Abstract
Dynamic oscillatory shear rheological characteristics of honey samples from different floral sources were evaluated using frequency sweep test and stress sweep test at three different temperatures (10, 15, and 20°C). All honey samples showed liquid-like behavior because the loss modulus was significantly greater than the elastic modulus. Pine honey showed the highest complex viscosity (86.33 Pa s at 10°C), while the lowest complex viscosity was observed in citrus honey (22.15 Pa s) at the same temperature. Temperature dependency of complex viscosity of honey samples was modeled by Arrhenius model and activation energy values of honeys ranged from 83.13 to 97.46 kJ/mol. An efficient predictive model for complex viscosity values of honeys was constructed using adaptive neuro fuzzy inference system that showed satisfactory prediction performance with high coefficient of determination (0.995) and low root mean square error (0.04) in the validation period compared to artificial neural networks.
Keywords:
INTRODUCTION
Honey is a sweet and natural liquid-like food with high nutritional value produced by honeybees from plant nectar or honeydew. It contains readily digestible sugar, different organic acids, many amino acids, and different bioactive compounds.Citation[1, Citation2] Honey contains different sugar types depending on the variety, most of which are in monosaccharide form, namely, glucose and fructose, and also some disaccharides are present in honey.Citation[3] Honey is one of the most important food products produced in Turkey, which ranks fourth in honey production among the honey-producing countries. Many different honey types are present with different floral origins in the world and the common honey varieties are chestnut, citrus, pine, thyme, linden, sunflower, and cotton.Citation[4] Since honey is a semi-solid food product, its rheological characteristics as well as physicochemical properties are important for the food industries and consumers. One of the most important rheological characteristics of honey is viscosity. Apparent viscosity as a function of shear rate is related to steady state shear rheology and it is particularly critical during storage, handling, and processing.Citation[5, Citation6] The magnitudes of viscosity values and other rheological characteristics are influenced by different factors, including composition, temperature, amount and size of crystals, and moisture content. Due to the differences in variety, rheological characteristics and physicochemical properties change depending on the structure of the honey.Citation[3, Citation5]
Many different studies are present in the literature related to physicochemical properties and rheological characterization of different honey types from different countries.Citation[2, Citation3, Citation7– Citation11] According to the information given in the literature, honey is a Newtonian fluid. But some honey samples showed a thixotropic behavior due to the presence of colloids in their structure, such as buckwheat, heather, and white clover.Citation[3, Citation12]
Adaptive neuro fuzzy inference system (ANFIS) and artificial neural networks (ANN) are effective and novel nonlinear modeling techniques used in food science to model a complex nonlinear system. Effective predictive models can be constructed using ANFIS and ANN because foods have a complex nonlinear nature. There are different studies related to application of these nonlinear modeling techniques to estimate the different parameters of food samples, and effective predictive models were constructed.Citation[13– Citation16]
In our previous work, some Turkish honey samples were rheologically characterized.Citation[4] All honey samples studied showed Newtonian behavior in steady state shear rheological measurements. Until today, no study has appeared in the literature focusing on the dynamic mechanical spectra of Turkish honeys. For that reason, the main aim of this study was to determine the dynamic mechanical properties of some Turkish honey samples and temperature dependency of some important rheological parameters. Also, it was aimed that the construction of predictive models using effective nonlinear modeling techniques, such as ANFIS and ANN, would estimate the complex viscosity values of sample depending on the variety, measurement temperature, and frequency.
MATERIALS AND METHODS
Honey Samples
Three honey varieties (citrus, flower, and pine) used in the present study were obtained from Anavarza Company (Anavarza Brand, Sezen Food Co., Adana, Turkey). Citrus and flower honeys were nectar honeys, while pine honey was from honeydew. Before the rheological measurements, commercial grade honeys were preconditioned because the viscosity may be influenced by air bubbles and crystals present in the honey. For this purpose, the honey samples were held at 55°C in a water bath for 1 h, and afterwards placed overnight in an oven (Nuve, Ankara, Turkey) at 30°C.
Physicochemical Analysis
The dry matter content of the samples was determined according to the standard procedures.Citation[17] Ash content was found by incinerating samples at 625°C in a muffle oven (Protherm, Ankara, Turkey) after drying and pH of honey samples was determined using a pH meter (WTW-Inolab, Weilheim, Germany) in a solution of 10% (w/v) honey in distilled water at 25°C. Water activity of the samples at 20°C was determined using an Aqualab water activity (Aw) meter (Decagon, Pullman, WA, USA). A colorimeter (Lovibond RT Series Reflectance Tintometer, Amesbury, England) was used to determine the color values of the samples; the color values were recorded as L (brightness), a (±red–green), and b (±yellow–blue). Each analysis was repeated three times.
Dynamic Mechanical Analysis
Dynamic mechanical properties of honey samples were determined using a stress/strain controlled rheometer (RheoStress 1, Haake, Karlsruhe, Germany) equipped with a temperature control unit (Thermo-Haake, Karlsruhe, Germany). Cone and plate geometry was used (cone diameter 35 mm, angle 4°, and gap size 0.140 mm) for the measurements. Before applying the frequency sweep test, the stress sweep test procedure was carried out to determine the linear viscoelastic region (LVR) of samples. Using this test, variation of dynamic mechanical spectra of honey varieties versus increased stress was characterized and LVR of samples over a stress range of 0.1–10 Pa at constant frequency (1 Hz) was determined since LVR is essential for the frequency sweep test application. In an oscillatory frequency sweep test, dynamic mechanical spectra of the honey sample were evaluated in the frequency range of 0.1–10 Hz at constant stress (within the range of LVR) at three different temperatures (10, 15, and 20°C). Low temperatures were selected for the rheological measurements since honey is very sensitive to the temperature. A sinusoidal stress or strain with an increasing frequency was applied to the honey sample at selected temperatures. The elastic modulus G′ and the viscous modulus G′′ are calculated as a function of frequency:Citation[18]
The overall response of the sample against the oscillatory shear may be characterized using the equations of complex modulus G* and complex viscosity η*:
The temperature dependency of complex viscosity and complex modulus of honey samples at a specified frequency (1 Hz) was described by the Arrhenius model:Citation[19, Citation20]
The activation energy was obtained by linear plotting of ln (η* and G*) against (1/T). The mean absolute percentage error (MAPE) was assessed according to the following equation to determine the deviance of calculated viscosity by the Arrhenius model from the observed value:
Nonlinear Modeling Procedure
The fuzzy logic toolbox of MATLAB 7.0.1 was used for the construction of the ANFIS model. Before starting the modeling, experimental complex viscosity data of samples were classified into three groups. Half of the selected data (90 data points) was determined as training data matrix. For the testing and validation of a fuzzy model system, 45 data points different from the data selected for training were used, separately. In the construction of the fuzzy model, three membership functions and product-of-sigmoidals membership function type (psig mf) for input and constant membership function type for output were used and models were constructed using ANFIS and Sugeno-type fuzzy inference system. Determination of coefficient (R 2), root mean square error (RMSE), and mean absolute error (MAE) were calculated as comparison criteria for the evaluation of developed model parameters. Honey type, measurement frequency of dynamic mechanical spectra, and temperature were selected as input while the complex viscosity was output. Ten learning epochs and a radius of 0.5 were used for the development and implementation of models.
ANN modeling was carried out using MATLAB 7.0.1. (Statistics Toolbox, MatLab 7.0.1., The MathWorks, Inc., MA, USA). It has one or more hidden layers, whose computation nodes are called hidden neurons. Computed outputs are compared with experimental outputs, and obtained errors are back propagated to obtain the appropriate weight adjustments necessary to minimize errors in this modeling technique.
In the present study, the ANN was trained using the Levenberg–Marquardt technique because this technique is more powerful and faster compared to the conventional gradient descent technique.Citation[21, Citation22] The number of hidden layer neurons was determined using a simple trial–error technique in all applications. The ANN networks training were stopped after 50 epochs since the variation of error was too small after this period. The number of nodes in a layer was determined as 3-3-1 for ANN.
Statistical Analysis
The statistical analysis software package MINITABCitation[23] for Windows Release 13 was used for the statistical analysis. The effect of independent variables on the dependent variable was determined using one-way Analysis of Variance (ANOVA) with the general linear model procedure. Duncan Multiple Range Test was used to determine the difference between mean combination values using MstatC software.Citation[24]
RESULTS AND DISCUSSION
Physicochemical Properties
The results of physicochemical analysis of honey samples were tabulated in . As can be seen from the table, moisture content of samples showed no significant differences (p > 0.05). These results are consistent with the results obtained in our previous work. Moisture content of honey samples were found to be close to each other and very important differences were not observed among the moisture of them, which is determined by the refractometric method.Citation[4] The ash content of the sample was determined in the range of 0.08–0.80%. The difference among the ash content of the samples was found to be significant (p < 0.05). The highest ash content was determined for pine honey, which is honeydew, compared to nectar honeys. Similar results were obtained in different studies. Ouchemoukh et al.Citation[25] found that the highest ash content (0.54%) for honeydew botanical origin and nectar honeys showed lower ash content compared to honeydew. A similar trend was determined by Kayacier and Karaman.Citation[4] pH value of the honey samples ranged from 4.45 to 5.63. It can be said that Turkish honeys have an acidic characteristic. The lowest pH was measured for citrus honey; the differences were statistically significant (p < 0.05). Pine honey, a honeydew honey, showed the highest pH. Similar results were reported by Terrab et al.Citation[26] They found the average pH values of the citrus and honeydew honey to be 3.55 and 4.28, respectively. Nanda et al.Citation[27] stated that the acidic character of honeys is attributed to the presence of organic acid and variations in those constituents may be caused by a variation in acidity among the different honeys. Water activity values were determined to be low (0.57–0.58) as expected because honey has a high amount of sugar and no significant difference among the water activity of samples was shown (p > 0.05). Kayacier and KaramanCitation[4] reported that there was no difference among the water activity values of pine, citrus, and flower honey (p > 0.05), and the values were in the range of 0.51–0.52. In another study, water activity values of the honey samples were in the range of 0.528–0.663.Citation[28]
Table 1 Physicochemical properties of different honey samples
Citrus honey was found to be the highest bright honey sample because L values were found to be the highest (19.29) compared to the pine (8.93) and flower (10.81) and differences were found to be significant (p < 0.05). On the contrary, redness was determined to be high in pine honey with a high value (5.82), while the lowest was in citrus honey (4.03). The b value of citrus honey showed a significant difference compared to pine and flower honey (p < 0.05) and ranged between 11.23–17.30.
Rheological Characteristics
Frequency sweep test results
It is well known that most of the honey samples show a Newtonian flow behavior.Citation[3, Citation7– Citation9] As stated in our previous work, pine, citrus, and flower honeys studied in the present work showed Newtonian flow behavior. The shear stress of Turkish honey samples increased while the apparent viscosity remained constant with increasing shear rate.Citation[4] illustrates the oscillatory shear rheological parameters of honey samples obtained from frequency sweep test. It is clear that complex viscosity of pine, flower, and citrus honey remained constant with increasing frequency. Independence of complex or apparent viscosity of sample from shear rate or frequency means typical liquid like macromolecular solution.Citation[29] Hence, it could be claimed that rheology of honey samples is similar to that of a liquid-like macromolecular solution. Complex viscosity of pine honey was measured to be 44.63 Pa s at 1 Hz and 15°C, while the complex viscosity of flower and citrus were 21.73 and 12.91 Pa s, respectively (). Complex modulus as a function of loss modulus and storage modulus increased with increasing frequency and showed a similar behavior to loss modulus because it has a high impact on complex modulus because of its high value; the highest complex modulus value was observed for the pine honey (280.43 Pa) at 1 Hz and 15°C, while the lowest was observed for the citrus honey (81.14 Pa). Loss modulus that is a measure of the energy lost as viscous dissipation and indicates liquid behavior was found to be very high. The highest loss modulus value was measured for pine honey for all temperatures compared to those of others (). Storage modulus (G′) that is a measure of the energy stored in the material was found to be very low for all honey samples and it increased with increasing frequency (). It could be said that storage modulus is less important than loss modulus due to its very low values.Citation[11, Citation30] Also, small fluctuations were observed in storage modulus for all honeys because samples do not have elastic behavior. For that reason, loss modulus was characterized mostly compared to storage modulus in the present study. Because of the liquid-like nature of the honey samples, change of loss modulus (G″) with frequency is more important compared to the storage modulus with respect to providing knowledge about viscoelasticity of honey samples. For that reason, dependency of loss modulus of honey samples on angular frequency was modeled using a power law type relationship:
Table 2 Dynamic mechanical spectra values of different honeys at 1 Hz
Table 3 Slopes and intercepts of ln G″ versus ln ω data of different honeys at different temperatures
Figure 1 Oscillatory shear rheological parameters obtained from frequency sweep test of different honeys at 15°C.
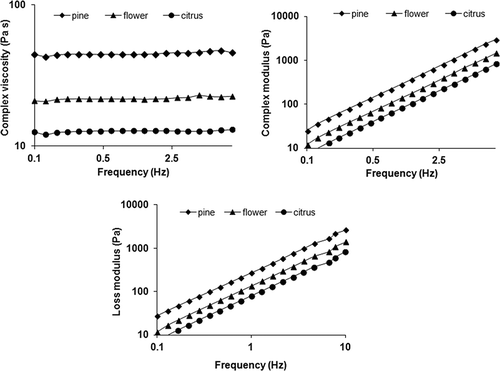
shows the effect of temperature on the complex viscosity and complex modulus of three different Turkish honey samples. Temperature increment caused a decrement in the complex viscosity and complex modulus as expected because it is a well known phenomenon that increment in temperature caused a decrease in viscosity of fluids due to the increment of kinetic energy in the structure of fluids.Citation[32] The complex viscosity of pine honey was measured to be 86.33 Pa s at 10°C while it decreased to 21.02 at 20°C. Similarly, complex modulus of pine honey was determined to be 542.47 Pa while it decreased to 132.07 due to the increase in temperature to 20°C. Citrus honey showed the lowest complex viscosity and complex modulus values compared to others (). Apparent viscosity of citrus honey was determined to be the lowest compared to pine and flower in our previous work.Citation[4]
Figure 2 Effect of temperature on complex viscosity and complex modulus obtained from frequency sweep test of different honeys. (a, b) Pine honey; (c, d) flower honey; and (e, f) citrus honey.
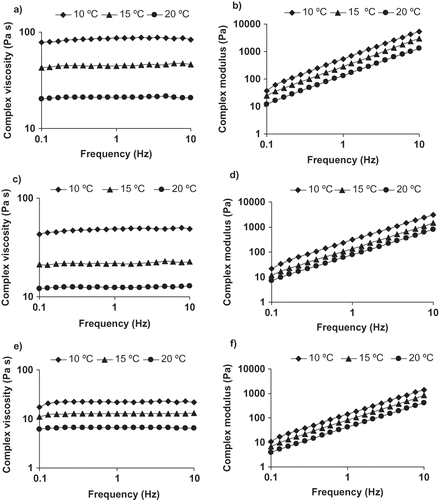
The effect of temperature on the dynamic mechanical spectra of Turkish honeys was determined and temperature dependency of parameters was adequately described using the Arrhenius equation with the determination coefficient of 0.991 or greater. The measured and computed complex viscosity and complex modulus as a function of temperature at 1 Hz are superimposed in . The mean absolute percentage error for the honey samples was in the range of 1.39–1.76 (). KleijnenCitation[33] reported that the recommended upper limit on the acceptability of MAPE is 10%. It could be concluded that the description of the complex viscosity and complex modulus as a function of temperature using Arrhenius is satisfactory. In other studies, Arrhenius equation was successfully used for describing the apparent viscosity of honey samples with high coefficient of determination.Citation[3, Citation4, Citation7] Activation energy calculated for the complex viscosity and complex modulus change as a function of temperature, Arrhenius constants, and coefficient of determinations were tabulated in . The differences based on the honey variety in activation energy values were found to be significant (p < 0.05). The activation energy was in the range of 83.13–97.46 kJ/mol for complex viscosity, while it was in the range of 83.12–97.45 kJ/mol for complex modulus. The highest E a was calculated for the complex viscosity and complex modulus of pine honey while the citrus honey had the lowest value. In general, activation energy values were inconsistent with those reported in the literature. The activation energy of Greek honeys calculated from apparent viscosity values varied between 69.1–93.8 kJ/mol.Citation[28] In another study, the activation energy of Polish honeys calculated from apparent viscosity was in the range of 92.3–105.3 kJ/mol.Citation[1] From these results, it can be said that dynamic mechanical spectra of honeys varies depending on the type of honey. All honey samples showed a liquid-like nature because their loss modulus values were higher than storage modulus. Using the rheological data obtained from the present study, the processing conditions of honeys depending on the honey type can be determined. And also, pumping power for the processes can be calculated using the viscosity values of the honey samples. Citrus honey flows easily in the pipe lines compared to pine honey due to its low viscosity.
Figure 3 The observed and predicted complex viscosity and complex modulus obtained using Arrhenius model of honey samples as a function of temperature at constant frequency (1 Hz) (•: observed data; −: predicted data).
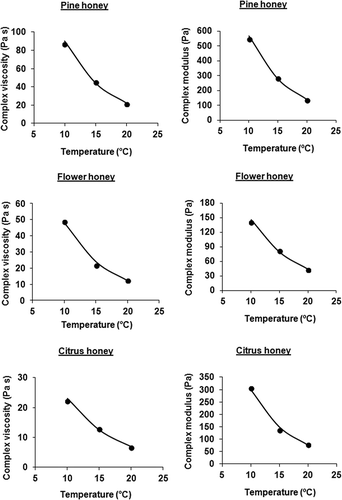
Table 4 Arrhenius model constants obtained from different honeys
Stress sweep test results
illustrates the dynamic mechanical spectra of honey samples obtained from a stress sweep test at a constant temperature (15°C). It is clear that the complex viscosity values of samples did not change in the range of 0.1–10 Pa. And also, the highest complex viscosity value was observed for the pine honey as in the frequency sweep test of honey samples. Citrus honey samples showed the lowest complex viscosity. A similar trend was also observed for the complex modulus. Storage modulus decreased with increasing stress and loss modulus that is important for the honey because they show liquid-like flow behavior did not change with increasing stress, and linear viscoelastic region was observed in the range of 0.1–10 Pa. Similar results were reported by Da Costa and Pereira.Citation[34] They determined that the complex modulus that is a function of loss modulus and storage modulus of wild flora pure honey remained constant with increasing stress from 0.1 to 10 Pa. It means that the stress in this range can be applied to the honey without adulterating or breaking the structure of the sample.
Figure 4 Oscillatory shear rheological parameters obtained from stress sweep test of different honeys at 15°C.
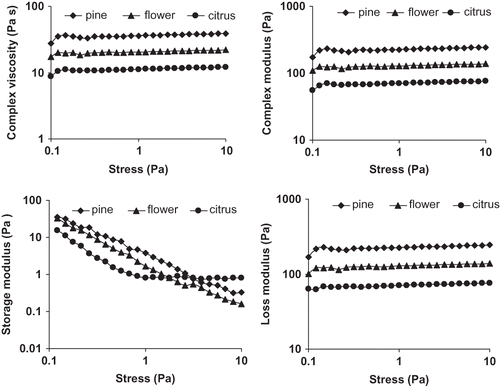
The effect of temperature on the complex viscosity and complex modulus for all Turkish honey samples was illustrated in . As can be seen from the figure, complex viscosity and complex modulus remained constant with increasing stress during a stress sweep test at all measurement temperatures and a decrement was observed with increment in temperature. The highest complex viscosity was determined for pine honey while the lowest was for citrus honey. The effect of temperature in the decrement of complex viscosity and complex modulus was found to be statistically significant (p < 0.05). shows the change in loss modulus, which is one of the most important dynamic mechanic parameters for all honey samples at different temperatures. As it can be seen from the figure, loss modulus decreased with increment of temperature as expected and increased with the increment of frequency and remained constant with the increment of stress. The highest loss modulus value was observed for pine honey after both frequency sweep and stress sweep test. Similar results were reported by Yoo,Citation[11] who investigated the temperature dependency of dynamic rheology of Korean honeys and determined that the magnitudes of loss modulus (G″), which are related to liquid-like response, decreased with increasing temperature and also an increment in loss modulus was observed with the increment of angular frequency. In another study, complex modulus as a function of loss modulus and storage modulus increased with an increase in angular velocity, indicating a more pronounced viscoelastic behavior of honey samples.Citation[34] Ahmed et al.Citation[2] reported that the viscous modulus (loss modulus, G″) was significantly higher compared to the elastic modulus (storage modulus, G′) throughout the frequency range employed confirming the liquid-like nature of the honey. Also, they stated that the viscous modulus of honey samples systematically increased as a function of angular frequency, while inconsistent elastic modulus data were observed in the rheogram. The lower elastic modulus means that there is a weak particle-particle interaction and there is no network formation in honey structure.Citation[2]
Nonlinear modeling results
Complex viscosity values of all honey samples were modeled using adaptive neuro fuzzy inference system (ANFIS) and artificial neural network (ANN) that are effective nonlinear modeling techniques to estimate the interval complex viscosity values at different frequency and temperature. summarizes the testing performance of ANFIS and ANN models for complex viscosity values of honey samples. As can be seen from the table, the best fit was observed for ANFIS model because of high coefficient of determination (R 2 ≥ 0.995) in both test and validation data compared to the ANN model. ANFIS model showed rather good performance for the estimation of complex viscosity depending on honey type, temperature, and frequency. RMSE values for test and validation data were calculated to be 1.003 and 3.131 in the ANFIS model, while these values were 1.037 and 4.988 in the ANN model, respectively. and illustrate the measured and computed complex viscosity values of honey samples using ANFIS and ANN modeling techniques in training, test, and validation periods, respectively. As can be seen from those figures, a very good fit was obtained from the model. A regression equation can be used for the computation of complex viscosity values obtained from the model in both figures. It could be said that the ANFIS was well trained for the modeling of complex viscosity of honey samples since its predicted values are a close match of the measured one. As can be seen from and , training performance of ANFIS was higher compared to ANN because of high coefficient of determination. Due to the good training of the ANFIS model, it predicted the actual complex viscosity values very well compared to ANN ( and ). Karaman and KayacierCitation[15] applied the ANFIS modeling techniques for the prediction of the apparent viscosity of date and apricot molasses and they concluded that the ANFIS model can be efficiently used for the prediction of apparent viscosity of molasses with coefficients of determination greater than 0.979. Similarly, Gänzle et al.Citation[35] stated that fuzzy inference systems can be efficiently used with the precise rules for the construction of effective predictive models to estimate the different parameters in nonlinear systems. As stated in the literature, physicochemical and rheological properties, such as viscosity or stability of foods, are regarded as complex nonlinear systems.Citation[13] It was found that the ANFIS was an efficient technique to be used for the prediction of viscosity and stability for mayonnaise in the food industry because the average percentage errors in the modeling of viscosity and stability were found to be 5 and 8%, respectively, in the validation period. Kavdir and GuyerCitation[36] developed an apple grading system using a fuzzy logic modeling system and the result showed that fuzzy logic was 89% in general agreement with results given by experts.
Figure 7 Measured and computed complex viscosity values of honeys using ANFIS model in training (a, b), test (c, d), and validation (e, f) period. Measured (•); ANFIS (—).
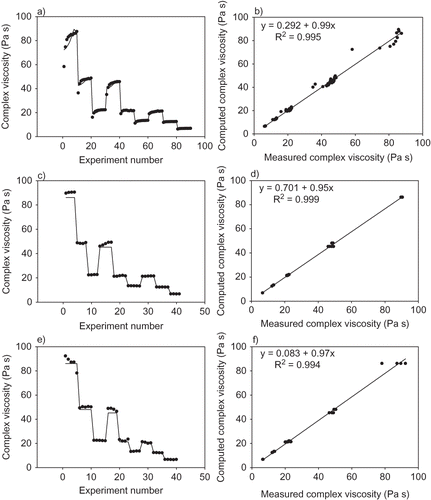
Figure 8 Measured and computed complex viscosity values of honeys using ANN model in training (a, b), test (c, d), and validation (e, f) period. Measured (•); ANN (—).
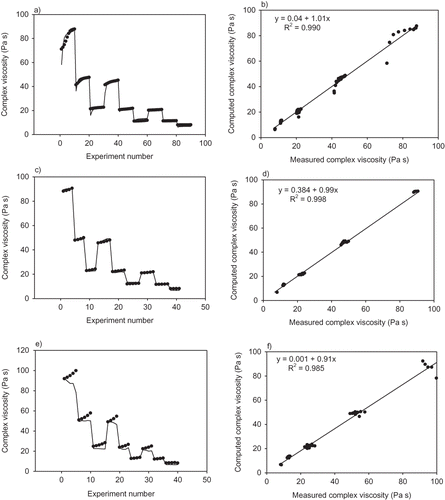
Table 5 Testing performance of ANFIS and ANN model for estimation of complex viscosity of honeys
CONCLUSION
All honey samples showed Newtonian flow behavior and their complex viscosity remained constant with increasing frequency and stress. The highest complex viscosity values were measured in pine honey, while the lowest values were in citrus honey. It was determined that the viscous modulus (loss modulus) of all honey samples was significantly higher than elastic modulus (storage modulus) during the frequency and stress range employed. Increasing frequency and stress provided an increment in loss modulus and complex modulus. Temperature dependency of complex viscosity and complex modulus was modeled by Arrhenius model with a coefficient of determination greater than 0.991. An increment in temperature caused a significant decrement in complex viscosity and complex modulus. Nonlinear modeling techniques, such as ANFIS and ANN, used for the prediction of complex viscosity performed well with coefficient of determination greater than 0.995 and 0.985, respectively. It could be concluded that ANFIS model can be used effectively for the estimation of complex viscosity values of different honey samples.
REFERENCES
- Juszczak , L. and Fortuna , T. 2006 . Rheology of selected Polish honeys . Journal of Food Engineering , 75 : 43 – 49 .
- Ahmed , J. , Prabhu , S.T. , Raghavan , G.S.V. and Ngadi , M. 2007 . Physico-chemical, rheological, calorimetric and dielectric behavior of selected Indian honeys . Journal of Food Engineering , 79 : 1207 – 1213 .
- Bhandari , B. , D'arcy , B. and Chow , S. 1999 . Rheology of selected Australian honeys . Journal of Food Engineering , 41 : 65 – 68 .
- Kayacier , A. and Karaman , S. 2008 . Rheological and some physicochemical characteristics of selected Turkish honeys . Journal of Texture Studies , 39 : 17 – 27 .
- White , Jr. and Honey , J.W. 1978 . Advances in Food Research , 24 : 288 – 354 .
- Assil , H.I. , Sterling , R. and Sporns , P. 1991 . Crystal control in processed liquid honey . Journal of Food Science , 56 : 1034 – 1041 .
- Mossel , B. , Bhandari , B. , D'arcy , B. and Caffin , N. 2000 . Use of Arrhenius model to predict rheological behaviour in some Australian honeys . LWT–Food Science and Technology , 33 : 545 – 552 .
- Sopade , P.A. , Halley , P. , Bhandari , B. , D'arcy , B. , Doebler , C. and Caffin , N. 2000 . Application of the Williams–Landel–Ferry model to the viscosity–temperature relationship of Australian honeys . Journal of Food Engineering , 56 : 67 – 75 .
- Junzheng , P. and Changying , J. 1998 . General rheological model for natural honeys in China . Journal of Food Engineering , 36 : 165 – 168 .
- Al-Malah , K.I.M. , Abu-Jdayil , B. , Zaitoun , S. and Al-Majeed Ghzawi , A. 2001 . Application of WLF and Arrhenius kinetics to rheology of selected dark-colored honey . Journal of Food Process Engineering , 24 : 341 – 357 .
- Yoo , B. 2004 . Effect of temperature on dynamic rheology of Korean honeys . Journal of Food Engineering , 65 : 459 – 463 .
- Rao , M.A. 1977 . Rheology of liquid foods—A review . Journal of Texture Studies , 8 : 135 – 168 .
- Abu Ghoush , M. , Samhouri , M. , Al Holy , M and Herald , T. 2008 . Formulation and fuzzy modeling of emulsion stability and viscosity of a gumeprotein emulsifier in a model mayonnaise system . Journal of Food Engineering , 84 : 348 – 357 .
- Lee , S.Y. , Hana , M.A. and Jones , D.D. 2008 . An adaptive neuro fuzzy inference system for modeling mechanical properties of tapioca starch-poly(lactic acid) nanocomposite foams . Starch , 60 : 159 – 164 .
- Karaman , S. and Kayacier , A. 2011 . Effect of temperature on rheological characteristics of molasses: Modeling of apparent viscosity using Adaptive Neuro Fuzzy Inference System (ANFIS) . LWT–Food Science and Technology , 44 ( 8 ) : 1717 – 1725 . 2011
- Zheng , H. , Jiang , B. and Lua , H. 2011 . An adaptive neural-fuzzy inference system (ANFIS) for detection of bruises on Chinese bayberry (Myrica rubra) based on fractal dimension and RGB intensity color . Journal Food Engineering , 104 ( 4 ) : 663 – 667 .
- AOAC . 2000 . Official Methods of Analysis , 17th , Arlington , VA : Association of Official Analytical Chemists, Inc .
- Steffe , J.F. 1996 . Rheological Methods in Food Process Engineering , 358 – 360 . East Lansing , MI : Freeman Press .
- Saravacos , G.D. 1970 . Effect of temperature on viscosity of fruit juices and purées . Journal of Food Science , 35 : 122 – 125 .
- Rao , M. , Cooley , M.J. and Vitali , A.A. 1984 . Flow properties of concentrated juices at low temperatures . Food Technology–Chicago , 38 : 113 – 119 .
- Hagan , M.T. and Menhaj , M.B. 1994 . Training feed forward networks with the Marquardt algorithm . IEEE Transactions on Neural Networks , 6 : 861 – 867 .
- Kisi , O. 2007 . Streamflow forecasting using different artificial neural network algorithms . ASCE Journal of Hydraulic Engineering , 12 : 532 – 539 .
- MINITAB . 2000 . Computer program, MINITAB release 13.0 for Windows , State College, PA: Minitab Inc .
- Mstat , C. 1986 . Version 4.00 , East Lansing , MI : Michigan State University .
- Ouchemoukh , S. , Louaileche , H. and Schweitzer , P. 2007 . Physicochemical characteristics and pollen spectrum of some Algerian honeys . Food Control , 18 : 52 – 58 .
- Terrab , A. , Diez , M.J. and Heredia , F.J. 2002 . Characterization of Moroccan unifloral honeys by their physicochemical characteristics . Food Chemistry , 79 : 373 – 379 .
- Nanda , V. , Sarkar , B.C. , Sharma , H.K. and Bawa , A.S. 2003 . Physico-chemical properties and estimation of mineral content in honey produced from different plants in Northern India . Journal of Food Composition and Analysis , 16 : 613 – 619 .
- Lazaridou , A. , Biliaderis , C.G. , Bacandritsos , N. and Sabatini , A.G. 2004 . Composition, thermal and rheological behaviour of selected Greek honeys . Journal of Food Engineering , 64 : 9 – 21 .
- Morris , E.R. 1989 . “ Polysaccharide solution properties: Origin, rheological characterization and implications for food systems ” . In Frontiers in Carbohydrate Research—1: Food Application , Edited by: Millan , R.P. , BeMiller , J.N. and Chandrasekavan , R. 132 – 163 . New York : Elsevier Applied Science Publisher .
- Doublier , J.L. and Cuvelier , G. 1996 . “ Gums and hydrocolloids: functional aspect ” . In Carbohydrates in Foods , Edited by: Eliasson , A.C. 283 – 318 . New York : Marcel Dekker Inc .
- Rao , M.A. and Cooley , H.J. 1992 . Rheological behavior of tomato paste in steady and dynamic shear . Journal of Texture Studies , 23 : 415 – 425 .
- Katsuta , K. and Kinsella , J.E. 1990 . Effects of temperature on viscoelastic properties and activation energies of whey protein gels . Journal of Food Science , 55 : 1296 – 1302 .
- Kleijnen , J.P.C. 1987 . Statistical Tools for Simulation Practitioners , 1 – 429 . New York : Marcel Dekker .
- Da Costa , C.C. and Pereira , R.G. 2002 . The influence of propolis on the rheological behavior of pure honey . Food Chemistry , 76 : 417 – 421 .
- Gänzle , M.G. , Kilimann , K.V. , Hartmann , C. , Vogel , R. and Delgado , A. 2007 . Data mining and fuzzy modelling of high pressure inactivation pathway of Lactococcus lactis . Innovative Food Science Emerging Technologies , 8 : 461 – 468 .
- Kavdir , I. and Guyer , D.E. 2003 . Apple grading using fuzzy logic . Turkish Journal of Agricultural and Forestry , 27 : 375 – 382 .