Abstract
In this study, inhibitory effects of bayleaf, rosemary, sage, and thyme hydrosols on the growth of Staphylococcus aureus inoculated on fresh-cut apples were investigated using several sigmoidal models (modified Gompertz, logistic, Richards, Stannard, and Whiting and Buchanan). In addition, these models were compared to describe the inactivation of S. aureus by fitting the experimental data using nonlinear models and various statistical parameters (the mean percentage error, the mean bias error, the root mean square error, the modeling efficiency, and chi-square parameters) as well as determination of coefficient (R2). The modified Gompertz, logistic, and Stannard models exhibited better fits than Richards and Whiting and Buchanan models regarding these statistical parameters. Rosemary and thyme hydrosols were found to be the most efficient sanitizers in reducing the S. aureus numbers. Results demonstrate that predictive models could be utilized for describing the inactivation or survival of S. aureus on fresh-cut apple with the effects of the treatments with plant hydrosols.
INTRODUCTION
Staphylococcus aureus is a facultative anaerobic, gram positive, and spherical-shaped bacterium with a diameter of 1–1.3 μm. Staphylococcal illnesses are resulted from enterotoxins produced by S. aureus in the foodstuff.[ Citation 1 ] Staphylococcal food poisoning is prevailed all over the world.[ Citation 2 ] Between 1979–1982, staphylococcal food poisoning constituted about 2% of reported outbreaks in Britain.[ Citation 3 ] Recently, S. aureus has been reported to be the second common foodborne pathogen in the USA and the third in France.[ Citation 4 ,Citation 5 ]
Humans are the main source of contamination of foods with S. aureus, since people carry the bacteria in their nose or on their hands as well as in their respiratory system. Moreover, air, dust, and food contact surfaces can also play a role as vehicles in the transfer of S. aureus to foods.[ Citation 6 ] Although enterotoxigenic S. aureus does not compete well with other microorganisms normally present in fresh products, the presence of S. aureus in fresh-cut fruit and vegetables has been well documented.[ Citation 7 ] In a study, staphylococci were isolated from approximately 20% of retail samples of strawberries and mushrooms in Norway.[ Citation 8 ] Nguz et al.[ Citation 9 ] detected S. aureus in 83.9% of fresh-cut organic vegetables produced in Zambia. Recently, Huong et al.[ Citation 10 ] have found that 22.2% of ready-to-eat foods, including sticky rice and fermented meat, were contaminated with S. aureus.
A number of antimicrobial compounds have been tested to minimize/eliminate the bacterial contamination of fresh-cut fruit and vegetables with food-borne pathogens including S. aureus. Chlorine-based sanitizers are widely used for this aim in the fresh-cut produce industry; however, reductions in bacterial populations of fruit and vegetables provided by those chemicals are less than 2 log cfu/g. Furthermore, some carcinogenic by-products may be formed because of the reaction between chlorine-based sanitizers and the organic material.[ Citation 11 ] Therefore, there is a great tendency to find alternative sanitizers all over the world. For this purpose, in addition to chlorine-based chemicals, some other sanitizing agents, such as electrolyzed water, essential oils, organic acids, and ozone, have been also evaluated as a way of sanitizing fresh-cut fruit and vegetables.[ Citation 12 − Citation 15 ]
Predictive food microbiology has drawn great attention in recent years.[ Citation 16 − Citation 18 ] Different mathematical models have been used to predict the behavior of microorganisms in food systems.[ Citation 18 ,Citation 19 ] An appropriate mathematical model, which can be constructed and properly validated, enables the prediction of the effect of changing treatment conditions on the microbial evolution.[ Citation 20 ]
The mathematical models used in predictive food microbiology can be classified as primary, secondary, and tertiary models.[ Citation 21 ] The primary models refer to mathematical expressions describing the response of the microbial load as a function of time under particular environmental and cultural conditions.[ Citation 22 ] The secondary models characterize the response of the parameters calculated with the primary models as a function of one or more environmental conditions, such as temperature, pH, water activity, etc.[ Citation 20 ] In a tertiary model, one or more primary and secondary models are combined with a user-friendly interface in a computer program to produce primary model-predicted output.[ Citation 23 ]
To the best of our knowledge, no study has appeared to focus on inhibitory effects of plant hydrosols on Staphylococcus aureus inoculated in fresh fruit and vegetables. Therefore, in this study, it was aimed to determine the effect of some plant hydrosols obtained from different parts of some spices (bayleaf, rosemary, sage, and thyme) in reducing the numbers of S. aureus in fresh-cut apples. Another objective was to describe the survival kinetics of S. aureus in sliced apples treated with these plant hydrosols using the primary models (modified Gompertz, logistic, Richards, Stannard, and Whiting and Buchanan models).
MATERIALS AND METHODS
Preparation of Plant Hydrosols and Apple Samples
Thyme (Thymus vulgaris L.), rosemary (Rosmarinus officinalis L.), sage (Salvia officinalis L.), and bayleaf (Laurus nobilis L.) plants were obtained from a local spice market in Kayseri, Turkey. In this study, leaves of thyme and bayleaf, and flowers of sage and rosemary were used as plant materials. The plant hydrosols were produced as outlined by Sagdic.[ Citation 24 ] Fifty grams of plant material was ground and placed into a flask (1 L) with 500 mL of distilled water (1:10 w/v) and hydrodistilled for 1 h with a Clevenger apparatus. During the hydrodistillation process, essential oil was removed through the cooling tunnels. Hydrosols were kept in sterile bottles at 4°C until use.
Fresh unprocessed apples were purchased from a local supermarket in Kayseri, Turkey and stored at 4°C until use. Apples were initially washed with cold tap water for 5 min to remove undesired residues and reduce native microbial load and then cut into pieces (1 × 1 × 1 cm3, each approximately 1 g) with a sterile knife to use them in the experiments.
Bacterial Culture Preparation and Contamination into Foods
Stock cultures of Staphylococcus aureus ATCC 25923 were obtained from Kayseri Agriculture Control Protection Management, Turkey. The culture was activated in Nutrient Broth (Merck, Darmstadt, Germany) at 37°C for 24 h.
A dip inoculation method was used to contaminate the sliced apples with S. aureus. For this purpose, stock inoculum solution with the targeted inoculation level of 106 cfu/mL was prepared by transferring 24 h Nutrient Broth culture of S. aureus into 500 mL of Ringer solution (1% v/v) and sliced apples (100 g) were immersed in it. The mixture was shaken for 1 min by hand to distribute the inoculum homogenously on the sliced apple samples and then they were kept in a biological safety cabinet (NuAire model Nu-425-200, Plymouth, MN, USA) at 22 ± 2°C for 1 h. Then the inoculated apple samples were filtered using rough filter paper under aseptic conditions.[ Citation 25 ]
Washing Treatment of Apple Samples with Plant Hydrosols
Sterility of the plant hydrosols was verified before use. Apple pieces were also tested for the presence of S. aureus and the bacterium was not detected. The contaminated sliced apple samples were washed by immersing 50 g of sample in sterile bottles containing 100 mL of sanitizing hydrosol for 0, 20, 40, and 60 min while control samples were immersed in sterile tap water. Bottles were covered following the addition of the sliced apple samples and subjected to continuous shaking. Finally, 10 g of the sliced apple samples were removed from the bottle by a sterile tweezer after 20, 40, and 60 min.[ Citation 25 ]
Enumeration of S. aureus Cells
Initially, 10 g of sanitized apple sample was transferred into sterile bottles containing 90 mL of sterile maximum recovery diluent solution and shaken vigorously by hand for 1 min. Next, 1 mL of homogenized solution was serially diluted in test tubes containing 9 mL of sterile maximum recovery diluent solution until 106. Initial counts and the number of surviving S. aureus cells were determined by spread-plating 1 ml of diluted (or nondiluted) samples on duplicate plates of Baird Parker Agar-BPA (Merck, Germany). The plates were incubated at 37°C for 48 h, after which all the characteristic visible bright black colonies on BPA were counted. The number of surviving cells was expressed as log N/N0 (N after treatment/initial N0).
Fitting of the Survival Models
Mathematical models that were developed for inactivation of S. aureus were fitted at different times of treatment of fresh cut apples with plant hydrosols. Curves were fitted to the natural logarithm of the counted S. aureus numbers, as detailed below, to estimate the inactivation rates using models. The nonlinear equations were used to fit to experimental data for inactivation of S. aureus by nonlinear regression analysis using Statistica (v8, StatSoft Inc., Tulsa, OK, USA). The Marquardt-Levenberg algorithm, a search method to minimize the sum of squares of the differences between the fitted and experimental data, was used to calculate the set of parameters and regression coefficient (R 2). Among the models studied, the application of the modified Gompertz and logistic models for the description of microbial survival/inactivation and growth has been well documented and commonly described in the literature.[ Citation 18 ,Citation 26 − Citation 28 ] The other models, Richards, Stannard,[ Citation 26 ] and Whiting and Buchanan models,[ Citation 29 ] were also used to calculate the set of parameters and regression coefficients (R 2).
The modified Gompertz (EquationEq. 1), logistic (EquationEq. 2), Richards (EquationEq. 5), Stannard (EquationEq. 6), and Whiting and Buchanan (EquationEq. 7) models were used as the empirical models to fit the microbial survival curves of S. aureus.
For the modified Gompertz model, the maximum specific inactivation rate (log cfu/g/min) was calculated from:
Comparison of the Fitted Models
The performance of the all mathematical models used in this study to predict the inactivation kinetics of S. aureus in fresh-cut apples was compared using various statistical parameters, i.e., the mean percentage error (MPE), the mean bias error (MBE), the root mean square error (RMSE), the modeling efficiency (EF), and chi-square (χ2) as well as determination of coefficient (R 2). These parameters were used to evaluate the models using the differences between experimental data and model estimates.[ Citation 30 ] These parameters were calculated as follows:
RESULTS AND DISCUSSION
Volatile Components of Plant Hydrosols
Major volatile compounds of plant hydrosols determined previously by Tornuk et al.[ Citation 25 ] were presented in . As can be seen in the table, carvacrol was detected in both thyme and sage hydrosols while thymol was another component found in thyme hydrosol in relatively high amounts. Meanwhile, α-terpineol and eugenol were the most abundant volatile compounds of rosemary and bayleaf hydrosols, respectively ().
Table 1 Major volatile compounds of plant hydrosols.[ Citation 25 ]
There has been a limited number of studies showing the volatile compositions of hydrosols from different plants. However, it may be expected that volatile components of hydrosols were similar to essential oils with lower amounts. In this case, volatile composition of an essential oil from a particular plant is comparable with the hydrosol. Porte and Godoy[ Citation 31 ] detected carvacrol in the volatile composition of thyme essential oil in addition to thymol, p-cymene, and γ-terpinene. Although Pintore et al.[ Citation 32 ] determined α-terpineol as one of the volatile compounds in rosemary essential oil from different origins with insignificant levels, α-pinene, camphor, verbenone, and bornyl acetate were the major compounds in the composition. In another study, 1,8-cineole (39.5–69.2%), linalool (5.6–15.3%), and α-terpineol (2.2–13%) were found as the main volatiles of bayleaf hydrosols.[ Citation 33 ]
Effects of Plant Hydrosols and Evaluation of the Biological Parameters
shows the derived parameters from modeling the survival number of S. aureus on treated fresh-cut apples with different plant hydrosols. All models gave reasonably good fits, with high R 2 values. As can be seen, these models were three- or four-parameter models that were used to describe the sigmoidal inactivation curve. In the three-parameter models, the estimates have one more degree of freedom, which can be important in the case of use of an activation curve with a small number of experimental points. All three-parameter models have a biological meaning. On the other hand, the four-parameter models give shape parameter and their explanation is difficult in terms of biological aspect.[ Citation 34 ]
Table 2 Parameters derived from the predictive modeling of the survival of Staphylococcus aureus in fresh-cut apples treated with different plant hydrosols
The maximum specific inactivation rates (μ), the derived parameter from the modified Gompertz and logistic models are given in . It can be clearly seen that the inactivation rate values obtained from these two models were determined from −0.053 to −0.201 log cfu/g/min. The negative signs of the μ values indicate inactivation. The similar results were also obtained with the a (the count increment) values of the other models; Richards, Stannard, and Whiting and Buchanan models indicated inactivation rates with negative signs.
illustrates the predicted data obtained by the fitting of experimental data with different predictive models. As can be seen from , model predictions gave similar results in some cases. For example, in the graphic showing the fitting results of the effect of thyme hydrosol treatment on S. aureus population, all models predicted the bacterial inactivation in a similar way with each other and with high accuracy.
Figure 1 Fitting of the modified Gompertz, logistic, Richards, Stannard, and Whiting and Buchanan models to the experimental (observed) data obtained from the survival of S. aureus in fresh-cut apples: (a) bay leaf, (b) rosemary, (c) sage, and (d) thyme hydrosols.
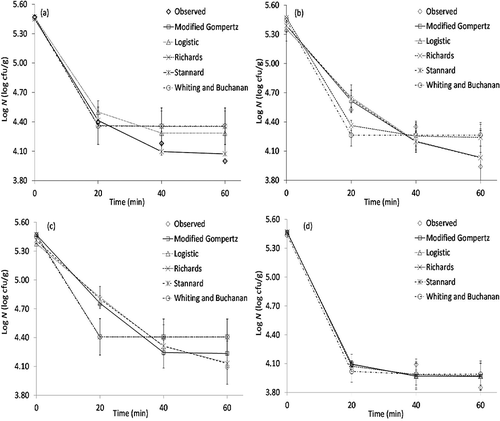
As for the effectiveness degree of the plant hydrosols based on the biological estimating parameters given in , rosemary and thyme hydrosols appeared to be the most effective sanitizers. also indicates the results of the experimental data. The initial number of S. aureus was 5.47 log cfu/g. Each hydrosol treatment resulted in remarkable reductions in the counts of S. aureus even in the first 20 min of treatment time and that rosemary and thyme hydrosol was the most effective sanitizer, considerably decreasing the number of S. aureus in the tested treatment times (20, 40, and 60 min). S. aureus counts decreased to the level of 3.85 log cfu/g after the sliced-apple samples were treated with the thyme hydrosol for 60 min. As for the rest of the plant hydrosols, the efficiency of the rosemary and sage hydrosols was also time dependent and additional reductions took place with increasing the times of treatments.
To summarize, observed and fitted inactivation responses indicated that hydrosol application showed remarkable inhibitory effect on S. aureus. These results were consistent with the reports in the literature.[ Citation 24 ,Citation 35 − Citation 37 ] Antibacterial activity of plant hydrosols may possibly be due to the presence of carvacrol and thymol.[ Citation 31 ,Citation 38 ] On the other hand, a great number of the studies have been conducted to determine the antibacterial effects of various plant materials, such as essential oils, extracts, and hydrosols.[ Citation 24 ,Citation 36 ,Citation 37 ,Citation 39 − Citation 41 ] Karatas and Ertekin[ Citation 37 ] proved in vitro antibacterial activities of essential oils obtained from four parts of sage against S. aureus. In a previous study,[ Citation 24 ] inhibitory effect of thyme hydrosol on S. aureus was revealed. Sagdic and Ozcan[ Citation 41 ] reported that oregano hydrosol showed the highest inhibition effect on S. aureus than did other hydrosols obtained from rosemary, sage, and black thyme. Hydrosols, also known to be aromatic water or distillate water, are residual liquid materials obtained after distillation of essential oils.[ Citation 42 ]
Model Comparisons
Microbial inhibition or inactivation is the second most studied area of modeling of microbiological food safety. A model used for describing the inhibition rate of a particular microorganism may present a linear decrease of population over time under an inactivation or preservation treatment.[ Citation 23 ] Several models have been utilized to fit models better for evaluation of microbial inactivation kinetics in various food models. Erkmen[ Citation 18 ] analyzed the inactivation curve of Salmonella Typhimurium under high hydrostatic pressures at different temperatures using the modified Gompertz model. In the study of Chen,[ Citation 43 ] linear, Weibull, and log-logistic functions were used to obtain survival curves of six foodborne pathogens in ultra-high-temperature (UHT) whole milk exposed to high hydrostatic pressure; as a result, they found that the log-logistic and Weibull models consistently showed better fits to the inactivation data than did the linear model. Bialka et al.[ Citation 44 ] reported that the Weibull model fitted better than the log-linear model in predicting the inactivation of Escherichia coli O157:H7 and Salmonella enterica in raspberries and strawberries by their exposure to ozone or pulsed UV-light.
Table 3 Regression parameters evaluating the performance of modified Gompertz, logistic, Richards, Stannard, and Whiting and Buchanan models
In this study, performance of the derived models was compared with each other. indicates the performance of the derived mathematical models evaluated by the statistical test parameters: MPE, MBE, RMSE, EF, and χ2. It was reported that higher values of EF and lower of MPE, MBE, RMSE, and χ2 implies the better goodness of an individual model. [ Citation 30 ] In this respect, when EF, RMSE, and χ2 values are taken into consideration, modified Gompertz, logistic, and Stannard models exhibited better results as compared to the other models regarding each hydrosol treatment. The correlations between experimental and predicted data obtained from the modified Gompertz, logistic, Richards, Stannard, and Whiting and Buchanan models used for determination of the effects of hydrosol treatments on the survival of S. aureus are shown in . In all cases, there was good agreement between experimental and predicted data with R 2 values between 0.800 and 0.996 ().
CONCLUSIONS
Plant hydrosols were successful in reducing the count of S. aureus inoculated on fresh-cut apples, and remarkable decreasing effect of each hydrosol was observed even at the treatment time (20 min). The inhibition effects of rosemary and thyme hydrosols were higher than those of bayleaf and sage hydrosols. All the mathematical models had a good fit with relatively high R 2 values. Modified Gompertz, Logistic, and Stannard models exhibited better fits than Richards and Whiting and Buchanan models in respect of the statistical comparison tools. This study revealed that the mathematical models studied could be used successfully to explain the microbial inactivation responses under different hydrosol applications.
REFERENCES
- Loir , Y.V. , Baron , F. and Gautier , M. 2003 . “ Staphylococcus aureus and food poisoning ” . In Genetics and Molecular Research Vol. 2 , 63 – 76 .
- Lawrynowicz-Paciorek , M. , Kochman , M. , Piekarska , K. , Grochowska , A. and Windyga , B. 2007 . The distribution of enterotoxin and enterotoxin-like genes in Staphylococcus aureus strains isolated from nasal carriers and food samples . International Journal of Food Microbiology , 117 : 319 – 323 .
- Woolaway , M.C. , Bartlett , C.L.R. , Wieneke , A.A. , Gilbert , R.J. , Murrell , H.C. and Aureli , P. 1986 . International outbreak of staphylococcal food poisoning caused by contaminated lasagne . Journal of Hygiene , 96 : 67 – 73 .
- Zhang , S. , Iandolo , J.J. and Stewart , G.C. 1998 . The enterotoxin D plasmid of Staphylococcus aureus encodes a second enterotoxin determinant (sej) . FEMS Microbiology Letters , 168 : 227 – 233 .
- Kerouanton , A. , Hennekinne , J.A. , Letertre , C. , Petit , L. , Chesneau , O. , Brisabois , A. and De Buyser , M.L. 2007 . Characterization of Staphylococcus aureus strains associated with food poisoning outbreaks in France . International Journal of Food Microbiology , 115 : 369 – 375 .
- Argudin , M.A. , Mendoza , M.C. and Rodicio , M.R. 2010 . Food poisoning and Staphylococcus aureus enterotoxins . Toxins , 2 : 1751 – 1753 .
- Beuchat, L.R. Surface decontamination of fruits and vegetables eaten raw: A review. 1998. http://www.who.int/foodsafety/publications/fs_management/en/surface_decon.pdf (http://www.who.int/foodsafety/publications/fs_management/en/surface_decon.pdf) (Accessed: 25 July 2011 ).
- Johannessen , G.S. , Loncarevic , S. and Kruse , H. 2002 . Bacteriological analysis of fresh produce in Norway . International Journal of Food Microbiology , 77 : 199 – 204 .
- Nguz , K. , Shindano , J. , Samapundo , S. and Huyghebaert , A. 2005 . Microbiological evaluation of fresh-cut organic vegetables produced in Zambia . Food Control , 16 : 623 – 628 .
- Huong , B.T.M. , Mahmud , Z.H. , Neogi , S.B. , Kassu , A. , Nhien , N.V. , Mohammad , A. , Yamato , M. , Ota , F. , Lam , N.T. , Dao , H.T.A. and Khan , N.C. 2010 . Toxigenicity and genetic diversity of Staphylococcus aureus isolated from Vietnamese ready-to-eat foods . Food Control , 21 : 166 – 171 .
- Gil , M.I. , Selma , M.V. , Lopez-Galvez , F. and Allende , A. 2009 . Fresh-cut product sanitation and water disinfection: problems and solutions . International Journal of Food Microbiology , 134 : 37 – 45 .
- Kim , B.S. , Kwon , J.Y. , Kwon , K.H. , Cha , H.S. and Jeong , J.W. 2006 . Antimicrobial effect of cold ozonated water washing on fresh-cut lettuce . Acta Horticulturae , 699 : 235 – 242 .
- Singh , N. , Singh , R.K. , Bhunia , A.K. and Stroshine , R.L. 2002 . Efficacy of chlorine dioxide, ozone, and thyme essential oil or a sequential washing in killing Escherichia coli O157: H7 in lettuce and baby carrots . LWT−Food Science and Technology , 35 : 720 – 729 .
- Koseki , S. and Isobe , S. 2006 . Effect of ozonated water treatment on microbial control and on browning of iceberg lettuce (Lactuca sativa L.) . Journal of Food Protection , 69 : 154 – 160 .
- Sengun , I.Y. and Karapinar , M. 2005 . Effectiveness of household natural sanitizers in the elimination of Salmonella typhimurium on rocket (Eruca sativa Miller) and spring onion (Allium cepa L.) . International Journal of Food Microbiology , 98 : 319 – 323 .
- Whiting , R.C. and Buchanan , R.L. 1994 . Microbial modeling, scientific status summary . Food Microbiology , 48 : 113 – 120 .
- Baranyi , J. and Roberts , T.A. 1995 . Mathematics of predictive food microbiology . International Journal of Food Microbiology , 26 : 199 – 218 .
- Erkmen , O. 2009 . Mathematical modeling of Salmonella typhimurium inactivation under high hydrostatic pressure at different temperatures . Food and Bioproducts Processing , 87 : 68 – 73 .
- Bozkurt , H. and Erkmen , O. 1999 . Modeling in protection and prediction of food quality . Food Technology , 4 : 63 – 70 .
- Geeraerda , A.H. , Valdramidisa , V.P. , Devlieghereb , F. , Bernaertb , H. , Debevereb , J. and Van Impe , J.F. 2004 . Development of a novel approach for secondary modelling in predictive microbiology: Incorporation of microbiological knowledge in black box polynomial modelling . International Journal of Food Microbiology , 91 : 229 – 244 .
- Aggelis , G. , Samelis , J. and Metaxopoulos , J. 1998 . A novel modelling approach for predicting microbial growth in a raw cured meat product stored at 38°C and at 128°C in air . International Journal of Food Microbiology , 43 : 39 – 52 .
- Marks , B.P. 2008 . Status of microbial modeling in food process models . Comprehensive Reviews in Food Science and Food Safety , 7 : 137 – 143 .
- Black , D.G. and Davidson , P.M. 2008 . Use of modeling to enhance the microbiological safety of the food system . Comprehensive Reviews in Food Science and Food Safety , 7 : 159 – 167 .
- Sagdic , O. 2003 . Sensitivity of four pathogenic bacteria to Turkish thyme and oregano hydrosols . LWT−Food Science and Technology , 36 : 467 – 473 .
- Tornuk , F. , Cankurt , H. , Ozturk , I. , Sagdic , O. , Bayram , O. and Yetim , H. 2011 . Efficacy of various plant hydrosols as natural food sanitizers in reducing Escherichia coli O157: H7 and Salmonella typhimurium on fresh cut carrots and apples . International Journal of Food Microbiology , 148 : 30 – 35 .
- Zwietering , M.H. , Jongenburger , I. , Rombouts , F.M. and Van't Riet , K. 1990 . Modeling of the bacterial growth curve . Applied and Environmental Microbiology , 56 : 1875 – 1881 .
- Buchanan , R.L. 1993 . Predictive food microbiology . Trends Food Science and Technology , 4 : 6 – 11 .
- Erkmen , O. 2008 . Modeling the effects of sucuk production technique on Listeria monocytogenes, aerobic bacteria and lactic acid bacteria during ripening and storage . Food and Bioproducts Processing , 86 : 220 – 226 .
- Buchanan , R.L. , Golden , M.H. , Whiting , R.C. , Phillips , J.G. and Smith , J.L. 1994 . Non-thermal inactivation models for Listeria monocytogenes . Journal Food Science , 59 : 179 – 188 .
- Togrul , H. and Arslan , N. 2004 . Mathematical model for prediction of apparent viscosity of molasses . Journal of Food Engineering , 62 : 281 – 289 .
- Porte , A. and Godoy , R.L.O. 2008 . Chemical composition of Thymus vulgaris L. essential oil from the Rio de Janerio State (Brazil) . Journal of the Serbian Chemical Society , 73 ( 3 ) : 307 – 310 .
- Pintore , G. , Usai , M. , Bradesi , P. , Juliano , C. , Boatto , G. , Tomi , F. , Chessa , M. , Cerri , R. and Casanova , J. 2002 . Chemical composition and antimicrobial activity of Rosmarinus officinalis L. oils from Sardinia and Corsica . Flavour and Fragrance Journal , 17 : 15 – 19 .
- Di Leo Lira , P. , Retta , D. , Tkacik , E. , Ringuelet , J. , Coussio , J.D. , Van Baren , C. and Bandoni , A.L. Essential oil and by-products of distillation of bay leaves (Laurus nobilis L.) from Argentina . Industrial Crops and Products 2009 , 30 ( 2 ) 259 – 264 .
- Erkmen , O. 2000 . Predictive modelling of Listeria monocytogenes inactivation under high pressure carbon dioxide . LWT−Food Science and Technology , 33 : 514 – 519 .
- Deans , S.G. and Svoboda , K.P. 1989 . Antimicrobial activity of summer savory (Satureja hortensis L.) essential oil and its constituents . Journal of Horticultural Science , 64 : 205 – 210 .
- Chorianopoulos , N.G. , Giaouris , E.D. , Skandamis , P.N. , Haroutounian , S.A. and Nychas , G.J.E. 2008 . Disinfectant test against monoculture and mixed-culture biofilms composed of technological, spoilage and pathogenic bacteria: Bactericidal effect of essential oil and hydrosol of Satureja thymbra and comparison with standard acid-base sanitizers . Journal of Applied Microbiology , 104 : 1586 – 1596 .
- Karatas , H. and Ertekin , S. 2010 . Antimicrobial activities of the essential oils of four Salvia species from Turkey . Journal of Medicinal Plants Research , 4 : 1238 – 1240 .
- Farag , R.S. , Daw , Z.Y. , Hewedi , F.M. and El-Baroty . 1989 . G.S.A. Antimicrobial activity of some Egyptian spice essential oils . Journal of Food Protection , 52 : 665 – 667 .
- Kivanc , M. and Akgul , A. 1986 . Antibacterial activities of essential oils from Turkish spices and citrus . Flavour Fragrance Journal , 1 : 175 – 179 .
- Sagdic , O. , Kuscu , A. , Ozcan , M. and Ozcelik , S. 2002 . Effects of Turkish spice extracts at various concentrations on the growth of Escherichia coli O157 . H7. Food Microbiology , 19 : 473 – 480 .
- Sagdic , O. and Ozcan , M. 2003 . Antibacterial activity of Turkish spice hydrosols . Food Control , 14 : 141 – 143 .
- Tajkarimi , M.M. , Ibrahim , S.A. and Cliver , D.O. 2010 . Antimicrobial herb and spice compounds in food . Food Control , 21 : 1199 – 1218 .
- Chen , H. 2007 . Use of linear, Weibull, and log-logistic functions to model pressure inactivation of seven foodborne pathogens in milk . Food Microbiology , 24 : 197 – 204 .
- Bialka , K.L. , Demirci , A. and Puri , V.M. 2008 . Modeling the inactivation of Escherichia coli O157: H7 and Salmonella enterica on raspberries and strawberries resulting from exposure to ozone or pulsed UV-light . Journal of Food Engineering , 85 : 444 – 449 .