Abstract
An experimental study on noninvasive methods for simultaneous prediction of pears' internal quality was investigated. This method is based on Fourier transform near infrared (FT-NIR) spectrometry with fiber optics in the region between 800 and 2500 nm. A total of 248 pear samples were used to develop the calibration models. The quality indices included soluble solids content (SSC) and titratable acidity (TA). Partial least squares (PLS) regression and principle component regression (PCR) were carried out describing the relationships between the data sets of laboratory data and the FT-NIR spectra. Calibration models based on the different spectral ranges and with several data pre-processing techniques (smoothing, multiplicative signal correction, standard normal variate, etc) were also compared. Performance of different calibration models was assessed in terms of root mean square errors of prediction (RMSEP) and correlation coefficients (r) of validation set of samples. The best predictive models had a RMSEP of 0.320, 0.019, and correlation coefficient (r) equal to 0.93, 0.89 for SSC and TA, respectively. It is concluded that FT- NIR spectrometry could be an easy to facilitate, reliable, accurate and fast method for noninvasive prediction of pears' internal quality.
INTRODUCTION
Quality evaluation of agricultural products is an important cue for both producers and consumers. Pear is a nutrition fruit for which there is heavy demand and which fetches a good price in the world market. To ensure the supply of high-quality pear, it is important to select pear with the proper degree of maturation, which is, however, not defined categorically in the literature. Various scientists have considered internal quality from different perspectives.[Citation1] Soluble solids content and acidity are the properties most likely to match the consumer's perception of internal quality, but they currently require a destructive measurement.[Citation2] Therefore, the development of a reliable, noninvasive method for the internal quality prediction of pears, before harvest and at the packing site, is critical to the success of the pear industry.
Near-infrared spectroscopy (NIRS) has been used in a variety of agricultural and food technology applications.[Citation3–4] In this technique, information about the internal quality of the fruits is obtained by measuring the absorption of near-infrared light by functional groups and scattering at specific wavelength.[Citation5] Since the 1980s, most research concerning the quality determination of fruits were achieved with dispersive NIRS.[Citation6–10] These instruments use grating to separate the individual frequencies of the radiation leaving the sample. Fourier transform NIR (FT-NIR) spectrometry and multiple data analysis techniques have had significant impact on the determination of changes in food composition. The FT-NIR instruments do have some major advantages: (1) higher speed because all frequencies are measured simultaneously; (2) simplified mechanics, the moving mirror or quartz wedge being the only moving part in the instrument with a reduced risk of mechanical breakdown; (3) self-calibrating with a He-Ne laser and wavelengths, and therefore never needing calibration by the user;[Citation9] and (4) high spectral signal-to-noise ratio obtained from FT-NIR instruments helps to detect constituents present in quite low concentrations. Previous researches for measuring sugar content in fruit juices have proved that FT-NIR method has some potential use in fruit industry.[Citation11]
The overall objective of the present research was to evaluate the feasibility of rapid prediction of internal quality of intact pear fruit using FT-NIR spectroscopic technology. The research would focus on the prediction performance of calibration models with different harvest time. The specific objectives of the research were: (1) to report the information obtained by FT-NIR spectrometry on internal quality indices of intact pear; (2) to establish relationships between the FT-NIR measurements and the major internal quality indices which relate to the maturity of the pear; (3) to compare the prediction performance of calibration models with different data pre-processing and calibration techniques; and (4) to find out the optimal wavelength range and for developing the best calibration models.
MATERIALS AND METHODS
System of FT-NIR Measurements
The FT-NIR measurement was equipped with Nexus FT-NIR spectrometer (Thermo Nicolet, Corporation, USA) and a smart near-IR fiber port (Type 847–072200) (). FT-NIR spectrometer was equipped with an interferometer, an InGaAs detector, and a wide band light source (50 W) quartz halogen to provide interactance measurements. Pears were placed steadily upon the fruit holder, with the stem-calyx axis horizontal. In the head of the bifurcated cable, the source and detector fibers were situated randomly, the light was guided to the sample by source fibers, and from the sample with the detector fibers to Nexus FT-NIR spectrometer, which had a spectral range of 800–2500 nm and the interferograms (64) were co-added followed by strong Beer-Norton apodization. The mirror velocity was 0.9494 cm/s and the resolution was 16 cm−1. In order to avoid the surface reflectance and guarantee subsurface penetration of the light into the pear flesh, the bifurcated optical probe was placed at a 75° angle to the level.
Fruit Samples
The experiment was performed over one month and the pears were picked during about four weeks before the commercial picking date at the experimental station of Zhejiang University. The harvest dates ensured a good range of fruit SSC and TA levels. For each measurement session, pears were randomly picked at eye level in the middle of the canopy of different trees and marked its sunny side and shade side for each pear. The pears were transported to the laboratory and stored under ambient conditions (24°C and 57% relative humidity) before being examined by the FT-NIR measurement. All measurements including spectral collection and quality analysis were carried out on the same day or the day after harvesting. In total 262 pears were collected in the experiment and all of them had been sized by weight.
Internal Quality Indices Determination
SSC and TA measurements were then performed in the afternoon on all fruit. For these measurements the fruit were cut in half and the juice extracted using a manual fruit squeezer (model: HL-56, Shanghai, China). Samples of the filtered juice were then taken for SSC measurement by digital refractometer (model: WYT-4, Quanzhou, China) by the China standard for SSC measurement in fruit (GB12295-90), and TA was measured on approximately 10 g of cortical tissue and reported as percentages, by fruit fresh weight, in terms of the malic acid equivalent. The tissue was macerated and juice, filtered from the homogenate, titrated to an endpoint at pH of 8.02 using 0.05 N NaOH. Titrations were undertaken using an autotitrator (model: ZD-2 Titrino□ Shanghai, China) according to the China standard about TA measurement in fruit (GB/T12456-90).
Multivariate Data Analysis
Region selection
The selection of the portion of the spectra to be used in calibration is crucial and determines the quality of the process. Wavelengths above 2000 nm were discarded due to the insensitivity of the InGaAs detector above this value, where the intensity spectrum drops drastically and the absorbance spectrum becomes noisy (). In this work, three regions were used in the calibration process, one being a subset of the other. The three regions used were 814–1155 nm, 1155–1834 nm, and 814–1834 nm. The latter suppresses the color information contained in the first region. In other words, the influence of the visible spectrum, its reddish component, is of particular interest.
Spectral correction
In the experiments, light scattering affects the path length, and in the transmittance case sample size variability is also a relevant factor. Three types of path length correction algorithm were used in this work. The first correction scheme assumes that the path length can be undecided. The standard normal variate (SNV) is another approach to deal with this problem.[Citation1] This algorithm removes the scattering by normalizing each spectrum by the standard deviation of the responses across the entire spectral range. Multiplicative scatter correction (also known as multiplicative signal correction (MSC) is the third algorithm that can be used. This technique (commonly used in chemometrics) regresses a measured spectrum against a reference spectrum and corrects the former using its slope.[Citation12] In this work, the mean spectrum of the calibration set was used as the reference spectrum. These three algorithms were programmed in the TQ Analyst v6.0.
Regression method
The Nicolet TQ Analyst v6.0 (Madison, Wisconsin, USA) was used for processing the data and NIR models were developed for the calibration part of the sample data set. Processed spectral data such as the first derivative, log (1/R) and its second derivative were analyzed for partial least square (PLS) and principle component regression (PCR). Typical spectra of log (1/R) and D 2 log (1/R) are presented in . Models were formulated which related the NIR spectra SSC, and TA in each tested pears. The error associated with the results of such a model is defined by the standard error of calibration (RMSEC): The RMSEC and RMSEP are calculated as follows:
with
ŷi = predicted value of the i-th observation; yi = measured value of the i-th observation; Ic = number of observations in calibration set; Ip = number of observations in prediction se. For each preprocessing sequence (e.g., spectral region, path length correction, smoothing, and derivation), the regression methods were used to develop a calibration procedure, using RMSECV to determine the optimum number of factors to be included in the model. Mean center was used for all calibrations.
RESULTS AND DISCUSSION
Soluble Solids Content and Titratable Acidity Distributions
The means, ranges, and standard deviations (SDs) of pear quality parameters are summarized in . The mean SSC, and TA measurements were 12.82%, (SD = 0.69), and 0.139% (SD = 0.033), respectively, where the bracketed values are the standard deviations. The SSC measurements (n = 248) were fairly normally distributed around the mean (max = 14.30%, min = 10.98%) but the TA distribution (n = 248) was skewed with the high-end tail stretching further than the corresponding low-end tail (max = 0.253%, min = 0.095%). Among the samples analyzed for the different parameters, some of them were selected for calibration by OMNIC 6.1a software, and the remaining samples were used for the validation set () and the correlation coefficients between the pear parameters is summarized in . explains some internal quality evolutions observed in a pear: when soluble solids increase with internal quality, the TA parameter decreases. The internal quality leads to a decrease of acidity and there is a general trend between acidity and soluble solids content, but all observed evolutions are dependent on the varieties and growth condition.
Table 1 Summary statistics of parameters of pear samples in this experiment
Table 2 Means, ranges, and SDs of pear samples of calibration and validation sets
Table 3 Correlation coefficients between SSC and TA parameters about 248 pear samples
Spectral Correction for Calibration Models
In the experiments (interactance), light scattering affects the path length, and in the interactance case sample size variability is also a relevant factor. Therefore, there is a need for some kind of correction in order to minimize the error when applying Beer's equation to the spectra.[Citation12] Three types of path length correction algorithm were used in this work. The results for SSC, TA and VA calibration models of PLS regression methods for log (1/R) using different spectral correction is presented in . explains the effect of different spectral correction on prediction models and the best prediction results were given a when using MSC-treated spectrum.
Table 4 The results for SSC and TA calibration models of PLS regression methods for log (1/R) using different spectral correction in the wavelength range of 814–1834 nm
Wavelength Selection for Calibration Models
Developing the calibration models involved deciding how many wavelengths to use and selecting an optimal of wavelengths, wavelengths where the data were noisy and provided little predictive ability were eliminated prior to selection of regressions. In this work, three regions were used in the calibration process, one being a subset of the other. The three regions included three effective NIR regions, two of them are ranges 814–1155 nm and 814–1834 nm, known to contain typical absorption bands for carbohydrate, sugar and water absorbance, and the third is regions 1155–1834 nm known to contain typical absorption bands for acidity groups (C-O stretch from COOH at 1607 nm, O-H stretch from carboxylaciditys at 1127 nm and C = O stretch from saturated and un saturated carboxylaciditys at 1437 nm). The calibration set was selected with the aim of providing strong calibration for SSC or TA by maximizing the variability among sample compositions and obtaining a wide range of spectra to avoid outliers in the validation set. Selection of the optimum wavelength range for best prediction model was done by PLS analysis in TQ Analyst 6.2.1 (Nicolet, Co., USA). The SSC and TA prediction results of different wavelengths regions are presented in .
Table 5 The results for calibration models of PLS regression methods for the log (1/R), means values of RMSEC, RMSEP, and RMSECV, the correlation coefficients of determination, r with 814–1834 nm, 1155–1834 nm, and 814–1155 nm wavelength ranges
The optimum wavelength range for best prediction models of SSC and TA was at 814–1834 nm region with a relative high correlation coefficient of determination r2 = 0.863 and 0.799, a relatively low RMSEC of 0.256 and 0.02, a relatively low RMSEP of 0.320 and 0.019, respectively, and was therefore selected as the optimum wavelength range for SSC and TA predictions ().
Statistical Calibration Models
It is desirable that a model has a low error of calibration, with as few factors as possible. The selection of calibration models based on this criterion, with the total described variance considered to be appropriate (about 90%), was conducted by means of the OMNIC 6.0 software. The results for the selected calibration models of PLS and PCR for the log (1/R), its first derivative D 1 log (1/R), and its second derivative D 2 log (1/R), are presented in .
Table 6 The results for calibration models of PLS and PCR methods for the log (1/R), first derivative D 1log (1/R), and its second derivative D 2 log (1/R), means values of RMSEC and the coefficients of determination, r 2
In general, the PLS method seems to produce the best calibration results using D 2 log (1/R) for SSC and TA. An example to the calibration results of SSC by the NIR system versus laboratory measurements is represented in (PLS model, in conjunction with the following figures). Although PCR models seem to produce a reasonable RMSEC, a non-monotonic relationship of RMSEC to the number of factors (undesired behavior) prevents confident use of these models for prediction purposes.
Statistical Prediction Analysis
Based on the above, PLS regression models for SSC and TA were applied to the prediction data set for log (1/R). The root mean standard error of prediction (RMSEP) was calculated by means of the same formula as the RMSEC. The RMSEP represents the sensitivity of prediction. The results of these predictions are presented in . Comparison between SMLR and PCR results indicates that the PLS method is preferable. PLS prediction results for SSC and TA are presented in scatter plots, in and , respectively. In all figures, the ordinate and abscissa axes represent the measured and the fitted values of the appropriate parameters, respectively. The correlation between the measured and predicted values of the parameters is high for SSC and moderate for TA.
Table 7 The results for prediction errors of PLS regression method and r 2, the coefficients of determination for the log (1/R) based on about 33% of the data set, for SSC and TA parameters
Figure 4 Predictions of partial least square regression by the FT-NIR system versus laboratory measurements of soluble solids content of pear fruit.
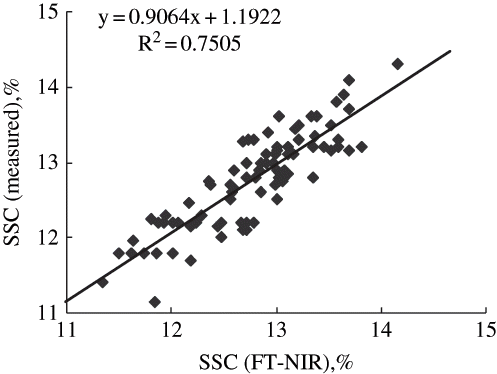
Figure 5 Predictions of partial least square regression by the FT-NIR system versus laboratory measurements of titratable acidity of pear fruit.
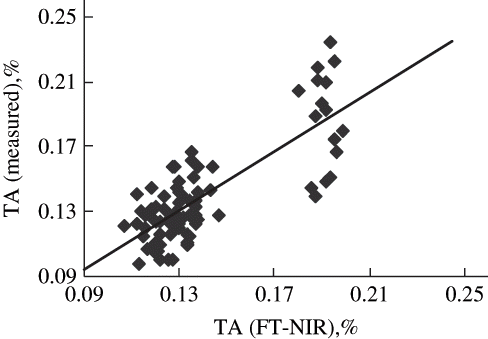
Due to the randomized data split process for calibration and prediction sets, some graphs show discontinuity (e.g. the TA between 0.14 and 0.18 g/kg). However, the lake of data observed in the prediction results () may affect in the calibration results (). The low acidity of the fruit might have caused the relative insensitivity for NIR prediction. The slope, offset, correlation, root mean standard error of cross validation (RMSECV) and number of PLS factors for best regression models for the log (1/R) based on about 33% of the data set, for SSC and TA parameter in 814–1834 nm wavelength ranges also are given in .
Table 8 Slope, offset, correlation, root mean standard error of cross validation (RMSECV) and number of PLS factors for best regression models for the log (1/R), based on about 33% of the data set, for SSC and TA parameters
CONCLUSION
This research has established a system for the non-destructive evaluation the internal quality of pears in different harvest date based on FT-NIR spectrometry. By means of multivariate calibration techniques (PLS and PCR) relationships were established between reflectance spectra and SSC or TA parameter. However, the log (1/R) spectra with PLS was found to provide the best prediction of the physical properties of the pear. The nondestructive FT-NIR measurements provided good estimates of the internal quality indices of the pear fruit, especially the SSC. The predicted values were highly correlated with destructively measured values for SSC.
ACKNOWLEDGMENTS
The authors wish to thank the Natural Science Foundation of China (Projects 60468002, 30560064) and the Program for New Century Excellent Talents in University, No. NCET-04-0524, for financial support.
REFERENCES
- Peirs , A.N. , Scheerlinck , K.T. and Nicolai , B.M. 2002 . Comparison of Fourier Transform and Dispersive Near-infrared Reflectance Spectroscopy for Apple Measurements . Biosystems Engineering , 81 : 305 – 311 .
- Belton , P.S. , Kemsley , E.K. , McCann , M.C. , Ttofis , S. , Wilson , R.H. and Delgadillo , I. 1995 . The Identification of Vegetable and Matter Using Fourier Transform Infrared Spectroscopy . J. Food Chem. , 54 : 437 – 441 .
- Wang , D. , Dowell , F.E. , Ram , M.S. and Schapaugh , W.T. 2004 . Classification of Fungal-Damaged Soybean Seeds Using Near-Infrared Spectroscopy . International Journal of Food Properties , 7 : 75 – 82 .
- Wang , D. , Dowell , F.E. , Lan , Y. , Pasikatan , M. and Maghirang , E. 2005 . Determining Pecky Rice Kernels Using Visible and Near Infrared Spectroscopy . International Journal of Food Properties , 5 : 629 – 639 .
- Schmilovitch , Ze. ev. , Mizrach , A. , Hoffman , A. and Haim , E. 2000 . Determination of Mango Physiological Indices by Near-infrared Spectrometry . J. Postharvest Biolo. Technol. , 19 : 245 – 252 .
- Kawano , S. , Fujiwara , K. and Iwamoto , M. 1993 . Nondestructive determination of sugar content in Satsuma mandarin using near infrared (NIR) transmittance . Journal of Japanese Society Horticultural Science , 62 : 465 – 470 .
- Lammertyn , J. , Nicolai , B. , Ooms , K. , De Smedt , V. and De Baerdemaeker , J. 1998 . Non-Destructive Measurement of Acidity, Soluble Solids and Firmness of Jonagold Apples Using NIR-Spectroscopy . Transactions of the ASAE , 41 : 1089 – 1094 .
- Peirs , K.H.S. , Dull , G.G. , Leffler , R.G. and Kays , S.J. 1999 . Spatial Variability of Soluble Solids or Dry-matter Content within Individual Fruits, Bulbs, or Tubers: Implications for the Development and Use of NIR Spectrometric Techniques . HortScience , 34 : 114 – 118 .
- Lu , R. 2001 . Predicting Firmness and Sugar Content of Sweet Cherries Using Near-Infrared Diffuse Reflectance Spectroscopy . Transactions of the ASAE , 44 : 1265 – 1271 .
- Wise , B.M. and Gallagher , N.B. 2000 . PLS_Toolbox 2.1 for use with MATLAB™ , Manson, WA : Eigenvector Research Inc. .
- Banwell , C.N. 1983 . Fundamentals of Molecular Spectroscopy , 3rd , London : McGraw-Hill .
- Rubinson , K.A. and Rubinson , J.F. 2000 . Contemporary Instrumental Analysis , Englewood Cliffs, NJ : Prentice-Hall .