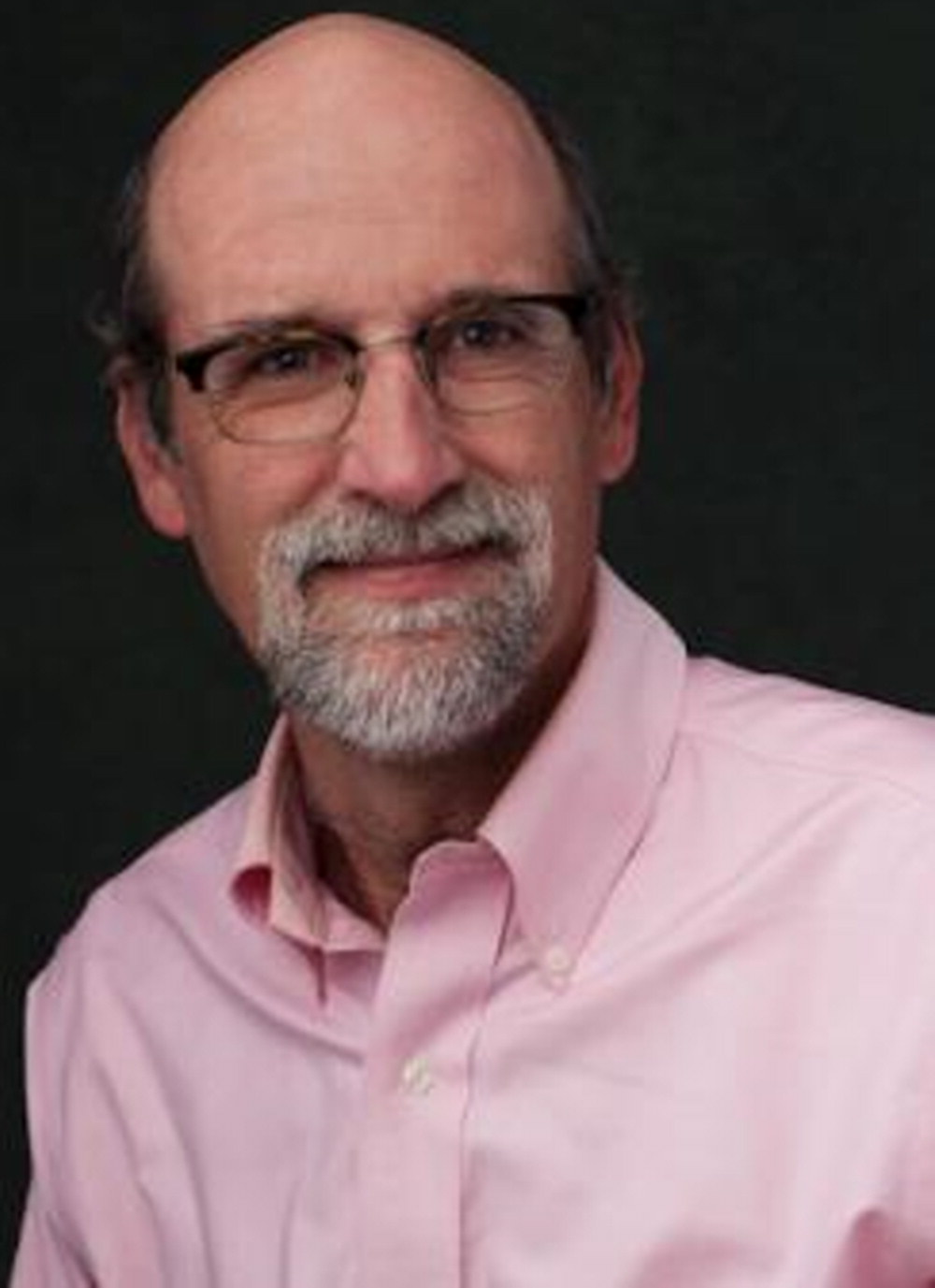
Jeff Witmer
Jeff Witmer is Professor of Mathematics at Oberlin College. He is a fellow of the American Statistical Association and editor of the Journal of Statistics Education. This interview took place via email on January 2–March 24, 2019.