ABSTRACT
Objective
To investigate the usefulness of inertial measurement units (IMUs) in the assessment of motor function of the upper limb (UL) in accordance with the international classification of functioning (ICF).
Data sources
PubMed; Scopus; Embase; WoS and PEDro databases were searched from inception to 1 February 2022.
Methods
The current systematic review follows PRISMA recommendations. Articles including IMU assessment of UL in stroke individuals have been included and divided into four ICF categories (b710, b735, b760, d445). We used correlation meta-analysis to pool the Fisher Z-score of each correlation between kinematics and clinical assessment.
Results
A total of 35 articles, involving 475 patients, met the inclusion criteria. In the included studies, IMUs have been employed to assess the mobility of joint functions (n = 6), muscle tone functions (n = 4), control of voluntary movement functions (n = 15), and hand and arm use (n = 15). A significant correlation was found in overall meta-analysis based on 10 studies, involving 213 subjects: (r = 0.69) (95% CI: 0.69/0.98; p < 0.001) as in the d445 (r = 0.71) and b760 (r = 0.64) ICF domains, with no heterogeneity across the studies.
Conclusion
The literature supports the integration of IMUs and conventional clinical assessment in functional evaluation of the UL after a stroke. The use of a limited number of wearable sensors can provide additional kinematic features of UL in all investigated ICF domains, especially in the ADL tasks when a strong correlation with clinical evaluation was found.
Introduction
Stroke is one of the leading causes of disability worldwide and can result in permanent motor impairment in 80% of cases.Citation1,Citation2 Up to 85% of patients show an initial deficit in the upper limb (UL) and problems remain, 3–6 months later in 55% to 75% of patients.Citation3 Common manifestations of UL motor impairment include muscle weakness or contracture, changes in muscle tone, joint laxity, and impaired motor control. These impairments induce disabilities in common activities such as reaching, picking up objects, and holding onto objectsCitation4 and consequently affect the ability to perform activities of daily livingCitation5 and quality of life.Citation6,Citation7 Despite the importance of UL motor recovery, in clinical and experimental settings, evaluation methods are subjectively scored by clinicians, and the assessment results vary individuallyCitation8 and present lack of sensitivity to subtle changes in motor performance throughout the rehabilitation process.Citation9 Understanding UL impairments is principally complex for two reasons: 1) the impairments are not static and change during time due to motor recovery and 2) multiple impairments may be observed simultaneously, i.e. a patient may present with weakness of the arm and hand immediately after a stroke, which may not have resolved when spasticity sets in a few weeks or months later, resulting in a chronic impairment.Citation10 In these chronic conditions, there is the need to apply a biopsychological model that considers the illness and health such as the result of an interaction between biological, psychological, and social factors.Citation11 above concept is the pillar of the World Health Organization’s International Classification of Functioning Disability and Health (ICF) that aims to provide a comprehensive, universal, and international classification of health, health-related domains, and a list of environmental factors via a schematic coding scheme. This model tries to improve communication between health care workers, researchers, policy makers, and the public including people with disability and data comparison across countries. In this respect, this improved communication only slightly involved the assessment of motor function. In fact, while numerous measures are readily available for the evaluation of UL function, no single measure is available to encapsulate the entire range of activities performed by the UL according to the ICF.Citation12 The Fugl-Meyer Assessment scale (FMA) is the most commonly used measure for UL evaluation after a stroke.Citation13 Moreover, with respect to the ICF, the FMA only deals with the body function domain.Citation9 For this reason, more than one measurement scale is often used for evaluation in about 70% of studies (i.e., FMA-WMFT).Citation13 Nevertheless, in this multiple outcomes assessment, only a part of ICF domains is covered (body function and activity).Citation9,Citation13 Moreover, relatively few studies have applied the ICF model to identify the contributions of specific UL impairments, such as muscular weakness, pain, and sensory loss, as predictors of activity and participation.Citation14
To improve the accuracy of impairment assessment of UL, instrumental evaluation can be coupled with clinical assessment or clinical scales and tests can be sensorised. In this perspective, wearable sensors are useful, noninvasive, low-cost, and objective tools that are being extensively used to assess motor and functional impairment in many neurological diseases.Citation15–17 In the last decade, the use of inertial motion units (IMUs), a subclass of wearable sensors, for clinical purposes has moved from laboratory to ambulatory-based settings. More recently, we are witnessing a transition to unsupervised real-world environments thanks to their affordability, unobtrusiveness, and higher ecological validity with respect to conventional motion analysis.Citation18,Citation19 Several fields of medicine, like orthopedic, neurological, physical medicine, and rehabilitation and occupational, have benefited from wearable IMUs for the assessment of the residual motor function both for the planning of the intervention and the assessment of the efficacy of the treatment over time.Citation20,Citation21 In particular, research on clinical movement analysis in the past 5 years has focused on the use of IMUs for the assessment of neurological gait and balance disorders,Citation22 orthopedic gait disorders,Citation23 and neurological and orthopedic disorders of the upper limb in standardized laboratory or clinical settings.Citation24 While all this has led to significant benefits, the vast amounts of data generated by these sensors carry issues related to privacy and to the extraction of clinically relevant and interpretable information by practitioners, which may discourage their use in the clinical practice.Citation19 A recent review suggests that IMUs can be practical options to assess motor function during the execution of activities of daily life, as well as tracking of complex upper limb movements,Citation25 even though this aspect is not fully recognized in clinical practice, and a better understanding is necessary to guide future clinical applications of wearable technology.Citation26 Similarly, the effectiveness of wearable technologies for the treatment of stroke needs further investigations to solve the unsatisfactory sample sizes and the lack in the methodological approaches of studies currently available.Citation27
The aim of the present review is to evaluate the usefulness of IMUs for the kinematics assessment of specific functions of the UL (in accordance with the ICF) in patients with stroke. Specifically, we reported an overview of kinematics variables and provided a first quantification of their correlation with clinical evaluation through meta-analysis.
Methods
The current systematic review was performed following Preferred Reporting Items for Systematic Reviews and Meta-Analyses (PRISMA) recommendationsCitation28 and was registered in the PROSPERO database (CRD42021240847). We try to respond to the following research questions: Can IMUs objectively evaluate UL motor functions in clinical settings in people with stroke? Is there an association between IMUs assessment and clinical evaluation?
A literature search of multiple electronic databases (PubMed; Scopus; Embase; WoS and PEDro) was conducted from inception to 1 February 2022. We combined Mesh Terms and free-terms as keywords “(wearable sensors) or (inertial sensors) or (accelerometers) or (sensor) or (IMUs) AND (upper limb) AND ((neurological disease) or (stroke)) AND (kinematics)” (Complete search strategy is available in Appendix A). We selected articles that meet the following inclusion criteria: 1) stroke population; 2) upper extremity assessment through inertial measurement units; 3) English language. Exclusion criteria were: 1) other neurological disease; 2) other wearable sensors such as chemical, optical, and electrical sensors; 3) methodological study design (not involving humans); 4) gray literature.
All results have been uploaded to an online database and screened simultaneously and independently by three reviewers (AB, AMC, and PP). At the end of the process, in the event of no agreement, a fourth reviewer (GV) was consulted.
ICF classification
The ICF includes several domains that are organized in a hierarchical tree structure. The first classification level defines two categories: “functioning and disability” and “contextual factors.” Functioning and disability subheading including “body functions and structures” and “activities and participation.” In the first subheading, we included the following domains: b710, mobility of joint functions; b735, muscle tone functions; and b760, control of voluntary movement functions. In the second subheading, we included the d445, hand and arm use domain. Further ICF’s subheading such as b730, muscle power functions; b740, muscle endurance functions; d440, and fine hand use were excluded because they were not directly investigable via IMUs. All selected studies were labeled with an ICF subheading according to the outcome goals: the relevant assignment criterion is reported in . The studies that reported data across two or more domains were labeled consistently (the complete allocation is available in Appendix B).
Table 1. Selected domains of the World Health Organization’s International Classification of Functioning Disability and Health (ICF).
Kinematics parameters
For each ICF domain, we investigated the use of IMU-based indices such as spatiotemporal parameters and global features (i.e., smoothness, symmetry, and spectral parameters) of UL movements (). The information on signal processing procedures is available in the fifth column of each synoptic table (). In the above-mentioned tables, we reported when the variable of interest has been estimated from the three-dimensional (3D) orientation of the sensor case (eventually mediated by a kinematic model). We also reported if sensor orientation has been computed using a sensor fusion algorithm either based on accelerometer, gyroscope, and magnetometer data (i.e., 9-axis) or on accelerometer and gyroscope only data (i.e., 6-axis). In the case of a custom-made sensor device, the sensor fusion algorithm is based on algorithms designed in previous studies.
Figure 1. Schematic representation of spatio-temporal and kinematic parameters recorded through inertial measurement units. The investigated spatio-temporal and kinematics variables are reported into the white boxes.
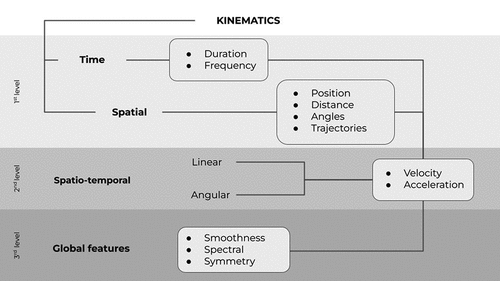
Table 2. Synoptic table of studies included in the passive range of motion assessment (b710) of the ICF domain.
Table 3. Synoptic table of studies included in the muscle tone functions (b735) of the ICF domain.
Table 4. Synoptic table of studies included in the voluntary movements assessment (b760) of the ICF domain.
Table 5. Synoptic table of studies included in the object manipulation and daily life activity assessment (d445) of the ICF domain.
Statistical analysis
All studies that reported a correlation between clinical assessment and IMU parameters in stroke population (linear or not linear) have been included in the meta-analysis. For the studies that reported more than one significant correlation result, we calculated the mean of the significant correlation coefficients. The correlation coefficient of each study has been transformed into the Fisher Z-score with the appropriate formula (linear or not linear). We pooled each Z-score and sample size with a random-effect model meta-analysis with a 95% of confidence interval (IC). Then, a reverse formula was used to transform the Z-score into an r value (rounded down). We used the following ranges for the interpretation of correlation coefficient: 0–0.10 negligible; 0.10–0.39 weak; 0.40–0.69 moderate; 0.70–0.89 strong; 0.90–1 very strong.Citation49 Heterogeneity between studies was assessed by computing the Q-statistics and I2 index. Substantial statistical heterogeneity was assumed if the Q-statistic was significant (p-value < 0.05) and I2 was higher by 75%. Characterization of variances across studies were calculated as no heterogeneity, low heterogeneity, moderate heterogeneity, and high heterogeneity, respectively, for the I2 values<25%, 25% to 50%, 50% to 75%, and >75%. To investigate publication bias, we performed the Egger’s regression test and the Begg-Mazumdar’s test.Citation50 In addition, subgroup analysis has been performed with respect to ICF categories.
Results
A total of 711 articles were found. After the duplicate removal (137), 574 articles were screened. After screening of titles and abstracts, 530 articles have been excluded, because they do not meet the inclusion criteria. A total of 44 full-text articles have been examined. Eight studies have been excluded during full-text check, in conclusion, 35 articles have been included in the systematic review (flow chart of studies screening is available in ). The included studies have been divided, in one or more than one domain, with respect to preview ICF classification. IMUs have been employed to assess passive range of motion (ROM) (b710/n = 6), spasticity (b735/n = 4), motor control (b760/n = 15), and ability in performing activities of daily living (ADL) (d445/n = 15) (the complete allocation is available in Appendix B). TenCitation33,Citation36,Citation37,Citation42,Citation44,Citation46–48,Citation51,Citation52 out of 35 studies investigated the kinematics variables in both ULs applying IMUs on affected and unaffected side.
Figure 2. Flow diagram of studies selection process (PRISMA ver. 2020).Citation28 *All records were excluded by humans.
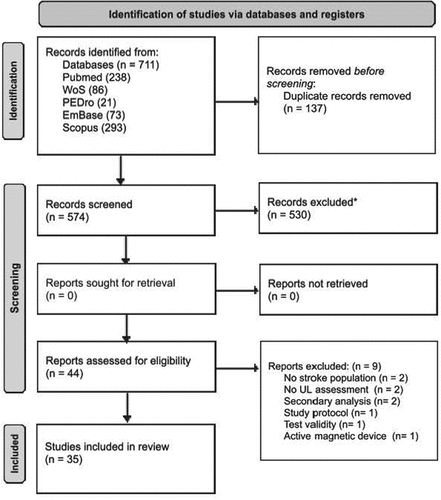
Passive range of motion assessment (b710)
Seven studies have assessed ROM through IMUs in a sample of 74 patients,Citation29–34 with a range of IMUs’ number from twoCitation30 to seven.Citation32
The ROM test was performed in three different ways: active ROM (AROM),Citation29,Citation33,Citation34 passive ROM,Citation31,Citation32 and robot-assisted ROM.Citation30
The most used kinematic variables were the joint angles of shoulder, elbow, wrist, and fingers either in a single plane or in three dimensions (3D). The most investigated tasks were single shoulder and elbow flexion-extension. Linear trajectories and velocity of anatomical landmarks as well as UL’s smoothness have also been investigated in a limited number of studies.
Muscle tone functions (b735)
Regarding the proposed ICF subdivision, spasticity is the least investigated domain through IMUs.
Four studies have used IMUs to assess muscle tone impairment on 82 stroke patients.37–40.
The number of IMUs ranged from oneCitation53 to three.Citation54,Citation55 Three studiesCitation53,Citation54,Citation56 performed a passive stretch reflex of the elbow flexors; additionally, Kim and colleagues (2020)Citation53 tested the elbow extensors; and Ang and colleagues (2018)Citation54 also tested shoulder, wrist, and thumb. Chen et al., 2021Citation55 performed a multimodal acquisition combining an IMU sensor with a surface EMG system (Ultium™ Biomechanics System, Noraxon Ltd, USA) during a guided flexion extension of the elbow.
Two studies used the Modified Ashworth Scale as clinical assessment,Citation53,Citation54 and one of these performed a correlation analysis with kinematics data,Citation54 the other study used the Tardieu scale.Citation56
The choice of the kinematic variable was heterogeneous among the selected studies. In particular, angular velocity and acceleration were used to assess the spasticity in two studies,Citation53,Citation54 while elbow flexion-extension ROM was used in the study of Paulis and colleagues (2011).Citation56
Voluntary movements assessment (b760)
Fifteen studies used IMUs to assess voluntary movements on 218 stroke patientsCitation29,Citation30,Citation37,Citation44,Citation48,Citation51,Citation52,Citation57–64 with a number of IMUs’ ranging from oneCitation64 to fourteen.Citation37
A high heterogeneity with respect to the required tasks was found across studies. Generally, reaching tasks or clinical tests that mimic reaching and grasping tasks have been administered. Two studies used non-immersive virtual reality with exergaming.Citation29,Citation64
Regarding the required tasks, several kinematics variables were chosen to evaluate the voluntary movements. The most recurrent variables were timing, position, velocity (linear), acceleration, and smoothness. Additionally, angles, symmetry, and spectral analysis have also been investigated.
Object manipulation and daily life activity assessment (d445)
Fifteen studies have used IMUs to assess object manipulation or activity of daily life tasks on 154 stroke patients,Citation30,Citation35–48 with a number of IMUs’ ranging from oneCitation35,Citation41 to seventeen.Citation46
Kinematic investigation during daily life tasks took into consideration a diverse number of variables. Despite the high heterogeneity in the choice of the observed variables, some parameters are more recurrent in many studies. Specifically, the number of repetitions, joint ROM, and the symmetry of movement (with respect to healthy subjects or with respect to the contralateral limb) were the most frequent kinematics variables selected. Secondly, the position, acceleration, and smoothness of the movements were investigated in about one-third of the selected studies. Additionally, trunk stability, trunk compensation, and spectral analysis have been investigated.
Clinical vs kinematics correlation meta-analysis
A total of 10 studies were included in the quantitative analysis of the correlation between IMUs parameters and clinical assessment, and the total sample size was 213. Six out of the ten studies included in the meta-analysis investigate the correlation of kinematics with the Fugl-Meyer Assessment (FMA) scale,Citation34,Citation44,Citation46,Citation57,Citation60,Citation64 three studies with the Action Research Arm Test (ARAT),Citation36,Citation42,Citation57 two with the Modified Ashworth Scale (MAS),Citation30,Citation54 and one with the Modified Barthel Index (MBI).Citation57 Meta analysis shows an overall Fisher Z-score of 0.83 (95% CI: 0.69/0.98; p < 0.001), Fisher Z-transformation (r 0.69) indicating a moderate correlation (). With no heterogeneity across the studies (Q-s = 6.7; p = 0.7; I2 = 0). Risk of bias assessed with Egger’s test (t = 3.23, p < 0.05) and Begg-Mazumdar’s test (t = 1.25, p = 0.2) indicated that there was a potential publication bias (Funnel plot in Appendix C). Subgroup analysis for ICF d445 domain performed on 51 stroke patients, showed an overall Fisher Z-score of 0.88 (95% CI: 0.58/1.18; p < 0.001), Fisher Z-transformation (r = 0.71) indicating a strong correlation. With no heterogeneity across the studies (Q-s = 0.87; p = 0.6; I2 = 0). Risk of bias assessed with Egger’s test (t = 1.41, p = 0.4) and Begg-Mazumdar’s test (t = 0.52, p = 0.6) indicated that there was not a potential publication bias. In the subgroup meta-analysis for ICF b760 domain performed on 107 stroke patients, show an overall Fisher Z-score of 0.76 (95% CI: 0.56/0.96; p < 0.001), Fisher Z-transformation (r = 0.64) indicating a moderate correlation. With no heterogeneity across the studies (Q-s = 0.14; p = 0.98; I2 = 0). Risk of bias assessed with Egger’s test (t = −0.5, p = 0.3) and Begg-Mazumdar’s test (t = −2.04, p = 0.04) indicated that there was a potential publication bias. The subgroup meta-analysis for ICF b710 domain performed on 40 stroke patients shows an overall Fisher Z-score of 1.28 (95% CI: −0.03/2.59; p < 0.056), Fisher Z-transformation (r = 0.85) indicating a strong correlation. With high heterogeneity across the studies (Q-s = 3.59; p = 0.058; I2 = 72).
Figure 3. Forest plot for the random effect model correlation meta-analysis between kinematics and clinical data. The continuous line indicates no correlation (right and left, positive and negative correlation, respectively). The dashed line indicates the pooled Z-score. ES: effect size; CI: confidence interval; W: weight; V: variance; Sig.: statistical significance; N: sample number. StudiesCitation30,Citation44 allocated to more than one ICF’ subgroups were considered for meta-analysis only in their dominant subgroup.
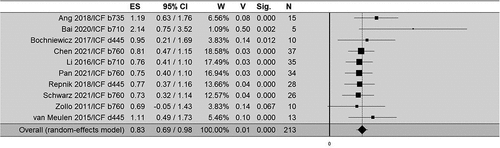
Discussion
The aim of the present systematic review was to give an overview of the use of wearable IMUs for the kinematic assessment of clinical features of the UL in accordance with the ICF in patients with stroke. The literature suggests that a limited number of sensors are functional in obtaining kinematic information on the functional activity of the upper limb in a simple and safe way. The use of IMUs alone or in association with other clinical or instrumental assessment tools has been used to collect objective data on the functions of the UL. The positive correlations with other instruments’ acquisition and clinical tests, and the high precision in the test-retest and intra-test reliability, promote the adoption of wearables as appropriate solutions to assess UL functions, especially in the assessment of activity of daily living.
Regarding the assessment of the range of motion (ICF – b710), kinematic variables such as 2D and 3D angles are the most utilized to describe the movements of UL joints. Consistent with the evaluation aim, the angles recorded during passive, active, or robot-assisted movements provide useful information regarding the ROM performed. In this respect, even a limited number of sensors can be enough to assess this function. Trajectory, smoothness, and symmetry can be investigated to expose additional information about the quality of ROM. The scientific literature suggests some useful indications: (i) 3D sensor orientation estimated by a 9-axis can be used to calculate the joints’ angles during passive or active mobilization; (ii) IMUs can detect the compensation during movement execution (i.e. trunk compensation); (iii) considering the individual variability of joints’ angles, the assessment of both ULs through IMUs can provide a symmetry index between affected and unaffected side defining a tailored ROM measure.
Muscle tone (ICF – b735) of the UL is the least common aspect investigated via IMUs with heterogeneity in the protocols and kinematics variables selected. Clinically, spasticity is defined as a motor disorder characterized by a velocity-dependent increase in the tonic stretch reflexCitation65 often difficult to clinically evaluate. Therefore, it is acknowledged that quantified, instrumented methods should be used to provide a more accurate and valid assessment of spasticity.Citation66 In this respect, biomechanical and electrophysiological methods (i.e., isokinetic dynamometers) have been investigated as potential instruments, but none of these techniques provide an easy and reliable assessment of spasticity for the use in the clinical routine.Citation67 Interestingly, our investigation shows that angular velocity and accelerations recorded via IMUs during rapid mobilization of UL were fruitfully adopted. Three sensors, at most, are sufficient to measure spasticity in a joint target (i.e., the elbow). This information can support the use of wearables to objectively quantify spasticity on which assessment there are still many limits. To summarize, spasticity is often clinically hard to quantify and present a lower inter-rater reliability. Although limited, scientific literature reports encouraging suggestions about the use of IMUs in the assessment of spasticity: (i) angular displacement (velocity and acceleration) is the suitable kinematic variable recordable via 3D sensor orientation estimated by a 9-axis; (ii) an excellent inter-rater reliability was observed in kinematics investigations with respect to the fair result in clinical inter-rater reliability.
Voluntary movements assessment (ICF – b760) is, with the ADLs, the most investigated function of UL, and they share some similarities. In the included studies in this domain was observed a high heterogeneity of variables and protocols. Such movements are typically assessed during clinical tests or integrated in more complex setups, eventually including robot-aided therapiesCitation64 or exergaming.Citation51 The kinematic data recorded during clinical evaluation showed a high statistical correlation with clinical score, favoring the increasing acceptability of wearable IMUs among clinical personnel. Noteworthy, IMU-based scores may provide additional information about the change in movement quality that clinical tools do not detect.Citation51 In different application contexts, either rehabilitation or home-monitoring,Citation61 a plethora of IMU-based parameters were fruitfully adopted to support clinical assessment and decision-making. In the context of voluntary movements, more than in other ICF categories, many global parameters have been proposed, such as spectral parameters, somewhere highlighted as better informative than traditional temporal or kinematics parameters. In brief, the assessment of voluntary movements via IMUs present high heterogeneity, moreover the literature provides useful information: (i) the investigation of voluntary movement was recurrently performed via instrumentation of clinical test (i.e. Mingazzini, finger-to-nose test); (ii) some IMUs variables (i.e. smoothness or trajectories) can reveal information about movement quality that cannot be recorded with timed or quantitative tests.
As mentioned above, the ability to perform activities of daily living and the manipulation of objects (ICF – d445) is one of the most investigated functions of UL. These skills are, in general, the elective goal of rehabilitation.Citation68 In fact, the capacity to reach and keep in mouth or explore the surroundings can be considered the hegemonic function of UL. Probably, for this reason, ADL skills were investigated more frequently in comparison to the other functions. Moreover, the improvement in the ability to perform ADL can be considered as the result of the recovery in the other skills, such as motor control, range of motion and muscle tone. The investigation of ADL skills can be useful when a comprehensive evaluation of UL is needed, without focusing on the subcomponents of movement. While it is recognized that the ADLs are complex tasks, the selected studies proposed to characterize them using simple kinematic scores such as the number of repetitions, joint angles, and symmetry (with respect to the healthy subjects or contralesional side). This information can be useful to quantify the use of impaired limb with respect to the non-lesioned limb, to evaluate the volume of space explored, and to assess the quality and quantity of movement during all-day life. David and colleagues (2021)Citation68 synthesized the UL functions during ADL in four aspects: (a) amount of use (duration and/or intensity), (b) hand preference, (c) type of task, and (d) quality of movement. As a result of the present systematic review, the most recurrent kinematics variables cited above can provide a satisfactory response to the main questions of investigation. Noteworthy, additional global features (such as smoothness) are also considered to investigate specific aspects of UL function. Quantitative analysis shows a strong correlation between kinematics variables and clinical assessment (r = 0.71 p < 0.001) recorded during activities of daily living tasks. The homogeneity across the studies and the low risk of bias indicate that wearables provide an objective measure with excellent compliance with clinical observations. To conclude, concerning the IMUs assessment of ADL, emerging evidence suggests: (i) kinematics variables establish high correlation with clinical assessment of every-day activities; (ii) simple and complex kinematics can be successfully used to evaluate linear or spatial components and global features, respectively; (iii) the investigation of both ULs can provide information about symmetry during movement sharing in bimanual tasks; (iv) IMUs can be used to quantify the UL use during daily life activities in their home.
Advantages
The use of IMUs allows us not only to objectively evaluate movement, ROM, spasticity, and ability in ADL skills but also to obtain information that is often not identified with the common clinical tests. Some parameters of global kinematics can broaden and/or deepen the evaluation of the functional capacity of the UL, often easily linked to the clinical observation of the clinical signs such as movement fluidity or symmetry. As shown for the lower limb, IMUs can provide clinically relevant data on movement quality in addition to the traditional outcome.Citation69
The assumption that clinical performance is equivalent to real-world performance may not be true and new technologies are needed to objectively measure real-world activity.Citation70 In this respect, IMU technology represents an added value in terms of evaluation of the UL functions in the real world, having an impact in measuring free-living function in a more objective, realistic, and ecological way.Citation71
The increased availability of inexpensive commercial devices might influence the clinical decision-making process.Citation12 In this respect, machine learning approaches could be used to detect specific characterization of UL movements to adapt treatments to subjects’ disabilities. Specifically, these algorithms on a large kinematics dataset can be effective to identify patterns of activity recognition, movement classification, or clinical assessment emulation in stroke patients.Citation72
Limitations and future perspectives
As a limitation of the study, less than a third of the studies performed a both-side IMUs detection, limiting the evaluation capacity of bimanual activities, especially in the ADL tasks. Moreover, in the literature are not provided the sensitivity of kinematics measures in the differentiation of minimal clinical difference. Finally, the current number of papers on the topic did not support a thorough meta-analysis especially when considering the papers falling in specific ICF domains. Noteworthy, the preliminary correlation meta-analysis clearly supported our conclusions , highlighting the increasing interest in using IMU technology to perform the functional assessment in and outside the clinical environment. As a matter of fact, functional UL evaluation with IMUs does not simply improve standard clinical evaluations, but radically changes their paradigm, providing information on functional domains and on subjects’ motor behavior not previously explored. This powerful possibility opens the chance to deliver rehabilitation in different settings (home, inpatient, and outpatient), to measure treatment content (amount, quality, and efficacy of the movement) and to better personalize rehabilitation programs. In the future, the amount of data recognized from the IMUs might offer a unique opportunity to improve the continuum of therapy from hospital to home environment encoding single and bimanual ADLs tasks.
Conclusion
The literature provides information about the use of IMUs in all four investigated ICF domains. The results of the present review show that kinematics assessments by IMUs provide additional data (i.e., global features) on motor function, muscle tone, range of motion, and ability to perform the activity of daily living in a clinical or ecological setting. The most investigated domain is the functional capacity of the upper limb during the movements of daily activities. A limited number of IMUs devices are sufficient to provide useful information on UL performance. The strong correlation between clinical and kinematics variables supports the choice of IMUs to objectively evaluate UL movement. Indeed, IMUs can increase the knowledge on the real use of the UL during activities of daily life in the home-setting.
Author contributions
AMC and GV contributed to the conception of the study. AMC, PP, AB, and GV contributed to data collection. AMC carried out the data analysis. AMC and GV wrote the first draft of the manuscript. GM and GK revised the manuscript. All the authors reviewed and approved the final version of the manuscript.
Disclosure statement
No potential conflict of interest was reported by the authors.
Additional information
Funding
References
- Feigin VL, Norrving B, Mensah GA. Global burden of stroke. Circ Res. 2017;120(3):439–448. doi:10.1161/CIRCRESAHA.116.308413.
- Bernhardt J, Hayward KS, Kwakkel G, et al. Agreed definitions and a shared vision for new standards in stroke recovery research: the stroke recovery and rehabilitation roundtable taskforce. Neurorehabil Neural Repair. 2017;31(9):793–799. doi:10.1177/1545968317732668.
- Feys HM, De Weerdt WJ, Selz BE, et al. Effect of a therapeutic intervention for the hemiplegic upper limb in the acute phase after stroke: a single-blind, randomized, controlled multicenter trial. Stroke. Apr 1998;29(4):78. doi:10.1161/01.STR.29.4.785.
- Hatem SM, Saussez G, Della Faille M, et al. Rehabilitation of motor function after stroke: a multiple systematic review focused on techniques to stimulate upper extremity recovery. Front Hum Neurosci. 2016;10:442. 5-92. Published 2016 Sep 13. 10.3389/fnhum.2016.00442
- Rafsten L, Meirelles C, Danielsson A, Sunnerhagen KS. Impaired motor function in the affected arm predicts impaired postural balance after stroke: a cross sectional study. Front Neurol. 2019;10:912. Published 2019 Aug 21. doi:10.3389/fneur.2019.00912.
- Lieshout ECCV, van de Port IG, Dijkhuizen RM, Visser-Meily JMA. Does upper limb strength play a prominent role in health-related quality of life in stroke patients discharged from inpatient rehabilitation? Top Stroke Rehabil. Oct, 2020;27(7):525–533. doi:10.1080/10749357.2020.1738662.
- Martino Cinnera A, Bonnì S, Pellicciari MC, Giorgi F, Caltagirone C, Koch G. Health-related quality of life (HRQoL) after stroke: positive relationship between lower extremity and balance recovery. Top Stroke Rehabil. Oct, 2020;27(7):534–540. doi:10.1080/10749357.2020.1726070.
- Li HT, Huang JJ, Pan CW, Chi HI, Pan MC. Inertial sensing based assessment methods to quantify the effectiveness of post-stroke rehabilitation. Sensors (Basel). 2015;15(7):16196–16209. doi:10.3390/s150716196.
- Longhi M, Merlo A, Prati P, Giacobbi M, Mazzoli D. Instrumental indices for upper limb function assessment in stroke patients: a validation study. J Neuroeng Rehabil. 2016 Jun 8;13(1):52. doi:10.1186/s12984-016-0163-4.
- Raghavan P. Upper limb motor impairment after stroke. Phys Med Rehabil Clin N Am. 2015;26(4):599–610. doi:10.1016/j.pmr.2015.06.008.
- Wade DT, Halligan PW. The biopsychosocial model of illness: a model whose time has come. Clin Rehabil. Aug, 2017;31(8):995–1004. doi:10.1177/0269215517709890.
- Lang CE, Bland MD, Bailey RR, Schaefer SY, Birkenmeier RL. Assessment of upper extremity impairment, function, and activity after stroke: foundations for clinical decision making. J Hand Ther. Apr-Jun, 2013;26(2):104–115. doi:10.1016/j.jht.2012.06.005.
- Santisteban L, Térémetz M, Bleton JP, Baron JC, Maier MA, Lindberg PG. Upper limb outcome measures used in stroke rehabilitation studies: a systematic literature review. PLoS One. 2016 May 6;11(5):e0154792. doi:10.1371/journal.pone.0154792.
- Faria-Fortini I, Michaelsen SM, Cassiano JG, Teixeira-Salmela LF. Upper extremity function in stroke subjects: relationships between the international classification of functioning, disability, and health domains. J Hand Ther. Jul-Sep, 2011;24(3):257–265. doi:10.1016/j.jht.2011.01.002.
- Brognara L, Palumbo P, Grimm B, Palmerini L. Assessing gait in Parkinson’s disease using wearable motion sensors: a systematic review. Diseases. 2019 Feb 5;7(1):18. doi:10.3390/diseases7010018.
- Johansson D, Malmgren K, Alt Murphy M. Wearable sensors for clinical applications in epilepsy, Parkinson’s disease, and stroke: a mixed-methods systematic review. J Neurol. Aug, 2018;265(8):1740–1752. doi:10.1007/s00415-018-8786-y.
- Summa A, Vannozzi G, Bergamini E, Iosa M, Morelli D, Cappozzo A. Multilevel upper body movement control during gait in children with cerebral Palsy. PLoS One. 2016 Mar 21;11(3):e0151792. doi:10.1371/journal.pone.0151792.
- Iosa M, Picerno P, Paolucci S, Morone G. Wearable inertial sensors for human movement analysis. Expert Rev Med Devices. Jul, 2016;13(7):641–659. doi:10.1080/17434440.2016.1198694.
- Picerno P, Iosa M, D’souza C, Benedetti MG, Paolucci S, Morone G. Wearable inertial sensors for human movement analysis: a five-year update. Expert Rev Med Devices. Oct, 2021;12(sup1):1–16. doi:10.1080/17434440.2021.1988849.
- Iosa M, De Sanctis M, Summa A, Bergamini E, Morelli D, Vannozzi G. Usefulness of magnetoinertial wearable devices in neurorehabilitation of children with cerebral palsy. Appl Bionics Biomech. 2018; 2018:5405680. 10.1155/2018/5405680.
- Tramontano M, Bergamini E, Iosa M, Belluscio V, Vannozzi G, Morone G. Vestibular rehabilitation training in patients with subacute stroke: a preliminary randomized controlled trial. NeuroRehabilitation. 2018;43(2):247–254. doi:10.3233/NRE-182427.
- Vienne A, Barrois RP, Buffat S, Ricard D, Vidal PP Inertial sensors to assess gait quality in patients with neurological disorders: a systematic review of technical and analytical challenges. Front Psychol. 2017 May18; 8:817. 10.3389/fpsyg.2017.00817
- Martino Cinnera A, Morone G, Marrano S, Vannozzi G, Picerno P. Feasibility of using wearable inertial sensors for assessing gait changes after total knee arthroplasty: a systematic review and meta-analysis. Minerva Orthopedics. Oct, 2021;72(5):498–508. doi:10.23736/S2784-8469.21.04137-7.
- Carnevale A, Longo UG, Schena E, et al. Wearable systems for shoulder kinematics assessment: a systematic review. BMC Musculoskelet Disord. 2019 Nov 15;20(1):546. doi:10.1186/s12891-019-2930-4.
- Maceira-Elvira P, Popa T, Schmid AC, Hummel FC. Wearable technology in stroke rehabilitation: towards improved diagnosis and treatment of upper-limb motor impairment. J Neuroeng Rehabil. 2019 Nov 19;16(1):142. doi:10.1186/s12984-019-0612-y.
- Smuck M, Odonkor CA, Wilt JK, Schmidt N, Swiernik MA. The emerging clinical role of wearables: factors for successful implementation in healthcare. NPJ Digit Med. Published 2021 Mar 10 2021;4(1):45. doi:10.1038/s41746-021-00418-3.
- Parker J, Powell L, Mawson S. Effectiveness of upper limb wearable technology for improving activity and participation in adult stroke survivors: systematic review. J Med Internet Res. 2020 Jan 8;22(1):e15981. doi:10.2196/15981.
- Page MJ, McKenzie JE, Bossuyt PM, et al. The PRISMA 2020 statement: an updated guideline for reporting systematic reviews. J Clin Epidemiol. Jun 2021;134:178–189. doi:10.1016/j.jclinepi.2021.03.001.
- Bai L, Pepper MG, Yan Y, Phillips M, Sakel M. Low cost inertial sensors for the motion tracking and orientation estimation of human upper limbs in neurological rehabilitation. IEEE Access. 2020;8:54254–54268.
- Bai L, Pepper MG, Yan Y, Phillips M, Sakel M Quantitative measurement of Upper limb motion pre- and post-treatment with Botulinum Toxin. Meas. 2021 Jan 15; 168:108304. 10.1016/j.measurement.2020.108304
- Bertomeu-Motos A, Blanco A, Badesa FJ, Barios JA, Zollo L, Garcia-Aracil N. Human arm joints reconstruction algorithm in rehabilitation therapies assisted by end effector robotic devices. J Neuroeng Rehabil. 2018 Feb 20;15(1):10. doi:10.1186/s12984-018-0348-0.
- Bhagubai MMC, Wolterink G, Schwarz A, Held JPO, Van Beijnum BF, Veltink PH Quantifying pathological synergies in the upper extremity of stroke subjects with the use of inertial measurement units: a pilot study. IEEE J Transl Eng Health Med. 2020 Dec 7; 9:2100211. 10.1109/JTEHM.2020.3042931
- Kang S, Shin JH, Kim IY, Lee J, Lee JY, Jeong E. Patterns of enhancement in paretic shoulder kinematics after stroke with musical cueing. Sci Rep. 2020 Oct 22;10(1):18109. doi:10.1038/s41598-020-75143-0.
- Li J, Pan B, Jin T, et al. A single task assessment system of upper-limb motor function after stroke. Technol Health Care. 2016 Apr 29;24(Suppl s2):S707–15. doi:10.3233/THC-161199.
- Biswas D, Corda D, Baldus G, et al. Recognition of elementary arm movements using orientation of a tri-axial accelerometer located near the wrist. Physiol Meas. Sep 2014;35(9):1751–1768. doi:10.1088/0967-3334/35/9/1751.
- Bochniewicz EM, Emmer G, McLeod A, Barth J, Dromerick AW, Lum P. Measuring functional arm movement after stroke using a single wrist-worn sensor and machine learning. J Stroke Cerebrovasc Dis. Dec, 2017;26(12):2880–2887. doi:10.1016/j.jstrokecerebrovasdis.2017.07.004.
- Held JPO, Klaassen B, Eenhoorn A, et al.Inertial sensor measurements of upper limb kinematics in stroke patients in clinic and home environmentFront Bioeng Biotechnol2018 Apr 12610.3389/fbioe.2018.00027
- Lin BS, Hsiao PC, Yang SY, Su CS, Lee IJ. Data glove system embedded with inertial measurement units for hand function evaluation in stroke patients. IEEE Trans Neural Syst Rehabil Eng. Nov, 2017;25(11):2204–2213. doi:10.1109/TNSRE.2017.2720727.
- Mahmoud SS, Cao Z, Fu J, Gu X, Fang Q Occupational therapy assessment for upper limb rehabilitation: a multisensor-based approach. Front Digit Health. 2021 Dec 17; 3:784120. 10.3389/fdgth.2021.784120
- Mazomenos EB, Biswas D, Cranny A, et al. Detecting elementary arm movements by tracking upper limb joint angles with MARG sensors. IEEE J Biomed Health Inform. Jul 2016;20(4):1088–1099. doi:10.1109/JBHI.2015.2431472.
- Michaelsen SM, Gomes RP, Marques AP, et al. Using an accelerometer for analyzing a reach-to-grasp movement after stroke. Motriz: Revista de Educação Física. Oct/Dec 2013;19(4):746–752. doi:10.1590/S1980-65742013000400012.
- Repnik E, Puh U, Goljar N, Munih M, Mihelj M. Using inertial measurement units and electromyography to quantify movement during action research arm test execution. Sensors (Basel). 2018 Aug 22;18(9):2767. doi:10.3390/s18092767.
- Schwarz A, Bhagubai MMC, Wolterink G, Held JPO, Luft AR, Veltink PH. Assessment of upper limb movement impairments after stroke using wearable inertial sensing. Sensors (Basel). 2020 Aug 24;20(17):4770. doi:10.3390/s20174770.
- Schwarz A, Veerbeek JM, Held JPO, Buurke JH, Luft AR Measures of interjoint coordination post-stroke across different upper limb movement tasks. Front Bioeng Biotechnol. 2021 Jan 28; 8:620805. 10.3389/fbioe.2020.620805
- Thies SB, Tresadern PA, Kenney LP, et al. Movement variability in stroke patients and controls performing two upper limb functional tasks: a new assessment methodology. J Neuroeng Rehabil. 2009 Jan 23;6(1):2. doi:10.1186/1743-0003-6-2.
- van Meulen FB, Reenalda J, Buurke JH, Veltink PH. Assessment of daily-life reaching performance after stroke. Ann Biomed Eng. Feb, 2015;43(2):478–486. doi:10.1007/s10439-014-1198-y.
- van Meulen FB, Klaassen B, Held J, et al. Objective evaluation of the quality of movement in daily life after stroke. Front Bioeng Biotechnol. 2016 Jan 13; 3: 210. 10.3389/fbioe.2015.00210.
- Wade E, Chen C, Winstein CJ. Spectral analyses of wrist motion in individuals poststroke: the development of a performance measure with promise for unsupervised settings. Neurorehabil Neural Repair. Feb, 2014;28(2):169–178. doi:10.1177/1545968313505911.
- Schober P, Boer C, Schwarte LA. Correlation coefficients: appropriate use and interpretation. Anesth Analg. 2018;126(5):1763–1768. doi:10.1213/ANE.0000000000002864.
- Gjerdevik M, Heuch I. Improving the error rates of the Begg and Mazumdar test for publication bias in fixed effects meta-analysis. BMC Med Res Methodol. 2014;14(1):109. doi:10.1186/1471-2288-14-109.
- Hesam-Shariati N, Trinh T, Thompson-Butel AG, Shiner CT, Redmond SJ, McNulty PA. Improved kinematics and motor control in a longitudinal study of a complex therapy movement in chronic stroke. IEEE Trans Neural Syst Rehabil Eng. Apr, 2019;27(4):682–691. doi:10.1109/TNSRE.2019.2895018.
- Park E, Lee K, Han T, Nam HS. Automatic grading of stroke symptoms for rapid assessment using optimized machine learning and 4-limb kinematics: clinical validation study. J Med Internet Res. 2020 Sep 16;22(9):e20641. doi:10.2196/20641.
- Kim JY, Park G, Lee SA, Nam Y. Analysis of machine learning-based assessment for elbow spasticity using inertial sensors. Sensors (Basel). 2020 Mar 14;20(6):1622. doi:10.3390/s20061622.
- Ang WS, Geyer H, Chen I-M, Ang WT. Objective assessment of spasticity with a method based on a human upper limb model. IEEE Trans Neural Syst Rehabil Eng. 2018;26(7):1414–1423. doi:10.1109/TNSRE.2018.2821197.
- Chen Y, Yu S, Cai Q, et al. A spasticity assessment method for voluntary movement using data fusion and machine learning. Biomedical Signal Processing and Control. 2021;65:65, 102353. doi:10.1016/j.bspc.2020.102353.
- Paulis WD, Horemans HL, Brouwer BS, Stam HJ. Excellent test-retest and inter-rater reliability for Tardieu Scale measurements with inertial sensors in elbow flexors of stroke patients. Gait Posture. Feb, 2011;33(2):185–189. doi:10.1016/j.gaitpost.2010.10.094.
- Chen ZJ, He C, Gu MH, Xu J, Huang XL Kinematic evaluation via inertial measurement unit associated with upper extremity motor function in subacute stroke: a cross-sectional study. J Healthc Eng. 2021 Aug 19; 2021:4071645. 10.1155/2021/4071645
- Nikmaram N, Scholz DS, Großbach M, et al. Musical sonification of arm movements in stroke rehabilitation yields limited benefits. Front Neurosci. 2019 Dec 20; 13: 1378. 10.3389/fnins.2019.01378.
- Nie JZ, Nie JW, Hung NT, Cotton RJ, Slutzky MW. Portable, open-source solutions for estimating wrist position during reaching in people with stroke. Sci Rep. 2021 Nov 18;11(1):22491. doi:10.1038/s41598-021-01805-2.
- Pan B, Huang Z, Jin T, Wu J, Zhang Z, Shen Y. Motor function assessment of upper limb in stroke patients. J Healthc Eng. 2021;2021:6621950. Feb 24; 2021. doi:10.1155/2021/6621950.
- Rau CL, Chen YP, Lai JS, et al. Low-cost tele-assessment system for home-based evaluation of reaching ability following stroke. Telemed J E Health. Dec 2013;19(12):973–978. doi:10.1089/tmj.2012.0300.
- Salazar AJ, Silva AS, Silva C, et al. Low-cost wearable data acquisition for stroke rehabilitation: a proof-of-concept study on accelerometry for functional task assessment. Top Stroke Rehabil. Jan-Feb 2014;21(1):12–22. doi:10.1310/tsr2101-12.
- Tedim Cruz V, Bento VF, Ribeiro DD, Araújo I, Branco CA, Coutinho P. A novel system for automatic classification of upper limb motor function after stroke: an exploratory study. Med Eng Phys. Dec, 2014;36(12):1704–1710. doi:10.1016/j.medengphy.2014.09.009.
- Zollo L, Rossini L, Bravi M, Magrone G, Sterzi S, Guglielmelli E. Quantitative evaluation of upper-limb motor control in robot-aided rehabilitation. Med Biol Eng Comput. Oct, 2011;49(10):1131–1144. doi:10.1007/s11517-011-0808-1.
- Smania N, Picelli A, Munari D, et al. Rehabilitation procedures in the management of spasticity. Eur J Phys Rehabil Med. Sep 2010;46(3):423–438.
- Bar-On L, Aertbeliën E, Molenaers G, Dan B, Desloovere K. Manually controlled instrumented spasticity assessments: a systematic review of psychometric properties. Dev Med Child Neurol. Oct, 2014;56(10):932–950. doi:10.1111/dmcn.12419.
- Biering-Sørensen F, Nielsen JB, Klinge K. Spasticity-assessment: a review. Spinal Cord. 2006;44(12):708–722. doi:10.1038/sj.sc.3101928.
- David A, Subash T, Varadhan SKM, Melendez-Calderon A, Balasubramanian S A Framework for Sensor-Based Assessment of Upper-Limb Functioning in Hemiparesis. Front Hum Neurosci. 2021 Jul 22; 15:667509. 10.3389/fnhum.2021.667509
- Porciuncula F, Roto AV, Kumar D, et al. Wearable Movement Sensors for Rehabilitation: a Focused Review of Technological and Clinical Advances. PM&R. 2018 Sep;10(Suppl 9S2):S220–232. Erratum in: PM R. 2018 Dec;10(12):1437. doi:10.1016/j.pmrj.2018.06.013.
- Winstein CJ, Stein J, Arena R, et al. Guidelines for Adult Stroke Rehabilitation and Recovery: a Guideline for Healthcare Professionals from the American Heart Association/American Stroke Association [published correction appears in Stroke. Stroke. 2016;47(6):e98–169. 2017 Feb;48(2):e78] [published correction appears in Stroke. 2017 Dec;48(12):e369]. doi:10.1161/STR.0000000000000098.
- Lang CE, Barth J, Holleran CL, Konrad JD, Bland MD. Implementation of Wearable Sensing Technology for Movement: pushing Forward into the Routine Physical Rehabilitation Care Field. Sensors (Basel). Published 2020 Oct 10 2020;20(20):5744. doi:10.3390/s20205744.
- Boukhennoufa I, Zhai X, Utti V, Jackson J, McDonald-Maier KD. Wearable sensors and machine learning in post-stroke rehabilitation assessment: a systematic review. Elsevier, Biomedical Signal Processing and Control. January 2022;71: 10.1016/j.bspc.2021.103197